- 1Department of Epidemiology and Data Science, Amsterdam University Medical Centers, Vrije Universiteit Amsterdam, Amsterdam, Netherlands
- 2Centre for Comparative Welfare Studies, Aalborg University, Aalborg, Denmark
This study examines occupation-based differences in life expectancy and the extent to which health accounts for these differences. Twentyseven-year survival follow-up data were used from the Dutch population-based Longitudinal Aging Study Amsterdam (n = 2,531), initial ages 55–85 years. Occupation was based on longest-held job. Results show that the non-skilled general, technical and transport domains had an up to 3.5-year shorter life expectancy than the academic professions, accounting for the compositional characteristics age and gender. Statutory retirement age could be made to vary accordingly, by allowing a proportionally greater pension build-up in the shorter-lived domains. Health accounted for a substantial portion of the longevity difference, ranging from 20 to 66%, depending on the health indicator. Thus, health differences between occupational domains today can be used as a means to tailor retirement ages to individuals’ risks of longevity. These data provide a proof of principle for the development of an actuarially fair method to determine statutory retirement ages.
Introduction
Actuarial fairness is considered a key aspect of a just pension system (Schokkaert and Van Parijs, 2003; De Tavernier, 2021) and can be understood as “equal treatment for equal risks” (Landes, 2015, p. 521). For a pension system to be actuarially fair, an individual’s contributions (plus interests) should equal the individual’s expected benefits. Expected benefits are directly linked to the expected duration of time during which they will be enjoyed, i.e., the individual’s life expectancy. It is well-known, however, that life expectancy is strongly socially stratified (Kunst and Mackenbach, 1994). Moreover, in European countries the longevity gap between lower and higher socio-economic positions has been shown to be increasing (Unger and Schulze, 2013; Östergren et al., 2017; Tanaka et al., 2019). Thus, equal treatment through a uniform statutory retirement age does not do justice to the socially highly unequally distributed life opportunities and is not actuarially fair (Unger and Schulze, 2013). Given a uniform statutory retirement age, Barnay (2007) showed that executives and the intermediate professions benefit much more from the pension system than manual workers. Likewise, several authors concluded that such pension systems generate a Matthew effect, redistributing means from the less well-off to the better-off (Myles, 2003; Schokkaert and Van Parijs, 2003; Queisser and Whitehouse, 2006; Simonovits, 2015). When contributions and benefits would be adapted to differences in life expectancy, redistribution towards an actuarially fair system could take place. This study proposes a mechanism through which this could be achieved.
Various policies affect actuarial fairness of pension systems. One way to make pension policies actuarially fair is through early retirement regulations. Some European countries offer early retirement possibilities for specific categories of workers in highly demanding jobs. In their overview, Natali et al. (2016) find a wide variety of classifications and interpretations of what constitutes arduous jobs across countries, and note that the concept is often not clearly delineated. This is the case for the new Finnish years-of-service pension (Finnish Centre for Pensions, 2015), for instance, where discretion is given to medical doctors to make decisions in individual cases. In other countries, such as Belgium and the Netherlands, social partners continue to disagree about which jobs fall in this category, illustrating the arbitrary nature of decisions about which jobs are particularly demanding.
This study aims to develop a more objective method to determine statutory retirement ages rooted in actuarial fairness by quantifying occupation-based life expectancies. Linking the resulting life expectancies to pension build-up would improve actuarial fairness of pension systems. Thus, workers in occupational domains with a shorter life expectancy should be facilitated to retire earlier by adjusting their pension build-up. It is important to note that by taking actuarial fairness as our point of departure, the question of whether inequalities in life expectancy are actually caused by occupational exposure differences is not relevant (indeed, selection into occupations might play an important role, leading to compositional differences, see Ravesteijn et al., 2018). The principle merely requires that pension policies are adjusted to observed differences in longevity: contributions and expected benefits should balance out–no matter what causes occupation-based differences in life expectancy.
Earlier evidence shows that numerous factors impact longevity, including physical and mental health related factors, social conditions, and heredity (e.g., Goldman et al., 2016; Iacob et al., 2016; Suemoto et al., 2017). Among these, social stratification indicators such as level of education and level of occupation play an important role. Many of these studies considered occupation as a proxy for socio-economic position. Indeed, in the United Kingdom occupational class has been used as the standard indicator of social class (Llena-Nozal et al., 2004). But also in studies outside the United Kingdom, occupation has been used as such, e.g., in Canada (Tjepkema et al., 2013), in Denmark (Brønnum-Hansen et al., 2020), and in other European countries (Tanaka et al., 2019). In these studies, the occupational classification represents a hierarchy of skill level, rather than actual occupations. It may be argued that social class is the main factor at work in occupation-related differences in health. Early studies by Moore and Hayward (1990) and Johnson and colleagues (1999), however, showed that important mortality differences between occupations exist that are not accounted for by social status, income, and education. A recent study by Leinonen et al. (2018) showed evidence of variation in sickness absence between four large industrial sectors despite the fact that these were examined within particular occupational classes. These studies support the unique contribution of occupation to health outcomes.
Evidence on the impact of employment characteristics other than job level on mortality comes from studies on working conditions. In a meta-analysis of 17 studies, Amiri and Behnezhad (2020) reported that in people with job strain, which is a combination of high demands and low control at work, the risk of mortality is 20% higher than in the reference group. Also physical demands have been shown to be associated with increased risk of mortality (Mikkola et al., 2019). In a recent study from our own group, people at age 55 who had physically strenuous jobs regarding repetitive movements and use of force had a 1.5–2.0 years shorter life expectancy than their counterparts with less strenuous jobs, and people who had jobs with a low variation in activities and low autonomy had a 1.1–2.5 years shorter life expectancy than their counterparts with jobs with high variation and autonomy (de Wind et al., 2020). Working conditions may be concentrated in specific occupations, but essentially cut across occupations. The findings from studies on working conditions are relevant for the management of older workers’ balance of work capacity and work demands, which is to be achieved mainly in the workplace and at the company level. For pension policy, however, it is useful to consider a higher aggregate level.
Studies addressing the impact on mortality of occupation per se are scarce, although they have a long tradition (Fox and Adelstein, 1978). Moore and Hayward (1990) showed that in the United States between 1966 and 1983, service workers had a 4.5-year shorter life expectancy from age 55 than professionals. In another US study, Johnson and colleagues (1999) found increasing risks of mortality across the occupational spectrum from the highly skilled occupations to less-skilled and generally more labor-intensive occupations. A more recent South Korean study of workers enrolled in a national insurance program showed that workers in elementary occupations had a twice as high mortality rate as professionals (Lee et al., 2016). A recent study in the United Kingdom was based on linkage of census data with 10-year follow-up registry mortality data and distinguished 60 occupations (Katikireddi et al., 2017). It showed that among men, the highest mortality rates were in elementary construction, housekeeping and factory workers, whereas health professionals had the lowest mortality. Among women, factory workers and garment trade workers had high, and teachers and business professionals had low mortality rates.
In this study, we will specifically address differences in longevity by broad occupational domains, as they closely correspond to industrial sectors. A sector is defined as a group of industries with the same main economic activity; the latter is indicated by the predominant type of occupation (Statistics Netherlands, 2021). Using sector as a study unit offers a number of advantages from a policy perspective. First, it suits the actuarial fairness argument as, in most countries, pension entitlements and contributions are linked to employment sector. Second, variations in pension policies can be decided by social partners in sector-specific collective bargaining, and occupational pension schemes often already are sector-specific (De Preter et al., 2012; Wiß 2015). Third, sector of employment is information that is easily accessible for governments and is readily available in many state registers. In Netherlands, the country in which our study is based, the focus on sector has direct applicability, as negotiations between unions of employees and employers are conducted sector-wise, and pension funds, the institutes that provide the work-related pension benefits, are organized within sectors. Hence, we formulate a first research question:
- What are quantitative differences in longevity between occupational domains?
We are aware of the issue that in developing occupation-based retirement ages, the calculation of occupation-based life expectancy involves mortality follow-up of individuals over time until enough individuals should have deceased in order to construct reliable mortality tables. This can only be done on historical data. However, occupations change over the years in terms of task use and demands (Romeu Gordo and Skirbekk, 2013; Cassidy, 2017), so that occupation-based life expectancies of earlier generations may not be generalized to those working in the same occupations today. To overcome this problem, we test to what extent the relation between occupational domain and life expectancy is accounted for by health in the historical data. We are not aware of earlier studies testing the role of health. However, if indeed health acts as an explanatory factor of this relationship, we can use health differences between domains today as a means to tailor statutory retirement ages to individuals’ risks of longevity, thereby making pension systems more actuarially fair. This leads to our second research question:
- To what extent are health indicators explanatory factors in the association between occupational domain and longevity?
Materials and Methods
Sample
Data are used from the 1992–93 baseline cycle of the Dutch population-based Longitudinal Aging Study Amsterdam (LASA), linked to vital status follow-up from municipal registries. LASA is a prospective study of cohorts based in three regions of the Netherlands that together form a representative sample for the Netherlands (Deeg et al., 2002; Hoogendijk et al., 2020). The baseline sample included 3,107 55–85-year-olds. Older ages and men were oversampled, so that each 5-year age group included about 250 male and 250 female participants. Among these participants, for 19 the vital status was unkown, 173 responded to a short version of the interview which did not include questions about current or past work, 80 missed one or more of the work questions, and 304 never had a job. Excluding these participants left a study sample size of 2,531.
Dependent Variable
Vital status is traced periodically through the Municipal Personal Records database which covers all residents in the Netherlands. For the current study, mortality ascertainment up to December 31, 2019 was used, providing about 27 years of mortality follow-up. At the probing date, 84.0% if the study sample had died.
The dependent variable is operationally defined as the Realized Probability of Dying (RPD). The RPD is an individual measure of survival time relative to the total population, based on sex and age at baseline (Deeg et al., 1989). As such, the RPD belongs to the family of relative survival measures (Rutherford et al., 2012). We opt for this individual measure of survival rather than using commonly used group-based methods to predict survival time, such as Cox proportional hazards models, for several reasons. First, an individual measure is more accurate than group-based approaches. Second, it lends itself as the dependent variable in linear regression models, with the advantage that examination of explanatory factors (our second research question) is straightforward (Mood, 2010). Third, as the RPD is based on the age and sex of each individual participant, differences the in age-sex composition across occupational domains are accounted for. Differences in RPD can be transformed into differences in number of years of life expectancy.
Using life tables based on the total population for subsequent years (1992 through 2019) during the study period, the RPD compares for each individual of a specific age and sex this person’s survival probability with the overall survival probability of the Dutch population of that age and sex, from the starting month of the study through the years up to December 31, 2019. In formula:
where n is the total number of calendar years during which the participant is followed up to death or end-of-study, di is the probability of death according to the life table in calendar year i (i = 1 … n), ai is the age in calendar year i, and s is the sex of the participant.
Possible values of the RPD lie between 0 and 1. These values introduce a rank order among all sample subjects. The reference population has a mean RPD of 0.50. If the RPD is greater than 0.50, this means that the subject has lived a relatively short time; if it is less than 0.50, the subject has lived a relatively long time after baseline. For example, the value of a man’s RPD is 0.80 if 80% of his age and sex peers in the total population are still alive at the time of his death. The name “realized probability of dying” comes from the notion that the individual has “realized” the probability of death when a certain percentage of the reference population is expected to be still alive. The actual amount of survival time needed to reach a particular RPD varies according to the age and sex of the individual at baseline, with older people needing less time to achieve a lower RPD than younger people, and men needing less time than women. For example, a man aged 65 years when first participating in LASA in 1993, who dies after 20 years in 2013, has an RPD of 0.39. By comparison, a woman aged 65 years in 1993 will have the same RPD of 0.39 when she dies after 24 years, in 2017.
For those participants still alive at the end of the study period (December 31, 2019), i.e. 16.0% of the study sample (n = 406), a value of the Realized Probability of Dying is imputed. The RPD for these participants is estimated by assuming that their remaining survival time corresponds to the median population survival time from end-of-follow-up onward. This amounts to multiplying the probability of reaching their age at the end of 2019 by 0.5. For example, a woman aged 65 when examined in 1993, reaches the age of 91 in 2019 with probability 0.30. If she is still alive at the end of 2019, her imputed RPD is 0.15, implying that it is expected that she will die when only 15% of her 1993 cohort is still alive. This approach is derived from standard actuarial methods.
If the study sample’s RPD shows a uniform distribution, the survival distribution of the sample represents that of the total population. In this case, the logit of the RPD (LRPD = log(RPD/(1-RPD))) approaches a normal distribution with mean 0, and can be used as the dependent variable in analysis of variance and linear regression analysis. In our study sample, the mean (standard deviation) of the RPD and the LRPD are 0.50 (0.28) and 0.05 (1.66), respectively, and thus its survival is very close to that of the total population.
Independent Variables
Occupational Domains
For baseline participants who currently did paid work, their precise job description was recorded. For those who had done paid work in the past, the job description of their longest-held job was recorded. For those participants whose current job was not the longest-held job, data on their longest-held job were used, because workers may have moved to less strenuous jobs and for them, the association of occupation with longevity may be underestimated (Moore and Hayward, 1990). Based on the job characteristics domain (e.g., agricultural, care, teaching), required skill level (i.e., elementary through scientific, based on required education, training period, and work experience), and tasks (e.g., cattle breeding, nursing, instructing), jobs were classified into 43 occupational categories according to the Netherlands Standard Classification of Occupations 1992 (Statistics Netherlands, 2001; Rijs et al., 2014). These categories were condensed into 13 broad occupational domains by collapsing the skill levels within one domain. For the current study, seven of the largest domains were selected: the non-skilled general, technical, transport, administrative/commercial, care, agriculture, and pedagogical (teaching) domains. The technical domain includes jobs such as construction, machine work, electro-technical maintenance. The administrative/commercial domain includes jobs such as book keeper, buying clerk, hotel manager. The care domain includes (para)medical and social care and services jobs. Together, these seven domains constitute 91.7% of the sample. Domains that had less than 90 cases were grouped and served as the reference category for comparison of survival time. This category includes the following domains: natural science, juridical/security, cultural/linguistic, social science, and management. All had a survival time longer than average (Supplementary Table A). The mean (standard deviation) of the LRPD of the reference group was −0.21 (1.61).
Health
In order to facilitate implementation in the practice of pension insurance, we selected five health measures that are commonly retrievable from register data for initial analyses, i.e., sickness days, number of medications, hospital admission, outpatient visits, and general practitioner contact. For sensitivity analyses, we selected seven other health measures that have been proven to be a “best” predictor set for longevity (Suemoto et al., 2017).
Sickness days. These were self-reported as the number of days during the past month that participants had been ill to such extent that they had to stay in bed, with response categories 1) no days, 2) 1–3 days, 3) 4–7 days, 4) more than 1 week but less than a month, 5) all month.
Number of medications. The medications which the participants were using were recorded by the interviewer by inspecting the medicine containers. The total number of medications used was included in the analyses.
Hospital admission was self-reported. In order to avoid recall bias, hospital admission was not asked longer back than 6 months.
Outpatient visits. Contact with a medical specialist or psychiatrist was self-reported and also pertained to the past 6 months.
General practice contact. Contact with the participant’s general practitioner was self-reported and pertained to the past 6 months.
Four chronic conditions, i.e., obstructive lung disease, cardiovascular disease, diabetes, and cancer, were self-reported. Comparison with general practitioner records showed a satisfactory agreement (Kriegsman et al., 1996).
Disability was self-reported using the Global Activity Limitation Index, which asks about activity limitation that has lasted at least 3 months. It is coded as 1) no limitations, 2) mild limitations, 3) severe limitations (Van Oyen et al., 2006).
Self-rated health was measured using the single, widely used question “How is your health in general?,” with codes from 1) very good, to 5) poor.
Cognitive impairment was ascertained using the Dutch translation of the MiniMental State Exam (MMSE, Folstein et al., 1975). On 23 questions and tasks, respondents received one or more points when they gave the correct answer or performed the task correctly. Scores range from (0) all answers incorrect, to (30) unimpaired.
Covariates
Other independent variables, for descriptive purposes, include socio-demographic characteristics: age, gender, education in years, and occupational skill level. The latter variable was coded from 1) elementary to 5) scientific. Work status was included in the analyses, because evidence shows that health tends to improve after retirement (Eibich, 2015), and that before retirement age, people who currently do paid work are in better health than people who do not do paid work (Scharn et al., 2019). Work status was defined using two dummy variables: doing paid work versus not doing paid work, and being fully retired versus not fully retired.
Statistical Analysis
The socio-demographic and health characteristics of the seven occupational domains were compared using the chi-square test for dichotomous variables, and ANOVA’s F-test for continuous variables. Likewise, the distribution of the LRPD across the domains was examined using ANOVA’s F-test. To provide an illustration of this distribution, the domain-specific remaining life expectancies from age 65 were calculated based on the mean RPD of each domain, and shown in a figure. Subsequently, a series of linear regression analyses was performed to test survival time differences. The first model included only the domain dummy variables and age; in a second model work status was added. A next series of linear regression models included in addition one health variable at a time, and examined to what extent this health variable accounted for the association between domain membership and survival time, by comparing the coefficient of each domain dummy in the models without and with the health variable and calculating the percentage decline in this coefficient. In a final model, all five health variables were included and again, the percentage decline in the domain dummy coefficients was calculated. In a sensitivity analysis, the domain coefficients in the models without and with the ‘best’ predictor set of seven health variables were compared. As 14.6% of the sample had missing values on one or more variables, 12.8% of which was due to medication use only, multiple imputation was applied. Fifteen imputations were performed. If a health variable showed a non-linear association, its quadratic term was tested in the case of a continuous variable, or dummies were created.
Results
Descriptives
At baseline, 333 participants currently had a paid job, whereas 2,198 had no current job but had held a job earlier in life. 76 participants had moved from their longest-held job to their current job, 71 of which currently worked in a different occupational domain.
Table 1 shows the distribution of socio-demographic characteristics across the domains. The percentage of males is highest in the transport domain (92.9%), followed by the agricultural and technical domains (79.4 and 77.5%, respectively), and lowest in the care domain (12.5%). The average level of education is highest in the teaching domain (13.6 years) and lowest in the non-skilled general domain (6.8 years). Likewise, average skill levels are highest in the teaching and “other” domains (4.3 and 4.0) and lowest in the elementary domain (1.0), but among the other five domains, there are no clear differences (circa 2.6). Note, that the standard deviation of years of education is relatively high in the care domain, reflecting that this domain includes substantial numbers of both lower and higher educated workers. The highest percentages of currently paid workers are observed in the agriculture and teaching domains (21.1 and 17.1%, respectively). In the non-skilled general, care and administrative domains, the highest percentages of people without paid work younger than 65 years and not retired are observed (21.9, 21.3, and 21.2%, respectively). The highest percentages of (early) retirees are found in the transport and technical domains (76.8 and 74.7%, respectively). Differences between domains regarding all socio-demographic characteristics are statistically significant at p < 0.001, indicating cross-domain heterogeneity.
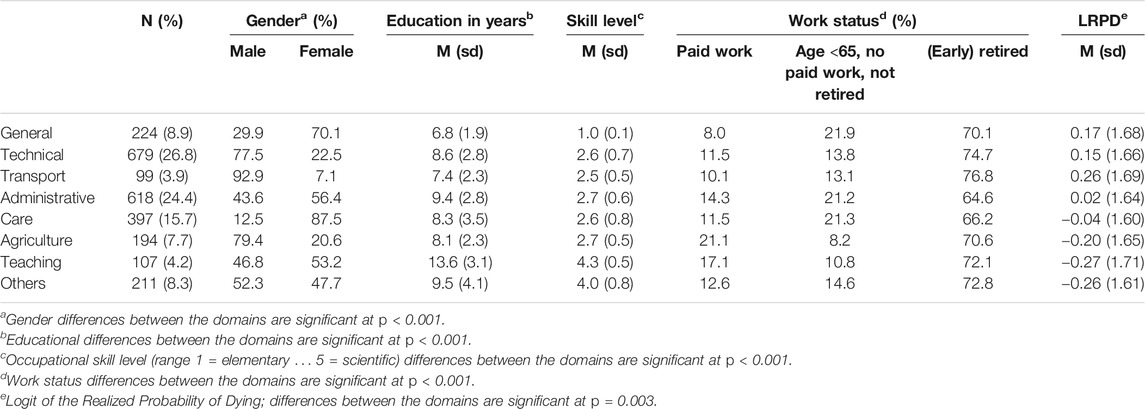
TABLE 1. Socio-demographic characteristics of the occupational domains selected. Source: Longitudinal Aging Study Amsterdam, 1992–93 (n = 2,531).
The distribution of the five key health indicators across the occupational domains is shown in Table 2. Although health differences between the selected domains and the “other” domain are apparent, only few differences reach statistical significance at p < 0.05. Considering only these differences, it is seen that workers in the technical domain have relatively many and in the agriculture domain have relatively few sick days; workers in the non-skilled general domain use relatively many medications and are relatively often admitted to a hospital; and workers in the technical domain have relatively few family physician contacts.
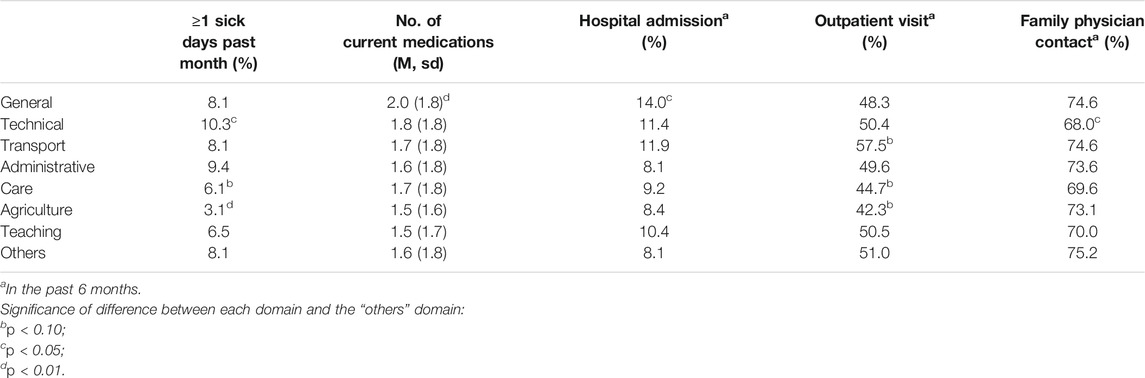
TABLE 2. Key health characteristics of the occupational domains selected. Source: Longitudinal Aging Study Amsterdam, 1992–93 (n = 2,531).
Association of Occupational Domain With Life Expectancy
The Logit of the Realized Probability of Dying (LRPD) ranges from +0.26 for the transport domain to −0.27 for the teaching domain (overall cross-domain difference: p = 0.003, Table 1). Figure 1 shows the LRPD-derived remaining life expectancies for men and women at age 65. The transport domain is characterized by the shortest life expectancy, i.e., 14.7 years for men and 20.0 years for women. The teaching domain is characterized by the longest life expectancy, i.e., 18.3 years for men and 23.1 years for women. For men, the difference amounts to 3.6 years; for women, this is 3.1 years. In-between are, in ascending order, the non-skilled general, technical, administrative, care, and agriculture domains. The population median of the survival time at age 65 is 16.5 years for men and 21.5 years for women. Thus, on the lowest end of the spectrum, the transport domain’s life expectancy is 1.8 years below the population median for men and 1.5 years for women. On the other end of the spectrum, the survival time advantage of the teaching domain is 1.8 years for men and 1.6 years for women. These differences would imply that male workers in the transport domain would be allowed for example a 1 + 1.8/16.5 = 1.1 greater pension build-up during their working years, accompanied by a proportionally earlier statutory retirement age than male workers in a domain with a median life expectancy.
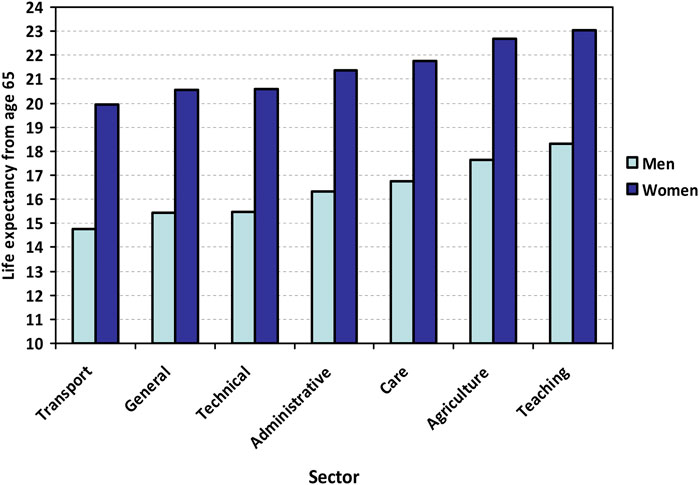
FIGURE 1. Life expectancy by occupational domain for men (light colors) and women (dark colors) at age 65. Note: The data are based on analysis of domain specific relative survival using the full sample, the results of which are compared to the population-based sex-specific survival curves at age 65 in order to obtain life expectancies (Supplementary Figure A). Source: Longitudinal Aging Study Amsterdam, 1992–93 to 2019.
A further test of these differences using linear regression analysis (model 1 in Table 3) yields statistically significantly higher LRPDs for, in descending order, the transport, the non-skilled general, the technical, and the administrative domain as compared to the other, non-defined domains (Bs ranging from 0.503 to 0.264; p < 0.05). Adding work status to the regression model somewhat decreases the regression coefficients, such that the coefficient for the administrative section no longer reaches statistical significance (model 2 in Table 3).
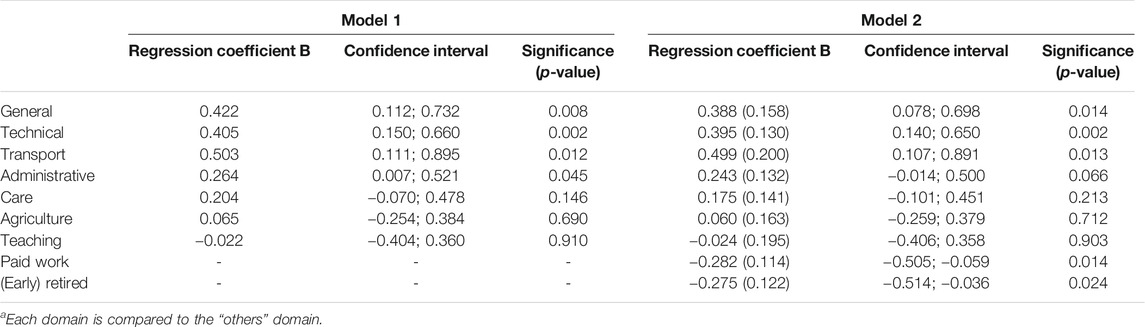
TABLE 3. Linear regression models of LRPD on occupational domain1, adjusted for age (model 1), and adjusted for age and work status (model 2). Source: Longitudinal Aging Study Amsterdam (N = 2,531).
The Role of Health
To test the role of health, first, in five separate regression analyses, one for each health variable, the occupational domain coefficients are compared between non-health-adjusted and health-adjusted models (Supplementary Table B). It is observed that number of sick days, number of medications, hospital admission, and outpatient visits are strongly associated with survival time, but family physician contact is not. Of all health variables, the number of medications explains the largest portion, ranging from 16.2% for the non-skilled general domain to 9.1% for the administrative domain. Contact with the family physician does not contribute any explanatory value.
The final model including all five health variables yields similar decreases in the B-coefficients of the non-skilled general, transport, technical, and administrative domains, ranging between 19.0 and 20.9% (Table 4).
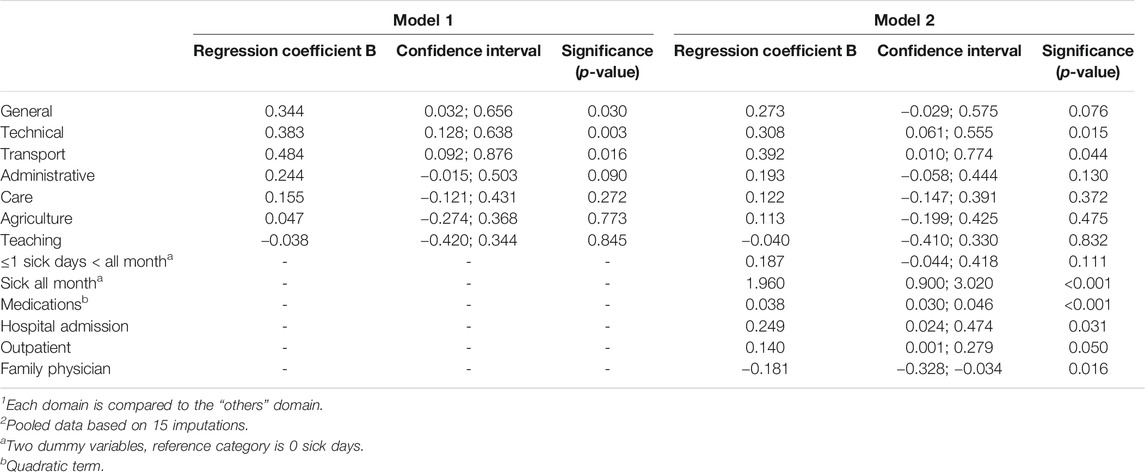
TABLE 4. Linear regression models of LRPD on occupational domain1, adjusted for age and work status (model 1), and additionally adjusted for five health variables (models 2). Imputed data (n = 2,531)2. Source: Longitudinal Aging Study Amsterdam, 1992–93 to 2019.
Sensitivity Analysis
The ‘best’ predictor set of seven health measures accounts for a substantially larger portion of the association between occupational domains and longevity (Supplementary Table C). The greatest decrease in the B-coefficient is observed for the non-skilled general domain, amounting to 66.5%. For the technical and transport domains, the percentage decrease is 48.1 and 41.9%, respectively. For the administrative domain, the portion accounted for is 20.2%, and thus similar to that using the initial five health measures.
Discussion
In this study, we provided evidence regarding the extent of quantitative differences in longevity between occupational domains. As a proof of principle for the determination of actuarially fair statutory retirement ages, we indeed found domain differences, in that the non-skilled general, technical, and transport domains had significantly shorter survival times than the domains with academic professions. These findings correspond to the scarce literature that links occupational domain to mortality (e.g., Moore and Hayward, 1990; Johnson et al., 1999, Katikireddi et al., 2017, Lee et al., 2016). Furthermore, we found for four of our five health indicators which are presumably retrievable from registries, that they explained a substantial portion of the association of domain with longevity. The joint contribution of these health variables was about 20% for the domains with the shortest life expectancy. The contribution of a “best” predictor set of health indicators ranged from 66.5 to 20.2%, with the largest percentage in the domain with the shortest life expectancy. These findings suggest that health differentiates among occupational domains in a similar way as life expectancy.
Comparison of the differences in life expectancy found in our study with the pertinent scientific literature is not straightforward. In reports from the few studies addressing the association of occupation or occupational domain with mortality, the findings are commonly expressed as risk ratios or percentages excess mortality. Therefore, differences in life expectancy expressed in years, such as our study reports, are not directly comparable. The only study reporting between-domain differences in life expectancy in years, to our knowledge, showed a maximum difference of 4.5 years at age 55 (Moore and Hayward, 1990). Considering the mean life expectancy at age 55 is larger than at age 65, this difference is comparable to the one we found at age 65. Our earlier study on work conditions and life expectancy at age 55 showed a maximum difference between unfavorable and favorable work conditions of 2.6 years for men and 2.3 for women (de Wind et al., 2020). The latter are smaller than the domain differences found in the current study. A German study in 16–65-year-olds of the effect of working conditions on self-rated health found a 16-months “ageing effect” of high physical strain, and a 6-months effect of low control (Ravesteijn et al., 2018). Although the use of a different health measure and different occupational characteristics do not allow a close comparison with our own study, these effects are also smaller than the domain-effects on survival time in our current study. Thus, occupational domain is certainly a relevant criterion to differentiate shorter from longer life expectancies.
It may be argued that health behaviors are a major factor at work in occupation-related differences in health, because unhealthy behaviors are shared within occupations (Fox and Adelstein, 1978; Johnson et al., 1999). The implication would be that differences in longevity by occupational domain arise from behavior that is independent from employment and thus should not be accounted for in pension policy. One study of workers in the construction sector compared the variance explained in work ability by health conditions and health behaviors on the one hand and work-related factors on the other hand (Alavinia et al., 2007). Health behaviors, including obesity, physical activity, and smoking, explained less than 1% over and above age and occupational status. In contrast, the explanatory value of the work-related factors, including both physical and psychosocial demands, was 22%. Several other studies on work ability adjusted for health behaviors and provide evidence that occupational factors impact health outcomes independently of health behavior (Lund and Csonka, 2003; Andersen et al., 2016; Schram et al., 2021). In an extra analysis of our own dataset, we have added the lifestyle factors smoking, heavy alcohol consumption, obesity, and minutes spent on physical activity (walking, doing a sport) as confounders to our basic analytic model (i.e., model 2 in Table 3). Interestingly, these factors acted as suppressors, i.e., the regression coefficients for the general, technical and transport domains became stronger. This was due to the fact that the domain “other” included substantially more current smokers and heavy alcohol consumers than the specified domains, while this category had a relatively long survival time. In our study, therefore, unhealthy behaviour does not explain the association between domains and longevity. These findings once again support the relevance of occupational domain for pension policy.
From the identification of health indicators as explanatory factors, two kinds of implications can be noted. One is, in line with our second research question, to differentiate between occupational domains in determining pensionable ages. The other one is to improve health in the workplace. Numerous observational studies on work and health have noted the latter implication. However, health intervention studies in the workplace so far do not show very promising results, i.e. effect sizes are small, if any (e.g., Hazelzet et al., 2019; Söderbacka et al., 2020). Until effective interventions are designed, differentiation of pension policies based on health differences between occupational domains is recommendable to reach greater actuarial fairness.
Strengths and Limitations
We emphasize that our findings should be considered as preliminary. Nevertheless, we can already state some strengths and limitations. The sample used is representative for the older Dutch population in 1992–93. Our analyses use occupational domain as the basis, because domains closely correspond to industrial sectors, and in the Netherlands pension policies are negotiated by social partners in sector-specific collective bargaining and occupational pension funds are organized by sector. Thus, implementation of our model is facilitated. The measure of survival time used is sensitive to inter-individual differences, because it is based on age and gender, and is prospective. Furthermore, our exposure variable was longest-held job rather than current job, so that health selection through transition to less strenuous jobs was precluded (Moore and Hayward, 1990).
In addition to these strengths, there are some weaknesses. First, the sample is relatively small when it comes to studying occupational domains more comprehensively. We selected the seven largest domains, based on a rule of thumb that their sample size is not smaller than 90. This makes it difficult to compare our findings to studies that had much larger sample sizes available and were able to make finer distinctions (e.g., Katikireddi et al., 2017). Also, the reference category consisted of the non-selected domains, in which a variety of smaller domains was collapsed, although they generally consisted of professional occupations, with concomitant greater survival times. With a larger sample size, we might have been able to observe clearer survival differences between domains. Furthermore, as there are gender differences in lifetime careers, even within a domain (Cambois et al., 2017; Riekhoff and Järnefelt 2017; Amiri and Behnezhad, 2020) and women tend to live longer than men, gender-specific analyses would have been preferable, but were not possible due to the relatively small sample size. However, as the survival measure LRPD is based on sex and age, gender differences in longevity were accounted for.
A second issue is that our sample has a broad age range (55–85 years). This implies that survival effects were examined of working in occupational domains, the exposure to which may have taken place decades earlier. Possibly, the effects of this exposure have weakened over the years. Regardless, we still find substantial survival time differences between domains. The long-lasting effect of working conditions on health is supported by studies of post-retirement health that showed that the health effects of poor working conditions lasted as long as 15 years post-retirement (Gueorguieva et al., 2009; de Breij et al., 2019).
A third limitation is that we were not able to incorporate the fact that individuals do not remain in the same occupation throughout their full careers (Kreiner et al., 2018). However, we did include the job that the participants had held during the longest time. Moreover, for participants currently working, their longest-held job was used if their current job was not the same as their longest-held job. Unfortunately, we did not have information on the duration of working in each occupational domain. In future research, using such information, work histories may be constructed for those participants who changed domain during their working career. Then, “weights” could be assigned to career years depending on domain. For example, 1 year working in non-skilled general occupations could be counted as 1.1 years in pension build-up.
A fourth issue may be that we initially selected health measures that could be retrieved relatively easily from registers available to pension funds. Although four of the five measures proved to be strongly associated with survival time, together they explained only 20%, a substantial yet relatively small portion of the association of occupational domain and survival time. In a sensitivity analysis, more direct health measures that have been shown to be strong and consistent predictors of longevity (Suemoto et al., 2017), including diseases and impairments, explained much greater portions, with a maximum of 66% for non-skilled general occupations. Although these measures are less likely to be available in registers, they confirm that for the longevity of non-skilled and skilled manual occupational domains, health is a strong explanatory factor.
Fifth, it may be argued that we included only a few covariates. Again, this choice was motivated by the likely availability of covariates in registers available to pension funds. We would like to stress, meanwhile, that we were primarily interested in longevity differences between occupational domains per se, because of their applicability in practice. Thus, we did not pursue a study of the unique predictive ability of domain for survival time given other individual and work-related characteristics.
As a final point, it must be acknowledged that working conditions within an occupational domain may vary substantially, which may result in substantial differences in life expectancy. Translated to industrial sectors, thus, unfairness within sectors may remain when applying sector-based pension rules. This may have implications for public acceptance of such rules, and may reduce predictability of pension benefits and timing.
To apply research findings in practice, the uncertainty that surrounds estimates and that is inherent in survey research should be minimized. As a further step, therefore, we recommend that the same research questions are addressed using national data from countries that have long-standing population-wide registry data available. Using such data would solve the sample size related limitations of our study. Due to somewhat different occupational distributions and prevalence of part-time work, however, the effects of having a certain occupation on health and life expectancy might vary across countries and time periods. Hence, the comparison would also act as a “robustness check” of the proposed mechanism, to see if its performance is dependent on certain circumstances. For the current study, however, we started with Dutch data with a long survival follow-up in order to provide a proof of principle.
Conclusion
In this study, we aimed to provide a proof of principle of a mechanism to reach greater actuarial fairness by linking retirement ages to occupation-based life expectancies. We showed that the non-skilled general, technical, and transport domains have a shorter life expectancy than the professional domains, amounting to 3.5 years for a man, and 3.1 years for a woman aged 65 years. Statutory retirement age could be made to vary accordingly, by allowing a proportionally greater pension build-up in the shorter-lived domains. Also, we were able to show that health accounted for a substantial portion of the association between occupational domain and longevity. Thus, health differences between domains today can be used as a means to tailor retirement ages to individuals’ risks of longevity. This method provides a basis for pension policies of greater actuarial fairness, by linking pension build-up and statutory retirement ages to occupation-based life expectancies, instead of holding on to a one-size-fits-all statutory retirement age.
Data Availability Statement
A publicly available dataset was analyzed in this study. This dataset can be found here: https://lasa-vu.nl/en/request-data/. Data from the Longitudinal Aging Study Amsterdam are available for use for specific research questions provided that an agreement is made up.
Ethics Statement
The studies involving human participants were reviewed and approved by the Medical Ethics Committee, VU University Medical Center, archive number 92/138. The participants provided their informed consent to participate in this study.
Author Contributions
Conceptualization, WT and DD; methodology, DD and SB; writing - original draft preparation, DD and WT; writing - revision and editing, DD, SB, and WT.
Funding
The Longitudinal Aging Study Amsterdam is supported by a grant from the Netherlands Ministry of Health, Welfare and Sport, Directorate of Long-Term Care. This study was funded by the Network for Studies on Pensions, Aging and Retirement, grant number CRG 2018.02.
Conflict of Interest
The authors declare that the research was conducted in the absence of any commercial or financial relationships that could be construed as a potential conflict of interest.
Publisher’s Note
All claims expressed in this article are solely those of the authors and do not necessarily represent those of their affiliated organizations, or those of the publisher, the editors and the reviewers. Any product that may be evaluated in this article, or claim that may be made by its manufacturer, is not guaranteed or endorsed by the publisher.
Supplementary Material
The Supplementary Material for this article can be found online at: https://www.frontiersin.org/articles/10.3389/fsoc.2021.675618/full#supplementary-material
References
Alavinia, S. M., van Duivenbooden, C., and Burdorf, A. (2007). Influence of Work-Related Factors and Individual Characteristics on Work Ability Among Dutch Construction Workers. Scand. J. Work Environ. Health 33 (5), 351–357. doi:10.5271/sjweh.1151
Amiri, S., and Behnezhad, S. (2020). Job Strain and Mortality Ratio: a Systematic Review and Meta-Analysis of Cohort Studies. Public Health 181, 24–33. doi:10.1016/j.puhe.2019.10.030
Andersen, L. L., Fallentin, N., Thorsen, S. V., and Holtermann, A. (2016). Physical Workload and Risk of Long-Term Sickness Absence in the General Working Population and Among Blue-Collar Workers: Prospective Cohort Study with Register Follow-Up. Occup. Environ. Med. 73 (4), 246–253. doi:10.1136/oemed-2015-103314
Barnay, T. (2007). Redistributive Impact of Differential Mortality in the French Pay-As-You-Go System. Geneva Pap. Risk Insur Issues Pract. 32 (4), 570–582. doi:10.1057/palgrave.gpp.2510145
Brønnum-Hansen, H., Foverskov, E., and Andersen, I. (2020). Occupational Inequality in Health Expectancy in Denmark. Scand. J. Public Health 48, 338–345. doi:10.1177/1403494819882138
Cambois, E., Garrouste, C., and Pailhé, A. (2017). Gender Career divide and Women's Disadvantage in Depressive Symptoms and Physical Limitations in France. SSM Popul. Health 3, 81–88. doi:10.1016/j.ssmph.2016.12.008
Cassidy, H. (2017). Task Variation within Occupations. Ind. Relat. 56 (3), 393–410. doi:10.1111/irel.12179
de Breij, S., Qvist, J. Y., Holman, D., Mäcken, J., Seitsamo, J., Huisman, M., et al. (2019). Educational Inequalities in Health after Work Exit: the Role of Work Characteristics. BMC Public Health 19 (1), 1515. doi:10.1186/s12889-019-7872-0
De Preter, H., Mortelmans, D., and Van Looy, D. (2012). Retirement Timing in Europe: Does Sector Make a Difference? Ind. Relat. J. 43 (6), 511–526. doi:10.1111/j.1468-2338.2012.00699.x
De Tavernier, W. (2020). “Indexing the Retirement Age to Life Expectancy,” in Alte und neue sociale Ungleichheiten bei Berufsaugabe und Rentenübergang. Ergebnisse des EXTEND-Projektes [Old and new social inequalities in working life and retirement. Results from the EXTEND-project]. Editors G. Naegele, and M. Hess (Wiesbaden: Springer VS), 133–138. doi:10.1007/978-3-658-31663-1_6
de Wind, A., Sewdas, R., Hoogendijk, E. O., van der Beek, A. J., Deeg, D. J. H., and Boot, C. R. L. (2020). Occupational Exposures Associated with Life Expectancy without and with Disability. Int. J. Environ. Res. Public Health 17 (17), E6377. doi:10.3390/ijerph17176377
Deeg, D. J., van Oortmarssen, G. J., Habbema, J. D., and van der Maas, P. J. (1989). A Measure of Survival Time for Long-Term Follow-Up Studies of the Elderly. J. Clin. Epidemiol. 42 (6), 541–549. doi:10.1016/0895-4356(89)90150-9
Deeg, D. J., van Tilburg, T., Smit, J. H., and de Leeuw, E. D. (2002). Attrition in the Longitudinal Aging Study Amsterdam. The Effect of Differential Inclusion in Side Studies. J. Clin. Epidemiol. 55, 319–328. doi:10.1016/S0895-4356(01)00475-9
Eibich, P. (2015). Understanding the Effect of Retirement on Health: Mechanisms and Heterogeneity. J. Health Econ. 43, 1–12. doi:10.1016/j.jhealeco.2015.05.001
Finnish Centre for Pensions (2015). Years-of-service Pension: Rare Arrangement in Europe. Online available at: http://www.etk.fi/en/uutinen/years-of-service-pension-rare-arrangement-in-europe/. (retrieved 03 06, 2017).
Folstein, M. F., Folstein, S. E., and McHugh, P. R. (1975). Mini-Mental State: a Practical Method for the Clinician. J. Psychiatr. Res. 12, 190–198. doi:10.1016/j.jhealeco.2015.05.001
Fox, A. J., and Adelstein, A. M. (1978). Occupational Mortality: Work or Way of Life? J. Epidemiol. Community Health 32, 73–78. doi:10.1136/jech.32.2.73
Goldman, N., Glei, D. A., and Weinstein, M. (2016). What Matters Most for Predicting Survival? A Multinational Population-Based Cohort Study. PloS ONE 11 (7), e0159273. doi:10.1371/journal.pone.0159273
Gueorguieva, R., Sindelar, J. L., Falba, T. A., Fletcher, J. M., Keenan, P., Wu, R., et al. (2009). The Impact of Occupation on Self-Rated Health: Cross-Sectional and Longitudinal Evidence from the Health and Retirement Survey. J. Gerontol. B Psychol. Sci. Soc. Sci. 64 (1), 118–124. doi:10.1093/geronb/gmb00610.1093/geronb/gbn006
Hazelzet, E., Picco, E., Houkes, I., Bosma, H., and de Rijk, A. (2019). Effectiveness of Interventions to Promote Sustainable Employability: A Systematic Review. Int. J. Environ. Res. Public Health 16, 1985. doi:10.3390/ijerph16111985
Hoogendijk, E. O., Deeg, D. J. H., de Breij, S., Klokgieters, S. S., Kok, A. A. L., Stringa, N., et al. (2020). The Longitudinal Aging Study Amsterdam: Cohort Update 2019 and Additional Data Collections. Eur. J. Epidemiol. 35 (1), 61–74. doi:10.1007/s10654-019-00541-2
Iacob, S., Hersant, B., Mezi, M. S., and Meningaud, J. P. (2016). Factors that May Enhance Longevity: A Literature Review and a Comprehensive Update for Aesthetic Surgeons. Aesthet. Plast Surg. 40, 625–631. doi:10.1007/s00266-016-0648-3
Johnson, N. J., Sorlie, P. D., and Backlund, E. (1999). The Impact of Specific Occupation on Mortality in the U.S. National Longitudinal Mortality Study. Demography 36 (3), 355–367. doi:10.2307/2648058
Katikireddi, S. V., Leyland, A. H., McKee, M., Ralston, K., and Stuckler, D. (2017). Patterns of Mortality by Occupation in the UK, 1991-2011: a Comparative Analysis of Linked Census and Mortality Records. Lancet Public Health 2, e501–512. doi:10.1016/S2468-2667(17)30193-7
Kreiner, C. T., Nielsen, T. H., and Serena, B. L. (2018). Role of Income Mobility for the Measurement of Inequality in Life Expectancy. Proc. Natl. Acad. Sci. U S A. 115 (46), 11754–11759. doi:10.1073/pnas.1811455115
Kriegsman, D. M., Penninx, B. W., van Eijk, J. T., Boeke, A. J., and Deeg, D. J. (1996). Self-reports and General Practitioner Information on the Presence of Chronic Diseases in Community Dwelling Elderly. A Study on the Accuracy of Patients' Self-Reports and on Determinants of Inaccuracy. J. Clin. Epidemiol. 49, 1407–1417. doi:10.1016/S0895-4356(96)00274-0
Kunst, A. E., and Mackenbach, J. P. (1994). The Size of Mortality Differences Associated with Educational Level in Nine Industrialized Countries. Am. J. Public Health 84, 932–937. doi:10.2105/AJPH.84.6.932
Landes, X. (2015). How Fair Is Actuarial Fairness?. J. Bus. Ethics 128 (3), 519–533. doi:10.1007/s10551-014-2120-0
Lee, H.-E., Kim, H.-R., Chung, Y. K., Kang, S.-K., and Kim, E.-A. (2016). Mortality Rates by Occupation in Korea: A Nationwide, 13-year Follow-up Study. Occup. Environ. Med. 73, 329–335.
Leinonen, T., Viikari-Juntura, E., Husgafvel-Pursiainen, K., and Solovieva, S. (2018). Cause-specific Sickness Absence Trends by Occupational Class and Industrial Sector in the Context of Recent Labour Market Changes: a Finnish Panel Data Study. BMJ Open 8, e019822. doi:10.1136/bmjopen-2017-019822
Llena-Nozal, A., Lindeboom, M., and Portrait, F. (2004). The Effect of Work on Mental Health: Does Occupation Matter?. Health Econ. 13, 1045–1062. doi:10.1002/hec.929
Lund, T., and Csonka, A. (2003). Risk Factors in Health, Work Environment, Smoking Status, and Organizational Context for Work Disability. Am. J. Ind. Med. 44, 492–501. doi:10.1002/ajim.10298
Mikkola, T. M., von Bonsdorff, M. B., Salonen, M. K., Kautiainen, H., Ala-Mursula, L., Solovieva, S., et al. (2019). Physical Heaviness of Work and Sitting at Work as Predictors of Mortality: A 26-Year Follow-Up of the Helsinki Birth Cohort Study. BMJ Open 9, e026280. doi:10.1136/bmjopen-2018-026280
Mood, C. (2010). Logistic Regression: Why We Cannot Do what We Think We Can Do, and what We Can Do about it. Eur. Sociol. Rev. 26 (1), 67–82. doi:10.1093/esr/jcp006
Moore, D. E., and Hayward, M. D. (1990). Occupational Careers and Mortality of Elderly Men. Demography 27 (1), 31–53. doi:10.2307/2061551
Myles, J. (2003). What justice Requires: Pension Reform in Ageing Societies. J. Eur. Soc. Pol. 13 (3), 264–269. doi:10.1177/09589287030133004
Natali, D., Spasova, S., and Vanhercke, B. (2016). Retirement Regimes for Workers in Arduous or Hazardous Jobs in Europe: A Study of National Policies. Brussels: European Commission.
Östergren, O., Lundberg, O., Artnik, B., Bopp, M., Borrell, C., Kalediene, R., et al. (2017). Educational Expansion and Inequalities in Mortality—A Fixed-Effects Analysis Using Longitudinal Data from 18 European Populations. PLoS ONE 12 (8), e0182526. doi:10.1371/journal.pone.0182526
Queisser, M., and Whitehouse, E. R. (2006). Neutral or Fair? Actuarial Concepts and Pension-System Design. OECD Social, Employment and Migration Working Papers. No. 40. (Paris: OECD Publishing). doi:10.1787/1815199X
Ravesteijn, B., Kippersluis, H. V., and Doorslaer, E. V. (2018). The Wear and Tear on Health: What Is the Role of Occupation? Health Econ. 27 (2), e69–e86. doi:10.1002/hec.3563
Riekhoff, A.-J., and Järnefelt, N. (2017). Gender Differences in Retirement in a Welfare State with High Female Labour Market Participation and Competing Exit Pathways. Eur. Sociol. Rev. 33 (6), 791–807. doi:10.1093/esr/jcx077
Rijs, K. J., van der Pas, S., Geuskens, G. A., Cozijnsen, R., Koppes, L. L., van der Beek, A. J., et al. (2014). Development and Validation of a Physical and Psychosocial Job-Exposure Matrix in Older and Retired Workers. Ann. Occup. Hyg. 58 (2), 152–170. doi:10.1093/annhyg/met052
Romeu, G. L., and Skirbekk, V. (2013). Skill Demand and the Comparative Advantage of Age: Jobs Tasks and Earnings from the 1980s to the 2000s in Germany. Labour Econ. 22, 61–69. doi:10.1016/j.labeco.2012.09.003
Rutherford, M. J., Dickman, P. W., and Lambert, P. C. (2012). Comparison of Methods for Calculating Relative Survival in Population-Based Studies. Cancer Epidemiol. 36, 16–21. doi:10.1016/j.canep.2011.05.010
Scharn, M., van der Beek, A. J., Suanet, B., Huisman, M., and Boot, C. R. L. (2019). Societal Participation of Individuals Aged 55-64 Years with and without Chronic Disease. Eur. J. Public Health 29 (1), 93–98. doi:10.1093/eurpub/cky122
Schokkaert, E., and Van Parijs, P. (2003). Debate on Social justice and Pension Reform. J. Eur. Soc. Pol. 13 (3), 245–263. doi:10.1177/09589287030133003
Schram, J. L., Oude Groeniger, J., Schuring, M., Proper, K. I., van Oostrom, S. H., Robroek, S. J., et al. (2021). Working Conditions and Health Behavior as Causes of Educational Inequalities in Self-Rated Health: an Inverse Odds Weighting Approach. Scand. J. Work Environ. Health 47 (2), 127–135. doi:10.5271/sjweh.3918
Simonovits, A. (2015). Benefit-Retirement Age Schedules and Redistribution in Public Pension Systems. Czech J. Econ. Finance 65 (5), 362–376.
Söderbacka, T., Nyholm, L., and Fagerström, L. (2020). Workplace Interventions that Support Older Employees' Health and Work Ability - a Scoping Review. BMC Health Serv. Res. 20, 472. doi:10.1186/s12913-020-05323-1
Statistics Netherlands (2001). Standaard Beroepenclassificatie 1992 [Netherlands Standard Classification of Occupations 1992]. Edition 2001. Heerlen, Netherlands: Statistics Netherlands. [In Dutch].
Statistics Netherlands. (2021). Standaard Bedrijfsindeling 2008; Toelichting [Standard Classification of Industries 2008; Explanation]. [In Dutch]. Available at: https://opendata.cbs.nl/statline#/CBS/nl/dataset/83583NED/table?ts=1625662947369. (Accessed July 7, 2021).
Suemoto, C. K., Ueda, P., Beltrán-Sánchez, H., Lebrão, M. L., Duarte, Y. A., Wong, R., et al. (2017). Development and Validation of a 10-year Mortality Prediction Model: Meta-Analysis of Individual Participant Data from Five Cohorts of Older Adults in Developed and Developing Countries. J. Gerontol. A. Biol. Sci. Med. Sci. 72 (3), 410–416. doi:10.1093/gerona/glw166
Tanaka, H., Nusselder, W. J., Bopp, M., Brønnum-Hansen, H., Kalediene, R., Lee, J. S., et al. (2019). Mortality Inequalities by Occupational Class Among Men in Japan, South Korea and Eight European Countries: a National Register-Based Study, 1990-2015. J. Epidemiol. Community Health 73, 750–758. doi:10.1136/jech-2018-211715
Tjepkema, M., Wilkins, R., and Long, A. (2013). Cause-specific Mortality by Occupational Skill Level in Canada: a 16-year Follow-Up Study. Chronic Dis. Inj. Can. 33 (4), 195–203. doi:10.24095/hpcdp.33.4.01
Unger, R., and Schulze, A. (2013). Can We Really (All) Work Longer? Trends in Healthy Life Expectancy According to Social Stratum in Germany. Comparative Pop. Stud. – Z. Bevölkerungswissenschaft 38 (3), 562–582. doi:10.4232/10.CPoS-2013-03en10.12765/cpos-2013-03
Van Oyen, H., Van der Heyden, J., Perenboom, R., and Jagger, C. (2006). Monitoring Population Disability: Evaluation of a New Global Activity Limitation Indicator (GALI). Soz. Praventivmed. 51, 153–161. doi:10.1007/s00038-006-0035-y
Keywords: retirement age, occupation, longevity, health, longitudinal study
Citation: Deeg DJ, De Tavernier W and de Breij S (2021) Occupation-Based Life Expectancy: Actuarial Fairness in Determining Statutory Retirement Age. Front. Sociol. 6:675618. doi: 10.3389/fsoc.2021.675618
Received: 03 March 2021; Accepted: 05 August 2021;
Published: 23 August 2021.
Edited by:
Moritz Hess, Hochschule Niederrhein, GermanyReviewed by:
Elias Naumann, Harvard University, United StatesAart-Jan Riekhoff, Finnish Centre for Pensions (ETK), Finland
Copyright © 2021 Deeg, De Tavernier and de Breij. This is an open-access article distributed under the terms of the Creative Commons Attribution License (CC BY). The use, distribution or reproduction in other forums is permitted, provided the original author(s) and the copyright owner(s) are credited and that the original publication in this journal is cited, in accordance with accepted academic practice. No use, distribution or reproduction is permitted which does not comply with these terms.
*Correspondence: Dorly J.H. Deeg, djh.deeg@amsterdamumc.nl