- 1Institute of Sensors, Signals and Systems, School of Engineering and Physical Sciences, Heriot-Watt University, Edinburgh, United Kingdom
- 2Hill & Mountain Research Centre, Scotland’s Rural College (SRUC), Edinburgh, United Kingdom
Agroforestry can be defined as an agroecosystem whereby soil is used holistically and synergistically by various stakeholders including farmers, livestock, and plants. As such, agroforestry offers numerous benefits that include conservation of biodiversity, regulation of pests and diseases, increased quality of soil, air and water, efficient cycling of nutrients, and resilience to climate change. Review of published studies in agroforestry shows however that research in this area could benefit from increased real-time, spatial and temporal measurements. This situation is to be contrasted with that of precision agriculture in monocultures and precision livestock farming where progress made in sensor systems has attracted considerable research interest. It is advocated in this review article that wireless sensor networks could also significantly impact agroforestry through the monitoring of the local real-time interactions that occur between the various components constituting agroforestry systems. This review article proposes therefore the new field of data-driven agroforestry which lies at the intersection of precision agriculture, precision livestock farming, permaculture, and agroforestry. Data-driven agroforestry has the potential to not only help farmers harness the interactions between the different components of an agroforestry system to their advantage but also shine light on fundamental interactions between soil, plants, trees, and livestock while offering a sustainable agricultural method beneficial to all agroforestry stakeholders.
1 Introduction
Agroforestry is a multi-functional land use system that can be basically described as agriculture with trees and livestock. Agroforestry systems take advantage of the mostly poorly understood interactions that occur amongst crops, trees and livestock. Agroforestry has been demonstrated to present numerous benefits which include conservation of biodiversity1, regulation of natural pests and diseases, regulation of soil, increased air and water quality, efficient cycling of nutrients, and resilience to climate change (Rao et al., 1997; Mateo-Sagasta et al., 2009; Smith et al., 2013; Khan et al., 2021). This form of land management is not a novel idea, and dates back to at least the middle ages in the developing world, and probably earlier in other cultures (Steppler and Nair, 1987).
John Bene from Canada’s International Development Research Centre was the first in the early 1970s to coin the term agroforestry and highlight the benefits of a multi-functional land use system2. Further progress led to the establishment of the International Council for Research in Agroforestry (ICRAF) in 1978 to promote agroforestry research in developing countries (Steppler and Nair, 1987). Since then, agroforestry has attracted strong interest as evidenced by the compilation of articles shown in Figure 1 where the number of publications in the electronic database Scopus™ was obtained by using the keywords “agroforestry,” “precision agriculture,” and “agroforestry and sensors.” It is not surprising that the exponential increase in the number of research articles coincide with the birth of the ICRAF. Most of the research articles however focus on tropical climates and rely on a posteriori measurements.
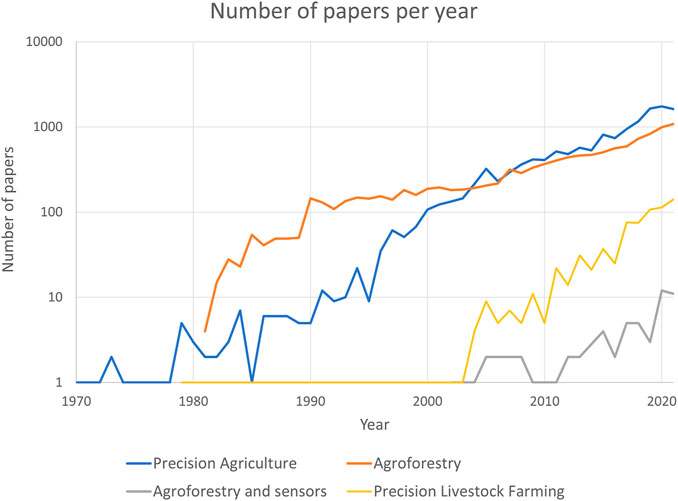
FIGURE 1. Yearly number of published papers. The keywords used in the electronic database Scopus™ were “agroforestry,” “precision agriculture,” “precision livestock farming,” and “agroforestry and sensors.” Note the logarithmic scale on the vertical axis.
Figure 1 shows that the increase in precision agriculture research coincide with the rise of technological development, especially with the Internet of Things (IoT). Precision agriculture is defined as a management strategy that considers temporal and spatial variability to improve the sustainability of agricultural production3 (Mulla and Khosla, 2016). Similarly to precision agriculture, precision livestock farming (PLF) is becoming increasingly popular. Precision livestock farming refers to the management and monitoring of livestock through the use of real-time, continuous monitoring of animal health, welfare, production, reproduction, and environmental impact (Berckmans, 2017). As such, they both rely on the heavy use of sensors whose relevant sensing modalities will be reviewed in this paper. This type of management system based on a data-driven approach has the potential to meet the increasing demand for food while ensuring sustainable farming practices (Precision farming, 2018).
In contrast, few data-driven approach studies exist for agroforestry systems as shown in the remarkably small number of papers combining agroforestry and sensors, which might be due to the lack of knowledge in wireless sensor technology from the agroforestry community. Research in agroforestry could benefit from sensors and wireless sensor networks that are deployed today in typical monocropping agricultural systems. This paper attempts therefore to investigate the sensors used in precision agriculture and see how these could benefit agroforestry.
Section 2 presents a review of agroforestry in temperate climates and highlights the main gaps in current agroforestry research. A broad review of precision agriculture is provided in Section 3 with an emphasis on the type of sensors and the manner of which wireless sensor networks that are currently being used in agriculture. Section 4 presents an overview of precision livestock farming and the types of sensors used in livestock farming. Permaculture is presented in Section 5 and compared to precision farming and agroforestry. A new field of study, called data-driven agroforestry (DDA), is advocated for in Section 6 where the potential of combining the non-invasive nature of miniaturized sensors and their mode of interrogation with agroforestry methods. In addition, this section includes suggestions of sensors to be used to measure the interactions in an agroforestry system as well as areas that still need development.
2 Principles of agroforestry
As the world population increases, there is a growing need for multi-functional land use systems that can meet the multiple demands of food and fuel production, environmental and biodiversity protection, and has the capacity for adaptation and resilience to climate change (Hislop and Claridge, 2000; Smith et al., 2013). Agroforestry can be a sustainable alternative to traditional agricultural practices by reducing its negative impacts by, for example, regulating soil, water, and air quality (Hislop and Claridge, 2000; Smith et al., 2013; Augère-Granie, 2020).
Currently, agriculture accounts for 70% of water withdrawals worldwide and is one of the major contributors to water pollution, according to the Food and Agriculture Organization (FAO) (Mateo-Sagasta et al., 2009). Farms discharge large quantities of agrochemicals, organic matter, drug residues, sediments, and saline drainage into bodies of water (Mateo-Sagasta et al., 2009). In most high-income countries, this agricultural pollution has overtaken contamination from settlements and industries as the main factor in the degradation of inland and coastal waters (Mateo-Sagasta et al., 2009). This pollution causes eutrophication in aquatic ecosystems and human health disorders (Mateo-Sagasta et al., 2009).
Moreover, there is growing evidence that agroforestry systems increase overall productivity (Augère-Granie, 2020; Khan et al., 2021). In agroforestry systems, productivity can be measured using the Land Equivalent Ratio which compares the yield from growing two or more components together versus the yield from growing them separately (Augère-Granie, 2020). Moreover, agroforestry systems do not need as much pesticide and fertilizer as traditional monocropping systems, thus reducing the cost of farming inputs (Perks et al., 2018; Augère-Granie, 2020). When livestock are introduced into the system, agroforestry can also reduce feeding costs (Perks et al., 2018). Apart from crops and livestock, agroforestry systems have the ability to produce outputs from the trees such as food, fuel, fodder, fibre, and timber which could yield an increase in economic profit due to the multiple outputs from this type of system (Augère-Granie, 2020). In addition, these multiple outputs reduce the risk associated with producing one product in the event of shortage periods due to adverse weather conditions or crop disease (Hislop and Claridge, 2000; Perks et al., 2018).
Agroforestry systems also benefit from increased animal welfare by providing protection from wind, rain, sun and the cold (Augère-Granie, 2020). Moreover, shrubs or trees offer protection from predators and encourages natural animal behaviour such as foraging (Augère-Granie, 2020). Lastly, agroforestry systems can also have recreational benefits (Hislop and Claridge, 2000; Augère-Granie, 2020). The motivation behind agroforestry is therefore multi-fold. Discovering the means to monitor, understand, and manage the interactions of the various agents within this ecosystem is the key to unlocking its full potential.
2.1 Types of agroforestry systems
There are several types of agroforestry systems used around the world. As shown in Figure 2, common configurations include (Mosquera-Losada et al., 2018):
• Silvopastoral systems which consist of a combination of trees or shrubs with forage and livestock (Mosquera-Losada et al., 2018).
• Silvoarable systems which are widely spaced woody vegetation intercropped with annual or perennial crops, also known as alley cropping (Mosquera-Losada et al., 2018).
• Forest farming systems which are forested areas used for harvesting crops for medicinal, ornamental, or culinary uses (Mosquera-Losada et al., 2018).
• Homegardens which combine trees or shrubs with vegetable production in urban areas (Mosquera-Losada et al., 2018).
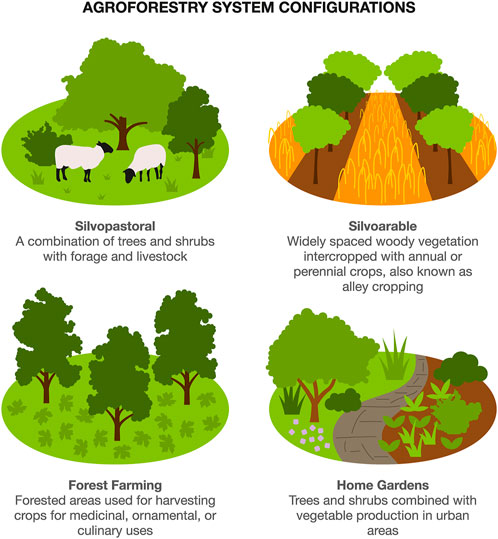
FIGURE 2. Types of agroforestry systems. Silvopastoral and silvoarable agroforestry systems are the most common in Europe.
Silvopastoral and silvoarable systems tend to be more frequent in Scotland and the United Kingdom and thus will only be considered here. Within silvopastoral and silvoarable agroforestry systems, there are several options for combining trees and shrubs with crops or livestock such as (Mosquera-Losada et al., 2018; Perks et al., 2018):
• Windbreaks and riparian buffer strips made of trees or shrubs to act as a shelterbelt.
• Rows of trees or shrubs (Perks et al., 2018).
• Single trees or tree cluster arrangements. These are best applied to silvopastoral systems (Perks et al., 2018).
Depending on the agroforestry practice used, the arrangement of components, and the types of trees, shrubs, and crops planted, there will be differing interactions that take place thus, each having potentially different outcomes.
2.2 Effects of interactions in agroforestry systems
The nature of interactions taking place in agroforestry systems arise from the addition of trees or shrubs to arable or livestock systems. These interactions affect the following areas (Rao et al., 1997; Smith et al., 2013):
• Soil fertility including soil chemical, physical and biological interactions.
• Competition for soil water, nutrients, and sunlight.
• Microclimate
• Pests and diseases
• Soil conservation
These will be further explained in the next sections.2.2.1 Soil fertility
Sustainable agriculture is highly dependent on a large community of soil organisms interacting with diverse crops to recycle nutrients (Østergård et al., 2009). The most important determinants of soil quality and health are the amount of soil organic matter and the level of microbial diversity (Østergård et al., 2009). These improve soil structure and water retention making soil more tolerant to stresses like droughts and compaction. They also help sequester carbon and mitigate the effects of soil salinity, acidity and alkalinity (Østergård et al., 2009).
Improved physical soil properties include better soil aggregation, lower bulk density, lower resistance to water penetration, and improved soil porosity (Rao et al., 1997). This results in improved water infiltration, higher saturated hydraulic conductivity, and higher water holding capacity (Rao et al., 1997). These properties originate primarily from increased soil organic matter and root activity (Rao et al., 1997). For instance, the decay of roots leaving behind channels increases infiltrability and enhances groundwater recharge (Bargués Tobella et al., 2014; Bayala and Prieto, 2020). Through the process of hydraulic lift, trees or shrubs with deep roots can lift and redistribute water and nutrients, inaccessible to crops to the upper layers of the soil thus acting as bio-irrigators to adjacent plants (Bayala and Prieto, 2020). During the dry season, trees obtain most of their water from deep soil levels when upper soil levels are dry and can distribute this water to the upper levels for the crops to access (Bayala and Prieto, 2020). This can help increase survival and growth of crops by providing a buffer against droughts and ensure productivity in regions with erratic rainfall (Bayala and Prieto, 2020).
Furthermore, because of the access of the tree to nutrients in deeper soil layers, once leaves fall onto the soil and decompose, increasing soil organic matter, these nutrients get cycled into the upper soil layers making them accessible to crops in this way as well (Rao et al., 1997; Bayala and Prieto, 2020). This promotes microbial processes and decomposition which release nutrients from organic matter and soil minerals (Rao et al., 1997; Bayala and Prieto, 2020).
Common mycorrhizal networks (CMN) also play a role in linking plants together (Alaux et al., 2021). These are formed by mycorrhizal fungi which make up a complex and dense network of mycelium in soil allowing the plant’s roots to access otherwise inaccessible soil. This network allows for an exchange of nutrients and water affecting plant resistance and tolerance to stresses (Alaux et al., 2021). There is evidence that sustainable agricultural practices, such as agroforestry, have a positive effect on CMNs (Battie-Laclau et al., 2020; Alaux et al., 2021).
2.2.2 Competitive landscape
Competition for resources including water, nutrients, and light occurs between the different components of an agroforestry system (Rao et al., 1997).
Root distribution from many trees overlaps the upper soil layers with shallower root systems from the crops which could generate competition for water and nutrients (Bayala and Prieto, 2020). This can be mitigated with methods such as tree pruning which has proven to reduce roots in the upper soil levels (Bayala and Prieto, 2020).
Another form of competition stems from the shade from the trees that can limit the amount of light received by crops under the canopy, but also protect livestock from sun overexposure as explained in the next section (Hislop and Claridge, 2000). However, protection from the trees can extend the grass growth season and increase the soil temperature in early spring and late autumn (The Woodland Trust, 2017).
2.2.3 Microclimate
Another result from planting trees or shrubs is their effect on the creation of a microclimate through the alteration of temperature, humidity and wind speed (Hislop and Claridge, 2000). In urban areas, tree planting can result in temperature change by several degrees (Vaz Monteiro et al., 2019). Within agriculture, trees can improve animal welfare by providing shelter for outdoor livestock from rain, wind, and sun (Perks et al., 2018). Moreover, the lowered wind speeds can reduce significant problems with wind erosion of soils (Perks et al., 2018). In addition, the tree’s canopy offers protection from raindrop impact reducing the formation of soil crusts which limits water infiltration (Bargués Tobella et al., 2014).
Heat increase is expected in the future which will affect animal pregnancy rates, weight, milk production, and overall animal health (Smith et al., 2022). The shade provided by trees, specifically in silvopastoral systems, can ease some of the issues that will be encountered in the future (Smith et al., 2022).
2.3 Agroforestry systems in temperate climates
Most of the research studies published in agroforestry have focused on tropical climates probably due to the higher popularity of agroforestry systems in tropical climates. This review article concentrates on temperate systems, more specifically silvopastoral systems (Bergez et al., 1997; Teklehaimanot et al., 2002; Morgan-Davies et al., 2008; The Woodland Trust, 2014; The Woodland Trust, 2015; Beckert et al., 2016; Lunka and Patil, 2016; Upson et al., 2016; The Woodland Trust, 2017; McAdam, 2018; Pantera et al., 2018; Smith, 2018; Lehmann et al., 2020; Varah et al., 2020) and silvoarable systems (Pantera et al., 2018; Smith, 2018; Lehmann et al., 2020; Varah et al., 2020).
For such studies, the age of the agroforestry systems needs to be considered as the time it takes for farm production from trees is a lot greater than that of annual crops and livestock (Raskin and Osburn, 2019). The amount of time required depends on the tree species and the end use of the tree product (Raskin and Osburn, 2019). The economics of agroforestry are further discussed in (Hislop and Claridge, 2000) and (Raskin and Osburn, 2019). Table 1 presents a breakdown of the location and age of the agroforestry systems reviewed.
Articles consulted in this review have been separated according to objectives of agroforestry systems including increase of productivity (Morgan-Davies et al., 2008; The Woodland Trust., 2014; The Woodland Trust., 2015; The Woodland Trust., 2017; Pantera et al., 2018); Teklehaimanot et al., 2002; Lehmann et al., 2020) and environmental benefits (Bergez et al., 1997; The Woodland Trust., 2014; The Woodland Trust., 2015; Beckert et al., 2016; Lunka and Patil, 2016; Upson et al., 2016; McAdam, 2018; Pantera et al., 2018; Varah et al., 2020), and also as to whether the interactions described were studied from a scientific or technological point of view (Bergez et al., 1997; Smith, 2018). The management of the agroforestry system will differ depending on the pursued objectives of the agroforestry plot. The following sub-sections discuss and group the most relevant papers by objective and summarize the performance metrics used to measure and quantify this objective.
2.3.1 Productivity
The productivity objective considers the increased economic profit that can be harvested from the agroforestry system. This includes crop, tree, and livestock yield (direct performance metrics) as well as the decrease of livestock mortality and overall livestock welfare (indirect performance metrics). A review of all performance metrics and key performance indicators related to this objective is provided in Table 2.
Morgan-Davies et al. combined hill sheep production with native woodland creation in a Scottish mountain valley and compared initial bio-economical modelling results and data over a 5-year period of study (Morgan-Davies et al., 2008). Some of the defined performance metrics are indicated in Table 2. Results demonstrate that, with the exception of the expected slow rate of woodland establishment, results exceeded expectation according to the combined system economics where actual returns were 20% more than predicted (Morgan-Davies et al., 2008).
In (Pantera et al., 2018), Pentarra et al. reviewed different European agroforestry systems to understand how the integration of understory crops such as chickpeas and barley, and/or livestock within high value tree systems like apple orchards and olive groves can affect production, environmental, or economic aspects. Their review illustrates that this land management system provides financial benefits from the extra products provided and reduces required management, societal benefits from the aesthetics of the landscape, and environmental benefits including improved carbon sequestration for climate regulation and reduced water pollution (Pantera et al., 2018).
The case study in (The Woodland Trust, 2017) demonstrated how mob grazing, tree and hedge planting can improve farm productivity and soil health while decreasing input costs. A decrease in lamb mortality was witnessed due to the new availability of shelter, higher biodiversity in the soil measured by worm counting, and a longer grass growing season (The Woodland Trust, 2017). Similarly in (The Woodland Trust, 2014), the planting of trees and shrubs helped reduce lamb mortality due to hypothermia. Trees also have the effect of reducing water pollution and poaching (The Woodland Trust, 2014). In addition, the case study in (The Woodland Trust, 2015) showed how the planting of trees improved soil conditions, provided shelter to dairy cows as well as nutritional and medicinal fodder to the herd.
Teklehaimanot et al. compared the growth of trees evenly dispersed in the plot against those planted in clumps in a silvopastoral system (Teklehaimanot et al., 2002). Livestock productivity was measured to be the same regardless of the planting pattern or species (Teklehaimanot et al., 2002). In addition, the growth of sycamore trees was found to be lower if highly spaced rather than in conventional forestry, while planting trees in small clumps reduced tree protection costs (Teklehaimanot et al., 2002).
Lehmann et al. assessed in (Lehmann et al., 2020) the agronomic productivity and economic viability of diverse agroforestry systems in Europe. Five agroforestry systems were reviewed and the Land Equivalent Ratio was calculated providing evidence for agronomic productivity and economic viability of agroforestry systems (Lehmann et al., 2020).
2.3.2 Environmental benefits
Environmental benefits include biodiversity, soil health, and water quality as shown in Table 3. Key performance indicators include biodiversity and soil indicators, tree measurements and productivity.
Varah et al. (2020) studied whether agroforestry can benefit wild pollinators. The study looked at wild insect pollinator abundance, species richness, and pollination services in six organic agroforestry and monocrop systems in the United Kingdom. They measured pollinator abundance by transect walking, species richness with pan traps, and pollination services with phytometers. Agroforestry systems in the United Kingdom were judged to be able to play a part in sustainable agriculture by supporting a greater number of wild insect pollinators, greater pollination service and in some sites greater wild bee species (Varah et al., 2020).
Beckert et al. compared the carbon sequestration potential of silvopastoral and woodland plots by taking soil samples, treating them in a laboratory, and calculating the amount of soil organic carbon (Beckert et al., 2016). They showed that silvopasture had similar or even greater soil carbon stocks compared to woodland plots (Beckert et al., 2016).
Canopy interception, soil water infiltration, and bulk density were compared in (Lunka and Patil, 2016) between a control pasture and two silvopastoral planting configurations composed of trees planted evenly and grazed, and trees clumped in fenced-off ungrazed plots, to characterize the potential of these configurations to reduce local flood risk. Soil water infiltration was measured using a single ring infiltrometer; bulk density was determined by extracting a soil sample and analysing it in a laboratory. Canopy interception measurements were made using throughfall gauges. The results suggest that silvopastoral systems are more likely to benefit from clumped and ungrazed tree configurations because of enhanced infiltration, lower soil compaction, and increased canopy interception (Lunka and Patil, 2016).
To the best of the authors’ knowledge and at the time of writing, references (Bergez et al., 1997; McAdam, 2018) and (Upson et al., 2016) are the only ones that specifically mention the use of in-field sensing. In (McAdam, 2018), MacAdam demonstrates that silvopastoral systems can significantly extend the grazing season to improve grass utilisation. They measured soil carbon storage by sampling and analysing the soil. Total tree carbon content was measured by excavating the tree and analysing it. Soil resistance to penetration, which gives an indication of water infiltration potential, was measured weekly using a penetrologger, an instrument allowing in situ measurement of soil penetration. Soil moisture was also measured weekly. The method of measurement is however not specified. In (Bergez et al., 1997), photosynthetically active radiation (PAR) was measured using a PAR quantum DRP-1 sensor to determine how widely spaced eight-year-old trees in a silvopastoral system affect light reaching the sward and how the light is modified depending on the tree planting density, weather, and development of foliage area of the trees. Other measurements included overall pasture production measured by the carrying capacity which is dependent on the weight and number of animals. The result recorded a small amount of radiation intercepted by the tree canopies which led to no significant differences observed in the annual carrying capacity (Bergez et al., 1997). The study in (Upson et al., 2016) determined the effect of woodland and silvopastoral systems on soil bulk density and organic carbon content. The authors measured bulk density, fine root mass, and soil organic carbon in the laboratory, and used an in situ capacitive soil moisture sensor (Upson et al., 2016). They showed that, 14 years after planting, the silvopastoral agroforestry system stored about 5% more carbon than the equivalent areas of separate woodland and pasture (Upson et al., 2016).
2.3.3 Interactions studies
Only two papers in the review had the objective of understanding interactions of the components of an agroforestry system (Bergez et al., 1997; Smith, 2018). In (Smith, 2018) the authors reported on the interactions between trees, crops, and both wild animals and livestock by measuring factors such as tree and animal interactions (number of trees browsed by livestock), pasture production, and earthworm abundance. They found that, in the first 6 years, there was no significant impact of trees on alley crops. The protection of the trees from livestock damage was however essential in the early years.
2.4 Discussion
Despite the substantial amount of research in agroforestry, only three papers used any type of in situ soil measurements (Bergez et al., 1997; Upson et al., 2016; McAdam, 2018). Most of the papers used destructive, remote, or expensive methods of measurement. No papers reported the use of data-driven approaches to studying these temporal and spatial interactions in real-time. A large area of research is still yet to be investigated.
3 Precision agriculture
Precision agriculture (PA) is defined as a management strategy that takes account of the temporal and spatial variability to support management decisions for improved resource efficiency, productivity, quality, profitability, and sustainability of agricultural production4. The main goal of PA is to apply just enough resources to sustain or increase productivity (Zarco-Tejada et al., 2014). Precision agriculture benefits the environment by this reduced use of farming inputs to avoid serious negative environmental effects (Bongiovanni and Lowenberg-DeBoer, 2004; Mateo-Sagasta et al., 2009). The rapid technological advancements in sensors, big data analytics, and cloud computing have increased the use and interest in precision agriculture.
A key technology enabling PA is Wireless Sensor Networks (WSN). These are reviewed first in this section, followed by communication protocols. Lastly, sensors used in precision agriculture are presented and grouped into three categories: soil, environmental, and plant. Their working principles are then explained.
3.1 Wireless sensor networks
A Wireless Sensor Network is a network of wireless sensors, called nodes, which communicate data wirelessly to a base station or coordinator node (Jawad et al., 2017). WSNs can be used in agriculture to collect, monitor, and analyse data from the field (Kumar and Ilango, 2018). In agricultural settings, WSNs should monitor in situ a large area with precise field information, helping minimize the burden of farmers in terms of efforts and manpower needed to retrieve the necessary information (Haule and Michael, 2014; Kumar and Ilango, 2018).
WSNs can suffer from short communication range, low energy efficiency, poor communication bandwidth, and poor fault tolerance (Jawad et al., 2017). It is likely that communication bandwidth is unlikely to be an issue as the amount of information requested, although in real-time, does not need to be collected at a high bit rate. Topography and presence of obstacles in agricultural fields could result in a loss or weakening of signals (Jawad et al., 2017). Sensor nodes are mainly limited by their battery, thus energy efficiency or energy harvesting are imperative to prolong battery life, potential solutions of which are provided in (Jawad et al., 2017). Lastly, sensor nodes are fault prone due to issues such as battery depletion and hardware fault (Ojha et al., 2015).
3.1.1 Wireless communication protocols
Some of the different wireless protocols and standards used in agriculture include ZigBee, Bluetooth, Wi-Fi, GPRS/2G/3G, Long Range Radio (LoRa), and SigFox. Table 4 compares these along with the Narrowband IoT (NB-IoT) and 5G, although they are not yet widely used in agriculture.

TABLE 4. Summary of WSN communication technology (Jawad et al., 2017; Mekki et al., 2019; Tang et al., 2021).
The ZigBee protocol is characterised by its low cost and low power consumption due to its low duty cycle and sleep mode (Jawad et al., 2017). However, communication range is short so may not be suitable for all uses (Jawad et al., 2017). Bluetooth is widely available, easy to use, and has low power consumption with Bluetooth Low Energy (BLE) (Jawad et al., 2017). However, it has a short communication range that could make it impractical for some agricultural purposes (Jawad et al., 2017). Wi-Fi technology adopts data redundancy techniques but requires a high amount of power, long communication time, and a large data payload (Jawad et al., 2017). Throughput and delay are variable in GPRS technology (Ojha et al., 2015). Its performance also depends on the volume of consumers that share communication channels and resources (Ojha et al., 2015). LoRa is characterised by its low power consumption, and long communication range making it suitable for agricultural domains (Jawad et al., 2017; Gresl et al., 2021). However, LoRa gateways have a limited message capacity (Jawad et al., 2017). It is also complex and needs substantial hardware implementation (Jawad et al., 2017). SigFox has low power consumption and long range, however it has low data rates and slow transmission (Jawad et al., 2017). Narrowband-IoT, along with SigFox and LoRa, are part of the low power wide area networks (LPWAN) (Mekki et al., 2019). However unlike SigFox and LoRa, NB-IoT deployment is limited to LTE base stations (Mekki et al., 2019). Most farms, especially in rural areas, may not have LTE coverage thus this option may be unsuitable for agricultural purposes (Mekki et al., 2019). The 5G network is characterised by its high speed and low latency (Tang et al., 2021). However, 5G uses a low frequency band and therefore a short range but achieves high connectivity through the use of small base stations (Tang et al., 2021). The application of 5G can be suitable to farming due to its wide coverage, low energy consumption, and low cost devices (Tang et al., 2021).
Due to its low power consumption and long communication range, currently LoRa is possibly today the best option for agricultural settings. In general, most agricultural measurements do not need a high data rate as the measurements do not change with a high frequency. Due to the relatively low data rates, the sensors only use a small amount of power thus, a small solar panel would likely be sufficient to power the sensor. Table 5 suggests estimates for minimum data rates needed for soil, environmental, and plant measurements.
3.2 Remote sensing in precision agriculture
The high availability of satellites and unmanned aerial vehicles (UAV) and their high spatial, temporal, and spectral resolution have made remote sensing technologies more popular in agricultural settings (Sishodia et al., 2020). Remote sensing techniques can help detect pests and diseases, measure production5, crop stress, crop nutrient status, and soil moisture, just to name a few (Messina and Modica, 2020; Sishodia et al., 2020).
3.3 Measurands and sensors in precision agriculture
Quantification of soil quality, environmental conditions, and effects on plant phenotypes will depend on the measurands to monitor, the sensing modalities to use, and the type of sensor to perform the measurement. Table 6 summarises the types of measurands used in that regard. The following sections provide a breakdown of the different types of measurements and an overview of the sensors used to quantify the measurands.
3.3.1 Soil measurements
Soil has multiple important uses: it helps filter water, decompose materials, and mitigates the effects of climate change by acting as a sink for greenhouse gases. There are several aspects of soil that need to be taken into consideration in order to asses soil quality, understand the effects of farm management, and interpret the interactions that take place from different components on the soil.
3.3.1.1 Soil moisture
Effective water usage is of high importance in agriculture (Barapatre and Patel, 2019). Soil moisture is a significant factor in determining yield; thus, soil moisture sensors can help farmers use water with efficacy and efficiency (Barapatre and Patel, 2019). Monitoring soil moisture provides important information on soil health and moisture retention which are key indicators of sustainable agroecosystems (Kashyap and Kumar, 2021). Soil moisture is the most sensed indicator as shown in Table 7, with 61% of papers reviewed quantifying this measurand.
Resistive and capacitive sensors are the two main types of sensors used for soil moisture. Resistive sensors such as FC-286 and SEN133227 use two probes to send a current through the soil and measure the resistance to quantify the moisture (Barapatre and Patel, 2019). Dry soil is a poor conductor, thus the less moisture, the more resistive the soil (Barapatre and Patel, 2019). However, resistive sensors are limited because of corrosion of the probes that could cause inaccurate measurements (Barapatre and Patel, 2019). In addition, adding fertilizer could decrease the soil resistance even if no water is added (Barapatre and Patel, 2019).
Another resistive soil moisture sensor is the WATERMARK granular matrix sensor8. It uses gypsum wafers surrounded by a porous granular filler material with electrodes embedded within it which is buried in the soil (Kashyap and Kumar, 2021). Gypsum begins to dissolve from the soil moisture which allows for ions to move within the porous material reducing the resistance between electrodes (Kashyap and Kumar, 2021). These sensors are easy to manufacture and low price, however they have poor accuracy, with errors ranging between 10% and 25%. In addition, the gypsum is affected by temperature and has a slow response to soil changes, about 24 h (Enciso et al., 2007; Kashyap and Kumar, 2021).
Capacitive sensors such as the EC-59, SEN019310, and 10HS11, measure the dielectric permittivity constant of the soil (Barapatre and Patel, 2019). Such sensors are made out of a corrosion resistant material making them less susceptible to corrosion than resistive soil moisture sensors (Barapatre and Patel, 2019). They are also more specific as inputs such as fertilizers will not significantly alter their readings (Barapatre and Patel, 2019). These types of sensors operate at a frequency in the order of 10 MHz (Kashyap and Kumar, 2021). Both the EC-5 and 10HS operate at a frequency of 70 MHz which minimizes the salinity and textual effects of the soil. In addition, the 10HS has longer probes compared to EC-5 and SEN0193 thus allowing for measurement of up to 1 L of soil volume compared to 0.2 L for EC-5. This makes the 10HS better at averaging varying soil moisture and characterizing spatial variability. Figure 3A shows examples of these sensors.
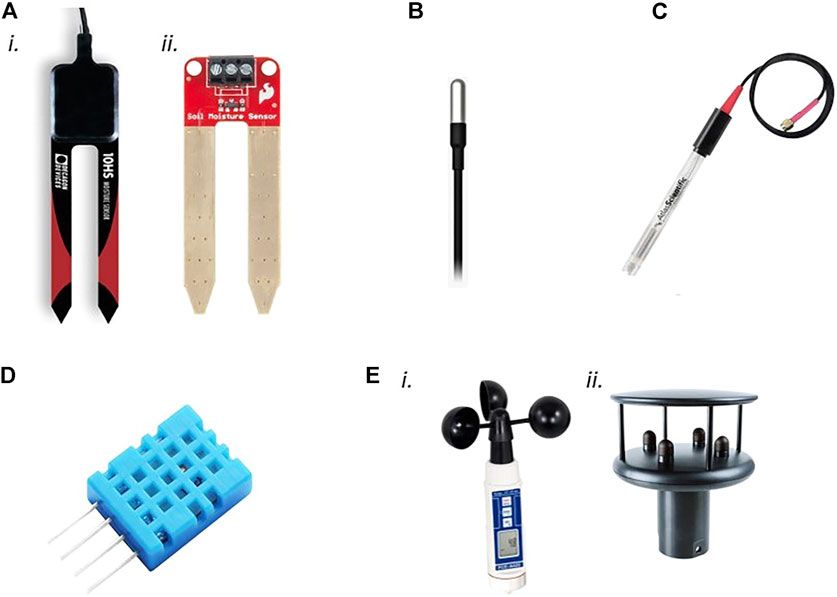
FIGURE 3. (Ai) 10HS capacitive soil moisture sensor. (Aii) SEN13322 resistive soil moisture sensor. (B) RT-1 soil temperature sensor. (C) Atlas Scientific soil pH sensor. (D) DHT11 temperature and humidity environment sensor. (Ei) PCE-A420 cup anemometer. (Eii) Windsonic M ultrasonic anemometer. All image permissions given by respective companies.
3.3.1.2 Soil temperature
Soil temperature influences all processes that occur in the soil including water content, acquisition of mineral nutrients, and storage of organic carbon (Pregitzer and King, 2005). In addition, soil temperature affects plant physiological aspects like root growth and composition, and function of soil microbial communities (Pregitzer and King, 2005). There are several factors that affect soil temperature such as atmospheric properties, surface properties like land cover and topography, and soil properties such as porosity and bulk density (Lehnert, 2014).
The DS18B20 is a digital thermometer that operates without an external power supply12. Power can be supplied through the control line which charges an internal capacitor supplying power to the device when the bus is low. The sensor measures temperatures ranging from −55°C to 125°C with a user-configurable resolution of 0.5°C, 0.25°C, 0.125°C, or 0.0625°C. The DS18B20 has an accuracy of ±0.5°C from -10°C to 85°C.
Figure 3B shows an examples of the RT-113 soil temperature sensor.
3.3.1.3 Soil nutrients, pH and texture
Nitrogen (N), phosphorous (P), and potassium (K) are considered the most important macronutrients as they are responsible for assimilating proteins, synthesizing metabolic energy, and producing ATP (Burton et al., 2020). These nutrients vary spatially across the agricultural field. Limited nutrients can result in a reduction of plant growth and a change in plant structure (Burton et al., 2020). Excess of nutrients which are not absorbed, are leached into ground and surface water during rainfall and irrigation which can pollute local water supplies harming humans and biodiversity (Burton et al., 2020).
In situ soil nutrient sensing currently remains a technical challenge (Burton et al., 2020). Most soil nutrient measurements involve random grid sampling to obtain soil cores. The cores are transported to a laboratory where the cores are analysed using methods such as Kjeldahl wet digestion, dumas combustion, and gas chromatography mass spectrometry (Muñoz-Huerta et al., 2013; Burton et al., 2020). Although these methods are highly accurate, they are expensive, time-consuming, and do not allow for real-time in situ sensing (Burton et al., 2020).
Overall, in situ soil nutrient sensors involve optical, or electrochemical methods (Burton et al., 2020). Optical sensors identify soil nutrients based on the magnitude of energy absorbed and reflected by nutrient ions (Burton et al., 2020). Electrochemical sensors use ion-selective electrodes to initiate a current or voltage output that reflects the concentration of target ions (Burton et al., 2020).
Very few articles reviewed measure soil nutrients. One of them uses the ISL29125 light sensor to measure the reflection characteristics of the N, P, and K minerals found in soil (Grimblatt et al., 2019). The measurement is done through the reflection characteristics of these minerals based on the Lambert-Beers Law. At the time of writing, the described system was not tested, thus there are no results as to how accurate this method of measurement is.
In (Golicz et al., 2019) authors investigate the potential for a smartphone to assess soil nutrients through the use of commercially available, single use, nitrate and phosphate sensitive test strips. The smartphone application can relate the reaction colour of the strips to the concentration of soil nutrients. They concluded that smartphone-mediated soil analysis can be successful for nitrate-nitrogen,
In (Smolka et al., 2017) the researchers developed a mobile lab-on-a-chip device to measure soil nutrients including NO3, NH4, K, and PO4. The sensor uses capillary electrophoresis to separate different ions and pass them through a detector. Although the time to get the measurements was high, the sensors successfully detect soil nutrients.
Reference (Pei et al., 2019) demonstrates the potential for in situ estimation of soil profile properties. They use optical diffuse reflectance spectroscopy to measure soil properties in the field such as soil organic carbon, total nitrogen, moisture, soil texture, cation exchange capacity, calcium, magnesium, potassium, and pH (Pei et al., 2019).
There are further developments using handheld, portable X-ray fluorescence spectrometers and near infrared spectrometers which claim to be able to detect soil organic matter (SOM) and soil organic carbon (SOC). The authors of (Ravansari et al., 2021), which created14, developed an attachment for a portable x-ray fluoresence (PXRF) spectrometer, which cannot directly measure carbon or other light elements, using visible near infrared (Vis-NIR). This attachment captures antecedent x-rays from the PXRF and has initially proven to estimate soil nutrients. The S1 Titan developed by Bruker15, is a PXRF spectrometer. It can detect elements from magnesium to uranium at low concentrations, however as just mentioned, it cannot quantify light elements such as carbon. Thus, this does not give complete information on SOM or SOC.
Soil pH affects nutrient solubility and availability (McCauley et al., 2009). Factors that influence soil pH include organic matter decomposition,
Soil texture refers to the size distribution of soil particles (Dexter, 2004). Textures affect water and nutrient retention (Dexter, 2004; Grimblatt et al., 2019). Soil texture does not change frequently, thus such measurements are usually carried out once in a laboratory.
3.3.2 Environmental measurements
Environmental measures including air temperature, humidity, pressure, light intensity, and wind speed have significant effects on the health and productivity of crops and trees (Ferrante and Mariani, 2018). Temperature affects a plants metabolism as temperatures either too high or too low can compromise plant growth and damage crops (Ferrante and Mariani, 2018). Light allows the plant to convert water and carbon dioxide into carbohydrates through the process of photosynthesis. The correct light intensity is necessary to drive production, however too much or too little can affect humidity, crop transpiration, and water balance (Ferrante and Mariani, 2018). Overall, these external environmental measures could cause abiotic stress and result in a loss of productivity (Mariani and Ferrante, 2017; Ferrante and Mariani, 2018).
Temperature, humidity, atmospheric pressure, light intensity, and wind speed can also be helpful in correlating sensed soil data with environmental information. Such measurands are summarized in Table 8.
3.3.2.1 Temperature and humidity
A common type of temperature sensors is the Negative Temperature Coefficient (NTC) thermistor17. A NTC thermistor correlates resistance with temperature; as resistance increases, temperature decreases.
Relative humidity relates the current absolute humidity to highest possible absolute humidity based on the current temperature. In capacitive humidity sensors, humidity affects the permittivity of the dielectric constant18. In resistive humidity sensors, the resistance of the conductive film contained in the sensor changes inversely based on the humidity.
Both the DHT1119 and DHT2220 comprise of a NCT measurement component and a resistive-type humidity sensor. The DHT11, seen in Figure 3D, has a temperature sensing range of −20°C–60°C with an accuracy of ±1°C and a humidity sensing range of 5–95% with an accuracy of ±5%. The temperature measurement range of the DHT22 is from -40°C to 80°C for an accuracy of ±0.5%; the humidity ranges from 0 to 99.9% for an accuracy of ±2%.
The Si7021 I2C is a monolithic CMOS integrated circuit combining humidity and temperature sensors. The humidity range of 0–80% is provided with an accuracy of ±3%. The temperature range of -10°C–85°C has an accuracy of ±0.4°C.
Similarly to the DHT11 and DHT22, the C10-M53R is a resistive21 humidity sensor22. It has a humidity sensing range of 20–90% with an accuracy of ±5%.
3.3.2.2 Atmospheric pressure
In atmospheric pressure applications, absolute pressure measurements are used. This type of pressure relates pressure values to a vacuum.
A few types of pressure sensors uses capacitive and piezoresistive sensing modality. The capacitive pressure sensor has a flexible membrane which will deflect depending on the pressure applied, affecting the capacitance. The piezoresistive pressure sensor has a thin diaphragm that changes the resistance as pressure is applied to the diaphragm23.
The MPX4115A is an absolute, piezoresistive air pressure sensor24. It operates within a temperature range of −40°C-125°C and measures a pressure within the range of 15–115 kPa with an accuracy of ±1.5% (from 0°C to 85°C).
The BMP180 pressure sensor also uses piezoresistive technology25. It operates within a temperature range of −40°C to 85°C and measures a pressure within the range of 30–110 kPa with an accuracy of 12 Pa.
3.3.2.3 Light intensity
There are a few ways to measure light including the total energy of incoming light (short wave radiation) and photosynthetically active radiation (PAR) (van Iersel et al., 2013). Short wave radiation measures most of the spectrum of radiation from the sun whereas PAR only measures wavelengths ranging from 400 to 700 nm which is the spectrum range used by plants for photosynthesis (van Iersel et al., 2013). Short wave radiation measures solar radiation flux density using a pyranometer, and PAR measures photon irradiance using quantum sensors (van Iersel et al., 2013).
The light sensor BH175FVI is a digital ambient light sensor integrated circuit using an I2C bus26. It is a photodetector that measures the ambient light present and has a measurement variation of ±20% and an operating temperature range of -40°C–85°C. Although the authors in (Zhang et al., 2017) used this sensor for agricultural purposes, it is typically used for adjusting LCD screens and keypad back light not in the electronics industry.
3.3.2.4 Rain
Different types of information can be measured in relation to rainfall such as the presence of rain or the amount of rainfall within a period of time (van Iersel et al., 2013). The amount of rain fallen for a certain duration can be determined with a rain gauge (van Iersel et al., 2013). A version of this is a tipping bucket rain gauge. This involves rain being collected and funneled into a lever-like container. When one side fills, it tips to the other side draining all of the water it has collected and activating a switch (van Iersel et al., 2013; Tabada and Loretero, 2019). The number of times the switch is activated can be counted to calculate the amount of rainfall (van Iersel et al., 2013; Tabada and Loretero, 2019).
In general, a rain sensor detects if it is raining by measuring the resistance of the sensing board. The sensing board has copper tracks on the surface that works as a potentiometer where the resistance will depend on the water on the surface27. The rain sensor used in (Patokar and Gohokar, 2018) uses this type of rain sensing board connected to a control circuit board with a comparator that can adjust the sensitivity using a potentiometer. The main limitation of this sensor is that it is only sensing if it is currently raining instead of the amount of rain that has fallen, arguably a more important measurement in agricultural settings.
3.3.2.5 Wind
Wind affects evapotranspiration in plants and can cause soil erosion and animal mortality (van Iersel et al., 2013; Perks et al., 2018). Wind is typically measured using anemometers including cup anemometers, hot-wire anemometers, and ultrasonic anemometers (Wu et al., 2021). In cup anemometers, the wind rotates the cups at a rate proportional to the wind speed28. An example of a cup anemometer can be seen in Figure 3Ei Hot-wire anemometers use a thin, electrically heated wire which cools down as a result of the wind (Morris and Langari, 2012). The cooling effect reduces the resistance allowing the wind speed to be determined (Morris and Langari, 2012). These are not typically used in high wind areas as the thin wire could break (Morris and Langari, 2012; van Iersel et al., 2013). Ultrasonic anemometers use the property that airspeed affects the speed of an ultrasonic signal to get from one transducer to another (van Iersel et al., 2013). As these sensors have no moving or fragile parts, they tend to be used in areas of extreme weather conditions. An example of one of these sensors can be seen in Figure 3Eii.
3.3.3 Plant phenotypes
Plant phenotypes refer to observable characteristics in plants such as height and leaf area (Walter et al., 2015). A plant phenotype is determined by the environment and its genotype, or genetic background (Walter et al., 2015). Currently most plant phenotyping is done with imaging and remote sensing however, the advances made in computer vision and machine learning techniques have started being applied to plant phenotyping (Walter et al., 2015; Li et al., 2020). In (Li et al., 2020) the authors provide an overview of current techniques for image-based plant phenotyping. Moreover, there is research into developing smart plant wearables that can monitor plant health at a finer resolution than with remote sensing (Yin et al., 2021). Plant wearables can monitor the microclimate as well as plant measurements such as water potential, strain of plant tissue, and volatile organic compounds (Nassar et al., 2018; Yin et al., 2021). Plant growth is one of the measurements that can be obtained with these wearables using strain sensors based on piezoresistive, piezoelectric, and capacitive effects (Nassar et al., 2018; Lee et al., 2021)
Not very many papers studied in this review article provide information regarding plant measurements. Those that did use CMOS sensors and image processing techniques. In (Patokar and Gohokar, 2018) plant growth is monitored by taking images after specified time intervals. The authors in (Zhang et al., 2017) calculated the function leaf angle and chlorophyll concentration to judge if a plant is in good health. Function leaf angle measures leaf movement (Zhang et al., 2017). In a weak plant the function leaf angle is less than in a healthy plant (Zhang et al., 2017). Chlorophyll concentration also gives an indication to a plant’s health where higher chlorophyll concentration is correlated to a healthier plant (Zhang et al., 2017).
3.4 Discussion
Precision agriculture has been able to give farmers the necessary information to make informed management decisions on their farms based on spatial and temporal data. This allows them to make an efficient use of resources without affecting yield. However, most of these practices are concerned with monocropping. Although reducing the amount of farm input does positively affect the environment, there is more that can be done to sustain the growing population while protecting the environment.
Current practices in precision agriculture show the potential for efficient farming. This can be exploited in other farming practices, and land management uses, such as in agroforestry. In addition, there is a need for better methods for soil nutrient sensing. These measurements could provide farmers with important information on fertilizer use and prevent the negative effects of excess fertilizers.
4 Precision livestock farming
By 2050, the use of animal products is expected to double (Schillings et al., 2021). The increasing population and decreasing number of farmers puts a strain on animal farming resulting in larger herd sizes and challenges in herd management (Berckmans, 2014; Berckmans, 2017; Schillings et al., 2021). The larger herd sizes raises concerns over disease transfer from animals to humans (Berckmans, 2014). Moreover, livestock farming has a high environmental impact attributing over 92% of ammonia emissions (Berckmans, 2014). There are also growing concerns over animal welfare as demonstrated by the Animal Health Law in the European Union adopted in 201629 (Berckmans, 2017; Aquilani et al., 2022).
Precision livestock farming (PLF) aims to assist in the management of livestock through the use of real-time, continuous monitoring of animal health, welfare, production and reproduction, and environmental impacts (Berckmans, 2017). Through the use of real-time and continuous monitoring, PLF can detect small yet significant changes in animal behaviour and warn the farmer when something goes wrong allowing for early action (Berckmans, 2017; Aquilani et al., 2022). PLF can assist in increasing productivity with less inputs (Berckmans, 2017). Furthermore, the continuous and real-time monitoring can provide customers a guarantee of food safety and quality along the supply chain (Berckmans, 2014, 2017).
This section takes a look at sensors used in precision livestock farming, their advantages and disadvantages, and what they have to offer data-driven agroforestry.
4.1 PLF measurements
There are several attributes that can be measured to assist in the management of livestock. Based on the recently published review (Aquilani et al., 2022) these are summarized here.
• Animal identification
• Body weight measurements
• Animal location
• Detecting animal behaviours
• Virtual fencing
• Reproductive monitoring
There are several methods of animal identification that have been recently reviewed in (Aquilani et al., 2022). Electrical identification systems typically use radio frequency identification tags (RFID) which transmit information to a tag reader (Aquilani et al., 2022). Examples of information that can be stored include animal growth, medication, drinking or eating habits and so on (Aquilani et al., 2022). RFID tags can be grouped into boluses, ear tags, and injectable glass tags, although ear tags are the most widely used (Aquilani et al., 2022).
Body weight measurements are necessary in livestock farming to determine production and stocking rates, for instance (Wangchuk et al., 2018). The commonly used weighing scale, although accurate, is time-consuming, costly and stressful to the animals (Wangchuk et al., 2018). Walk-over-weigh platforms can be placed in restricted entry points, for instance for feed, water, or milking (Dickinson et al., 2013; Aquilani et al., 2022). These platforms combined with RFID technology can allow for identifying the animal and recording their weight with no unnecessary animal handling, reducing animal stress (Dickinson et al., 2013; Aquilani et al., 2022). Apart from walk-over-weigh platforms, image analysis can also be used to estimate body weight (Aquilani et al., 2022). However, these techniques have mostly been tested in indoor settings (Aquilani et al., 2022).
Real-time and continuous information on animal location can give the farmer instant information about where every animal in the herd is located. This is typically done using GPS tracking or with top-view cameras (Aquilani et al., 2022). The use of GPS may not always be realistic due to their current high cost especially if they are needed for each animal; however, there is work in reducing the cost of these (Aquilani et al., 2022). In addition, there is the possibility of having some animals fitted with Bluetooth devices instead of GPS, thus reducing the overall cost (Aquilani et al., 2022). Furthermore, GPS tracking is limited by its battery lifespan making it unsuitable for animals not often handled by humans (Aquilani et al., 2022). There is work in this area to use solar panels, or kinetic energy to power the GPS tracker. Another limitation of using GPS for animal tracking, is the loss of reception that can occur due to topography or weather (Aquilani et al., 2022). To work around these limitations, top-view cameras can also be used alongside image processing techniques to monitor the herd (Aquilani et al., 2022). However, it has been noted that animal size and topography affect sensitivity and precision (Aquilani et al., 2022). The use of top-view cameras significantly lowers the cost compared to the cost of the use of GPS tracking (Aquilani et al., 2022).
Animal welfare can be determined through the understanding of animal behaviour, which includes grazing behaviour, animal-to-animal interaction, or jaw movements for feed or drink intake (Schillings et al., 2021; Aquilani et al., 2022). Sensors for detecting animal behaviour can include GPS, accelerometers, inertial measurement units (IMU), pressure sensors, and acoustic sensors (Aquilani et al., 2022).
Virtual fencing is used for controlled grazing (Aquilani et al., 2022). Farmers can make and change boundaries where the animals can graze replacing the need for physical fencing allowing greater flexibility to the farmer in choosing grazing areas (Aquilani et al., 2022). Virtual fences can also reduce labour, improve management of the herd, and enhance the protection of environmentally-sensitive areas (Campbell et al., 2019). When animals approach a boundary their GPS mounted collar plays a sound to let the know they should stop, if ignored the animal is given an electric shock (Aquilani et al., 2022). There is concern over animal welfare regarding the electric shock, however using only audio cues is not as effective (Aquilani et al., 2022).
PLF can also be used for reproductive monitoring by alerting the farmer when an animal is ready to reproduce or has started parturition (Aquilani et al., 2022). The need for remote oestrus detection is based on the breeding management of the farm (Aquilani et al., 2022). For planned breeding, real-time sensing of the oestrus cycle is necessary (Aquilani et al., 2022). Typically done in the past with pedometers and accelerometers, recent work has been done using wireless intravaginal probes for cattle measuring conductivity, temperature, movement, and position. Parturition can be important to detect especially if quick intervention is needed (Aquilani et al., 2022). This can be detected in sheep using GPS and accelerometers placed on the tail of animals for identifying behaviour changes such as walking speed and direction.
In (Aquilani et al., 2022), the authors provide information about the commercially available solutions for monitoring and managing livestock, and for studying behaviour.
4.2 Discussion
Advantages of PLF include the ease of managing and monitoring a big herd. This is especially useful in pasture based systems where the farmer’s contact with the animal tends to be less frequent (Aquilani et al., 2022). The ability for an automatic, continuous, and real-time monitoring will become increasingly important as herd sizes increase in the future (Berckmans, 2017; Aquilani et al., 2022). In addition, the continuous monitoring of individual animals using wearable sensors, for example, allows for the understanding the animal’s baseline behaviour. Small changes in behaviour that may not be obvious to an observer can allow for early detection of diseases or injuries and allow for early intervention (Schillings et al., 2021). This can minimize the spread of diseases and minimise painful experiences for animals thus increasing animal welfare (Schillings et al., 2021).
Generally, PLF will decrease the amount of contact the farmer has with the animal. This lack of positive contact can affect the human-animal relationship affecting productivity and animal welfare (Schillings et al., 2021). Furthermore, PLF suffers the same limitations as precision agriculture in terms of the limitation of its technology, such as cost and battery life span (Aquilani et al., 2022).
In conclusion, precision livestock farming has the ability to support management decisions and early interventions through the use of real-time, continuous monitoring of livestock affecting animal welfare, production and reproduction rates, and the environmental impact of livestock farming. Similarly to precision agriculture, PLF can also give farmers the tools to increase production while reducing inputs by understanding the animal’s needs.
Both precision agriculture and precision livestock farming rely on the use of sensors and wireless sensor networks to manage plants and livestock through real-time monitoring techniques. The techniques used in PLF, just like for PA, can be exploited for other agricultural practices, such as in silvopastoral agroforestry systems.
5 Principles of permaculture
The term permaculture was coined in the mid 1970s by Bill Mollinson and David Holmgren (Holmgren, 2002). Originally defined as a method of sustainable, or permanent, agriculture, permaculture has since evolved to a holistic design process for complex systems and design of social systems (Holmgren, 2002; Krebs and Bach, 2018). Permaculture is now defined as “consciously designed landscapes which mimic the patterns and relationships found in nature, while yielding an abundance of food, fibre and energy for provision of local needs” (Holmgren, 2002). It conceptualises the design of sustainable socio-ecological land use systems, recognizing that they are interlinked with social systems (Holmgren, 2002; Krebs and Bach, 2018). Three basic ethical design principles are used to guide and provide an organisational framework (Holmgren, 2002):
• Care for the earth (soil, biodiversity, living things)
• Care for people (care for self and others)
• Limit to consumption and reproduction, and redistribute surplus.
The foundations of these principles are inspired by the observation of natural processes (Hirschfeld and Acker, 2021). The performance of crops or livestock in terms of yield and nutrition is directly related to the environment and any intervening inputs (Hirschfeld and Acker, 2021). Industrialized agriculture has a short-term gain in efficiency, but the regular use of synthetic fertilizers and pesticides compromise environmental integrity impeding the longevity of these systems (Hirschfeld and Acker, 2021). In contrast as a result of its design principles, permaculture emphasizes crop diversity, the use of perennial and annual crops, and organic or chemical-free management of the soil and plants (Krebs and Bach, 2018; Hirschfeld and Acker, 2021).
5.1 Permaculture and agroforestry
As a result of the permaculture design principles, permaculture utilises a mix of crops with perennials (Krebs and Bach, 2018). However, permaculture also encapsulates a wider concept: the relationship of mankind with nature, and the design and redesign of all natural resource management systems, so that they support the health and well-being of all present and future generations (Holmgren, 2002). That being said, agroforestry can help meet the needs of the permaculture design principles (Krebs and Bach, 2018).
5.2 Permaculture and precision farming
Limited research has been carried out concerning the use of sensor technology for permaculture. The authors of (Ismail and Seddik, 2014) developed and tested a low cost and low power WSN in a laboratory environment. They did not propose sensors to measure certain properties for a given goal or output of the permaculture system. Moreover, the authors describe permaculture as sustainable farming instead of viewing it as a more holistic approach.
Although not using in situ sensing, the authors in (Flores et al., 2020) demonstrate the capability of using an unmanned aerial vehicle (UAV) in permaculture to monitor biodiversity, system component interactions, land cover and land use, and landscape heterogeneity over time using aerial photography. This could potentially to be a good starting point for more advanced sensing techniques.
6 Data-driven agroforestry
Section 2 demonstrated the lack of real-time spatial and temporal data in agroforestry systems. Sections 3, 4 presented the potential of precision agriculture and precision livestock farming in providing data to allow farmers the ability to better manage their farm. An overview of the holistic approach offered by permaculture was provided in Section 5.
This review article proposes a new field of research called Data-Driven Agroforestry (DDA) which combines the sustainable and holistic aspect of permaculture with the data-driven approach used in PA and PLF so that these principles can be applied to agroforestry systems. This approach can allow farmers to harness the interactions in an agroforestry system to their benefit while using a sustainable agricultural method that will provide numerous benefits to their crops, trees, and livestock. Figure 4 provides a visual representation of data-driven agroforestry, and its overlap with precision agriculture, precision livestock farming, permaculture, and agroforestry.
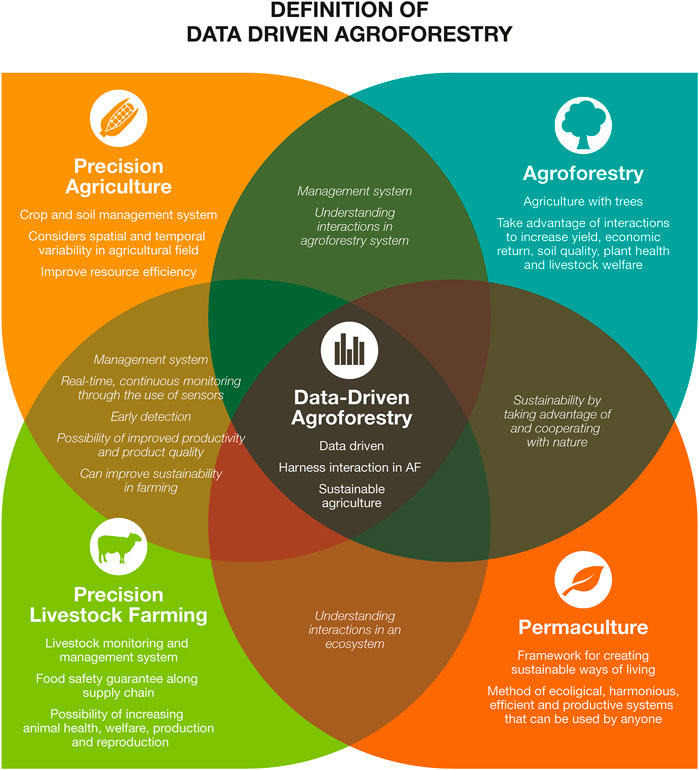
FIGURE 4. Visual representation of Data-Driven Agroforestry and its relationship with Precision Agriculture, Precision Livestock Farming, Permaculture, and Agroforestry.
6.1 Measurand suggestions
Based on the information collated in Sections 2, 3, 4, Figure 5 gives a representation of the possible measurands for soil, plants, productivity, microclimate, biodiversity, and animal welfare. Details of the additional measurands needed in data-driven agroforestry are provided in the relevant sub-sections.
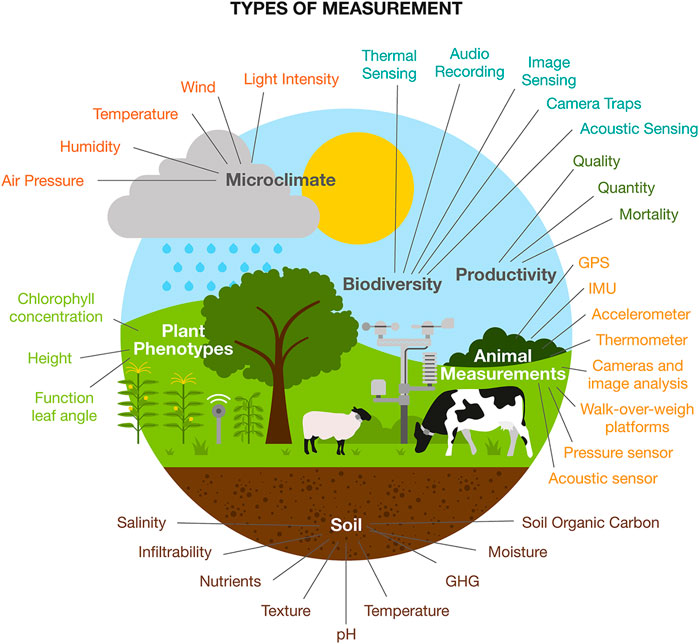
FIGURE 5. Pictorial representation of the various performance metrics and the contribution of the measurands to quantify these performance metrics.
6.1.1 Productivity
Productivity deals with the economic profit that could be generated by the system including crop, tree, livestock yield, and decrease of mortality. Quality of the final product can help determine the economic return. This performance metric uses the raw measurements obtained by the sensors and interpret them in the light of the local socio-economic conditions of the agroforestry system.
6.1.2 Biodiversity
Measuring insects and animals can determine variations of biodiversity in an agroforestry system. In addition, the determination of quantification of pests can allow for better management of pesticides (Lima et al., 2020). Such systems include thermal, image, and acoustic sensing (Shafi et al., 2019; Lima et al., 2020). Further sensing and detection of other animal and insects can be done with camera traps and audio recording devices (Muller-Karger et al., 2018).
6.1.3 Microclimate
Microclimate sensing includes environmental humidity, temperature, atmospheric pressure, light intensity, and wind speed. As the review for precision agriculture shows, most of these sensors are already widely used and could be readily applied in DDA systems.
6.1.4 Animal measurements
The change in microclimate in agroforestry systems due to the addition of trees, can offer protection to livestock from the weather (Perks et al., 2018). This can increase animal comfort thus improving animal welfare and productivity (Lemes et al., 2021). The addition of trees encourages natural animal behaviour like foraging and provides an alternate food sources for livestock, reducing the cost for feeding and the emissions that come with producing animal feed (Perks et al., 2018; Augère-Granie, 2020). Furthermore, trees can remove ammonia and nitrate from the environment thus potentially reducing the overall environmental impact of livestock farming (Perks et al., 2018).
As discussed in Section 4, animal monitoring methods include the use of GPS, Bluetooth, RFID tags, accelerometers, pressure and acoustic sensors, and cameras and image processing techniques. These technologies can help farmers manage livestock within data-driven agroforestry systems.
6.1.5 Plant measurements
As previously mentioned, the addition of trees can alter the microclimate and create competition of resources which could have effects on the crops or plants (Rao et al., 1997; Hislop and Claridge, 2000). Monitoring plants and crops can help identify and rectify any issues as they arise thus allowing for better the management of the agroforestry system. Section 3.3.3 discusses some methods of measurements for understanding a plant’s health in precision agriculture which could benefit DDA.
6.1.6 Soil measurands
Environmental measurands in agroforestry systems were considered in Section 2.3.2. From the review in precision agriculture, soil measurements, such as soil moisture and soil temperature, have the potential to determine soil health and understand the interactions within the soil that lead to these environmental benefits. However, some measurands that could be useful in data-driven agroforestry did not come up in the review of sensors used in precision agriculture, possibly due to the lack of or absence of these in situ sensors. These include soil organic carbon, soil infiltration, soil nutrients and soil greenhouse gas emissions.
In (Chen et al., 2020) the authors propose measuring soil organic carbon using in situ visible near-infrared spectroscopy. They concluded that, coupled with deep learning algorithms, the sensing modality has the potential for large-scale soil organic carbon monitoring (Chen et al., 2020). In (Huang et al., 2019) the authors use a peristaltic pump, camera, and computer with a mathematical model to estimate the soil water infiltration. None of the papers studied in the review specify the pH sensors, however these are widely used (Ananthi et al., 2017).
Soil can also store and emit greenhouse gasses (GHG) including carbon dioxide (CO2), nitrous oxide (N2O), and methane (CH4) (Oertel et al., 2016; Yamulki, 2017). Some of these greenhouse gas emissions are due to aerobic production in the stems and branches by microorganisms, and through soil produced gases that are transported through the stem (Yamulki, 2017). Measurements that can affect soil GHG emissions include soil moisture, temperature, pH, UV radiation, and plant or tree species just to name a few (Oertel et al., 2016; Yamulki, 2017). Measuring the soil GHG emissions is necessary for better quantifying global greenhouse gas budgets which can benefit decisions for land management (Oertel et al., 2016; Yamulki, 2017). The review in (Oertel et al., 2016) includes a review of soil GHG measurement methods such as chamber systems, micrometeorological methods, remote sensing, airborne methods, and laboratory approaches.
Chamber methods are the most common for measuring GHG (Butterbach-Bahl et al., 2016; ISO 20951, 2019). There are two main types of chambers: open and closed (Butterbach-Bahl et al., 2016). Open chambers measure the gas concentration at the inlet and outlet and the gas flux can be calculated by taking the difference of concentrations at the two measurement points (Yu et al., 2013). There are two types of closed chambers: static and dynamic. Closed static chambers typically operate manually where samples are taken and analyzed in a laboratory (Heinemeyer and McNamara, 2011). On the other hand, closed dynamic chambers are a closed loop system that tend to be automated and in situ (Heinemeyer and McNamara, 2011; Yu et al., 2013). These methods only account for GHG emissions of the small area of the field where the measurements are taken. Furthermore, due to their inherent intrusiveness, chamber systems have the potential to alter soil and microclimate conditions affecting the measured concentrations (Görres et al., 2016). In addition, in most chamber systems, CO2 measurements tend to be underestimated due to the headspace increase during the sampling period (Heinemeyer and McNamara, 2011; Yu et al., 2013). However, these tend to be most noticeable in closed, static chambers (Heinemeyer and McNamara, 2011). On the other hand, chambers are easy to use and useful for assessing short term changes (Jalota et al., 2018).
Paper (Görres et al., 2016) compares two commercial soil GHG dynamic chamber systems the LI-8100A30 and AGPS31. The LI-8100A is an automated system for measuring CO2. It has a multiplexer allowing for either one or multiple chambers and uses an infrared gas analyzer to measure CO2 and H2O concentrations. The AGPS31 is an automatic vial collector, but was fitted with a multiplexer and gas analyzer for the study (Görres et al., 2016). Alternatively, the GT5000 Terra32 and DX401533 are gas analysers that can also be used to calculate GHG in soil. They both use Fourier transform infrared spectroscopy (FTIR) to identify gases and their concentrations.
Another method is the eddy covariance method, a micrometeorological method, which measures vertical turbulent fluxes of water, CO2, heat, CH4, and volatile organic components in the atmosphere (Jalota et al., 2018). Differently from the chamber method, this technique can measure GHG over a large area and without disturbing the soil (Jalota et al., 2018). However, it has a high operational cost and is dependent on weather conditions such as air turbulence (Jalota et al., 2018).
6.2 Discussion
Based on the reviews of precision agriculture, precision livestock farming and agroforestry, Table 9 was generated which shows the measurands, sensing modalities and examples of sensor used in precision agriculture, agroforestry, and precision livestock farming. This table shows a striking lack of knowledge transfer between these different areas. Precision agriculture and precision livestock farming have a lot to offer to provide farmers a data-driven approach to agroforesty. Taking advantage of this can fill the current gaps in agroforestry research by providing a temporal and spatial, real-time understanding of an agroforestry system. However, as noted from Table 9, there is a need for further development in areas such as measuring biodiversity, soil nutrient content and GHG emissions, and plant growth and health that could assist in gathering important information for DDA and help in the management of agroforestry systems.
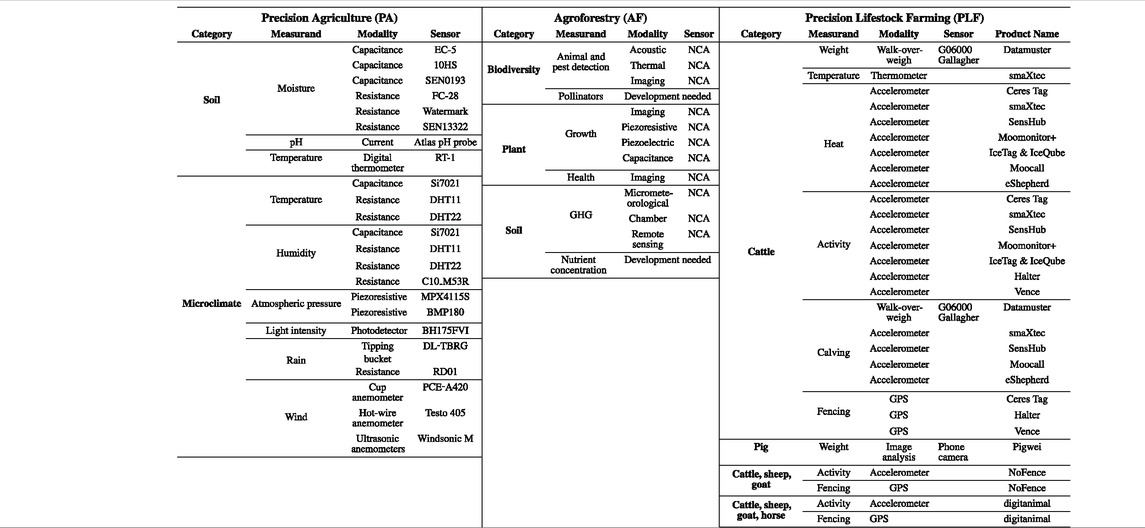
TABLE 9. Performance metrics, measurands, and sensors that could be potentially used in data-driven agroforestry. PLF based on Aquilani et al. (2022). Not commercially available (NCA).
7 Conclusion and future work
This paper presented an up-to-date review of agroforestry and precision agriculture. Table 9 identifies gaps in the current research. Firstly, there is a need for in situ real-time, autonomous sensing of soil nutrients and soil GHG. Current methods for these measurements are destructive and expensive, thus are unsuitable for data-driven agroforestry. In addition, there is the need for commercialised plant growth and health sensors, as well as for biodiversity sensing. Overall, most measurements for agroforestry rely on laboratory analysis of samples. From the review of AF, there were limited occurrences of measurements with sensors.
The review for precision agriculture gave an overview of the sensors used in wireless sensor networks in agriculture and their use for specific performance metrics. This information helped come up with the proposed measurements and sensors that could be used in data-driven agroforestry. Similarly, the precision livestock farming section gave information about the types of attributes measured and the method of measurement in PLF. Next, an overview of permaculture was presented and compared to precision farming and agroforestry. Lastly, data-driven agroforestry was presented. This method of agriculture combines PA and PLF practices with agroforestry providing an understanding of the real-time interactions that occur within agroforestry. Based on the agroforestry, PA, and PLF reviews, proposed measurements and sensors were identified that could be used in data-driven agroforestry.
Data-driven agroforestry has the potential to help farmers harness the interactions occurring between the different components of the system for a multitude of benefits such as increased yield, environmental benefits, and animal welfare. In addition, it can promote a more sustainable agricultural method that can eventually sustain population growth as witnessed today.
Author contributions
EB, MD, and JH contributed to the conception of this work. EB conducted the review and prepared the manuscript under the supervision of MD, JH, KB, and DA. All authors contributed to manuscript revision, read, and approved the submitted version.
Acknowledgments
EB would like to acknowledge and thank the financial contribution provided for her PhD under the Doctoral Training Programme (DTP) by the Engineering and Physical Sciences research Council (EPSRC) of the United Kingdom Research and Innovation (UKRI). Permissions for image use was provided by the following companies: Meter provided Figures 3Ai,B, SparkFun Figure 3Aii, Atlas Scientific Figure 3C, Aosong Electronic Figure 3D, PCE Instruments Figure 3Ei, and RPR Figure 3Eii.
Conflict of interest
The authors declare that the research was conducted in the absence of any commercial or financial relationships that could be construed as a potential conflict of interest.
Publisher’s note
All claims expressed in this article are solely those of the authors and do not necessarily represent those of their affiliated organizations, or those of the publisher, the editors and the reviewers. Any product that may be evaluated in this article, or claim that may be made by its manufacturer, is not guaranteed or endorsed by the publisher.
Footnotes
1https://www.worldagroforestry.org/about/agroforestry
2https://www.worldagroforestry.org/about/history
3https://www.ispag.org/about/definition
4https://www.ispag.org/about/definition
5https://www.fao.org/soils-portal/about/en/
6https://naylampmechatronics.com/sensores-temperatura-y-humedad/47-sensor-de-humedad-de-suelo-fc-28.html
7https://www.sparkfun.com/products/13322
8https://irrometer.com/sensors.html
9https://www.metergroup.com/environment/products/ec-5-soil-moisture-sensor/
10https://wiki.dfrobot.com/Capacitive_Soil_Moisture_Sensor_SKU_SEN0193
11http://www.labcell.com/media/24207/10hs-manual.pdf
12https://datasheets.maximintegrated.com/en/ds/DS18B20.pdf
13https://www.metergroup.com/en/meter-environment/products/rt-1-soil-temperature-sensor
15https://www.bruker.com/en/products-and-solutions/elemental-analyzers/handheld-xrf-spectrometers/S1-TITAN.html
16https://atlas-scientific.com/probes/consumer-grade-ph-probe/
17https://www.ametherm.com/blog/thermistors/temperature-sensor-types
18https://www.linquip.com/blog/types-of-humidity-sensors/
19http://www.aosong.com/en/products-21.html
20http://www.aosong.com/en/products-22.html
21https://cdn-learn.adafruit.com/assets/assets/000/035/931/original/Support_Documents_TechnicalDocs_Si7021-A20.pdf
22http://c1170156.r56.cf3.rackcdn.com/UK_SHN_C10-M53R_DS.pdf
23https://www.avnet.com/wps/portal/abacus/solutions/technologies/sensors/pressure-sensors/types
24https://www.nxp.com/docs/en/data-sheet/MPXAZ4115A.pdf
25https://cdn-shop.adafruit.com/datasheets/BST-BMP180-DS000-09.pdf
26https://www.mouser.com/datasheet/2/348/bh1750fvi-e-186247.pdf
27https://www.watelectronics.com/rain-sensor/
28https://www.metoffice.gov.uk/weather/guides/observations/how-we-measure-wind
29https://ec.europa.eu/food/animals/animal-health/animal-health-law_en
30https://licor.app.boxenterprise.net/s/jtpq4vg358reu4c8r4id
31https://www.uit-gmbh.de/en/product-details__762/getProdInfos_-_1795/
32https://www.gasmet.com/wp-content/uploads/2019/09/Gasmet-GT5000-Terra-Technical-Datasheet-ID-10233.pdf
33https://www.gasmet.com/uk/wp-content/uploads/sites/10/2018/03/Gasmet-DX4015-Technical-Data-ID-7090.pdf
References
Abagissa, A. T., Behura, A., and Pani, S. K. (2018). “Iot based smart agricultural device controlling system,” in 2018 Second International Conference on Inventive Communication and Computational Technologies (ICICCT) (IEEE), 26–30.
Alaux, P.-L., Zhang, Y., Gilbert, L., and Johnson, D. (2021). Can common mycorrhizal fungal networks be managed to enhance ecosystem functionality? Plants, People, Planet 3, 433–444. doi:10.1002/ppp3.10178
Ananthi, N., Divya, J., Divya, M., and Janani, V. (2017). “Iot based smart soil monitoring system for agricultural production,” in 2017 IEEE Technological Innovations in ICT for Agriculture and Rural Development (TIAR) (IEEE), 209–214.
Aquilani, C., Confessore, A., Bozzi, R., Sirtori, F., and Pugliese, C. (2022). Review: Precision livestock farming technologies in pasture-based livestock systems. Animal 16, 100429. doi:10.1016/j.animal.2021.100429
Barapatre, P., and Patel, J. N. (2019). Determination of soil moisture using various sensors for irrigation water management. Int. J. Innovative Technol. Explor. Eng. (IJITEE) 8, 576–582.
Bargués Tobella, A., Reese, H., Almaw, A., Bayala, J., Malmer, A., Laudon, H., et al. (2014). The effect of trees on preferential flow and soil infiltrability in an agroforestry parkland in semiarid Burkina Faso. Water Resour. Res. 50, 3342–3354. doi:10.1002/2013wr015197
Battie-Laclau, P., Taschen, E., Plassard, C., Dezette, D., Abadie, J., Arnal, D., et al. (2020). Role of trees and herbaceous vegetation beneath trees in maintaining arbuscular mycorrhizal communities in temperate alley cropping systems. Plant Soil 453, 153–171. doi:10.1007/s11104-019-04181-z
Bayala, J., and Prieto, I. (2020). Water acquisition, sharing and redistribution by roots: Applications to agroforestry systems. Plant Soil 453, 17–28. doi:10.1007/s11104-019-04173-z
Beckert, M. R., Smith, P., Lilly, A., and Chapman, S. J. (2016). Soil and tree biomass carbon sequestration potential of silvopastoral and woodland-pasture systems in north east scotland. Agroforest. Syst. 90, 371–383. doi:10.1007/s10457-015-9860-4
Berckmans, D. (2017). General introduction to precision livestock farming. Anim. Front. 7, 6–11. doi:10.2527/af.2017.0102
Berckmans, D. (2014). Precision livestock farming technologies for welfare management in intensive livestock systems. Rev. Sci. Tech. OIE. 33, 189–196. doi:10.20506/rst.33.1.2273
Bergez, J.-É., Dalziel, A., Duller, C., Eason, W., Hoppe, G., and Lavender, R. (1997). Light modification in a developing silvopastoral system in the UK: A quantitative analysis. Agrofor. Syst. 37, 227–240. doi:10.1023/a:1005916925339
Bongiovanni, R., and Lowenberg-DeBoer, J. (2004). Precision agriculture and sustainability. Precis. Agric. 5, 359–387. doi:10.1023/b:prag.0000040806.39604.aa
Burton, L., Dave, N., Fernandez, R. E., Jayachandran, K., and Bhansali, S. (2018). Smart gardening iot soil sheets for real-time nutrient analysis. J. Electrochem. Soc. 165, B3157–B3162. doi:10.1149/2.0201808jes
Burton, L., Jayachandran, K., and Bhansali, S. (2020). Review—the “real-time” revolution for in situ soil nutrient sensing. J. Electrochem. Soc. 167, 037569. doi:10.1149/1945-7111/ab6f5d
Butterbach-Bahl, K., Sander, B. O., Pelster, D., and Díaz-Pinés, E. (2016). “Quantifying greenhouse gas emissions from managed and natural soils,” in Methods for measuring greenhouse gas balances and evaluating mitigation options in smallholder agriculture (Cham: Springer), 71–96.
Cabaccan, C. N., Cruz, F. R. G., and Agulto, I. C. (2017). “Wireless sensor network for agricultural environment using raspberry pi based sensor nodes,” in 2017IEEE 9th International Conference on Humanoid, Nanotechnology, Information Technology, Communication and Control, Environment and Management (HNICEM IEEE), 1–5.
Campbell, D. L. M., Lea, J. M., Keshavarzi, H., and Lee, C. (2019). Virtual fencing is comparable to electric tape fencing for cattle behavior and welfare. Front. Vet. Sci. 6, 445. doi:10.3389/fvets.2019.00445
Chen, S., Xu, D., Li, S., Ji, W., Yang, M., Zhou, Y., et al. (2020). Monitoring soil organic carbon in alpine soils using in situ vis-nir spectroscopy and a multilayer perceptron. Land Degrad. Dev. 31, 1026–1038. doi:10.1002/ldr.3497
Dexter, A. R. (2004). Soil physical quality: Part i. theory, effects of soil texture, density, and organic matter, and effects on root growth. Geoderma 120, 201–214. doi:10.1016/j.geoderma.2003.09.004
Dickinson, R., Morton, J., Beggs, D., Anderson, G., Pyman, M., Mansell, P., et al. (2013). An automated walk-over weighing system as a tool for measuring liveweight change in lactating dairy cows. J. Dairy Sci. 96, 4477–4486. doi:10.3168/jds.2012-6522
Dursun, M., and Ozden, S. (2011). A wireless application of drip irrigation automation supported by soil moisture sensors. Sci. Res. Essays 6, 1573–1582.
Enciso, J., Porter, D., and Peries, X. (2007). Irrigation monitoring with soil water sensors (Spanish). Texas: Texas FARMER Collection.
Fahmi, N., Huda, S., Prayitno, E., Al Rasyid, M. U. H., Roziqin, M. C., and Pamenang, M. U. (2017). “A prototype of monitoring precision agriculture system based on wsn,” in 2017 International Seminar on Intelligent Technology and Its Applications (ISITIA) (IEEE), 323–328.
Ferrante, A., and Mariani, L. (2018). Agronomic management for enhancing plant tolerance to abiotic stresses: High and low values of temperature, light intensity, and relative humidity. Horticulturae 4, 21. doi:10.3390/horticulturae4030021
Flores, J. J. M., Bagunu, A. K., and Buot, I. E. (2020). Documenting permaculture farm landscapes in the Philippines using a drone with a smartphone. Methodol. Support. Sustain. Dev. Agric. Nat. Resour. Manag. 71.
Foughali, K., Fathallah, K., and Frihida, A. (2018). Using cloud iot for disease prevention in precision agriculture. Procedia Comput. Sci. 130, 575–582. doi:10.1016/j.procs.2018.04.106
Golicz, K., Hallett, S. H., Sakrabani, R., and Pan, G. (2019). The potential for using smartphones as portable soil nutrient analyzers on suburban farms in central east China. Sci. Rep. 9, 1–10. doi:10.1038/s41598-019-52702-8
Görres, C.-M., Kammann, C., and Ceulemans, R. (2016). Automation of soil flux chamber measurements: Potentials and pitfalls. Biogeosciences 13, 1949–1966. doi:10.5194/bg-13-1949-2016
Gresl, J., Fazackerley, S., and Lawrence, R. (2021). Practical precision agriculture with lora based wireless sensor networks.
Grimblatt, V., Ferré, G., Rivet, F., Jego, C., and Vergara, N. (2019). “Precision agriculture for small to medium size farmers—An iot approach,” in 2019 IEEE International Symposium on Circuits and Systems (ISCAS) (IEEE), 1–5. doi:10.1109/ISCAS.2019.8702563
Haule, J., and Michael, K. (2014). “Deployment of wireless sensor networks (wsn) in automated irrigation management and scheduling systems: A review,” in Proceedings of the 2nd Pan African international conference on science, computing and telecommunications (PACT 2014) (IEEE), 86–91.
Heinemeyer, A., and McNamara, N. P. (2011). Comparing the closed static versus the closed dynamic chamber flux methodology: Implications for soil respiration studies. Plant Soil 346, 145–151. doi:10.1007/s11104-011-0804-0
Hirschfeld, S., and Acker, R. V. (2021). Review: Ecosystem services in permaculture systems. Agroecol. Sustain. Food Syst. 45, 794–816. doi:10.1080/21683565.2021.1881862
Holmgren, D. (2002). Permaculture: Principles & pathways beyond sustainability. Hepburn, Victoria: Holmgren Design Services).
Huang, Z., Sun, L., Liu, Y., Liu, Y.-F., López-Vicente, M., Wei, X.-H., et al. (2019). Alfalfa planting significantly improved alpine soil water infiltrability in the qinghai-Tibetan plateau. Agric. Ecosyst. Environ. 285, 106606. doi:10.1016/j.agee.2019.106606
Ismail, A., and Seddik, K. (2014). “The design and implementation of a constrained wsn for permaculture farming in Egypt,” in Proceedings of the 2014 IEEE Emerging Technology and Factory Automation (Barcelona: ETFA), 1–8. doi:10.1109/ETFA.2014.7005166
ISO 20951 (2019). Soil Quality — guidance on methods for measuring greenhouse gases (CO2, N2O, CH4) and ammonia (NH3) fluxes between soils and the atmosphere. Geneva, Switzerland: Standard, ISO.
Jalota, S., Vashisht, B., Sharma, S., and Kaur, S. (2018). “Chapter 1 - emission of greenhouse gases and their warming effect,” in Understanding climate change impacts on crop productivity and water balance. Editors S. Jalota, B. Vashisht, S. Sharma, and S. Kaur (Academic Press), 1–53. doi:10.1016/B978-0-12-809520-1.00001-X
Jawad, H. M., Nordin, R., Gharghan, S. K., Jawad, A. M., and Ismail, M. (2017). Energy-efficient wireless sensor networks for precision agriculture: A review. Sensors 17, 1781. doi:10.3390/s17081781
Kashyap, B., and Kumar, R. (2021). Sensing methodologies in agriculture for soil moisture and nutrient monitoring. IEEE Access 9, 14095–14121. doi:10.1109/ACCESS.2021.3052478
Keswani, B., Mohapatra, A. G., Mohanty, A., Khanna, A., Rodrigues, J. J., Gupta, D., et al. (2019). Adapting weather conditions based iot enabled smart irrigation technique in precision agriculture mechanisms. Neural comput. Appl. 31, 277–292. doi:10.1007/s00521-018-3737-1
Khan, N., Jhariya, M. K., Raj, A., Banerjee, A., Meena, R. S., Bargali, S. S., et al. (2021). Agroforestry and its services for soil management and sustainability. Singapore: Springer Singapore, 353–377. doi:10.1007/978-981-16-3207-5_11
Kiani, F., and Seyyedabbasi, A. (2018). Wireless sensor network and internet of things in precision agriculture.
Krebs, J., and Bach, S. (2018). Permaculture—Scientific evidence of principles for the agroecological design of farming systems. Sustainability 10, 3218. doi:10.3390/su10093218
Kumar, S. A., and Ilango, P. (2018). The impact of wireless sensor network in the field of precision agriculture: A review. Wirel. Pers. Commun. 98, 685–698. doi:10.1007/s11277-017-4890-z
Lee, G., Wei, Q., and Zhu, Y. (2021). Emerging wearable sensors for plant health monitoring. Adv. Funct. Mat. 31, 2106475. doi:10.1002/adfm.202106475
Lehmann, L. M., Smith, J., Westaway, S., Pisanelli, A., Russo, G., Borek, R., et al. (2020). Productivity and economic evaluation of agroforestry systems for sustainable production of food and non-food products. Sustainability 12, 5429. doi:10.3390/su12135429
Lehnert, M. (2014). Factors affecting soil temperature as limits of spatial interpretation and simulation of soil temperature. Acta Univ. Palacki. Geogr. 45, 5–21.
Lemes, A. P., Garcia, A. R., Pezzopane, J. R. M., Brandão, F. Z., Watanabe, Y. F., Cooke, R. F., et al. (2021). Silvopastoral system is an alternative to improve animal welfare and productive performance in meat production systems. Sci. Rep. 11, 1–17. doi:10.1038/s41598-021-93609-7
Li, Z., Guo, R., Li, M., Chen, Y., and Li, G. (2020). A review of computer vision technologies for plant phenotyping. Comput. Electron. Agric. 176, 105672. doi:10.1016/j.compag.2020.105672
Lima, M. C. F., de Almeida Leandro, M. E. D., Valero, C., Coronel, L. C. P., and Bazzo, C. O. G. (2020). Automatic detection and monitoring of insect pests—A review. Agriculture 10, 161. doi:10.3390/agriculture10050161
Lunka, P., and Patil, S. D. (2016). Impact of tree planting configuration and grazing restriction on canopy interception and soil hydrological properties: Implications for flood mitigation in silvopastoral systems. Hydrol. Process. 30, 945–958. doi:10.1002/hyp.10630
Mariani, L., and Ferrante, A. (2017). Agronomic management for enhancing plant tolerance to abiotic stresses—Drought, salinity, hypoxia, and lodging. Horticulturae 3, 52. doi:10.3390/horticulturae3040052
Mateo-Sagasta, J., Marjani, S., and Turral, H. (2009). Executive summary. Rome: Food and Agriculture Organization of the United Nations).Water pollution from agriculture: A global review
McAdam, J. (2018). “Silvopastoral agroforestry-an option to support sustainable grassland intensification,” in European Agroforestry Conference-Agroforestry as Sustainable Land Use, 4th (EURAF).
McCauley, A., Jones, C., and Jacobsen, J. (2009). Soil ph and organic matter. Nutr. Manag. module 8, 1–12.
Mekki, K., Bajic, E., Chaxel, F., and Meyer, F. (2019). A comparative study of lpwan technologies for large-scale iot deployment. ICT Express 5, 1–7. doi:10.1016/j.icte.2017.12.005
Messina, G., and Modica, G. (2020). Applications of uav thermal imagery in precision agriculture: State of the art and future research outlook. Remote Sens. 12, 1491. doi:10.3390/rs12091491
Morgan-Davies, C., Waterhouse, A., Pollock, M., and Holland, J. (2008). Integrating hill sheep production and newly established native woodland: Achieving sustainability through multiple land use in scotland. Int. J. Agric. Sustain. 6, 133–147. doi:10.3763/ijas.2007.0248
Morris, A. S., and Langari, R. (2012). “Chapter 16 - flow measurement,” in Measurement and instrumentation. Editors A. S. Morris, and R. Langari (Boston: Butterworth-Heinemann), 425–459. doi:10.1016/B978-0-12-381960-4.00016-4
Mosquera-Losada, M., Santiago-Freijanes, J., Rois-Díaz, M., Moreno, G., Den Herder, M., Aldrey-Vázquez, J., et al. (2018). Agroforestry in europe: A land management policy tool to combat climate change. Land Use Policy 78, 603–613. doi:10.1016/j.landusepol.2018.06.052
Mulla, D., and Khosla, R. (2016). Historical evolution and recent advances in precision farming. Soil-specific farming Precis. Agric. 1–35.
Muller-Karger, F. E., Hestir, E., Ade, C., Turpie, K., Roberts, D. A., Siegel, D., et al. (2018). Satellite sensor requirements for monitoring essential biodiversity variables of coastal ecosystems. Ecol. Appl. 28, 749–760. doi:10.1002/eap.1682
Muñoz-Huerta, R. F., Guevara-Gonzalez, R. G., Contreras-Medina, L. M., Torres-Pacheco, I., Prado-Olivarez, J., and Ocampo-Velazquez, R. V. (2013). A review of methods for sensing the nitrogen status in plants: Advantages, disadvantages and recent advances. Sensors 13, 10823–10843. doi:10.3390/s130810823
Nassar, J. M., Khan, S. M., Villalva, D. R., Nour, M. M., Almuslem, A. S., and Hussain, M. M. (2018). Compliant plant wearables for localized microclimate and plant growth monitoring. npj Flex. Electron. 2, 24–12. doi:10.1038/s41528-018-0039-8
Oertel, C., Matschullat, J., Zurba, K., Zimmermann, F., and Erasmi, S. (2016). Greenhouse gas emissions from soils—A review. Geochemistry 76, 327–352. doi:10.1016/j.chemer.2016.04.002
Ojha, T., Misra, S., and Raghuwanshi, N. S. (2015). Wireless sensor networks for agriculture: The state-of-the-art in practice and future challenges. Comput. Electron. Agric. 118, 66–84. doi:10.1016/j.compag.2015.08.011
Østergård, H., Finckh, M. R., Fontaine, L., Goldringer, I., Hoad, S. P., Kristensen, K., et al. (2009). Time for a shift in crop production: Embracing complexity through diversity at all levels. J. Sci. Food Agric. 89, 1439–1445. doi:10.1002/jsfa.3615
Pantera, A., Burgess, P. J., Losada, R. M., Moreno, G., López-Díaz, M., Corroyer, N., et al. (2018). Agroforestry for high value tree systems in europe. Agroforest. Syst. 92, 945–959. doi:10.1007/s10457-017-0181-7
Pascual, A., Rivera, R., Gómez, R., and Domínguez-Lerena, S. (2019). Monitoring water-soil dynamics and tree survival using soil sensors under a big data approach. Sensors 19, 4634. doi:10.3390/s19214634
Patokar, A. M., and Gohokar, V. V. (2018). “Precision agriculture system design using wireless sensor network,” in Information and communication technology (Springer), 169–177.
Pei, X., Sudduth, K. A., Veum, K. S., and Li, M. (2019). Improving in-situ estimation of soil profile properties using a multi-sensor probe. Sensors 19, 1011. doi:10.3390/s19051011
Perks, M., Khomik, M., Bathgate, S., Chapman, S., Slee, B., Yeluripati, J., et al. (2018). Agroforestry in scotland – potential benefits in a changing climate.
Pregitzer, K., and King, J. (2005). “Effects of soil temperature on nutrient uptake,” in Nutrient acquisition by plants (Springer), 277–310.
Rao, M., Nair, P., and Ong, C. (1997). Biophysical interactions in tropical agroforestry systems. Agrofor. Syst. 38, 3–50. doi:10.1023/a:1005971525590
Rathinam, D. D. K., Surendran, D., Shilpa, A., Grace, A. S., and Sherin, J. (2019). “Modern agriculture using wireless sensor network (wsn),” in 2019 5th International Conference on Advanced Computing & Communication Systems (ICACCS IEEE), 515–519.
Ravansari, R., Wilson, S. C., Wilson, B. R., and Tighe, M. (2021). Rapid pxrf soil organic carbon and organic matter assessment using novel modular radiation detector assembly. Geoderma 382, 114728. doi:10.1016/j.geoderma.2020.114728
Sahitya, G., Balaji, N., Naidu, C. D., and Abinaya, S. (2017). “Designing a wireless sensor network for precision agriculture using zigbee,” in 2017 IEEE 7th International Advance Computing Conference (IACC) (IEEE), 287–291.
Sanjeevi, P., Prasanna, S., Siva Kumar, B., Gunasekaran, G., Alagiri, I., and Vijay Anand, R. (2020). Precision agriculture and farming using internet of things based on wireless sensor network. Trans. Emerg. Tel. Tech. 31, e3978. doi:10.1002/ett.3978
Schillings, J., Bennett, R., and Rose, D. C. (2021). Exploring the potential of precision livestock farming technologies to help address farm animal welfare. Front. Anim. Sci. 2. doi:10.3389/fanim.2021.639678
Shafi, U., Mumtaz, R., García-Nieto, J., Hassan, S. A., Zaidi, S. A. R., and Iqbal, N. (2019). Precision agriculture techniques and practices: From considerations to applications. Sensors 19, 3796. doi:10.3390/s19173796
Sishodia, R. P., Ray, R. L., and Singh, S. K. (2020). Applications of remote sensing in precision agriculture: A review. Remote Sens. 12, 3136. doi:10.3390/rs12193136
Smith, J. (2018). “Interactions between trees, crops and animals: Experiences in a novel bioenergy-livestock system in the UK,” in European Agroforestry Conference-Agroforestry as Sustainable Land Use, 4th (EURAF).
Smith, J., Pearce, B. D., and Wolfe, M. S. (2013). Reconciling productivity with protection of the environment: Is temperate agroforestry the answer? Renew. Agric. Food Syst. 28, 80–92. doi:10.1017/S1742170511000585
Smith, M. M., Bentrup, G., Kellerman, T., MacFarland, K., Straight, R., Ameyaw, L., et al. (2022). Silvopasture in the USA: A systematic review of natural resource professional and producer-reported benefits, challenges, and management activities. Agric. Ecosyst. Environ. 326, 107818. doi:10.1016/j.agee.2021.107818
Smolka, M., Puchberger-Enengl, D., Bipoun, M., Klasa, A., Kiczkajlo, M., Śmiechowski, W., et al. (2017). A mobile lab-on-a-chip device for on-site soil nutrient analysis. Precis. Agric. 18, 152–168. doi:10.1007/s11119-016-9452-y
Steppler, H. A., and Nair, P. (1987). Agroforestry: A decade of development. Nairobi: International Council for Research in Agroforestry.
Tabada, M. T., and Loretero, M. E. (2019). Application of a low-cost water level circuit for an accurate pulse detection of a tipping-bucket rain gauge as an alternative method for reed switch sensors. Environ. Monit. Assess. 191, 294–298. doi:10.1007/s10661-019-7459-3
Tang, Y., Dananjayan, S., Hou, C., Guo, Q., Luo, S., and He, Y. (2021). A survey on the 5g network and its impact on agriculture: Challenges and opportunities. Comput. Electron. Agric. 180, 105895. doi:10.1016/j.compag.2020.105895
Teklehaimanot, Z., Jones, M., and Sinclair, F. (2002). Tree and livestock productivity in relation to tree planting configuration in a silvopastoral system in north wales, UK. Agrofor. Syst. 56, 47–55. doi:10.1023/a:1021131026092
The Woodland Trust (2017). Mob grazing and creating new hedgerows for livestock shelter in Cumbria. Lincolnshire: Woodland Trust.
Upson, M. A., Burgess, P. J., and Morison, J. (2016). Soil carbon changes after establishing woodland and agroforestry trees in a grazed pasture. Geoderma 283, 10–20. doi:10.1016/j.geoderma.2016.07.002
van Iersel, M. W., Chappell, M., and Lea-Cox, J. D. (2013). Sensors for improved efficiency of irrigation in greenhouse and nursery production. HortTechnology 23, 735–746. doi:10.21273/horttech.23.6.735
Varah, A., Jones, H., Smith, J., and Potts, S. G. (2020). Temperate agroforestry systems provide greater pollination service than monoculture. Agric. Ecosyst. Environ. 301, 107031. doi:10.1016/j.agee.2020.107031
Vaz Monteiro, M., Phillip, H., Morison, J. I., and Doick, K. J. (2019). The role of urban trees and greenspaces in reducing urban air temperatures
Walter, A., Liebisch, F., and Hund, A. (2015). Plant phenotyping: From bean weighing to image analysis. Plant methods 11, 14–11. doi:10.1186/s13007-015-0056-8
Wangchuk, K., Wangdi, J., and Mindu, M. (2018). Comparison and reliability of techniques to estimate live cattle body weight. J. Appl. Animal Res. 46, 349–352. doi:10.1080/09712119.2017.1302876
Wu, S.-C., Tzou, J.-C., and Ding, C.-Y. (2021). A low-cost system for measuring wind speed and direction using thermopile array and artificial neural network. Appl. Sci. 11, 4024. doi:10.3390/app11094024
Yin, H., Cao, Y., Marelli, B., Zeng, X., Mason, A. J., and Cao, C. (2021). Smart agriculture systems: Soil sensors and plant wearables for smart and precision agriculture (adv. Mater. 20/2021). Adv. Mat. 33, 2170156. doi:10.1002/adma.202170156
Yu, K., Hiscox, A., and DeLaune, R. D. (2013). Greenhouse gas emission by static chamber and eddy flux methods. Methods Biogeochem. Wetl. 10, 427–437. doi:10.2136/sssabookser10.c22
Zarco-Tejada, P. J., Hubbard, N., and Loudjani, P. (2014). Precision agriculture – an opportunity for eu farmers - potential support with the cap2014-2020.
Keywords: data-driven agroforestry, agroforestry, precision agriculture, precision livestock farming, sensors, wireless sensor networks
Citation: Ramil Brick ES, Holland J, Anagnostou DE, Brown K and Desmulliez MPY (2022) A review of agroforestry, precision agriculture, and precision livestock farming—The case for a data-driven agroforestry strategy. Front. Sens. 3:998928. doi: 10.3389/fsens.2022.998928
Received: 20 July 2022; Accepted: 08 August 2022;
Published: 23 September 2022.
Edited by:
Lorena Parra, Universitat Politècnica de València, SpainReviewed by:
Javier Rocher Morant, Universitat Politècnica de València, SpainManoj Kumar Jhariya, Sant Gahira Guru Vishwavidyalaya, India
Copyright © 2022 Ramil Brick, Holland, Anagnostou, Brown and Desmulliez. This is an open-access article distributed under the terms of the Creative Commons Attribution License (CC BY). The use, distribution or reproduction in other forums is permitted, provided the original author(s) and the copyright owner(s) are credited and that the original publication in this journal is cited, in accordance with accepted academic practice. No use, distribution or reproduction is permitted which does not comply with these terms.
*Correspondence: Elisa S. Ramil Brick, esr1@hw.ac.uk