- Laboratory of Microbial Ecology, Research and Development Institute for the Agri-Environment (IRDA), Quebec, QC, Canada
Introduction and methods: As part of a study on control methods of common scab disease impact on potato yield and quality, high-throughput sequencing was used to measure the effects of soil fumigant chloropicrin alone or in combination with a Bacillus species-based biostimulant on soil bacterial diversity in terms of richness and composition, as well as on soil bacterial network interactions.
Results and discussion: The results showed that common scab caused significant net yield losses of more than 46.25% in potatoes of control plots (T1), while the use of the fumigant alone (T3) and the use of the fumigant with the biostimulant (T4) reduced net yield losses to less than 2.5%. These treatments also promoted gross yield increases of 23.5 cwt. acre-1 (7.06%) and 28 cwt. acre-1 (8.41%) respectively. The study found that using the soil fumigant chloropicrin significantly and persistently altered the composition of the soil bacterial community over the growing season. The modifications of the soil bacterial community induced by the inoculation of the Bacillus species-based biostimulant are distinct by the end of the growing season depending on whether the soil has been fumigated (T4) or not (T2). Interestingly, artificial network inference analysis showed that the T2 treatment had the highest number of edges and linkages, contrary to the T3 treatment that had the lowest number of edges and linkages. The fumigation alone treatment leads to a reduction in interactions, while the application of the biostimulant, in both non-fumigated and fumigated soil, results in increased interactions and a higher number of connections within a phylum or between different taxa. Furthermore, the treatment combining the fumigant and the biostimulant exhibits a moderate increase in various network properties, providing evidence for the positive effect of biostimulant inoculation on bacterial communities in fumigated soils. Our results provide a more detailed understanding of the bacterial community structure and diversity in the soil of the different treatments. Moreover, deciphering network interactions in soil bacterial communities is fundamentally important for research in soil microbial ecology of potato cropping systems.
Introduction
Potato (Solanum tuberosum L.) is one of the most important food crops in the world. During cropping season, potato production faces numerous constraints, including a high vulnerability to diseases and pests (1, 2). Under the genus Streptomyces, which represents spore-forming bacteria that are commonly found in the soil, several species (S. scabies, S. acidiscabies, S. turgidiscabies, S. europaeiscabiei, S. stelliscabiei and S. aureofaciens) act as plant pathogens by producing phytotoxins such as thaxtomin A (3–5). The txtAB genes involved in thaxtomin biosynthesis act as pathogenicity determinants while other genes like nec1 and tomA are virulence determinants (4). These genes are clustered within a mobile pathogenicity island (PAI) (6). The PAI can be horizontally transferred among genetically distinct strains (7), that may lead to new pathogenic species (8). The CS causes different skin defects with shallow or deep-pit tuber necrosis according to the potato cultivar sensitivity and the Streptomyces strains involved (5) and is a recurrent plant disease found all over the world (9). The pathogen infects the surface of potato tubers, resulting in the formation of corky, scaly lesions and scab symptoms may vary according to cultivar susceptibility, environmental conditions, infection date or virulence of the Streptomyces species (10). These unsightly blemishes reduce the market value of potatoes and often render them unmarketable. In addition to the cosmetic damage, common scab can lead to a decrease in tuber yield and size, impacting potato crop productivity and profitability (11, 12). Symptoms development can be severe due to environmental conditions, including warm and dry seasons, combined with physicochemical properties of the soil (13). Virulent spores may survive many years in the soil. Some of these Streptomyces species are of particular importance in Québec (14) and other potato growing provinces in Canada causing significant economic losses estimated between 15 and 17 million dollars per year in Canada (1).
The economic impact of common scab on potato production is important. Crop losses due to common scab can range from moderate to severe, with estimates of yield reductions reaching up to 30-50% in severely affected fields. In regions where potato production is a vital economic activity, such losses can lead to food scarcity, higher potato prices, and economic strain on farmers and stakeholders. Furthermore, the cost of disease management practices, such as chemical treatments and soil fumigation, adds an economic burden on growers, making effective management strategies crucial (4, 15).
Traditional methods for common scab management have primarily relied on the use of chemical pesticides and soil fumigation (16). While these practices can provide temporary relief, they come with several limitations. Prolonged pesticide use can lead to the development of pesticide-resistant pathogen strains and environmental pollution, affecting non-target organisms and ecosystems. Additionally, soil fumigation can disrupt the soil’s natural microbial balance, further exacerbating the problem and reducing the overall soil health and sustainability (17).
Given the environmental and economic drawbacks of conventional management strategies, there is an urgent need for more effective, sustainable, and environmentally friendly approaches to combat common scab in potato crops. The inoculation of biostimulant bacterial strains acting as biocontrol agent against plant pathogens and acting as plant growth-promoting bacteria (PGPR) is an element of a strategy for sustainable agriculture (18). In addition to their biocontrol agent capability, biostimulant bacteria enhance plant growth by producing phytohormones like auxins, siderophores, and by activating plant defense pathways (9).
Soil microorganisms play a key role in soil biogeochemical cycles and ecosystem conservation (19). Given the importance of microbial diversity in ecological functioning that promote soil health, plant productivity and protection against soil-borne diseases (20), agronomists and growers may benefit of a better understanding of how agricultural practices, such as soil chemical fumigation with or without biostimulant influence the diversity of soil microbial communities. Chemical fumigation is a non-specific control method that has been used against soil pathogenic fungi, bacteria, nematodes, weeds, and insects in high-value crop systems (16, 21). In Canadian potato crop systems, the most used chemical fumigants are chloropicrin and metam-sodium (22). Although chemical fumigation effectively reduces soil-borne diseases (23, 24), studies have shown that chemical fumigants impact non-targeted microorganisms, consequently altering the soil bacterial community diversity and decreasing bacterial Chao1 index (17, 25). According to a study investigating the efficacy of chloropicrin as a possible alternative fumigant to methyl bromide in tomato production systems (26), results showed that chloropicrin can kill more than 85% of soil bacteria, fungi and Actinobacteriota, when control efficiency is estimated using a cultivation-dependent bioassay method to monitor pathogens inoculum survival on Petri plates containing potato dextrose agar.
Although biofumigation has been proposed as an alternative to chemical fumigation (27), it has been found to alter bacterial and fungal β-diversity while decreasing bacterial and fungal species richness under controlled environments (22). Studies that have compared the effects of chemical fumigation and biofumigation on soil bacterial communities (28, 29) showed that fumigation with chloropicrin had the greatest and most persistent impact on bacterial diversity, whereas fumigation with metam-sodium used alone and biofumigation with mustard plant residues led to transient decreases in bacterial diversity.
Given that fumigation with chloropicrin used to control soil-borne pathogens, weeds and nematodes may still destroy beneficial soil microorganisms, it becomes urgent to mitigate this undesirable impact by promoting the rapid microbial repopulation of the soil using microorganisms known to stimulate plant growth. It has been shown that the use of biostimulants can enhance soil nutrients availability, plant nutrients uptake and assimilation, and increase mineral content in plant-based food (30). This is the case of a biostimulant which is a mix of eight bacterial isolates of five Bacillus species (B. amyloliquefaciens, B. licheniformis, B. megatarium, B. methylotrophicus (syn. B. velezensis) and B. subtilis). This Bacillus species-based biostimulant increases microbial activity leading to increased nutrient availability that affect plant growth, including root growth and root diameter, as well as impacting other soil factors such as the capacity of retention of soil water (31). Indeed, the Bacillus species-based biostimulant may maintain plant growth during abiotic stress situations (31). However, to our knowledge no study has yet used powerful tools such as high throughput sequencing (HTS) of 16S rRNA to assess the combined effect of Bacillus species based biostimulant and chloropicrin fumigant on soil bacterial community to control common scab in potato production.
Acquiring a better understanding of the interactions among different bacterial species within a community is a key purpose in microbial ecology (32). Soil microbiome studies have become more accessible and cost-effective, enabling the analysis of large datasets. Machine learning techniques, such as penalized regression, support vector machine, random forest, artificial neural network, and deep neural networks, are increasingly being used to explore the complex relationships between the microbiome and various host phenotypes. These methods can identify important features or biomarkers in microbiome data and predict host phenotypes based on microbiome composition (33, 34).
Thus, our study aimed using HTS 16S rRNA and network inference analysis to assess the effect of a fumigant (chloropicrin) and a Bacillus species-based biostimulant, applied alone or in combination, on soil bacterial communities and their interactions. The impact of the treatments on agronomic performance of potato plants, specifically on the potato yield and the incidence of common scab were measured. The hypotheses of the study were that: (i) fumigation would increase potato yield and reduce the yield losses caused by common scab, while altering interactions within the soil bacterial community; (ii) biostimulant inoculated in non-fumigated or fumigated soils would increase potato yield and also reduce net yield losses caused by plant pathogenic Streptomyces species (iii) biostimulant inoculated in non-fumigated or fumigated soils would increase species richness, promote modification of the bacterial community composition and increase the positive interactions among bacterial phyla in comparison to bacterial communities of non-fumigated or fumigated alone soils. Our specific objectives consisted to i) evaluate the agronomic performance of potato plants and the incidence of common scab on potato yield; ii) compare the effects of the treatments applied alone or in combination at five time-periods in the growing season on the quantity, diversity in richness and composition of soil bacterial community; iii) estimate the relationships and interactions between the soil bacterial populations.
Materials and methods
Field experiment design
Experiment was carried out on 16 potato plots of 6 rows of 6 m in length, planted in a field of Patates Dolbec inc. located in Saint-Ubalde (46.729085, -72.266851) (QC, Canada). Experiments were conducted in a randomized complete block design with four repetitions in an area of 532.53 m2 in 2018. Soil physicochemical properties and nutrient content are described in Table 1. Planting was done at a density of 33,300 plants ha-1. The two rows in the center of each plot were used to determine the potato yield, the next two rows were used for soil composite and plant biomass sampling, while the outer rows represent a treatment buffer zone. Main plots involved four treatments: T1 (non-fumigated soil without biostimulant application), T2 (non-fumigated soil with biostimulant application), T3 (fumigated soil without biostimulant application) and T4 (fumigated soil with biostimulant application).
The fumigation of plots T3 and T4 was done by a well-trained soil fumigation service provider using Chloropicrin Pic Plus fumigant (Synagri, QC, Canada) injected under pressure with shank-injectors penetrating the first 15 cm of the soil. The fumigation process took place on May 19th, 2018. This method involved applying the fumigant to the soil to control soil-borne pests and diseases. Regarding the biostimulant application, the product Alpine Bio20 is a mix of eight bacterial isolates of five Bacillus species applied at around 5 x 105 CFU.mL-1 (AgBrima, QC, Canada). The application was done by inoculating the Bacillus species-based biostimulant at 30 L.ha-1 to the furrow on the potato seed pieces at plantation. This process occurred on May 29th, 10 days after the soil fumigation and during the plantation (10DAF and 0DAP). The Table 2 presents the milestones of the project.
Composite of four soil cores (4.5 cm diameter and 0-20 cm depth) were sampled, one core in each corner of the rows adjacent to center rows of a plot at five time-periods during the growing season (Table 2). Subsequently, potatoes were harvested over 2 x 5 m (± 32 plants) targeting the two central rows of each plot.
Assessing plant agronomic performance and incidence of common scab
Two plants per row adjacent to the two centered rows were randomly sampled for a total of four plants per plot one hundred one days after planting. The roots were shaken to remove all adhering soil and placed in a paper bag, while the aerial parts of the plants were placed in a paper bag to determine plant root and plant aerial biomass respectively. Following the harvest of the tubers from each of the two central rows (2 x 5 m), the number of plants and the numbers and weights of the tubers were determined to obtain the total gross yield and the yield for each of the following five caliber sizes:< 2 in.; from 21/8 to 21/2 in.; from 21/2 to 23/4 in.; from 23/4 to 3 in.; > 3 in. Among the harvested tubers, a subsample of 25 tubers of the caliber size from 21/2 to 23/4 in. and 15 tubers of >3 in. size were examined for each plot to quantify the visual recovery index of superficial and deep-pit common scab symptoms and to determine common scab disease index. The common scab index, including superficial and deep-pit common scab was estimated as previously described by (35) according to the formula:
where CI: common scab index, SI: sum of indices and NTO: number of tubers observed. For each plot, tubers showing superficial common scab symptoms on more than 10% of their surface and tubers showing deep-pit symptoms were used to estimate the gross yield losses, the remaining harvested tubers representing the marketable yield.
Soil samples collection and DNA extraction
The DNA extraction was carried out as described (36) In order to collect and prepare soil samples for DNA extraction, a 200 g aliquot of each soil sample was manually homogenized and sieved to 6 mm, then a 0.5 g subsample of the 6 mm sieved soil was added to 2 mL tubes containing 1.4 g of matrix E beads and 1 mL of lysis buffer that were supplied with the FastDNA™ SPIN Kit for Soil (MP Biomedicals, Solon, OH, USA), as described by (37). The homogenization step was done using a FastPrep-24™instrument (MP Biomedicals, Solon, OH, USA). Quality control of DNA extracts was evaluated by spectrophotometry (Biophotometer, Eppendorf, Mississauga, ON, Canada) with a G1.0 microcuvette (mCuvette, Eppendorf) at 230, 260, and 280 nm.
Quantitative PCR analysis
The Eub338 and Eub518 primers sequences “ACTCCTACGGGAGGCAGCAG” and “ATTACCGCGGCTGCTGG” from Fierer et al. (38) were used to perform amplifications on a CFX96 instrument (Biorad, Hercules, CA) using SYBR green qPCR mix (Qiagen, Toronto, ON) as described (39). Briefly, initial activation step was 15 min at 95°C, followed by 40 cycles of 95°C for 1 min, 30 s at the annealing temperature 53°C and 1 min at 72°C for extension. Standard curves were generated from a known quantity of amplified DNA fragments, diluted over a 4-log range. This method was used to quantify the relative abundance of bacteria in the soil samples expressed as the number of targeted sequences (Amplified Units) per gram of dry soil (AU g-1 dry soil). These values were normalized, and a log transformation was applied.
Library construction and sequencing
The prokaryote rRNA 16S (V4 region) gene from the soil DNA extracts were prepared for Illumina sequencing as described (39). Briefly, the gene was amplified using the 515FB and 806RB primers (40, 41) via a two-step dual-indexed PCR approach that was specifically designed for Illumina instruments by the Plateforme d’analyses geínomiques (IBIS, Universiteí Laval, Quebec City, QC, Canada).
Statistical and bioinformatic analyses
The agronomic data collected were subjected to one-way analysis of variance (ANOVA) using SAS (Statistical Analysis System) software, version 9.3., following the mixed linear model procedure. Treatments, time-periods, repetitions and the interactions mean were compared by performing post hoc Duncan’s test at the p-value threshold of P ≤ 0.05.
Sequence analysis was performed under QIIME2 (42) using the DADA2 filtration approach (43) for determining the Amplicon Sequence Variants (ASV). The taxonomic assignment of ASVs was performed using a pre-trained classifier based on Silva (version 138) reference database (44) and a classification approach (45, 46).
R Studio software (version 4.1.3) was used to analyze the diversity indices, and the relative abundance of the most abundant taxa with treatment, sampling time points and blocks were considered as fixed factors. Non-normal data were normalised and scaled. Each of the inferred single DNA sequences recovered from a high-throughput analysis of marker genes, namely ASV, were subjected to several analyzes. Indeed, bacterial alpha-diversity (Shannon), richness (Chao1), evenness (Pielou’s evenness), and observed number ASVs were estimated via Phyloseq package (47) using an Anova, following by a post hoc Duncan’s test at the p-value threshold of p ≤ 0.10, based on the selected ASVs table at a depth of 6 890 sequences per sample. Non-metric multidimensional scaling (NMDS) and hierarchical classification analysis were performed using the Bray–Curtis distance matrix, using microeco package (48). Analysis of the β-diversity of the bacterial community, as well as the multilevel pairwise comparisons between treatments and sampling time-periods were done using a permutational multivariate analysis of variance (PERMANOVA) via the Adonis function (with 999 permutations) as described by (49). Differential gene expression analysis based on the negative binomial distribution was carried out using DESeq2 package described by (50). In order to analyse the changes in ASVs abundance and composition over time, we used Venn diagram via the package microeco (48).
To investigate the relationships between bacterial populations within the community in soil, we utilized an artificial network to examine co-occurrence patterns, by calculating all possible Spearman’s rank correlations between ASVs (2558 ASVs) at the correlation threshold (P = 0.0001). Indeed, Spearman correlation based on WGCNA package through the RMT theory (32) was applied to select the correlation threshold (COR_p_thres = 0.01, COR_cut = 0.8). This previous filtering step was applied to remove poorly represented ASVs and reduced network complexity, facilitating the determination of the core soil community, as described by (51). To summarize the variation in relative abundance of ASVs within a module (as recommended by (34), we used the module eigen-gene, following the method described by Deng et al. (32). We identified communities or modules within the network using modularity, as described by (52). To assign each node’s role based on its within-module and among-module connectivity, we followed the approach outlined by (53). Various common network properties were measured to describe the network’s topological properties. This included connectedness, which is one of the most important measurements for summarizing hierarchical structures (54). Node degree, also known as connectivity, is the most commonly used concept for describing the topological property of a node in a network (55). Clustering coefficient, which describes how well a node is connected with its neighbors (56). Density, which is closely related to the average connectivity (57). Betweenness, which is used to describe the ratio of paths that pass through the ith node (a high betweenness node can serve as a broker similar to stress centrality, (58). Centralization of degree, which is close to 1 for a network with a star topology and close to 0 for a network where each node has the same connectivity (57). Then, statistical analyses were carried out in the R studio environment, using microeco (48) and igraph (59) packages. Finally, we visualized the resulting network with node colors indicating calculated modules using Gephi’s program (60) via the ForceAtlas2 (61) and Fruchterman-Reingold layouts algorithms.
To estimate the number of nodes connected between different taxa or within one taxon in the soil, the function plot_sum_links was used to present the positive links from the function cal_sum_links via the chorddiag package (https://github.com/mattflor/chorddiag).
Results
The results showed the use of the fumigant alone or with a biostimulant reduced common scab and deep-pit scab disease indices and the net yield losses to less than 2.5%. The fumigant persistently altered the soil bacterial community composition over the growing season. The inoculation of the biostimulant caused distinct modifications to bacterial composition depending on whether the soil has been fumigated or not. Artificial network inference analysis showed that fumigation leads to a reduction in network interactions, while the biostimulant increased the interactions and the number of connections within a phylum or between different taxa. Detailed results will be presented in the next sections.
Agronomic performance of potato plants and incidence of common scab disease
Several variables (number of tubers per plant; percentage of tubers showing superficial scab lesions; percentage of tubers showing deep-pit scab lesions; superficial scab index, deep-pit scab index, gross yields and marketable yield) were measured to assess the agronomic performance of potato plants and the incidence of common scab disease in control plots and in plots that received the application of the fumigant Chloropicrin Pic Plus alone or in combination with the Bacillus-species-based biostimulant (Table 3). Variability was observed among the plots depending on the combination of variables and treatments. The biostimulant alone (T2) as well as the fumigant alone (T3) or combined with the biostimulant (T4) treatments in comparison to the non-fumigated control soil (T1) favored a significant (p ≤ 0.05) increase in the number of tubers per plant (Table 3).
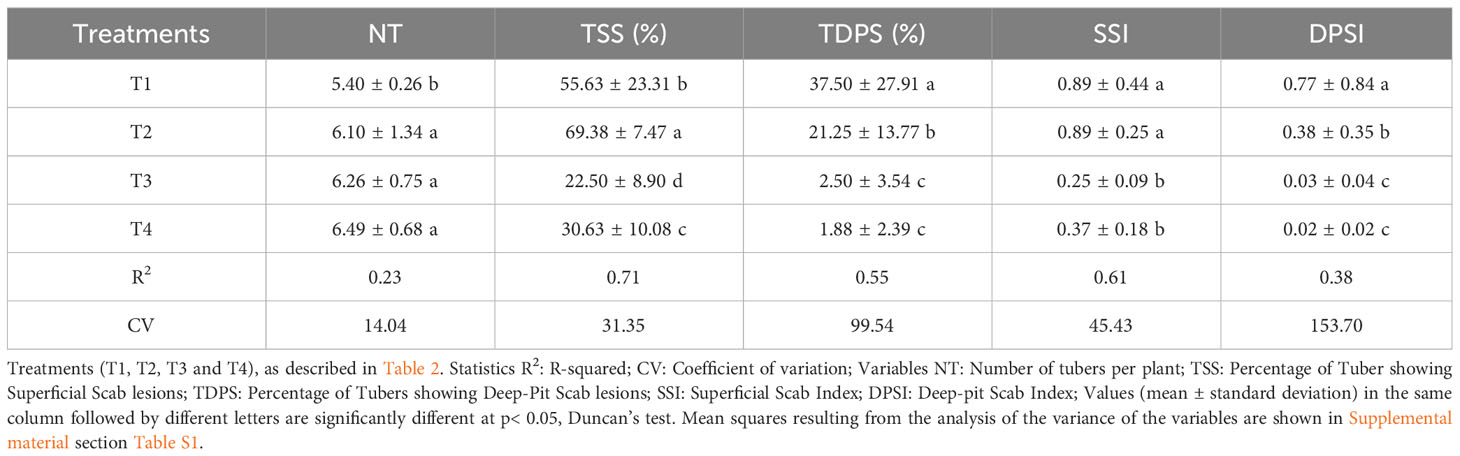
Table 3 Physio-agronomic performance of potato against common scab in soil treated with fumigant and biostimulant alone or in combination.
On average in control plots T1, 55.63% and 37.5% of tubers were respectively infected with superficial scab or with deep-pit scab, while T2 plots showed respectively 69.38% and 21.5% of diseased tubers. In fumigated plots T3, 22.5% and 2.50% of tubers were respectively infected with superficial scab or with deep-pit scab, while T4 plots showed respectively 30.63% and 1.8% of diseased tubers (Table 3). The fumigation treatment alone or in combination with biostimulant significantly (p ≤ 0.05) reduced the superficial scab index (SSI) and the deep-pit scab index (DPSI) values calculated for potato tubers harvested in T3 and T4 plots. The biostimulant inoculation of non-fumigated soil (T2) significantly (p ≤ 0.05) increased the percentage of tubers showing superficial scab symptoms, while it decreased the percentage of tubers showing deep-pit symptoms and decrease by half the deep-pit scab index in comparison to those from T1 plots (Table 3).
As shown in Figure 1, the average potato gross yields were 332.98, 317.23, 356.43 and 360.43 cwt. acre-1 in respectively T1, T2, T3 and T4 plots, with a significant (p ≤ 0.05) difference observed between the fumigated and non-fumigated plots. The fumigated soil in T3 and T4 plots promoted a yield increase of respectively 23.5 cwt. acre-1 (7.06%) and 28 cwt. acre-1 (8.41%), in comparison to the average gross yield of T1 plots. The common scab disease intensity affected the potato marketable yield especially when considering tubers showing symptoms of superficial lesions covering more than 10% of tuber surface and tubers showing any deep-pit symptoms. It caused potato marketable yield losses of 46.30% in control plots (T1), while the losses were significantly (p ≤ 0.05) reduced to respectively 23.13% (T2), 2.50% (T3) and 2.50% (T4) in treated plots.
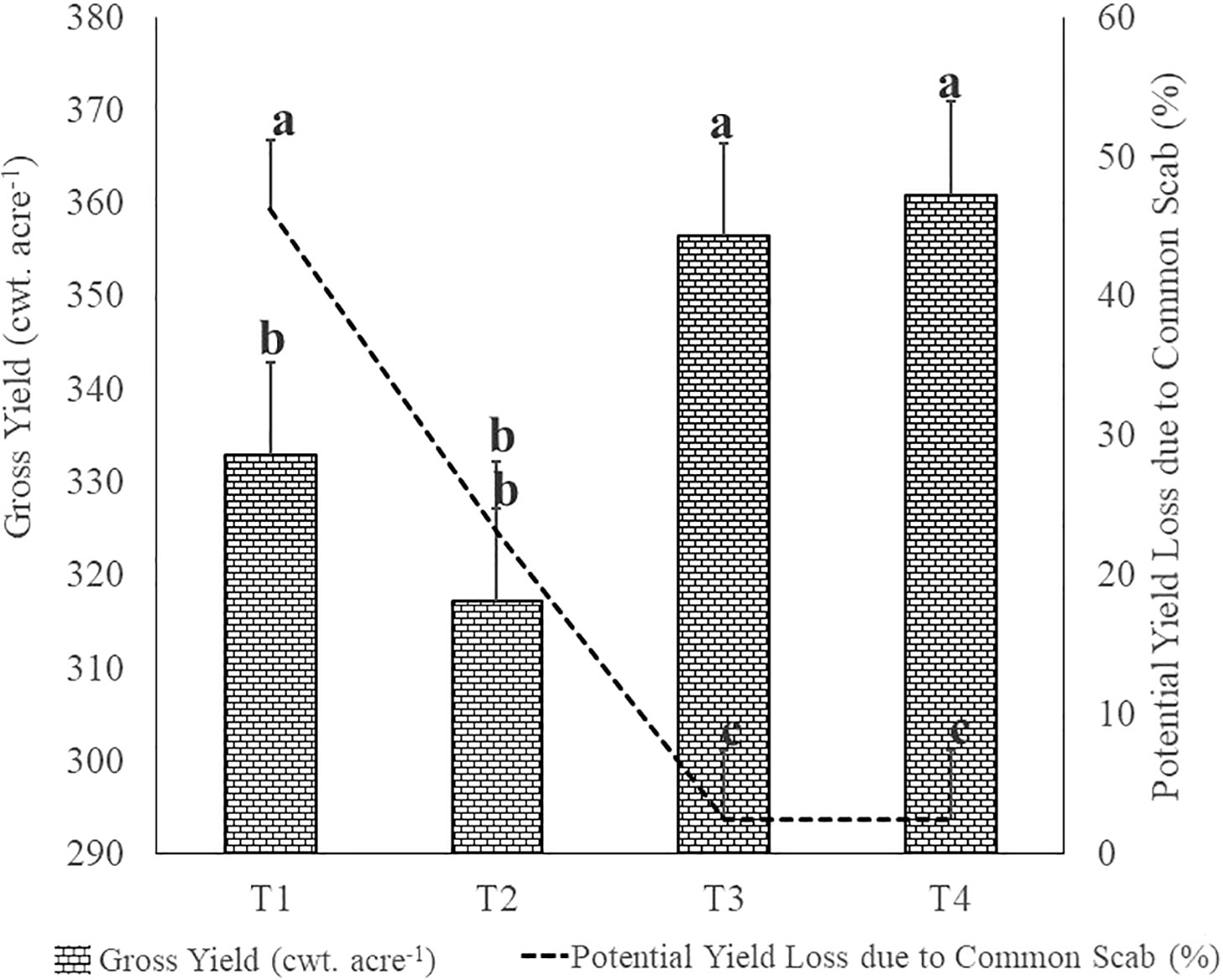
Figure 1 Effects of the treatments on Potato Gross Yield and on relative proportion of Potential Yield Losses due to the severity of common scab (superficial lesions coverage of tuber surface ≥ 10%) and the presence of deep-pit scab symptoms. The letters indicate significant difference at p< 0.05 according to one-way analysis of variance (ANOVA) among treatments as described in Table 2. T1: Non-fumigated soil without biostimulant, T2: Non-fumigated soil with biostimulant, T3: Fumigated soil without biostimulant, T4: Fumigated soil with biostimulant.
Relative abundance of bacteria in soil throughout the growing season
The relative abundance of bacteria in the soil was assessed using a qPCR protocol with specific primers of V3-4 regions of bacterial 16S rRNA and it showed a significant effect of the fumigation of the soil over the growing season periods. Variability was observed in the number of bacteria among treated soil as a function of season periods (Figure 2). At pre-treatment application period P1, the average quantity of bacteria was estimated at 9.60± 0.07 Log AU g-1 dry soil among all experimental plots. At P2 (6 days after fumigation), an average of 9.51± 0.09 Log AU g-1 dry soil for unfumigated plots T1 and T2 plots were detected in comparison to an average of 9.43± 0.07 Log AU g-1 dry soil in fumigated T3 and T4 plots (data not shown). Overall, we observed that the short-term effect of fumigation was not perceptible using qPCR to estimate the number of soil bacteria.
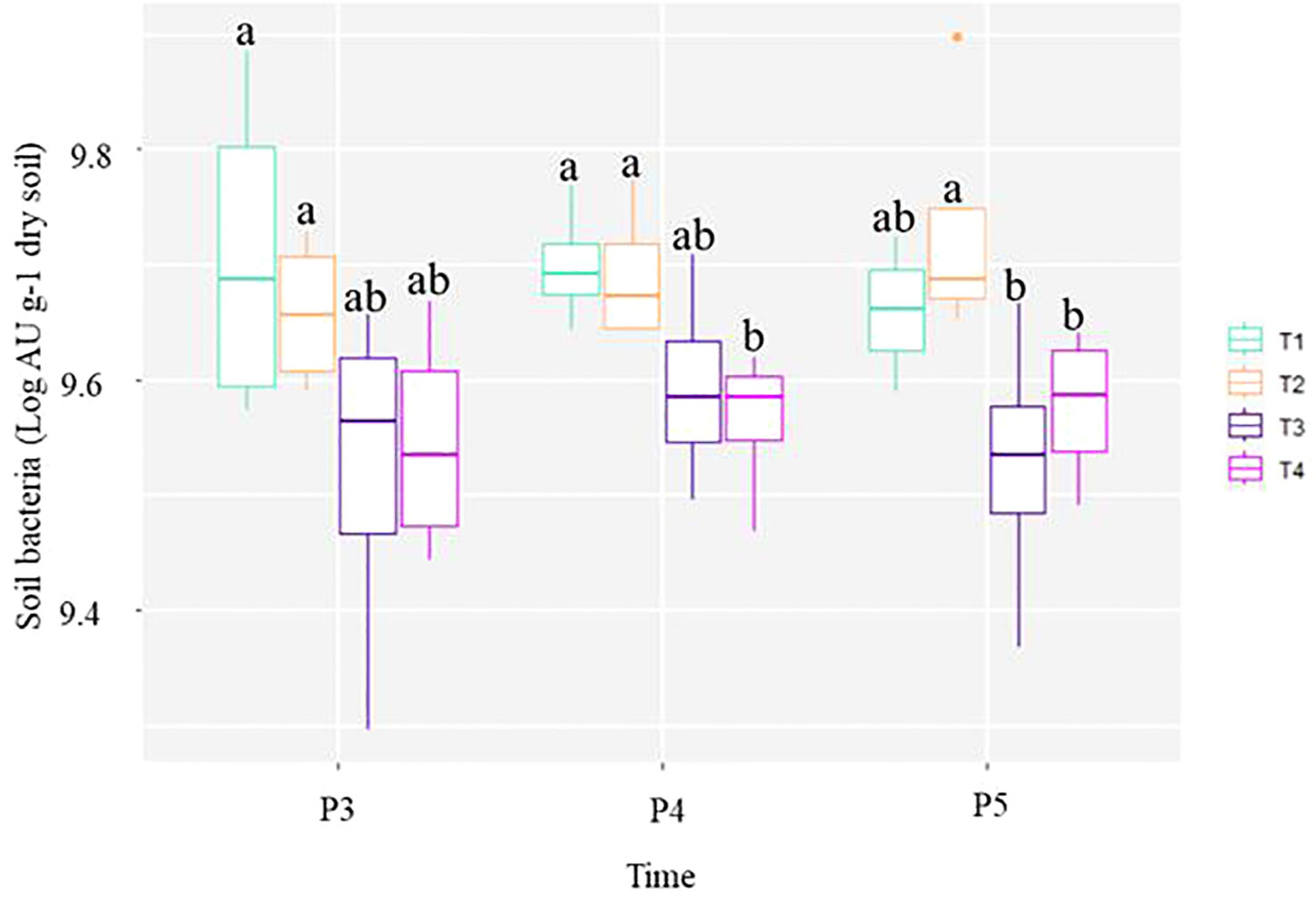
Figure 2 Box and whisker plots showing the quantitative abundances of soil bacteria at each treatment and sampling period point. The line inside the box represents the median, while the whiskers represent the lowest and highest values within the 1.5 interquartile range (IQR). Treatments comprised: non-fumigated soil without biostimulant (T1), Non-fumigated soil with biostimulant (T2), Fumigated soil without biostimulant (T3), Fumigated soil with biostimulant (T4). Significant differences (Duncan test, P ≤ 0.05) among treatment means for individual sampling points are represented by letters above the boxes.
Figure 2 shows that the average total number of bacteria in T1 and T2 plots remained at the same level throughout the study. On the other hand, the average number of bacteria in T4, started to significantly (p ≤ 0.05) decrease at P4, and both fumigated treatments (T3 and T4) showed significant decrease at P5. The overall trend depicted in the Figure 2 indicates that bacterial counts were higher in soils that were not fumigated. This suggests that the chloropicrin treatment effect of lowering the number of bacteria in soil may takes few weeks to be perceived by qPCR. The study showed that in comparison to the number of soil bacteria estimated in control T1 plots from P3 to P5 sampling dates, chloropicrin fumigation caused a persistent decrease of bacteria number in T3 and T4 plots throughout the growing season. Additionally, the application of the biostimulant in the furrow at plantation in unfumigated (T2) or fumigated (T4) soils tends to slightly increase the soil bacteria ribosomal amplified unit per gram of dry soil detected at P5 sampling date by the end of the growing season (Figure 2).
Alpha-diversity of bacterial communities
Four alpha diversity indices were measured to evaluate the effect of treatments and soil sampling time-periods on the alpha-diversity of bacterial communities (Figure 3).
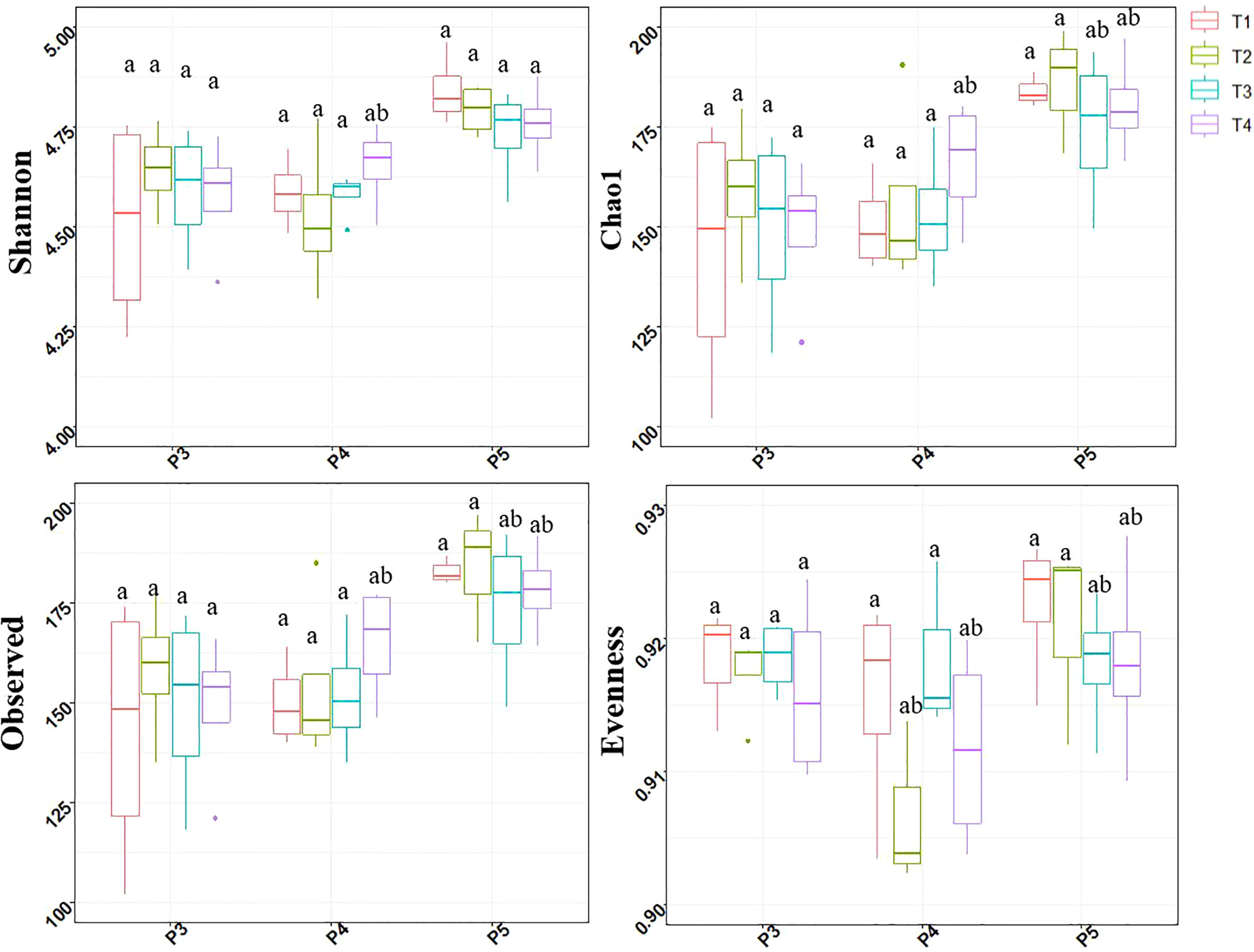
Figure 3 Box and whisker plots showing the Shannon diversity, Pielou’s evenness, Chao1 richness and observed ASV’s number distributions at each treatment and sampling period point. Treatments included: non-fumigated soil without biostimulant (T1), Non-fumigated soil with biostimulant (T2), Fumigated soil without biostimulant (T3), Fumigated soil with biostimulant (T4). Significant differences (Duncan test, P ≤ 0.05) among treatment means for individual sampling points are represented by letters above the boxes.
The treatments had no effect on alpha-diversity indices at P3 periods. At P4 period the combination of fumigation and biostimulant (T4) slightly increased the Shannon, Chao1 and Observed indices while the Evenness indice values observed in biostimulant inoculated T2 and T4 plots were reduced. At P5 period, the Chao1, Observed and Evenness indices values observed in fumigated soils (T3 and T4) were slightly lower.
Soil bacterial diversity composition as a function of treatments over time
To compare the soil bacterial diversity composition of each of the four treatments sampled at each time-period, we performed a permutational multivariate analysis of variance which showed highly significant (p ≤ 0.05) differences between the different treatments and sampling time-periods (Table 4), suggesting that the soil bacterial communities composition varies between treatments and times.
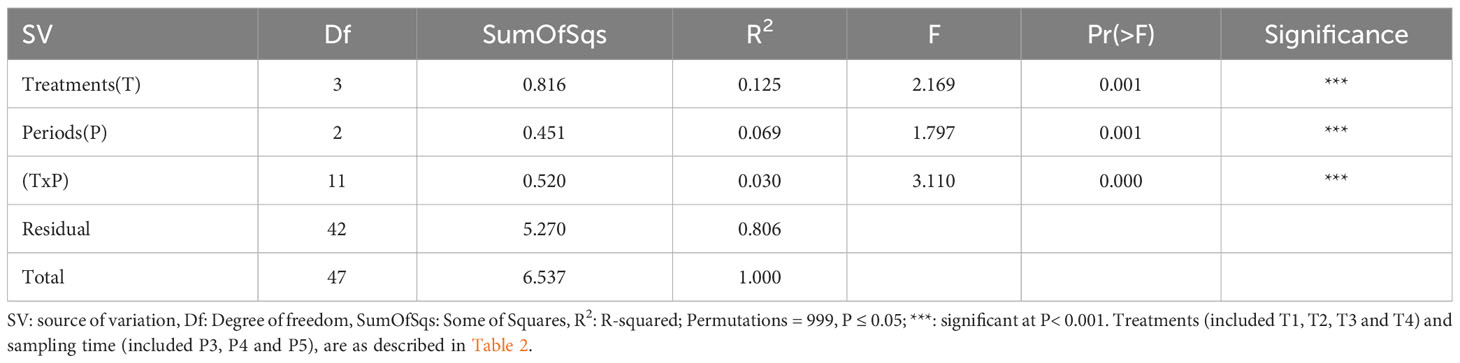
Table 4 Permutation test for adonis under reduced model of bacterial community composition in soils.
Furthermore, the NMDS analysis revealed that there was an evolution in the bacterial community composition in soils under potato cultivation throughout the season (Figure 4). At the initial stage (P2), six days after the fumigation and four days before the inoculation of Bacillus species-based biostimulant in the furrow at plantation, we observed that the bacterial communities in the soils showed no clear dissimilarity. This suggests that the short-term effect of fumigation was not yet perceivable based on the NMDS analysis of soil bacterial communities. However, as the potato growing season progressed from P3 to P5, we observed a significant (p ≤ 0.05) dissimilarity between communities of the treatments involving non-fumigated soils (T1 and T2) and fumigated soils (T3 and T4), as presented in Figure 4 and Table 5. If overall, the bacterial community compositions of the soils inoculated with the biostimulant did not show significant dissimilarities among non-fumigated soils (T1 vs T2) or among fumigated soils (T3 vs T4) at periods P3 to P5 (Table 5), it is however interesting to observe that Table S2 (Supplemental material) shows a significant (p ≤ 0.05) dissimilarity between the bacterial composition of fumigated soil T3 and those of non-fumigated soils T1 and T2 at periods P3 to P5, while the bacterial composition of fumigated soil inoculated with biostimulant T4 and those of soils T1and T2 are not significantly dissimilar at the same periods. This may indicate that the biostimulant had induced a slight effect on the variability of the bacterial community composition in fumigated soils of treatment T4.
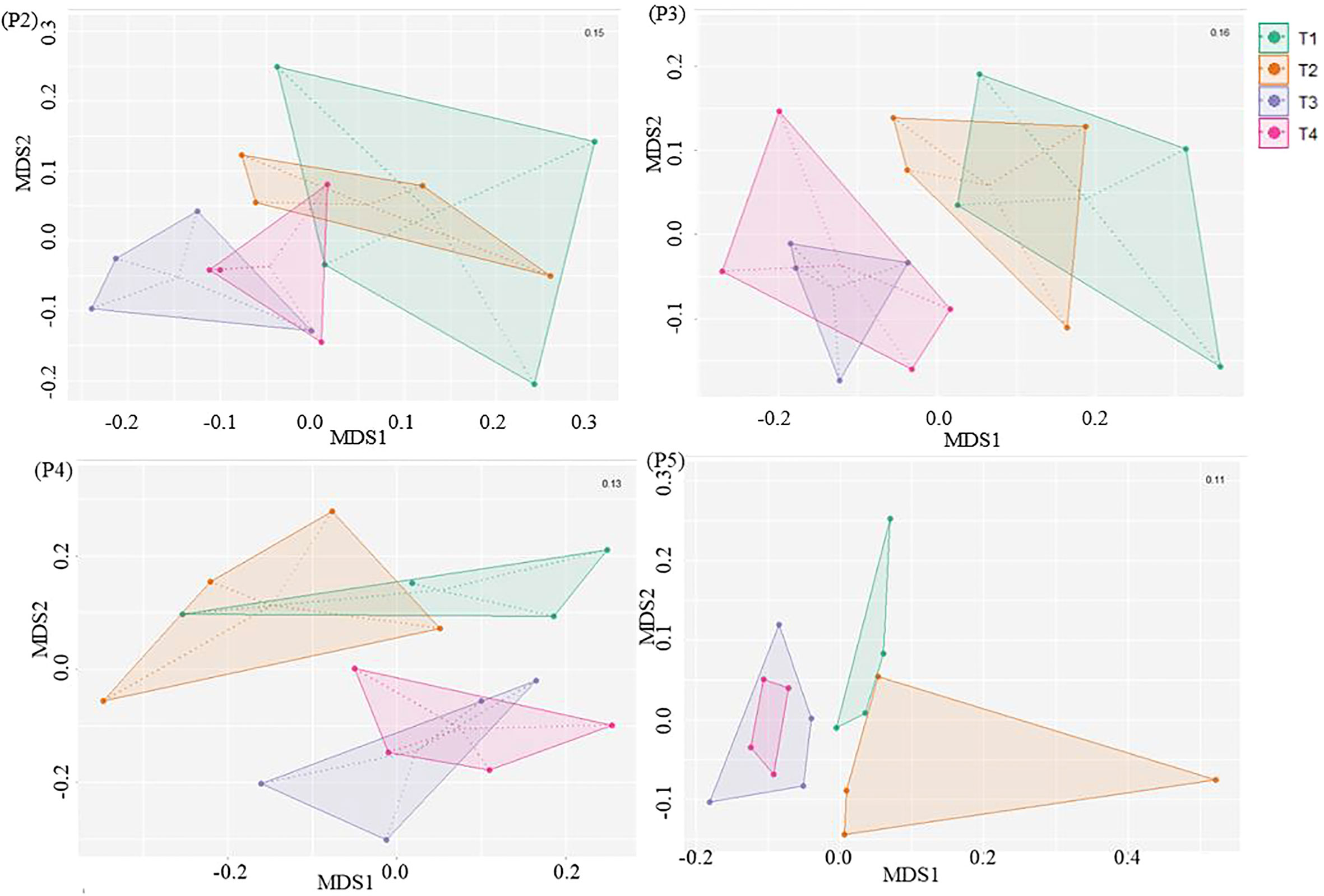
Figure 4 NMDS ordination based on Bray–Curtis distances comparing the bacterial community compositions among treatments at sampling time-period P2, P3, P4 and P5. Treatments and sampling time-periods were as described in Table 2.
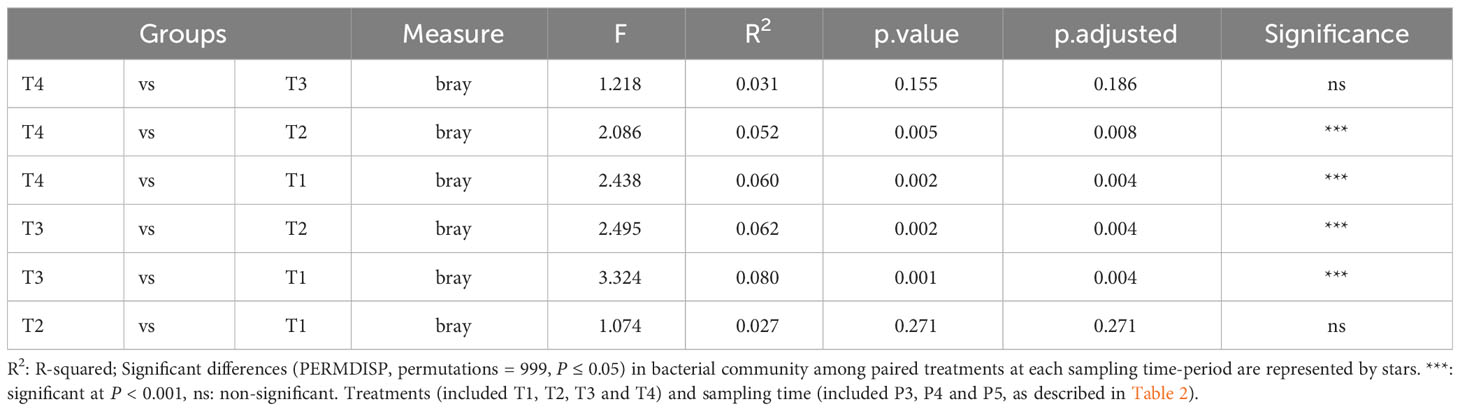
Table 5 PERMDISP test implemented to check multivariate homogeneity of treatments dispersions (variances) of bacterial community compositions in soils sampled over all P3, P4 and P5 periods.
Bacterial community composition at phylum level
We analyzed changes in the proportions of twelve main bacterial phyla over time. Our results showed that fumigation alone and biostimulant inoculated in non-fumigated or fumigated soils treatments affected several phyla and most of them represented relative proportion< 2% of the bacterial community. As shown in Figure 5, the fumigation alone (T3) resulted in a significant (p ≤ 0.05) reduction of the relative proportions of several bacterial phyla at different time-periods over the growing season. The reduction effect was mostly detected at P3 period in Crenarchaeota, Planctomycetota and Myxococcota and at P4 period in Verrucomicrobiota, while in P5 period the reduction effect of fumigation alone was observed in Firmicutes, Crenarchaeota and Methylomirabilota. Interestingly, the relative proportions of phyla Gemmatimonadota (P4 and P5), Proteobacteria (P5) and Myxococcota (P5) slightly increased in T3 plots.
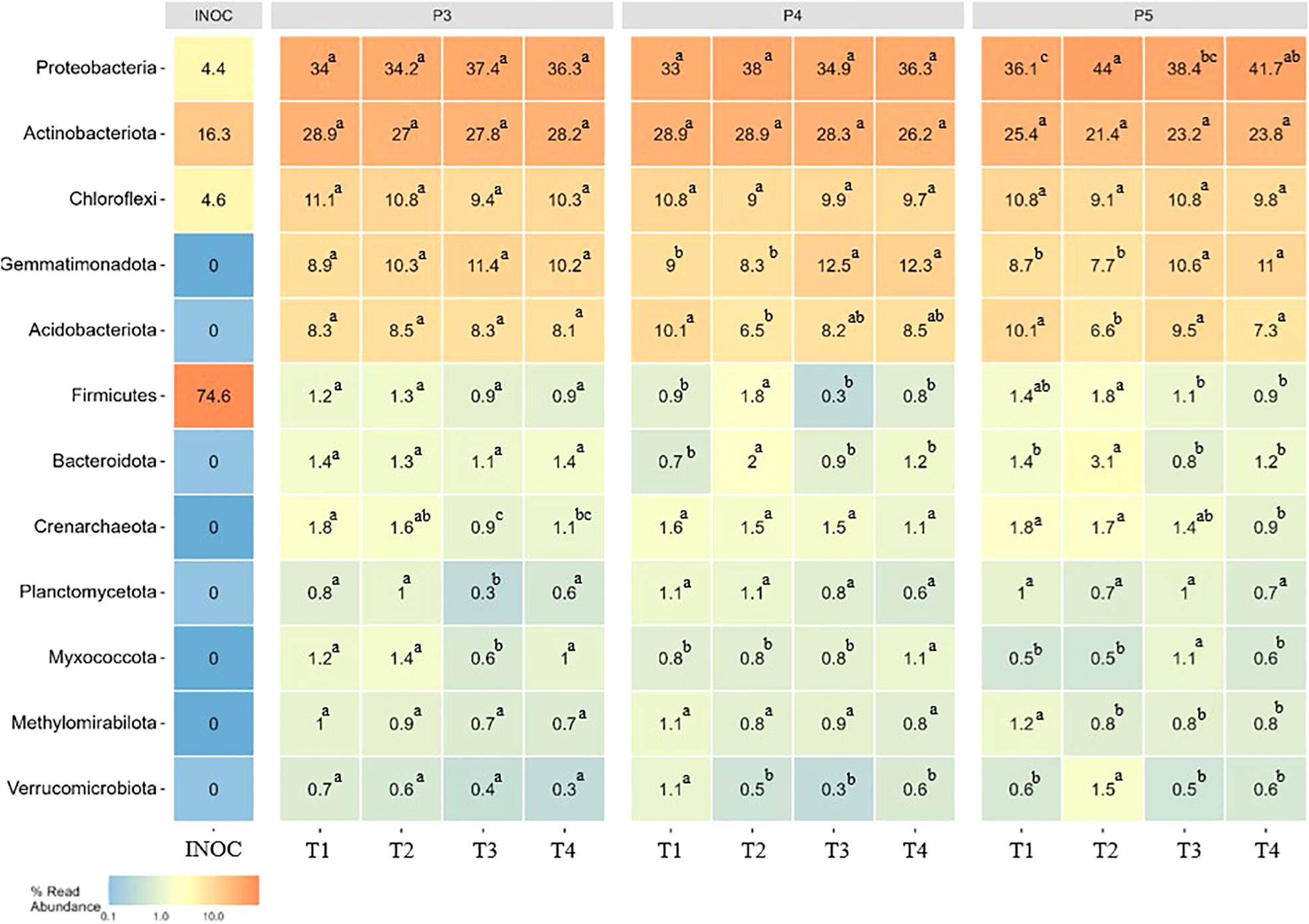
Figure 5 Heatmaps showing the relative abundance of 12 top bacterial phyla in different treatments over time. Treatments and sampling time-periods are as described in Table 2. Significant differences (p ≤ 0.05) among treatment means for bacterial phyla are represented by letters. The column INOC indicates the relative proportions of phyla that were detected in the biostimulant applied in the furrow at potato plantation.
The inoculation of the Bacillus species-based biostimulant alone (T2) modified the relative proportions of few phyla among the 12 most abundant one (Figure 5). The significant (p ≤ 0.05) decrease effect was mostly detected at P4 period in Verrucomicrobiota, at P5 in Methylomirabilota and at P4 and P5 periods in Acidobacteriota, while the biostimulant showed a significant (p ≤ 0.05) increased effect on Firmicutes and Bacteroidota at P4 and P5 periods and on Proteobacteria and Verrumicrobiota at P5 period only (Figure 5).
The combined use of the biostimulant and fumigant (T4) resulted in a reduction of the decrease effect caused by the fumigation alone (T3) treatment as shown by a significant (p ≤ 0.05) increase of the relative proportions of Crenarchaeota and Planctomycetota (P3), Myxococcota (P3 and P4) and of Proteobacteria at P5 period (Figure 5). The relative proportions of Crenarchaeota and Myxococcota decreased at P5. Otherwise, the T4 treatment showed the same effect as the T3 treatment on the relative proportion of the other phyla during the season.
These results suggest that the inoculation of the biostimulant significantly modified the soil bacterial community composition in a way that seems to be different when considering the non-fumigated or the fumigated soils at least for the 12 most abundant phyla that were considered in this analysis (Figure 5).
Bacterial community composition at ASVs level
The differential analysis using DESeq2 enabled the identification of ASVs that were positively or negatively affected by the T2, T3, and T4 treatments compared to the reference T1 treatment (Figure 6A). The analysis revealed significant clustering of bacterial ASVs communities (at the phylum and family level) in the fumigated soils (T3 and T4) compared to the treatment involving non-fumigated soils inoculated with biostimulant (T2) throughout the periods P3 and P4. However, at period P5, the analysis revealed significant clustering of ASVs composition affected by treatments T2 and T3, suggesting that treatment T4 had induced significant changes in the bacterial community composition of the fumigated soil to no longer be clustered with T3 ASVs composition. Our findings highlight the significant influence of both the treatments (biostimulant and fumigation) and the time periods on specific ASVs that were positively or negatively affected among the soil bacterial community composition. In comparison to the bacterial community composition of T1, the number of ASVs positively affected by the treatments decreased from P3 to P5 periods, while the number of ASVs negatively affected progressively increased from P3 to P5 periods. (Figure 6A). Among the ASVs detected by the DESeq2 analysis, most were from a wide spectrum of taxonomic families of the phyla Proteobacteria and Actinobacteriota, while few were from Chloroflexi, Gemmatimonadota and Acidobacteriota phyla. The results of the DESeq2 analysis shown in Figure 6A are in relative accordance with results shown in Figure 5.
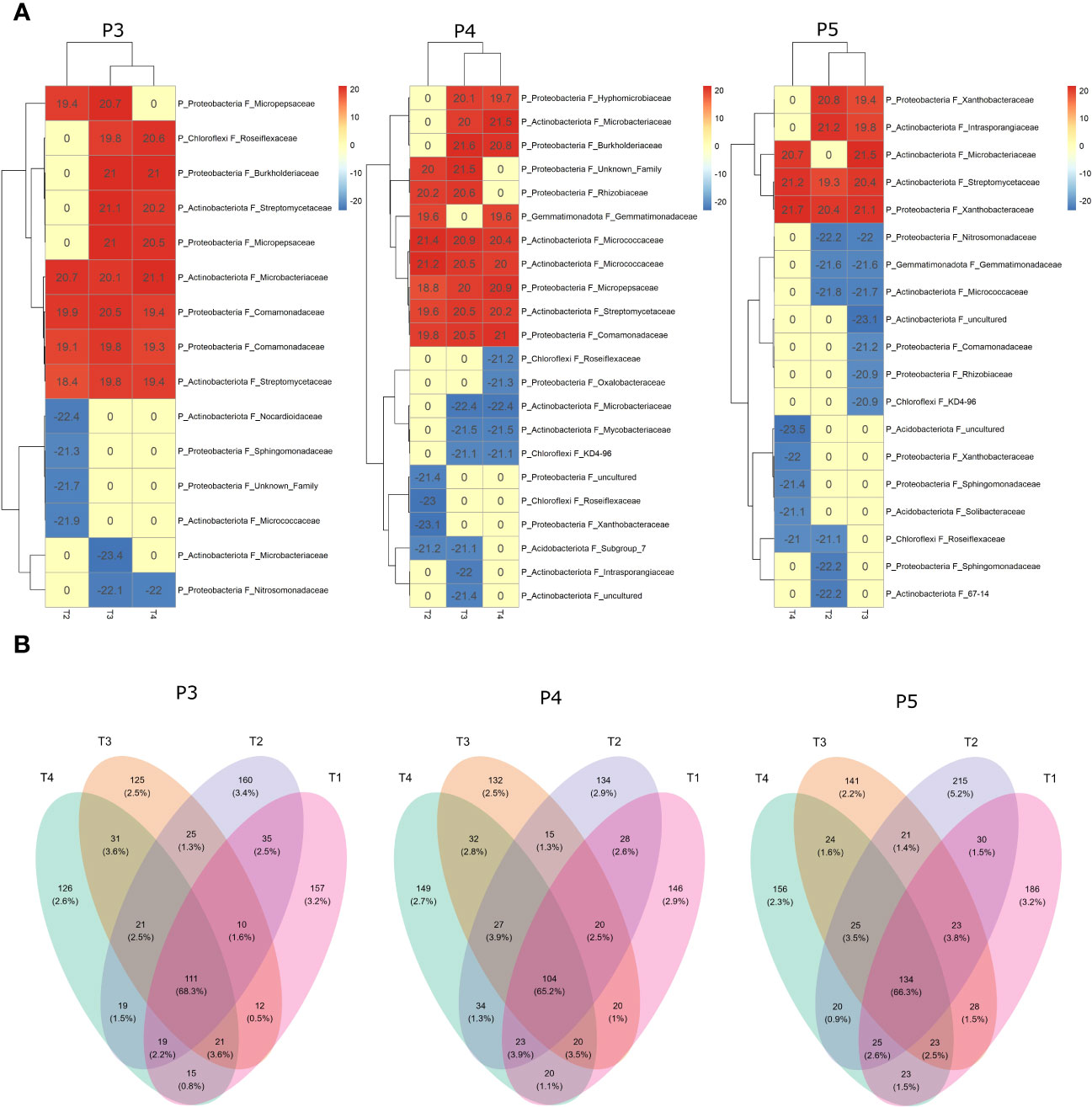
Figure 6 Differential analysis using DESeq2 (A) and Venn diagram (B) showing the overlapping rates of bacterial ASVs abundance under different treatments and time-periods during growing season. Treatments (T1, T2, T3 and T4) and sampling time-periods (P3, P4 and P5) were as described in Table 2.
We examined the impact of Bacillus species-based biostimulant and chloropicrin fumigation treatments on the number and proportion represented by the ASVs detected in the soil bacterial community. Our analysis focused on the changes in ASV abundance among various treatments combinations over time illustrated by the Venn diagrams (Figure 6B). During the period P3, we identified a total of 887 retained ASVs. Among these, 157 and 160 ASVs were exclusively found in respectively untreated control plots (T1) or biostimulant alone treated plots (T2), while 125 and 126 ASVs were exclusively found in fumigated plots (T3) or biostimulant inoculated in fumigated plots (T4). We also observed 35 ASVs that were shared between T1 and T2 for a total of 352 ASVs detected exclusively in non-fumigated soils, while a total of 282 ASVs were exclusively detected in fumigated soils at P3. Furthermore, 111 ASVs were detected in at least one plot of each treatment and those ASVs represented 68.3% of the total proportion represented by all the ASVs in the bacterial community at P3 period.
During the period P4, we identified a total of 904 retained ASVs. Among these, 146 and 134 ASVs were exclusively found in respectively untreated control plots (T1) or biostimulant alone treated plots (T2), while 132 and 149 ASVs were exclusively found in fumigated plots (T3 and T4). We also observed 28 ASVs that were shared between T1 and T2 for a total of 308 ASVs detected exclusively in non-fumigated soils, while a total of 313 ASVs were exclusively detected in fumigated soils at P4. Furthermore, 104 ASVs were detected in at least one plot of each treatment and those ASVs represented 65.2% of the total proportion represented by all the ASVs in the bacterial community at P4 period.
During the period P5, we identified a total of 1074 retained ASVs. Among these, 186 and 215 ASVs were exclusively found in respectively untreated control plots (T1 and T2), while 141 and 156 ASVs were exclusively detected in fumigated plots (T3) or biostimulant inoculated in fumigated plots (T4). We also observed 30 ASVs that were shared between T1 and T2 for a total of 431 ASVs detected exclusively in non-fumigated soils, while a total of 321 ASVs were exclusively found in fumigated soils at P5. Furthermore, 134 ASVs were detected in at least one plot of each treatment and those ASVs represented 66.3% of the total proportion of all the ASVs in the bacterial community at P5 period.
Overall, we observed a significant (p ≤ 0.05) reduction in the average 306 ASVs exclusively detected in both T3 and T4 fumigated plots across the sampling dates from P3 to P5 in comparison to the average 364 ASVs exclusively detected in T1 and T2 non-fumigated plots. An average 116 ASVs were detected in at least one plot of each treatment and they represented 66.6% of the bacterial community from P3 to P5 periods.
In summary, our findings indicate that fumigation caused the greatest reduction in common scab incidence and yield loss, while biostimulant inoculation alone reduced the incidence of deep-pit scab symptoms and yield losses to a lesser extent. In addition, fumigation and inoculation of the biostimulant in non-fumigated or fumigated soils significantly affected an average number of ASVs that represented in proportion almost a third of the bacterial community composition. These ASVs are mainly distributed among phyla and families having a relative proportion of less than 2% within the bacterial community of the treated soils. These effects persisted throughout the season and the results of DESeq2 and Venn diagram analyzes suggest that ASVs are affected differently depending on whether the biostimulant has been inoculated into non-fumigated or fumigated soils.
Effects of treatments on network interactions within the soil bacterial community
The number of nodes connected between different taxa or within one taxon in the soil were estimated to describe the effects of chloropicrin fumigation and Bacillus species-based biostimulant on the number of connections within and between different bacterial taxa in soil. Indeed, the total number of linkages (edges) for each treatment was determined by summing the edges for each phylum level. Figure 7 presents the sum of the linkage numbers for each of the four treatments at the phylum level. The linkage numbers per phylum profile observed for each treatment is quite distinctive and may highlight anticipated effects of the treatment on selected phyla.
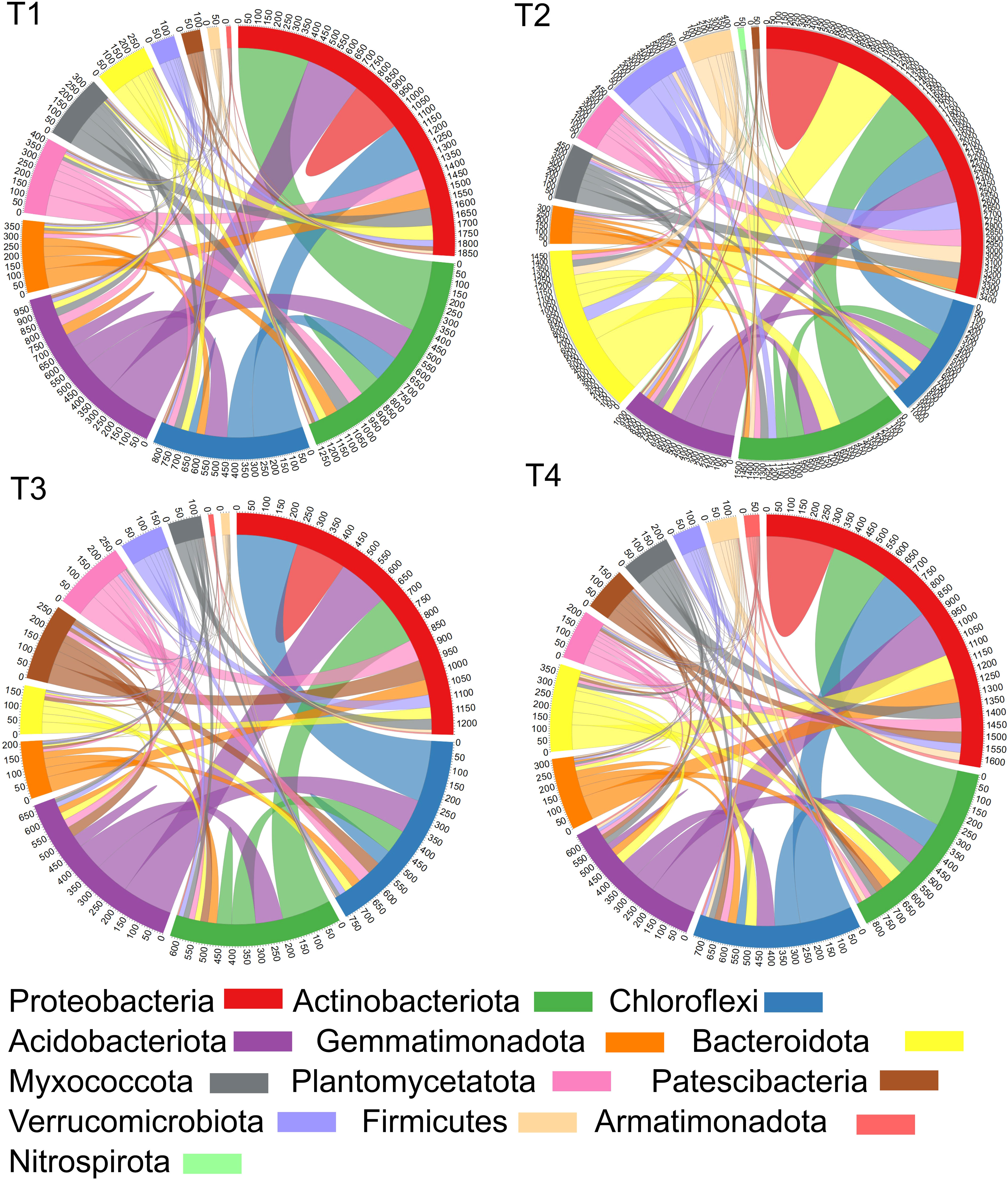
Figure 7 Plots showing the number of nodes connected between different bacterial phyla or within one phylum. It has been calculated through the sum of their edges, where the numbers along the outside of the circular plot represent how many edges or linkages are related with a Phylum. Treatments (T1, T2, T3 and T4) and sampling time-periods (P3, P4 and P5) were as described in Table 2.
Our study showed that chloropicrin fumigation (T3) reduced interactions within the soil bacterial community while the application of biostimulant to non-fumigated (T2) or fumigated (T4) soils favored a significant increase of the number of nodes connected between different phyla or within one phylum. Interestingly, we observed for the Firmicutes, the phylum grouping the Bacillus species, that the application of Bacillus species-based biostimulant promoted an eight-fold increase of the number of edges in non-fumigated soil (T2 vs T1) and two-fold in fumigated soil (T4 vs T3).
For the Proteobacteria phylum, the control soils (T1) showed approximately 1850 edges associated with ASVs in Proteobacteria. Among these, around 300 edges connected ASVs within Proteobacteria, around 400, 300, and 350 edges connected ASVs from Proteobacteria with Actinobacteriota, Chloroflexi, and Acidobacteriota respectively, while lower number of edges among the remaining 500 edges connected with eight other phyla (Figure 7). Five phyla (Proteobacteria, Actinobacteriota, Chloroflexi and Acidobacteriota) showed high proportions of edges connected with other phyla. In contrast, fumigated soils without biostimulant application (T3) bacterial community showed around 1250 edges associated with ASVs in Proteobacteria which is 32% less edges than those estimated in T1 plots. Among the 1250 edges, around 200 edges connected ASVs within Proteobacteria, and approximately 200, 250, and 200 edges connected ASVs from Proteobacteria with other ASVs from Actinobacteriota, Chloroflexi, and Acidobacteriota, respectively, while lower number of edges among the remaining 400 edges connected to eight other phyla. Also, in comparison to the number of connections per phylum profile seen in T1 plots, the T3 profile showed a decreased number of edges for eight among thirteen phyla (Proteobacteria, Actinobacteriota, Chloroflexi, Acidobacteriota, Gemmatimonadota, Bacteroidota, Myxococcota, Planctomycetota) suggesting that the fumigation decreased significantly the connection status among phyla (Figure 7).
The inoculation of biostimulant in non-fumigated soil (T2) showed quite distinctive connections profile in comparison to those of T1 or T3 plots. The T2 vs T1 treatment increased the number of edges per phylum for 10 among 13 phyla while with T2 vs T3 treatment this increase was observed for 11 phyla. Around 3400 edges were associated with ASVs of Proteobacteria in T2 plots which is around 84% more edges than those estimated in T1 plots. Among these, approximately 700 edges connected ASVs within Proteobacteria, and around 500, 350, and 350 edges connected ASVs from Proteobacteria with other ASVs from Actinobacteriota, Chloroflexi, and Acidobacteriota, respectively. The remaining 1500 edges connected with the eight other phyla, and notably, a greater increase of edges was estimated in Bacteroidota, Firmicutes and Verrucomicrobiota phyla in T2 connections profile (Figure 7).
Finally, in T4 plots approximately 1650 edges were associated with ASVs in Proteobacteria which is around 33% more edges than those counted in T3 plots and 11% less than in T1. In comparison to T3 profile, T4 treatment has increased the edges number for five phyla (Proteobacteria, Actinobacteriota, Gemmatimonadota, Bacteroidota, Myxococcota) and decreased the edges number for five phyla (Chloroflexi, Acidobacteriota, Planctomycetota, Patescibacteria, Verrucomicrobiota). In comparison to T2 profile, the number of edges per phylum induced by T4 treatment remained lower in 10 phyla (Figure 7).
Analysis of modular structure and eigengene artificial networks inference in the soil bacterial community
In this study, ecological relationships between soil bacterial communities treated with fumigant and bacteria-based biostimulant against potato’s common scab were predicted by building indirected hypergraphs of complex systems from the behavior of their components in soil bacterial communities. Network interactions were established for each of the four treatments (Figure 8) by combining all significant pairwise relationships. The modular structure analysis statistics, including connectedness, connectivity, density, betweenness, and centralization, are presented in Table 6.
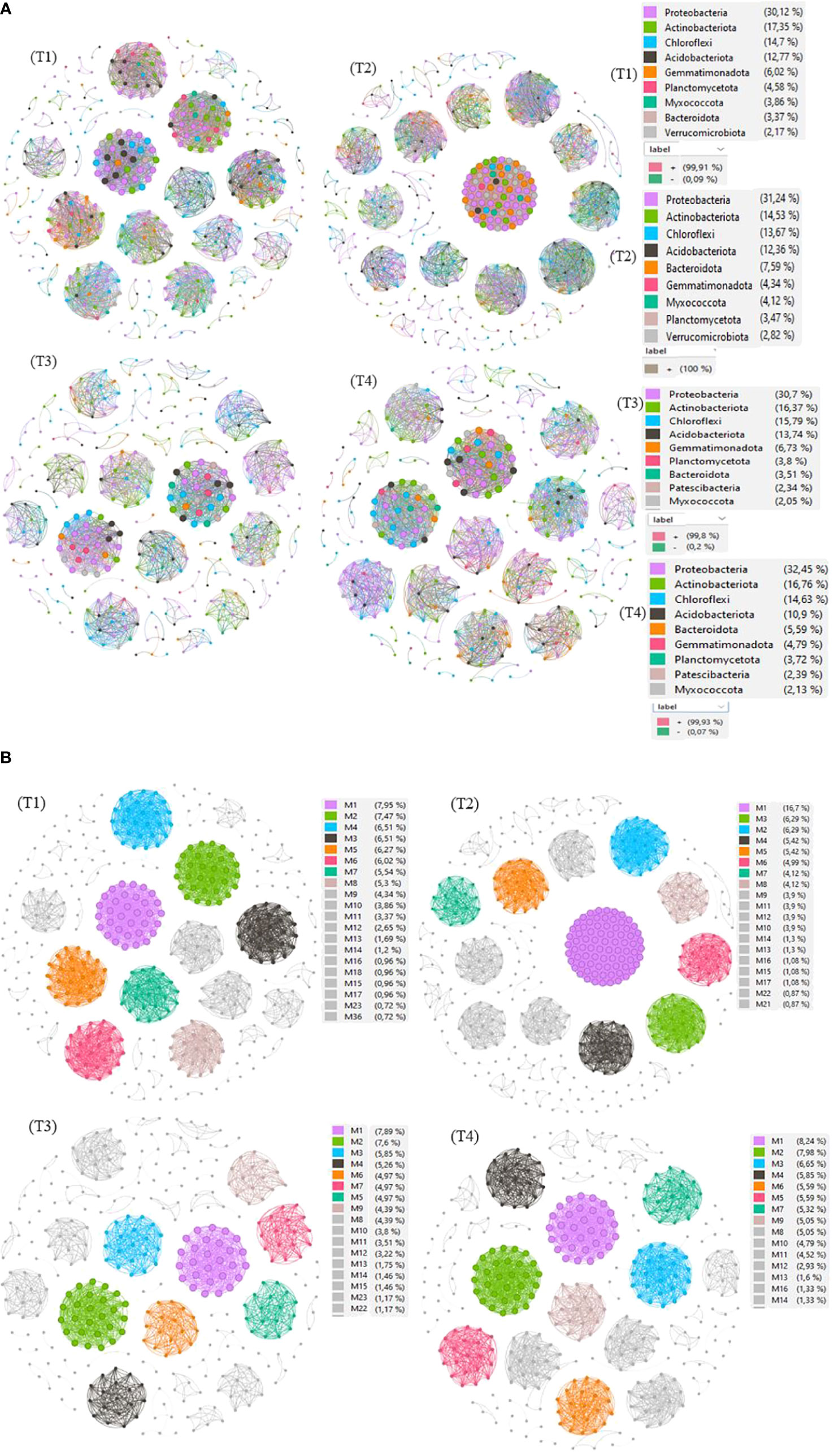
Figure 8 Co-occurring network of bacterial communities at Phylum level (A) and modularity class (B) across treatments. The nodes in network are colored by modularity class. The connections stand for a strong (Spearman’s ρ > 0.8) and significant (p< 0.01) correlations. The node sizes reflect their degree of connection (edge numbers assigned to the node) in non-fumigated soil without biostimulant (T1), Non-fumigated soil with biostimulant (T2), Fumigated soil without biostimulant (T3) and Fumigated soil with biostimulant (T4).
The results revealed several insights. Firstly, the T2 treatment, which involved non-fumigated soil inoculated with a biostimulant, exhibited the highest number of edges (5686), linkages (461), average degree (24), network diameter (4), density (0.05), betweenness (1.01), and centralization (0.11). This treatment also had 64 modules with relative abundances ranging from 0.01% to 3.48% and 100% positive interactions. In contrast, the T3 treatment (fumigated soil) showed the lowest values for these network properties. Specifically, T3 had 1967 edges, 342 linkages, and 61 modules with relative abundances ranging from 0.01% to 1.70%, with 99.8% and 0.2% of positive and negative interactions, respectively.
The analysis indicated that fumigation treatment reduced interactions within the soil bacterial community, whereas biostimulant application increased the number of nodes and linkages, both between different taxa and within a taxon (Figure 8A). These results suggest a shift from competition to cooperation in utilizing available nutrients. Additionally, the biostimulant treatment resulted in an increase in the ratio of positive associations, as well as in network diameter and density. Conversely, fumigation alone led to a decrease in interactions. Moreover, the application of the biostimulant, in both non-fumigated and fumigated soils, increased interactions and the number of connections between different taxa or within a taxon. Notably, the treatment combining the fumigant and biostimulant exhibited a moderate increase in various network properties, providing evidence for the positive effect of inoculation of biostimulant on bacterial communities in fumigated soils.
Figure 8A also provides insights into the direction of network interactions, indicating whether they are positive or negative. In plots that received the T4 treatment (fumigated soil treated with biostimulant), we observed a moderate number of connectedness/edges (2851), linkages (376), average degree (15.16), average path length (1), network diameter (2.00), clustering coefficient (1.00), density (0.04), betweenness (0.67), centralization (0.04), and modules (58). The relative abundances of these modules ranged from 0.01% to 0.89%, with 99.93% positive interactions and 0.07% negative interactions. The correlations between the modules’ own genes were used to define the own gene network, and further details about the components of each module can be found in Figure 8B and Supplementary Table S3.
In summary, our findings highlight the intricate modular structure and network dynamics within the soil bacterial community under different treatments. The application of biostimulant and fumigation had significant effects on the network properties, with biostimulant treatment promoting increased interactions and connections. These results contribute to our understanding of the ecological relationships in the soil bacterial community and the potential benefits of biostimulant application for enhancing microbial interactions in fumigated soils.
Discussion
Performance of potato plants and incidence of common scab
Our study aimed to investigate the impact of a Bacillus species-based biostimulant to reduce negative effects on soil bacterial community of chloropicrin fumigation used for potato production against common scab. By conducting our study as a field experiment, we aimed to determine the effects of the treatments under real-world effectiveness context. The high economic losses caused by common scab in potato crops highlight the need for sustainable disease management strategy effective against this endemic soil-borne disease. Our study found that common scab causes significant losses in marketable potato yield, with more than 46% loss. This is consistent with previous research which has estimated that common scab causes significant economic losses of between 15 and 17 million dollars per year in Canada (1). The biostimulant, consisting of eight bacterial isolates of five species of Bacillus, when applied alone in the furrow on seed potatoes, showed that it could interact with microbial activities in the soil (62). Our study reports that the fumigation alone treatment (T3) caused the greatest reduction of the number of tubers showing common scab and deep-pit scab symptoms, the common scab and the deep-pit scab indices, and it significantly reduced the gross and marketable yield losses. Meanwhile, biostimulant inoculation alone (T2) reduced the deep-pit scab index and the marketable yield losses to a lesser extent. The combination of chemical fumigation and biostimulant application (T4) did not show additive positive impact on potato yield and on reducing common scab and deep pit scab incidence. However, the inoculation of the biostimulant in non-fumigated or fumigated soils showed slight effect on bacterial alpha-diversity and distinctive effects on soil bacterial community composition.
Relative abundance and alpha-diversity indices of soil bacteria
Our findings showed that on average, the chloropicrin fumigation alone or with a biostimulant inoculated at plantation on potato seeds in furrow 10 days after fumigation induced a reduction of 1.38 Log AU g-1 dry soil in bacterial abundance by the end of the growing season. Gullino et al. (26) reported that chloropicrin fumigation can effectively eliminate more than 85% of soil bacteria, fungi, and Actinobacteriota. In their study on tomato production systems, they evaluated the efficacy of chloropicrin as a possible alternative fumigant to methyl bromide using a cultivation-dependent bioassay method to monitor pathogens inoculum survival on Petri plates containing potato dextrose agar. While the average bacterial abundance reduction efficacy, estimated by qPCR, is much lower than the efficacy previously reported, our results still showed that chloropicrin treatment alone significantly greatly reduced common scab and deep-pit scab indices and marketable potato yield losses.
Experimental factors such as soil properties, rate of DNA recovery in soil extracts and time-periods used to monitor treatment effects may have affected the qPCR estimation efficacy of bacterial abundance in our soil samples. However, using high throughput sequencing (HTS) of 16S rRNA to assess the combined effects of Bacillus species-based biostimulant and chloropicrin fumigant on soil bacterial communities, we gained more detection sensitivity than the level obtained by cultivation-dependent methods. HTS can also provide the extensive detection and identification efficacy that is needed for a comprehensive understanding of the intricate interactions between the biostimulant, the fumigant, and the soil bacterial community. HTS may provide valuable insights into this potential strategy for a more sustainable management of common scab disease.
Overall, our findings indicate that in fumigated soils, with or without the inoculation of a biostimulant 10 days after the fumigation, the abundance of soil bacteria was slightly but significantly lowered from P3 to P5 periods. Although the mechanism of soil microbial resilience to fumigation is not entirely clear, one proposed explanation (63) is that the relatively short half-life of the effective compound methyl isothiocyanate, which is decomposed from chloropicrin in soil within about 7 days, could play a role. Overall, all four alpha-diversity indices showed higher values at P5 period, and the treatments showed only slight effect on the alpha-diversity estimated with Chao1, Observed and Evenness indices. Nonetheless, the fumigation and biostimulant treatments showed more significant effects on bacterial community composition.
Treatments effects on soil bacterial community composition
Our study, based on molecular biology detection methods (qPCR, HTS), found that the short-term effects of fumigation were not yet noticeable on bacterial communities in soil sampled 6 days after the application of fumigation (P2). However, from 61 DAF (P3) to 111 DAF (P5), bacterial community composition of non-fumigated soils (T1 and T2) were significantly different from those of fumigated soils (T3 and T4), indicating that the fumigation had the most marked and persistent impact on bacterial diversity composition. This finding indicates that the bacterial diversity composition in the T3-treated soil could not recover or evolve during the growing season in such a way to be less dissimilar than the bacterial diversity composition observed in control plots T1, as reported by previous studies conducted under controlled environment (17, 22, 25).
Our results revealed that each treatment T3 (fumigation alone), T2 (biostimulant alone) and T4 (combination fumigation and biostimulant) induced a profile of specific effects linked to changes in the relative proportions of the 12 most abundant bacterial phyla in these plots. The T3 fumigation treatment alone reduced the proportions of 7 phyla (Crenarchaeota, Planctomycetota, Myxococcota (P3-P4), Methylomirabilota, Verrucomicrobiota, Firmicutes and Acidobacteriota). The first 6 phyla have relative proportions ≤ 2% in soil samples. The T3 treatment also increased the relative proportions of 3 phyla (Myxococcota (P5), Gemmatimonadota and Proteobacteria), the last 2 phyla have relative proportions ≥ 8%. T2 and T4 biostimulant treatments induced distinct profiles of specific effects depending on whether the biostimulant was inoculated into non-fumigated (T2) or fumigated (T4) soil.
Thus, the T2 treatment caused a significant reduction in the relative proportion of the phyla Verrucomicrobiota (P4), Methylomirabilota (P5) and Acidobacteriota (P5) and a significant increase in the relative proportion of the phyla Firmicutes and Bacteroidota (P4 and P5) and Verrucomicrobiota and Proteobacteria (P5). Meanwhile, T4 treatment showed a distinctive profile of specific effects by causing an increase in the relative proportion of Crenarchaeota and Planctomycetota (P3), Myxococcota (P3 and P4) and Proteobacteria (P5) phyla and a significant reduction in the relative proportion of Crenarchaeota and Myxococcota phyla at P5 period. It should be noted that the inoculation of the biostimulant in the fumigated soils (T4) makes it possible to diminish the effect of reduction of the relative proportion of the phyla Crenarchaeota, Planctomycetota, Myxococcota caused by the T3 fumigation treatment.
DESeq2 analysis demonstrated that T3, T2 and T4 treatments significantly affect the ASVs of a limited number of phyla, mainly Proteobacteria and Actinobacteriota, but the ASVs are associated with a broader spectrum of taxonomic families. The DESeq2 analysis revealed significant clustering of treatment’s ASVs composition affected by periods P3, P4 and P5. This suggests that treatment T4 had induced significant changes in the bacterial community composition of the fumigated soils at P5 period to no longer be clustered with T3 ASVs composition as it was the case at periods P3 and P4. Our findings highlight the significant influence of both the treatments (biostimulant and fumigation) and the time periods on specific ASVs that were positively or negatively affected in the treated soils. Our results are consistent with those reported by Li et al. (64), who found that chloropicrin fumigation exposure enriched the abundance of Pseudarthrobacter, Sphingomonas, Pseudomonas, Domibacillus, Nocardioides, and Paenibacillus at the genus level, many of which have known beneficial functions in promoting crop growth, antagonizing plant pathogens, and interacting with soil nitrogen cycling.
Previous studies have found that in bacterial community, Proteobacteria, Actinobacteriota and Firmicutes were more abundant while Acidobacteriota were less abundant in soils exposed to chloropicrin (17, 25, 65). Also, our findings are consistent with previous studies that reported that chloropicrin fumigation altered bacterial phyla relative abundance and sustained changes of bacterial community compared to the effects of other non-fumigated treatments (22, 64).
The application of a Bacillus species based-biostimulant on seed potato in furrow at plantation in either non-fumigated or fumigated soils has shown a positive impact on the bacterial community. This suggests that the application of Bacillus species-based biostimulant, in combination with other soil treatments such as fumigants or fungicides, may significantly promote diverse soil microbial communities, which in turn can sustain soil health and plant growth. It should be noted that the specific effects of biostimulants may vary depending on the type of treatment and the time point during the growing season at which measurements are taken. In a recent study (66), it was suggested that the use of biostimulants, such as the Bacillus-species inoculant based on eight strains used in our study, could promote the growth and diversity of beneficial microorganisms in the soil, potentially leading to improved soil health and plant growth. Our findings support this notion, since the application of biostimulant in non-fumigated soils (T2 treatment) promotes a persistent high proportion of Firmicutes in T2 plots.
Similarity-based artificial network inference within bacterial communities
Our study on the diversity of bacterial communities in soils treated with fumigant and bacteria-based biostimulant against potato’s common scab goes beyond the traditional focus on the number and abundance of species, and instead focuses on interactions among species. This is very important because previous research has shown that species interactions can be more important to ecosystem functioning than species richness and abundance, especially in complex ecosystems (67, 68). Previous research demonstrated the application of network analysis to describe interactions between microbial community features (51, 69, 70). This study builds upon previous research by using indirected hypergraphs to predict ecological relationships between soil microorganisms in bacterial communities treated with fumigant and bacteria-based biostimulant against potato’s common scab. The results indicated that the application of a mix of five Bacillus species-based biostimulants in non-fumigated soils had the highest number of edges and linkages, suggesting a more complex and interconnected microbial community. On the other hand, the chloropicrin fumigated soils had the lowest number of edges and linkages, indicating a less diverse microbial community. The results demonstrate an increase in the ratio of positive associations, as well as in the network diameter and density of the associations, with the biostimulant. This shift in bacterial interrelationships would indicates a transition from competition to cooperation for utilizing available nutrients (71, 72). Furthermore, the fumigation alone treatment leads to a reduction in interactions, while the application of the biostimulant, in both non-fumigated and fumigated soils, results in increased interactions and a higher number of connections between different phyla or within a phylum which revealed that fumigation and other types of fungicides exposure altered the network structure in microbial communities (72–75). The treatment combining the fumigant and the biostimulant exhibits a moderate increase in various network properties, providing evidence for the positive effect of inoculation on bacterial communities in fumigated soils. Our study suggests that the implementation of integrated pest management practices can offer a sustainable approach against common scab, thus reducing reliance on chemical pesticides and promoting environmentally friendly alternatives. By emphasizing the importance of biostimulants as effective and eco-friendly tools for disease management, this study contributes to the development of more sustainable agricultural practices.
Network clusters can represent a group of bacteria with similar or related functions (52, 76). Furthermore, we found that the clustering agreements in the bacterial networks differed between fumigated and non-fumigated soils, respectively, implying potential functional changes, as described in previous studies with fungicides tebuconazole and boscalid (73, 77). Overall, our findings provide insight into how different soil treatments can impact soil microbial communities and their interactions. These ecological networks between bacterial species are highly structured, presenting numerous interactions among network components. Our study also showed that in the bacterial community, any two species can be linked by just a few other neighbor species, indicating small bacterial network property (70). This suggests that the information, materials, and energy can be easily transported throughout the entire network system (32). Our study provides new insight into the architecture of mutualistic, competitive, and trophic networks and how these networks respond to environmental changes (78) due to chemical fumigant/biostimulant treatment. This study adds to the existing literature on the effects of chemical fumigation and bacterial-based biostimulant on soil bacterial community (79). Additionally, this study further demonstrates the importance of deciphering network interactions in bacterial community by building indirected hypergraphs of complex systems in microbial ecology and by increasing our knowledge on the behavior of the components in soil bacterial community exposed to fumigant and bacteria-based biostimulant to control potato common scab. Our study contributes to the existing knowledge in the field of common scab management using chloropicrin fumigation with or without bacterial species biostimulant in potato production. Specifically, we highlight the novel insights gained from HTS and the artificial network inference analysis, which allowed us to elucidate the complex interactions between soil bacterial communities, biostimulant and fumigant in the context of common scab incidence.
Conclusion
The findings of this study are consistent with previous research that has shown that chemical fumigation can have negative impacts on soil microbial communities, while the use of biostimulant can have positive effects. This study also highlights the complex and dynamic nature of soil bacterial phyla and specific taxons in the community and their interactions, which can have important implications for plant and soil health and crop productivity. Our findings highlight the intricate modular structure and network dynamics within the soil bacterial community. The application of biostimulant and fumigation had significant effects on the network properties, with biostimulant treatment promoting increased interactions and connections. These results contribute to our understanding of the ecological relationships in the soil bacterial community and the potential benefits of biostimulant application for enhancing microbial interactions in fumigated soils. Our study suggests that implementing integrated disease management practices can be beneficial to improve bacterial composition in fumigated soil, leading to improve level of positive interactions among bacterial community that may sustain effective common scab control and improved potato crop performance. We have highlighted the significance of using biostimulants as environmentally friendly alternatives to conventional chemical treatments, thus contributing to sustainable agricultural practices. Further investigation is warranted, such as exploring the long-term effects of biostimulant application on soil microbial communities and potato crop health and investigating the specific mechanisms by which Bacterial-based biostimulants improve soil microbial diversity and functions. By pointing out these future research opportunities, we hope to encourage further exploration and advancement in the field.
Data availability statement
The data presented in the study are deposited in the National Library of Medicine, NIH, SRA repository, accession number PRJNA1010157 (https://www.ncbi.nlm.nih.gov/sra/PRJNA1010157).
Author contributions
RH contributed to the conception and design of the study, to get the funding, and to analyse the results. TJ and JD’A-P contributed to the sampling and HTS analysis, to compile and organize the database. HT performed the bioinformatic and statistical analysis and wrote the draft of the manuscript. All authors contributed to the article and approved the submitted version.
Funding
The authors declare that this study received funding from the Ministry of Agriculture, Fisheries and Food, as part of the Program to support the development of agriculture and agri-food in the region (project # PADAAR-1819 -4051-011CN) and received in-kind support from Patates Dolbec inc.. The funders and Patates Dolbec’s suppliers were not involved in the study design, collection, analysis, interpretation of data, the writing of this article or the decision to submit it for publication.
Acknowledgments
Authors are grateful to Dr. Elmer Iquira for his insightfull comments on the draft of the manuscript and to Philippe Parent and Juliette Lévesque for their agronomic expertise and to Vanessa Villeneuve and Véronique Gagné for their technical supports
Conflict of interest
The authors declare that the research was conducted in the absence of any commercial or financial relationships that could be construed as a potential conflict of interest.
Publisher’s note
All claims expressed in this article are solely those of the authors and do not necessarily represent those of their affiliated organizations, or those of the publisher, the editors and the reviewers. Any product that may be evaluated in this article, or claim that may be made by its manufacturer, is not guaranteed or endorsed by the publisher.
Supplementary material
The Supplementary Material for this article can be found online at: https://www.frontiersin.org/articles/10.3389/fsoil.2023.1208909/full#supplementary-material
References
1. Hills K, Collins H, Yorgey G, McGuire A, Kruger C. Improving soil health in pacific northwest potato production: a review. Am J Potato Res (2020) 97(1):1–22. doi: 10.1007/s12230-019-09742-7
2. Papp O, Kocsis T, Biró B, Jung T, Ganszky D, Abod É. ‘Co-Inoculation of Organic Potato with Fungi and Bacteria at High Disease Severity of Rhizoctonia solani and Streptomyces species Increases Beneficial Effects’. Microorganisms (2021) 9(10):2028. doi: 10.3390/microorganisms9102028
3. Wanner LA. A survey of genetic variation in streptomyces isolates causing potato common scab in the United States. Phytopathology (2006) 96(12):1363–71. doi: 10.1094/PHYTO-96-1363
4. Wanner LA. A patchwork of streptomyces species isolated from potato common scab lesions in north america. Am J Potato Res (2009) 86(4):247–64. doi: 10.1007/s12230-009-9078-y
5. Leiminger J, Frank M, Wenk C, Poschenrieder G, Kellermann A, Schwarzfischer A. Distribution and characterization of Streptomyces species causing potato common scab in Germany. Plant Pathol (2013) 62(3):611–23. doi: 10.1111/j.1365-3059.2012.02659.x
6. Lerat S, Simao-Beaunoir A-M, Beaulieu C. Genetic and physiological determinants of Streptomyces scabies pathogenicity. Mol Plant Pathol (2009) 10(5):579–85. doi: 10.1111/j.1364-3703.2009.00561.x
7. Healy FG, Wach M, Krasnoff SB, Gibson DM, Loria R. The txtAB genes of the plant pathogen Streptomyces acidiscabies encode a peptide synthetase required for phytotoxin thaxtomin A production and pathogenicity. Mol Microbiol (2000) 38(4):794–804. doi: 10.1046/j.1365-2958.2000.02170.x
8. Kers JA, Cameron KD, Joshi MV, Bukhalid RA, Morello JE, Wach MJ, et al. A large, mobile pathogenicity island confers plant pathogenicity on Streptomyces species. Mol Microbiol (2005) 55(4):1025–33. doi: 10.1111/j.1365-2958.2004.04461.x
9. Sarwar A, Latif Z, Zhang S, Zhu J, Zechel DL, Bechthold A. Biological control of potato common scab with rare isatropolone C compound produced by plant growth promoting streptomyces A1RT. Front Microbiol (2018) 9. doi: 10.3389/fmicb.2018.01126
10. Cullen DW, Lees AK. Detection of the nec1 virulence gene and its correlation with pathogenicity in Streptomyces species on potato tubers and in soil using conventional and real-time PCR. J Appl Microbiol (2007) 102(4):1082–94. doi: 10.1111/j.1365-2672.2006.03146.x
11. Bukhalid RA, Loria R. Cloning and expression of a gene from Streptomyces scabies encoding a putative pathogenicity factor. J Bacteriol (1997) 179(24):7776–83. doi: 10.1128/jb.179.24.7776-7783.1997
12. Wanner LA, Haynes KG. Aggressiveness of streptomyces on four potato cultivars and implications for common scab resistance breeding. Am J Potato Res (2009) 86(5):335–46. doi: 10.1007/s12230-009-9088-9
13. Wanner LA, Kirk WW. Streptomyces – from basic microbiology to role as a plant pathogen. Am J Potato Res (2015) 92(2):236–42. doi: 10.1007/s12230-015-9449-5
14. Beaulieu C, Goyer C, Beaudoin N. Interaction between pathogenic streptomycetes and plants: the role of thaxtomins. In Plant-Microbe Interactions. Barka EA, Clément C eds. (2008) Trivandrum, India: Research Signpost, 117–33.
15. Braun S, Gevens A, Charkowski A, Allen C, Jansky S. Potato common scab: a review of the causal pathogens, management practices, varietal resistance screening methods, and host resistance. Am J Potato Res (2017) 94(4):283–96. doi: 10.1007/s12230-017-9575-3
16. Huang B, Li J, Wang Q, Guo M, Yan D, Fang W, et al. Effect of soil fumigants on degradation of abamectin and their combination synergistic effect to root-knot nematode. PloS One (2018) 13(6):e0188245. doi: 10.1371/journal.pone.0188245
17. Fang W, Yan D, Wang X, Huang B, Wang X, Liu J, et al. Responses of nitrogen-cycling microorganisms to dazomet fumigation. Front Microbiol (2018) 9:2529. doi: 10.3389/fmicb.2018.02529
18. Shennan C. Biotic interactions, ecological knowledge and agriculture. Philos Trans R Soc Lond B Biol Sci (2008) 363:717–39. doi: 10.1098/rstb.2007.2180
19. Fierer N. Embracing the unknown: disentangling the complexities of the soil microbiome. Nat Rev Microbiol (2017) 15(10):579–90. doi: 10.1038/nrmicro.2017.87
20. van Elsas JD, Chiurazzi M, Mallon CA, Elhottovā D, Krištůfek V, Salles JF. Microbial diversity determines the invasion of soil by a bacterial pathogen. Proc Natl Acad Sci (2012) 109(4):1159–64. doi: 10.1073/pnas.1109326109
21. Huang B, Yan D, Ouyang C, Zhang D, Zhu J, Liu J, et al. Chloropicrin fumigation alters the soil phosphorus and the composition of the encoding alkaline phosphatase PhoD gene microbial community. Sci Total Environ (2020) 711:135080. doi: 10.1016/j.scitotenv.2019.135080
22. Sennett LB, Goyer C, Burton DL, Zebarth BJ, Whitney S. Chemical fumigation and biofumigation alter soil bacterial community diversity and composition. FEMS Microbiol Ecol (2022) 98(4):fiac026. doi: 10.1093/femsec/fiac026
23. De Cal A, Treceño AM, Salto T, Aranda JML, Melgarejo P. Effect of chemical fumigation on soil fungal communities in Spanish strawberry nurseries. Appl Soil Ecol (2005) 28(1):47–56. doi: 10.1016/j.apsoil.2004.06.005
24. Collins HP, Alva A, Boydston RA, Cochran RL, Hamm PB, McGuire A, et al. Soil microbial, fungal, and nematode responses to soil fumigation and cover crops under potato production. Biol Fertility Soils (2006) 42(3):247–57. doi: 10.1007/s00374-005-0022-0
25. Li J, Huang B, Wan Q, Li Y, Fang W, Han D, et al. Effects of fumigation with metam-sodium on soil microbial biomass, respiration, nitrogen transformation, bacterial community diversity and genes encoding key enzymes involved in nitrogen cycling. Sci Total Environ (2017) 598:1027–36. doi: 10.1016/j.scitotenv.2017.02.058
26. Gullino ML, Minuto A, Gilardi G, Garibaldi A, Ajwa H, Duafala T, et al. Efficacy of preplant soil fumigation with chloropicrin for tomato production in Italy. Crop Prot (2002) 21(9):741–9. doi: 10.1016/S0261-2194(02)00031-5
27. Chen D, Zebarth BJ, Goyer C, Comeau LP, Nahar K, Dixon T. Effect of Biofumigation on Population Densities of Pratylenchus species and Verticillium species and Potato Yield in Eastern Canada. Am J Potato Res (2022) 99(3):229–42. doi: 10.1007/s12230-022-09875-2
28. Wang Q, Ma Y, Yang H, Chang Z. Effect of biofumigation and chemical fumigation on soil microbial community structure and control of pepper Phytophthora blight. World J Microbiol Biotechnol (2014) 30(2):507–18. doi: 10.1007/s11274-013-1462-6
29. Wei F, Passey T, Xu X. Amplicon-based metabarcoding reveals temporal response of soil microbial community to fumigation-derived products. Appl Soil Ecol (2016) 103:83–92. doi: 10.1016/j.apsoil.2016.03.009
30. Dziugieł T, Wadas W. Effect of plant biostimulants on macronutrient content in early crop potato tubers. Agronomy (2020) 10(8):1202. doi: 10.3390/agronomy10081202
31. Agbrima. ALPINE Bio20™. (2023). Available at: https://www.agbrima.com/_files/ugd/03495e_3e461adb1759427381ae38f04da57853.pdf.
32. Deng Y, Jiang YH, Yang Y, He Z, Luo F, Zhou J. Molecular ecological network analyses. BMC Bioinf (2012) 13:113. doi: 10.1186/1471-2105-13-113
33. Rashidi HH, Tran NK, Betts EV, Howell LP, Green R. Artificial intelligence and machine learning in pathology: the present landscape of supervised methods. Acad Pathol (2019) 6:2374289519873088. doi: 10.1177/2374289519873088
34. Namkung J. Machine learning methods for microbiome studies. J Microbiol (2020) 58(3):206–16. doi: 10.1007/s12275-020-0066-8
35. Driscoll J, Coombs J, Hammerschmidt R, Kirk W, Wanner L, Douches D. Greenhouse and field nursery evaluation for potato common scab tolerance in a tetraploid population. Am J potato Res (2009) 86(2):96–101. doi: 10.1007/s12230-008-9065-8
36. Overbeek W, Jeanne T, Hogue R, Smith DL. Effects of microbial consortia, applied as fertilizer coating, on soil and rhizosphere microbial communities and potato yield. Front Agron (2021) 3. doi: 10.3389/fagro.2021.714700
37. Jeanne T, Parent S.-É., Hogue R. Using a soil bacterial species balance index to estimate potato crop productivity. PloS One (2019) 14(3):e0214089. doi: 10.1371/journal.pone.0214089
38. Fierer N, Jackson JA, Vilgalys R, Jackson RB. Assessment of soil microbial community structure by use of taxon-specific quantitative PCR assays. Appl Environ Microbiol (2005) 71(7):4117–20. doi: 10.1128/AEM.71.7.4117-4120.2005
39. Jeanne T, D’Astous-Pagé J, Hogue R. Spatial, temporal and technical variability in the diversity of prokaryotes and fungi in agricultural soils. Front Soil Sci (2022) 2:945888 (Accessed: 11 November 2022. doi: 10.3389/fsoil.2022.945888
40. Apprill A, McNally S, Parsons R, Weber L. Minor revision to V4 region SSU rRNA 806R gene primer greatly increases detection of SAR11 bacterioplankton. Aquat Microbial Ecol (2015) 75(2):129–37. doi: 10.3354/ame01753
41. Parada AE, Needham DM, Fuhrman JA. Every base matters: assessing small subunit rRNA primers for marine microbiomes with mock communities, time series and global field samples. Environ Microbiol (2016) 18(5):1403–14. doi: 10.1111/1462-2920.13023
42. Bolyen E, Rideout JR, Dillon MR, Bokulich NA, Abnet CC, Al-Ghalith GA, et al. Reproducible, interactive, scalable and extensible microbiome data science using QIIME 2. Nat Biotechnol (2019) 37(8):852–7. doi: 10.1038/s41587-019-0209-9
43. Callahan BJ, McMurdie PJ, Rosen MJ, Johnson AJA, Han AW, Holmes SP. DADA2: High-resolution sample inference from Illumina amplicon data. Nat Methods (2016) 13(7):581–3. doi: 10.1038/nmeth.3869
44. Quast C, Pruesse E, Yilmaz P, Gerken J, Schweer T, Yarza P, et al. The SILVA ribosomal RNA gene database project: improved data processing and web-based tools. Nucleic Acids Res (2013) 41(Database issue):D590–596. doi: 10.1093/nar/gks1219
45. Bokulich NA, Kaehler BD, Rideout JR, Dillon M, Bolyen E, Knight R, et al. Optimizing taxonomic classification of marker-gene amplicon sequences with QIIME 2’s q2-feature-classifier plugin. Microbiome (2018) 6(1):90. doi: 10.1186/s40168-018-0470-z
46. Robeson MS, O’Rourke DR, Kaehler BD, Ziemski M, Dillon MR, Foster JT, et al. RESCRIPt: Reproducible sequence taxonomy reference database management. PloS Comput Biol (2021) 17(11):e1009581. doi: 10.1371/journal.pcbi.1009581
47. McMurdie PJ, Holmes S. phyloseq: an R package for reproducible interactive analysis and graphics of microbiome census data. PloS One (2013) 8(4):e61217. doi: 10.1371/journal.pone.0061217
48. Liu C, Cui Y, Li X, Yao M. microeco: an R package for data mining in microbial community ecology. FEMS Microbiol Ecol (2021) 97(2):fiaa255. doi: 10.1093/femsec/fiaa255
49. Anderson MJ. A new method for non-parametric multivariate analysis of variance. Austral Ecol (2001) 26(1):32–46. doi: 10.1111/j.1442-9993.2001.01070.pp.x
50. Love MI, Huber W, Anders S. Moderated estimation of fold change and dispersion for RNA-seq data with DESeq2. Genome Biol (2014) 15(12):550. doi: 10.1186/s13059-014-0550-8
51. Barberán A, Bates ST, Casamayor EO, Fierer N. Using network analysis to explore co-occurrence patterns in soil microbial communities. ISME J (2012) 6(2):343–51. doi: 10.1038/ismej.2011.119
52. Newman MEJ. Modularity and community structure in networks. Proc Natl Acad Sci United States America (2006) 103(23):8577–82. doi: 10.1073/pnas.0601602103
53. Guimerà R, Nunes Amaral LA. Functional cartography of complex metabolic networks. Nature (2005) 433(7028):895–900. doi: 10.1038/nature03288
54. Krackhardt D. Graph theoretical dimensions of informal organization. Comput Organ Theory (1994) 89:89–111.
55. Guimerà R, Sales-Pardo M, Amaral LAN. Classes of complex networks defined by role-to-role connectivity profiles. Nat Phys (2007) 3(1):63–9. doi: 10.1038/nphys489
56. Ravasz E, Somera AL, Mongru DA, Oltvai ZN, Barabási AL. Hierarchical organization of modularity in metabolic networks. Science (2002) 297(5586):1551–5. doi: 10.1126/science.1073374
57. Wasserman S, Faust K. Social network analysis: Methods and applications. New York, NY, US: Cambridge University Press (Social network analysis: Methods and applications (1994). p. 825. doi: 10.1017/CBO9780511815478
58. Brandes U, Erlebach T. Network analysis. Berlin, Heidelberg: Springer (Lecture Notes in Computer Science (2005). doi: 10.1007/b106453
59. Csárdi G, Nepusz T. The igraph software package for complex network research (2006). Available at: https://www.semanticscholar.org/paper/The-igraph-software-package-for-complex-network-Cs%C3%A1rdi-Nepusz/1d2744b83519657f5f2610698a8ddd177ced4f5c.
60. Bastian M, Heymann S, Jacomy M. Gephi: an open source software for exploring and manipulating networks. Proc Int AAAI Conf Web Soc Media (2009) 3(1):361–2. doi: 10.1609/icwsm.v3i1.13937
61. Jacomy M, Venturini T, Heymann S, Bastian M. ForceAtlas2, a continuous graph layout algorithm for handy network visualization designed for the gephi software. PloS One (2014) 9(6):e98679. doi: 10.1371/journal.pone.0098679
62. Souza R, de Ambrosini A, Passaglia LMP. Plant growth-promoting bacteria as inoculants in agricultural soils’. Genet Mol Biol (2015) 38(4):401–19. doi: 10.1590/S1415-475738420150053
63. Ajwa HA, Trout T, Mueller J, Wilhelm S, Nelson SD, Soppe R, et al. Application of alternative fumigants through drip irrigation systems. Phytopathology® (2002) 92(12):1349–55. doi: 10.1094/PHYTO.2002.92.12.1349
64. Li X, Skillman V, Dung J, Dung J. Legacy effects of fumigation on soil bacterial and fungal communities and their response to metam sodium application. Environ Microbiome (2022) 17(1):59. doi: 10.1186/s40793-022-00454-w
65. Toyota K, Ritz K, Kuninaga S, Kimura M. Impact of fumigation with metam sodium upon soil microbial community structure in two Japanese soils. Soil Sci Plant Nutr (1999) 45(1):207–23. doi: 10.1080/00380768.1999.10409336
66. Zhan Y, Yan N, Miao X, Li Q, Chen C. Different responses of soil environmental factors, soil bacterial community, and root performance to reductive soil disinfestation and soil fumigant chloropicrin. Front Microbiol (2021) 12:796191. doi: 10.3389/fmicb.2021.796191
67. Montoya JM, Pimm SL, Solé RV. Ecological networks and their fragility. Nature (2006) 442(7100):259–64. doi: 10.1038/nature04927
68. Bascompte J. Networks in ecology. Basic Appl Ecol (2007) 8:485–90. doi: 10.1016/j.baae.2007.06.003
69. Taketani RG, Moitinho MA, Mauchline TH, Melo IS. Co-occurrence patterns of litter decomposing communities in mangroves indicate a robust community resistant to disturbances. PeerJ (2018) 6:e5710. doi: 10.7717/peerj.5710
70. Wu X, Yang J, Ruan H, Wang S, Yang Y, Naeem I, et al. The diversity and co-occurrence network of soil bacterial and fungal communities and their implications for a new indicator of grassland degradation. Ecol Indic (2021) 129:107989. doi: 10.1016/j.ecolind.2021.107989
71. Banerjee S, Kirkby CA, Schmutter D, Bissett A, Kirkegaard JA, Richardson AE, et al. Network analysis reveals functional redundancy and keystone taxa amongst bacterial and fungal communities during organic matter decomposition in an arable soil. Soil Biol Biochem (2016) 97:188–98. doi: 10.1016/j.soilbio.2016.03.017
72. Ge A-H, Liang ZH, Xiao J-L, Zhang Y, Zeng Q, Xiong C, et al. Microbial assembly and association network in watermelon rhizosphere after soil fumigation for Fusarium wilt control. Agriculture Ecosyst Environ (2021) 312:107336. doi: 10.1016/j.agee.2021.107336
73. Han L, Xu M, Kong X, Liu X, Wang Q, Chen G, et al. Deciphering the diversity, composition, function, and network complexity of the soil microbial community after repeated exposure to a fungicide boscalid. Environ pollut (2022) 312:120060. doi: 10.1016/j.envpol.2022.120060
74. Zhang H, Song J, Zhang Z, Zhang Q, Chen S, Mei J, et al. Exposure to fungicide difenoconazole reduces the soil bacterial community diversity and the co-occurrence network complexity. J Haz Mat (2021) 405:124208. doi: 10.1016/j.jhazmat.2020.124208
75. Zhang X, Xue C, Fang D, He X, Wei M, Zhuo C, et al. Manipulating the soil microbiomes during a community recovery process with plant beneficial species for the suppression of Fusarium wilt of watermelon. AMB Express (2021) 11(1):87. doi: 10.1186/s13568-021-01225-5
76. Jiao S, Lu Y, Wei G. Soil multitrophic network complexity enhances the link between biodiversity and multifunctionality in agricultural systems. Global Change Biol (2022) 28(1):140–53. doi: 10.1111/gcb.15917
77. Han L, Kong X, Xu M, Nie J. Repeated exposure to fungicide tebuconazole alters the degradation characteristics, soil microbial community and functional profiles. Environ pollut (2021) 287:117660. doi: 10.1016/j.envpol.2021.117660
78. Thébault E, Fontaine C. Stability of ecological communities and the architecture of mutualistic and trophic networks. Science (2010) 329(5993):853–6. doi: 10.1126/science.1188321
Keywords: chloropicrin, Bacillus species-based biostimulant, common scab, bacterial community, 16S amplicon-targeted high throughput sequencing, machine-learning analysis, and network Interactions
Citation: Tekeu H, Jeanne T, D’Astous-Pagé J and Hogue R (2023) Artificial network inference analysis reveals the impact of biostimulant on bacterial communities in fumigated soil for potato production against common scab. Front. Soil Sci. 3:1208909. doi: 10.3389/fsoil.2023.1208909
Received: 19 April 2023; Accepted: 14 August 2023;
Published: 12 September 2023.
Edited by:
Rodrigo Gouvea Taketani, Rothamsted Research, United KingdomReviewed by:
Amita Kaundal, Utah State University, United StatesVishal Tripathi, Graphic Era University, India
Copyright © 2023 Tekeu, Jeanne, D’Astous-Pagé and Hogue. This is an open-access article distributed under the terms of the Creative Commons Attribution License (CC BY). The use, distribution or reproduction in other forums is permitted, provided the original author(s) and the copyright owner(s) are credited and that the original publication in this journal is cited, in accordance with accepted academic practice. No use, distribution or reproduction is permitted which does not comply with these terms.
*Correspondence: Richard Hogue, richard.hogue@irda.qc.ca