- 1São Carlos Institute of Physics, University of Sao Paulo (USP), Sao Carlos, Brazil
- 2Institute of Mathematical Sciences and Computing, University of Sao Paulo, Sao Carlos, Brazil
In this paper we discuss how nanotech-based sensors and biosensors are providing the data for autonomous machines and intelligent systems, using two metaphors to exemplify the convergence between nanotechnology and artificial intelligence (AI). These are related to sensors to mimic the five human senses, and integration of data from varied sources and natures into an intelligent system to manage autonomous services, as in a train station.
Introduction
The rapid progress in autonomous systems with artificial intelligence (AI) has brought an expectation that machines and software systems will soon be able to perform intellectual tasks as efficiently as humans (perhaps even better), to the extent that in a near future, for the first time in history such systems may be generating knowledge, with no human intervention (Rodrigues et al., 2021). This tremendous achievement will only be realized if these manmade systems can acquire, process and make sense of a lot of combined data from the environment, in addition to mastering natural languages. The latter requirement appears particularly challenging since machine learning (ML) and other currently successful AI approaches are not yet sufficient to interpret text (Rodrigues et al., 2021). Another stringent requirement is in the capability of continuously acquiring information with sensors and biosensors, in many cases having to emulate human capabilities. As for shorter-term applications, medical diagnosis and any other type of diagnosis are among the topics that may benefit most from AI. This is due to a convergence with multiple technologies that are crucial for diagnosis, namely the nanotech-based methodologies which allow for ubiquitous sensing and biosensing to be integrated into diagnosis and surveillance systems (Rodrigues et al., 2016). Diagnosis is essentially a classification task, for which ML has been proven especially suited, in spite of its limitations in performing tasks that require interpretation (Buscaglia et al., 2021).
Living beings depend on sensing for their survival, growth, reproduction, and interaction. Humans, in particular, use their five senses (touch, sight, hearing, smell and taste) at all times to monitor their environment and interact with it, where most of the sensorial detection relies on pattern recognition. Through history, a range of devices and instruments have been developed to augment and assist human monitoring capability, but the field of sensing (and biosensing) became well established only in the final decades of the 20th century. By way of illustration, in a search in the Web of Science in May, 2021, for published papers containing these terms only a few dozen papers per year were found for the period from 1900 to 1950, most of which unrelated to sensor devices, but rather associated with sensorial phenomena. In the 1970s, an order of magnitude of 1,000 papers published per year was reached, and in the 1990s the annual numbers increased to 10,000 and 20,000, whereas in the last 2 years the number of papers published ranged between 140,000 and 150,000 per year. This outstanding increase in scientific production was obviously a consequence of the progress in research on novel materials, including manmade as well as natural materials adapted for sensing purposes. Though Nature provided inspiration from the early stages, particularly with sensing in animals, two reasons contributed to the field expanding as if entirely independent of human (or animal) sensing. First, real-time monitoring was unfeasible in many sensing applications, and integration of different types of sensors—e.g., to emulate the five senses—remained mostly elusive. Another significant distinction lies in the underlying detection principles: unlike the natural sensors in living beings, normally the analysis of data from manmade sensors does not rely on pattern recognition methods.
The significant progresses in analytical techniques and in sensors and biosensors as nanotech products introduce opportunities for bridging these gaps. Cheap sensors are now routinely fabricated that permit ubiquitous sensing and real-time monitoring in specific applications. Some of these sensors can be wearable and even implantable, and may be more sensitive than their corresponding sensors in humans. An archetypical example is that of an electronic tongue (e-tongue), whose sensitivity for some tastes can be as high as 10,000 times the average sensitivity value for humans (Riul Jr. et al., 2010). Sensing and biosensing employ distinct detection principles, which permits integrating different types of data (e.g., obtained with electronic tongues and electronic noses). Furthermore, computational methods are now available to process the large amounts of data generated with ubiquitous sensing and real-time monitoring, with the bonus of a substantially expanded capacity in terms of memory and processing power, compared to living beings. These methods also allow exploiting pattern recognition strategies, thus bringing the sensing tasks somewhat closer to how sensing is performed by humans. Last, but not least, sensing principles are much broader than those prevailing in living beings, which may open novel ways to fabricate intelligent systems and robots with unprecedented capabilities.
In this paper we focus on two aspects: 1) sensing systems that mimic human senses to exemplify how manmade sensors are being developed with bioinspiration; 2) sensors and biosensors aimed at integration into intelligent systems, in which a discussion will be presented of the stringent requirements that must be fulfilled for data analysis based on computational methods, especially machine learning.
Mimicking the Five Senses
Today’s technology allows for mimicking the five human senses (Guerrini et al., 2017) with the multiple methodologies discussed below and briefly illustrated with one or two examples, as it is not our purpose to review the major contributions in any of these areas. We shall also distinguish between sensing systems conceived as artificial counterparts of human organs and those simply performing similar functions, even if their shape and nature have nothing to do with the sensing organs.
Touch
Pressure and strain sensors have been developed toward creating electronic skins (Lipomi et al., 2011; Hammock et al., 2013), ionic skins (Qiu et al., 2021) or epidermal electronics (Kim et al., 2011). The terms used may vary and so do the functions performed by e-skins or ionic skins, which can go well beyond those of a human (or other animals) skin. The e-skins share nevertheless the following features: they should be flexible, stretchable, self-healing, and possess the ability to sense temperature, and wide ranges of pressures (not only touch) and strain. With recent developments in nanomaterials and self-healable polymers, it has been possible to obtain e-skins with augmented performance in comparison to their organic counterparts, especially in superior spatial resolution and thermal sensitivity (Hammock et al., 2013). Future improvements are focused on adding functionalities for specific purposes. For health applications, for instance, biosensors may be incorporated to monitor body conditions and detect diseases, while antimicrobial coatings may be employed to functionalize the e-skins to assist in wound healing (Yang et al., 2019). Within the paradigm of epidermal electronics, on the other hand, the systems envisaged may include not only sensors, but also transistors, capacitors, light-emitting diodes, photovoltaic devices and wireless coils (Kim et al., 2011). Self-powered tactile sensors produced with piezoelectric polymer nanofibers are indicative of these capabilities (Liu et al., 2021a). An example of the sensing ability of self-healing hydrogels is in detecting distinct body movements, including from speaking which can be relevant for speech processing in the future (Liu et al., 2021b). We shall return to this point when discussing the hearing sense.
Taste
Taste sensing has been mimicked for decades with electronic tongues (e-tongues), which normally contain an array of sensing units with principles of detection based mostly on electrochemical methods (Winquist, 2008) and impedance spectroscopy (Riul et al., 2002). The rationale behind an e-tongue is that humans perceive taste as a combination of five basic tastes, viz. sweet, salty, sour, bitter and umami, thus meaning that the brain receives from the sensors in the papillae signals that are not specific to any given chemical compound. This is the so-called global selectivity principle (Riul et al., 2002), according to which the sensing units, in contrast to biosensors, do not need to contain materials with specific interactions with the samples. Obviously, obtaining sensing units with high sensitivity require their manufacturing materials to be judiciously chosen, bearing in mind the intended application. For example, if an e-tongue is to be used to distinguish liquids with varied acidity levels, polyanilines can be selected for the sensing units since their electrical properties are very sensitive to the pH. In practice, an e-tongue typically comprises four to six sensing units made of nanomaterials or nanostructured polymer films, where the distinct sensing units are expected to yield different responses for a given liquid. This variability is important to establish a “finger print” for the liquids under analysis, which may have similar properties. An e-tongue may take different shapes. While the majority comprise sensing units with nanostructured films deposited over areas of the order of cm2, microfluidic e-tongues have also been produced (Shimizu et al., 2017). This is an advantageous arrangement because it requires small amounts of samples for the measurements and allows for multiplex sensing in miniaturized setups.
Though conceived to mimic the tasting function, e-tongues may also be employed in several tasks unrelated to taste. Hence, in addition to their use in evaluating taste in wines, juices, coffee (Riul Jr et al., 2010), taste masking in pharmaceutical drugs (Machado et al., 2018), e-tongues have been utilized in detecting poisoning and pollution in waters, fuel adulteration and soil analysis (Braunger et al., 2017). Three other aspects are worth mentioning about e-tongues. The first is related to the incorporation of biosensors as one (or more) of the sensing units in the arrays. The overall selectivity can be enhanced in these so-called bioelectronic tongues, as demonstrated for the discrimination of two similar tropical diseases (Perinoto et al., 2010). A second aspect refers to data analysis since the use of a global selectivity concept requires assessing the combined responses of various sensing units. As a consequence, a considerable amount of data configurations is generated which must be analyzed with statistical and computational methods. Reduction in the dimensionality of the data representation is thus a central operation. The methods often applied to e-tongue data include principal component analysis (PCA) (Jolliffe and Cadima, 2016) and interactive document mapping (IDMAP) (Minghim et al., 2006). With these methods, the response measured for one sample—e.g., one impedance spectrum—is mapped as a graphical marker, and markers are spatialized so that those markers depicting samples with similar responses will be placed close to each other. Hence, one may identify visual clusters of similar elements, which would suggest a correct classification of the samples is possible in case one univocal cluster exists for each class. If the number of samples is too large, the visualization of clusters on a map is not efficient due to overlapping of markers and clusters. The data may still be processed with machine learning algorithms (Neto et al., 2021), which can be either supervised or unsupervised. In supervised learning, it may be also possible to correlate the e-tongue response with human taste (Ferreira et al., 2007). The third aspect is associated with the combination of e-tongues and electronic noses (e-noses, described below). Especially for drinks and beverages such as coffee and wine, flavor perception depends on taste and smell combined, and therefore it is advisable to employ e-tongues in conjunction with e-noses (Rodriguez-Mendez et al., 2014).
Smell
Electronic noses (e-noses) are the counterparts of e-tongues for smell, being also based on global selectivity concepts where arrays of vapour-sensing devices are employed to mimic the mammalian olfactory system (Rakow and Suslick, 2000). Similar to e-tongues, varied principles of detection can be exploited, including electrical, electrochemical, and optical measurements, or any type of measurement used in gas sensors. The materials for building the sensing units are selected to allow for interaction with various types of vapours, as with metalloporphyrin dyes whose optical properties are affected significantly by ligating vapours such as alcohols, amines, ethers, phosphines and thiols (Rakow and Suslick, 2000). The e-noses are mostly obtained with nanostructured films, e.g., Langmuir-Blodgett (LB) (Barker et al., 1994) and, as in biosensor, may include sensing units capable of specific interaction with analytes. An example of the latter was an e-nose with field-effect transistors made with carbon nanotubes functionalized with lipid nanodiscs containing insect odorant receptors (Murugathas et al., 2019). Their selective electrical response to the corresponding ligands for the odorant receptors allowed for distinguishing the smells from fresh and rotten fish (Murugathas et al., 2019).
Sight
Spectacular developments have been witnessed in computational vision owing to the widespread deployment of all sorts of cameras. Though one may argue that the availability of high-quality cameras is far from sufficient for mimicking the sight sense, recent breakthroughs in the field of computational vision demonstrated that artificial systems can already equal, or even surpass the human ability in executing certain image or video analysis tasks (Ng et al., 2018). Facial recognition, for example, can certainly be performed with superior performance by intelligent systems employing deep learning strategies (NandhiniAbirami et al., 2021). Also, cameras may be used to detect and observe phenomena beyond human capabilities, as in the case of infrared vision (Havens and Sharp, 2016). The challenge of replicating the functionality of the human eye in a single device is, nevertheless, still formidable, especially if prosthetic eyes are desired, since a fully functional analogue of the eye remains a long-term goal (Regal et al., 2021). Research on novel materials for bionic eyes and special cameras focuses essentially on bioinspired and biointegrated electronics to fabricate deformable and self-healable devices that preserve functionality while being deformed (Lee et al., 2021). This is analog to other types of devices to mimic human senses, e.g. electronic skin and electronic ear (see below). Another feature shared by these mimicking systems is the need to process large amounts of data, in many cases of entirely different natures. A recent example is represented by an evaluation of withering of tea leaves upon combining data from near-infrared spectroscopy, an electronic eye, and a colorimetric sensing array, which were treated with machine learning algorithms in a machine vision system (Wang et al., 2021).
Hearing
The hearing ability may be mimicked with the so-called electronic ears (Solanki et al., 2017) and other devices, which basically work with strain or pressure sensors. The simplest ones are chemiresistive sensors containing nanomaterials, whose response varies with the mechanical stimulus with sufficient sensitivity to detect whistling, breathing and speaking (Solanki et al., 2017). In terms of materials for sensing, as already mentioned they are similar to those employed for the touch sensors and electronic skins. One issue yet not exploited to a reasonable extent is speech processing. Current applications involving speech processing—prevailing in intelligent assistants, for example—use high-quality microphones to acquire sound. The new developments in wearable strain and pressure sensors allow us to envisage sound acquisition directly from the human (or other living being) body. This would revolutionize the working principles of speech processing, especially in the biomedical area as it would enable real-time online monitoring. Obviously, such an intrusive data collection approach raises ethical issues, for any type of user utterance would be captured and recorded. Another thread is represented by innovative applications made possible by the wide availability of low-cost autonomous microphones, such as the study of environmental soundscapes, for purposes that go from monitoring biodiversity in natural environments (bioacoustics monitoring) (Gibb et al., 2019) or the ocean fauna (Sánchez-Gendriz and Padovese, 2017).
Integration of Sensing and Biosensing Into Intelligent Systems
Some of the examples associated with the five human senses are already representative of integrated systems based on artificial intelligence. It is relevant, however, that well beyond these examples many other types of sensors and biosensors exist which allow for monitoring substances, phenomena, and processes. In spite of the advances in integrating sensors and biosensors, as mentioned here, we should stress that real-time monitoring and seamless integration of multiple sensing devices using different technologies are still in an embryonic stage, as discussed next with a hypothetical scenario of an autonomous transportation station in a metropolitan area.
Autonomous Public Spaces and Infrastructures
Intelligent systems supported by sensing and biosensing are likely to be employed in any type of application involving control and actuation. Particularly challenging will be such integration in large infrastructures, for instance in public spaces and combining multiple initiatives. As an illustration, let us consider a station serving a busy town area, integrating train and bus services, plus a parking lot, as illustrated in Figure 1. Let us imagine it is connected to a large green park area close to a river, with plenty of vegetation, pedestrian and cycling lanes. City administrators want this area to be a safe zone for users of the station and park. The station should be environmentally sustainable, and they also plan to partner with other city managers to run a preventive health care program targeted at the population of users and with researchers from the local university to study and preserve the fauna in the park. One may think of the sensing devices deployed in such a scenario. There will be video cameras for real time monitoring for purposes of adjusting train timetables according to the population flow and also of critical areas and spots for security, water and air quality sensors in the station and in the park and surroundings. The site may include a photovoltaic plant, for which energy generation by the plant and energy consumption both in the station and the park areas are continuously tracked and adjusted as necessary. Light-sensitive sensors can switch illumination on/off; sound sensors at multiple spots in the park monitor animal diversity and how the operation of the busy station affects their behavior. Station users are encouraged to stop and collect fundamental health indicators such as blood pressure, glycemic and cholesterol levels, and oriented towards medical assistance when issues are identified; the program may keep track of and approach those users for whom critical issues have been identified.
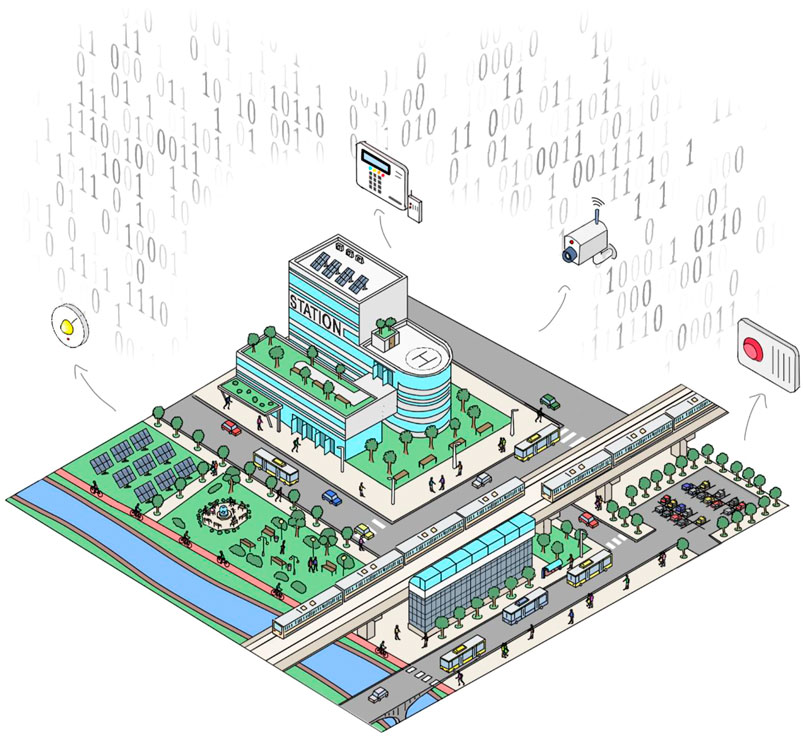
FIGURE 1. A busy urban transportation station and the multiple sensors supporting its automated operation integrated with multiple initiatives of public interest, that continuously generate data of diverse types.
A hypothetical integrated AI system to manage the station autonomously is depicted in the flow chart in Figure 2, which brings an oversimplified abstract view of an intelligent data processing approach. The top layer represents the data sources, including the multitude of sensing devices to monitor internal and external risks in the facilities. These sensors will be continuously generating data of a variety of types, e.g., images, audios, measurements, text forms and documents. It must handle multiple data types, as the data is produced by sensing devices that will include those related to the human senses, i.e., part of the sensing will be sight, touch, taste, smell, and hearing. The diverse data types will demand treatment (storage, filtering, processing) and curation, as indicated the middle block in the figure. Furthermore, some processes will be required to verify if the data makes sense, whether the datasets have sufficient quality as input information for the AI system, represented as a single block for simplicity—though more likely it would consist of multiple integrated systems. Finally, the system will need to learn data representations for algorithmic processing. This AI system will be responsible for the analysis (what happened, where, which is the danger or threat level), then a corresponding action—in some cases in real time. Analysis tasks essentially consist of looking for specific patterns in the data indicating the occurrence of an anomalous situation, or a particular category of event, which in turn must trigger the corresponding actions from the different systems represented in the bottom of the figure. Such a complex scenario consists essentially of a combination of devices for monitoring (quantities and processes) and responding (reacting) to the measurements. The nature of the application may change entirely, e.g., we could think of diagnosis in a medical care facility, or the integrated operating room of a smart city, but the core components of such systems are essentially the same. The implication is that sensing must be ubiquitous and is bound to generate huge quantities of data, which can be connected to the Internet, thus enabling tasks and services to be executed and controlled remotely (Alzahrani, 2017).
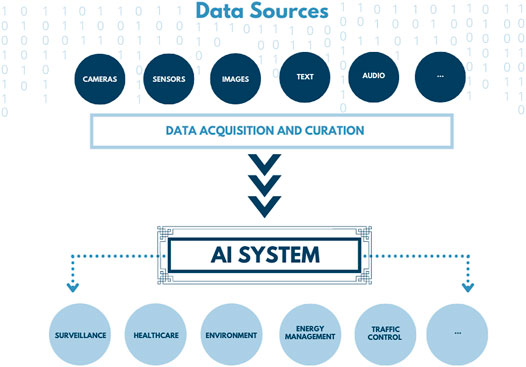
FIGURE 2. Schematic representation of the automated system to manage the train station, which should contain various layers of devices and control systems. The top layer represents the varied data sources, whose data will be acquired and curated in dedicated repositories. These will feed the AI system responsible for processing the data and providing the input for the various applications (surveillance, energy management, etc). The AI module and these applications will comprise a number of independent analysis and control systems.
Existing AI Technology and Major Challenges
The proposal outlined in Figure 2, of a sensor enabled integrated AI system with multiple controls over its environment, may seem a far-fetched view of AI applications today. Yet, a careful analysis of its components indicates that existing technologies are already sufficient for implementing most of the tasks. Indeed, the literature is rich with examples of applications representative of all the components in the figure. Automation has been observed in a multitude of tasks for which the input is digital data, such as object detection in images, or natural language translation, or traffic control, with performance levels equivalent or superior to that of human operators. Smart integration of the required components is, however, a mammoth endeavor. For example, the management and curation of the data from such disparate natures and formats (i.e., images, text, audio, videos, sensing data) is a tremendous challenge. The architecture of the AI system will be highly complex as it must be prepared to detecting and handling multiple ordinary and anomalous situations timely. Even more relevant is that such a system, despite its complexity, is limited in that only classification tasks will be performed efficiently, as already mentioned. Nonetheless, full autonomous operation demands other relevant tasks, such as assessing risks and making decisions based on such assessments. These latter tasks will require at least some degree of interpretation, and therefore current technologies are still not sufficient for the autonomous operation envisaged.
The various issues involved in dealing with big data and machine learning for applications such as the autonomous station have been discussed in reviews and opinion papers (Oliveira et al., 2014; Rodrigues et al., 2016; Paulovich et al., 2018; Rodrigues et al., 2021). We observe a synergistic movement driven by the combination of data generation at unprecedented levels of detail, variety and velocity with massive computing capability. This is crucial to introduce some kind of “intelligence” into systems that process data to execute complex tasks. For instance, data now plays an “active” role in science discovery, meaning that rather than solely supporting hypothesis verification, data collected at massive scales with ubiquitous and networked sensing devices connected to “things” (the “Internet of Things”) can support “intelligent” automation of complex tasks and foster active search for hidden hypotheses.
It must be stressed that building complex autonomous systems as the hypothetical case of the transportation station will demand considerable human effort. Human experts need to be prepared to inspect a system´s underlying algorithms and supervise task execution and decision making during development and operation, in order to ensure it complies with the intended goals. This is different than just inspecting results yielded by a standalone algorithm on a relatively small data set. ML algorithms can be trained to identify patterns from data, but data quality is a fundamental issue (otherwise, “garbage-in, garbage-out”). Moreover, ensuring quality and correctness of the outcomes may demand considerable user supervision. The complexity posed by the sheer scale of data generation and processing, plus the need to handle distinct data types produced by multiple sources in an integrated manner, requires substantial changes in the role of the experts. It also modifies the type of expertise required. Help can be found from researchers working in the field known as “visual analytics” (Endert et al., 2017), in which the goal is to study ways of introducing effective user interaction into data processing and model learning. An additional concern addressed with visual representations is to enhance model interpretability. Domain experts in charge of analysis, as well as decision makers, must be well informed on the concepts behind the techniques, understand their limitations and learn how to parametrize algorithms properly and how to interpret the results and the performance measures. Techniques for data and model visualization can contribute to empowering the mutual roles of a human expert and a ML algorithm in conducting analysis tasks.
Concluding Remarks
The integration of sensing and biosensing with machine learning algorithms to develop sophisticated autonomous systems has been explored here using two metaphors. In the first, we highlighted recent developments in nanotechnology to fabricate devices that can mimic the five human senses. The motivation behind this choice was not only because these nanotech devices constitute an essential requirement for autonomous entities, but also due to the data processing involved. The other metaphor was related to an autonomous transportation station where we illustrated how an integrated “intelligent” system could manage the station using the input data from a multitude of sensors. This latter example was intended as a mere illustration, for there is a virtually endless list of complex problems could be similarly tackled at a large scale with systems directly fed with data from sensors, with limited human intervention. These range from traffic control to population health and environmental monitoring, precision agriculture to manufacturing processes, amongst others. The wider dissemination of solutions based on machine learning from data provided by sensors is an inescapable trend. Their successful application depends less on technological issues and more on tackling important conceptual and practical ethical, legal and social issues regarding data collection and usage. Yet, some technological issues remain, such as seamless integration of data from different systems and ensuring continuously adaptive learning in highly dynamic environments.
Data Availability Statement
The original contributions presented in the study are included in the article/Supplementary Material, further inquiries can be directed to the corresponding author.
Author Contributions
All authors listed have made a substantial, direct, and intellectual contribution to the work and approved it for publication.
Funding
INEO, CAPES, CNPq (301847/2017–7) and FAPESP (2018/22214–6) (Brazil).
Conflict of Interest
The authors declare that the research was conducted in the absence of any commercial or financial relationships that could be construed as a potential conflict of interest.
The handling editor declared a past co-authorship/collaboration on a previous publication with the authors.
Publisher’s Note
All claims expressed in this article are solely those of the authors and do not necessarily represent those of their affiliated organizations, or those of the publisher, the editors, and the reviewers. Any product that may be evaluated in this article, or claim that may be made by its manufacturer, is not guaranteed or endorsed by the publisher.
References
Alzahrani, S. M. (2017).Sensing for the Internet of Things and its Applications, In 5th International Conference on Future Internet of Things and Cloud Workshops. Prague: FiCloudW, 88–92. doi:10.1109/FiCloudW.2017.94
Barker, P. S., Chen, J. R., Agbor, N. E., Monkman, A. P., Mars, P., and Petty, M. C. (1994). Vapour Recognition Using Organic Films and Artificial Neural Networks. Sensors Actuators B: Chem. 17, 143–147. doi:10.1016/0925-4005(94)87042-X
Braunger, M., Shimizu, F., Jimenez, M., Amaral, L., Piazzetta, M., Gobbi, Â., et al. (2017). Microfluidic Electronic Tongue Applied to Soil Analysis. Chemosensors 5, 14. doi:10.3390/chemosensors5020014
Buscaglia, L. A., Oliveira, O. N., and Carmo, J. P. (2021). Roadmap for Electrical Impedance Spectroscopy for Sensing: A Tutorial. IEEE Sensors J., 1. doi:10.1109/JSEN.2021.3085237
Endert, A., Ribarsky, W., Turkay, C., Wong, B. L. W., Nabney, I., Blanco, I. D., et al. (2017). The State of the Art in Integrating Machine Learning into Visual Analytics. Comp. Graphics Forum 36 (8), 458–486. doi:10.1111/cgf.13092
Ferreira, E. J., Pereira, R. C. T., Delbem, A. C. B., Oliveira, O. N., and Mattoso, L. H. C. (2007). Random Subspace Method for Analysing Coffee with Electronic Tongue. Electron. Lett. 43, 1138–1139. doi:10.1049/el:20071182
Gibb, R., Browning, E., Glover‐Kapfer, P., and Jones, K. E. (2019). Emerging Opportunities and Challenges for Passive Acoustics in Ecological Assessment and Monitoring. Methods Ecol. Evol. 10, 169–185. doi:10.1111/2041-210X.13101
Guerrini, L., Garcia-Rico, E., Pazos-Perez, N., Alvarez-Puebla, R. A., and Smelling, Seeing. (2017). Smelling, Seeing, Tasting-Old Senses for New Sensing. ACS Nano 11, 5217–5222. doi:10.1021/acsnano.7b03176
Hammock, M. L., Chortos, A., Tee, B. C.-K., Tok, J. B.-H., and Bao, Z. (2013). 25th Anniversary Article: The Evolution of Electronic Skin (E-Skin): A Brief History, Design Considerations, and Recent Progress. Adv. Mater. 25, 5997–6038. doi:10.1002/adma.201302240
Havens, K. J., and Sharp, E. J. (2016). Thermal Imaging Techniques to Survey and Monitor Animals in the Wild: A Methodology. Amsterdam: Academic Press. ISBN 978-0-12-803384-5. doi:10.1016/C2014-0-03312-6
Jolliffe, I. T., and Cadima, J. (2016). Principal Component Analysis: a Review and Recent Developments. Phil. Trans. R. Soc. A. 374, 20150202. doi:10.1098/rsta.2015.0202
Ng, J. Y., Neumann, J., Davis, L. S., and Davis, L. S. (2018). ActionFlowNet: Learning Motion Representation for Action Recognition, IEEE Winter Conference on Applications of Computer Vision (WACV), 1616–1624. doi:10.1109/WACV.2018.00179
Kim, D.-H., Lu, N., Ma, R., Kim, Y.-S., Kim, R.-H., Wang, S., et al. (2011). Epidermal Electronics. Science 333, 838–843. doi:10.1126/science.1206157
Lee, W., Yun, H., Song, J.-K., Sunwoo, S.-H., and Kim, D.-H. (2021). Nanoscale Materials and Deformable Device Designs for Bioinspired and Biointegrated Electronics. Acc. Mater. Res. 2, 266–281. doi:10.1021/accountsmr.1c00020
Lipomi, D. J., Vosgueritchian, M., Tee, B. C.-K., Hellstrom, S. L., Lee, J. A., Fox, C. H., et al. (2011). Skin-like Pressure and Strain Sensors Based on Transparent Elastic Films of Carbon Nanotubes. Nat. Nanotech 6, 788–792. doi:10.1038/NNANO.2011.184
Liu, Q., Jin, L., Zhang, P., Zhang, B., Li, Y., Xie, S., et al. (2021). Nanofibrous Grids Assembled Orthogonally from Direct-Written Piezoelectric Fibers as Self-Powered Tactile Sensors. ACS Appl. Mater. Inter. 13, 10623–10631. doi:10.1021/acsami.0c22318
Liu, X., Ren, Z., Liu, F., Zhao, L., Ling, Q., and Gu, H. (2021). Multifunctional Self-Healing Dual Network Hydrogels Constructed via Host-Guest Interaction and Dynamic Covalent Bond as Wearable Strain Sensors for Monitoring Human and Organ Motions. ACS Appl. Mater. Inter. 13, 14612–14622. doi:10.1021/acsami.1c03213
Machado, J. C., Shimizu, F. M., Ortiz, M., Pinhatti, M. S., Carr, O., Guterres, S. S., et al. (2018). Efficient Praziquantel Encapsulation into Polymer Microcapsules and Taste Masking Evaluation Using an Electronic Tongue. Bcsj 91, 865–874. doi:10.1246/bcsj.20180005
Minghim, R., Paulovich, F. V., and de Andrade Lopes, A. (2006). “Content-based Text Mapping Using Multi-Dimensional Projections for Exploration of Document Collections,” in Visualization and Data Analysis 2006. Of SPIE-IS&T Electronic Imaging. Editors Robert. F. Erbacher, Jonathan. C. Roberts, Matti. T. Gröhn, and Katy. Börner (SPIE), 6060, 60600S. doi:10.1117/12.650880
Murugathas, T., Zheng, H. Y., Colbert, D., Kralicek, A. V., Carraher, C., and Plank, N. O. V. (2019). Biosensing with Insect Odorant Receptor Nanodiscs and Carbon Nanotube Field-Effect Transistors. ACS Appl. Mater. Inter. 11, 9530–9538. doi:10.1021/acsami.8b19433
Nandhini Abirami, R., Durai Raj Vincent, P. M., Srinivasan, K., Tariq, U., and Chang, C.-Y. (2021). Deep CNN and Deep GAN in Computational Visual Perception-Driven Image Analysis. Complexity 2021, 1–30. doi:10.1155/2021/5541134
Neto, M. P., Soares, A. C., Oliveira, O. N., and Paulovich, F. V. (2021). Machine Learning Used to Create a Multidimensional Calibration Space for Sensing and Biosensing Data. Bcsj 94, 1553–1562. doi:10.1246/bcsj.20200359
Oliveira, Jr., O. N., Neves, T. T. A. T., Paulovich, F. V., and de Oliveira, M. C. F. (2014). Where Chemical Sensors May Assist in Clinical Diagnosis Exploring “Big Data”. Chem. Lett. 43, 1672–1679. doi:10.1246/cl.140762
Paulovich, F. V., De Oliveira, M. C. F., and Oliveira, O. N. (2018). A Future with Ubiquitous Sensing and Intelligent Systems. ACS Sens. 3, 1433–1438. doi:10.1021/acssensors.8b00276
Perinoto, Â. C., Maki, R. M., Colhone, M. C., Santos, F. R., Migliaccio, V., Daghastanli, K. R., et al. (2010). Biosensors for Efficient Diagnosis of Leishmaniasis: Innovations in Bioanalytics for a Neglected Disease. Anal. Chem. 82, 9763–9768. doi:10.1021/ac101920t
Qiu, W., Zhang, C., Chen, G., Zhu, H., Zhang, Q., Zhu, S., et al. (2021). Colorimetric Ionic Organohydrogels Mimicking Human Skin for Mechanical Stimuli Sensing and Injury Visualization. ACS Appl. Mater. Inter. 13, 26490–26497. doi:10.1021/acsami.1c04911
Rakow, N. A., and Suslick, K. S. (2000). A Colorimetric Sensor Array for Odour Visualization. Nature 406, 710–713. doi:10.1038/35021028
Regal, S., Troughton, J., Djenizian, T., and Ramuz, M. (2021). Biomimetic Models of the Human Eye, and Their Applications. Nanotechnology 32, 302001. doi:10.1088/1361-6528/abf3ee
Riul, A., Dos Santos, D. S., Wohnrath, K., Di Tommazo, R., Carvalho, A. C. P. L. F., Fonseca, F. J., et al. (2002). Artificial Taste Sensor: Efficient Combination of Sensors Made from Langmuir−Blodgett Films of Conducting Polymers and a Ruthenium Complex and Self-Assembled Films of an Azobenzene-Containing Polymer. Langmuir 18, 239–245. doi:10.1021/la011017d
Riul Jr., A., Dantas, C. A. R., Miyazaki, C. M., and Oliveira Jr., O. N. (2010). Recent Advances in Electronic Tongues. Analyst 135, 2481–2495. doi:10.1039/c0an00292e
Rodrigues, J. F., Florea, L., de Oliveira, M. C. F., Diamond, D., and Oliveira, (2021). Big Data and Machine Learning for Materials Science. Discov. Mater. 1, 12. doi:10.1007/s43939-021-00012-0
Rodrigues, J. F., Paulovich, F. V., de Oliveira, M. C., and de Oliveira, O. N. (2016). On the Convergence of Nanotechnology and Big Data Analysis for Computer-Aided Diagnosis. Nanomedicine 11, 959–982. doi:10.2217/nnm.16.35
Rodriguez-Mendez, M. L., Apetrei, C., Gay, M., Medina-Plaza, C., de Saja, J. A., Vidal, S., et al. (2014). Evaluation of Oxygen Exposure Levels and Polyphenolic Content of Red Wines Using an Electronic Panel Formed by an Electronic Nose and an Electronic Tongue. Food Chem. 155, 91–97. doi:10.1016/j.foodchem.2014.01.021
Sánchez-Gendriz, I., and Padovese, L. R. (2017). Temporal and Spectral Patterns of Fish Choruses in Two Protected Areas in Southern Atlantic. Ecol. Inform. 38, 31–38. doi:10.1016/j.ecoinf.2017.01.003
Shimizu, F. M., Todão, F. R., Gobbi, A. L., Oliveira, O. N., Garcia, C. D., and Lima, R. S. (2017). Functionalization-free Microfluidic Electronic Tongue Based on a Single Response. ACS Sens. 2, 1027–1034. doi:10.1021/acssensors.7b00302
Solanki, V., Krupanidhi, S. B., and Nanda, K. K. (2017). Sequential Elemental Dealloying Approach for the Fabrication of Porous Metal Oxides and Chemiresistive Sensors Thereof for Electronic Listening. ACS Appl. Mater. Inter. 9, 41428–41434. doi:10.1021/acsami.7b12127
Wang, Y., Liu, Y., Cui, Q., Li, L., Ning, J., and Zhang, Z. (2021). Monitoring the Withering Condition of Leaves during Black tea Processing via the Fusion of Electronic Eye (E-Eye), Colorimetric Sensing Array (CSA), and Micro-near-infrared Spectroscopy (NIRS). J. Food Eng. 300, 110534. doi:10.1016/j.jfoodeng.2021.110534
Winquist, F. (2008). Voltammetric Electronic Tongues - Basic Principles and Applications. Microchim Acta 163, 3–10. doi:10.1007/s00604-007-0929-2
Keywords: sensors, biosensors, artificial intelligence, machine learning, ELECTRONIC TONGUE, electronic skin, visual analytics
Citation: Oliveira ON and Oliveira MCF (2021) Sensing and Biosensing in the World of Autonomous Machines and Intelligent Systems. Front. Sens. 2:752754. doi: 10.3389/fsens.2021.752754
Received: 03 August 2021; Accepted: 06 September 2021;
Published: 17 September 2021.
Edited by:
Dermot Diamond, Dublin City University, IrelandReviewed by:
Stanislav Moshkalev, State University of Campinas, BrazilJuyoung Leem, Stanford University, United States
Copyright © 2021 Oliveira and Oliveira. This is an open-access article distributed under the terms of the Creative Commons Attribution License (CC BY). The use, distribution or reproduction in other forums is permitted, provided the original author(s) and the copyright owner(s) are credited and that the original publication in this journal is cited, in accordance with accepted academic practice. No use, distribution or reproduction is permitted which does not comply with these terms.
*Correspondence: Osvaldo N. Oliveira Jr, Y2h1QGlmc2MudXNwLmJy