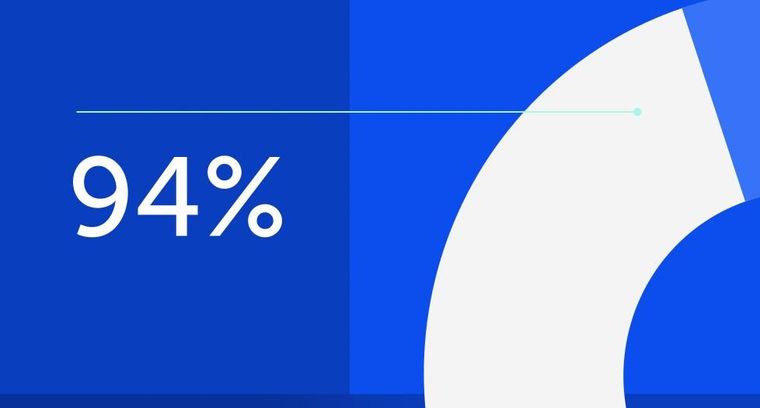
94% of researchers rate our articles as excellent or good
Learn more about the work of our research integrity team to safeguard the quality of each article we publish.
Find out more
REVIEW article
Front. Soil Sci., 29 August 2022
Sec. Soil Biogeochemistry & Nutrient Cycling
Volume 2 - 2022 | https://doi.org/10.3389/fsoil.2022.927452
This article is part of the Research TopicGreenhouse gas measurements in underrepresented areas of the worldView all 8 articles
Livestock are an important source of livelihoods in agricultural systems in sub-Saharan Africa (SSA), while also being the largest source of national greenhouse gas (GHG) emissions in most African countries. As a consequence, there is a critical need for data on livestock GHG sources and sinks to develop national inventories, as well as conduct baseline measurements and intervention testing to mitigate GHG emissions and meet ambitious national climate goals. Our objective was to review studies on GHG emissions from livestock systems in SSA, as well as soil carbon storage in livestock-dominated systems (i.e., grasslands and rangelands), to evaluate best current data and suggest future research priorities. To this end, we compiled studies from SSA that determined emission factors (EFs) for enteric methane and manure emissions, along with studies on soil organic carbon (SOC) stocks in SSA. We found that there has been limited research on livestock GHG emissions and SOC relative to national ambitions for climate change mitigation in SSA. Enteric methane emission factors (EFs) in low productivity cattle systems may be lower than IPCC Tier 1 default EFs, whereas small ruminants (i.e. sheep and goats) had higher EFs compared to IPCC Tier 1 EFs. Manure EFs were equal to or lower than IPCC Tier 1 EFs for deposited manure (while grazing), manure applied as fertilizer, and manure management. SOC stocks for grasslands and rangelands in SSA show broad agreement with IPCC estimates, but there was a strong geographic bias and many studies did not report soil type, bulk density, or SOC stocks at >30 cm depth. In general, the largest data gaps included information for manure (quantity, quality, management), small ruminants, agropastoral/pastoralist systems, and in general from West Africa. Future research should focus on filling major data gaps on locally appropriate mitigation interventions and improving livestock activity data for developing Tier 2 GHG inventories in SSA. At the science-policy interface, all parties would benefit from enhanced coordination within the research community and between researchers and African governments to improve Tier 2 inventories and harmonize measurement for mitigation in livestock systems in SSA.
Livestock are a major source of agricultural livelihoods and provide multiple important services within smallholder and pastoral systems in sub-Saharan Africa (SSA). Livestock in SSA are multi-purpose, since animals are kept for food and nutrition security, as a form of insurance and “savings account”, and for cultural reasons (1; 2). In mixed crop-livestock systems, livestock provide traction for ploughing and manure as fertilizer (3, 4). Mixed crop-livestock systems, which integrate livestock keeping with crop production, are predominantly rain-fed and widespread throughout humid and tropical highland regions of SSA, whereas agropastoral and grazing-based pastoral systems predominate in arid and semi-arid regions (5).
Livestock systems and livelihoods in SSA are under threat from multiple stresses, including climate change, land fragmentation and degradation, invasive species, and competition for feed (6–8). With respect to climate change, livestock are affected directly by increased temperatures due to negative physiological effects of heat stress on animal metabolism and productivity (9, 10), and indirectly via increased disease burden, reduced water availability, and lower quantity and quality of feed (11–13). At the same time, the agriculture sector as whole contributes to climate change by producing a large proportion (~34% on CO2-eq basis as of 2018) of national anthropogenic greenhouse gas (GHG) emissions for countries in SSA. The vast majority of agricultural GHGs are from livestock – methane (CH4) emissions from enteric fermentation and manure management account for ~21% of GHG emissions, while nitrous oxide (N2O) from applied, deposited, and managed manure account for ~11% of emissions across all sectors in SSA (http://edgar.jrc.ec.europa.eu/overview.php?v=432_AP, last access: April 2022). Carbon storage in grasslands and rangelands, particularly as soil organic carbon (SOC) stocks, can also be affected by livestock and grazing management. SOC sequestration is proposed as a practice for offsetting livestock emissions in grassland and rangeland ecosystems in SSA, but at present SOC storage potential for these ecosystems has not been well-quantified (14, 15).
Given the importance of livestock emissions in SSA, the livestock sector has been specifically incorporated into climate change policies at the national and international level in many African countries through participation in the United Nations Framework Convention on Climate Change and the Paris Climate Agreement. Most countries conditionally mention livestock in their Nationally Determined Contributions (NDCs) and/or Adaptation Plans, or specific large-scale low-emissions development projects (16). To date, 30 countries in SSA (61%) have made some mention of livestock in their conditional commitments on GHG mitigation in either their 2016 or 2021 NDCs, while 21 countries (43%) prioritized livestock for adaptation in their updated NDCs in 2021 (see Supporting Table 1; 17; 18). To meet their NDCs, African nations need reliable GHG inventories and GHG monitoring approaches that can track the efficacy of mitigation efforts.
However, most national GHG emissions estimates in SSA use the IPCC Tier 1 methodology to estimate national GHG inventories for livestock systems. The Tier 1 method uses fixed, default emission factors (EFs) (19) to compute emissions per head of livestock. Tier 1 EFs for SSA are based on modeling studies, and more recent in situ research has shown that IPCC Tier 1 EFs may not be appropriate for livestock in SSA (20–25). Moreover, because it relies on fixed EFs, the Tier 1 approach cannot capture trends in emissions over time due to changes in management or other factors (26) and is therefore not suitable for measurement, reporting, and verification (MRV) purposes and tracking progress toward mitigation goals outlined in NDCs. For SOC stocks, IPCC Tier 1 methods use default SOC stocks depending on soil type and climate, while changes in SOC stocks depend on fixed stock change factors (SCFs) that vary depending land cover type and management practices. But, there is a paucity of data on SOC stocks and management impacts in grasslands and rangelands in SSA with which to validate IPCC default SOC stocks and SCFs (27)
For significant GHG emission sources in national GHG inventories, such as livestock in SSA, the IPCC recommends the use of IPCC Tier 2 or higher (Tier 3) methods. These can either be via direct measurements of GHG emissions, estimated EFs based on locally or regionally specific activity data, or modelling that uses locally specific data (Figure 1) (28). Higher Tier inventories may also be useful because they potentially allow for more accurate identification of emissions hotspots, targeting of mitigation interventions, and carbon payment schemes (4, 29). But, development of national inventories from IPCC Tier 1 to higher IPCC Tiers has been hampered by the lack of resources and unavailability of data (30, 31). As a result, only six countries in SSA have thus far developed IPCC Tier 2 inventories (Benin, Ethiopia, Kenya, Namibia, Senegal, South Africa) (Mwape, pers. comm.).
Figure 1 Flow diagram showing activity data and model input requirements for indirect estimation of Tier 2 emission factors for livestock based on IPCC guidelines. Lower part of the diagram shows additional data requirements for “feed intake data” using direct and indirect estimation for estimating feed intake. NE, net energy requirements; GE, gross energy intake.
In addition to improved inventories, there is a need to collect baseline data, as this will allow for critically assessments of the impacts of interventions on GHG emissions and SOC stock changes for livestock systems in SSA. Baselining requires in situ data on livestock GHG emissions and SOC stocks from the diverse range systems present on the continent. This ensures that mitigation measures tracked through Tier 2 inventories are locally appropriate. Baseline GHG assessments and intervention testing are also required for realistic upscaling of emissions from the local to regional scale using process-based biogeochemical and life-cycle assessment models to complement national inventories and assess the efficacy of mitigation interventions (32). Baseline measurements and changes in SOC stocks, along with estimates of biomass carbon and net ecosystem exchange are also required to determine the full GHG budget of African grassland and rangeland ecosystems and whether these systems are net GHG sources or sinks after including emissions from livestock (i.e. enteric CH4 and manure emissions) (33). Given the lack of direct GHG measurements, activity data, and SOC stocks for the breadth of conditions present in livestock systems in SSA, African nations face a challenge to develop appropriate Tier 2 inventories and MRV systems of viable intervention strategies (34).
Emissions of enteric CH4 from ruminant livestock are positively correlated with total feed intake and negatively correlated with feed digestibility. Higher feed intake increases passage of feed through ruminant digestive systems and thus increases CH4 emissions, whereas feed of high digestibility is utilized more efficiently and reduces CH4 emissions intensity (i.e., CH4 emitted per unit of animal product) (19). Manure emissions of N2O are positively related to both feed intake and feed quality. Increased feed intake increases excreta output and higher increased dietary protein and N content increase faecal N content and reduces C:N ratios, both of which tend to increase N2O emissions due increased concentrations of labile N (35). Manure CH4 emissions are driven by excreta output and correlated with manure quality, while also being elevated under anaerobic conditions, so manure management and environmental conditions have a major impact on manure CH4 emissions (IPCC, 2019, Ch. 10). Rainfall affects manure emissions through alternate wetting and drying of the substrate, which can increase N2O emissions since both aerobic (nitrification of to ) and anaerobic conditions (denitrification of or to N2O) are often necessary to convert mineral N to N2O (36). In addition, N2O can also be produced through nitrifier-denitrification under aerobic condtions (37) Methane emissions from manure are dependent on the presence of anaerobic conditions (38).
Impacts of livestock grazing on SOC storage in grasslands and rangelands in SSA may be positive or negative depending on depending on climate, grazing intensity, and other environmental factors (39–41). A number of management practices have been proposed to increase SOC sequestration in grasslands and rangelands in SSA. For example, long-term exclosure of livestock using fencing has been proposed in areas with severe degradation in order to allow for recovery of both native vegetation and SOC stocks (42, 43). Grazing management practices that improve productivity and SOC sequestration include reductions in grazing intensity and paddocking, and more complex management regimes, such as high density – short duration grazing that mimics grazing patterns in natural ecosystems (44).
To facilitate transformation of African livestock systems and to support the development of Tier 2 inventories and MRV strategies, there is an urgent need to identify knowledge gaps and map priorities for future research. To our knowledge, no comprehensive review has been conducted to assess the current state of existing research and data availability on livestock GHG emissions, while also considering challenges and future research priorities for the continent. Here we review the literature for GHG emissions from livestock systems in SSA (specifically studies containing country-specific Tier 2 EF estimates, livestock activity data, and mitigation intervention measures), and compare EFs and activity data from the literature with IPCC Tier 1 2006 and/or 2019 default EFs. We further examine research on SOC stocks and potential SOC sequestration in grassland and rangeland ecosystems in SSA and compare with IPCC default SOC stocks and SCFs. We organize the review by dividing livestock systems into components based on IPCC guidelines: enteric CH4 emissions, manure emissions, and carbon storage in grassland/rangeland ecosystems. Subsequently, we assess the key challenges and future research priorities on livestock GHG emissions in SSA. While the focus of this review is on livestock GHG emissions in SSA, we emphasize that reductions in GHG emissions from the livestock sector must be realized as co-benefits of improved management practices that enhance productivity and food and nutrition security while adapting to a changing climate, maintaining or enhancing biodiversity, and reducing the risk of emerging zoonotic diseases (45–47).
We conducted a systematic literature search through Web of Science to obtain studies measuring livestock GHG emissions in SSA for each category of emissions (enteric, manure, carbon storage in grassland and rangeland ecosystems) (see Supporting Material A.1 for additional details). For all categories, we restricted the search to true ruminants (cattle, sheep, and goats) and did not include pseudo-ruminants (horses, mules, asses, camels) or monogastrics (pigs, poultry). We chose to focus on ruminant livestock due to their high population and ubiquitous presence in livestock systems in SSA, as well their relatively high emissions compared to other species (particularly due to enteric CH4 emissions). We searched Google Scholar to ensure that gray literature (including reports from national GHG inventories) or other publications not found in the Web of Science search were captured. All publications were screened to select only those that had qualitatively or quantitatively estimated emissions from livestock using one of the following methods: for enteric CH4 emissions, we included studies that used direct methods (e.g. automatic chambers, SF6, etc.) or indirect estimation based on feed intake and activity data. We excluded modeling studies, in vitro estimates, and laser methane detector studies for enteric CH4. For manure emissions, we only included publications that contained direct measurements of N2O and/or CH4 emissions from ruminant livestock and met the minimum criteria described by Beltran et al. (48) and van der Weerden etal. (49) (see Supporting Material A.3). Additionally, we included indirect estimates of emissions from manure management from Tier 2 inventories and modeling studies (if the latter were based on in situ field measurements). For carbon storage in grassland and rangeland ecosystems, we focused on studies that measured baseline soil organic carbon (SOC) stocks and/or changes in SOC stocks due to management practices for comparison with the IPCC default SOC stock values and stock change factors, respectively. We also examined studies of C uptake and balance for grassland and rangeland ecosystems using micrometeorological techniques (e.g. eddy covariance) or C balance methods, which do not appear in IPCC guidelines and are therefore reported in the Supporting Material (Supporting Material A.5, Supporting Material B)
Following the literature search and screening of publications, we disaggregated data from the selected publications to create separate databases for enteric CH4, GHG emissions from manure management, and SOC stocks or changes therein for grasslands/rangelands. We also disaggregated activity data associated with emissions based on the IPCC 2019, guidelines, if reported (50).
For enteric CH4, we classified methods as either direct measurement (Figure 1D), such as animal respiration chambers (51), sulfur hexafluoride tracer (SF6) (52), and GreenFeed (53) (we also included open path laser under direct methods) or indirect estimation (i.e., based on feed digestibility and activity data; Figure 1E) (20). We disaggregated data from each study into separate observations for ease of analysis and comparison with IPCC default EFs for enteric CH4 emissions (50), and divided data by species (i.e., cattle, sheep, goats). For publications with more than one species, data for each species were treated as separate observations.
We classified cattle into “high” and “low” productivity systems based on IPCC 2019 Tier 1a guidelines. The guidelines on enteric CH4 emissions divide cattle into “dairy” and “other cattle” categories, and into multiple distinct sub-classes based on age and sex or physiological state. We therefore divided and compared EFs for cattle by productivity level according to IPCC guidelines into the following sub-classes: mature females, mature females – grazing, mature males, draft bullocks, bulls – grazing, growing/replacement, calves on forage. For small ruminants, the IPCC only provides Tier 1 default EFs and does not distinguish by productivity level for Africa. For all species, we classified observations by agro-ecological zone and modified FAO livestock production system. Additional details on methods for classification of observations into “high” and “low” productivity, cattle sub-classes, agro-ecological zone, and livestock production system can be found in Supporting Material A.2.
This created a database of enteric CH4 Tier 2 EFs for which we calculated means ± 95% confidence interval (CI) by productivity level and sub-class for cattle (Supporting Table 2) and at the species level for small ruminants (Supporting Table 3). A subset of studies with Tier 2 EFs collected activity data, and we extracted activity data for each study and/or observation where available. Activity data extracted included live-weight, milk yield, milk fat, milk protein, feed digestibility, and dietary crude protein. For each cattle sub-class and productivity level (“high”, low”) we calculated mean and CI for activity data values. For cattle, we then compared calculated Tier 2 CH4 EFs and activity data values with IPCC Tier 1a defaults from both the 54 and 2019 guidelines. For small ruminants, only Tier 1 default values were available for CH4 EFs and activity data, and these values were not updated between the 2006 and 2019 IPCC guidelines.
We disaggregated data for GHG emissions from manure management based on GHG species (CH4, N2O) and IPCC emissions category, as described in Supporting Material A.1 of this publication. Here, emissions based on IPCC 2019 categories from manure deposited on pasture, range, and paddock are referred to as “deposited manure”, emissions from manure used as organic fertilizer are referred to as “applied manure”, and emissions from manure management. For manure management, we included measurements emissions from manure in overnight livestock enclosures (generally referred to as ‘bomas’ or ‘kraals’ in SSA) and compare with IPCC EFs for ‘drylot’ manure management (54, p. 10.62).
Compared to the IPCC guidelines, we simplified our categorization and use of the term “manure”. The IPCC guidelines categorize N2O emissions from deposited manure and applied manure under soil emissions (55, p. 11.5), whereas N2O and CH4 from manure management, along with CH4 emissions from deposited manure, are reported under livestock emissions (50, p. 10.167) (CH4 emissions from applied manure are not considered in the IPCC guidelines). To avoid confusion, we do not distinguish “manure” as falling within either managed soils or livestock, but instead discuss all manure N2O and CH4 emissions together. Following disaggregation by GHG and simplified manure categories, we further disaggregated the data for N2O emissions from deposited manure by manure component (“dung” or “urine”) and climate (“wet” and “dry”), following (23) and IPCC guidelines.
Additionally, IPCC guidelines specify that full Tier 2 estimations of manure N2O emissions require country-specific data on, inter-alia, N leaching/runoff and NH3/NOx volatilization. Since none of the studies on manure N2O emissions reported here measured N leaching or NH3/NOx volatilization, EFs calculated from these studies should be referred to as country-specific N2O emission estimates. These country-specific N2O emission estimates should be useful for national inventory improvement and provide a useful benchmark for assessing the adequacy of IPCC Tier 1 default EFs for manure in SSA.
The disaggregation process created a database of observations for emissions from deposited manure, applied manure, and manure management in SSA (Supporting Table 4). We calculated mean EFs and CI separately for each manure category. If EFs were not reported, we calculated the EFs manually based on GHG measurements reported in the publication. We then compared mean EF values for each manure category with updated 2019 IPCC Tier 1 default values. For applied manure, we included observations that applied manure and mineral fertilizer simultaneously and included these in mean EF calculations due to minimal differences in emissions between studies with and without mineral fertilizer (Supporting Figure 1). In addition to EFs, we extracted activity data for each study and/or observation, if available. Activity data on deposited manure were extracted for manure N and C or volatile solid (VS) content, soil type, and manure N application rate. Activity data for applied manure were collected for soil type and manure N application rate. Activity data for manure management were collected for management system type and manure N and C or VS content (Figure 1C).
We obtained SOC stocks from all available depth intervals and treatments in each individual study, with each depth interval and treatment constituting a single observation in the database (Supporting Table 5). If SOC was reported as a concentration or percentage, we multiplied by bulk density and depth to obtain the SOC stock (Mg C ha-1) (Supporting Equation 1). In cases where bulk density was only available as the mean or had a single depth interval for multiple SOC concentrations, we used the best available value for bulk density to calculate SOC stocks for all associated observations in the study. We excluded studies that reported SOC concentration but did not report bulk density (see details in Supporting Material A.4). To obtain SOC stocks to a depth of 30 cm for comparison with IPCC, we summed all observations from the various separate depth intervals <30 cm that occurred in the same soil profile (e.g. summing SOC stocks for intervals 0-5, 5-10, 10-30 into one aggregated stock for SOC at <30 cm). Depth intervals >30 cm at any measure were excluded (e.g. 20-40 cm). SOC stocks for depth intervals >30 cm were included in separate calculations of SOC stocks for all depths measured, but were not compared with IPCC reference values.
The IPCC uses a stock change method to calculate changes in SOC for national inventories (see Supporting Material A.4 for more details) and provides default SOC stocks for “reference conditions” to a depth of 30 cm for several climate and soil types. Changes in SOC for different land uses and management practices are calculated using the IPCC method by multiplying SOC stocks for the reference condition(s) by a specific stock change factor for each individual land use and management practice (Supporting Equation 2). We therefore disaggregated SOC stock data by climate and soil type for comparison with IPCC reference SOC stocks to a depth of 30 cm, with our data consisting of two climates (tropical dry: <1000 mm yr-1; tropical wet: 1000-2000 mm yr-1) (54, 3.39) and four soil types (high activity clay, low activity clay, sandy, and volcanic soils) (53).
Although “reference condition” SOC stocks correspond to undisturbed native vegetation with minimal management, we found that mean SOC stocks for observations with undisturbed native grasslands/rangelands in our dataset (45.9 ± 16.0 Mg C ha-1; n = 12) were nearly identical to mean SOC stocks for observations classified as low to moderate grazing (45.0 ± 40.8; n = 141) (Supporting Table 6). Additionally, the IPCC default SCF for grasslands with low to medium grazing (SCF=1.0) is the same as grasslands under reference condition (SCF=1.0) (56). We therefore included observations with low to moderate grazing in our comparison with IPCC default reference SOC stocks.
We did not include SOC stocks for observations that were characterized as “high intensity grazing” or “severely degraded” in the comparison with IPCC default SOC stocks. However, SCFs for different grassland/rangeland management practices have default values listed by IPCC (Supporting Table 6), and these can be calculated as the simple response ratio of SOC stocks between a specific management practice and a designated control. Therefore, to compare with IPCC default SCFs for these management categories, we calculated SCFs for “high intensity grazing” and “severely degraded” separately as the response ratio between these categories and the calculated mean for undisturbed/low to moderate grazing in our dataset.
Finally, we evaluated the SOC sequestration potential of two grassland/rangeland management practices, namely long-term exclusion of livestock from grazing areas for rehabilitation purposes (i.e., “livestock exclosures”) and improved grazing management (e.g., lower stocking rates, paddocking, etc.), which consisted of several different improved grazing practices compared to an unimproved control practice. We computed the simple response ratio between treatment and control management practices in the individual studies for comparison with the IPCC default SCFs for “improved grassland” management (depths <=30 cm), as well as the mean SOC sequestration rate (Mg C ha-1 yr-1) for these practices.
We found a total of 21 studies or inventories across all countries in SSA with Tier 2 enteric CH4 EFs. This resulted in 93 observations for Tier 2 EFs for cattle and small ruminants (sheep and goats). We found that cattle accounted for most studies and observations, with 15 studies containing 70 observations (79% of all observations), while there were six studies containing 19 observations for small ruminants (21% of all observations). Nine studies used direct methods for estimating or measuring CH4 emissions, of which six studies used open-circuit respiration chambers, two used the sulfur hexafluoride tracer (SF6) and one study used the open path laser technique (Supporting Table 7). All studies using direct measurements (Figure 1D) took place in either Kenya (four studies) or South Africa (five studies). Most studies and observations used indirect estimation (Figure 1E) to obtain enteric CH4 EFs by estimating feed intake. Nearly all studies using the indirect EF estimation approach calculated feed intake indirectly based on animal energy requirements and feed digestibility. Most studies for enteric CH4 were conducted in East Africa (62% for cattle; 56% for small ruminants) or South Africa (23% for cattle; 33% for small ruminants), with only three studies in West Africa (two on cattle, one on small ruminants) (Supporting Table 7). There were no studies with enteric CH4 measurements in Central Africa.
For production systems, the most observed system was semi-intensive (i.e., smallholder mixed crop-livestock), which accounted for 43% of observations for cattle and 44% for small ruminants. Intensive systems (i.e., commercially-oriented stall-feeding) had a somewhat lower representation (30%) and were mainly conducted in South Africa, but the proportion of intensive systems in the data was high compared to the low prevalence of these systems at the continental scale (<10%) (57). In contrast, there were few observations from agropastoral and pastoral systems (22%) despite their high prevalence at the continental scale.
When comparing observed EFs to IPCC defaults, we find that enteric EFs for cattle in high productivity systems (41% of observations, n=28) are close to IPCC Tier 1a (2019) defaults for most age classes (mean difference: +4.5%; range: -10 to +18%) (Figure 2A). Low productivity systems accounted for the remaining 59% of enteric cattle EF observations (n=41), and mean EFs were lower than 2019 Tier 1a defaults for all age classes except for growing/replacement (mean difference: -15%; range: -43 to +4.6%) (Figure 2B). However, the variability of enteric EF values in the reviewed literature is high, particularly for the cattle growing/replacement category, which also accounted for most observations in low productivity systems.
Figure 2 Mean of enteric CH4 emission factors (EFs) for cattle in sub-Saharan Africa by class and compared to 54 Tier 1 and IPCC 2019 Tier 1a defaults. Error bars for calculated mean EFs are expressed as ± 95% CI, whereas errors bars for IPCC default EFs express default uncertainty as a percent (50%). Panel (A) shows high productivity cattle systems, panel (B) shows low productivity cattle systems. Mat. Females/males stand for mature females/males. n = number of individual observations for each sub-class.
There were six studies and 18 total observations reporting enteric CH4 EFs for small ruminants in SSA, with half of the studies conducted in South Africa. We found similar numbers of observations for sheep (10) and goats (9). We did not break down observations for small ruminant EFs by productivity level or age class due to the small number of studies and because IPCC guidelines do not provide default live-weight for sub-categories of small ruminants. The mean EFs in the reviewed literature were slightly higher than IPCC defaults for both sheep and goats (Figure 3). This effect is likely attributable to relatively high EFs for observations from South Africa and Ethiopia.
Figure 3 Mean of enteric CH4 emission factors (EFs) for small ruminants (sheep and goats) in sub-Saharan Africa compared to IPCC defaults EFs (EFs for small ruminants did not change between 2006 and 2019). Error bars for calculated mean EFs are expressed as ± 95% CI, whereas errors bars for IPCC default EFs express default uncertainty as a percent (50%). n = number of observations for each species.
We analyzed the activity data reported for studies with EFs for enteric CH4 in the previous section related to liveweight, methane conversion factor (Ym), milk yield, milk fat, milk protein content, feed digestibility, and crude protein in feed. For cattle activity data, 79% (11 studies) measured live-weights (Figure 1J) as part of the Tier 2 calculations, whereas the remaining three studies relied on literature values for cattle live-weights. Mean reported live-weights for high productivity cattle systems was generally much higher than IPCC 2019 Tier 1a default values for all age classes (404 vs. 250 kg for mature dairy females, 503 vs 275 kg for mature non-dairy females, 649 vs 540 kg for mature males, 393 vs 204 kg for growing/replacement, and 165 vs 82 kg for calves). Mean reported live-weights in low productivity systems were higher than IPCC for most age classes, including mature females (292 vs. 275 kg), mature non-dairy females (420 vs. 275 kg), growing/replacement (207 vs 185 kg), and calves (73 vs 57 kg), but lower for draft bullocks (241 vs 340 kg) (Supporting Table 8).
The methane conversion factor (Ym) (Figure 1A), which is defined as the proportion of feed intake converted to CH4 (19) is only available through direct measurements such as those using chamber measurements. Five studies using direct measurements for cattle reported Ym values (mean: 8.4 ± 0.5%) that were higher by 17-23% compared to the IPCC 2019 defaults of 6.5% for dairy cattle and 7.0% for other cattle (i.e., beef or multi-purpose), though this was still within range of uncertainty prescribed by IPCC guidelines. The IPCC 2019 defaults for Ym are identical for low and high productivity systems in SSA (Supporting Figure 2A).
For studies that directly measured milk yields (Figure 1H) as part of the EF calculations, 63% measured milk yields and mean milk yield was consistently higher than IPCC 2019 defaults in both low (mean: 3.87 ± 0.85 L d-1; range: +63 to +225%) and high productivity systems (mean: 9.45 ± 2.24 L d-1; range: +64 – 227%) (Supporting Figure 2B). In contrast to cattle milk yields, milk fat and protein content were similar to IPCC default values (Figure 1H; Supporting Figures 2C, D), though a few studies measured milk quality.
Four studies measured feed digestibility indirectly as part of EF calculations, while one study measured digestible energy. All studies measured dry matter digestibility or organic matter digestibility, rather than using digestibility of feed expressed as digestible energy as a fraction of gross energy, as per IPCC guidelines (Figure 1K) (50, Ch. 10). However, we assumed that dry matter digestibility would be similar to digestible energy based on local studies in SSA showing minimal differences in GE between feed and faeces on a per kg basis (Korir, pers. comm.). Results based on dry matter digestibility had a mean digestibility of 61%, which was similar to the IPCC 2019 default of 58% for “other cattle”, but somewhat higher than the value for “dairy cattle” (50: 51%) (Supporting Figure 2E).
For small ruminants, live-weights and feed digestibility were the only activity data measured in tandem with Tier 2 EF studies (Supporting Figure 3). Mean measured values for live-weights were slightly lower than IPCC 2019 defaults for both sheep (43 reported vs. 45 kg IPCC) and goats (37 vs 40 kg). Mean feed digestibility for small ruminants was 57% (n=6), but the IPCC does not provide specific defaults for small ruminant feed digestibility.
There were five studies using direct methods to investigate mitigation interventions for enteric CH4 emissions from cattle in SSA. A feeding study conducted by Ali et al. (58) using respiration chambers in Kenya found that feeding Holstein/Boran crossbred heifers on a diet of low-quality roughage (grass from native pasture) plus sweet potato vine silage reduced enteric CH4 yield by ~8% compared to a roughage-only diet (from 30.6 to 28.1 g CH4 kg-1 DMI). By contrast, supplementation with urea+molasses blocks showed no difference with the roughage only diet (30.6 g CH4 kg-1 DMI).
A feeding trial in South Africa using the SF6 tracer technique found that increasing dietary feed concentrate levels to a basal diet of pasture for exotic purebred (Jersey) cows reduced CH4 yield by ~14% from the lowest (29.1 g CH4 kg-1 DMI) to highest concentrate feeding level (25.1 g CH4 kg-1 DMI) (59). Another study in South Africa found that increasing dietary nitrate levels decreased CH4 emissions from Jersey cows on seeded pasture diets, with CH4 yield declining from 21.8 g CH4 kg-1 DMI in the control diet to 20.1 g CH4 kg-1 DMI (-8%) for low and 18.7 g CH4 kg-1 DMI (-14%) for high nitrate feeding levels (60).
Two studies examined mitigation interventions for enteric CH4 from small ruminants in South Africa. One study found that feeding exotic (Merino) sheep increasing levels of high-digestibility hay (Lespedeza cuneata) reduced enteric CH4 yield by ~21% (13.8 g CH4 kg-1 DMI for 60% L. cuneata hay) or ~19% (14.3 g CH4 kg-1 DMI for 90% L. cuneata hay) compared to sheep on a basal diet of low quality hay (17.6 g CH4 kg-1 DMI) (61). Adejoro et al. (62) found that several condensed tannin feed additives reduced enteric CH4 yield from exotic Merino sheep. Compared to the control without tannins (35.5 g CH4 kg-1 DMI), enteric CH4 yield were reduced by 12% for sheep supplemented with commercial tannins (21.7 g CH4 kg-1 DMI), by 30% for crude Acacia tannin extract (17.2 g CH4 g kg-1 DMI), and 19% for encapsulated Acacia tannin extract (20.0 g CH4 g kg-1 DMI).
We found a total of 14 studies that measured ruminant manure GHG emissions in SSA (Supporting Table 9). There were six studies with direct GHG measurements on deposited manure, seven on applied manure, and three on manure management. There were three studies with indirect estimates of emissions from manure management. All except of one study (goat manure) focused on cattle manure. All studies consisted of direct N2O measurements that allowed us to obtain N2O EFs, whereas we were only able to compute CH4 EFs directly from three studies. All studies with measurements for deposited manure and manure management were conducted in Kenya, whereas applied manure had three studies from Zimbabwe and four studies from Kenya.
For N2O from deposited manure, we disaggregated observations by excreta type (‘dung’, ‘urine’, ‘dung + urine’) and by climate (‘wet’, ‘dry’) to compare with IPCC default EFs for these categories (Figure 4A). Studies were conducted in both dry and wet seasons, but we found no difference in EFs between seasons (Supporting Figure 4) and therefore report all observations per annum. For deposited manure CH4, IPCC guidelines do not disaggregate by excreta type or climate, so we only report the overall mean EF here (Figure 4B). We found that all deposited manure studies focused on N2O and CH4 emissions from cattle dung or urine in dry climates, with no studies conducted in wet climates (i.e., >1000 mm annual precipitation). For N2O, the deposited manure EF was lower by 29% for cattle dung (0.05 ± 0.02%) compared to 2019 IPCC default N2O EFs for dry climates (mean value of 0.07% for dung), whereas the mean N2O EF for cattle urine in dry climates (0.32 ± 0.17%) was identical to the 2019 IPCC default mean value (0.32% for urine). The mean CH4 EF for deposited manure (0.69 ± 0.34 kg CH4 animal-1 year-1) was 31% lower than the IPCC default value for non-dairy cattle (1 kg CH4 animal-1 year-1), albeit based on a small number of observations.
Figure 4 Calculated means of EFs for different categories of manure in sub-Saharan Africa. Bars show mean 95% CI of reported observations and IPCC 2019 defaults ± uncertainty (expressed as percentage) for N2O (A) and CH4 EFs (B) for manure on pasture, range, and paddock (deposited manure) disaggregated by climate (‘wet’, ‘dry’) and excreta type (‘dung’, ‘urine’); (C) N2O EF for manure applied as organic fertilizer (applied manure); EFs for N2O (D) and CH4 (E) for manure managed as “solid storage”; EFs for N2O (F) and CH4 (G) for manure managed as “drylot” (i.e. livestock enclosures). The EF for N2O are reported as the proportion of N in manure that is emitted as N2O. The EFs for CH4 from deposited manure (A) are reported in kg of CH4 emitted per animal per year, whereas manure management EFs (D–G) are reported in g CH4 emitted per kg of manure volatile solid (VS). n = number of observations for each manure category and GHG used for mean ± 95% CI calculation.
For N2O emissions from applied manure (Figure 4C), we followed IPCC guidelines and disaggregated by climate (‘wet’, ‘dry’) but not by excreta type (dung or urine) or animal species. Emissions of CH4 from applied manure (i.e., daily spread) were not reported in any of the studies reviewed here. The N2O EFs for applied manure consisted of cattle manure applied to crops in ‘dry’ climates (Kenya, Zimbabwe), whereas the ‘wet’ climate data was derived from cattle manure applied to fields in Kenya, with one observation for goat in Kenya. The mean applied manure N2O EF for the ‘wet’ climate (mean: 0.02%) was substantially lower (-97%) than the IPCC default (0.60%). The ‘dry’ climate N2O EF for applied manure (-0.01) was also much lower (-102%) compared to the IPCC default for ‘dry’ climates (0.50%). For ‘dry’ climates, we removed outlier values with very high N2O emissions from one study in Zimbabwe where manure was applied to drained wetland soils. Inclusion of extreme data points from this study increased the mean N2O EF to 1.08%, which was 116% larger than the IPCC default (Supporting Figure 5).
We found only two published studies (plus one unpublished study) with direct measurements and EFs for manure management (Figure 1C). One study reported emissions from solid storage (“manure heaps” or “stockpiles”) of cattle manure in Kenya (Figure 4D, E). In this study, N2O EFs (0.6 ± 0.1%) were 40% lower than 2019 IPCC defaults for solid storage (1%), and reported CH4 EFs (2.5 ± 0.4 g CH4 kg-1 VS) were 43% lower than the 2019 IPCC default (4.4 g CH4 kg-1 VS). The other two manure management studies measured emissions from overnight livestock enclosures (i.e., ‘bomas’, ‘kraals’), which we classified as ‘drylot” according to IPCC guidelines. Both studies were conducted in dry climates under extensive livestock systems in Kenya and had N2O EFs ranging from 1.34 to 2.43% N2O-N compared to IPCC default for ‘drylot’ of 2.0% N2O-N (Figure 4F) (63; Leitner, pers. comm.) A preliminary estimate of CH4 EF for cattle enclosures was 2.65 ± 0.4 g CH4 kg-1 VS, which was somewhat higher than the default for ‘drylots’ (1.70 ± 0.5 g CH4 kg-1 VS) (Figure 4G) (Leitner, pers. comm.).
There were no direct studies on any other manure management systems (such as slurry tank, composting, anaerobic digester) from SSA that fulfilled quality criteria. We found multiple indirect estimates of Tier 2 CH4 EFs from manure management in national inventories, but comparison with direct measurements was difficult because reported EFs are in different units (i.e., kg CH4 head-1 yr-1) and represent composite values from several different manure management practices. However, compared against the 54 default for “solid storage”, the mean indirect CH4 EF was higher than the IPCC default value for cattle (2.7 vs 1.0 kg CH4 head-1 yr-1) (Supporting Figure 6).
We found a total of 50 studies reporting SOC for grassland and rangeland ecosystems, resulting in a database with 355 observations from 14 countries in SSA (Supporting Table 5). Aggregation of intervals by depth for with IPCC resulted SOC stocks for 176 observations <=30 cm and 221 observations aggregated for the entire soil profile (i.e. including depths >30cm). Most observations (74%) consisted of SOC sampled at depths <=30 cm, with an overall average sampling depth of ~30 cm across 16 separate depth intervals measured (ranging from 0-5 to 100-140 cm). There were large regional disparities in the number of observations from grasslands and rangelands, with East (n = 162) and Southern Africa (n = 146) accounting for 46 and 41% of observations, whereas West Africa (n = 47) represented only 13% (Supporting Table 10). Similar to enteric CH4 and manure emissions, three countries – South Africa, Ethiopia, and Kenya – accounted for 68% of all observations in the SOC dataset.
A large proportion of studies and observations did not report the soil type (50%; n = 178), with many only reporting soil texture class. The most reported soil type was low activity clay (20%; n = 72), followed by high activity clay (16%, n = 56), sandy (8%, n = 30), and volcanic soils (5%, n = 19). There were more observations from dry climates (83%, n = 293) than wet climates (17%, n = 60). The most frequent study designs were land use comparisons (23 studies and 165 observations), livestock exclosure studies (12 studies, 110 observations), and land degradation studies (6 studies, 27 observations). Some studies also included stocking/grazing management as part of the main study design (8 studies, 72 observations).
Calculated mean SOC stocks <=30 cm (mean sampling depth: ~18cm) for grassland/rangeland soils under undisturbed native vegetation and low to moderate grazing were generally similar to IPCC reference SOC stocks for most soil types in both dry and wet climates (Figure 5). Exceptions to this included volcanic soils in dry climates and high activity clay and volcanic soils in wet climates. Unclassified soils (i.e., soil type not reported in study) were the most numerous grouping and had the highest mean SOC stocks in both climate types. The overall means for SOC stocks <=30 cm in both dry (33 Mg C ha-1) and wet climates (83 Mg C ha-1) were higher than the IPCC reference SOC stocks for all individual soil types. We found that including depth intervals >30 cm to calculate SOC stocks deeper in the soil profile resulted in an overall mean SOC stock of 54.1 Mg C ha-1, which was 27% higher than the mean SOC stock of 42.5 Mg C ha-1 at <=30 cm across all climate and soil types. This implies that SOC sampling at depths less than or equal to the IPCC default of 30 cm may miss a substantial portion of the total SOC stock in the profile for grassland and rangeland soils in SSA (Supporting Figure 7).
Figure 5 Calculated means of soil organic carbon (SOC) stocks (Mg C ha-1) for depth intervals <=30 cm for grasslands and rangelands in sub-Saharan Africa compared to IPCC default reference condition SOC stocks to 30 cm for different climates and soil types. Bars show SOC stocks in (A) tropical dry climates (<1000 mm yr-1) and (B) tropical wet climates (1000 – 2000 mm yr-1) for high activity clay, low activity clay, sandy, and volcanic soils. Error bars correspond ± 95% CI for reported observations, whereas error bars for IPCC 2019 defaults express uncertainty as percentage of the mean. n = number of observations used for mean ± 95% CI calculation.
The calculated SCF as a simple response ratio of SOC stocks for “high intensity grazing” (0.93; n = 8) was similar to the IPCC default SCF (0.90) for this management practice. However, the SCF calculated based on SOC stocks for “severely degraded” soils was much lower (0.32; n = 14) than the IPCC default SCF (0.70) (Supporting Table 6). The SCF for livestock exclosures compared to the control (grazed) was 1.22, which corresponded to a mean SOC sequestration rate of 0.46 (± 0.66) Mg C ha-1 yr-1 (mean study duration: 19.8 yrs). The SCF for improved grazing management practices against the control was 1.23 and the mean SOC sequestration rate was 0.79 (± 0.93) Mg C ha-1 yr-1 (mean study duration: 22.9 yrs). The SCFs for both exclosures and improved grazing management were close to the IPCC default SCF for tropical improved grassland management (1.17).
Enteric CH4 emissions from ruminant livestock are estimated to account for ~67% of emissions from the agricultural sector in SSA (http://edgar.jrc.ec.europa.eu/overview.php?v=432_AP, last access: April 2022), so improving estimates and reducing uncertainty should be a high research priority. Our results reveal that while there has been research progress on enteric CH4 emissions from SSA in recent years, there are still relatively few studies and several major data gaps for important categories. Low productivity cattle had generally lower Tier 2 EFs compared to IPCC Tier 1 defaults, implying that studies relying on Tier 1 values for cattle inventories may overestimate emissions. Small ruminants had higher Tier 2 EFs compared to IPCC Tier 1 defaults, but this was based on limited data and it is unclear whether these values are representative on the continental scale. IPCC Tier 1 EFs for enteric CH4 could thus be improved by including results presented here, rather than relying mainly on modeling inputs to obtain continental scale EFs for African livestock systems.
Research priorities for enteric CH4 EFs moving forward should address the nearly complete lack of data on pastoral and agropastoral systems, which predominate in arid and semi-arid regions and account for most of the livestock population across all species in SSA (63% of cattle, 82% of sheep, and 70% of goats) (64). Although small ruminants have lower enteric CH4 emissions per head compared to cattle, their population in SSA is expected to rise due to climate change (65) and is large relative to the small number of studies conducted thus far. More research is needed to characterize the diversity of smallholder mixed-crop livestock systems and associated EFs, while also linking CH4 emissions and productivity levels (66). Research on all species and production systems is urgently needed for West, Central, and Southern Africa (outside of South Africa) to improve geographic representation.
Filling data gaps on enteric CH4 emissions will require additional studies using both direct (Figure 1D) and indirect methods (Figure 1E). Respiration chamber experiments are considered the “gold standard” for directly measuring CH4 (51), but they have limitations with respect to animal mobility, diet selection, and environmental interactions (67). Other direct methods, such as the SF6 tracer technique, can provide complementary measurements of enteric CH4 emissions under more realistic field conditions, which is particularly relevant for grazing and pastoral/agropastoral systems (52, 68). The Greenfeed system (C-Lock Inc., Rapid City, USA) may be more useful for in situ measurements for stall-feeding systems (e.g., intensive and smallholder mixed crop-livestock) (53). We currently do not consider the laser methane detector method to be suitable for developing EFs, but this approach could be useful for ranking mitigation options (69). Ongoing advances that increase the accuracy of laser methane detector could reduce the costs of enteric CH4 measurements and make this method more attractive for SSA (70). Indirect methods enable estimates outside of laboratory in “real-world” conditions, and they have proven effective for estimating Tier 2 EFs for livestock systems in SSA (20, 21). Indirect estimations based on household surveys can highlight heterogeneity in enteric CH4 emissions and productivity between farms and may be useful in identifying drivers and developing mitigation strategies for enteric emissions (71, 72).
Accurate activity data are essential for developing Tier 2 national inventories and MRV, and it has been suggested that collecting Tier 2 activity data may provide a better return on investment in improving national GHG inventories compared to experimental research on enteric CH4 emissions (34). Multiple studies in SSA have analyzed uncertainty and sensitivity of activity data and other Tier 2 model inputs (e.g., coefficients) and found that feed digestibility and methane conversion factor (Ym) are usually the two most important factors. Live-weight and live-weight gain for dairy cattle, as well as total population of cattle in different production systems are additional major sources of uncertainty (73, 74).
Diet composition and feed digestibility (Figure 1K) are likely the most important activity data for Tier 2 enteric CH4 EFs because feed digestibility affects several other variables for both enteric CH4 and manure emissions (see Section 4.2; Figure 1). Diet composition and feed digestibility for livestock systems in SSA are highly uncertain because feed sources are diverse, and exhibit strong seasonal and geographic variability, especially for mixed crop-livestock systems (2, 20, 21, 75). Further studies characterizing diet composition are needed for representative livestock systems and could conducted using relatively low cost, out-of-the-box survey tools such as the Rural Household Multi-Indicator Survey (RHoMIS, 76). Constructing a comprehensive database of existing studies of diet composition and digestibility of common feeds in SSA should be high priority for improving Tier 2 enteric CH4 estimates and inventories (77).
We found that studies in SSA had a consistently higher mean Ym (Figure 1A) for cattle compared to IPCC defaults. This could be explained by low feed quality (i.e., low digestibility, high neutral detergent fibre) and more seasonal variations in feed intake (i.e., sub-maintenance feeding for part of the year) compared to systems on which IPCC Ym estimates are based (59, 60, 74, 78–82 Korir, pers. comm., unpublished). Further, although available studies using mainly indirect methods suggest that Tier 2 cattle EFs are lower than IPCC Tier 1 defaults, most studies were based on IPCC defaults for Ym. Using higher Ym values such as those found in studies reported here (Supporting Figure 2) instead of defaults would likely result in higher CH4 EFs for cattle in SSA. This discrepancy highlights the need for improved data to inform models and develop EFs for livestock across a range of production systems, species, and breeds in SSA.
Other important activity data, such as livestock population and herd structure (Figure 1B), and live-weights (i.e., animal performance data) (Figure 1J) must be collected via government surveys and/or through collaborations with non-government organizations. These activity data should be high priority for African governments to reduce uncertainty in GHG inventories, but so far only six countries have collated Tier 2 level activity data for enteric CH4 emissions (Mwape, pers. comm.). Activity data collection in the field is costly and labor intensive, and in many instances literature values are used in lieu of field measurements to reduce the costs of Tier 2 EF estimates (83–85). Future research is needed to assess the sensitivity of enteric CH4 emissions estimates to different methods and sources of activity data, and to reduce costs of activity data collection more generally (71). The scientific research community can contribute to government-led efforts by providing decision support and developing low-cost tools for data collection. For example, simple methods based on heart girth measurements have been developed for estimating cattle live-weights, which could be useful for national-scale data collection (86). Stratifying the collection of activity data by agro-ecological zone and livestock system rather than administrative units (e.g., counties, districts) has the potential to reduce costs by allowing usage of EFs and activity data for similar systems in other countries, but more work is required to assess the compatibility of this type of stratification with national surveys that rely on administrative units.
Finally, appropriate CH4 mitigation intervention trials for livestock systems in SSA are critical to assess mitigation potential, target interventions, and MRV in the livestock sector, but we found only two studies testing mitigation practices for livestock outside of South Africa. Most current projections of the CH4 mitigation potential from livestock in SSA rely on modelled data, which may be constrained by limited data for African systems (87). Further research on economically feasible and locally appropriate mitigation options should be prioritized for important livestock systems in SSA (66, 88).
We found that studies directly measuring manure CH4 and N2O emissions in SSA have been relatively limited in terms of focus and geographic scope to this point, and that the mean reported EFs for N2O were similar to IPCC Tier 1 EFs for deposited manure, but lower than IPCC Tier 1 EFs for applied manure and manure management. Mean N2O EFs for deposited manure in this review were an order of magnitude lower than N2O EFs reported for cattle dung and urine in other tropical systems outside SSA (23). This can be explained by low feed intake and poor feed quality for cattle in SSA resulting in low N concentrations in dung and urine, as well as high C:N ratios in dung (36, 89, 90). In addition, feed intake levels and dietary N content (Figure 1L) influence N partitioning between dung and urine because lower feed intake and dietary N content result in less N being excreted via urine and a lower urine-N:dung-N ratio for low productivity systems in SSA (34:66 or lower) compared to high productivity systems (66:34) (90, 91). Urine-N consists mainly of urea and ammonium, which is more easily converted to N2O than the organic N from dung, so a low urine-N:dung-N ratio reduces manure N2O emissions (92, 93). However, low-N diets restrict livestock production, so this must be put into context with livestock productivity to support livelihoods and food and nutrition security. Lower EFs than IPCC CH4 EFs for deposited manure may be related to higher dung C:N ratios and warm and dry climatic conditions, which cause smaller and drier dung patches and therefore reduce the potential for anoxic conditions and CH4 production (89, 94–96).
For manure applied as fertilizer, the low reported N2O EF for manure management may also be related to low feed quality, which results in low manure N concentrations and high C:N ratios. Similarly, the low CH4 EF study may be attributable to feed quality since high fiber, high ash contents, and low N concentrations are thought to reduce the activity of methanogenic microorganisms (24). Manure management practices may affect the physical and chemical properties of applied manure and therefore associated emissions of N2O in the field (97).
These results indicate that inventories relying on IPCC Tier 1 default values, even with the new 2019 refinement, may still overestimate GHG emissions from manure in SSA. National inventories at the Tier 1 level for N2O and CH4 emissions from applied and deposited manure require data on N excretion/application rates, along with activity data on livestock population by species. Emissions from manure management require additional data on the proportion of animals in each manure management system. Tier 1 inventories could be improved by replacing IPCC default EFs with country or regionally-specific N2O and CH4 EFs for manure based on locally conducted field studies, such as those presented here. For deposited manure, country- or region-specific N2O EFs should be developed separately for urine and dung and should also consider climate type (23). Given the current bias in the data toward cattle in dry climates, there is a need for more research to determine manure EFs from multiple species across the diverse range of livestock systems, soil types, and climatic conditions present in SSA.
Even more surprising is the lack of in situ studies measuring GHG emissions from manure management systems in SSA (Figure 1C). Emissions from several important manure management systems (particularly pit storage, feedlot, liquid/slurry, anaerobic digester, and passive composting) have yet to be characterized and this should be high priority. There is also a paucity of data on the proportion of manure in different manure management systems for SSA – data manure management systems has not been collected at the national level for inventories (Mwape, pers. comm.) and there are only few studies determining the state of existing manure management systems in SSA at broad geographic scales (97). The lack of data on the proportion of manure managed under different systems is the largest contributor to uncertainty in emissions from manure management in SSA (26, 73), so the research community should prioritize development of low-cost tools (such as household interviews) to help governments acquire the requisite data and reduce uncertainty for GHG inventories of manure management. No studies have examined the potential for GHG mitigation from manure management, but research indicates that existing manure management practices in SSA are inefficient in terms of conserving nutrients and reducing losses from volatilization, leaching, runoff, and emissions (66).
In addition to the general lack of data on manure management in SSA, we found only two studies with direct estimates of emissions from livestock enclosures. Livestock enclosures and other areas where livestock congregate in grazing-based rangeland systems, such as around water pans, accumulate large volumes of manure, which is not removed or managed, and can remain GHG emission hotspots for years to decades after use (98). For SSA, emissions from livestock enclosures have been shown to be orders of magnitudes higher than from background soils and manure patches deposited during grazing activity, and are estimated to account for 5-10% of N2O emissions on the continent (63). Although we compared with default IPCC values for ‘drylot’ management, these critical manure management are overlooked by the IPCC because they do not fit into any of the manure management system classifications and this should be rectified by adding overnight enclosures as a category in future IPCC guidelines. Further, multiple interventions have been proposed to mitigate GHG emissions from livestock enclosures, including moving enclosures more frequently (after days or weeks, instead of months or years) to reduce manure accumulation and subsequent GHG emissions in these areas (63), or remove manure periodically from enclosures instead of leaving it to decompose.
Tier 1 inventories for manure emissions can be enhanced by better activity data on N excretion rates. Feed quantity and quality affect manure N2O and CH4 emissions, and the lower N content (Figure 1L) of feed and consequently manure in African systems should be accounted for when calculating N excretion rates for national inventories. N excretion rates depend critically on feed intake and digestibility, and studies have shown that feed digestibility is the most uncertain factor for all three types of manure emissions (73, 74). Thus, improved activity data on diet composition, feed digestibility, feed crude protein or N content, and livestock feeding situations (e.g., feed scarcity) should be the highest priority for reducing uncertainty in emissions of both enteric CH4 and manure N2O and CH4 emissions.
Finally, while short-term research should prioritize improving Tier 1 estimates of emissions from manure in SSA as described in this section, much of this research will contribute to the longer-term goal of moving towards Tier 2 inventories for manure GHGs. Tier 2 inventories will require country- or regionally-specific EFs for manure across a range of different soil types, management practices, and climatic conditions. In addition to such measurements, Tier 2 inventories will require spatially explicit data on soil properties at the national scale, some of which has recently become available for SSA through digital soil data platforms (99). Data on manure N losses via leaching/runoff and NH3/NOx volatilization is required for Tier 2 N2O EFs and this should be high priority for research moving forward due to limited studies in SSA, since the lack of data renders the magnitude and importance of these pathways highly uncertain at present (98).
Although indirectly connected to livestock emissions, SOC stocks for grasslands and rangelands are included in national inventories using the stock change method described above, and changes in SOC stocks due to land use or management practices contribute to the overall GHG budget for inventories. Improved management of grasslands and rangelands to sequester SOC has been proposed as one of the few methods available for offsetting livestock GHG emissions from extensive pastoralist production systems in SSA (100, 101). SOC sequestration on agricultural land has also been proposed as a major mechanism for offsetting fossil fuel emissions through international initiatives such as the ‘4 per 1000’ program (102).
Development of Tier 2 inventories for SOC stocks requires the development of country-specific SOC stocks for “reference conditions” (i.e., undisturbed native vegetation) across all climate and soil types present in each country, regardless of land use. We obtained SOC stocks for a single land use (grassland/rangelands), but our results imply that SOC stocks for undisturbed native vegetation and those with low to moderate grazing may be interchangeable.
There was a strong geographic bias in the SOC data and there is a need for extensive SOC data collection in West and Southern Africa. However, in the absence of widely available country-specific SOC data, African countries may be able to develop Tier 2 SOC inventories by using data from countries with similar climate and soil types. Tier 2 SOC inventories could also be developed by extracting the appropriate data from recently developed SOC databases, such as those provided by the Africa Soil Information Service (AfSIS) (103, 104).
Development of Tier 2 inventories will require country-specific stock change factors for various grassland and rangeland management practices, and we found that SCFs calculated from the literature were largely similar to IPCC values. Mean SOC sequestration rates for livestock exclosures and improved grazing were both positive, albeit with high uncertainty, and within the range of reported SOC storage in grasslands and rangelands more generally (i.e., 0.11 – 1.0 Mg C ha-1 yr-1) (105). However, management practices can have positive or negative effects on SOC depending on environmental conditions (39–41), so more research on these practices across a range of conditions is required.
Tier 2 inventories should follow IPCC guidelines on reporting SOC stocks, which strongly recommend calculating SOC stocks on a mass-equivalent (i.e. adjusted for bulk density) rather soil-volume equivalent (i.e., fixed depth) basis (53). Failure to adjust for bulk density has been shown to lead to inaccurate estimates of SOC stocks, particularly due to changes in land use or management (106–108). However, our initial literature search of SOC stocks found that >25% of studies did not report bulk density and were therefore not included in this review.
Taken together, this implies a strong need to conduct rigorous SOC sampling across the breadth of grassland/rangeland management practices present in SSA to reduce uncertainty in reference SOC stocks and provide accurate SCF values for country-specific Tier 2 SOC inventories. Further research is needed to examine changes in SOC stocks over time, along with changes in biomass carbon and net ecosystem exchange (see Supporting Material B.1, Supporting Table 11) due to changes in vegetation, management, and global change forces (e.g., climate change, CO2 fertilization effects, degradation, woody encroachment, etc.) Finally, our results show that depths >30 cm store a large proportion of total SOC (Supporting Figure 7), so sampling at greater depths and accounting for this in national inventories may be an overlooked aspect within IPCC guidelines.
Our results demonstrate that there has been some research progress on livestock GHG emissions and SOC stocks in SSA with a marked increase in the number of studies reporting these measurements over the past several years, which is encouraging. Nevertheless, academic research and African governments face a number of challenges with respect to livestock and GHG emissions, including insufficient finance for climate-related goals, lack of research capacity, and poor research infrastructure (28). Research on climate change and the environment in SSA is underfunded (109), and often lacks qualified personnel and resources (110). Research infrastructure and equipment for monitoring GHG emissions is lacking in SSA for several reasons (remote locations, poor infrastructure, energy supply issues, poor maintenance, etc.), which makes research on climate change mitigation and adaptation difficult (28).
Despite these challenges, there have been notable successes in forwarding the agenda of livestock and GHGs in SSA. For example, the Mazingira Centre at the CGIAR’s International Livestock Research Institute has established a hub for scientific research, capacity building, and cutting-edge research infrastructure on the environment in SSA with a focus on livestock in East Africa. Such initiatives may provide a blueprint for upscaling to other regions, and recent analysis shows that the cost of developing a pan-African GHG monitoring infrastructure is relatively low (estimated at $630M USD over the next 30 years) (31). To meet government objectives on livestock and climate, such initiatives could benefit from greater coordination at the science-policy interface through enhanced collaboration and institutional arrangements between and within the research community and African governments (111, 112).
MG conducted all literature searches, data analyses, and writing of the manuscript. CA and SL provided primary oversight and contributions to the manuscript writing and analysis process. KB-B, LDT, DK, LM, AM, PN, DP, MR, TVDW, and AW all provided major feedback, comments, and suggestions in terms of both the overall direction and specific changes to the manuscript and associated literature searches and analyses. All authors contributed to the article and approved the submitted version.
This study was funded by the German Federal Ministry for Economic Cooperation and Development (BMZ issued through GIZ) through the “Programme of Climate Smart Livestock” (PCSL, Programme No. 2017.0119.2). Additional funding was provided by EU DeSIRA project “Earth observation and environmental sensing for climate-smart sustainable agropastoral ecosystem transformation in East Africa” (ESSA). Support for this study was provided through Mitigate+: Research for Low Emissions Food Systems. We would like to thank all funders who supported this research through their contributions to the CGIAR Trust Fund. This review also benefitted from conversations with Laura Cramer, Gretchen Gettel, Alice Onyango, and Yuhao Zhu.
The authors declare that the research was conducted in the absence of any commercial or financial relationships that could be construed as a potential conflict of interest.
All claims expressed in this article are solely those of the authors and do not necessarily represent those of their affiliated organizations, or those of the publisher, the editors and the reviewers. Any product that may be evaluated in this article, or claim that may be made by its manufacturer, is not guaranteed or endorsed by the publisher.
The Supplementary Material for this article can be found online at: https://www.frontiersin.org/articles/10.3389/fsoil.2022.927452/full#supplementary-material
1. Weiler V, Udo HM, Viets T, Crane TA, De Boer IJ. Handling Multi-Functionality of Livestock in a Life Cycle Assessment: The Case of Smallholder Dairying in Kenya. Curr. Opin. Environ. Sustain. (2014) 8:29–38. doi: 10.1016/j.cosust.2014.07.009
2. Ndung’u PW, Kirui P, Takahashi T, du Toit CJL, Merbold L, Goopy JP. Data Describing Cattle Performance and Feed Characteristics to Calculate Enteric Methane Emissions in Smallholder Livestock Systems in Bomet County, Kenya. Data Brief (2021) 39:107673. doi: 10.1016/j.dib.2021.107673
3. Mekonnen S, Descheemaeker K, Tolera A, Amede T. Livestock Water Productivity in A Water Stressed Environment in Northern Ethiopia. Exp. Agric. (2011) 47(S1):85–98. doi: 10.1017/S0014479710000852
4. Herrero M, Grace D, Njuki J, Johnson N, Enahoro D, Silvestri S, et al. The Roles of Livestock in Developing Countries. Animal (2013) 7(s1):3–18. doi: 10.1017/S1751731112001954
5. Herrero M, Havlík P, Valin H, Notenbaert A, Rufino MC, Thornton PK, et al. Biomass Use, Production, Feed Efficiencies, and Greenhouse Gas Emissions From Global Livestock Systems. Proc. Natl. Acad. Sci. U.S.A. (2013) 110(52):20888–93. doi: 10.1073/pnas.1308149110
6. Thornton PK, Herrero M. Integrated Crop–Livestock Simulation Models for Scenario Analysis and Impact Assessment. Agric Syst (2001) 70(2):581–602. doi: 10.1016/S0308-521X(01)00060-9
7. Rojas-Downing MM, Nejadhashemi AP, Harrigan T, Woznicki SA. Climate change and livestock: Impacts, adaptation, and mitigation. Climate Risk Manage (2017) 16:145–63. doi: 10.1016/j.crm.2017.02.001
8. Mapfumo L, Muchenje V, Mupangwa JF, Scholtz MM, Washaya S, Mapfumo L, et al. Dynamics and Influence of Environmental Components on Greenhouse Gas Emissions in Sub-Saharan African Rangelands: A Review. Anim. Product. Sci. (2021) 61(8):721–30. doi: 10.1071/AN20564
9. Collier RJ, Gebremedhin KG. Thermal biology of domestic animals. Annu Rev Anim Biosci (2015) 3:513–32.
10. Rahimi J, Ago EE, Ayantunde A, Berger S, Bogaert J, Butterbach-Bahl K, et al. Modelling Gas Exchange and Biomass Production in West African Sahelian and Sudanian Ecological Zones. Geoscie. Model Dev. Discuss. (2021) 14(6):1–39. doi: 10.5194/gmd-2020-417
11. Thornton PK, Boone RB, Galvin KA, BurnSilver SB, Waithaka MM, Kuyiah J, et al. Coping Strategies in Livestock-Dependent Households in East and Southern Africa: A Synthesis of Four Case Studies. Hum. Ecol. (2007) 35(4):461–76. doi: 10.1007/s10745-007-9118-5
12. Thornton PK, van de Steeg J, Notenbaert A, Herrero M. The Impacts of Climate Change on Livestock and Livestock Systems in Developing Countries: A Review of What We Know and What We Need to Know. Agric. Syst. (2009) 101(3):113–27. doi: 10.1016/j.agsy.2009.05.002
13. Rahimi J, Mutua JY, Notenbaert AMO, Marshall K, Butterbach-Bahl K. Heat Stress Will Detrimentally Impact Future Livestock Production in East Africa. Nat. Food (2021) 2(2):88–96. doi: 10.1038/s43016-021-00226-8
14. Tessema B, Sommer R, Piikki K, Söderström M, Namirembe S, Notenbaert A, et al. Potential for Soil Organic Carbon Sequestration in Grasslands in East African Countries: A Review. Grassland. Sci. (2020) 66(3):135–44. doi: 10.1111/grs.12267
15. Mgalula ME, Wasonga OV, Hülsebusch C, Richter U, Hensel O. Greenhouse Gas Emissions and Carbon Sink Potential in Eastern Africa Rangeland Ecosystems: A Review. Pastoralism (2021) 11(1):19. doi: 10.1186/s13570-021-00201-9
16. Vernooy R, Bouroncle C. Climate-Smart Agriculture: In Need of a Theory of Scaling (Working Paper) (2019). Available at: https://cgspace.cgiar.org/handle/10568/99822.
17. Wilkes A, Reisinger A, Wollenberg EK, van Dijk S. Measurement, reporting and verification of livestock GHG emissions by developing countries in the UNFCCC: current practices and opportunities for improvement (2017). Available at: https://cgspace.cgiar.org/handle/10568/89335.
18. Rose S, Khatri-Chhetri A, Stier M, Wilkes A, Shelton SW, Arndt C, et al. Livestock Management Ambition in the New and Updated Nationally Determined Contributions: 2020-2021: Analysis of Agricultural Sub-Sectors in National Climate Change Strategies (2021). Available at: https://cgspace.cgiar.org/handle/10568/115885.
19. IPCC. Chapter 6: Grasslands. In: 2019 Refinement to the 2006 IPCC Guidelines for National Greenhouse Gas Inventories (2019) Intergovernmental Panel on Climate Change.
20. Goopy JP, Onyango AA, Dickhoefer U, Butterbach-Bahl K. A New Approach for Improving Emission Factors for Enteric Methane Emissions of Cattle in Smallholder Systems of East Africa – Results for Nyando, Western Kenya. Agric. Syst. (2018) 161:72–80. doi: 10.1016/j.agsy.2017.12.004
21. Ndung’u PW, Bebe BO, Ondiek JO, Butterbach-Bahl K, Merbold L, Goopy JP, et al. Improved region-specific emission factors for enteric methane emissions from cattle in smallholder mixed crop: livestock systems of Nandi County, Kenya. Anim Production Sci (2018) 59(6):1136–46. doi: 10.1071/AN17809
22. Goopy JP, Korir D, Pelster D, Ali AIM, Wassie SE, Schlecht E, et al. Severe below-maintenance feed intake increases methane yield from enteric fermentation in cattle. Br J Nutr (2020) 123(11):1239–46. doi: 10.1017/S0007114519003350
23. Zhu Y, Butterbach-Bahl K, Merbold L, Leitner S, Pelster DE. Nitrous Oxide Emission Factors for Cattle Dung and Urine Deposited Onto Tropical Pastures: A Review of Field-Based Studies. Agri. Ecosyst Environ. (2021) 322:107637. doi: 10.1016/j.agee.2021.107637
24. Leitner S, Ring D, Wanyama GN, Korir D, Pelster DE, Goopy JP, et al. Effect of Feeding Practices and Manure Quality on CH4 and N2O Emissions From Uncovered Cattle Manure Heaps in Kenya. Waste. Manage (2021) 126:209–20. doi: 10.1016/j.wasman.2021.03.014
25. Ndao S. Analysis of Inputs Parameters Used to Estimate Enteric Methane Emission Factors Applying a Tier 2 Model: Case Study of Native Cattle in Senegal. Animal Feed Science and Nutrition - Production, Health and Environment. IntechOpen. (2021). doi: 10.5772/intechopen.99810.
26. Wilkes A, Pica-Ciamarra U, Opio C. Livestock activity data guidance (L-ADG). In: methods and guidance on compilation of activity data for Tier 2 livestock GHG inventories. Livestock Activity Data Guidance (L-ADG): Methods and Guidance on Compilation of Activity Data for Tier 2 Livestock GHG Inventories (2020). Available at: https://www.cabdirect.org/cabdirect/abstract/20203565720.
27. von Fromm SF, Hoyt AM, Lange M, Acquah GE, Aynekulu E, Berhe AA, et al. Continental-Scale Controls on Soil Organic Carbon Across Sub-Saharan Africa. Soil (2021) 7(1):305–32. doi: 10.5194/soil-7-305-2021
28. López-Ballesteros A, Beck J, Bombelli A, Grieco E, Lorencová EK, Merbold L, et al. Towards a Feasible and Representative Pan-African Research Infrastructure Network for GHG Observations. Environ.Res. Lett. (2018) 13(8):085003. doi: 10.1088/1748-9326/aad66c
29. Uwizeye A, de Boer IJM, Opio CI, Schulte RPO, Falcucci A, Tempio G, et al. Nitrogen Emissions Along Global Livestock Supply Chains. Nat. Food (2020) 1(7):437–46. doi: 10.1038/s43016-020-0113-y
30. FAO. The Share of Agri-Food Systems in Total Greenhouse Gas Emissions: Global, Regional and Country Trends 1990–2019. Rome, Italy: FAO (2021). Available at: https://www.fao.org/publications/card/en/c/CB7514EN/.
31. Merbold L, Scholes RJ, Acosta M, Beck J, Bombelli A, Fiedler B, et al. Opportunities for an African Greenhouse Gas Observation System. Reg. Environ. Change (2021) 21(4):104. doi: 10.1007/s10113-021-01823-w
32. Mottet A, Henderson B, Opio C, Falcucci A, Tempio G, Silvestri S, et al. Climate Change Mitigation and Productivity Gains in Livestock Supply Chains: Insights From Regional Case Studies. Reg. Environ. Change (2017) 17(1):129–41. doi: 10.1007/s10113-016-0986-3
33. Assouma MH, Hiernaux P, Lecomte P, Ickowicz A, Bernoux M, Vayssières J. Contrasted Seasonal Balances in a Sahelian Pastoral Ecosystem Result in a Neutral Annual Carbon Balance. J Arid. Environ. (2019) 162:62–73. doi: 10.1016/j.jaridenv.2018.11.013
34. Rosenstock TS, Wilkes A. Reorienting Emissions Research to Catalyse African Agricultural Development. Nat. Climate Change (2021) 11(6):463–5. doi: 10.1038/s41558-021-01055-0
35. Dijkstra J, Oenema O, van Groenigen JW, Spek JW, van Vuuren AM, Bannink A. Diet Effects on Urine Composition of Cattle and N2O Emissions. Animal (2013) 7:292–302. doi: 10.1017/S1751731113000578
36. Zhu Y, Merbold L, Leitner S, Wolf B, Pelster D, Goopy J, et al. Interactive Effects of Dung Deposited Onto Urine Patches on Greenhouse Gas Fluxes From Tropical Pastures in Kenya. Sci. Tot. Environ (2021) 761:143184. doi: 10.1016/j.scitotenv.2020.143184
37. Butterbach-Bahl K, Baggs EM, Dannenmann M, Kiese R, Zechmeister-Boltenstern S. Nitrous Oxide Emissions From Soils: How Well do We Understand the Processes and Their Controls? Philos. Trans. R. Soc. London. Ser B. Biol. Sci. (2013) 368(1621):20130122. doi: 10.1098/rstb.2013.0122
38. Cai Y, Chang SX, Cheng Y. Greenhouse Gas Emissions From Excreta Patches of Grazing Animals and Their Mitigation Strategies. Earth-Science. Rev. (2017) 171:44–57. doi: 10.1016/j.earscirev.2017.05.013
39. McSherry ME, Ritchie ME. Effects of Grazing on Grassland Soil Carbon: A Global Review. Global Change Biol. (2013) 19(5):1347–57. doi: 10.1111/gcb.12144
40. Byrnes RC, Eastburn DJ, Tate KW, Roche LM. A Global Meta-Analysis of Grazing Impacts on Soil Health Indicators. J. Environ. Qual. (2018) 47(4):758–65. doi: 10.2134/jeq2017.08.0313
41. Ritchie ME. Grazing Management, Forage Production and Soil Carbon Dynamics. Resources (2020) 9(4):49. doi: 10.3390/resources9040049
42. Oduor CO, Karanja NK, Onwonga RN, Mureithi SM, Pelster D, Nyberg G. Enhancing Soil Organic Carbon, Particulate Organic Carbon and Microbial Biomass in Semi-Arid Rangeland Using Pasture Enclosures. BMC Ecol. (2018) 18:45. doi: 10.1186/s12898-018-0202-z
43. Abegaz A, Tamene L, Abera W, Yaekob T, Hailu H, Nyawira SS, et al. Soil Organic Carbon Dynamics Along Chrono-Sequence Land-Use Systems in the Highlands of Ethiopia. Agric. Ecosyst. Environ. (2020) 300:106997. doi: 10.1016/j.agee.2020.106997
44. Chaplot V, Dlamini P, Chivenge P. Potential of grassland rehabilitation through high density-short duration grazing to sequester atmospheric carbon. Geoderma (2016) 271:10–7. doi: 10.1016/j.geoderma.2016.02.010
45. Bett BK. Using the One Health Approach to Prevent and Manage Zoonotic Diseases (2020). Available at: https://cgspace.cgiar.org/handle/10568/110312.
46. Morand S. Emerging Diseases, Livestock Expansion and Biodiversity Loss are Positively Related at Global Scale. Biol. Conserv. (2020) 248:108707. doi: 10.1016/j.biocon.2020.108707
47. Pilling D, Bélanger J, Hoffmann I. Declining Biodiversity for Food and Agriculture Needs Urgent Global Action. Nat. Food (2020) 1(3):144–7. doi: 10.1038/s43016-020-0040-y
48. Beltran I, Van der Weerden TJ, Alfaro MA, Amon B, de Klein CAM, Grace P, et al. DATAMAN: A Global Database of Nitrous Oxide and Ammonia Emission Factors for Excreta Deposited by Livestock and Land-Applied Manure. J. Environ. Qual. (2021) 50(2):513–27. doi: 10.1002/jeq2.20186
49. van der Weerden TJ, Noble A, de Klein CAM, Hutchings N, Thorman RE, Alfaro MA, et al. Ammonia and Nitrous Oxide Emission Factors for Excreta Deposited by Livestock and Land-Applied Manure. J. Environ. Qual. (2021) 50(5):1005–23. doi: 10.1002/jeq2.20259
50. IPCC. Chapter 10: Emissions From Livestock and Manure Management. In: 2019 Refinement to the 2006 IPCC Guidelines for National Greenhouse Gas Inventories (2019) Intergovernmental Panel on Climate Change.
51. Conant RT, Cerri CEP, Osborne BB, Paustian K. Grassland management impacts on soil carbon stocks: a new synthesis. Ecol Appl (2017) 27(2):662–8. doi: 10.1002/eap.1473
52. Pinares-Patiño CS, Lassey KR, Martin RJ, Molano G, Fernandez M, MacLean S, et al. Assessment of the Sulphur Hexafluoride (SF6) Tracer Technique Using Respiration Chambers for Estimation of Methane Emissions From Sheep. Anim. Feed. Sci Technol. (2011) 166–167:201–9. doi: 10.1016/j.anifeedsci.2011.04.067
53. Hammond KJ, Waghorn GC, Hegarty RS, Hammond KJ, Waghorn GC, Hegarty RS. The GreenFeed System for Measurement of Enteric Methane Emission From Cattle. Anim. Product. Sci. (2016) 56(3):181–9. doi: 10.1071/AN15631
54. IPCC. Chapter 3: Consistent Representation of Lands. In: 2006 IPCC Guidelines for National Greenhouse Gas Inventories (2006) Intergovernmental Panel on Climate Change.
55. IPCC. Chapter 11: N2O Emissions From Managed Soils, and CO2 Emissions From Lime and Urea Application. In: 2019 Refinement to the 2006 IPCC Guidelines for National Greenhouse Gas Inventories (2019) Intergovernmental Panel on Climate Change.
56. IPCC. Chapter 2: Generic Methodlogies Applicable to Multiple Land-Use Categories. In: 2019 Refinement to the 2006 IPCC Guidelines for National Greenhouse Gas Inventories (2019) Intergovernmental Panel on Climate Change.
57. Robinson T, Thornton P, Franceschini G, Kruska R, Chiozza F, Notenbaert A, et al. Global Livestock Production Systems. In: Global Livestock Production Systems (2011) Food and Agriculture Organization of the United Nations:Rome, Italy. Available at: https://www.cabdirect.org/cabdirect/abstract/20123268130.
58. Ali AIM, Wassie SE, Korir D, Merbold L, Goopy JP, Butterbach-Bahl K, et al. Supplementing Tropical Cattle for Improved Nutrient Utilization and Reduced Enteric Methane Emissions. Animals (2019) 9(5):210. doi: 10.3390/ani9050210
59. van Wyngaard JDV, Meeske R, Erasmus LJ. Effect of Concentrate Level on Enteric Methane Emissions, Production Performance, and Rumen Fermentation of Jersey Cows Grazing Kikuyu-Dominant Pasture During Summer. J Dairy. Sci. (2018) 101(11):9954–66. doi: 10.3168/jds.2017-14327
60. van Wyngaard JDV, Meeske R, Erasmus LJ. Effect of Dietary Nitrate on Enteric Methane Emissions, Production Performance and Rumen Fermentation of Dairy Cows Grazing Kikuyu-Dominant Pasture During Summer. Anim. Feed. Sci. Technol. (2018) 244:76–87. doi: 10.1016/j.anifeedsci.2018.08.005
61. Patra AK. Recent Advances in Measurement and Dietary Mitigation of Enteric Methane Emissions in Ruminants. Front Veterinary Sci (2016) 3:39. doi: 10.3389/fvets.2016.00039
62. Adejoro FA, Hassen A, Akanmu AM. Effect of Lipid-Encapsulated Acacia Tannin Extract on Feed Intake, Nutrient Digestibility and Methane Emission in Sheep. Animals (2019) 9(11):863. doi: 10.3390/ani9110863
63. Butterbach-Bahl K, Gettel G, Kiese R, Fuchs K, Werner C, Rahimi J, et al. Livestock Enclosures in Drylands of Sub-Saharan Africa are Overlooked Hotspots of N 2 O Emissions. Nat. Commun. (2020) 11(1):4644. doi: 10.1038/s41467-020-18359-y
64. Gilbert M, Nicolas G, Cinardi G, Van Boeckel TP, Vanwambeke SO, Wint GRW, et al. Global Distribution Data for Cattle, Buffaloes, Horses, Sheep, Goats, Pigs, Chickens and Ducks in 2010. Sci. Data (2018) 5(1):180227. doi: 10.1038/sdata.2018.227
65. Ogutu JO, Piepho H-P, Said MY, Ojwang GO, Njino LW, Kifugo SC, et al. Extreme Wildlife Declines and Concurrent Increase in Livestock Numbers in Kenya: What Are the Causes? PLoS One (2016) 11(9):e0163249. doi: 10.1371/journal.pone.0163249
66. Rufino MC, Brandt P, Herrero M, Butterbach-Bahl K. Reducing Uncertainty in Nitrogen Budgets for African Livestock Systems. Environ. Res. Lett. (2014) 9(10):105008. doi: 10.1088/1748-9326/9/10/105008
67. O’Kelly JC, Spiers WG. Effect of Monensin on Methane and Heat Productions of Steers Fed Lucerne Hay Either Ad Libitum or at the Rate of 250 G/Hour. Aust. J. Agric. Res. (1992) 43(8):1789–93. doi: 10.1071/ar9921789
68. Pinares-Patiño C, Gere J, Williams K, Gratton R, Juliarena P, Molano G, et al. Extending the Collection Duration of Breath Samples for Enteric Methane Emission Estimation Using the SF6 Tracer Technique. Animals (2012) 2(2):275–87. doi: 10.3390/ani2020275
69. Sorg D. Measuring Livestock CH4 Emissions With the Laser Methane Detector: A Review. Methane (2022) 1(1):38–57. doi: 10.3390/methane1010004
70. du Toit CJL, Niekerk WA, Meissner HH, Erasmus LJ, Coertze RJ, du CJL, et al. Methane emissions from sheep fed Eragrostis curvula hay substituted with Lespedeza cuneata. Anim Production Sci (2020) 60(15):1777–84. doi: 10.1071/AN19257
71. Ndung’u PW, Takahashi T, du Toit CJL, Robertson-Dean M, Butterbach-Bahl K, McAuliffe GA, et al. Farm-Level Emission Intensities of Smallholder Cattle (Bos Indicus; B. Indicus–B. Taurus Crosses) Production Systems in Highlands and Semi-Arid Regions. Animal (2022) 16(1):100445. doi: 10.1016/j.animal.2021.100445
72. Wilkes A, Wassie S, Odhong’ C, Fraval S, van Dijk S. Variation in the Carbon Footprint of Milk Production on Smallholder Dairy Farms in Central Kenya. J. Cleaner. Product. (2020) 265:121780. doi: 10.1016/j.jclepro.2020.121780
73. Government of Kenya, Ministry of Agriculture, Livestock, Fisheries and Cooperative: State Department of Livestock. Inventory of GHG Emissions From Dairy Cattle in Kenya 1995-2017 (2020). Available at: https://www.ccacoalition.org/en/resources/inventory-ghg-emissions-dairy-cattle-kenya-1995-2017.
74. Wilkes A, Wassie SE, Tadesse M, Assefa B, Abu M, Ketema A, et al. Inventory of Greenhouse Gas Emissions From Cattle, Sheep and Goats in Ethiopia, (1994-2018) Calculated Using the IPCC Tier 2 Approach. Environment and Climate Change Directorate of the Ministry of Agriculture. (2020).(Addis Ababa, Ethiopia:CGIAR Research Program on Climate Change, Agriculture and Food Security (CCAFS)) .Available at: https://cgspace.cgiar.org/handle/10568/110982.
75. Onyango AA, Dickhoefer U, Rufino MC, Butterbach-Bahl K, Goopy JP. Temporal and Spatial Variability in the Nutritive Value of Pasture Vegetation and Supplement Feedstuffs for Domestic Ruminants in Western Kenya. Asian-Australasian. J Anim Sci (2018) 32(5):637–47. doi: 10.5713/ajas.18.0114
76. Hammond J, Fraval S, van Etten J, Suchini JG, Mercado L, Pagella T, et al. The Rural Household Multi-Indicator Survey (RHoMIS) for Rapid Characterisation of Households to Inform Climate Smart Agriculture Interventions: Description and Applications in East Africa and Central America. Agric Syst (2017) 151:225–33. doi: 10.1016/j.agsy.2016.05.003
77. Sorg D, Difford GF, Mühlbach S, Kuhla B, Swalve HH, Lassen J, et al. Comparison of a laser methane detector with the GreenFeed and two breath analysers for on-farm measurements of methane emissions from dairy cows. Comput Electron Agric (2018) 153:285–94. doi: 10.1016/j.compag.2018.08.024
78. Kurihara M, Magner T, Hunter RA, McCrabb GJ. Methane Production and Energy Partition of Cattle in the Tropics. Br. J. Nutr (1999) 81(3):227–34. doi: 10.1017/S0007114599000422
79. Appuhamy J. A. D. R. N., France J, Kebreab E. Models for Predicting Enteric Methane Emissions From Dairy Cows in North America, Europe, and Australia and New Zealand. Global Change Biol. (2016) 22(9):3039–56. doi: 10.1111/gcb.13339
80. Hellwing ALF, Weisbjerg MR, Brask M, Alstrup L, Johansen M, Hymøller L, et al. Prediction of the Methane Conversion Factor (Ym) for Dairy Cows on the Basis of National Farm Data. Anim. Product. Sci. (2016) 56(3):535–40. doi: 10.1071/AN15520
81. Jayasundara S, Ranga Niroshan Appuhamy JAD, Kebreab E, Wagner-Riddle C. Methane and Nitrous Oxide Emissions From Canadian Dairy Farms and Mitigation Options: An Updated Review. Can. J. Anim. Sci. (2016) 96(3):306–31. doi: 10.1139/cjas-2015-0111
82. Niu M, Kebreab E, Hristov AN, Oh J, Arndt C, Bannink A, et al. Prediction of Enteric Methane Production, Yield, and Intensity in Dairy Cattle Using an Intercontinental Database. Global Change Biol. (2018) 24(8):3368–89. doi: 10.1111/gcb.14094
83. Kouazounde JB, Gbenou JD, Babatounde S, Srivastava N, Eggleston SH, Antwi C, et al. Development of Methane Emission Factors for Enteric Fermentation in Cattle From Benin Using IPCC Tier 2 Methodology. Animal (2015) 9(3):526–33. doi: 10.1017/S1751731114002626
84. Ndao Séga, Moulin C-H, Traoré EH, Diop M, Bocquier F. Contextualized Re-Calculation of Enteric Methane Emission Factors for Small Ruminants in Sub-Humid Western Africa is Far Lower Than Previous Estimates. Trop. Anim. Health Product. (2019) 51(4):919–28. doi: 10.1007/s11250-018-1775-4
85. Ndao S, Traoré EH, Ickowicz A, Moulin C-H. Estimation of Enteric Methane Emission Factors for Ndama Cattle in the Sudanian Zone of Senegal. Trop. Anim. Health Product. (2020) 52(6):2883–95. doi: 10.1007/s11250-020-02280-z
86. Goopy JP, Pelster DE, Onyango A, Marshall K, Lukuyu M, Goopy JP, et al. Simple and Robust Algorithms to Estimate Liveweight in African Smallholder Cattle. Anim. Product. Sci (2017) 58(9):1758–65. doi: 10.1071/AN16577
87. FAO, New Zealand Agricultural Greenhouse Gas Research Centre. Options for Low Emission Development in the Kenya Dairy Sector: Reducing Enteric Methane for Food Security and Livelihoods (2017). Available at: https://ccacoalition.org/en/resources/options-low-emission-development-kenya-dairy-sector-reducing-enteric-methane-food-security.
88. Balehegn M, Kebreab E, Tolera A, Hunt S, Erickson P, Crane TA, et al. Livestock Sustainability Research in Africa With a Focus on the Environment. Anim. Front. (2021) 11(4):47–56. doi: 10.1093/af/vfab034
89. Zhu Y, Merbold L, Pelster D, Diaz-Pines E, Wanyama GN, Butterbach-Bahl K. Effect of Dung Quantity and Quality on Greenhouse Gas Fluxes From Tropical Pastures in Kenya. Global Biogeochem. Cycle. (2018) 32(10):1589–604. doi: 10.1029/2018GB005949
90. Zhu Y, Merbold L, Leitner S, Xia L, Pelster DE, Diaz-Pines E, et al. Influence of Soil Properties on N2O and CO2 Emissions From Excreta Deposited on Tropical Pastures in Kenya. Soil Biol. Biochem. (2020) 140:107636. doi: 10.1016/j.soilbio.2019.107636
91. Rufino MC, Rowe EC, Delve RJ, Giller KE. Nitrogen Cycling Efficiencies Through Resource-Poor African Crop–Livestock Systems. Agri. Ecosyst. Environ. (2006) 112(4):261–82. doi: 10.1016/j.agee.2005.08.028
92. Chadwick D, Sommer S, Thorman R, Fangueiro D, Cardenas L, Amon B, et al. Manure Management: Implications for Greenhouse Gas Emissions. Anim. Feed. Sci. Technol. (2011) 166–167:514–31. doi: 10.1016/j.anifeedsci.2011.04.036
93. Petersen SO, Blanchard M, Chadwick D, Del Prado A, Edouard N, Mosquera J, et al. Manure Management for Greenhouse Gas Mitigation. Animal (2013) 7:266–82. doi: 10.1017/S1751731113000736
94. Jarvis SC, Lovell RD, Panayides R. Patterns of Methane Emission From Excreta of Grazing Animals. Soil Biol. Biochem. (1995) 27(12):1581–8. doi: 10.1016/0038-0717(95)00092-S
95. Holter P. Methane Emissions From Danish Cattle Dung Pats in the Field. Soil Biol.Biochem. (1997) 29(1):31–7. doi: 10.1016/S0038-0717(96)00267-2
96. Mori A, Hojito M. Methane and Nitrous Oxide Emissions Due to Excreta Returns From Grazing Cattle in Nasu, Japan. Grassland. Sci (2015) 61(2):109–20. doi: 10.1111/grs.12081
97. Ndambi OA, Pelster DE, Owino JO, de Buisonjé F, Vellinga T. Manure Management Practices and Policies in Sub-Saharan Africa: Implications on Manure Quality as a Fertilizer. Front Sustain. Food Syst (2019) 3:29. doi: 10.3389/fsufs.2019.00029
98. Carbonell V, Merbold L, Díaz-Pinés E, Dowling TPF, Butterbach-Bahl K. Nitrogen Cycling in Pastoral Livestock Systems in Sub-Saharan Africa: Knowns and Unknowns. Ecol. Applicat. (2021). doi: 10.1002/eap.2368
99. Summerauer L, Baumann P, Ramirez-Lopez L, Barthel M, Bauters M, Bukombe B, et al. The Central African Soil Spectral Library: A New Soil Infrared Repository and a Geographical Prediction Analysis. SOIL (2021) 7(2):693–715. doi: 10.5194/soil-7-693-2021
100. Dabasso BH, Taddese Z, Hoag D. Carbon Stocks in Semi-Arid Pastoral Ecosystems of Northern Kenya. Pastoralism (2014) 4(1):5. doi: 10.1186/2041-7136-4-5
101. Henderson BB, Gerber PJ, Hilinski TE, Falcucci A, Ojima DS, Salvatore M, et al. Greenhouse Gas Mitigation Potential of the World’s Grazing Lands: Modeling Soil Carbon and Nitrogen Fluxes of Mitigation Practices. Agri. Ecosyst. Environ. (2015) 207:91–100. doi: 10.1016/j.agee.2015.03.029
102. Minasny B, Malone BP, McBratney AB, Angers DA, Arrouays D, Chambers A, et al. Soil Carbon 4 Per Mille. Geoderma (2017) 292:59–86. doi: 10.1016/j.geoderma.2017.01.002
103. Leenaars JGB, Van Oostrum AJM, Gonzalez MR. Africa Soil Profiles Database, Version 1.2. A Compilation of Georeferenced and Standardised Legacy Soil Profile Data for Sub-Saharan Africa (With Dataset) (2014). Available at: https://research.wur.nl/en/publications/africa-soil-profiles-database-version-12-a-compilation-of-georefe.
104. Hengl T, Heuvelink GBM, Kempen B, Leenaars JGB, Walsh MG, Shepherd KD, et al. Mapping Soil Properties of Africa at 250 M Resolution: Random Forests Significantly Improve Current Predictions. PLoS One (2015) 10(6):e0125814. doi: 10.1371/journal.pone.0125814
105. Evans M, Jones CS, Duncan AJ. The sub-Saharan Africa feeds composition database (2021). Available at: https://cgspace.cgiar.org/handle/10568/118004.
106. Wuest SB. Correction of Bulk Density and Sampling Method Biases Using Soil Mass Per Unit Area. Soil Sci. Soc. America J. (2009) 73(1):312–6. doi: 10.2136/sssaj2008.0063
107. Wendt JW, Hauser S. An Equivalent Soil Mass Procedure for Monitoring Soil Organic Carbon in Multiple Soil Layers. Eur. J. Soil Sci. (2013) 64(1):58–65. doi: 10.1111/ejss.12002
108. von Haden AC, Yang WH, DeLucia EH. Soils’ Dirty Little Secret: Depth-Based Comparisons can be Inadequate for Quantifying Changes in Soil Organic Carbon and Other Mineral Soil Properties. Global Change Biol. (2020) 26(7):3759–70. doi: 10.1111/gcb.15124
109. Adenle AA, Manning DT, Arbiol J. Mitigating Climate Change in Africa: Barriers to Financing Low-Carbon Development. World Dev. (2017) 100:123–32. doi: 10.1016/j.worlddev.2017.07.033
110. North MA, Hastie WW, Hoyer L. Out of Africa: The Underrepresentation of African Authors in High-Impact Geoscience Literature. Earth-Science. Rev. (2020) 208:103262. doi: 10.1016/j.earscirev.2020.103262
111. Costa C, Seabauer M, Schwarz B, Dittmer K, Wollenberg E. Scaling Soil Organic Carbon Sequestration for Climate Change Mitigation (2020) (Wageningen, the Netherlands:CGIAR Research Program on Climate Change, Agriculture and Food Security (CCAFS)). Available at: https://cgspace.cgiar.org/handle/10568/114846.
112. Cramer L. Report From Kenya’s Climate Smart Agriculture Multi-Stakeholder Platform (CSA MSP) Livestock Learning Platform Meeting.CCAFS Report (2021) Wageningen, the Netherlands: CGIAR Research Program on Climate Change, Agriculture and Food Security (CCAFS). Available at: https://cgspace.cgiar.org/handle/10568/113685.
Keywords: livestock, manure, enteric, soil carbon, Africa, greenhouse gas emissions (CH4 N2O CO2)
Citation: Graham MW, Butterbach-Bahl K, du Toit CJL, Korir D, Leitner S, Merbold L, Mwape A, Ndung’u PW, Pelster DE, Rufino MC, van der Weerden T, Wilkes A and Arndt C (2022) Research Progress on Greenhouse Gas Emissions From Livestock in Sub-Saharan Africa Falls Short of National Inventory Ambitions. Front. Soil Sci. 2:927452. doi: 10.3389/fsoil.2022.927452
Received: 24 April 2022; Accepted: 14 June 2022;
Published: 29 August 2022.
Edited by:
Derrick Y.F. Lai, The Chinese University of Hong Kong, ChinaReviewed by:
Eduardo Vázquez, University of Bayreuth, GermanyCopyright © 2022 Graham, Butterbach-Bahl, du Toit, Korir, Leitner, Merbold, Mwape, Ndung’u, Pelster, Rufino, van der Weerden, Wilkes and Arndt. This is an open-access article distributed under the terms of the Creative Commons Attribution License (CC BY). The use, distribution or reproduction in other forums is permitted, provided the original author(s) and the copyright owner(s) are credited and that the original publication in this journal is cited, in accordance with accepted academic practice. No use, distribution or reproduction is permitted which does not comply with these terms.
*Correspondence: Michael W. Graham, bS5ncmFoYW1AY2dpYXIub3Jn
Disclaimer: All claims expressed in this article are solely those of the authors and do not necessarily represent those of their affiliated organizations, or those of the publisher, the editors and the reviewers. Any product that may be evaluated in this article or claim that may be made by its manufacturer is not guaranteed or endorsed by the publisher.
Research integrity at Frontiers
Learn more about the work of our research integrity team to safeguard the quality of each article we publish.