- Pedometrics, Landscape Analysis and GIS Laboratory, Soil and Water Sciences Department, University of Florida, Gainesville, FL, United States
Foundations Of Pedometrics And Digital Soil Mapping
The discipline of pedometrics combines pedology (i.e., understanding of the physical, chemical, and biological soil properties, patterns, and their genesis) and quantitative modeling of soils. Pedometrics research has focused extensively to model soil properties and associated uncertainties from field to large landscape scale (1, 2). Artificial intelligence (AI), specifically machine learning (ML), and deep learning (DL) algorithms, have advanced a profound transformation of the discipline with new challenges.
The conceptual frameworks underlying pedometrics and digital soil mapping (DSM) have been rooted in factorial models that relate soils and factors that influence soil formation, so-called soil-environmental covariates (3, 4). These soil factorial frameworks have moved from the conceptual CLORPT soil formation model1 (5, 6), the spatially and temporally explicit SCORPAN framework2 (7) toward the spatially and temporally explicit STEP-AWBH model frame3 (8–11). The general approach of soil-factorial modeling using STEP-AWBH input variables to predict a soil property or class is showcased in Figure 1. This latter mental frame accounts for soil-landscape conditions (STEP factorsiii) and the dynamics of the atmosphere/climate (A), water/hydrosphere (W), biosphere (B), as well as human activities (H) in the social, cultural, economic, and political domains (e.g., land management, carbon credit markets, economic incentives and programs, human resource capital). For example, the STEP-AWBH frame facilitates the incorporation of short-term temporally varying AWBH factors such as short-duration climatic variables (e.g., rainfall-runoff events preceding soil observations) and also long-term climatic patterns and variations (e.g., 40-year average annual precipitation and the 40-year amplitude of temperature variation preceding soil observations) that have impacted pedogenesis in a study region. The STEP-AWBH frame is anchored in system theory that views the totality of an ecosystem integrating a multiplicity of domains. Thus, STEP-AWBH has moved factorial models closer to mechanistic Earth simulation models through the incorporation of pedological, biogeochemical, socio-cultural, economic, and political factors in the modeling process of soils.
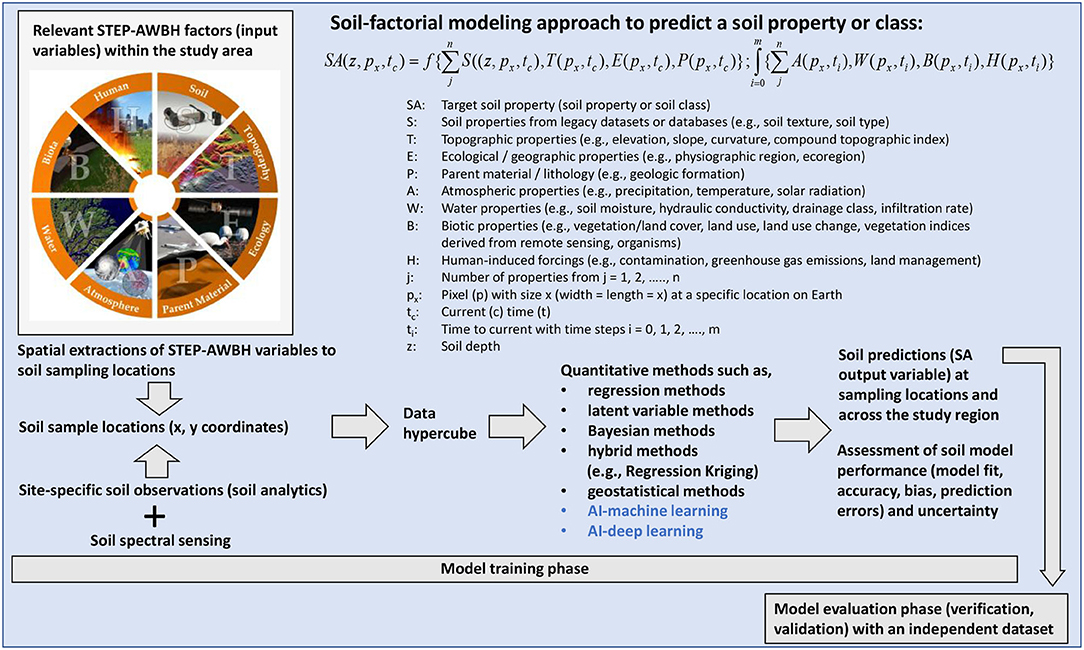
Figure 1. Overview soil-factorial modeling based on the STEP-AWBH model [after (8)].
In general, conceptual factorial soil models have been implemented using purely spatial quantitative approaches (e.g., geostatistical methods), environmental correlation approaches (e.g., fitting methods such as multivariate regression, ML, and DL), and hybrid methods (e.g., regression kriging) (12–14). Purely mechanistic space-time simulations of soil genesis are still at the frontiers of pedometrics due to the following challenges (1) labor and costs to collect soil data and up-to-date soil-environmental datasets, (2) algorithms that appropriately model the pedosphere across spatial and temporal scales, (3) the subjectivity and idiosyncrasy of human's impact onto landscapes that varies widely due to needs, people's values and beliefs, and (4) insufficient incorporation of social, cultural, economic, and political dimensions into the modeling process. Although factorial soil models intended to provide a mechanistic framework for soil formation they have been used predominantly as functional fit models which formalize relationships between soil-forming factors and the resulting soils.
Knowledge Vs. Data-Driven Soil-Factorial Modeling
Knowledge-Based Soil-Factorial Models
Factorial soil prediction models have dominated the field of pedometrics to assess soil quality, security, health, fertility, productivity, and more (15, 16). From a pedological perspective, these kinds of models are one-directional and aim to predict soils (S) across space: Soil-forming factors → S. Thus, such CLORPT inspired soil prediction models are deterministic assuming causality between soil-environmental covariates (cause) and soils (effect). The underlying philosophical paradigms are (1) constructivism focused on the integration of new knowledge and understanding of soils that are part of the totality of the environment, and (2) participatory epistemology which asserts that meaning arises through the participation of humans with the environment. For example, for a soil scientist meaning arises through the study of soils, for a farmer meaning arises through cropping and sustainability of soils, for an environmentalist meaning arises through care and protection of soils. The constructivist and participatory paradigms view humans and all life forms as agents that participate in the formation and use of soils (B and H → S); and vice versa, soils shape land use, impact water flux, influence climatic conditions, etc. (S → SCORPAN; STEP-AWBH). Such knowledge-oriented view acknowledges feedback loops between soil-forming factors and S adopting an integrative system perspective that honors meaning, connectivity, and understanding of soil-people relations (17).
Challenges remain in knowledge-based soil-factorial models to populate SCORPAN and STEP-AWBH factors, especially B and H factors. A vast amount of DSM applications have populated the B factor and H factor using land cover, land use, or spectral signatures derived from proximal sensors or remote sensors [e.g., (12, 14, 18–21)]. These soil prediction models fall short to give voice to the diversity of anthropogenic impacts onto soils and reduce the H factor to a quantifiable variable in the environmental system; or worse, neglect to populate the H factor. Such commodification of B and H factors ignores how socio-cultural spheres (e.g., land use management, people's beliefs and valuation of nature and soils) and economic-political domains (e.g., conservation programs, cash crop markets, and environmental regulations) interact with soilscapes.
Data-Driven Soil Factorial Models
The adoption of AI-ML and AI-DL into soil science has pivoted research goals from understanding of soil formation and patterns toward the search for “the best” performing soil prediction model. Literally, “running the machine” to identify the ideal relations between SCORPAN or STEP-AWBH variables and a target soil property (S). Recently, Padarian et al. (22) provided a comprehensive review of digital soil models using DL and Khaledian and Miller (23) reviewed ML methods for predictive soil mapping.
Generally, prediction refers to the prediction of a future state, although factorial soil models are unsuitable to forecast or backcast because pedological understanding conveys that the relations between soil-forming factors and S change over time (24). Thus, factorial models have been predominantly used in digital soil mapping to compute spatially explicit predictions of soil properties. For example, geospatial soil predictions for soil organic carbon, bulk density, cation exchange capacity, pH, soil texture fractions, and depth to bedrock were made by Hengl et al. (25) using Random Forest, Gradient Boosting, and neural networks. Padarian et al. (22) used Convolutional Neural Networks and Cubist to predict soil layers and soil organic carbon, while (26) used various ML algorithms (among them Random Forest, Bagged Regression Tree, Boosted Regression Tree, Support Vector Machine) to predict soil total carbon and multiple carbon fractions.
The soil factorial modeling approach is not suitable to project soil properties into the future because this would involve extrapolation of soil predications that are associated with high uncertainty. Thus, there are limitations of factorial soil modeling to address Grande challenge such as the assessment of future soil and food security. Instead, state-of-the-art factorial models are now widely used in the pedometrics community to compute relations between soil-forming factors and S and apply these machine-fitting models to unsampled locations within a given study region. For example, Minasny et al. (27), Ross et al. (28), Wadoux et al. (29), Xiong et al. (11), and Yang et al. (30) computed soil organic carbon using various AI machine-fitting algorithms with Random Forest being one of the most popular method in soil science. AI-ML and AI-DL technology is poised to optimize through brute fitting of inputs and outputs (31).
The implications of AI-based factorial soil modeling are striking. AI models aim to compute the “perfect” connections between soil-forming factors and S to describe the soil-ecosystem adopting a purely empirical data-driven perspective. Computational advancements have fostered to apply AI-DL algorithms, which refers to ML using multiple layers, nodes, and weighting factors of adjustable computing elements (32). Artificial neural networks, such as Convolutional Neural Networks (CNN), adopt the DL paradigm to fit inputs (soil-forming factors) and output (a specific soil property or class) accounting for the complexity of real-world soil-ecosystems (e.g., CNN-DSM application by 28). According to Liao (33), “deep learning announces its prediction without explaining (in human terms) how it arrived at that prediction” (p. 7). AI-DL soil models idealize the R2 of 1.0 and are able to minimize error metrics like no other statistical or geostatistical method. AI-DL models use nodes or layers of nodes to represent pedological knowledge in abstract form that lacks transparency for soil scientists, land managers, and the general public.
Importantly, this movement from knowledge-based toward data-driven AI-based soil-factorial modeling is a profound paradigm shift from earlier research focused on understanding of the humans-soil-environmental domain toward soils mapped into machine code. AI-based soil modeling relaxes deterministic-mechanistic assumptions of causality between soil-environmental covariates (cause) and soils (effect); instead, associations between covariates and soil(s) are optimized to identify the best performing model(s). The latter associations may be spurious, lack transparency, human meaning-making and trust in the model, and have vulnerable sensibility to so-called one pixel-attacks (33). By changing one pixel in an image, Harvard researchers were able to get a DL algorithm to classify an image of a car as a dog (33). How are pixel-attacks impacting soil maps and models? What are the implications of adding/changing one soil observation or a covariate (e.g., one pixel in a remote sensing image) in an AI-DL soil prediction model?
What Is “the Perfect” Soil Model of the Future?
The pivotal shift from pedological knowledge-discovery of soil-landscape patterns and genesis toward machine optimized soil models has created enthusiasm, critique, and controversy in the pedometrics research community. Wadoux et al. (34) demonstrated that ML can find relevant soil patterns even with meaningless pseudo-variables, such as digital portrait photos of pedometricians, that successfully predicted soil organic carbon using the machine learning algorithm Random Forest.
The prevalent trends in SCORPAN and STEP-AWBH facilitated modeling of soils entail increased usage of (1) latent variable and AI-ML methods, specifically the popular Random Forest approach [e.g., (35, 36)], (2) hidden nodes, layers, and weighting factors in AI-DL methods [e.g., (29, 37)], and (3) applications of automated mapping techniques at global scale (25, 38) to identify the best fit between soil, soil-environmental covariates and spectral data, minimize uncertainty and bias, and optimize the accuracy and precision of soil predictions. Such machine-focused research applications reveal “the perfect” soil model in terms of error and uncertainty metrics. However, these kind of machine-generated soil models are rooted in abstract soil-environmental relations of high-orders of dimensionality and complex non-linear interactions among input and output variables. Challenges remain to enhance the transparency and meaning of AI soil models. In AI, black-box (AI-DL) or gray-box (AI-ML) conceptual frameworks are adopted, the former AI-DL limiting insights into the soil-environmental relations that govern the soil prediction model. In contrast, “the perfect” soil models using non-AI approaches aim to maximize soil knowledge discovery (e.g., assessing soil carbon or identifying which STEP-AWBH variable relates most strongly with a specific S) which is meaningful to people and researchers alike.
The challenge for future soil-factorial model applications is to account for—prior selection of relevant soil-environmental covariates (i.e., SCORPAN or STEP-AWBH factors) based on pedological knowledge as well as rigorous posterior soil model interpretation. Xiong et al. (11) provided a holistic soil-landscape modeling framework that combines knowledge and data-driven AI approaches to assess soil organic carbon in Florida. Xiong et al.'s strategic approach is able to discern (1) all-relevant sets (i.e., strongly and weakly relevant STEP-AWBH variables to assess S which has value to understand the mechanisms underlying the formation of S), (2) minimal-optimal set (i.e., to identify a parsimonious and transparent model for end-users), and (3) irrelevant variables (e.g., pseudo-variables that are not meaningful to explain pedological processes). Challenges remain to harmonize and reconcile knowledge-based and data-driven AI soil modeling approaches to account for the diversity of soil-landscapes.
Author Contributions
The author confirms being the sole contributor of this work and has approved it for publication.
Funding
SG was funded by the University of Florida.
Conflict of Interest
The author declares that the research was conducted in the absence of any commercial or financial relationships that could be construed as a potential conflict of interest.
Publisher's Note
All claims expressed in this article are solely those of the authors and do not necessarily represent those of their affiliated organizations, or those of the publisher, the editors and the reviewers. Any product that may be evaluated in this article, or claim that may be made by its manufacturer, is not guaranteed or endorsed by the publisher.
Acknowledgments
I would like to thank the critical discussion within the Pedometrics Commission of the International Union of Soil Science for the inspiration to write this short article.
Footnotes
1. ^CLORPT soil formation model with CL, climate; O, organisms; R, relief; P, parent material; and T, time to form soils (5, 6).
2. ^SCORPAN model with S, soil class or soil attribute; O, organism (fauna, flora, vegetation, or human activity); R, relief (topography, landscape attributes); P, parent material, lithology; A, age or time factor, and N, space, spatial position (7).
3. ^STEP-AWBH model with S: Ancillary soil properties (e.g., soil texture, soil spectral data), T, Topographic properties (e.g., elevation, slope, curvature, compound topographic index); E, Ecological/geographic properties (e.g., physiographic region, ecoregion); P, Parent material; geologic properties (e.g., geologic formation); A, Atmospheric properties (e.g., precipitation, temperature, solar radiation); W, Water properties (e.g., surface runoff, infiltration rate); B, Biotic properties (e.g., vegetation/land cover, land use, land use change, spectral indices derived from remote sensing, organisms); H, Human-induced forcings (e.g., contamination, greenhouse gas emissions) (8).
References
1. Minasny B, McBratney AB. Digital soil mapping: a brief history and some lessons. Geoderma. (2015) 264:301–11. doi: 10.1016/j.geoderma.2015.07.017
3. McKenzie NJ, Philip Ryan J. Spatial prediction of soil properties using environmental correlation. Geoderma. (1999) 89:67–94. doi: 10.1016/S0016-7061(98)00137-2
4. Grunwald S. What do we really know about the space–time continuum of soil landscapes? In: Grunwald S, editors. Environmental Soil-Landscape Modeling: Geographic Information Technologies and Pedometrics. New York, NY: CRC Press (2006). p. 3–36.
6. Jenny H. Factors of Soil Formation: A System of Quantitative Pedology. Mineaola, NY: Courier Dover Publications (1992).
7. McBratney AB, Mendonça Santos ML, Minasny B. On digital soil mapping. Geoderma. (2003) 117:3–52. doi: 10.1016/S0016-7061(03)00223-4
8. Grunwald S, Thompson JA, Boettinger JL. Digital soil mapping and modeling at continental scales: finding solutions for global issues. Soil Sci Soc Am J. (2011) 75:1201–13. doi: 10.2136/sssaj2011.0025
9. Ma Y, Minasny B, Malone BP, McBratney AB. Pedology and digital soil mapping (DSM). Eur J Soil Sci. (2019) 70:216–35. doi: 10.1111/ejss.12790
10. Thompson JA, Roecker SM, Grunwald S, Owens PR. Digital soil mapping: interactions with and applications for hydropedology. In: Lin HS, editors. Hydropedology – Synergistic Integration of Pedology and Hydrology. Cambridge, MA: Elsevier B.V, Academic Press (2012). p. 665–709.
11. Xiong X, Grunwald S, Myers DB, Kim J, Harris WG, Comerford NB. Holistic environmental soil-landscape modeling of soil organic carbon. Environ Model Softw. (2014) 57:202–15. doi: 10.1016/j.envsoft.2014.03.004
12. Arrouays D, Lagacherie P, Hartemink AE. Digital soil mapping across the globe. Geoderma Reg Digital Soil Mapp Across Globe. (2017) 9:1–4. doi: 10.1016/j.geodrs.2017.03.002
13. Keskin H, Grunwald S. Regression kriging as a workhorse in the digital soil mapper's toolbox. Geoderma. (2018) 326:22–41. doi: 10.1016/j.geoderma.2018.04.004
14. Malone BP, Odgers NP, Stockmann U, Minasny B, McBratney AB. Digital soil mapping of soil classes and continuous soil properties. In: McBratney AB, Minasny B, Stockmann U, editors. Pedometrics. Cham: Springer (2018). p. 372–413.
15. Lehmann J, Bossio DA, Kögel-Knabner I, Rillig MC. The health concept and future prospects of soil. Nat Rev Earth Environ. (2020) 1:544–53. doi: 10.1038/s43017-020-0080-8
16. Mizuta K, Grunwald S, Cropper WP Jr., Bacon AR. Developmental history of soil concepts from a scientific perspective. Appl Sci J. (2021) 11:4275. doi: 10.3390/app11094275
17. Grunwald S, Mizuta K, Ceddia MB, Pinheiro EFM, Kastner-Wilcox RK, Gavilan CP, et al. The meta soil model: an integrative multi-model framework for soil security. In: Field DJ, Morgan CLS, McBratney AB, editors. Global Soil Security. Cham: Springer Nature Publ (2017). p. 305–18.
18. Grunwald S. Multi-criteria characterization of recent digital soil mapping and modeling approaches. Geoderma. (2009) 152:195–207. doi: 10.1016/j.geoderma.2009.06.003
19. Grunwald S, Vasques GM, Rivero RG. Fusion of soil and remote sensing data to model soil properties. Adv Agron. (2015) 131:1–109. doi: 10.1016/bs.agron.2014.12.004
20. Peng Y, Xiong X, Adhikari K, Knadel M, Grunwald S, Greve H, et al. Modeling soil organic carbon at regional scale by combining multi-spectral images with laboratory spectra. PLoS ONE. (2015) 10:e0142295. doi: 10.1371/journal.pone.0142295
21. Poggio L, Gimona A, Brewer MJ. Regional scale mapping of soil properties and their uncertainty with a large number of satellite-derived covariates. Geoderma. (2013) 209–10:1–14. doi: 10.1016/j.geoderma.2013.05.029
22. Padarian J, Minasny B, McBratney AB. Using deep learning for digital soil mapping. Soil. (2019) 5:79–89. doi: 10.5194/soil-5-79-2019
23. Khaledian Y, Miller BA. Selecting appropriate machine learning methods for digital soil mapping. Appl Math Model. (2020) 81:401–18. doi: 10.1016/j.apm.2019.12.016
24. Weil RR, Brady NC. The Nature and Properties of Soils. 14th ed. New York, NY: Pearson International (2016).
25. Hengl T, Mendes de Jesus J, Heuvelink BMG, Gonzalez MR, Albert M, Blagotić A, et al. SoilGrids250m: global gridded soil information based on machine learning. PLoS ONE. (2017) 12:e0169748. doi: 10.1371/journal.pone.0169748
26. Keskin H, Grunwald S, Harris WG. Digital mapping of soil carbon fractions with machine learning. Geoderma. (2019) 339:40–58. doi: 10.1016/j.geoderma.2018.12.037
27. Minasny B, McBratney AB, Malone BP, Wheeler I. Digital mapping of soil organic carbon. Adv Agron. (2013) 118:1–47. doi: 10.1016/B978-0-12-405942-9.00001-3
28. Ross CW, Grunwald S, Vogel JG, Markewitz D, Jokela EJ, Martin TA, et al. Accounting for two-billion tons of stabilized soil carbon. Sci Tot Environ. (2019) 134615:1–11. doi: 10.1016/j.scitotenv.2019.134615
29. Wadoux AMJ-C, Padarian J, Minasny B. Multi-source data integration for soil mapping using deep learning. Soil. (2019) 5:107–19. doi: 10.5194/soil-5-107-2019
30. Yang R-M, Zhang GL, Liu F, Lu YY, Yang F, Yang F, et al. Comparison of boosted regression tree and random forest models for mapping topsoil organic carbon concentration in an alpine ecosystem. Ecol Indic. (2016) 60:870–8. doi: 10.1016/j.ecolind.2015.08.036
31. Liao SM, editors. Ethics of Artificial Intelligence. New York, NY: Oxford University Press (2020).
33. Liao SM. A short introduction to the ethics of artificial intelligence. In: Liao SM, editors. Ethics of Artificial Intelligence. New York, NY: Oxford University Press (2020). p. 1–42.
34. Wadoux AMJ-C, Samuel-Rosa A, Poggio L, Mulder VL. A note on knowledge discovery and machine learning in digital soil mapping. Eur J Soil Sci. (2020) 71:133–6. doi: 10.1111/ejss.12909
35. Adi SH, Grunwald S. Integrative environmental modeling of soil carbon fractions based on a new latent variable model approach. Sci Tot Environ. (2019) 134566:1–15. doi: 10.1016/j.scitotenv.2019.134566
36. Lamichhane S, Kumar L, Wilson B. Digital soil mapping algorithms and covariates for soil organic carbon mapping and their implications: a review. Geoderma. (2019) 352:395–413. doi: 10.1016/j.geoderma.2019.05.031
37. Ng W, Minasny B, Montazerolghaem M, Padarian J, Ferguson R, Bailey S, et al. Convolutional neural network for simultaneous prediction of several soil properties using visible/near-infrared, mid-infrared, and their combined spectra. Geoderma. (2019) 352:251–67. doi: 10.1016/j.geoderma.2019.06.016
Keywords: pedometrics, digital soil mapping, artificial intelligence, machine learning, deep learning, factorial soil models, SCORPAN, STEP-AWBH
Citation: Grunwald S (2021) Grand Challenges in Pedometrics-AI Research. Front. Soil Sci. 1:714323. doi: 10.3389/fsoil.2021.714323
Received: 25 May 2021; Accepted: 28 July 2021;
Published: 20 August 2021.
Approved by: Davey Jones, Bangor University, United Kingdom
Copyright © 2021 Grunwald. This is an open-access article distributed under the terms of the Creative Commons Attribution License (CC BY). The use, distribution or reproduction in other forums is permitted, provided the original author(s) and the copyright owner(s) are credited and that the original publication in this journal is cited, in accordance with accepted academic practice. No use, distribution or reproduction is permitted which does not comply with these terms.
*Correspondence: Sabine Grunwald, c2FiZ3J1QHVmbC5lZHU=