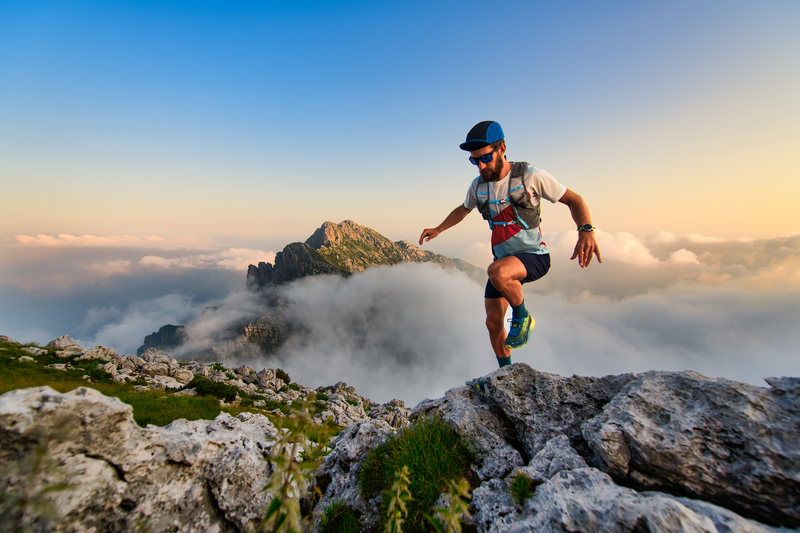
94% of researchers rate our articles as excellent or good
Learn more about the work of our research integrity team to safeguard the quality of each article we publish.
Find out more
REVIEW article
Front. Sens. , 14 September 2023
Sec. Biosensors
Volume 4 - 2023 | https://doi.org/10.3389/fsens.2023.1250756
Wound infections are a major problem worldwide, both for the healthcare system and for patients affected. Currently available diagnostic methods to determine the responsible germs are time-consuming and costly. Wound infections are mostly caused by various bacteria, which in turn produce volatile organic compounds. From clinical experience, we know that depending on the bacteria involved, a specific odor impression can be expected. For this reason, we hypothesized that electronic noses, i.e., non-invasive electronic sensors for the detection of volatile organic compounds, are applicable for diagnostic purposes. By providing a comprehensive overview of the state-of-research, we tested our hypothesis. In particular, we addressed three overarching questions: 1) which sensor technologies are suitable for the diagnosis of wound infections and why? 2) how must the (biological) sample be prepared and presented to the measurement system? 3) which machine learning methods and algorithms have already proven successful for the classification of microorganisms? The corresponding articles have critically been reviewed and are discussed particularly in the context of their potential for clinical diagnostics. In summary, it can already be stated today that the use of electronic noses for the detection of bacteria in wound infections is a very interesting, fast and non-invasive method. However, reliable clinical studies are still missing and further research is necessary.
Wounds, and chronic wounds in particular, represent a steadily increasing challenge for patients and for healthcare systems worldwide. One of the main reasons is the increasing life expectancy of the population. As the incidence of chronic wounds increases with age, body weight, and comorbidities, the number of affected individuals is expected to continue to rise (Weber and Deinsberger, 2022). It is estimated that 2%–3% of the adult population currently suffers from a chronic wound (Sen, 2021). For example, in Germany it is assumed that approximately 11.5% of people cared for by outpatient nursing services and approximately 7.8% of people cared for in nursing homes suffer from chronic wounds (Raeder et al., 2019). The most common manifestations of chronic wounds are venous leg ulcers, diabetic foot ulcers and pressure ulcers. It is estimated that the financial expenditure of the healthcare system for these patients in Germany amounts to at least five billion euros per year (Horch et al., 2008). In addition to the problems and costs caused by the wound itself, there may be other complications associated with patients with wounds. Here, wound infections are a typical and also feared problem that can develop into sepsis and thus a potentially life-threatening situation (Cecconi et al., 2018; Dissemond, 2023). So it becomes clear that wound infections are a multidisciplinary relevant problem that should be diagnosed and adequately treated as soon as possible.
If wound healing is interrupted and the wound tissue is damaged by the multiplication of microorganisms evoking a response from the host, a wound can be considered as clinically infected (International Wound Infection Institute, 2022). E. coli, P. aeruginosa and S. aureus are three common bacteria species associated with clinically infected wounds (Tian et al., 2009; Bessa et al., 2015; Maddocks, 2017). To provide a bacteria-specific medical treatment, culture-based and molecular-based methods from swabs are commonly used for diagnosis. These state-of-the-art techniques are, however, in terms of culture-based methods not generally error-free and overall quite time-consuming and costly (Ashrafi et al., 2017; Xu et al., 2020; Li et al., 2021).
Current literature reveals that bacteria produce specific volatile organic compounds (VOCs) (Bos et al., 2013; Wang et al., 2016). This is why besides gas chromatography mass spectrometers and ion mobility spectrometers, so called electronic noses (e-noses) could become valuable instruments for noninvasive bacteria detection (Daulton et al., 2020). E-nose systems generally consist of an array of sensors, each sensitive to multiple volatiles, a sample collection chamber for fresh air and for the headspace of the specimen, a transducer and the software unit for pattern recognition and further statistical analyses (Wilson, 2016; Saggio, 2020). Such instruments have been used to identify bacteria or to diagnose different diseases by, for example, analyzing and discriminating between the different VOC profiles of human breath (Bos et al., 2013; Wilson, 2016; Dragonieri et al., 2017). Despite their theoretical applicability, e-noses have not yet been widely implemented in clinical practice. However, a suitable device being able to detect bacteria in wound infections in an early stage could initiate an optimal treatment (Benjamens et al., 2020). As a result, worsening of the infection or favoring of antimicrobial resistances could be avoided (Daulton et al., 2020).
Therefore, with our review we aim to address the challenges and advancements in the use of e-noses to support wound infection diagnosis. We consider it from the a) microbiological, b) technical and c) software point of view. For this purpose, we a) relate the sample characteristics and sampling methods to their impact on the results, b) present and discuss the available e-nose sensor types with regard their suitability for the detection of bacteria in wounds, and c) present algorithms and machine learning (ML) methods already been used for improving the performance of the e-nose system in context of infection detection. We will continue to illustrate why there is not just one best technology and multiple solutions to this problem can be purposeful. Based on these aspects, existing challenges and missing steps will be investigated.
Altogether, we aim to provide an overview of this topic including the current progress, restrictions and giving an indication for working with these e-nose systems for bacteria detection in wound infections in clinical practice.
A literature search was performed via four different searching engines and databases, respectively (i.e., Google Scholar, PubMed, IEEE Xplore and Springer Link). At the beginning of the literature search, various combinations of the words electronic nose, e-nose, wound infection, wound, and chronic wound were searched for in the search engines and databases. It turned out that the perspective could be divided into three areas. Based on this knowledge, the search was thus split up and we searched for the areas algorithms, sensor technologies and samples. To do this, the words electronic nose, e-nose, algorithms, feature extraction, and machine learning were combined to bring out the current status of data processing. The search for sensor technologies was done by combining electronic nose, e-nose, sensor technologies, and sensor types. Then, the sample was regarded in more detail by searching for electronic nose, e-nose, bacteria, wound, volatiles, and infection. Mostly new publications were selected (newer than 2014) so that the current state-of-research is considered. However, if publications were older but still represented important foundations or findings, they have also been included. In general, publications were pre-selected by the title or abstract, followed by a verification, if the detection and classification of wound infections or bacteria with an e-nose (independent of the sensor type or bacteria species) was focused. The articles describing specific projects were included, if an e-nose was used to classify bacteria or wound infections, or if a review provided an overview of volatiles from bacteria and wounds. If a publication was mainly focused on improving the results of a previous project of the same group (e.g., by changing the algorithm), the recent publication was included. Additional publications were then found by using specific search terms and analyzing the literature listed in the references. It is possible that not all previously published papers were considered. Nevertheless, from our perspective, a comprehensive picture has emerged through this search. The aim of the literature review was therefore to get an overview of the current status of the development of a system for the early detection of wound infections. Furthermore, publications are to be presented that allow an entry point into this topic. Further, we aimed to learn about critical issues related to the work with e-noses. To achieve this, we paid special attention to the restrictions of the respective projects. Additionally, example projects were analyzed regarding the performance they reached and simplicity of the sampling methods.
There are three main sources for the production of VOCs associated with a wound: 1) infections, 2) dead tissue and 3) exudate (Samala and Davis, 2015). Thus, VOCs additionally reflect the host response to infections (Saviauk et al., 2018). Volatiles emitted from the skin are, however, diverse and affected by external factors, such as diet or use of fragranced products (e.g., sanitary and cosmetic products) (Ashrafi et al., 2017). Additionally, risk reducing measures like peri- and post-operative antimicrobial administration and surgical skin preparation alter the wound microbiome and thus the VOC profile (Ashrafi et al., 2017). Depending on the part of the body, as the location of infected tissues, the body can cause changes in bacterial metabolism and further, different species are found on the skin (Maddocks, 2017; Ratiu et al., 2020). It has further been shown that samples from different areas and sites of lesions present different VOC metabolites (Daneshkhah et al., 2020). There are differences between volatiles at the wound periphery and volatiles found in the center of the wound (colonized-but-healing and clinically infected areas) (Reeves et al., 2022). Also compressed and non-compressed skin have been shown to emit different volatility profiles (Daulton et al., 2020). Further, acetone and isoprene—VOCs known to be produced by bacteria but also dependend on the fluctuation of plasma glucose levels—are not applicable as wound infection biomarkers in patients with diabetes (Daneshkhah et al., 2020). Furthermore, necrotic tissue itself was shown to be another source of sore-related volatiles (Samala and Davis, 2015).
These facts in combination highlight that real wound conditions should be considered to assess the applicability of e-noses as wound infection diagnosis support. Specific volatiles produced by characteristic bacteria need to be identified to allow for monitoring the wound healing process and the step from colonization to an infection. Thus, to get sound results with e-nose systems, knowledge about characteristics of the (biological) sample, its preparation and presentation to a measurement system is essential.
Three bacteria species commonly present in infected wounds are E. coli, P. aeruginosa and S. aureus (Tian et al., 2009; Bessa et al., 2015; Maddocks, 2017). For example, Bessa et al. (Bessa et al., 2015) quantified wounds infected by S. aureus with 37%, by P. aeruginosa with 17% and by E. coli with 6%. Other mentioned bacteria species are S. epidermis, S. pyogenes, E. faecalis and A. baumannii (Tian et al., 2009; Ashrafi et al., 2017). Those and other bacteria species are associated with a variety of VOCs (Daulton et al., 2020), rather than with specific ones. Typically related VOCs, comprising shared and unique volatile metabolites of aforementioned common wound bacteria, can be found in (Ashrafi et al., 2017; Palma et al., 2018). Knowledge about the volatile smellprint of pathogen bacteria (Palma et al., 2018; Daulton et al., 2020) could thus be useful for their identification.
It was shown in (Palma et al., 2018) that it is possible to achieve an accuracy of 77% in identifying eleven different bacterial species using 18 VOCs. At the same time, for each bacterium there exists a set of VOCs suitable to predict the presence of the bacterium with a high accuracy of more than 90% (Palma et al., 2018).
Once multiple bacterial species are present, identifying microorganisms based on the corresponding VOCs becomes complex. However, it is nowadays known that especially chronic wounds harbor diverse bacterial populations (Dowd et al., 2008). Related to this fact, Bessa et al. found infections caused by two or more species, so called poly-microbial infections, in about 27% of the studied wounds (Bessa et al., 2015). Nevertheless, a decrease in microbial diversity has been associated with a worsening prognosis (Maddocks, 2017). The most common pathogen combination identified in such poly-microbial infections was based on S. aureus and P. aeruginosa—which together can cause biofilms (Serra et al., 2015). Those complex colonies of bacterial populations embedded in a protective extracellular polymeric substance (Gajula et al., 2020) can in turn lead to delayed wound healing. Further, presence of biofilms commonly results in failure of conventional pathogen identification methods (Ashrafi et al., 2017; Gajula et al., 2020; Xu et al., 2020), also for the most commonly applied method, the swab culture. As it has been reported that such biofilms are present in up to 80% of all chronic wounds, and in about 65% of all microbial infections (Perry et al., 2010; Ashrafi et al., 2017; He et al., 2017; Jamal et al., 2018; Mendoza et al., 2019), their impact on a correct pathogen diagnosis is high. It is thus promising that biofilms could be distinguished by e-noses (Thaler et al., 2008). However, in vivo biofilms show significant differences from biofilm formations in in vitro pure-culture systems (Xu et al., 2020). While the presence of different species and biofilms alter the VOC-fingerprints, volatile patterns of different strains of a single species are comparable (Kunze et al., 2013). This allows to transferring identified VOC-fingerprints to learn for other strains of the species (Kunze et al., 2013). It was further found that also the composition of the produced volatiles stays constant during the exponential growth of the microorganisms although the emission of volatiles increases with the number of colony-forming units (CFU) (Reidt et al., 2020). It should critically be noted here that not all studies distinguished precisely between colonization and wound infection.
The mere presence of microorganisms is not mandatorily accompanied with an infection as also non-infected wounds contain microorganisms. But although some bacteria are known to increase the risk of getting an infection and some species can induce diseases at lower concentrations than other types (International Wound Infection Institute, 2022) concrete biomarkers for early wound infection detection are hardly available. Therefore, the diagnoses of healthcare professionals are usually based on the clinical assessment and on the basis on their experience (Thet et al., 2020).
To implement such experience in approaches combining instrumental data and supervised learning methods samples requires proper labelling (Karakaya et al., 2020). Then it can be decided, whether the results actually influence the treatment or the clinical setting. For example, patients with multidrug-resistant pathogens, such as methicillin-resistant S. aureus (MRSA) must be treated in isolation, even if no relevant infection is present. In particular, it has to be defined, whether an infection should be detected in general or if the bacteria species should be identified. For both cases, the given label depends on the results of the reference method, which can be of microbiological nature or a diagnosis of a clinician. But particularly results obtained from swab cultures were in this regard found to be misleading (Daulton et al., 2020). This can be due to the fact that those culture-based methods are susceptible to overestimating skin commensals, which could cause incorrect causative pathogen identification (Ashrafi et al., 2017). Incorrect labelling can also be due to biofilms. Those cannot be detected without errors by culturing methods.
For this case, other reference methods as those mentioned before might be beneficial to get the best estimation of the infection status. In literature, bright-field microscopy and scanning electron microscopy have for instance been used to detect biofilms on wounds (Li et al., 2021). Besides, a wound infection detection system combining Field Asymmetric Ion Mobility Spectrometry with a particular algorithm outperformed conventional methods by detecting E. coli wound infections with a recognition rate of >96% (Sun et al., 2019).
Researchers typically analyze volatiles produced by bacteria grown in culture media (in vitro). This procedure allows targeting isolated bacterial VOCs, but neglects volatiles produced by e.g., changes in tissue. Further, in vitro VOCs will differ from those of bacteria in vivo due to different environmental factors and related to a more complex and varied growing regime (Daneshkhah et al., 2020; Ratiu et al., 2020). However, the choice of the growing regime in vitro also has an influence on the bacteria to be detected. For example, anaerobic bacteria are usually not detected in the cultures (Kramer et al., 2018).
If still a prediction model is trained with in vitro data, its performance drops when used for in vivo analysis. This could be due to the use of unsuitable sample carriers (Liang et al., 2018). So media or broths are no ideal models for a wound in clinical practice (Ashrafi et al., 2017), as standard growth media are highly dissimilar to the human body environment (Ratiu et al., 2020). Applying samples from real wounds should thus be more appropriate. Such an approach was presented by (Thuleau et al., 2018), who investigated biological wound samples and corresponding wound dressings obtained from patients with breast cancer. Headspace solid-phase micro extraction was used to collect VOCs from the headspace of the dressings (Thuleau et al., 2018), to provide sample carrier identical to prevailing conditions in clinical practice. In this study, five volatile compounds were identified in both, bacterial cultures and the headspace of 40% of the wound dressings (Thuleau et al., 2018). The VOCs were analyzed using gas chromatography separation coupled with a mass spectrometer. It can be noted that few publications currently study real infected human wound as a sample. One example of a pioneering study that analyzed data from real wounds in 80 patients to identify actual wound infections (Haalboom et al., 2019). Two swabs were thereby taken from one wound, whereof one was evaluated by conventional microbiology culture essay and one swab was evaluated by an e-nose analysis (Haalboom et al., 2019). The three sample preparation methods mentioned above are shown in Figure 1. Furthermore, Table 1 lists which microorganisms and sample carriers were included in which projects considered in this review article.
FIGURE 1. By different ways of sampling (A–C), the volatile substances of the bacteria species within the wound can be picked up with the electronic nose. The wound can be measured directly (A), a swab can be taken from the wound (B) or the bacteria from the wound can be cultured (C).
TABLE 1. Summary of published projects dealing with electronic noses in the field of wound infection detection and bacteria classification.
Many studies have been conducted to explore the basics and study bacteria in cultures with e-noses. Some projects have already investigated wounds in animals, which reflect the complexity of real wounds better than in vitro models. Research on these models allowed identifying solutions for the challenges described in this review. The next steps should be to transfer the gained knowledge into practice and study real infected wounds. In our opinion, the complexity of real wounds will not be taken into account, if this step is missed. Otherwise, there is a risk that the potential will not be evaluated correctly. We further believe that establishing standardized sampling methods, which are applicable in practice, is of major importance.
Lastly, the infection status of a wound is by no means unambiguous and the labels may therefore differ depending on the reference method. This should be considered and the performance of the algorithms should be related to the specific reference method.
Choosing an appropriate e-nose sensor technology and operating conditions have a major impact on a proper assessment of the smellprint of microorganisms (Reidt et al., 2020). In the following, those technologies are introduced that have already been applied for this task or might be promising candidates.
The majority of reviewed literature dealing with e-noses and wound infection detection applies MOS sensors (Sun et al., 2017). These sensors are inexpensive, show a long shelf life, response fast, and provide a broad detection range (5–500 ppm) (Wilson, 2016). For the detection of bacteria, even a commercially available e-nose from ‘AlphaMOS’ has been applied (Daneshkhah et al., 2020). However, MOS sensors are known to have weak precision, are susceptible to poisoning and sensitive to humidity (Karakaya et al., 2020). While the influence of humidity on the sensor performance can be decreased by integration of an independent humidity sensor (Reidt et al., 2020), well-known drift issues and lacking long-term stability still decrease sensor´s ability to provide stable signals over a sufficiently long period (Chai et al., 2022). Such a long-term stability is, however, crucial for long-term data collection to monitor wounds. In this regard it is beneficial that the application of different algorithms can reduce the drift by up to 64% (Romain and Nicolas, 2010; Abidin et al., 2018; Chai et al., 2022), resulting in increased sensor response stability.
Due to their fast response and inexpensiveness, also conducting polymer sensors have already widely been used in different applications (Nikolic et al., 2020; Wang et al., 2020). With a detection range of 0.1–100 ppm, these sensors can detect lower concentrations than MOS sensors. However, these sensors are also sensitive to humidity and temperature, have a limited sensor life and are affected from drift (Karakaya et al., 2020). Presumably, as for the MOS sensors, it could be possible to compensate for sensitivity to moisture with an independent sensor. Sensors based on polymer nanocomposites combine polymers with inorganic nanomaterials, graphene, or carbon nanotubes (John, 2020). The ‘Cyranose’, a handheld e-nose, is for example, based such nanocomposite sensors and was applied to identify bacteria species from diabetic wound infections in blood agar medium (Yusuf et al., 2015). The same working group compared the performance of ‘Cyranose’ with that of an MOS based e-nose called ‘PEN3’. Both devices were able to differentiate the evaluated bacteria species. Compared to MOS sensors, conducting polymer sensors show a resistance to poisoning. This resistance is beneficial, as a poisoned sensor results from an irreversible reaction and leads to an unstable sensor signal (Chai et al., 2022). Especially in clinical practice, however, unnoticed damaged sensors have consequential impacts.
Acoustic wave sensors, especially bulk acoustic wave and surface acoustic wave sensors, are advantageous due to their small size, low cost, robustness, good sensitivity, and fast response time. Their disadvantages cover that they are difficult to implement, have a poor signal-to-noise ratio, are sensitive to temperature (Karakaya et al., 2020) and are to date highly experimental (Mandal and Banerjee, 2022). Whether this technology is advantageous over MOS sensors for detecting wound bacteria has not yet been focused on, but might be a promising approach. Particularly as a portable device, these sensors were able to detect and discriminate between benzene, toluene, and xylene even at concentrations of 10 ppm (Matatagui et al., 2019).
Optoelectronic noses comprise instruments that detect changes in color (colorimetric sensors) of the sensor array and those that detect changes in light emissions released by the sample (fluorescence sensors). The underlying technology of optoelectronic noses overcomes several disadvantages of other sensor technologies, such as poor chemical specificity, drift, or sensitivity to humidity. They are further characterized by low cost, a light weight and high compactness, as well as by immunity to electromagnetic interferences, rapid sensor responses, and a very high sensitivity down to ppb with exquisite fingerprints of extremely similar mixtures (Li and Suslick, 2016; 2021; Karakaya et al., 2020). It is even possible to produce the sensor array by inkjet onto porous polymer membranes or paper (Li and Suslick, 2021). However, disadvantages cited are that they are harder to implement, less portable in some cases, and affected by light interference.
Literature provides information that pathogen detection was performed with colorimetric assays (Bordbar et al., 2020). In the exemplarily chosen study researchers detected bacterial volatiles in drinking water and human urine. Unique colorimetric patterns were achieved for each bacteria strain and it was further possible to accurately differentiate between infected and non-infected urine sample of patients. With a colorimetric sensor array it was also possible to identify bacterial cultures on blood agar plates within 3 h of detection, resulting in a sensitivity of 91% and a specificity of 99.4% (Lim et al., 2016). The detection time decreased as the number of CFUs increased. Another group (Li and Suslick, 2016) built a portable optoelectronic nose based on a colorimetric sensor array to monitor the freshness of meat. Such a sensor array would be beneficial for wound infection detection, because 1) the sensing device was assembled inside a hand-held analyzer which would allow for measurements close to the patient, 2) the limits of detection were low, 3) the device showed ‘excellent’ reproducibility over several printings of the arrays, the differences in the printings were lower than the differences among different meat samples. Such a system could provide improved long-term data stability as the sensor array as such is only used once. Further, no replacement of individual defective sensors is required as the whole sensor array is disposable.
Overall, optoelectronic noses may represent another suitable technology for detecting bacteria in wounds and classifying wound infections, even though, to our knowledge, this has not been directly addressed to date.
Electronic noses based on quartz crystal microbalance (QCM) sensors are sensitive towards mass changes down to 1 ng while responding fast. However, they are sensitive towards changes in temperature and humidity and suffer from a poor signal-to-noise ratio. Besides, these sensors are hard to implement. But still, they are stable, and it is possible to build portable devices (Karakaya et al., 2020). Particularly the high sensitivity and low detection limits would be beneficial for wound infection detection, as this allows for detecting less colony forming units and therefore a detection at early stage. Effects caused by changes in humidity are unfavorable, especially in clinical practice, but could possibly be compensated here as well. So one working group used a reference element to compensate for humidity drift in their QCM sensors for ammonia (Ushimi et al., 2015). Further, mathematical models in the data processing system were able to compensate humidity effects (Ushimi et al., 2015) allowing to predict a toluene gas concentration independently from humidity (Mumyakmaz et al., 2010). Additionally, a low-cost and portable e-nose based on QCM sensors was able to discriminate accurately seven volatile analytes (Julian et al., 2020). These included butanol, propanol, and methanol, which also play a role in differentiating bacteria by their metabolic volatiles (Bos et al., 2013; Julian et al., 2020). Further, due to the fast response of QCM-based sensors, 120 s for the measurement of the sample air was enough.
Another promising way to obtain best possible results is the use of hybrid sensor arrays, including different technologies as presented by (Broza et al., 2018). Interestingly, these hybrid arrays explicitly include the measurement of interfering VOCs to allow for clearer observation. Such combinations can lead to a power of data analysis. It can, however, be crucial to choose a proper subset of the hybrid array so that the test-set error can be further reduced. For example, the entire hybrid array may not perform better than an array with only one technology, but a subset of the sensors from the hybrid array does (Broza et al., 2018). Such approaches are to date only scarcely used for VOC detection. As one example, the work of (Seesaard et al., 2020) can be cited, who used a hybrid e-nose system to differentiate bacteria species. The sensor array consisted of three organic-inorganic nanocomposite gas sensors and three MOS gas sensors to analyze five different infection-causing bacteria species (E. coli, E. cloacae, P. aeruginosa, S. typhi and S. aureus). Using principal component analysis (PCA) and hierarchical cluster analysis, it was possible to differentiate the bacteria species.
MOS sensors have most commonly been applied in the reviewed studies. This might be due to their biggest advantages, as they are not expensive and can be assembled into a lab-made e-nose. For example, in (Jia et al., 2016; Liang et al., 2019; Astantri et al., 2020) an e-nose was built in the laboratory, whereas in (Haalboom et al., 2019) a commercially available e-nose was used. However, also other technologies provided promiseable results (Table 1). In summary, we identified several sensor technologies being capable of detecting bacteria based on their volatile components. As each of these sensor technologies has their advantages but also disadvantages for wound infection detection, we do not recommend one best technology for this use case. We rather suggest to make optimal use of the existing sensor technologies by adapting them to the respective project, taking the sample properties into account and improving the evaluation algorithms.
As previously mentioned, gas sensors can suffer from drift issues, or be sensitive towards environmental changes in temperature, humidity and background odors. This is why a sound usage of e-noses requires a considerate handling of the data—which in turn improves the sensing result.
The initial steps aim at reducing noise, normalizing data or manipulating the baseline (Bonah et al., 2020). Subsequent, the definition and extraction of suitable features is important to obtain robust information with less redundancy prior applying machine learning methods (Yan et al., 2016). Such pre-processed data is typically beneficial to train supervised machine learning models for identifying patterns (classifier). According supervised learning requires labeling (see section 3.1.2) of the training data. Inputs, such as sensing features, are mapped to known outputs (labels), for example, ‘infected’ or ‘non-infected’. Thereby these methods can be used for classification (Scott et al., 2019; Karakaya et al., 2020). However, the learning models achieve different results depending on the quality of the pre-processing (Yusuf et al., 2014). All previously mentioned steps are of fundamental importance for the proper application of e-noses. The general problem is not to achieve sufficient accuracy for a given dataset, but more to obtain stable results on new data over time with varying conditions. For these reasons, efforts are made to develop appropriate methods for suppressing interferences and minimizing the impact of changes in external factors.
Wound infection samples are neither easy nor fast to obtain, which leads to limited but often costly data sets. However, to train the classifier many samples are needed (He et al., 2017). One solution could be applying transfer learning, whereof models are generally characterized by the ability to transfer knowledge from one field to another. For improving the accuracy to classify wounds, they allow for implementing cheaper but unlabeled pollutant gas samples from other fields (He et al., 2017). If size and dimension of the dataset of unlabeled data combined with a suitable classifier is chosen correctly, a significant improvement can be achieved. For example, the accuracy obtained with the radial basis function classifier was further improved by 10% with transfer learning (He et al., 2017).
In clinical setting, minimizing exogenous volatiles is often challenging (Daulton et al., 2020). Interferences can, however, impact the detection of microbial VOCs by e-noses possibly leading to a deteriorated classification performance (Liang et al., 2017; Liang et al., 2018). This is why the prediction accuracy typically drops when models trained on laboratory data are directly used in clinical setting (Liang et al., 2019).
In general, there are two types of interferences a) non-target odor interference caused by interfering odors that influence the detection of the target odor and b) the interference from environmental factors, such as temperature and humidity (Liang et al., 2017). The latter can be compensated by using the responses of corresponding sensors as input to the classifier (Liang et al., 2017). The non-target odor interference appears as background interference or as suddenly appearing interference (Liang et al., 2017). To address the problems caused by background interferences, information in the sampling stage correlated with baseline samples were for example, removed by the suppression technique ‘CIRIS’ (Liang et al., 2017). Thereby it was possible to avoid deterioration in pathogen classification performance—even in the presence of ethanol in the air, which appears likely in clinical practice due to disinfection (Liang et al., 2017). A detailed overview of commonly used suppression methods for different types of interferences is given in (Liang et al., 2018), where it is also stated that further research is still needed.
Further, background interference can be due to the sample carrier (see section 3.1.3). Though, by applying a transfer learning technique, it was possible to compensate the noise odors of the carrier material and thus increasing the prediction accuracy from 29.18% to 82.55%. This allowed the use of only one predictive model for both sample carriers: animal wound and culture medium (Liang et al., 2019). Authors further mentioned that the method might be suitable for other common problems, such as transfer among multiple systems or sensor drift issues.
Several reasons can cause unintended sensor drift (e.g., aging, poisoning, or environmental changes) (Karakaya et al., 2020; Liu et al., 2020). Especially for MOS sensors, sensor drift causes changing patterns for the same VOC profiles (Karakaya et al., 2020; Liu et al., 2020). In addition to appropriate sensor material selection, algorithms can help to suppress drift and achieve more stable results over time. An often-used method for improving sensor stability is baseline manipulation (Karakaya et al., 2020). To overcome the disadvantages of baseline correction or component correction, however, transfer learning-based methods are applied (Liang et al., 2018; Liu et al., 2020). They further require less labeled data in the target domain, which is especially important for samples from wounds. To a certain extent, it is also possible to compensate for drift by means of suitable features (Tian et al., 2009). Moreover, a recently published drift compensation for an e-nose does not require calibration samples and increases reliability of the e-nose over time by compensating drift (Maho et al., 2022).
A complete e-nose sensor array may pick noisy information caused by environmental factors. Further, collected sensor data can be redundant, incomplete, and inconsistent (Qian et al., 2022). Selecting a subset of sensors can result in higher performance, (Yan et al., 2016; Sun et al., 2017). In the project of (Sun et al., 2017), the original array consisted of 34 sensors. Culture dishes containing bacteria were measured using an e-nose and then classified using support vector machine (SVM). Without any optimization, the recognition rate was 86.54%. Using linear discriminant analysis (LDA), the rate was increased to 96.15% while the number of sensors was reduced to 20. Even with 10 sensors, selected by LDA or Wilks’ Λ-statistic, the detection rate was still 95.19% (Sun et al., 2017). Another working group utilized the Hilbert-Schmidt Independence Criterion to optimize the sensor array (Qian et al., 2022). The test accuracy without optimization was 80.83% while using all 34 sensors. After optimization, an accuracy of 92% was obtained, with the number of sensors reduced to 18. One working group (Wijaya et al., 2016) optimized the sensor array using filter-based selection (using FCBF) to overcome the problem of redundancy and find the best-suited features. The related e-nose initially consisted of 11 MOS gas sensors. In the end, the number of sensors was reduced to seven and the general resolution factor was increased by 16%, also indicating a possible higher accuracy. As the classifier parameters also have a great influence, Yan et al. (Yan et al., 2016) used synchronous optimization (based on QPSO) to optimize the parameters of the classifier (RBF neural network). As a result, the number of sensors was reduced to six (there were 20 at the beginning), while the performance was increased. In sum, the best combination of feature extraction and pattern recognition algorithm with optimized parameters should be found, and not only excellent algorithms explored—which has already been stated by (Luo et al., 2018). Table 1 summarizes the projects that address the topic of wound infection detection or bacterial classification using e-noses. The technologies used, the sample carrier and the algorithms are listed.
This overview illustrates that numerous algorithms have been developed to solve challenges and classification tasks in order to achieve the best possible performance. It can been seen that different algorithms lead to similarly good results.
Numerous algorithms are already available to compensate for the disadvantages of certain sensor technologies and improve the performance over time. However, the final performance also depends on the combination of algorithms, extracted features and technology. For a sustainable implementation, we see an urgend need to apply the algorithms particularly outside the laboratory. Only thereby, the suitability of the algorithms can properly be evaluated. Accordingly, clinical studies are required to investigate the algorithms performance over time under real conditions. This should also address the question of how to monitor the performance of these algorithms in real applications over time.
Such clinical studies should take into account that the sample (3.1), the sensor technology (3.2) and the algorithms (3.3) should not be considered separately (Figure 2), particularly as they influence each other. Further, to prove the reliability of the obtained information, clinical results are required to merge them with those obtained by of e-noses.
FIGURE 2. The three sub-areas of the application of electronic noses related to the support of the diagnosis of wound infections. These areas influence each other and should be seen as a whole. Here, the different bubble sizes reflect the frequency of occurrence in the publications considered.
A structured approach to the diagnosis of patients with wounds is always advisable. The ABCDE rule represents a supportive method for this (Dissemond, 2017). Under “B”, the further examinations with regard to bacteria are designated. The current gold standard for wound diagnostics in this regard is the microbiological swab or tissue examination of a biopsy. These methods have advantages and disadvantages. However, a major disadvantage are always the longer time periods until a result is available. Exactly here lies a substantial advantage of the bacteria identification by means of e-nose. A diagnosis could be made that provides directly relevant information about the bacterial spectrum. In order to be able to evaluate the clinical relevance of this information in a differentiated manner, a clinical assessment of the patient must always be carried out. The term infection continuum is often used for this purpose to specify the various progressive stages from harmless bacterial contamination or colonization to a potentially dangerous systemic infection. Here, several successive clinical states are described (Dissemond, 2023). Until now, there were no uniformly accepted criteria for diagnosis of wound infections. The newly developed and validated TILI score, as a supplement to vital signs and serological values, now enables rapid objectification of local wound infections (Dissemond et al., 2020). The W.A.R. score can be used in addition to identify patients at increased risk of infection (Dissemond et al., 2011). With these easy-to-use tools, the indication for antiseptic wound therapy can be assessed individually, quickly, and without problems.
In general, the potential for harmful effects of microorganisms in a wound is influenced by several risk factors. The patient’s immune system and the type and number of bacteria determine the risk of overcoming the host resistance (International Wound Infection Institute, 2022). Common risk factors are overweight, smoking or poorly controlled diabetes (International Wound Infection Institute, 2022). Further, diabetic wounds, poor perfusion, fluctuations in plasma glucose levels, changes in metabolic pathways, or anti-inflammatory agents affect wound healing and thus the odor emitted from the wound (Ousey et al., 2017; Daneshkhah et al., 2020). Changes in pH have also been associated with wound infection status (section 3.1.1), so this information could also be fused. As discussed earlier, there is merit in making more robust and reliable diagnostic decisions by fusing different technologies (Wilson, 2016). Overall, fusing information from different scores and rules with information obtained by e-noses could significantly improve wound diagnostics (Figure 3). This can reduce the risk of the infection developing further into a systemic infection or even sepsis.
FIGURE 3. Different information from different sources should be fused with electronic nose measurements to improve the diagnosis of wound infections and compensate for the uncertainties of the measurements.
E-noses, as machine learning (ML)-based methods, face common Artificial-Intelligence-related challenges, especially if they are to be applied in healthcare projects. In general applying promising ML tools to realistic clinical practice settings is difficult, as it is much more complex to extend a model to this environment than to develop it only in a reductionist research context (Mateen et al., 2020). In fact, research projects tend to stop after achieving accurate results in the lab—and the difficult transfer into clinical practice, often associated with failure, is left to other interested experts (Mateen et al., 2020). This rather typical gap between development and eventual use is referred to as ‘artificial intelligence chasm’ in literature (Antoniou and Mamdani, 2021).
To close this gap, clinicians should be involved in the development process (Scott et al., 2019). Proper reporting is thereby essential to evaluate the weaknesses and strengths of the system in terms of its clinical suitability (Mateen et al., 2020). However, a clear pathway for translation of ML tools into clinical practice to assess the point at which the tool is ready and safe for use in real health contexts is lacking (Mateen et al., 2020). As described in literature, checklists and guidelines are a way to safely integrate ML-based tools into clinical practice and can provide a roadmap of expectations (Mateen et al., 2020). Further, assessment standards for safety and utility are needed, and replication studies must be conducted, as it is the case with general ML applications (Scott et al., 2019). Especially for wound infection detection, the replication studies are needed to test reproducibility and validity. This is a promising way for using e-noses safely in clinical practice for the detection of wound infections.
However, no clear definition on the part of regulatory bodies for classifying is given, whether a technology is based on artificial intelligence/ML or not (Benjamens et al., 2020). From authors point of view, a wound infection detection device is not a mere sensor array but a medical device based on machine learning as the performance largely depends on the features previously extracted, the algorithms chosen, and the data on which the model was trained. Such ML-based medical devices lack, however, transparency—but explainable and interpretable ML models are crucial to establish trust on the part of clinicians (Tonekaboni et al., 2019). In a survey it was noted that clinical staff did not mainly rank ML models by their accuracy to be used routinely. Conversely, models with lower accuracy would be accepted, if the reason for underperformance is known (Tonekaboni et al., 2019). So in the context of clinical diagnoses, it is important for clinicians to get a sense of when technology works and when it does not (Tonekaboni et al., 2019).
ML models require periodic reassessment. Over time, several influences may cause the model to no longer provide accurate results. In addition, the ongoing evaluation of the system is important as processes and clinical practice evolve over time and data set shifts may result in data sets that differ from those initially used, resulting in deviation of the performance (Antoniou and Mamdani, 2021). There is a need to discuss how to deal with sensor drift over the entire lifecycle.
Regulatory requirements pose important challenges to companies developing medical algorithms (Benjamens et al., 2020). As medical devices are associated with a high level of risk, strict requirements apply to the approval of those technologies by the regulatory process of the U.S. Food and Drug Administration (FDA) (Benjamens et al., 2020). The FDA plays a leading role in the adoption of ML-based medical technologies, having already approved several ML-based medical technologies. Nevertheless, the European Medicines Agency has also provided statements and guidelines about artificial intelligence (Benjamens et al., 2020). Further, it becomes challenging to evaluate compensation algorithms in their performance to be able to ensure safety. Overall, changes due to drift or sensor poisoning in addition to changes of the ML model may be challenging to consider, as they influence each other. However, in particular these questions cannot be answered yet, if only the laboratory context is considered. For this reason, practice-oriented studies are needed.
To assess the performance of e-nose set-ups under real conditions, clinical studies are needed. This includes evaluating several combinations of features, algorithms and sensor technologies, linking the individual knowledge. This would allow for reversely engineer the results to the sub-areas and answer the initial questions, such as whether the sensor technology is still suitable in use or whether the algorithms need to be adapted, again.
To further develop and validate the technology, studies could be conducted using real wound swabs or the headspace of human wounds as a sample. These data would be applicable to test the algorithms and formulate further challenges. Overall, the previously mentioned explainability is another essential aspect. Attempts should be made to work towards the use case and thus enhance both sustainability and acceptance. In this context, we primarily view sustainability as ensuring and monitoring algorithms for sustained performance and meeting initial requirements over time. The algorithms should sustainably meet the requirements specified at the beginning of the development.
Research is being done using e-noses for wound infection detection to get fast results close to the patient. Consequently, the performance of e-nose systems in the field of wound infection detection improves and bacteria can be identified by their VOCs profile.
Numerous influencing factors occurring in complex clinic settings can be minimized in their effects by various algorithms. Therefore, it seems that the detection of wound infections is possible and feasible. However, the deployment of the technology is associated with various hurdles, which is why good results in the laboratory are not yet a guarantee for use. Therefore, close contact should be maintained with clinicians during the development phase to successfully manage the difficult step of transfer and to develop a trustworthy device that fits into existing workflows. Attention should be paid to the biological sample and its preparation, the characteristics of the sensor technology and measurement device, and the choice of the algorithms. All these points must be coordinated to obtain the best possible result. However, it is important that wounds and wound infections are complex, and the mere presence of bacteria cannot be considered a sufficient criterion for the presence of an infection. For this reason, further work should be done towards information fusion to answer the question of whether the results can be improved by adding additional sources of information, thereby enabling results that are more robust. In summary, 1) the wound including the crucial VOCs for the diagnosis at different stages, 2) the standardization of sampling techniques, and 3) the improvement of the performance robustness under different influences in the clinical practice should be further investigated. This requires studies that address the practical use and include conditions found outside the laboratory.
Conceptualization JW and MP-H; funding acquisition MP-H; investigation JW; supervision MP-H; visualization JW; writing—original draft preparation JW, MP-H, and JD; writing—review and editing JW, MP-H, JD, and MM; All authors contributed to the article and approved the submitted version.
The authors acknowledge financial support by the project “SAIL: SustAInable Life-cycle of Intelligent Socio-Technical Systems” (Grant ID NW21-059C), which is funded by the program “Netzwerke 2021” of the Ministry of Culture and Science of the State of North Rhine Westphalia, Germany.
Authors would like to thank Selina Ramm (TH OWL) for valuable discussions and her critical view during the draft preparation.
The authors declare that the research was conducted in the absence of any commercial or financial relationships that could be construed as a potential conflict of interest.
All claims expressed in this article are solely those of the authors and do not necessarily represent those of their affiliated organizations, or those of the publisher, the editors and the reviewers. Any product that may be evaluated in this article, or claim that may be made by its manufacturer, is not guaranteed or endorsed by the publisher.
Abidin, M. Z., Asmat, A., and Hamidon, M. N. (2018). Comparative study of drift compensation methods for environmental gas sensors. IOP Conf. Ser. Earth Environ. Sci. 117, 012031. doi:10.1088/1755-1315/117/1/012031
Antoniou, T., and Mamdani, M. (2021). Evaluation of machine learning solutions in medicine. CMAJ 193, E1425–E1429. doi:10.1503/cmaj.210036
Ashrafi, M., Bates, M., Baguneid, M., Alonso-Rasgado, T., Rautemaa-Richardson, R., and Bayat, A. (2017). Volatile organic compound detection as a potential means of diagnosing cutaneous wound infections. Wound Repair Regen. 25, 574–590. doi:10.1111/wrr.12563
Astantri, P. F., Prakoso, W. S. A., Triyana, K., Untari, T., Airin, C. M., and Astuti, P. (2020). Lab-made electronic nose for fast detection of Listeria monocytogenes and Bacillus cereus. Vet. Sci. 7, 20. doi:10.3390/vetsci7010020
Benjamens, S., Dhunnoo, P., and Meskó, B. (2020). The state of artificial intelligence-based FDA-approved medical devices and algorithms: an online database. NPJ Digit. Med. 3, 118. doi:10.1038/s41746-020-00324-0
Bessa, L. J., Fazii, P., Di Giulio, M., and Cellini, L. (2015). Bacterial isolates from infected wounds and their antibiotic susceptibility pattern: some remarks about wound infection. Int. Wound J. 12, 47–52. doi:10.1111/iwj.12049
Bonah, E., Huang, X., Aheto, J. H., and Osae, R. (2020). Application of electronic nose as a non-invasive technique for odor fingerprinting and detection of bacterial foodborne pathogens: a review. J. Food Sci. Technol. 57, 1977–1990. doi:10.1007/s13197-019-04143-4
Bordbar, M. M., Tashkhourian, J., Tavassoli, A., Bahramali, E., and Hemmateenejad, B. (2020). Ultrafast detection of infectious bacteria using optoelectronic nose based on metallic nanoparticles. Sens. Actuators B Chem. 319, 128262. doi:10.1016/j.snb.2020.128262
Bos, L. D. J., Sterk, P. J., and Schultz, M. J. (2013). Volatile metabolites of pathogens: a systematic review. PLoS Pathog. 9, e1003311. doi:10.1371/journal.ppat.1003311
Broza, Y. Y., Vishinkin, R., Barash, O., Nakhleh, M. K., and Haick, H. (2018). Synergy between nanomaterials and volatile organic compounds for non-invasive medical evaluation. Chem. Soc. Rev. 47, 4781–4859. doi:10.1039/C8CS00317C
Cecconi, M., Evans, L., Levy, M., and Rhodes, A. (2018). Sepsis and septic shock. Lancet 392, 75–87. doi:10.1016/s0140-6736(18)30696-2
Chai, H., Zheng, Z., Liu, K., Xu, J., Wu, K., Luo, Y., et al. (2022). Stability of metal oxide semiconductor gas sensors: a review. IEEE Sens. J. 22, 5470–5481. doi:10.1109/JSEN.2022.3148264
Daneshkhah, A., Siegel, A. P., and Agarwal, M. (2020). Volatile organic compounds: potential biomarkers for improved diagnosis and monitoring of diabetic wounds. Wound Heal. tissue repair, Regen. diabetes 2020, 491–512. Elsevier. doi:10.1016/B978-0-12-816413-6.00023-X
Daulton, E., Wicaksono, A., Bechar, J., Covington, J. A., and Hardwicke, J. (2020). The detection of wound infection by ion mobility chemical analysis. Biosensors 10, 19. doi:10.3390/bios10030019
Dissemond, J. (2017). ABCDE rule in the diagnosis of chronic wounds. J. Dtsch. Dermatol Ges. 15, 732–733. doi:10.1111/ddg.13273
Dissemond, J., Assadian, O., Gerber, V., Kingsley, A., Kramer, A., Leaper, D. J., et al. (2011). Classification of wounds at risk and their antimicrobial treatment with polihexanide: a practice-oriented expert recommendation. Skin. Pharmacol. Physiol. 24, 245–255. doi:10.1159/000327210
Dissemond, J. (2023). Diagnostik und Therapie lokaler Wundinfektionen. Z Gerontol. Geriatr. 56, 48–52. doi:10.1007/s00391-021-01984-7
Dissemond, J., Strohal, R., Mastronicola, D., Senneville, E., Moisan, C., Edward-Jones, V., et al. (2020). Therapeutic index for local infections score validity: a retrospective European analysis. J. Wound Care 29, 726–734. doi:10.12968/jowc.2020.29.12.726
Dowd, S. E., Sun, Y., Secor, P. R., Rhoads, D. D., Wolcott, B. M., James, G. A., et al. (2008). Survey of bacterial diversity in chronic wounds using pyrosequencing, DGGE, and full ribosome shotgun sequencing. BMC Microbiol. 8, 43. doi:10.1186/1471-2180-8-43
Dragonieri, S., Pennazza, G., Carratu, P., and Resta, O. (2017). Electronic nose technology in respiratory diseases. Lung 195, 157–165. doi:10.1007/s00408-017-9987-3
Gajula, B., Munnamgi, S., and Basu, S. (2020). How bacterial biofilms affect chronic wound healing: a narrative review. Int. J. Surg. Glob. Health 3, e16. doi:10.1097/GH9.0000000000000016
Guo, X., Peng, C., Zhang, S., Yan, J., Duan, S., Wang, L., et al. (2015). A novel feature extraction approach using window function capturing and QPSO-SVM for enhancing electronic nose performance. Sensors 15, 15198–15217. doi:10.3390/s150715198
Haalboom, M., Gerritsen, J. W., and van der Palen, J. (2019). Differentiation between infected and non-infected wounds using an electronic nose. Clin. Microbiol. Infect. 25, 1288.e1–1288.e6. doi:10.1016/j.cmi.2019.03.018
He, P., Jia, P., Qiao, S., and Duan, S. (2017). Self-taught learning based on sparse autoencoder for e-nose in wound infection detection. Sensors 17, 2279. doi:10.3390/s17102279
Horch, R. E., Nord, D., Augustin, M., Germann, G., Leffler, M., and Dragu, A. (2008). Economic aspects of surgical wound therapies. Chirurg 79, 518–525. doi:10.1007/s00104-008-1500-3
International Wound Infection Institute (2022). Wound infection in clinical practice. Principles of best practice. Wounds Int, Available at: https://woundinfection-institute.com/wp-content/uploads/IWII-CD-2022-web-1.pdf (Assessed June 29, 2023).
Jamal, M., Ahmad, W., Andleeb, S., Jalil, F., Imran, M., Nawaz, M. A., et al. (2018). Bacterial biofilm and associated infections. J. Chin. Med. Assoc. 81, 7–11. doi:10.1016/j.jcma.2017.07.012
Jia, P., Huang, T., Wang, L., Duan, S., Yan, J., and Wang, L. (2016). A novel pre-processing technique for original feature matrix of electronic nose based on supervised locality preserving projections. Sensors 16, 1019. doi:10.3390/s16071019
John, B. (2020). “Polymer nanocomposite-based electrochemical sensors and biosensors,” in Nanorods and nanocomposites. Editors M. Sasani Ghamsari, and S. Dhara (London, England: IntechOpen).
Julian, T., Hidayat, S. N., Rianjanu, A., Dharmawan, A. B., Wasisto, H. S., and Triyana, K. (2020). Intelligent mobile electronic nose system comprising a hybrid polymer-functionalized quartz crystal microbalance sensor array. ACS Oomega 5, 29492–29503. doi:10.1021/acsomega.0c04433
Karakaya, D., Ulucan, O., and Turkan, M. (2020). Electronic nose and its applications: a survey. Int. J. Auto. Comp. 17, 179–209. doi:10.1007/s11633-019-1212-9
Kodogiannis, V. S. (2014). Point-of-care diagnosis of bacterial pathogens in vitro, utilising an electronic nose and wavelet neural networks. Neural Comput. Appl. 25, 353–366. doi:10.1007/s00521-013-1494-8
Kramer, A., Dissemond, J., Kim, S., Willy, C., Mayer, D., Papke, R., et al. (2018). Consensus on wound antisepsis: update 2018. Skin. Pharmacol. Physiol. 31, 28–58. doi:10.1159/000481545
Kunze, N., Göpel, J., Kuhns, M., Jünger, M., Quintel, M., and Perl, T. (2013). Detection and validation of volatile metabolic patterns over different strains of two human pathogenic bacteria during their growth in a complex medium using multi-capillary column-ion mobility spectrometry (MCC-IMS). Appl. Microbiol. Biotechnol. 97, 3665–3676. doi:10.1007/s00253-013-4762-8
Li, S., Renick, P., Senkowsky, J., Nair, A., and Tang, L. (2021). Diagnostics for wound infections. Adv. Wound Care 10, 317–327. doi:10.1089/wound.2019.1103
Li, Z., and Suslick, K. S. (2016). Portable optoelectronic nose for monitoring meat freshness. ACS Sensors 1, 1330–1335. doi:10.1021/acssensors.6b00492
Li, Z., and Suslick, K. S. (2021). The optoelectronic nose. Acc. Chem. Res. 54, 950–960. doi:10.1021/acs.accounts.0c00671
Liang, Z., Tian, F., Yang, S. X., Zhang, C., Sun, H., and Liu, T. (2018). Study on interference suppression algorithms for electronic noses: a review. Sensors 18, 1179. doi:10.3390/s18041179
Liang, Z., Tian, F., Zhang, C., Sun, H., Liu, X., and Yang, S. X. (2017). A correlated information removing based interference suppression technique in electronic nose for detection of bacteria. Anal. Chim. Acta 986, 145–152. doi:10.1016/j.aca.2017.07.028
Liang, Z., Tian, F., Zhang, C., and Yang, L. (2019). A novel subspace alignment-based interference suppression method for the transfer caused by different sample carriers in electronic nose. Sensors 19, 4846. doi:10.3390/s19224846
Lim, S. H., Mix, S., Anikst, V., Budvytiene, I., Eiden, M., Churi, Y., et al. (2016). Bacterial culture detection and identification in blood agar plates with an optoelectronic nose. Analyst 141, 918–925. doi:10.1039/c5an01990g
Liu, H., Li, Q., Li, Z., and Gu, Y. (2020). A suppression method of concentration background noise by transductive transfer learning for a metal oxide semiconductor-based electronic nose. Sensors 20, s20071913. doi:10.3390/s20071913
Luo, H., Jia, P., Qiao, S., and Duan, S. (2018). Enhancing electronic nose performance based on a novel QPSO-RBM technique. Sens. Actuators B Chem. 259, 241–249. doi:10.1016/j.snb.2017.12.026
Maddocks, S. E. (2017). Microbial communities within the chronic wound: the microbiom. Microbiology today magazine. Available at: https://microbiologysociety.org/publication/past-issues/the-microbiome/article/microbial-communities-within-the-chronic-wound.html (Assessed June 29, 2023).
Maho, P., Herrier, C., Livache, T., Comon, P., and Barthelmé, S. (2022). A calibrant-free drift compensation method for gas sensor arrays. Chemom. Intell. Lab. Sys 225, 104549. doi:10.1016/j.chemolab.2022.104549
Mandal, D., and Banerjee, S. (2022). Surface acoustic wave (SAW) sensors: physics, materials, and applications. Sensors 22, s22030820. doi:10.3390/s22030820
Matatagui, D., Bahos, F. A., Gràcia, I., and Del Horrillo, M. C. (2019). Portable low-cost electronic nose based on surface acoustic wave sensors for the detection of BTX vapors in air. Sensors 19, s19245406. doi:10.3390/s19245406
Mateen, B. A., Liley, J., Denniston, A. K., Holmes, C. C., and Vollmer, S. J. (2020). Improving the quality of machine learning in health applications and clinical research. Nat. Mach. Intell. 2, 554–556. doi:10.1038/s42256-020-00239-1
Mendoza, R. A., Hsieh, J.-C., and Galiano, R. D. (2019). “The impact of biofilm formation on wound healing,” in Wound healing - current perspectives. Editor K. Hakan Dogan (London, England: IntechOpen).
Mumyakmaz, B., Özmen, A., Ebeoğlu, M. A., Taşaltın, C., and Gürol, İ. (2010). A study on the development of a compensation method for humidity effect in QCM sensor responses. Sens. Actuators B Chem. 147, 277–282. doi:10.1016/j.snb.2010.03.019
Nikolic, M. V., Milovanovic, V., Vasiljevic, Z. Z., and Stamenkovic, Z. (2020). Semiconductor gas sensors: materials, technology, design, and application. Sensors 20, 6694. doi:10.3390/s20226694
Ousey, K., Roberts, D., and Gefen, A. (2017). Early identification of wound infection: understanding wound odour. J. Wound Care 26, 577–582. doi:10.12968/jowc.2017.26.10.577
Palma, S. I. C. J., Traguedo, A. P., Porteira, A. R., Frias, M. J., Gamboa, H., and Roque, A. C. A. (2018). Machine learning for the meta-analyses of microbial pathogens' volatile signatures. Sci. Rep. 8, 3360. doi:10.1038/s41598-018-21544-1
Perry, D. J., Fitzmaurice, D. A., Kitchen, S., Mackie, I. J., and Mallett, S. (2010). Point-of-care testing in haemostasis. Br. J. Haematol. 150, 501–514. doi:10.1111/j.1365-2141.2010.08223.x
Qian, J., Lu, M., Tian, F., and Liu, R. (2022). Study on sensor array optimization of medical electronic nose for wound infection detection. IEEE Trans. Circuits Syst. II Express Briefs 69, 1867–1871. doi:10.1109/TCSII.2021.3112597
Raeder, K., Strube-Lahmann, S., Müller-Werdan, U., Kottner, J., Lahmann, N. A., and Suhr, R. (2019). Prevalence and influencing factors of chronic wounds among clients of home care services in Germany. Z Evid. Fortbild. Qual. Gesundheitswes 140, 14–21. doi:10.1016/j.zefq.2019.01.001
Ratiu, I.-A., Bocos-Bintintan, V., Monedeiro, F., Milanowski, M., Ligor, T., and Buszewski, B. (2020). An optimistic vision of future: diagnosis of bacterial infections by sensing their associated volatile organic compounds. Crit. Rev. Anal. Chem. 50, 501–512. doi:10.1080/10408347.2019.1663147
Reeves, N., Phillips, S., Hughes, A., Maddocks, S., Bates, M., Torkington, J., et al. (2022). Volatile organic compounds in the early diagnosis of non-healing surgical wounds: a systematic review. World J. Surg. 46, 1669–1677. doi:10.1007/s00268-022-06548-3
Reidt, U., Helwig, A., Müller, G., Lenic, J., Grosser, J., Fetter, V., et al. (2020). Detection of microorganisms with an electronic nose for application under microgravity conditions. Gravit. Space Res. 8, 1–17. doi:10.2478/gsr-2020-0001
Romain, A. C., and Nicolas, J. (2010). Long term stability of metal oxide-based gas sensors for e-nose environmental applications: an overview. Sens. Actuators B Chem. 146, 502–506. doi:10.1016/j.snb.2009.12.027
Saggio, G. (2020). “Are sensors and data processing paving the way to completely non-invasive and not-painful medical tests for widespread screening and diagnosis purposes?,” in Proceedings of the 13th International Joint Conference on Biomedical Engineering Systems and Technologies, 207–214. SCITEPRESS - Science and Technology Publications.
Samala, R. V., and Davis, M. P. (2015). Comprehensive wound malodor management: win the RACE. Cleve Clin. J. Med. 82, 535–543. doi:10.3949/ccjm.82a.14077
Saviauk, T., Kiiski, J. P., Nieminen, M. K., Tamminen, N. N., Roine, A. N., Kumpulainen, P. S., et al. (2018). Electronic nose in the detection of wound infection bacteria from bacterial cultures: a proof-of-principle study. Eur. Surg. Res. 59, 1–11. doi:10.1159/000485461
Scott, I. A., Cook, D., Coiera, E. W., and Richards, B. (2019). Machine learning in clinical practice: prospects and pitfalls. Med. J. Aust. 211, 203–205.e1. doi:10.5694/mja2.50294
Seesaard, T., Thippakorn, C., Kerdcharoen, T., and Kladsomboon, S. (2020). A hybrid electronic nose system for discrimination of pathogenic bacterial volatile compounds. Anal. Methods 12, 5671–5683. doi:10.1039/D0AY01255F
Sen, C. K. (2021). Human wound and its burden: updated 2020 compendium of estimates. Adv. Wound Care 10, 281–292. doi:10.1089/wound.2021.0026
Serra, R., Grande, R., Butrico, L., Rossi, A., Settimio, U. F., Caroleo, B., et al. (2015). Chronic wound infections: the role of Pseudomonas aeruginosa and Staphylococcus aureus. Expert Rev. Anti Infect. Ther. 13, 605–613. doi:10.1586/14787210.2015.1023291
Sun, H., Tian, F., Liang, Z., Sun, T., Yu, B., Yang, S. X., et al. (2017). Sensor array optimization of electronic nose for detection of bacteria in wound infection. IEEE Trans. Ind. Electron 64, 7350–7358. doi:10.1109/TIE.2017.2694353
Sun, T., Tian, F., Bi, Y., Zhong, X., He, J., Yang, T., et al. (2019). Local warning integrated with global feature based on dynamic spectra for FAIMS data analysis in detection of clinical wound infection. Sens. Actuators B Chem. 298, 126926. doi:10.1016/j.snb.2019.126926
Thaler, E. R., Huang, D., Giebeig, L., Palmer, J., Lee, D., Hanson, C. W., et al. (2008). Use of an electronic nose for detection of biofilms. Am. J. Rhinol. 22, 29–33. doi:10.2500/ajr.2008.22.3126
Thet, N. T., Mercer-Chalmers, J., Greenwood, R. J., Young, A. E. R., Coy, K., Booth, S., et al. (2020). SPaCE swab: point-of-care sensor for simple and rapid detection of acute wound infection. ACS Sens. 5, 2652–2657. doi:10.1021/acssensors.0c01265
Thuleau, A., Dugay, J., Dacremont, C., Jemmali, Z., Elard, J., Ricke, Y., et al. (2018). Volatile organic compounds of malignant breast cancer wounds: identification and odors. Wounds 30, 337–344.
Tian, F., Xu, X., Shen, Y., Yan, J., He, Q., Ma, J., et al. (2009). Detection of wound pathogen by an intelligent electronic nose. Sens. Mater 21, 155–166.
Tonekaboni, S., Joshi, S., McCradden, M. D., and Goldenberg, A. (2019). What clinicians want: contextualizing explainable machine learning for clinical end use. Proc. Mach. Learn Res. 106, 359–380. doi:10.48550/arXiv.1905.05134
Trincavelli, M., Coradeschi, S., Loutfi, A., Söderquist, B., and Thunberg, P. (2010). Direct identification of bacteria in blood culture samples using an electronic nose. IEEE Trans. Biomed. Eng. 57, 2884–2890. doi:10.1109/TBME.2010.2049492
Ushimi, Y., Ito, Y., Yoriuchi, H., Kadota, M., Nozaki, Y., Hotta, Y., et al. (2015). Quartz crystal microbalance sensor for NH3 gas with compensation of humidity drift. Electron Comm. Jpn. 98, 1–7. doi:10.1002/ecj.11653
Wang, Y., Li, Y., Yang, J., Ruan, J., and Sun, C. (2016). Microbial volatile organic compounds and their application in microorganism identification in foodstuff. Trends Anal. Chem. 78, 1–16. doi:10.1016/j.trac.2015.08.010
Wang, Y., Liu, A., Han, Y., and Li, T. (2020). Sensors based on conductive polymers and their composites: a review. Polym. Int. 69, 7–17. doi:10.1002/pi.5907
Weber, B., and Deinsberger, J. (2022). Ulcus cruris venosum. Hautnah 21, 43–50. doi:10.1007/s12326-022-00487-5
Wijaya, D. R., Sarno, R., and Zulaika, E. (2019). Noise filtering framework for electronic nose signals: an application for beef quality monitoring. Comput. Electron Agric. 157, 305–321. doi:10.1016/j.compag.2019.01.001
Wijaya, D. R., Sarno, R., and Zulaika, E. (2016). Sensor array optimization for mobile electronic nose: wavelet transform and filter based feature selection approach. Int. Rev. Comput. Softw. 11, 659. doi:10.15866/irecos.v11i8.9425
Wilson, A. D. (2016). Recent progress in the design and clinical development of electronic-nose technologies. Nanobiosens Dis. Diagn 15. doi:10.2147/NDD.S66278
Xu, Y., Dhaouadi, Y., Stoodley, P., and Ren, D. (2020). Sensing the unreachable: challenges and opportunities in biofilm detection. Curr. Opin. Biotech. 64, 79–84. doi:10.1016/j.copbio.2019.10.009
Yan, J., Duan, S., Huang, T., and Wang, L. (2016). Hybrid feature matrix construction and feature selection optimization-based multi-objective QPSO for electronic nose in wound infection detection. Sens. Rev. 36, 23–33. doi:10.1108/SR-01-2015-0011
Yusuf, N., Abdullah, A. A., Omar, M. I., Zakaria, A., Kamarudin, L. M., Shakaff, A. Y. M., et al. (2014). “Comparison of various pattern recognition techniques based on e-nose for identifying bacterial species in diabetic wound infections,” in Advances in intelligent systems. Editors F. L. Gaol, and S. Zeadally (UK: WIT PressSouthampton), 43–60.
Keywords: bacteria, chronic wounds, antibiotic stewardship, machine learning, diagnostic support
Citation: Wörner J, Moelleken M, Dissemond J and Pein-Hackelbusch M (2023) Supporting wound infection diagnosis: advancements and challenges with electronic noses. Front. Sens. 4:1250756. doi: 10.3389/fsens.2023.1250756
Received: 30 June 2023; Accepted: 30 August 2023;
Published: 14 September 2023.
Edited by:
Alisa Rudnitskaya, University of Aveiro, PortugalReviewed by:
Ben Costello, University of the West of England, United KingdomCopyright © 2023 Wörner, Moelleken, Dissemond and Pein-Hackelbusch. This is an open-access article distributed under the terms of the Creative Commons Attribution License (CC BY). The use, distribution or reproduction in other forums is permitted, provided the original author(s) and the copyright owner(s) are credited and that the original publication in this journal is cited, in accordance with accepted academic practice. No use, distribution or reproduction is permitted which does not comply with these terms.
*Correspondence: Miriam Pein-Hackelbusch, bWlyaWFtLnBlaW4taGFja2VsYnVzY2hAdGgtb3dsLmRl
Disclaimer: All claims expressed in this article are solely those of the authors and do not necessarily represent those of their affiliated organizations, or those of the publisher, the editors and the reviewers. Any product that may be evaluated in this article or claim that may be made by its manufacturer is not guaranteed or endorsed by the publisher.
Research integrity at Frontiers
Learn more about the work of our research integrity team to safeguard the quality of each article we publish.