- 1State Key Laboratory of Atmospheric Boundary Layer Physics and Atmospheric Chemistry (LAPC), Institute of Atmospheric Physics, Chinese Academy of Sciences, Beijing, China
- 2Institute for Meteorology and Climate Research, Atmospheric Environmental Research (IMK-IFU), Karlsruhe Institute of Technology, Garmisch-Partenkirchen, Germany
- 3Beijing Meteorological Observation Center, Beijing Meteorological Service, Beijing, China
- 4State Key Laboratory of Vegetation and Environmental Change (LVEC), Institute of Botany, Chinese Academy of Sciences, Beijing, China
Nitrous oxide (N2O) emissions are highly variable due to the complex interaction of climatic and ecological factors. Here, we obtained in-situ annual N2O emission flux data from almost 180 peer-papers to evaluate the dominant drivers of N2O emissions from forests and unfertilized grasslands at a global scale. The average value of N2O emission fluxes from forest (1.389 kg Nha-1yr-1) is almost twice as large as that from grassland (0.675 kg Nha-1yr-1). Soil texture and climate are the primary drivers of global forest and grassland annual N2O emissions. However, the best predictors varied according to land use and region. Soil clay content was the best predictor for N2O emissions from forest soils, especially in moist or wet regions, while soil sand content predicted N2O emissions from dry or moist grasslands in temperate and tropical regions best. Air temperature was important for N2O emission from forest, while precipitation was more efficient in grassland. This study provides an overall understanding of the relationship between natural N2O emissions and climatic and environmental variables. Moreover, the identification of principle factors for different regions will reduce the uncertainty range of N2O flux estimates, and help to identify region specific climate change mitigation and adaptation strategies.
1 Introduction
Nitrous oxide (N2O) is the third most important long-lived trace greenhouse gas after CO2 and CH4. The average atmospheric N2O concentration has reached to 333.2 ppb in 2020, which is an increase of 23% with respect to pre-industrial levels (1). In natural ecosystems like forests and grasslands, N2O is primarily produced during re-mineralization of organic matter via the soil microbial processes of nitrification and denitrification (2, 3). Soils are the largest natural source of N2O, accounting for about 58% of the total natural source (4).
Natural soil N2O emissions are mainly controlled by climatic and ecological variables (5). Carbon to nitrogen ratios (C/N) have been shown to be a good predictor for N2O emissions from organic soils (6–9), while N2O emissions from grassland soils were positively correlated with soil clay content (Clay) and temperature, but negatively correlated with annual precipitation (10). In Brazil, soil pH, soil organic carbon (SOC), and Clay were good predictors for N2O emissions from unfertilized soils (11). Araujo et al. (2021) showed that annual temperature and soils properties (such as phosphorous, C/N ratio, clay and sand content, soil nitrate contents) were the main drivers for the spatial distribution of N2O emissions from grassland and forest soils in Argentina (12). However, the principle factors dominating N2O emissions have been shown to vary strongly among different regions (13), with the key drivers of global forest and grassland N2O emissions still not clear.
Precise estimates of the global N2O emissions from forests and grasslands are difficult to obtain owing to the high spatiotemporal heterogeneity of N2O flux caused by the complex production and emission processes. Nevertheless, process-based models, with detailed description of the N cycling process exist and are considered powerful tools for estimating regional or global N2O emissions (14). Various models have been developed to simulate N2O emissions from soils, such as DNDC (15), LPJ (16), and DLEM (17). However, few process-based models have been validated against in situ observations across a wider domain with various climatic and ecological conditions. Consequently, it’s difficult to verify the reliability of the regional or global estimates, which always have a high uncertainty (18, 19). Although the reliability of regional estimates is gradually reduced with a better understanding of the N cycling process (4, 5, 13, 20, 21), the principle factors and the feedback mechanisms of N2O emissions at regional scale are still highly uncertain (4, 5, 20, 22). Thus, identifying robust drivers of N2O emissions for different ecological zones is critical for reducing the uncertainty of global estimates.
Field data-oriented analysis can be an effective tool to better understand regulating factors of N2O emission from different ecosystems, if sufficient data is available (13). There have been data-oriented studies on forest and grassland N2O emissions at global scale (13, 23, 24). Kim et al. (2013) found that only annual temperature had a significant correlation with N2O emissions from natural soils (24). Zhuang et al. (2012) used the Artificial Neural Network (ANN) model to show that precipitation was the most sensitive variable for natural N2O emissions (13). However, both of them didn’t take the effect of soil texture into account, which also is known to be an important factor for natural N2O emissions (25). Stehfest et al. (2006) used linear mixed-effects model (LMM) to evaluate the effects of all possible continuous or discrete variables on N2O emissions. Finally, it verified that SOC, pH, bulk density, drainage, and vegetation type have a significant influence on N2O emissions from natural soils (23). LMMs are now widely used for analyzing datasets from multiple experiments (26). However, the observation length of 57% of the data used was less than 50 days, and only 12% of the data came from observations over more than 300 days. Thus, these datasets fail to accurately represent conditions over the entire vegetation cycle, resulting in large uncertainties. Since 1980s, a large number of long-term field N2O experiments has been conducted throughout the world, providing sufficient representative data for field data-oriented analysis (27, 28).
Consequently, the aim of this study was to assess whether the magnitude of annual N2O emissions from forest and unfertilized grasslands sites at global scale can be related to specific environmental factors (i.e. vegetation, soil pH, soil carbon content, soil nitrogen content, soil C/N ratio, soil bulk density, soil clay content, soil sand content, air temperature, precipitation, and N deposition etc.); and to explore whether the robust predictors are different for different land use and regions. To address these objectives, in-situ field measurements of annual N2O emissions with detailed reports of soil properties were collected from forest and unfertilized grassland sites covering a wide range of environmental conditions that varied from 44.83S to 64.27N in the latitude direction at a global scale.
2 Materials and method
2.1 Data sources
Data from field experiments were extracted from peer-reviewed scientific articles that reported N2O emission from forest or grassland soils across the world. The studies in scope of the analysis were searched by ISI Web of Science and China Knowledge Resource Integrated Database (CNKI) with key words of “nitrous oxide”, “denitrification”, “nitrification”, and “forest” and “grassland”. We compiled datasets following the criteria of: (a) the data were obtained by in-situ observational experiments; (b) the measurements were conducted with the static chamber technique. Those obtained with eddy covariance observation systems were excluded to avoid bias caused by differences in observational systems; (c) the experiments lasted at least one year, while at sites with a longer freezing period the time duration of the experiments could reduce to 8 months; (d) studies reporting soilN2O uptake reported were excluded.
Beside N2O fluxes, site information (such as experimental period, measurement frequency, longitude and latitude, elevation, and vegetation type), and other environmental information, such as climate (including mean annual temperature (MAT) and precipitation (MAP)), soil properties (including pH, soil organic carbon concentration (SOC), soil total nitrogen (TN), bulk density (BD), and sand and clay fractions in mineral soil), and N deposition (N_dep) were also obtained from relevant studies at the same site. In addition, MAT and MAP during the experimental period of each study were extracted from the Climatic Research Unit Time-Series (29) (CRU TS 3.22). The distributions of these factors were showed in Figures S1, S2.
Totally, 556 sets of N2O emission flux measurements were collected from about 180 scientific papers (Appendix 1). Among them, 355 datasets were of forests at 161 sites in 32 countries (Figure 1). The other 201 datasets were of grassland N2O emission fluxes at 81 sites in 25 countries. The observation sites distributed worldwide, and concentrated in Southern Asia, Europe, North America, and South America (Figure 1).
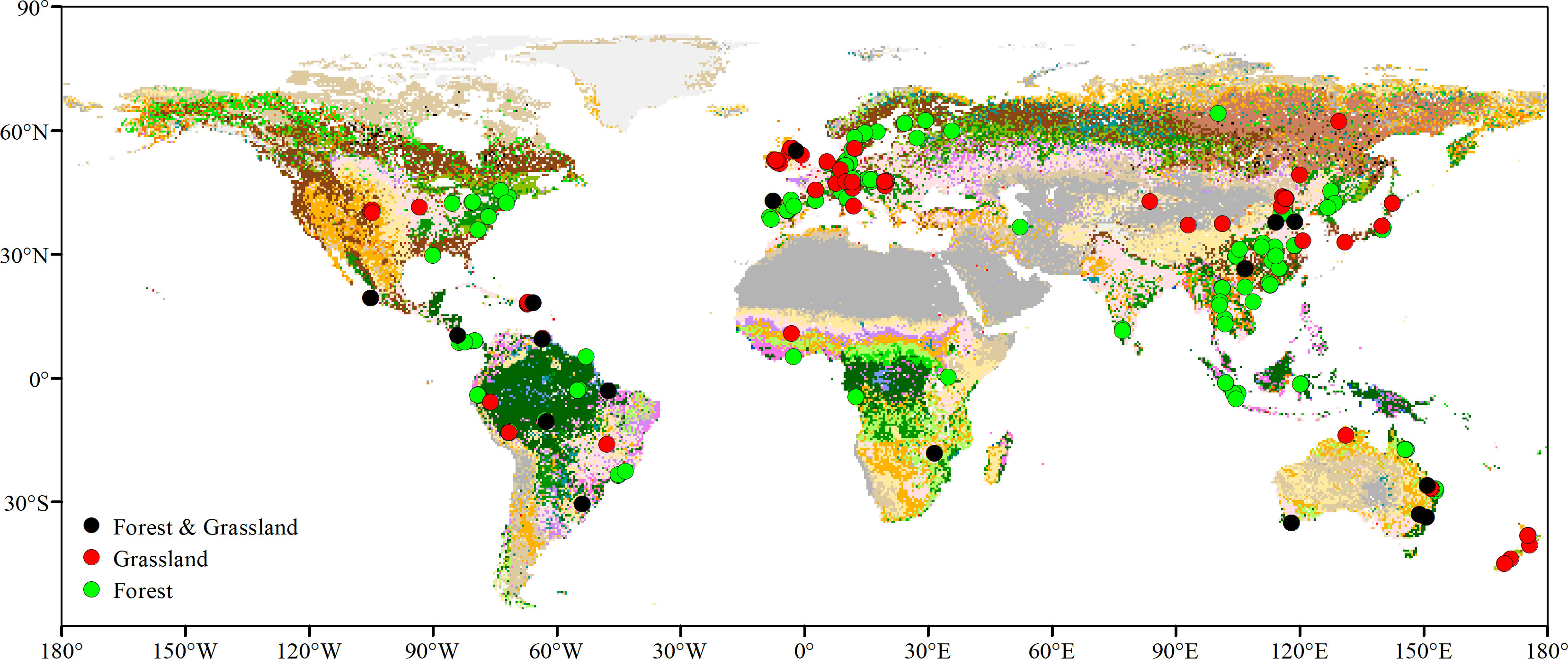
Figure 1 Global distribution of the experimental sites with measurements of annual N2O emission fluxes. The map was generated using ESRI ArcGIS 10.0 (URL: http://www.esri.com), and the coordinate system is WGS84. The base image was acquired and modified from GLC2000 database (30) (https://forobs.jrc.ec.europa.eu/products/glc2000/products.php).
2.2 Data preprocessing
We unified the metric unit of the N2O fluxes into kg N2O-N ha-1yr-1 from the diverse metrics reported in different studies. In case that the annual N2O emission flux was not given in the literature, it was calculated by summing up the time-weighted flux measurements in each year. Both dry and wet seasons in tropics and growing and non-growing seasons in temperate zones were included in the calculation of annual fluxes; for sites in high latitudes such as Siberia, the annual flux only included the cumulative fluxes of growing season.
The soil property was featured by pH, SOC, BD, TN, C/N, sand and clay content in this study soil depths (e.g., 0-5cm, 0-10cm, 0-20cm etc.) of different sources was normalized to 0-10cm. The linear regression functions between different layers for SOC, BD, and TN have been developed in our previous study, and we used the same transfer function to do the standardization of soil properties (19). Soil texture was classified into three groups: coarse, medium and fine soils. Soils with sand content no less than 65% and clay content less than 18% were defined as coarse soils. Soils with clay content no less than 35% belonged to the fine soil group (31). The climate zones were defined by temperature categories with four groups (cold temperate, warm temperate, subtropical, and tropical), and Dry-Wet (dry, moist, wet) conditions with three groups according to IPCC climate zones (32). The global vegetation was also categorized into five groups: evergreen coniferous forest, evergreen broadleaf forest, mixed coniferous and broadleaf forest, deciduous forest, and grasslands.
2.3 Statistical analysis
The normality of the N2O emission data were tested with Shapiro-Wilk test (33). We conducted a Spearman rank correlation analysis to identify edaphic and climatic factors (i.e. pH, SOC, TN, BD, sand, Clay, MAT, MAP, and N_dep) influencing N2O emissions. The non-parametric Kruskal-Wallis one-way ANOVA test was used to evaluate the variances across different groups of soil textures, climate zones, and vegetation in forest and grassland ecosystems.
LMMs (Linear mixed-effect model) analysis was applied to quantify the principle factors that correlate with N2O emissions. Study site was introduced into the model as a random factor, because clustering replicates by location could introduce spatial autocorrelation (34). Furthermore, given the positive skew distribution of forest and grassland N2O emissions and in preparation for LMM development, both (forest and grassland N2O emissions) were transformed by Box-Cox transformation (35). Finally, power transformation was made with the conversion coefficient 0.15, 0.10 for forest and grassland, respectively (Figure 2). The fit and accuracy assessment of the LMM were reported through model parameter significance test together with Akaike information criterion (AIC) and Bayesian information criterion (BIC) (36). Smaller AIC and BIC indicate better model performance.
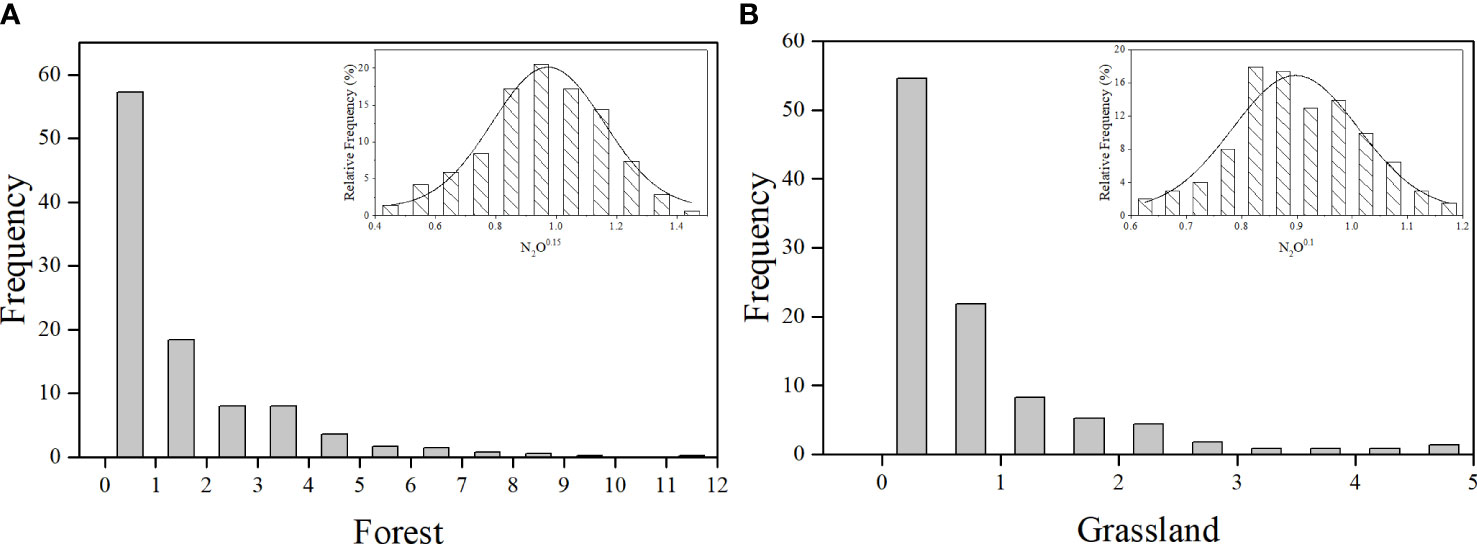
Figure 2 Frequency histogram of N2O emissions in forest (A) and grassland (B). The subgraph in the upper right is the distribution of N2O emissions after BOX-COX transformation.
3 Result
3.1 N2O emissions from global forest and grassland soils
According to the observation dataset, the average annual N2O emissions of global forest and grassland soils was 1.130 kg N2O-N ha-1yr-1, with a maximum value of 11.388 kg N2O-N ha-1yr-1, which occurred in a heavy textured (60% clay) lowland moist Amazonian forest (37). The annual N2O emission flux from global forest soils and grassland soils varied from 0.003 to 11.388 kg N2O-N ha-1yr-1, 0.007 to 4.800 kg N2O-N ha-1yr-1, respectively (Table 1). 58% of forest N2O emission and 79% of grassland N2O emission data fall within 0 to 1.0 kg N2O-N ha-1yr-1. The flux distributions of both ecosystems were non-normal (Figure 2). The power transformation coefficients were 0.15, 0.10 for forests and grasslands, respectively. The non-parametric Kruskal-Wallis variance analysis showed that there was a significant difference of annual N2O emissions between the two ecosystems. The median of forest annual N2O emission fluxes was significantly higher than that of grasslands (Table 1). Ecosystem type could explain 5% (y = 0.72xecosystem -0.04, R2 = 0.05, p<0.01; In the equation, Grassland = 1, and Forest =2) of the changes in annual N2O emission fluxes in natural soils.
3.2 The influence of environmental factors on N2O emissions
Spearman correlation analysis indicated that the annual N2O emissions from both forests and grasslands was positively correlated with Clay, MAT, and MAP, and had a significant negative correlation with Sand (Table 2). In forests, the annual N2O emissions had a significant negative relationship with pH, while in grassland the annual N2O emissions were positively related with C/N (Table 2). Among all variables, though Clay and MAP were targeted by Spearman correlation analysis as the two most important factors of N2O emissions in forests, the stepwise regression analysis selected MAT, Clay and N deposition instead of Clay and MAP. In grasslands, only MAP was chosen for the stepwise regression analysis of annual N2O emissions.
In forests, the median and average value of N2O emissions in the (sub-) tropics were significantly higher than that in temperate zones (Figure 3A). In grasslands, the median and average value of N2O emissions in tropical zones were significantly higher than that in temperate and subtropical zones (Figure 3B). The variance of annual N2O emission fluxes were always high in the tropics (Figures 3A, B). The average N2O emissions from grassland soils in global moist zones were significantly higher than in dry zones (Figure 3D). However, in forests there was no significant difference of N2O emissions between arid, moist and wet zones (Figure 3C). In summary, the principle climatic variable for N2O emissions differed between forests and grasslands.
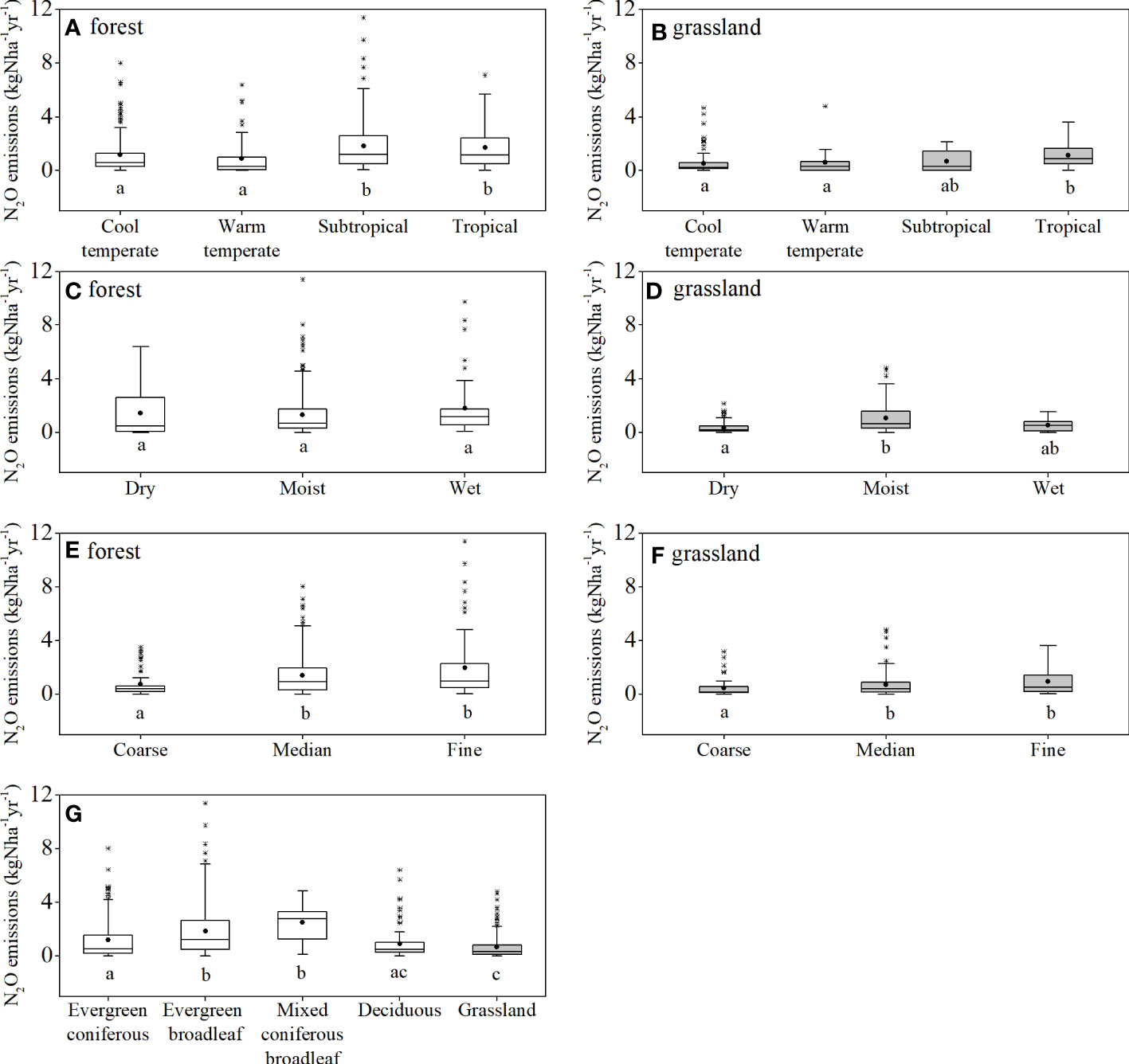
Figure 3 Box plot of N2O emissions in different climate zone, dry-wet, texture, and vegetation types. (A, C, E) are from forest, and (B, D, F) are from grassland. For (G), the blank boxes represent forest, and the grey box represents grassland. a, b, and c represent the significant different between the median value variances. Solid circles represent averages, lines within the boxes indicated medians, and upper and lower edges of the boxes represent the 25th and 75th percentiles. The stars are outliers.
Both the statistic median and mean of the N2O emissions from coarse texture soils were significantly lower than that of the medium and fine soils (Figures 3E, F). The finer the soil texture, the higher the magnitude of N2O emission. In addition, mixed coniferous and broadleaf forest and evergreen broadleaf forests had the relatively higher N2O emissions than deciduous forests and grasslands (Figure 3G).
3.3 LMMs of forest and grassland N2O emissions with different variables
For forest N2O emissions, the LMMs were tried by AIC and BIC criteria. We retained the model parameters by their statistical significance (Table 3). The pH, C/N, Clay, Sand, MAT, and MAP were all significant (P < 0.05) in LMMs, and the best-fit factor was Clay, which had the minimum AIC and BIC (Table 3). The importance of these variables ranked: Clay > Sand > MAT > C/N > MAP > pH. The additive effects of multi-variables were also tested, and four variables, i.e. pH, C/N, Clay and MAT were selected into the model, and the explanation of the fixed effect to the forest N2O emissions was 7.8% (P < 0.05) (Table 3). In addition, the best-fit model with mixed additive and two-way interactions (AIC=-335.3) could explain 11.1% (P < 0.05) of the forest N2O emission change. Furthermore, the effect significance of the variables differed among regions. For example, the positive effect of Clay on forest N2O emissions was more significant in fine textured soils in tropical or moist regions, while the negative effect of C/N was more significant in coarse soils of temperate or moist regions (Table S1).
For grassland N2O emissions, we ranked the influence of environmental covariates based on AIC and BIC. The result showed that, Sand was the most important continuous variable that had the best-fit LMM model of grassland N2O emission (Table 3). The importance rank was Sand > Clay > MAT, which indicated that soil texture was the principle factors for the spatial heterogeneity of global grassland N2O emission. In addition, by comparing the numerous models with additive or interaction effect of multi-variables LMM, only Sand was still the best-fit model. However, the explanation of the fixed effect of Sand was only 1.7% (P < 0.01), while the explanation of the fixed effect of MAP was 11.3% (P = 0.11) (Table 3). Sand had a significant negative effect on grassland N2O emission from temperate soils, tropical soils, or dry zone soils (Table S1). MAP mainly had a significant positive effect on grassland N2O emission from fine texture soils (Table S1).
The LMMs with continuous variables quantitatively reflect the effect of environmental factors on N2O emissions. In addition, the influences of categorical variables, such as climatic temperature zone, vegetation, soil texture, and dry-wet, on annual N2O emissions were also tested with LMMs (Table 4). Given that these categorical variables were classified based on the information of spatial distribution, we could directly obtain the primary factors that caused the spatial difference of N2O emissions. By comparing the AIC and BIC values, the variable with the best-fit LMM was the climatic temperature zone and dry-wet for forest and grassland, respectively (Table 4). But in contrast, the dry-wet and climatic temperature zone was not significant for global forest and grassland N2O emissions, respectively (Table 4). Soil texture was the second best-fit variable for both forest and grassland. The 2-way interaction LMMs indicated that the interaction of climate zone and soil texture (AIC=-299.4, BIC=-245.5), dry-wet and soil texture (AIC=-428.0, BIC=-395.0) had minimum values of AIC and BIC for forest and grassland, respectively (Table 4).
4 Discussion
The primary measurement systems for N2O emission are eddy covariance systems and the closed chamber technique (38, 39). Among those, the static chamber method has been widely used by collecting air samples in chambers and then analyzing with a gas chromatograph equipped with an electronic capture detector (40). Given that there is no systematic research on the difference between the two observation systems (41, 42), our study only considered the N2O emission data that observed by the static chamber method in this study. In addition, only studies that reported emissions were considered, while those reporting soil N2O uptake were neglected because N2O uptake in natural soils is very weak and reported uptake rates often range within the detection limit of the measuring system. Moreover, highest uptake rates are generally found in wetland and peatland ecosystems (43), while in forest and grassland soils these are only of minor importance. Furthermore, the limited observations of N2O uptake in forest and grassland soils are typically very episodic, driven by changes of during the year (44), while our analysis focuses on variations in annual emissions.
In this study, spearman analysis showed that the predominant variables (i.e. Clay, Sand, MAT, and MAP) that affected N2O emissions were the same for both forest and grassland (Table 2). These factors also have been proven to have significant effects on N2O emissions by the other studies (13, 24, 45). Moreover, the N2O emissions from both forest and grassland had a negative relationship with pH, which was in consistent with previous studies (46, 47). But only the correlation in forest was significant (Table 2), most likely be due to the fact that forest soils are more acidic than grassland soils (48) (Figure S1A, FigureS2A). Soil C/N has been reported as good predictor for N2O emissions from soils, especially for organic soils, with generally negative effects on N2O emissions (8). However, our study indicated a positive effect of soil C/N on N2O emissions (Table 2), which can probably be explained by the fact that our analysis only investigated a linear relationship, while the relationship between N2O emissions and soil C/N is probably better explained by a parabola, and we only found the same negative effect in temperate zone (Table S1). A recent meta-analysis also found a Gaussian curve relationship between annual N2O fluxes and C/N ratio in organic soils, which could explain the negative relationship when C/N was above 18 (9). However, soil C/N for most sites in our dataset was lower than 18 (Figure S1E, Figure S2E). In addition, atmospheric N deposition had no significant effect on annual N2O emissions from both forest and grassland (Table 2). Because atmospheric N depositions of most sites were less than 30kg Nha-1yr-1, which presumably was too low to significantly affect N2O emissions.
Soil N2O productions and emissions result from the synergistic effect of temperature, substrate availability, microbial community, and gas transport process etc. Due to the sensitivity of N2O production to these factors, N2O emissions are highly heterogeneous in time and space, even at small scales (49). Our analysis showed that soil sand and clay content were important drivers that affected global N2O emissions from both forest and grassland soils (Table 2). Soils with heavy clay content had higher N2O emissions, and this positive effect was more significant in moist and wet regions where usually highest emissions are observed (Table 2; Table S1). This generally agrees with previous reports (37, 50–53) and can be explained by the larger volume of small pores in finer soil, making it more conductive to denitrification and N2O production (25, 54). In addition, the clay particles are favorable for N mineralization rates (55), but not temperature sensitivity of N2O emissions (56–59). Furthermore, our results showed that LMM including Clay or Sand for forest and grassland, respectively, had the minimum AIC (Table 3). That is to say, Clay is the best predictor for forest N2O emissions, while Sand is the best predictor for grassland. However, there is currently no study on the importance of these two factors for different ecosystems at a global scale. We infer that this might be due to general differences in soil type of the two ecosystems. Because in our dataset, about 30% of grassland sites were classified as coarse textured soil, while only 16% of forest sites belonged to coarse groups (Table S2). This results in Sand being a more effective predictor for N2O emissions from grassland. A similar relationship was also found between mineral-associated organic carbon and soil sand content, which was also more effective in grassland than in forest (60).
The seasonal dynamics of soil temperature and soil moisture could reflect the seasonal course of N2O (61), while the annual dynamic of temperature and precipitation influence the spatial distribution and intern-annual dynamics of N2O (4). Spearman correlation analysis showed that the explanations of MAT and MAP on N2O emissions were relatively high (Table 2). Compared with temperature, precipitation is thought to be more important for N2O emissions from natural ecosystems (5, 13, 62). In this study, the LMMs’ result showed that precipitation was the most effective factor for global grassland N2O emission, although the fixed linear relationship was only significantly in dry and moist grassland (Table S1). The average N2O emissions from wet tropical grassland was significantly lower than that from moist grassland (Table S2). However, there was no significant difference of N2O emissions between the two zones for forests (Figure 3C). That can probably be attributed to the higher infiltration capacity of grassland soils resulting in more pronounced wetting and drying cycles and thus a stronger negative response of N2O emissions to rainfall compared to the wet or flooded soil conditions in the finer textured forest soils (51, 63). Furthermore, we found that temperature was more important than precipitation for N2O emissions from forest (Table 3). This is because of the significant direct or indirect effect of temperature on the soil enzyme activities and the resulting supply of N substrate of nitrification and denitrification (64). Increasing temperature could significantly favor N2O emissions by a strong biotic regulation via ammonia-oxidizing bacteria amoA gene abundance, while the effect of rainfall reduction was not significant (65). However, some study found that increased or decreased precipitation could promote or suppress N2O emissions to varying degrees (66). Sometimes, a decreasing soil water content could offset the positive effect of warming on N2O emissions (67). So, there are still large uncertainties regarding the feedback of N2O emission to the additive and interactive effects of temperature and precipitation in regional scale.
Up to now, estimates of simulation studies for N2O emissions from global forest and grassland soils vary from 2.61 Tg N2O-Nyr-1 to 11.48 Tg N2O-Nyr-1 (4, 5, 13, 20, 21). Using the results of our data analysis we calculated global N2O emissions for forest and grasslands by extrapolating average emissions for different groups, like climate, texture or vegetation classes without considering the effects of human management. According to the independent classify variable, we estimated that the N2O emissions form forests and grasslands were 5.22-5.55 Tg N2O-Nyr-1, 1.45-1.93 Tg N2O-Nyr-1 (Table S2), respectively: This estimate is at the higher end of the estimates of Zhang et al. (2019) (Forest:3.62 ± 0.16 Tg N2O-Nyr-1, grassland:1.40 ± 0.03 Tg N2O-Nyr-1) (5). If we use the interaction variables to classify, the N2O emissions form forests and grasslands were 4.11 Tg N2O-Nyr-1, 1.06 Tg N2O-Nyr-1 (Table S3), respectively, which was similar to the result of Tian et al. (2020) (natural soils, 5.6 Tg N2O-Nyr-1) (4). Although, these estimates have large uncertainties, they are well within the range of current estimates of global scale N2O emissions, showing that the difference of key factors of N2O emissions in different ecosystems or regions (Table 3; S1) can be a promising approach to estimate N2O from these ecosystems.
5 Conclusion
This study highlighted that natural forest soils are a strong natural source of N2O, with an average annual emission flux almost double that of grassland soils. For both ecosystems, soils with a high clay content in moist tropical climates appear to be a hotspot of N2O. In addition, grasslands in moist region have relatively higher N2O emissions than the other regions. Soil texture, annual mean temperature and precipitation are the most important factors that influence forest and grassland N2O emissions at a global scale. However, the best predictors varied according to land use and region. While clay content was the best predictor for N2O emissions from forest soils, especially in moist or wet regions, sand content predicted N2O emissions from dry or moist grasslands in temperate and tropical regions best. However, MAP, with a significant positive effect on N2O emissions from grassland soils in dry and moist regions, had the highest R2 of fixed effected of grassland N2O emissions.
Although the principle factors of soil N2O emission are varying in different regions, these simple statistical models can help to derive global estimates for specific ecosystems when no detailed data for process-based simulation models is available. Moreover, this study provides an overall view of them. According to the result of this study, the process-based model estimates could do calibration and validation with more representative sites that capture different primary factors in different regions, which is benefit to increase the reliability and spatial applicability of the model. In addition, in order to accurately evaluate the estimates’ uncertainty, the sensitivity differences of the principle factors in various regions also should be taken into account in future studies.
Data availability statement
The original contributions presented in the study are included in the article/Supplementary material. Further inquiries can be directed to the corresponding author.
Author contributions
LJ conducted all literature searches, data analyses, and writing of the manuscript. WZ helped with the project design. QZ and YT participated in the soil and climate information collection. WJ, SC, and TL all provided major feedback, comments, and suggestions in terms of both the overall direction and specific changes to the manuscript and associated literature searches and analyses. All authors contributed to the article and approved the submitted version.
Funding
This research was jointly supported by National Natural Science Foundation of China (Grant No. 41901069), China High-Resolution Earth Observation System (30-Y30F06-9003-20/22), the ‘China and Germany Postdoctoral Exchange Program’ jointly funded by the Office of the China Postdoctoral Council of the Ministry of Human Resources and Social Security and Institute for Meteorology and Climate Research (IMK-IFU), Karlsruhe Institute of Technology, and the German Federal Ministry of Education and Research (BMBF) under the “Make our Planet Great Again – German Research Initiative”, grant number 306060, implemented by the German Academic Exchange Service (DAAD).
Conflict of interest
The authors declare that the research was conducted in the absence of any commercial or financial relationships that could be construed as a potential conflict of interest.
Publisher’s note
All claims expressed in this article are solely those of the authors and do not necessarily represent those of their affiliated organizations, or those of the publisher, the editors and the reviewers. Any product that may be evaluated in this article, or claim that may be made by its manufacturer, is not guaranteed or endorsed by the publisher.
Supplementary material
The Supplementary Material for this article can be found online at: https://www.frontiersin.org/articles/10.3389/fsoil.2022.1094177/full#supplementary-material
References
1. WMO. WMO greenhouse gas bullletin. (2021). Available at: https://library.wmo.int/index.php?lvl=notice_display&id=21975#.Y48i5nZBzWh
2. Voss M, Bange HW, Dippner JW, Middelburg JJ, Montoya JP, Ward B. The marine nitrogen cycle: recent discoveries, uncertainties and the potential relevance of climate change. Philos Trans R Soc B Biol Sci (2013) 368(1621):20130121. doi: 10.1098/rstb.2013.0121
3. Canadell JG, Monteiro PMS, Costa MH, Cunha LCD, Cox PM, Eliseev AV, et al. Global carbon and other biogeochemical cycles and feedbacks. (2021) (Cambridge, United Kingdom and New York, NY, USA: Cambridge University Press) 673–816. doi: 10.1017/9781009157896.007
4. Tian H, Xu R, Canadell JG, Thompson RL, Winiwarter W, Suntharalingam P, et al. A comprehensive quantification of global nitrous oxide sources and sinks. Nature (2020) 586(7828):248–56. doi: 10.1038/s41586-020-2780-0
5. Zhang K, Zhu Q, Liu J, Wang M, Zhou X, Li M, et al. Spatial and temporal variations of N2O emissions from global forest and grassland ecosystems. Agric For Meteorol (2019) 266–267:129–39. doi: 10.1016/j.agrformet.2018.12.011
6. Ernfors M, von Arnold K, Stendahl J, Olsson M, Klemedtsson L. Nitrous oxide emissions from drained organic forest soils–-an up-scaling based on C:N ratios. Biogeochemistry (2007) 84(2):219–31. doi: 10.1007/s10533-007-9123-1
7. Leppelt T, Dechow R, Gebbert S, Freibauer A, Lohila A, Augustin J, et al. Nitrous oxide emission budgets and land-use-driven hotspots for organic soils in Europe. Biogeosciences (2014) 11(23):6595–612. doi: 10.5194/bg-11-6595-2014
8. Leifeld J. Distribution of nitrous oxide emissions from managed organic soils under different land uses estimated by the peat C/N ratio to improve national GHG inventories. Sci Total Environ (2018) 631–632:23–6. doi: 10.1016/j.scitotenv.2018.02.328
9. Yao Z, Yan G, Ma L, Wang Y, Zhang H, Zheng X, et al. Soil C/N ratio is the dominant control of annual N2O fluxes from organic soils of natural and semi-natural ecosystems. Agric For Meteorol (2022) 327(109198):1–11. doi: 10.1016/j.agrformet.2022.109198
10. Chatskikh D, Olesen JE, Berntsen J, Regina K, Yamulki S. Simulation of effects of soils, climate and management on N2O emission from grasslands. Biogeochemistry (2005) 76(3):395–419. doi: 10.1007/s10533-005-6996-8
11. Meurer KHE, Franko U, Stange CF, Rosa JD, Madari BE, Jungkunst HF. Direct nitrous oxide (N2O) fluxes from soils under different land use in Brazil–a critical review. Environ Res Lett (2016) 11(2):023001. doi: 10.1088/1748-9326/11/2/023001
12. Araujo PI, Piñeiro-Guerra JM, Yahdjian L, Acreche MM, Alvarez C, Alvarez CR, et al. Drivers of N2O emissions from natural forests and grasslands differ in space and time. Ecosystems (2021) 24(2):335–50. doi: 10.1007/s10021-020-00522-7
13. Zhuang Q, Lu Y, Chen M. An inventory of global N2O emissions from the soils of natural terrestrial ecosystems. Atmos Environ (2012) 47:66–75. doi: 10.1016/j.atmosenv.2011.11.036
14. Tian Z, Niu Y, Fan D, Sun L, Ficsher G, Zhong H, et al. Maintaining rice production while mitigating methane and nitrous oxide emissions from paddy fields in China: Evaluating tradeoffs by using coupled agricultural systems models. Agric Syst (2018) 159:175–86. doi: 10.1016/j.agsy.2017.04.006
15. Li C, Mosier A, Wassmann R, Cai Z, Zheng X, Huang Y, et al. Modeling greenhouse gas emissions from rice-based production systems: Sensitivity and upscaling. Glob Biogeochem Cycles 18(GB1043):1–19 . doi: 10.1029/2003GB002045
16. Sitch S, Smith B, Prentice IC, Arneth A, Bondeau A, Cramer W, et al. Evaluation of ecosystem dynamics, plant geography and terrestrial carbon cycling in the LPJ dynamic global vegetation model. Glob Change Biol (2003) 9:161–85. doi: 10.1046/j.1365-2486.2003.00569.x
17. Tian H, Chen G, Lu C, Xu X, Ren W, Zhang B, et al. Global methane and nitrous oxide emissions from terrestrial ecosystems due to multiple environmental changes. Ecosyst Health Sustain (2010) 1(1):1–20. doi: 10.1890/EHS14-0015.1
18. Huang Y, Yu Y, Zhang W, Sun W, Liu S, Jiang J, et al. Agro-c: A biogeophysical model for simulating the carbon budget of agroecosystems. Agric For Meteorol (2009) 149(1):106–29. doi: 10.1016/j.agrformet.2008.07.013
19. Yu L, Huang Y, Zhang W, Li T, Sun W. Methane uptake in global forest and grassland soils from 1981 to 2010. Sci Total Environ (2017) 607–608:1163–72. doi: 10.1016/j.scitotenv.2017.07.082
20. Xu R, Prentice IC, Spahni R, Niu HS. Modelling terrestrial nitrous oxide emissions and implications for climate feedback. New Phytol (2012) 196(2):472–88. doi: 10.1111/j.1469-8137.2012.04269.x
21. Tian H, Chen G, Lu C, Xu X, Ren W, Banger K, et al. Global land-atmosphere exchange of methane and nitrous oxide: Magnitude and spatiotemporal patterns. Biogeochem: Air - Land Exchange (2013) 10:19811–65. doi: 10.5194/bgd-10-19811-2013
22. Zhang F, Qi J, Li FM, Li CS, Li CB. Quantifying nitrous oxide emissions from Chinese grasslands with a process-based model. Biogeosciences (2010) 7(6):2039–50. doi: 10.5194/bg-7-2039-2010, 2010
23. Stehfest E, Bouwman L. N2O and NO emission from agricultural fields and soils under natural vegetation: Summarizing available measurement data and modeling of global annual emissions. Nutr Cycl Agroecosyst (2006) 74(3):207–28. doi: 10.1007/s10705-006-9000-7
24. Kim DG, Giltrap D, Hernandez-Ramirez G. Background nitrous oxide emissions in agricultural and natural lands: A meta-analysis. Plant Soil (2013) 373(1–2):17–30. doi: 10.1007/s11104-013-1762-5
25. Pelster DE, Chantigny MH, Rochette P, Angers DA, Rieux C, Vanasse A. Nitrous oxide emissions respond differently to mineral and organic nitrogen sources in contrasting soil types. J Environ Qual (2012) 41(2):427–35. doi: 10.2134/jeq2011.0261
26. Klein CAM, Alfaro MA, Giltrap D, Topp CFE, Simon PL, Noble ADL, et al. Global research alliance n2O chamber methodology guidelines: Statistical considerations, emission factor calculation, and data reporting. J Environ Qual (2020) 49(5):1156–67. doi: 10.1002/jeq2.20127
27. Keller M, Reiners WA. Soil-atmosphere exchange of nitrous oxide, nitric oxide, and methane under secondary succession of pasture to forest in the Atlantic lowlands of Costa Rica. Glob Biogeochem Cycles (1994) 8(4):399–409. doi: 10.1029/94GB01660
28. Becker H, Uri V, Aosaar J, Varik M, Mander Ü, Soosaar K, et al. The effects of clear-cut on net nitrogen mineralization and nitrogen losses in a grey alder stand. Ecol Eng (2015) 85:237–46. doi: 10.1016/j.ecoleng.2015.10.006
29. Harris I, Jones PD, Osborn TJ, Lister DH. Updated high-resolution grids of monthly climatic observations - the CRU TS3.10 dataset: UPDATED HIGH-RESOLUTION GRIDS OF MONTHLY CLIMATIC OBSERVATIONS. Int J Climatol (2014) 34(3):623–42. doi: 10.1002/joc.3711
30. Bartholome E, Belward AS. GLC2000: a new approach to global land cover mapping from Earth observation data. Int J Remote Sens (2005) 26(9):1959–1977. doi: 10.1080/01431160412331291297
31. Dörr H, Katruff L, Levin I. Soil texture parameterization of the methane uptake in aerated soils. Chemosphere (1993) 26(1–4):697–713. doi: 10.1016/0045-6535(93)90454-D
32. Paustian K, Ravindranath NH, van Amstel AR. Agriculture, forestry and other land use. In IPCC 2006: 2006 IPCC Guidelines for National Greenhouse Gas Inventories, Prepared by the National Greenhouse Gas Inventories Programme Eggleston HS, Buendia L, Miwa K, Ngara T, Tanabe K (eds). IGES, Japan (2006).
33. Shapiro SS, Wilk MB. An analysis of variance test for normality (Complete samples). Biometrika (1965) 52(3/4):591. doi: 10.2307/2333709
34. Crowther TW, Todd-Brown KEO, Rowe CW, Wieder WR, Carey JC, Machmuller MB, et al. Quantifying global soil carbon losses in response to warming. Nature (2016) 540(7631):104–8. doi: 10.1038/nature20150
35. Moulin AP, Glenn A, Tenuta M, Lobb DA, Dunmola AS, Yapa P. Alternative transformations of nitrous oxide soil flux data to normal distributions. Can J Soil Sci (2014) 94(1):105–8. doi: 10.4141/cjss2013-008
36. Charteris AF, Harris P, Marsden KA, Harris IM, Guo Z, Beaumont DA, et al. Within-field spatial variability of greenhouse gas fluxes from an extensive and intensive sheep-grazed pasture. Agric Ecosyst Environ (2021) 312:107355. doi: 10.1016/j.agee.2021.107355
37. Silver WL, Thompson AW, McGroddy ME, Varner RK, Dias JD, Silva H, et al. Fine root dynamics and trace gas fluxes in two lowland tropical forest soils. Glob Change Biol (2005) 11(2):290–306. doi: 10.1111/j.1365-2486.2005.00903.x
38. Laville P, Jambert C, Cellier P, Delmas R. Nitrous oxide fluxes from a fertilised maize crop using micrometeorological and chamber methods. Agric For Meteorol (1999) 20:19–38. doi: 10.1016/S0168-1923(99)00054-4
39. Kiese R, Butterbach-Bahl K. N2O and CO2 emissions from three different tropical forest sites in the wet tropics of Queensland, Australia. Soil Biol Biochem (2002) 34(7):975–87. doi: 10.1016/S0038-0717(02)00031-7
40. Zheng X, Mei B, Wang Y, Xie B, Wang Y, Dong H, et al. Quantification of N2O fluxes from soil–plant systems may be biased by the applied gas chromatograph methodology. Plant Soil (2008) 311(1–2):211–34. doi: 10.1007/s11104-008-9673-6
41. Pihlatie M, Rinne J, Ambus P, Pilegaard K, Dorsey JR, Rannik U, et al. Nitrous oxide emissions from a beech forest floor measured by eddy covariance and soil enclosure techniques. (2005) 2(4):377–87. doi: 10.5194/bg-2-377-2005
42. Jones SK, Famulari D, Di Marco CF, Nemitz E, Skiba UM, Rees RM, et al. Nitrous oxide emissions from managed grassland: a comparison of eddy covariance and static chamber measurements. Atmospheric Meas Tech (2011) 4(10):2179–94. doi: 10.5194/amt-4-2179-2011
43. Schlesinger WH. An estimate of the global sink for nitrous oxide in soils. Glob Change Biol (2013) 19(10):2929–31. doi: 10.1111/gcb.12239
44. Kroeze C, Bouwman L, Slomp CP. Sinks for nitrous oxide at the earth’s surface. In: Reay DS, Hewitt CN, Smith KA, Grace J, editors. Greenhouse gas sinks, 1st ed. UK: CABI (2007). doi: 10.1079/9781845931896.0227
45. Xia L, Lam SK, Kiese R, Chen D, Luo Y, van Groenigen KJ, et al. Elevated CO2 negates O3 impacts on terrestrial carbon and nitrogen cycles. One Earth (2021) 4(12):1752–63. doi: 10.1016/j.oneear.2021.11.009
46. Yamulki S, Harrison RM, Goulding KWT, Webster CP. N2O, NO and NO2 fluxes from a grassland: Effect of soil pH. Soil Biol Biochem (1997) 29(8):1199–208. doi: 10.1016/S0038-0717(97)00032-1
47. Rütting T, Huygens D, Boeckx P, Staelens J, Klemedtsson L. Increased fungal dominance in N2O emission hotspots along a natural pH gradient in organic forest soil. Biol Fertil Soils (2013) 49(6):715–21. doi: 10.1007/s00374-012-0762-6
48. Tzanakakis VA, Taylor AE, Bakken LR, Bottomley PJ, Myrold DD, Dörsch P. Relative activity of ammonia oxidizing archaea and bacteria determine nitrification-dependent N2O emissions in Oregon forest soils. Soil Biol Biochem (2019) 139:107612. doi: 10.1016/j.soilbio.2019.107612
49. Butterbach-Bahl K, Baggs EM, Dannenmann M, Kiese R, Zechmeister-Boltenstern S. Nitrous oxide emissions from soils: how well do we understand the processes and their controls? Philos Trans R Soc B Biol Sci (2013) 368(1621):20130122. doi: 10.1098/rstb.2013.0122
50. Corre M, Pennock D, Keseel CV, Elliott D. Estimation of annual nitrous oxide emissions from a transitional grassland-forest region in Saskatchewan, Canada. Canada Biogeochem (1999) 44:29–49. doi: 10.1007/BF00992997
51. Parton WJ, Holland EA, Del Grosso SJ, Hartman MD, Martin RE, Mosier AR, et al. Generalized model for NOx and N2O emissions from soils. J Geophys Res Atmospheres (2001) 106(D15):17403–19. doi: 10.1029/2001JD900101
52. Ullah S, Breitenbeck GA, Faulkner SP. Denitrification and N2O emission from forested and cultivated alluvial clay soil. Biogeochemistry (2005) 73(3):499–513. doi: 10.1007/s10533-004-1565-0
53. Xia L, Lam SK, Wolf B, Kiese R, Chen D, Butterbach-Bahl K. Trade-offs between soil carbon sequestration and reactive nitrogen losses under straw return in global agroecosystems. Glob Change Biol (2018) 24(12):5919–32. doi: 10.1111/gcb.14466
54. Sessitsch A, Weilharter A, Gerzabek MH, Kirchmann H, Kandeler E. Microbial population structures in soil particle size fractions of a long-term fertilizer field experiment. Appl Environ Microbiol (2001) 67(9):4215–24. doi: 10.1128/AEM.67.9.4215-4224.2001
55. Parfitt RL, Salt GJ. Carbon and nitrogen mineralisation in sand, silt, and clay fractions of soils under maize and pasture. Soil Res (2001) 39(2):361. doi: 10.1071/SR00028
56. Peiyuan C, Zhixuan C, Fenliang F, Chang Y, Alin S, Tingqiang L, et al. Soil texture: an easily overlooked factor affecting the temperature sensitivity of N2O emissions. (2022).
57. Ding F, Sun W, Huang Y. Net N2O production from soil particle size fractions and its response to changing temperature. Sci Total Environ (2019) 650:97–104. doi: 10.1016/j.scitotenv.2018.08.428
58. Weitz AM, Linder E, Frolking S, Crill PM, Keller M. N2O emissions from humid tropical agricultural soils: effects of soil moisture, texture and nitrogen availability. Soil Biol Biochem (2001) 33(7–8):1077–93. doi: 10.1016/S0038-0717(01)00013-X
59. Gu J, Nicoullaud B, Rochette P, Grossel A, Hénault C, Cellier P, et al. A regional experiment suggests that soil texture is a major control of N2O emissions from tile-drained winter wheat fields during the fertilization period. Soil Biol Biochem (2013) 60:134–41. doi: 10.1016/j.soilbio.2013.01.029
60. Cai A, Feng W, Zhang W, Xu M. Climate, soil texture, and soil types affect the contributions of fine-fraction-stabilized carbon to total soil organic carbon in different land uses across China. J Environ Manage (2016) 172:2–9. doi: 10.1016/j.jenvman.2016.02.009
61. Schaufler G, Kitzler B, Schindlbacher A, Skiba U, Sutton MA, Zechmeister-Boltenstern S. Greenhouse gas emissions from European soils under different land use: effects of soil moisture and temperature. Eur J Soil Sci (2010) 61(5):683–96. doi: 10.1111/j.1365-2389.2010.01277.x
62. Groffman PM, Brumme R, Butterbach-Bahl K, Dobbie KE, Mosier AR, Ojima D, et al. Evaluating annual nitrous oxide fluxes at the ecosystem scale. Glob Biogeochem Cycles (2000) 14(4):1061–70. doi: 10.1029/1999GB001227
63. Morishita T, Sakata T, Takahashi M, Ishizuka S, Mizoguchi T, Inagaki Y, et al. Methane uptake and nitrous oxide emission in Japanese forest soils and their relationship to soil and vegetation types. Soil Sci Plant Nutr (2007) 53(5):678–91. doi: 10.1111/j.1747-0765.2007.00181.x
64. Martins CSC, Nazaries L, Delgado-Baquerizo M, Macdonald CA, Anderson IC, Hobbie SE, et al. Identifying environmental drivers of greenhouse gas emissions under warming and reduced rainfall in bo\real–temperate forests. Funct Ecol (2017) 31(12):2356–68. doi: 10.1111/1365-2435.12928
65. Li L, Zheng Z, Wang W, Biederman JA, Xu X, Ran Q, et al. Terrestrial N2O emissions and related functional genes under climate change: A global meta-analysis. Glob Change Biol (2020) 26(2):931–43. doi: 10.1111/gcb.14847
66. Shi F, Chen H, Chen H, Wu Y, Wu N. The combined effects of warming and drying suppress CO2 and N2O emission rates in an alpine meadow of the eastern Tibetan plateau. Ecol Res (2012) 27(4):725–33. doi: 10.1007/s11284-012-0950-8
Keywords: forest, grassland, N2O emissions, global, drivers
Citation: Yu L, Zhang Q, Tian Y, Sun W, Scheer C, Li T and Zhang W (2022) Global variations and drivers of nitrous oxide emissions from forests and grasslands. Front. Soil Sci. 2:1094177. doi: 10.3389/fsoil.2022.1094177
Received: 09 November 2022; Accepted: 29 November 2022;
Published: 16 December 2022.
Edited by:
Shuo Jiao, Northwest A&F University, ChinaReviewed by:
Baobao Pan, The University of Melbourne, AustraliaJinyang Wang, Nanjing Agricultural University, China
Copyright © 2022 Yu, Zhang, Tian, Sun, Scheer, Li and Zhang. This is an open-access article distributed under the terms of the Creative Commons Attribution License (CC BY). The use, distribution or reproduction in other forums is permitted, provided the original author(s) and the copyright owner(s) are credited and that the original publication in this journal is cited, in accordance with accepted academic practice. No use, distribution or reproduction is permitted which does not comply with these terms.
*Correspondence: Wen Zhang, zhw@mail.iap.ac.cn