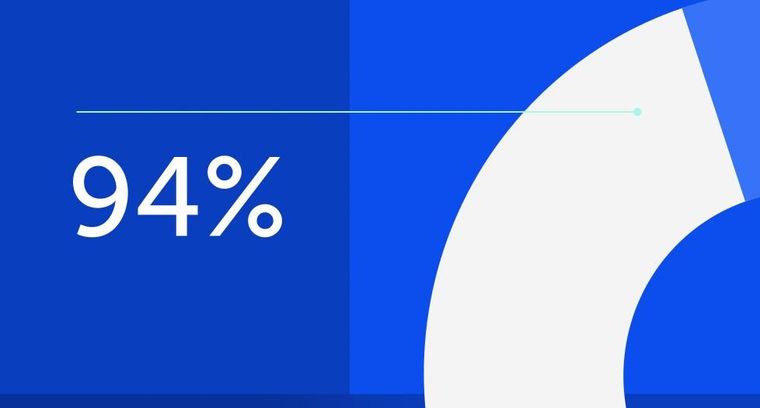
94% of researchers rate our articles as excellent or good
Learn more about the work of our research integrity team to safeguard the quality of each article we publish.
Find out more
ORIGINAL RESEARCH article
Front. Soil Sci., 21 March 2022
Sec. Soil Organic Matter Dynamics and Carbon Sequestration
Volume 2 - 2022 | https://doi.org/10.3389/fsoil.2022.831775
Irrigation is in the spotlight of land-use planning in semi-arid and sub-humid regions. It can be a promising practice to promote soil organic C storage (SOC), although it may also involve an increase in soil GHG emissions. Assessing the impact of its adoption on SOC storage is crucial to better understand its potential role in terms of agricultural sustainability and climate policies. In this study, we measured and modeled the changes in soil organic C storage and dynamics in the tilled soil layer (0–30 cm) of an experimental field on a calcareous soil with two different crops (maize, a C4 plant, and wheat, a C3 plant), cultivated with and without irrigation for 7 years. We hypothesized that changes in SOC storage occur when introducing irrigation and/or different crops in an agrosystem, and that they would be related to changes in the incorporation of crop residues, their partitioning between the labile and the stable fraction, and C losses by mineralization. Our results validated theses hypotheses only partially. Over the 7-year study period, irrigation significantly increased total (TOC) and sand-size (50–2,000 μm) particulate organic C (POC50−2,000) stocks in the tilled layer (0–30 cm): +7.1% TOC and +12.1% POC50−2,000 for maize, and +7.0 and +12.3% for wheat. A parallel two-pool SOC model based on TOC and POC50−2,000 fractions and the C3-C4 plant shift allowed understanding that the observed changes in SOC storage were most likely related to an increase in C inputs from crop residues, and to a more efficient incorporation of these residues with irrigation. The mean residence time of SOC in the two modeled pools did not allow to support our hypothesis of changes in SOC mineralization with irrigation. The limitations of SOC fractionation, which implied that some labile fractions might have been lost from POC50−2,000 and recovered in the fraction identified as slow-turning, together with the interaction of the carbonate-rich mineral phase of this soil can explain at least partially this observation. We conclude that irrigation can contribute to effectively increase SOC storage in the mid-term, but its effect might be dependent upon the type of crops and soil.
Irrigation is a traditional strategy to expand and sustain agriculture in semi-arid and sub-humid regions (1). It allows to increase yields and provides farmers with wider crop farming options and more flexible planting dates (2). It can also be seen as a tool for adapting to climate variability (3, 4), although its sustainability in some regions has been questioned (5, 6).
Assessing the impact of the adoption of irrigation on soil quality and functioning, and in particular on organic matter storage, seems necessary to better understand its benefits and drawbacks in terms of agricultural sustainability and climate policies. In terms of soil organic C (SOC) storage, irrigation may have positive effects in arid and semi-arid areas (7, 8). It is, for instance, one of the measures considered within those proposed in the “4 per 1,000” initiative framework (9), which aims to address the global challenge of securing food for a growing population, while ensuring climate change adaptation and mitigation (10, 11). However, it is also known that irrigation can also increase GHG emissions compared to rainfed management, especially of those GHG associated with soil (12, 13). For Virto et al. (14), the net effect on C balance may be modulated not only by soil characteristics but also by soil management, since different agricultural strategies on irrigated systems can had different potential to sequester SOC and/or reduce GHG emissions.
In this context, assessing the net effect of change from rainfed to irrigated agriculture on SOC is crucial. This effect has indeed not been clearly demonstrated. For example, only 8 out of 14 long-term field experiments reviewed by Trost et al. (15) across different climate zones showed higher SOC contents in irrigated plots compared with rainfed plots. These eight experiments were all located in arid or desert regions, in contrast with the other six experiments from wetter regions, which displayed no significant differences. As SOC content depends mostly on C input from plants and decomposition processes, these results were explained by a greater irrigated-induced increase in primary productivity in dry areas compared with wetter regions, while SOC decomposition rates were assumed to be similar at all sites (10). Nevertheless, an increase in decomposition rates may be associated with changes in the water regime of the soil, which can favor the soil microbial activity (16). Accordingly, Aguilera et al. (17) showed that in the Mediterranean region, both soil C inputs and SOC decomposition rates increased with irrigation, obtaining inconsistent results in terms of the net effects on SOC storage. Similar results were observed by Zhou et al. (18) in a global meta-analysis.
In summary, the variations induced by irrigation in the soil C cycle can be expected to be different depending on the effects of the new water and soil management practices on soil C inputs, and C stabilization mechanisms (15, 18, 19). The role of these aspects as drivers of SOC dynamics is widely accepted (20, 21), as well as the critical interaction of the soil physical-chemical characteristics and the nature of the mineral phase of the soil with them (22, 23). In this sense, the protective nature of carbonate-rich soil matrixes could play a significant role in irrigated calcareous soils. In addition to the well-known higher stability of organic forms in Ca-rich soils compared to other soils in similar conditions (24, 25), carbonates can be sensitive to both moisture changes and acidification by increased roots respiration (26, 27), which should be accounted for when assessing the environmental and agronomic impacts of irrigation adoption in calcareous soils (28). A need for site-specific research to correctly assess this effect in the long term is therefore needed (13, 17, 29, 30).
Despite this evidence, there is still little information available at the regional scale to conduct a detailed analysis of the effect of irrigation adoption on SOC dynamics (10), notably because of the lack of paired irrigated and non-irrigated trials (31). The assessment of SOC changes associated with a land-use change such as a change in crop and/or irrigation regime in a given area requires some considerations. An important one is whether the change of use is likely to imply a transition period in which SOC stocks are not at steady state. For example, in a regional study conducted in Navarre (NE Spain), a positive effect of irrigation in terms of SOC storage was observed (32), but with variable annual rates depending on crops, climate and/or soil type (33). Understanding the mechanisms of SOC dynamics during this transition period remains a major research question (10).
Different approaches have been developed to assess the potential changes in SOC storage and dynamics in agricultural soils. Isotopic studies combined with the monitoring of field-scale parameters represent a powerful method for this purpose, and are frequently used in SOC modeling (34–36). In particular, the 13C natural abundance approach represents a widespread option for temporal and spatial modeling of SOC, with great applicability to evaluate SOC turnover (37–40).
This approach, when used in chronological studies, allows for testing models aiming to estimate the turnover, or the mean residence time (MRT) of SOC, by simulating the processes driving SOC dynamics, the balance between the soil C inputs and outputs, and the influence of land use and management on SOC dynamics (10, 41, 42). They can have a variable degree of complexity (43). In this sense, the decomposition of C inputs in the soil and their progressive incorporation into the soil organic matter pool represent an extremely complex process (21), to which, at least, two-pools models may give a closer representation over the first few years, offering good fits to this decay process (44). In these two-pools models, a fast- and a slow-cycling pool are considered, according to their biological stability toward decomposition (45, 46) and, therefore, representing SOC pools with contrasting turnover rates. For example, Derrien and Amelung (39) studied how different structures of simple models of SOC dynamics, representing different types of SOC incorporation and stabilization dynamics, could affect the MRT of SOC. They concluded that identifying the best choice of model structure is essential to get as close to the natural system evaluated with a minimal error as possible. These authors studied different mechanistic processes controlling SOC stabilization, from the simplest one with one single SOC pool, to different combinations of two successive or two parallel SOC pools.
In this context, when different pools are considered in the model, a well-defined SOC fractionation protocol is required to separate and analyze fractions corresponding to these modeled pools (47). Indeed, in many cases, the convergence of the conceptual pools defined in models with their corresponding fractions of SOC can be considered the real challenge in their parametrization (48–50). As such, Six et al. (51) already observed that the isolation of functional pools of soil organic matter sensitive to land use changes can be elusive.
Poeplau et al. (52), in a study comparing fractionation methods to recover fractions with varying turnover rates, concluded that none of the 20 methods tested was able to properly isolate the most labile fractions representing the fast-cycling labile C pools described in biogeochemical models. In their study, the fraction most enriched in labile C recently arrived into the soil, was the coarse (sand size) light organic fraction or particulate organic matter. In fact, the organic C in the size fraction 50–2,000 μm has been often considered as functionally representing the fast cycling /labile organic C pool (53–55). The isolation of such fraction can however be elusive, and its effectiveness depends upon soil characteristics (56).
Carbon inputs from crop residues are also a critical parameter in these models (39), as observed by Keel et al. (57), who reported large uncertainties in the simulated changes of SOC by applying different approaches to estimate C inputs with the same decomposition model. In addition, it is known that the proportion of these residues which actually enters the soil at a given depth may depend on the physical distribution and fate of crop residues between harvesting and incorporation with tillage (58), and that changes in moisture conditions in the soil can affect the proportion of C actually incorporated per unit C in crop residues (59).
In this study, we aimed to investigate the changes in SOC storage and dynamics in a sub-humid calcareous Mediterranean soil 7 years after the introduction of sprinkler irrigation. For this purpose, a C3-to-C4 plant-shift experiment was set up in Enériz (Navarra, Spain) with two of the most widespread crops in the region, wheat and maize, taking advantage of the introduction of irrigation in an area where both crops can complete their growing season with and without irrigation.
We hypothesized that (i) irrigation and the crop conversion from wheat (a C3 plant) to maize (a C4 plant, with higher biomass production than wheat) would lead to changes in SOC storage, and (ii) that these changes would be related to changes in the incorporation of crop residues, their partitioning between the labile and the stable fraction, and C losses by mineralization.
The objectives of this work were (i) to study the relationship between the introduction of irrigation and its consequences in terms of C storage in both crops, and (ii) to model the process of SOC stabilization in these agroclimatic conditions, in order to (ii.1) define a basic mechanistic model that can explain the changes associated to the implementation of irrigation, and (ii.2) to understand how the introduction of irrigation can induce changes in this process.
The study field is located in Navarre, NE Spain (42°40′18.74″N; 1° 45′ 4.65″W) within a sub-humid temperate Mediterranean climate area. The mean annual temperature is 13.4°C, and mean annual rainfall and potential evapotranspiration (PET) are 565 and 722 mm, respectively. The average monthly distributions of PET and rainfall are shown in Figure 1. In this field, rainfed maize is in its geographical limit for profitable standard production, but can still be grown.
Figure 1. Monthly average rainfall (bars) and potential evapotranspiration (PET, solid line) data at the experimental site. Source data series: 1925–2018 (60).
The soil is a Calcic Haploxerept (61) or Calcic Cambisol (62), developed on carbonate-rich siltstones and sandstones. It is a fine-loamy deep soil, with 38% carbonates by mass (pH = 8.2), and average clay content of 29% in the upper horizon (0–30 cm).
This field was managed for at least five decades as a traditional rainfed dryland field under conventionally tilled (chisel plow) and cultivated with C3 crops [mostly wheat (Triticum aestivum L.) and barley (Hordeum vulgare L.)] before the area was converted to irrigated farmland. At that moment, in 2010, and taking advantage of the introduction of irrigation, a C3-to-C4 plant shift experiment was set up with the aim of long-term monitoring the effect of climate manipulation with irrigation under different crop systems. Winter wheat (T. aestivum L.) and maize (Zea mays L.), a C3 and C4 plant, respectively, corresponding to the two most widespread extensive crops in the region, and differing in their primary productivity, were selected for the experiment, and grown for 7 years with and without irrigation. This allows for long-term monitoring of different crop and soil parameters in both crops, while keeping a C3 control under irrigation for modeling purposes.
The experimental design was conceived to monitor four combinations of crops and irrigation regime: rainfed wheat (W-rf), irrigated wheat (W-irr), rainfed maize (M-rf), and irrigated maize (M-irr).
To that end, the field was split into two large areas of 2,600 m2, one with irrigation and the other without, given the impossibility of varying the level of irrigation and of controlling belowground water transfers on a small scale, and the differences in irrigation regime in both crops,. For these same reason, each area was then divided into two large subareas devoted to winter wheat and maize, respectively, and separated by a buffer due to the different irrigation and management schedules of wheat and maize. This design ensured an adequate distribution of irrigation in time and space for both crops. Winter wheat and maize were grown for the 7 years of the experiment.
Within each sub-area, a grid of 6 plots (6 × 10 m) separated by 4 m wide corridors was stablished for soil sampling. This allowed for a systematic sampling to ensure complete coverage of the field for good representativeness, and to avoid contour effects of small sampling plots, in each subarea with W-rf, W-irr, M-rf, and M-irr. This sampling strategy was used first for verification of the homogeneity of soil properties known to affect crop yields and SOC stabilization among the four subareas [namely texture (58), carbonates content and available water-holding capacity, AWHC, Supplementary Tables 1, 2]. Then, the soil in each of the 6 plots in the grid per crop-irrigation combination subarea was sampled every year except for year 5, after the incorporation of the crop residues biomass into the soil through tillage (in early fall for wheat and early spring for maize). Each year the sampling date was precisely determined by computing thermal integrals for each crop, so that the number of degree-days accumulated between residues incorporation and sampling was equivalent for both crops (data not shown).
In all cases, undisturbed (100 cm3 soil cores for bulk density) and disturbed composite samples were collected at 0–30 cm. For disturbed samples, five sub-samples were collected at random per plot, avoiding the perimeter first meter, and gently pooled to obtain one composite sample, and air-dried.
Sprinkler irrigation was used in this field for W-irr and M-irr. Because of the local distribution of rainfall (Figure 1), and the cycle and water demands of both crops, the irrigation schedule and amounts was different for W-irr and M-irr. Irrigation in W-irr was used in March, April, May and June, and it was continuous from June to September in M-irr. The average water supply by rainfall within the growing season each year, for the seven years of the experiment in wheat was of 182 ± 70 L m−2, and the average total water supply in the growing season per year, complemented by irrigation, in W-irr was of 281 ± 66 L m−2. For maize, the average water supply by rainfall within the growing season for the years of the experiment was of 143 ± 73 L m−2, and it was complemented with irrigation in M-irr to get an average amount of 709 ± 99 L m−2 in the growing season (63).
Crops and soils management were conducted following the local conventional practices for both crops (Table 1). Crop residues incorporation was done in early fall for wheat, and early spring for maize, through inversion tillage (0–30 cm). For both crops, the average dose of fertilizers and herbicides used in the area was applied (Table 1).
Crop yields were controlled during the seven growing seasons of the study by harvesting each individual plot with a Haldrup C-85 mechanical harvester (Haldrup, Denmark). Before harvesting, aboveground plant biomass (AGB) was quantified by counting the number of plants in 2 linear meters and collecting and dry-weighing 5 of these plants for maize. For wheat controls, before harvesting, plants were collected and dry-weighed within a sampling frame of 0.3 m2 in each plot (6 plots per subarea). From these plants, the harvest index (HI) was determined as the ratio of grain yield and total AGB. The total amount of aboveground crop residues potentially incorporated into the soil was then determined for each plot using HI and the measured grain yield at harvest. Belowground biomass was estimated using empirical allometric relations given by IPCC (64), as a percentage of AGB (24% in wheat, 22% in maize). The C concentration in each crop was analyzed (see following section), and used to convert AGB and belowground biomass into organic C data, as an expression of crop residues-C potentially incorporated into the soil. Accumulated crop residues-C within the 7 years of the study where accounted from annual crop residues-C estimations.
Prior to analyses, air-dried disturbed soil samples were sieved at 2 mm in a device allowing for complete disaggregation of large clods and fragments of organic debris, to ensure the highest possible recovery of aggregates and particles <2 mm, and thoroughly homogenized. Part of the fresh maize and wheat samples (leaves, stalks and cobs in maize) collected were dried at 105°C, finely ground and homogenized.
At year 0, standard methods were used to determine the soil particle-size distribution (65). Carbonates were analyzed using a calcimeter after complete reaction of a finely ground sample with HCl 1M (66). Also, AWHC was determined as the difference in water retained at−33 and −1,500 KPa in a pressure plate extractor (67) from disturbed and undisturbed samples, respectively.
Every sampling year, total organic C (TOC) was determined in soil samples by wet oxidation (Walkley-Black method) following the protocol given in Tiessen and Noir (68) due to the elevated carbonate content (69). The organic C in the particulate size fraction 50–2,000 μm (POC50−2,000) was isolated by the method described in Virto et al. (70) where 10 g of air-dry soil (<2 mm) were dispersed by shaking overnight in 150 mL of a 5% (NaPO3)6 solution (pH = 6.13), and sieved to collect the fraction > 50 μm (71). This fraction was chosen with the aim of keeping the soil organic matter model simple (two pools), considering it as functionally representing the fast cycling/labile organic C pool (53–55). Although mechanical dispersion methods such as shaking with glass beads have been reported to be more adequate for the isolation of this fraction, they seem not to be adequate in clay and carbonate-rich soils (56). The use of a (NaPO3)6 solution of pH 6.13 was therefore preferred, and the risk of organic C loss from this fraction by dissolution was minimized by reducing rinsing as much as possible. Organic C concentration in this fraction was measured by wet oxidation after drying and grinding the fraction to a powdery consistency (68).
TOC and POC50−2,000 stocks in the tilled layer were calculated for W-rf, W-irr, M-rf and M-irr using concentrations, and bulk density data for an equivalent dry soil mass (72) of 4,771 kg m−2, corresponding to the soil mass of the lowest recorded average bulk density in the tilled depth (0–30 cm) across plots and years, which was recorded in M-rf at year 7, to avoid corrections based in unknown bulk density and SOC concentrations beyond this depth (73, 74).
Three plots out of 6 were randomly chosen to analyze the natural abundance of 13C in TOC and POC50−2,000 samples, as well as in maize and wheat vegetal tissues. These analysis were performed on a CN elemental analyzer (NC 2500, Carlo Erba, Milano, Italy) coupled to a mass spectrometer (Thermo Quest-Finningan Delta Plus, Bremen, Germany). Acid fumigation (75), which was reported by previous studies to not affect the organic C concentration or isotopic signature (24), was used before analysis to remove soil carbonates.
For modeling purposes, the proportion of maize-C at time t in the chronological study (F(t)) in both TOC and POC50−2,000 was quantified in M-rf and M-irr samples considering that the isotope composition of SOC after the introduction of maize (δsample) can be expressed as (35):
where Cold and Cnew are the soil C contents from the old and the new crops (former C3 vegetation and maize, respectively), δsample is the δ13C of the soil studied sample, δold is the δ13C of the original SOC (t = 0) and δnew is the average δ13C of new maize plant material (−12.7 ± 0.1 ‰ in M-irr, and −13.5 ± 0.2‰ in M-rf).
F(t), the proportion of C derived from the new C4 crop is calculated as:
Values of F(t) were calculated for each plot independently, for TOC and POC50−2,000, in relation to the average value of δ13C in the W-rf plots (δold = −26.6 ± 1.2‰) which is considered to be representative of previous soil condition (76). For verification, δ13C from TOC and POC50−2,000 in W-rf and W-irr samples was measured at years 0, 2 and 7, and no significant differences were observed (data not shown). The amount of C4-C stored in any fraction and moment in time (C4-C stock) can be calculated as the product of C in that fraction and F(t). Thus, this amount for TOC (C4-TOC) and POC50−2,000 (C4-POC50−2,000) was calculated for the 7 years of study, except for year 5, in the M-rf and M-irr.
The changes in TOC and POC50−2,000 over time were analyzed, using the nlme package of R (77). Given that we were dealing with a longitudinal study design, the assumption of independence of TOC and POC50−2,000 data from the same plot in time typically does not hold. In addition, considering the characteristics of the experimental design, data of TOC and POC50−2,000 were considered embedded in a hierarchical structure, so that different plots are nested within each subarea, while the observations over time constitute a cross effect. This structure creates additional dependences for data from the same subarea, and for data from the same year. In order to take these dependences into account when analyzing crop, irrigation and year effects, different linear mixed models (LMMs) with fixed and random effects were considered. These included a mean structure based on crop, irrigation and year effects, and their (potential) interactions to analyze year-specific or non-specific crop and irrigation effects. The LMM incorporated the possibility of a subarea-specific, plot-specific, and year-specific intercepts, which allows the LMM to explain the existing correlation in the measurements. The sources of dependence generated by the hierarchical structure were incorporated into the LMM through a random effect that explains the dependence of the measurements of the same subarea, and another random effect nested in the previous one, that explains the dependence of the measurements of the same plot over the years.
The residual errors of the model conditionally maintained the classical assumption of independence (78), an assumption that was checked with a subsequent analysis (see below). We also analyzed the residual error variances, confirming the existence of statistically significant heteroscedasticity as a year-specific variance. Thus, the LMM equation for TOC measurements was the following:
Where TOCijt is the value of TOC measured for unit(plot) i (i = 1,…,24), which belongs to subarea j (j = 1,…,4), at year t (t = 1, 2, 3, 4, 6, and 7) with β0 denoting the intercept, β1 the year continuous effect, β2t the year-specific crop effect and β3 the constant irrigation effect. The random part of the model included two random effects: δSj, the specific subarea random intercept, and the specific plot random intercept (δPi) nested in the previous one. Finally, εit denotes the residual random error.
The random intercepts δSj and δPi are distributed independently with mean 0 and constant variances, , respectivelly, and the conditional distributions of the residuals, εit|δ, are distributed with mean 0, (E(εit|δ) = 0) and year-specific variance (Var(εit|δ) = σ2t) and independent of all random intercepts δSj and δPi.
The estimated standard deviation of the random base effects of zero mean for each subarea (δSj) and plot (δPi), as well as the conditional standard deviation of the random residue (εit) for each year t, are shown in Supplementary Table 3. The assumption of independence of the residual errors of the model was checked by studying the distribution of Pearson residuals against the fitted values of the model for each subarea (Supplementary Figure 1). No correlation among them related to the subareas was observed, which supports the assumption of independence between subareas. Finally, the normality of errors was tested by studying their deviation from a linear trend in a Q-Q plot (Supplementary Figure 2). Only small deviations were observed, supporting the hypothesis of normality.
The same approach and model were also applied for POC50−2,000 stocks data analysis.
Finally, considering the experimental design, accumulated C in crop residues was assessed by comparing the intervals of values observed in each subarea. Differences between subareas were only considered as significant when these intervals did not overlap.
Soil organic carbon dynamics in M-irr and M-rf were simulated with a two-pool modeling approach, with both pools submitted to exponential decomposition kinetics. Pool 1 (P1) consists of rapidly-decomposable organic matter with a fast decay rate (k1) and, consequently, a short MRT1 (MRT1 = 1/k1). Pool 2 (P2) represents a pool with a slower decay rate (k2) and a longer MRT2 (MRT2 = 1/k2).
There are different options for coupling the two pools. We tested a two sequential-pools model (sequential) and a two parallel-pools model (parallel) (Figure 2), as those described by Derrien and Amelung (39), adding a new parameter (Incorporated fraction, IF) to account for the increasingly acknowledged possibility that only a proportion of crop residues would be effectively incorporated as C inputs into the soil (79). In the sequential option, C inputs are first directed into P1. When C leaves P1, a proportion (tr) is transferred to P2 and the rest is lost as CO2. The C eventually leaving P2 is fully lost as CO2. In the parallel option, it is assumed that C inputs are directed into both pools P1 and P2. A proportion (s) is allocated to the pool P1, and the remainder (1 – s) enters the pool P2. There is no C exchange between the two pools. Carbon leaving P1 and P2 is lost as CO2.
Figure 2. Flow chart of the two two-pools models considered in this study for soil organic C dynamics, two sequential pools model (A) and two-parallel pools model (B), where Pool 1 cycles more rapidly than Pool 2.
At steady state, the SOC stocks in the two pools, Css_P1 and Css_P2, can be calculated as follows (39), for the sequential model:
and for the parallel model:
where I is the average amount of C inputs, and k1 and k2 are the decay rates of P1 and P2, respectively (Figure 2). The calculation of the mean or equivalent residence time in the whole system (MRTeq) is also possible from MRT1 and MRT2, plus tr or s data (see Supplementary Material).
Model parameters were estimated by calibrating the model with the 7-year dataset from the three plots monitored for their isotopic composition in M-rf and M-irr, and assuming that the POC50−2,000 fraction represented the fast-cycling pool P1. A General Likelihood Uncertainty Estimation (GLUE) (80, 81) calibration procedure was applied. This approach enables to simultaneously (i) optimize the model parameters in order to obtain the best fit between the modeled and empirical datasets and (ii) quantify the uncertainties associated with parameter estimates. This Monte Carlo type of approach is based upon making a large number of runs of a given model with different sets parameter values, chosen randomly from specified parameter distributions. On a basis of comparing predicted and observed responses, each set of parameter values is assigned a likelihood of being a simulator of the system (80).
The amounts of simulated total SOC (P1 + P2) and C in the labile fraction (P1) were initialized using the average measured values of TOC and POC50−2,000 before the onset of the experiment (year 0), respectively. For each of the model parameters to optimize (IF, MRT1, MRT2, tr, or s), an initial interval of possible values was defined: (0–1) for the proportions IF, tr and s, (0–50 years) for MRT1, and (50–200 years) for MRT2. Each model parameter was assigned a random value within its interval. The model was then run and the performance of the model run was determined using the Root Mean Square Error (RMSE):
where n is the number of observation dates, and Oi and Pi are the observed and predicted proportions of C for each observation date i, respectively (39).
This process was repeated 6,000 times. Within these 6,000 combinations of model parameters, all parameter combinations leading to a calculated new steady-state total carbon pool (TOCss) >2.5-fold the initial carbon pool were rejected, on the basis of a regional-scale study conducted in the same area by Antón et al. (33). In this study, it was observed that SOC stocks in agricultural fields converted to irrigation were up to 1.8 times greater than those in similar soils under rainfed cropping conditions after 20 years.
Finally, the 50 combinations of parameters that resulted in the lowest values of total RMSE (Equation 8), were used to define a new interval of possible values for each parameter for the next cycle. The modeled parameter intervals obtained after 5 cycles were used to estimate the range of optimal model parameters and quantify their associated uncertainty. The number of cycles and the random parameter combinations were defined in order to ensure the repeatability of the GLUE-optimization procedure.
As for carbon input data, differences in mean residence time (MRT1 and MRT2), incorporated fraction of crop residues (IF) and in the proportion of C input allocated to the pool P1 (s) between M-irr and M-rf were assessed by comparing the intervals of possible model parameters values obtained through the GLUE procedure. Differences were only considered as significant when these intervals did not overlap.
Accumulated C in crop residues after 7 years were significantly higher (no overlapping of the intervals), in the irrigated than in the rainfed subareas where maize was cultivated (M-irr vs. M-rf), while no differences were observed between the wheat subareas (W-irr vs. W-rf, Figure 3).
Figure 3. Accumulated C in crops residues (Mg ha−1) in the 7 years of study per crop-irrigation combination. M-irr: maize, irrigated; M-rf: maize, rainfed; W-irr: wheat, irrigated; W-rf: wheat, rainfed. The box plots represent the median (n = 6), 25th and 75th percentiles. *Represents significant differences (no overlapping of the intervals).
The changes in TOC and POC50−2,000 for each of the 6 plots in each subarea, as well as the average evolution the subareas (W-rf, W-irr, M-rf, and M-irr) are shown in Figures 4, 5. The TOC and POC50−2,000 stocks at year 0 showed no significant differences between these four subareas (Year 0 in Figures 4, 5). The correlation observed in TOC and POC50−2,000 measurements in adjacent years for all W-rf, W-irr, M-rf and M-irr, supported the use of a LMM model (Equation 3). The average effects of the studied factors (crops and irrigation) on TOC and POC50−2,000, as observed with the LMM model are shown in Table 2.
Figure 4. Total organic C (TOC) stocks (Mg ha−1) in the six plots per crop-irrigation combination, and average values over time (dashed line). M-irr, maize, irrigated (A); M-rf, maize, rainfed (B); W-irr, wheat, irrigated (C); W-rf, wheat, rainfed (D).
Figure 5. Particulate organic C (POC) stocks (Mg ha−1) in the six plots per crop-irrigation combination, and average values over time (dashed line). M-irr, maize, irrigated (A); M-rf, maize, rainfed (B); W-irr, wheat, irrigated (C); W-rf, wheat, rainfed (D).
Table 2. Estimations from the linear mixed-effects model (LMM) for the changes in time of TOC and POC50−2,000.
A significant trend of TOC (and POC50−2,000) to increase was observed over the years in the four subareas, confirming the increasing trends observed in Figures 4, 5. This observation implies that none of the subzones could be considered to be at steady-state in terms of C storage after 7 years of experimentation.
A significant mean effect associated with irrigation was detected. The LMM results explained this difference as an increase of 3.44 Mg C ha−1 in the mean value of SOC, and of 1.06 Mg ha−1 in the case of POC50−2,000 (Table 2). The LMM also showed an annual variation of SOC and POC50−2,000 associated with the different crops, which was of 1.02 Mg SOC ha−1 year−1 and of 0.49 Mg POC50−2,000 ha−1 year−1 in the case of maize, and of 0.51 Mg SOC ha−1 year−1 and of 0.03 Mg POC50−2,000 ha−1 year−1 for wheat (Table 2).
According to these trends observed in the LMM model, the overall effect of irrigation on TOC stocks in year 7 would be of +7.1% between M-irr and M-rf, and of +7.0% between W-irr and W-rf. In the case of POC50−2,000, the differences in year 7 between M-irr and M-rf would be of +13.7%, and of +13.9% between W-irr and W-rf.
Overall, the greatest observed difference between POC50−2,000 and TOC data was that the changes in POC50−2,000 were faster than those of TOC in all subareas (W-rf, W-irr, M-rf, and M-irr), with an average annual increase estimated for POC50−2,000 of +7.7% compared with +2.1% for TOC (Table 2).
The sequential model, built on the assumption that POC50−2,000 corresponds to the fast-cycling pool P1, was not able to satisfactory reproduce SOC dynamics in the maize plots (Figure 6—parameter values given Supplementary Tables 4, 5). It correctly reproduced the change in stocks in TOC and POC50−2,000, but it could not reproduce the C4-C incorporation patterns. Whatever the time after conversion, observations indicated larger C4-TOC stocks than C4-POC50−2,000 stocks, which is contradictory to simulations with a sequential model of SOC dynamics. In a sequential model, during the first years after the crop conversion, the new C4 carbon is almost completely in P1, and there is no or negligible amounts of C4-C in P2, as shown by the superposition of the simulated curves for C4-C in P1 and C4-C in (P1+P2) in Figure 6.
Figure 6. Simulations of organic C and C4-C stocks dynamics in P1 (gray dashed lines) and (P1+P2) (dark lines) with the sequential model. The 50 best fits are represented. Total organic C (TOC, gray dots) and particulate organic C (POC, dark dots) observed data are also displayed (n = 3). Organic C (A), C4-C stocks (B), in M-rf (maize, rainfed) on top panels and organic C (C), and C4-C stocks (D) in M-irr (maize, irrigated) on bottom panels.
Unlike the sequential model, the parallel model successfully reproduced the dynamics observed for TOC and POC50−2,000 in the maize plots (both total stocks and kinetics of new C4-C incorporation, Figure 7). The 50 best combinations of parameters provided by the GLUE approach for this model are given in Supplementary Tables 6, 7. They were used to gain quantitative insights into the effect of irrigation on SOC dynamics, and are explained in the following section for M-rf and M-irr plots.
Figure 7. Simulations of C and C4 stocks dynamics in P1 (gray dashed lines) and (P1+P2) (dark lines) with the parallel model. The 50 best fits are represented. TOC (gray dots) and POC (dark dots) observed data are also displayed (n = 3). Organic C (A), C4-C stocks (B), in M-rf (maize, rainfed) on top panels and organic C (C), and C4-C stocks (D) in M-irr (maize, irrigated) on bottom panels.
The intervals of possible model parameters generated by the GLUE optimization procedure for the irrigated and rainfed plots for MRT2, the split s parameter, and the incorporation factor of crop residues, IF (Figure 8) did not overlap. The proportion of C from crop residues incorporated into the soil (IF) was ~19% higher in the irrigated plots, from 0.36 (M-rf) to 0.43 (M-irr). MRT2 was also significantly higher in the irrigated plots (67.2 ± 3.6 years in M-rf against 86.3 ± 1.1 years in M-irr). The split of plant input (s) between P1 and P2 was slightly but significantly higher for the irrigated plots, favoring the incorporation into P1 by 8% in average over the whole set of parameters (0.39 ± 0.00 for M-rf against 0.42 ± 0.00 for M-irr).
Figure 8. Fifty best sets of parameters obtained for the parallel model with the GLUE procedure. Values of MRT1 (A), MRT2 (B), the split s parameter (C), and the proportion from crop residues incorporated into the soil (IF) (D) in the parallel model for rainfed maize (M-rf) and irrigated maize (M-irr). The boxplots represent the median (n = 50), 25th and 75th percentiles with the whiskers extending to the most extreme data points within the 1.5× of values generated by the model for each parameter. *Represents significant differences (no overlapping of the intervals of possible model parameters).
No effect was however observed for MRT1 (mean MRT1 obtained for the 50 best sets of parameters in M-rf was 49.8 ± 0.05 years against 49.3 ± 0.31 years for M-irr).
The first observation in the assessment of TOC and POC50−2,000 storage was that none of the four subareas (W-rf, W-irr, M-rf, and M-irr) was at steady state: all showed increments of both TOC and POC50−2,000 stocks in the 7 years of the study. In our hypothesis, this behavior was however only expected for the subareas including a change (irrigation and/or the introduction of maize, as in W-irr, M-rf and M-irr) from the original situation (rainfed C3 plants cropping, as in W-rf). An explanation for this can be the fact that cropping conditions were standardized when the experiment was designed, and carefully applied during the 7 years. In contrast, in the previous situation, the most extended rainfed agricultural practices in the area included low fertilization and frequent crop residues removal from the field (Apesteguía, personal comm.). In terms of the general assessment of organic C stabilization, this would imply that an improvement in fertilization routines and the restitution of crop residues can effectively increase SOC stocks in this region. Other studies have shown that adequate fertilization, especially with N, can result in improved SOC storage (19, 82). This observation was beyond the goals of this study, but seems relevant in the framework of present policies encouraging SOC storage in agricultural soils in the European Union (83).
Second, a relevant observation in relation to the objective of this study was that, unlike other studies reporting losses of SOC upon the adoption of irrigation (84–86), the TOC and POC50−2,000 stocks in the irrigated subareas were higher than in the rainfed subareas.
Thirdly, the fixed crop effect observed from the LMM (Table 2), implies a cumulative effect associated to wheat (wheat vs. maize year interaction in Table 2). From this, it is reasonable to suppose that the effect observed with irrigation could also respond to an annual cumulative evolution, but this could not be verified in this work due to the relatively short period of time since the trial set up.
From these last observations, it can be deduced that both the type of crop and the presence or not of irrigation had a role in the net final evolution of TOC and POC50−2,000 stocks in the four subareas. This implies that, under the conditions of the experimental field studied here, it is reasonable to consider that the introduction of irrigation, which results in higher C inputs to the soil, was a driver for the changes observed in SOC storage, as stated by the main hypothesis of this work.
Understanding the consequences of these changes in SOC cycling is therefore needed for a complete assessment of the final consequences of this land-use change.
In this sense, it was first observed that the change over time of POC50−2,000 was greater than that of TOC (Figures 4, 5; Table 2). This confirmed POC50−2,000, as isolated here, as a fraction behaving as an early indicator of SOC changes in the conditions of this study (87, 88), and which can be considered as a fast cycling pool in the mechanistic two-pools modeling approach used, in agreement with results from Poeplau et al. (52). These authors concluded that the separation of particles >50 and <50 μm, recovered after chemical dispersion as described in Sanderman et al. (89), yielded the strongest contrasts in terms of turnover rates. Cotrufo et al. (90) also described this fraction as useful to inform on organic C storage at different levels of protection, and as predominantly of plant origin and persisting in soil through physical protection in aggregates and/or microbial inhibition.
The fact that the parallel model (Figure 7) was a closer approximation to actual SOC dynamics in the studied soil than the sequential model option (Figure 6) suggested that, in the soil and cropping conditions of this experiment, the protection of organic C from crop residues can be direct, once they are incorporated into the soil. This can however also been partially explained if a small fraction of the labile fraction POC50−2,000 was transferred to the non-labile fraction in the fractionation process, as suggested by one recent re-assessment of different methodologies to isolate slow and fast cycling SOC fractions (56) We reduced rinsing, and used a non-alkaline solution, to minimize this aspect, but it is still possible that part of the most labile fraction was indeed accounted for in the non-labile fraction in our case.
Despite of this possible fractionation bias, there is consensus on the fact that the interaction of the organic and mineral components of the soil represents a major factor controlling SOC stabilization (20). The nature and proportion of the mineral compounds largely determine their functioning as stabilizers of SOC (91–93). In calcareous soils like the one studied here alkaline carbonates can be an important factor of aggregation (24, 94), which can result in longer-term organic matter protection in comparison to carbonates-free soils (95–97). In fact, it is a long known observation (98) that in calcareous soils SOC contents are usually higher than in soils without carbonates under equal conditions, especially in agricultural soils with low SOC levels (99, 100). The soil of this study had a carbonates content of 37% by mass in the upper layer (0–30 cm, Supplementary Table 2). Carbonates can promote the fast incorporation of crop residues into stable aggregates with slow cycling in comparison with non-calcareous soils, as observed by Fernández-Ugalde et al. (24), very likely as a result of carbonate dissolution and re-precipitation cycles. As explained by Rowley et al. (25), in such situations, part of the residues incorporated would be directly protected within aggregates of different sizes stabilized by carbonates. The elevated content of alkaline carbonates of the studied soil (38% carbonates by mass at 0–30 cm, Supplementary Table 2), could therefore at least partially explain this observation, as well as the relatively long MRTs of the fraction POC50−2,000.
Indeed, MRT1 values found in our calcareous soil were close to 40 years, which seems relatively long if we consider that the pool P1 conceptually represents a labile fraction of rapid renewal.
Other studies based on the natural abundance of 13C in soil samples and conducted on C3-C4 plant-shift experiments in other soil types and climate conditions, have found MRTs in the same order of magnitude, but smaller than our data, associated with the fraction 50–2,000 μm and with ρ > 1 g cm−3 recovered after chemical dispersion. These ranged from 13.8 to 28 years calculated from Derrien et al. (38) and Balesdent (101) on silty soils in humid temperate France, or 20.6 years from Dalal et al. (102) in warmer and more arid conditions in Queensland (Australia). Haile-Mariam et al. (53) reported between 5.6 and 12.8 years in three studies with 30 years of maize cultivation on three soils (Typic Fragiudalf, Mollic Ochracqualf, and Typic Haplustoll) in the MidWest of the US, and of 11.4 years in a Typic Hapludalf after 8 years of maize cultivation for this fraction. Paul (55), combining incubations and field samples data, described MRT values between 12 and 7.8 years in two Mollisols in Canada and Ohio. None of these soils was calcareous.
Therefore, although our results confirm the traditional vision of POC50−2,000 as a fraction with a faster turnover than bulk soil cycling (55, 90, 103, 104), they also open questions on the concept of precocity when referring to POC50−2,000, and on the interaction of soil and climate factors in this sense. It has to be also considered that the fractionation methodological approach followed in this study may also partially explain this observation.
Another relevant result from the modeling approach was that, in all cases, the optimized fitting of the observed TOC and POC50−2,000 data indicated that a large proportion of crop residues was not actually incorporated into the soil (parameter IF <50%).
Different reasons can explain this observation. First, a loss of crop residues left on the ground is possible before they are actually incorporated into SOC, as part of these residues (aerial crop parts) remained on the surface of the soil after harvest for a period of 4–5 months before incorporation by tillage. It has indeed been reported by local extension agents that wind and intense meteorological events, as well as the activity of wild fauna, can exert a significant reduction in the amount of biomass during this time (Apesteguía, personal comm.). Second, direct mineralization of aerial crop residues compared with belowground residues has been frequently reported as the cause of belowground crop biomass contributing more to SOC than aboveground biomass. Jackson et al. (79) analyzed 6 maize field experiments with a duration ranging between 2 and 15 years, and observed that, on average, 47 ± 20% of belowground C inputs were stabilized as SOC compared with 11 ± 4% of aboveground C inputs, showing great variability depending on different agroclimatic conditions or management. These authors emphasized that, although many models for C balances generally assume a direct relationship between crops biomass production, crop residues inputs, and SOC accumulation, there is yet little direct evidence for such a relationship.
Our investigation showed that the input of plant material into the soil was higher in the irrigated subarea with maize (M-irr) than in the rainfed subarea (M-rf), which can be explained by both the higher amount of C accumulated in crop residues potentially incorporated into the soil in this area (Figure 3), as well as by a higher incorporation of crop residues into the studied layer (0–30 cm) (IF data, Figure 8).
One explanation for this increased effective incorporation of crop residues could be that irrigation could induce a difference in the root distribution with depth resulting in a higher proportion of roots concentrated in the studied 0–30 cm soil layer compared with rainfed management. In rainfed systems within areas where water availability is limited, the soil exploration for available water results in crop root systems tending to expand, usually in depth (105), notably in the case of maize (106), while irrigation tends to increase maize root density in the topsoil (107). It is also possible that the granted moisture conditions during the growing season of maize in the irrigated subarea (Figure 1) would provide better conditions for an effective incorporation of crop residues (which were incorporated with tillage shortly before seeding) into the soil matrix by earthworms and other soil fauna. Irrigation can also enhance the leaching of water-soluble materials from crop residues, which should incorporate quickly to organo-mineral complexes (fraction <50 μm), particularly in Ca-rich soils (108). Also, the higher water availability in the soil can accelerate the transfer of organic matter from POM to the <50 μm fraction.
According to the modeling results, MRT1 was not significantly different in M-irr than in M-rf, and contrary to our expectation, MRT2 was higher in M-irr than M-rf. In relation to our hypothesis of irrigation affecting SOC mineralization due to a possible enhancement of microbial activity, and therefore resulting in shorter MRTs of SOC than rainfed cropping, these results do not allow to support it.
Finally, in relation to the effect of irrigation on the partitioning of crop residues C between the labile and the stable SOC fractions, the results of the parallel model showed a slight increase in s in M-irr compared to M-rf (Figure 8). This suggests that slightly less crop residues would be protected in the slow-cycling pool P2 with irrigation, but their protection would occur over a longer time because MRT2 increased with respect to M-rf.
The calculation of the mean residence time in the whole system (MRTeq, Equation 10 included in Supplementary Table 6) and the stock of SOC at the new steady state projected with the parallel model (Equations 4–7) enabled to balance the apparent diverging effects of irrigation on mineralization and stabilization processes. The MRTeq of M-irr was of 70.8 ± 0.5 years vs. 60.4 ± 2.2 years for M-rf (+17% with irrigation, see Supplementary Tables 6, 7), which indicates that an enhanced stabilization of SOC could be an effective consequence of the introduction of irrigation. This dominance of stabilization over mineralization was related to the observed difference in MRT2 between the irrigated and rainfed maize subareas (+28% in M-irr than in M-rf, Figure 8). In terms of projected SOC stocks in the new steady state equilibrium (TOCss), the projection was 2.50 times the initial value of TOC in M-irr, and 1.23 times in M-rf (Supplementary Tables 6, 7; Supplementary Figure 3), in line with the trend observed in TOC data in the first 7 years of this study, and within the range of field observations in a previous study in the region on calcareous soils (32, 33). The possible limitations of the fractionation procedure, together with the relatively short duration of the study, hinder however, to draw clear conclusions from these observations (58).
In summary, and as a general response to our working hypotheses, the modeling results indicated that the greatest difference between irrigated and rainfed maize subareas in SOC storage seemed related to crop residues incorporation, and not to SOC mineralization rates. Carbon inputs would therefore be here the major driver responsible for the observed and projected increase in SOC storage in the irrigated subarea, as increasingly claimed (10, 109, 110). A careful determination of the actual amount of crop residues entering the soil is then of the uttermost importance to accurately predict changes in SOC stocks and dynamics (111). This seems of especial relevance considering our results, where it was observed that introducing a system (irrigation) that multiplied the C accumulated in C residues by ~70% (M-irr vs. M-rf in Figure 3), did not automatically imply multiplying SOC stocks by the same proportion (Figure 4) in the period studied. New tools for monitoring root systems and in situ organic C incorporation from crops in the field are needed, and these must be integrated into simulation models (79, 112). Adequate allometric functions for estimating C inputs seem in this sense critical to select the appropriate modeling approach (113).
Changes in SOC and POC50−2,000 stocks over the 7-year study period where observed in the soil of the experimental field used in this study, with overall greater increments in the sprinkler-irrigated areas at 0–30 cm. This means that the hypothesis that irrigation can result in SOC gains when it results in higher C inputs to the soil and implemented in calcareous soils like the one used in this study, can be accepted.
The modeling approach based on the C3-C4 natural isotopic labeling study showed that the parallel two-pool model option seemed to be a closer representation of the agrosystem evaluated. The interaction of the mineral phase of this soil, with 38% carbonates in the studied depth, could explain at least partially this observation, because carbonates are known to directly promote the protection of fresh organic matter in the soil. This can also be partially explained if the SOC fractionation approach would result in a transfer of SOC from the labile to non-labile fractions. This highlights the relevant need for site-specific investigation to properly understand the response of soil and SOC to major management changes, and the need for adequate and case-specific fractionation approaches.
This two-pool model allowed understanding that the changes in SOC dynamic were most likely due not only to an increase in C inputs from crop residues associated to irrigation, but also because of a more efficient incorporation of these residues into SOC, in the studied topsoil layer. No relevant differences were observed in the model parameters explaining SOC portioning or mineralization.
This study represents therefore a contribution to deepen our understanding of SOC dynamics associated with changes in land use, and in particular, the introduction of irrigation in a field on a calcareous soil. It provides relevant information in assessing the impact of the introduction of irrigation on the net soil C balance, and on the C footprint of agrosystems, which are needed to better understand its significance in terms of agricultural sustainability and climate policies. Crop types, annual contributions of crop biomass incorporated into the soil, and agricultural management were observed as the most important drivers of this process in the soil and climate conditions of the studied field. The use of SOC cycling modeling showed to be a useful approach in this assessment.
The challenges associated to the expansion of irrigation remain significant and need to be carefully considered when such a strategy is considered at a regional or national scale. Our observations support the idea that this expansion can increase biomass productivity and favor SOC storage, but this should be considered in the context with other environmental and socioeconomic issues such as the competition for water use, greenhouse gasses emissions and/or food sovereignty.
The raw data supporting the conclusions of this article will be made available by the authors, without undue reservation.
IV and AE were responsible for the conception and design of the study and DD led model development in collaboration with RA. RA, IV, and DD organized the database and performed formal analysis. HU performing statistical analysis and GH providing guidance on modeling approaches. RA, IV, DD, and HU wrote the first draft of the manuscript and GH and AE contributed to review and editing. IV was responsible for the acquisition of funds. All authors have read and approved the submitted version.
The authors thank funding from INIA, Spanish Agency (Grant INIA RTA2009-00052-C02-02), and the European Commission Integrated Life Program (Life NADAPTA Project, LIFE 16 IPC/ES/000001). The authors would like to thank SILVATECH (Silvatech, INRAE, 2018. Structural and functional analysis of tree and wood Facility, doi: 10.15454/1.5572400113627854E12) from UMR 1434 SILVA, 1136 IAM, 1138 BEF and 4370 EA LERMAB from the research center INRAE Grand-Est Nancy for it contribution to isotopic analysis. SILVATECH facility is supported by the French National Research Agency through the Laboratory of Excellence ARBRE (ANR-11-LABX-0002-01). RA was awarded a pre-doctoral fellowship by Universidad Pública de Navarra, and part of this work is included in his Ph.D., thesis.
The authors declare that the research was conducted in the absence of any commercial or financial relationships that could be construed as a potential conflict of interest.
All claims expressed in this article are solely those of the authors and do not necessarily represent those of their affiliated organizations, or those of the publisher, the editors and the reviewers. Any product that may be evaluated in this article, or claim that may be made by its manufacturer, is not guaranteed or endorsed by the publisher.
The Instituto Navarro de Tecnologías e Innovación Agroalimentarias (INTIA) is thanked for the maintenance and crop management of the experimental field. In particular, Luis Orcaray, Marcos Apesteguía, and Nerea Arias are thanked for their involvement in this work. We also thank the staff and students at the Soil Science group of the Department of Sciences (Universidad Pública de Navarra), for their assistance during soil and biomass sampling, and Prof. Esther González for valuable advice in C4-plants physiology.
The Supplementary Material for this article can be found online at: https://www.frontiersin.org/articles/10.3389/fsoil.2022.831775/full#supplementary-material
1. Zaveri EB, Lobell D. The role of irrigation in changing wheat yields and heat sensitivity in India. Nat Commun. (2019) 10:4144. doi: 10.1038/s41467-019-12183-9
2. Salmon JM, Friedl MA, Frolking S, Wisser D, Douglas EM. Global rain-fed, irrigated, and paddy croplands: a new high resolution map derived from remote sensing, crop inventories and climate data. Int J Appl Earth Obs Geoinf. (2015) 38:321–34. doi: 10.1016/j.jag.2015.01.014
3. Tanasijevic L, Todorovic M, Pereira LS, Pizzigalli C, Lionello P. Impacts of climate change on olive crop evapotranspiration and irrigation requirements in the Mediterranean region. Agric Water Manag. (2014) 144:54–68. doi: 10.1016/j.agwat.2014.05.019
4. Patanita M, Tomaz A, Ramos T, Oliveira P, Boteta L, Dôres J. Water regime and nitrogen management to cope with wheat yield variability under the mediterranean conditions of Southern Portugal. Plants. (2019) 8:1–15. doi: 10.3390/plants8100429
5. Ricart S, Clarimont S. Modelling the links between irrigation, ecosystem services and rural development in pursuit of social legitimacy: results from a territorial analysis of the Neste System (Hautes-Pyrénées, France). J Rural Stud. (2016) 43:1–12. doi: 10.1016/j.jrurstud.2015.09.012
6. Velasco-Muñoz JF, Aznar-Sánchez JA, Batlles-delaFuente A, Fidelibus MD. Sustainable irrigation in agriculture: an analysis of global research. Water. (2019) 11:1–26. doi: 10.3390/w11091758
7. Lal R. Food security impacts of the “4 per Thousand” initiative. Geoderma. (2020) 374:114427. doi: 10.1016/j.geoderma.2020.114427
8. Pareja-Sánchez E, Cantero-Martínez C, Álvaro-Fuentes J, Plaza-Bonilla D. Soil organic carbon sequestration when converting a rainfed cropping system to irrigated corn under different tillage systems and N fertilizer rates. Soil Sci Soc Am J. (2020) 84:1219–32. doi: 10.1002/saj2.20116
9. Minasny B, Malone BP, McBratney AB, Angers DA, Arrouays D, Chambers A, et al. Soil carbon 4 per mille. Geoderma. (2017) 292:59–86. doi: 10.1016/j.geoderma.2017.01.002
10. Chenu C, Angers DA, Barré P, Derrien D, Arrouays D, Balesdent J. Increasing organic stocks in agricultural soils: knowledge gaps and potential innovations. Soil Tillage Res. (2019) 188:41–52. doi: 10.1016/j.still.2018.04.011
11. Rumpel C, Amiraslani F, Chenu C, Garcia Cardenas M, Kaonga M, Koutika L-S, et al. The 4p1000 initiative: opportunities, limitations and challenges for implementing soil organic carbon sequestration as a sustainable development strategy. Ambio. (2020) 49:350–60. doi: 10.1007/s13280-019-01165-2
12. Erb KH, Luyssaert S, Meyfroidt P, Pongratz J, Don A, Kloster S, et al. Land management: data availability and process understanding for global change studies. Glob Chang Biol. (2017) 23:512–33. doi: 10.1111/gcb.13443
13. Sanz-Cobena A, Lassaletta L, Aguilera E, Prado A, del Garnier J, Billen G, et al. Strategies for greenhouse gas emissions mitigation in Mediterranean agriculture: a review. Agric Ecosyst Environ. (2017) 238:5–24. doi: 10.1016/j.agee.2016.09.038
14. Virto I, Antón R, Enrique A, Orcaray L, Arias N. 19. Irrigation and SOC sequestration in the region of Navarre in Spain. In: Recarbonizing Global Soils – A Technical Manual of Recommended Sustainable Soil Management. Volume 4: Cropland, Grassland, Integrated Systems and Farming Approaches – Case Studies. Rome: FAO and ITPS (2021). doi: 10.4060/cb6598en
15. Trost B, Prochnow A, Drastig K, Meyer-Aurich A, Ellmer F, Baumecker M. Irrigation, soil organic carbon and N2O emissions. A review Agron Sustain Dev. (2013) 33:733–49. doi: 10.1007/s13593-013-0134-0
16. Moyano FE, Manzoni S, Chenu C. Responses of soil heterotrophic respiration to moisture availability: an exploration of processes and models. Soil Biol Biochem. (2013) 59:72–85. doi: 10.1016/j.soilbio.2013.01.002
17. Aguilera E, Guzmán GI, Álvaro-Fuentes J, Infante-Amate J, García-Ruiz R, Carranza-Gallego G, et al. A historical perspective on soil organic carbon in Mediterranean cropland (Spain, 1900–2008). Sci Total Environ. (2018) 621:634–48. doi: 10.1016/j.scitotenv.2017.11.243
18. Zhou X, Zhou L, Nie Y, Fu Y, Du Z, Shao J, et al. Similar responses of soil carbon storage to drought and irrigation in terrestrial ecosystems but with contrasting mechanisms: A meta-analysis. Agric Ecosyst Environ. (2016) 228:70–81. doi: 10.1016/j.agee.2016.04.030
19. Qiu L, Wu Y, Hao M, Shen J, Lei X, Liao W, et al. Simulation of the irrigation requirements for improving carbon sequestration in a rainfed cropping system under long-term fertilization on the Loess Plateau of China. Agric Ecosyst Environ. (2018) 265:198–208. doi: 10.1016/j.agee.2018.06.015
20. Schmidt MWI, Torn MS, Abiven S, Dittmar T, Guggenberger G, Janssens IA, et al. Persistence of soil organic matter as an ecosystem property. Nature. (2011) 478:49–56. doi: 10.1038/nature10386
21. Lehmann J, Kleber M. The contentious nature of soil organic matter. Nature. (2015) 528:60–8. doi: 10.1038/nature16069
22. Rasmussen C, Heckman K, Wieder WR, Keiluweit M, Lawrence CR, Berhe AA, et al. Beyond clay: towards an improved set of variables for predicting soil organic matter content. Biogeochemistry. (2018) 137:297–306. doi: 10.1007/s10533-018-0424-3
23. Vázquez E, Benito M, Espejo R, Teutscherova N. Response of soil properties and microbial indicators to land use change in an acid soil under Mediterranean conditions. Catena. (2020) 189:104486. doi: 10.1016/j.catena.2020.104486
24. Fernández-Ugalde O, Virto I, Barré P, Gartzia-Bengoetxea N, Enrique A, Imaz MJ, et al. Effect of carbonates on the hierarchical model of aggregation in calcareous semi-arid Mediterranean soils. Geoderma. (2011) 164:203–14. doi: 10.1016/j.geoderma.2011.06.008
25. Rowley MC, Grand S, Verrecchia ÉP. Calcium-mediated stabilisation of soil organic carbon. Biogeochemistry. (2018) 137:27–49. doi: 10.1007/s10533-017-0410-1
26. Bughio MA, Wang P, Meng F, Qing C, Kuzyakov Y, Wang X, et al. Neoformation of pedogenic carbonates by irrigation and fertilization and their contribution to carbon sequestration in soil. Geoderma. (2016) 262:12–9. doi: 10.1016/j.geoderma.2015.08.003
27. Bughio MA, Wang P, Meng F, Chen Q, Li J, Shaikh TA. Neoformation of pedogenic carbonate and conservation of lithogenic carbonate by farming practices and their contribution to carbon sequestration in soil. Zeitschrift Pflanzenernahrung Bodenkd. (2017) 180:454–63. doi: 10.1002/jpln.201500650
28. de Soto IS, Virto I, Barré P, Fernández-Ugalde O, Antón R, Martínez I, et al. A model for field-based evidences of the impact of irrigation on carbonates in the tilled layer of semi-arid Mediterranean soils. Geoderma. (2017) 297:48–60. doi: 10.1016/j.geoderma.2017.03.005
29. Apesteguía M, Virto I, Orcaray L, Enrique A, Bescansa P. Effect of the conversion to irrigation of semiarid mediterranean dryland agrosecoystems on soil carbon dynamics and soil aggregation. Arid L Res Manag. (2015) 29:399–414. doi: 10.1080/15324982.2015.1016245
30. Cayuela ML, Aguilera E, Sanz-Cobena A, Adams DC, Abalos D, Barton L, et al. Direct nitrous oxide emissions in Mediterranean climate cropping systems: emission factors based on a meta-analysis of available measurement data. Agric Ecosyst Environ. (2017) 238:25–35. doi: 10.1016/j.agee.2016.10.006
31. Bai Z, Caspari T, Gonzalez MR, Batjes NH, Mäder P, Bünemann EK, et al. Effects of agricultural management practices on soil quality: a review of long-term experiments for Europe and China. Agric Ecosyst Environ. (2018) 265:1–7. doi: 10.1016/j.agee.2018.05.028
32. Antón R, Arricibita FJ, Ruiz-sagaseta A, Enrique A, de Soto I, Orcaray L, et al. Soil organic carbon monitoring to assess agricultural climate change adaptation practices in Navarre, Spain. Reg Environ Chang. (2021) 21:63. doi: 10.1007/s10113-021-01788-w
33. Antón R, Virto I, Gonzalez J, Hernandez I, Enrique A, Bescansa P, et al. Extension of irrigation in semi-arid regions: what challenges for soil security? Perspectives from a regional-scale project in Navarre (Spain). In: Anne Richer de Forges DA, Carré F, McBratney AB, Bouma J, editors. Global Soil Security. Towards More Science-Society Interfaces. Leiden: CRC Press - Balkema (2019). p. 79–87.
34. Balesdent J. The turnover of soil organic fractions estimated by radiocarbon dating. Sci Total Environ. (1987) 62:405–8. doi: 10.1016/0048-9697(87)90528-6
35. Balesdent J, Mariotti A. Measurement of soil organic matter turnover using 13C abundance. In: Boutton TW, Yamasaki S. Mass Spectrometry of Soils. New York, NY: Marcel Dekker, Inc. (1996).
36. Balesdent J, Basile-Doelsch I, Chadoeuf J, Cornu S, Fekiacova Z, Fontaine S, et al. Turnover of deep organic carbon in cultivated soils: an estimate from a review of isotope data [Renouvellement du carbone profond des sols cultivés: Une estimation par compilation de données isotopiques]. Biotechnol Agron Soc Environ. (2017) 21:1–10. doi: 10.25518/1780-4507.13562
37. Dignac MF, Bahri H, Rumpel C, Rasse DP, Bardoux G, Balesdent J, et al. Carbon-13 natural abundance as a tool to study the dynamics of lignin monomers in soil: an appraisal at the Closeaux experimental field (France). Geoderma. (2005) 128:3–17. doi: 10.1016/j.geoderma.2004.12.022
38. Derrien D, Marol C, Balabane M, Balesdent J. The turnover of carbohydrate carbon in a cultivated soil estimated by 13C natural abundances. Eur J Soil Sci. (2006) 57:547–57. doi: 10.1111/j.1365-2389.2006.00811.x
39. Derrien D, Amelung W. Computing the mean residence time of soil carbon fractions using stable isotopes: impacts of the model framework. Eur J Soil Sci. (2011) 62:237–52. doi: 10.1111/j.1365-2389.2010.01333.x
40. Liu S, Zhang ZB, Li DM, Hallett PD, Zhang GL, Peng XH. Temporal dynamics and vertical distribution of newly-derived carbon from a C3/C4 conversion in an Ultisol after 30-yr fertilization. Geoderma. (2019) 337:1077–85. doi: 10.1016/j.geoderma.2018.11.021
41. Aalde H, Gonzalez P, Gytarsky M, Krug T, Kurz WA, Lasco RD, et al. Chapter 2, generic methodologies applicable to multiple land-use categories. In: 2006 IPCC Guidelines For national Greenhouse Gas Inventories: Volume 4 Agriculture, Forestry And Other Land Use, ed. IPCC (Hayama: The Institute for Global Environmental Strategies (IGES)) (2006).
42. Novara A, Gristina L, Kuzyakov Y, Schillaci C, Laudicina VA, La Mantia T. Turnover and availability of soil organic carbon under different Mediterranean land-uses as estimated by 13C natural abundance. Eur J Soil Sci. (2013) 64:466–75. doi: 10.1111/ejss.12038
43. Campbell EE, Paustian K. Current developments in soil organic matter modeling and the expansion of model applications: a review. Environ Res Lett. (2015) 10:123004. doi: 10.1088/1748-9326/10/12/123004
44. Jenkinson DS. The turnover of organic carbon and nitrogen in soil. Philos Trans R Soc London Ser B Biol Sci. (1990) 329:361–8. doi: 10.1098/rstb.1990.0177
45. Trumbore SE. Potential responses of soil organic carbon to global environmental change. Proc Natl Acad Sci USA. (1997) 94:8284–91. doi: 10.1073/pnas.94.16.8284
46. Batlle-Aguilar J, Brovelli A, Porporato A, Barry DA. Modelling soil carbon and nitrogen cycles during land use change. Sustain Agric. (2011) 2:499–527. doi: 10.1007/978-94-007-0394-0_23
47. Stockmann U, Adams MA, Crawford JW, Field DJ, Henakaarchchi N, Jenkins M, et al. The knowns, known unknowns and unknowns of sequestration of soil organic carbon. Agric Ecosyst Environ. (2013) 164:80–99. doi: 10.1016/j.agee.2012.10.001
48. Ellerbrock RH, Kaiser M. Stability and composition of different soluble soil organic matter fractions - Evidence from δ13C and FTIR signatures. Geoderma. (2005) 128:28–37. doi: 10.1016/j.geoderma.2004.12.025
49. Zimmermann M, Leifeld J, Schmidt MWI, Smith P, Fuhrer J. Measured soil organic matter fractions can be related to pools in the RothC model. Eur J Soil Sci. (2007) 58:658–67. doi: 10.1111/j.1365-2389.2006.00855.x
50. Blankinship JC, Berhe AA, Crow SE, Druhan JL, Heckman KA, Keiluweit M, et al. Improving understanding of soil organic matter dynamics by triangulating theories, measurements, and models. Biogeochemistry. (2018) 140:1–13. doi: 10.1007/s10533-018-0478-2
51. Six J, Merckx R, Kimpe K, Paustian K, Elliott ET. A re-evaluation of the enriched labile soil organic matter fraction. Eur J Soil Sci. (2000) 51:283–93. doi: 10.1046/j.1365-2389.2000.00304.x
52. Poeplau C, Don A, Six J, Kaiser M, Benbi D, Chenu C, et al. Isolating organic carbon fractions with varying turnover rates in temperate agricultural soils – A comprehensive method comparison. Soil Biol Biochem. (2018) 125:10–26. doi: 10.1016/j.soilbio.2018.06.025
53. Haile-Mariam S, Collins HP, Wright S, Paul EA. Fractionation and long-term laboratory incubation to measure soil organic matter dynamics. Soil Sci Soc Am J. (2008) 72:370–8. doi: 10.2136/sssaj2007.0126
54. Mazzilli SR, Kemanian AR, Ernst OR, Jackson RB, Piñeiro G. Priming of soil organic carbon decomposition induced by corn compared to soybean crops. Soil Biol Biochem. (2014) 75:273–81. doi: 10.1016/j.soilbio.2014.04.005
55. Paul EA. The nature and dynamics of soil organic matter: plant inputs, microbial transformations, and organic matter stabilization. Soil Biol Biochem. (2016) 98:109–26. doi: 10.1016/j.soilbio.2016.04.001
56. Just C, Poeplau C, Don A, van Wesemael B, Kögel-Knabner I, Wiesmeier M. A simple approach to isolate slow and fast cycling organic carbon fractions in Central European Soils—importance of dispersion method. Front Soil Sci. (2021) 1:e692583. doi: 10.3389/fsoil.2021.692583
57. Keel SG, Leifeld J, Mayer J, Taghizadeh-Toosi A, Olesen JE. Large uncertainty in soil carbon modelling related to method of calculation of plant carbon input in agricultural systems. Eur J Soil Sci. (2017) 68:953–63. doi: 10.1111/ejss.12454
58. Schiedung H, Tilly N, Hütt C, Welp G, Brüggemann N, Amelung W. Spatial controls of topsoil and subsoil organic carbon turnover under C3–C4 vegetation change. Geoderma. (2017) 303:44–51. doi: 10.1016/j.geoderma.2017.05.006
59. Coppens F, Garnier P, De Gryze S, Merckx R, Recous S. Soil moisture, carbon and nitrogen dynamics following incorporation and surface application of labelled crop residues in soil columns. Eur J Soil Sci. (2006) 57:894–905. doi: 10.1111/j.1365-2389.2006.00783.x
60. DDRMA GB (Departamento de Desarrollo Rural y Medio Ambiente Gobierno de Navarra) (2019). Estaciones meteorológicas (meteorological station data) [In Spanish]. Available online at: http://meteo.navarra.es/estaciones/mapadeestaciones.cfm (accessed Marzo 18, 2019).
62. IUSS Working Group WRB. World Reference Base for Soil Resources 2014, update 2015. International Soil Classification System for Naming Soils and Creating Legends for Soil Maps. World Soil Resources Reports No. 106. Rome: FAO (2015).
63. Aguacanal Sociedad concesionaria Zona I Canal de Navarra Local Agency. Consumos Multihidrante (Water Consumption) [In Spanish]. Available online at http://www.aguacanal.es/regantes/multihidrante/ (accessed December 20, 2018).
64. IPCC. 2006 IPCC Guidelines for National Greenhouse Gas Inventories, Prepared by the National Greenhouse Gas Inventories Programme, editors. Eggleston TK, Buendia HS, Miwa L. IGES (Hayama) (2006).
65. Klute A editor. Methods of Soil Analysis. Part 1. Physical and Mineralogical Methods. Madison: Agronomy S. American Society of Agronomy (1986).
66. Sherrod LA, Dunn G, Peterson GA, Kolberg RL. Inorganic Carbon Analysis by Modified Pressure-Calcimeter Method. Soil Sci Soc Am J. (2002) 66:299–305. doi: 10.2136/sssaj2002.2990
68. Tiessen H, Moir JO. Total and organic C. In: Carter MR, editors. Soil Sampling and Methods of Analysis. Ottawa, ON: CRC Press (1993). p. 187–99.
69. Apesteguia M, Plante AF, Virto I. Methods assessment for organic and inorganic carbon quantification in calcareous soils of the Mediterranean region. Geoderma Reg. (2018) 12:39–48. doi: 10.1016/j.geodrs.2017.12.001
70. Virto I, Imaz MJ, Enrique A, Hoogmoed W, Bescansa P. Burning crop residues under no-till in semi-arid land, Northern Spain - Effects on soil organic matter, aggregation, and earthworm populations. Aust J Soil Res. (2007) 45:414–21. doi: 10.1071/SR07021
71. Cambardella CA, Elliott ET. Particulate soil organic-matter changes across a grassland cultivation sequence. Soil Sci Soc Am J. (1992) 56:777. doi: 10.2136/sssaj1992.03615995005600030017x
72. Ellert BH, Bettany JR. Calculation of organic matter and nutrients stored in soils under contrasting management regimes. Can J Soil Sci. (1995) 75:529–38. doi: 10.4141/cjss95-075
73. Lee J, Hopmans JW, Rolston DE, Baer SG, Six J. Determining soil carbon stock changes: Simple bulk density corrections fail. Agric Ecosyst Environ. (2009) 134:251–6. doi: 10.1016/j.agee.2009.07.006
74. Poeplau C, Don A. Sensitivity of soil organic carbon stocks and fractions to different land-use changes across Europe. Geoderma. (2013) 192:189–201. doi: 10.1016/j.geoderma.2012.08.003
75. Harris D, Horwáth WR, van Kessel C. Acid fumigation of soils to remove carbonates prior to total organic carbon or CARBON-13 isotopic analysis. Soil Sci Soc Am J. (2001) 65:1853. doi: 10.2136/sssaj2001.1853
76. Rasse DP, Mulder J, Moni C, Chenu C. Carbon Turnover Kinetics with Depth in a French Loamy Soil. Soil Sci Soc Am J. (2006) 70:2097. doi: 10.2136/sssaj2006.0056
77. Pinheiro J, Bates D, DebRoy S, Sarkar D, R Core Team. nlme: Linear Nonlinear Mixed Effects Models. R package version 3.1-148. (2020). Available online at: https://cran.r-project.org/package=nlme (accessed February 21, 2019).
78. Galecki A, Burzykowski T. Linear Mixed-Effects models using R. A Step-by-Step Approach. New York, NY: Springer Science+Business Media (2013).
79. Jackson RB, Lajtha K, Crow SE, Hugelius G, Kramer MG, Piñeiro G. The ecology of soil carbon: pools, vulnerabilities, and biotic and abiotic controls. Annu Rev Ecol Evol Syst. (2017) 48:419–45. doi: 10.1146/annurev-ecolsys-112414-054234
80. Beven K, Binley A. The future and distributed models: model clibration and uncertainty prediction. Hydrol Process. (1992) 6:279–98. doi: 10.1002/hyp.3360060305
81. Beven K. Prophecy, reality and uncertainty in distributed hydrological modelling. Adv Water Resour. (1993) 16:41–51. doi: 10.1016/0309-1708(93)90028-E
82. Ladha JK, Reddy CK, Padre AT, van Kessel C. Role of nitrogen fertilization in sustaining organic matter in cultivated soils. J Environ Qual. (2011) 40:1756–66. J Environ Qual. 40:1756–66. doi: 10.2134/jeq2011.0064
83. Veerman C, Bastioli C, Biro B, Bouma J, Cienciala E, Emmett B, et al. Caring for Soil is Caring for Life - Publications Office of the EU (2020).
84. Nunes JM, López-Piñeiro A, Albarrán A, Muñoz A, Coelho J. Changes in selected soil properties caused by 30 years of continuous irrigation under Mediterranean conditions. Geoderma. (2007) 139:321–8. doi: 10.1016/j.geoderma.2007.02.010
85. Mudge PL, Kelliher FM, Knight TL, O'Connell D, Fraser S, Schipper LA. Irrigating grazed pasture decreases soil carbon and nitrogen stocks. Glob Chang Biol. (2017) 23:945–54. doi: 10.1111/gcb.13448
86. Da Gama JT, Nunes JR, Loures L, Piñeiro AL, Vivas P. Assessing spatial and temporal variability for some edaphic characteristics of Mediterranean rainfed and irrigated soils. Agronomy. (2019) 9:132. doi: 10.3390/agronomy9030132
87. Imaz MJ, Virto I, Bescansa P, Enrique A, Fernandez-Ugalde O, Karlen DL. Soil quality indicator response to tillage and residue management on semi-arid Mediterranean cropland. Soil Tillage Res. (2010) 107:17–25. doi: 10.1016/j.still.2010.02.003
88. Apesteguia M, Virto I, Orcaray L, Bescansa P, Enrique A, Jose Imaz M, et al. Tillage effects on soil quality after three years of irrigation in northern spain. Sustainability. (2017) 9:1476. doi: 10.3390/su9081476
89. Sanderman J, Fillery IRP, Jongepier R, Massalsky A, Roper MM, MacDonald LM, et al. Carbon sequestration under subtropical perennial pastures II: carbon dynamics. Soil Res. (2013) 51:771–80. doi: 10.1071/SR12351
90. Cotrufo MF, Ranalli MG, Haddix ML, Six J, Lugato E. Soil carbon storage informed by particulate and mineral-associated organic matter. Nat Geosci. (2019) 12:989–94. doi: 10.1038/s41561-019-0484-6
91. Torn MS, Trumbore SE, Chadwick OA, Vitousek PM, Hendricks DM. Mineral control of soil organic carbon storage and turnover content were measured by horizon down to the depth at which. Nature. (1997) 389:3601–3. doi: 10.1038/38260
92. Kaiser K, Guggenberger G. Mineral surfaces and soil organic matter. Eur J Soil Sci. (2003) 54:219–36. doi: 10.1046/j.1365-2389.2003.00544.x
93. Creamer CA, Foster AL, Lawrence C, McFarland J, Schulz M, Waldrop MP. Mineralogy dictates the initial mechanism of microbial necromass association. Geochim Cosmochim Acta. (2019) 260:161–76. doi: 10.1016/j.gca.2019.06.028
94. Fernández-Ugalde O, Virto I, Barré P, Apesteguía M, Enrique A, Imaz MJ, et al. Mechanisms of macroaggregate stabilisation by carbonates: implications for organic matter protection in semi-arid calcareous soils. Soil Res. (2014) 52:180–92. doi: 10.1071/SR13234
95. Boix-Fayos C, Calvo-Cases A, Imeson AC, Soriano-Soto MD. Influence of soil properties on the aggregation of some Mediterranean soils and the use of aggregate size and stability as land degradation indicators. Catena. (2001) 44:47–67. doi: 10.1016/S0341-8162(00)00176-4
96. Bouajila A, Gallali T. Soil organic carbon fractions and aggregate stability in carbonated and no carbonated soils in Tunisia. J Agron. (2008) 7:127–37. doi: 10.3923/ja.2008.127.137
97. Six J, Bossuyt H, Degryze S, Denef K. A history of research on the link between (micro)aggregates, soil biota, and soil organic matter dynamics. Soil Tillage Res. (2004) 79:7–31. doi: 10.1016/j.still.2004.03.008
99. Lopez-Sangil L, Rovira P. Sequential chemical extractions of the mineral-associated soil organic matter: an integrated approach for the fractionation of organo-mineral complexes. Soil Biol Biochem. (2013) 62:57–67. doi: 10.1016/j.soilbio.2013.03.004
100. Romanyà J, Rovira P. An appraisal of soil organic C content in Mediterranean agricultural soils. Soil Use Manag. (2011) 27:321–32. doi: 10.1111/j.1475-2743.2011.00346.x
101. Balesdent J. The significance of organic separates to carbon dynamics and its modelling in some cultivated soils. Eur J Soil Sci. (1996) 47:485–93. doi: 10.1111/j.1365-2389.1996.tb01848.x
102. Dalal RC, Thornton CM, Cowie BA. Turnover of organic carbon and nitrogen in soil assessed from δ13C and δ15N changes under pasture and cropping practices and estimates of greenhouse gas emissions. Sci Total Environ. (2013) 465:26–35. doi: 10.1016/j.scitotenv.2013.04.101
103. Cambardella CA, Elliot ET. Carbon and nitrogen distribution in aggregates from cultivated and native grassland soils. Soil Sci Soc Am J. (1993) 57:1071–6. doi: 10.2136/sssaj1993.03615995005700040032x
104. Jastrow D. Soil aggregate formation and the accrual of particulate and mineral-associated organic matter. Soil Biol Biochem. (1996) 28:665–76. doi: 10.1016/0038-0717(95)00159-X
105. Lynch JP. Steep, cheap and deep: an ideotype to optimize water and N acquisition by maize root systems. Ann Bot. (2013) 112:347–57. doi: 10.1093/aob/mcs293
106. Kondo M, Murty MVR, Aragones DV. Characteristics of root growth and water uptake from soil in upland rice and maize under water stress. Soil Sci Plant Nutr. (2000) 46:721–32. doi: 10.1080/00380768.2000.10409137
107. Mahgoub NA, Ibrahim AM, Ali OM. Effect of different irrigation systems on root growth of maize and cowpea plants in sandy soil. Eurasian J Soil Sci. (2017) 6:374–9. doi: 10.18393/ejss.319952
108. Rowley MC, Grand S, Spangenberg JE, Verrecchia EP. Evidence linking calcium to increased organo-mineral association in soils. Biogeochemistry. (2021) 153:223–41. doi: 10.1007/s10533-021-00779-7
109. Virto I, Barré P, Burlot A, Chenu C. Carbon input differences as the main factor explaining the variability in soil organic C storage in no-tilled compared to inversion tilled agrosystems. Biogeochemistry. (2012) 108:17–26. doi: 10.1007/s10533-011-9600-4
110. Keel SG, Anken T, Büchi L, Chervet A, Fliessbach A, Flisch R, et al. Loss of soil organic carbon in Swiss long-term agricultural experiments over a wide range of management practices. Agric Ecosyst Environ. (2019) 286:654. doi: 10.1016/j.agee.2019.106654
111. Unkovich M, Baldock J, Forbes M, Chapter Chapter 5 - Variability in Harvest Index of Grain Crops and Potential Significance for Carbon Accounting: Examples from Australian Agriculture. In: Advances in Agronomy. Vol 105. San Diego, CA: Academic Press (2010). p. 173–219. doi: 10.1016/S0065962113(10)05005964
112. Molon M, Boyce JI, Arain MA. Quantitative, nondestructive estimates of coarse root biomass in a temperate pine forest using 3-D ground-penetrating radar (GPR). J Geophys Res Biogeosci. (2017) 122:80–102. doi: 10.1002/2016JG003518
Keywords: carbon sequestration, irrigation, calcareous soils, maize, soil organic C modeling, stable carbon isotopes
Citation: Antón R, Derrien D, Urmeneta H, van der Heijden G, Enrique A and Virto I (2022) Organic Carbon Storage and Dynamics as Affected by the Adoption of Irrigation in a Cultivated Calcareous Mediterranean Soil. Front. Soil Sci. 2:831775. doi: 10.3389/fsoil.2022.831775
Received: 08 December 2021; Accepted: 18 February 2022;
Published: 21 March 2022.
Edited by:
Carsten W. Mueller, University of Copenhagen, DenmarkReviewed by:
Maren Oelbermann, University of Waterloo, CanadaCopyright © 2022 Antón, Derrien, Urmeneta, van der Heijden, Enrique and Virto. This is an open-access article distributed under the terms of the Creative Commons Attribution License (CC BY). The use, distribution or reproduction in other forums is permitted, provided the original author(s) and the copyright owner(s) are credited and that the original publication in this journal is cited, in accordance with accepted academic practice. No use, distribution or reproduction is permitted which does not comply with these terms.
*Correspondence: Iñigo Virto, aW5pZ28udmlydG9AdW5hdmFycmEuZXM=
Disclaimer: All claims expressed in this article are solely those of the authors and do not necessarily represent those of their affiliated organizations, or those of the publisher, the editors and the reviewers. Any product that may be evaluated in this article or claim that may be made by its manufacturer is not guaranteed or endorsed by the publisher.
Research integrity at Frontiers
Learn more about the work of our research integrity team to safeguard the quality of each article we publish.