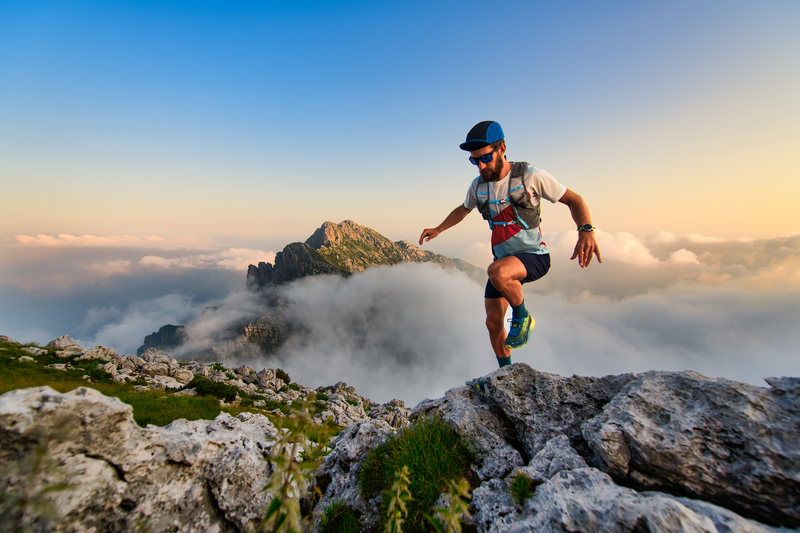
94% of researchers rate our articles as excellent or good
Learn more about the work of our research integrity team to safeguard the quality of each article we publish.
Find out more
METHODS article
Front. Water , 25 October 2024
Sec. Water Resource Management
Volume 6 - 2024 | https://doi.org/10.3389/frwa.2024.1468354
Flooding poses a severe global threat, necessitating advanced methodologies to assess and manage its risks effectively. This study introduces a novel approach that integrates Geographic Information System (GIS) with hydrologic-hydraulic modeling to evaluate the combined drivers of current and future flood risks. The method is applied to the development Bonita Bay in southwest Florida. It occurs in a region highly susceptible to flooding due to its low elevation and proximity to tidal waters. The innovative integration of GIS with hydrologic-hydraulic models enables detailed assessment and visualization of flood inundation areas under multiple flood drivers including design storms, land use changes, groundwater rise, and sea-level rise. This allows for the seamless simulation of complex flood interactions with only minor adjustments to the model for the identified drivers. The results indicate significant increases in initial water storage caused by sea and groundwater level rise and amplified storm runoff from land use changes. A 2% increase in flooded areas is projected with stronger design storms, and a 5% increase by 2,100 compared to 2024. This approach provides a robust framework for developing tailored flood mitigation strategies and can be adapted to various coastal regions globally.
Flooding is a worldwide threat causing significant socioeconomic and environmental damages that affect millions of people (Jonkman, 2005). The increasing frequency and intensity of flood events, driven by climate change and rapid urbanization, underscores the urgent need for effective flood management strategies. According to the World Economic Forum, about 1.81 billion people, or 23% of the world population, are at risk of flooding (McLennan, 2022). In in low-lying, densely populated coastal cities, this threat is particularly acute, ranging worldwide from the United States to the European coasts, Chinese and Indian megacities, and many other cities globally facing substantial risks (De Sherbinin et al., 2012; Sweet et al., 2022). In the United States alone, about 42% of the population lives in coastal areas that are susceptible to flooding (Peter Sheng et al., 2022; Shrestha et al. Long and Gao, 2023). The challenge of flooding is the greatest depending on the geographical locations. For Example, in Florida, United States, about 76.5% of the state population lives in coastal regions that must tackle the challenge of frequent flooding (Tenali and McManus, 2022). However, mitigating flooding on our dynamic planet is challenging due to the complex interactions between human activities and environmental factors, necessitating use of advanced methodologies to understand and address the intricate interplay of flood drivers in specific regions (Andersen and Marshall Shepherd, 2013; Fuller et al., 2010).
Research on flooding has predominantly focused on individual drivers such as flooding caused by sea lever rise (SLR), groundwater rise, extreme precipitation, and land use changes. However, these studies often overlook the compounded effects of multiple flood drivers, which can significantly exacerbate flood risks. For example, while SLR research typically focuses on natural shorelines, (e.g., Bosserelle et al., 2022), SLR can also raise groundwater tables and may impact stormwater drainage capacity (Davtalab et al., 2020; Rahimi et al., 2020). Heavy rainfall coupled with rapid land use change in a coastal watershed has amplified flooding and caused adverse economic and human impacts in recent years (Breinl et al., 2021; Schroeder et al., 2022; Viglione and Blöschl, 2009). It is also evident that human-induced landscape interventions such as urbanization, which often replaces natural landscapes with impervious surfaces like pavement and rooftops, reduces infiltration, increases surface runoff, and alters natural drainage patterns, thus causing localized flooding (Aguiar et al., 2020; Schroeder et al., 2022). Additionally, mining and agricultural intensification alters natural drainage patterns and flood peaks further aggravating flooding in coastal areas caused by SLR, groundwater rises and other factors (Su et al., 2017). The interlinkage of these drivers means that an imbalance of one factor can increase flooding due to another. Consequently, the significance of compound effects is rising (Rahimi et al., 2020). For example, a study on the 128.2 km2 San Leandro coastal watershed located in Oak flatland, CA, United States demonstrated a 2.8 km2 increase in flood area when considering a combined effect of SLR and groundwater level rise rather than considering them individually (Rahimi et al., 2020).
Developing a comprehensive approach for managing flood risk is a significant challenge due to the complex interplay between natural and human-caused factors. Natural phenomena, such as heavy rainfall, rapid snowmelt, storm surge, and hurricanes, can all trigger flooding events (Hirschboeck, 1988). Human activities like deforestation, urbanization, and climate change exacerbate these natural drivers, making flooding events more frequent and severe (Andersen and Marshall Shepherd, 2013; Kumar et al., 2021). For example, urban expansion can worsen flooding from SLR and extreme precipitation by creating more impervious surfaces, like pavements that reduces rechange the underlying aquifer, and this reduced infiltration increases surface runoff and elevate flood risk (Schroeder et al., 2022; Sukop et al., 2018). The intricate interplay of these natural and human factors complicated the development of a universal applicable approach to flood management.
Only a few studies have developed a method to investigate more than two flood drivers. Previous studies have addressed flooding in coastal urban watersheds (Saksena et al., 2021), investigated associated risks and estimated the loss of life and resources (Peter Sheng et al., 2022). Most research works in the literature are focused on flooding caused by individual drivers without a comprehensive approach to address combined effects. One study leveraged a climate model coupled with surge wave model and investigated flooding due to the combined effect of SLR and tropical cyclones in Florida highlighting potential increases in flood frequency (e.g., 1% event becoming a 3-year event, 0.2% becoming a 5-year event for 100 and 500-years respectively) (Peter Sheng et al., 2022). Similarly, Schroeder et al. (2022) developed a method integrating Interconnected Pond and Channel Routing (ICPR), now known as StormWise™ and Geographic Information Systems (GIS) to address urban expansion and groundwater rise, and Davtalab et al. (2020) used a similar approach to investigate the effect of SLR on groundwater rise for the rising concern of costal inundation in Southwest Florida (Davtalab et al., 2020; Schroeder et al., 2022). However, these limited studies lack integration of all possible drivers and the effects of coastal flooding complex and poorly understood.
There are previous studies that have focused on the combined impacts of extreme precipitation with land use change (Sempewo et al., 2024; Schroeder et al., 2022; Aguiar et al., 2020), SLR and storm surge (Sweet et al., 2022; Bosserelle et al., 2022; Fang et al., 2021), groundwater level rise (Howland and Thompson, 2024; Long and Gao, 2023; Davtalab et al., 2020), and hurricanes and SLR rise (Howland and Thompson, 2024) to estimate coastal flooding. These studies employed climate models, SLR projections, and historical tide gauge data to predict future flooding risks. However, most models have traditionally considered only a few drivers and are not specifically tailored to the groundwater mounding of coastal areas impacted by SLR.
Recognizing the catastrophic impacts of compound effects on costal communities, recent research has increasingly emphasized the importance of considering multiple drivers simultaneously. This study differs by integrating GIS with hydrology-hydraulic approach to capture the combined effects of multiple flood drivers. Our study aligns with Rahimi et al. (2020), who highlighted the critical role of compound effects, such as sea-level rise, groundwater rise, and precipitation, in coastal flooding. By analyzing combined flood drivers, these studies have demonstrated that the drainage capacity of existing infrastructure is significantly overwhelmed, leading to widespread flooding. This underscores the necessity of incorporating compound effects into coastal inundation assessments to accurately predict and mitigate future risks. This study further extends this understanding by incorporating land use change as an additional driver.
The research goal is to develop an integrated GIS and hydrology-hydraulic modeling approach for combined flood drivers, addressing the growing concern of flooding in Florida. The novelty of this approach lies in the seamless integration of ICPR model (Streamline Technology, 2018), which incorporates local hydrologic and hydraulic features, with ArcMap to analyze combined flood drivers in coastal regions, including precipitation, land use change, groundwater fluctuations, and SLR. With its extensive and diverse georeferenced graphical toolset and customizable 2D mesh, StormWise software simulates flood drivers, facilitates data logging, and enables visualization for informed decision-making. This method represents a significant advancement over traditional approaches by providing a comprehensive tool to evaluate the interactions between multiple flood drivers. As a test case, we apply this method to Bonita Bay Community, in southwest Florida to assess the present (2024) and future (2100) flooding scenarios. This involves combing various design storms, SLR, groundwater rises, and land-use changes reflecting the rapid urbanization of the area. By demonstrating its effectiveness in Bonita Bay, this approach can potentially be replicated in other coastal regions facing similar challenges.
The method leverages advancements in the hydrology-hydraulic models for surface and sub surface flow simulations coupled with the powerful capabilities of the Geographic Information System (GIS). Use of ArcGIS in spatial data preparation and visualization facilitates user-friendly input–output and allows for data visualization in a preferred format. Organizing the train/digital elevation model (DEM), soil data, land use/cover, and the spatial hydraulic data of the area of interest using is performed in ArcGIS. Accurate spatial distribution of meteorological and hydrological data is critical to understand the hydrologic processes such as the effect of rainfall pattern, sea and groundwater rise, infiltration, evapotranspiration, and runoff conditions.
The hydrology-hydraulic model assembles the processes of water flow in the surface and sub-surface, and incorporates additional hydroclimatic inputs, empowering users to define and integrate geometric configurations of the model to simulate the combined effects on flooding. Hydrology-hydraulic models leverage scientific principles to simulate engineering scenarios based on user-defined input parameters. This fosters modelers to select an appropriate hydrology-hydraulic model type to represent their area of concern. While most hydrology and hydraulic models operate separately, there are models that integrate the hydrologic processes and hydraulic analyses using both aspects. A prime example is the StormWise (previously ICPR4) model, widely used in Florida and other parts of the United States (Saksena et al., 2019, 2021). StormWise is an advanced hydrologic and hydraulic modeling software used to assess flood risks and map floodplains. It integrates surface water and groundwater flow models, emphasizing interactions between aquifer systems and surface water bodies for effective stormwater management.
Combining ArcMap and a hydrology-hydraulic model provides an opportunity to integrate the drivers and gives the flexibility for evaluating multiple simulations without changing much of the model setups. This fosters a unique approach in capturing the combined as well as the individual effects of the drivers on a specific area of interest. The combination is not only used for data analysis and simulation but also to interpret the results for decision-making. This gives an opportunity to identify and compare the inundation area by re-simulating the framework (Figure 1) based on the preassigned conditions. Furthermore, the synergy between ArcMap and hydrology-hydraulic model establishes an iterative process for formulating timeline scenarios that makes it flexible to assess multiple scenarios.
The developed framework champions a flexible approach to tackling the global challenge of flooding. The approach offers two unique advantages. Firstly, it quantifies flood inundation areas for specific drivers by following unique flow paths designed in Figure 1. This is achieved by modifying the driver while maintaining the geometric and hydraulic configuration. Secondly, it offers flexibility in scenario development, crucial for visualizing the impacts of individual or combined flood drivers. This empowers the development of current and future flood predictions, paving the way for effective mitigation strategies from the simulation results.
To understand historical and future flood risks, it is first necessary to establish a baseline scenario for the current conditions. This scenario captures all the factors influencing flooding within the simulation year. A crucial first step involves a critical literature review and thorough understanding of the study area. This helps identify the dominant flood drivers and existing drainage structures. These drivers, such as rainfall, SLR and groundwater level rise, and land use changes are then incorporated into hydrology-hydraulic model software. Additionally, spatial data are generated using ArcMap, drawing on information like Digital Elevation Models (DEMs), land use, soil characteristics, and existing and planned drainage infrastructure within the study area.
The establishment of the base scenario and predictions is site-specific, relaying on historical and current levels from monitoring as well as simulated values. Groundwater levels are determined using a stabilization simulation with the StormWise model, which employs projected tidal elevations as boundaries. The simulation runs until groundwater levels reaches steady state, with then serve as the initial condition for future scenario simulations. For SLR, regional predictions that encompass a range of uncertainties can be integrated into the model. This allows for a comparison of future conditions derived from both historical data and climate models. The methodology for combined drivers enables the simulation of multiple scenarios and their cumulative impact on flooding.
The model setup phase involves defining computational parameters, the study area geometric representation, boundary conditions, and various simulation scenarios. A typical initial simulation focuses on a design storm with a 25-year return period, which is a common permitting requirement in southwest Florida. However, additional simulations for different return periods are necessary to account for potential variations in precipitation. Similarly, if uncertainties exist regarding specific flood drivers, the modeler can conduct multiple simulations with varying driver quantities. Ultimately, the approach involves running a range of simulations, modifying individual drivers, and creating combined scenarios. This comprehensive approach ensures all potential flood drivers, and their interactions are considered throughout the simulation period.
To gain a thorough understanding of potential future flooding scenarios, this methodology explores a range of possibilities, including individual and combined changes in various drivers. Understanding the current flooding situation through a baseline scenario is crucial. This foundation then allows the development of future flood risk projections using the established modeling tools. The predicted outcomes hinge on how these key factors interact and influence flood events for the future specified timeframe.
The unique modeling approach presented in Figure 1 outlines a stepwise and flexible process to identify drivers, setup and simulate the base model, and conduct scenario-based modeling for all identified scenarios. The framework employs a targeted approach in which only the drivers and model parameters anticipated to change are modified, while all other critical parameters are preserved without necessitating a complete reconfiguration of the model. This ensures that the fundamental physical characteristics of the model remain consistent throughout the simulations. This approach offers two unique advantages. Firstly, it quantifies flood inundation areas for specific drivers by following unique steps that maintain process representation, as outlined in Figure 1. This is accomplished by modifying the driver while maintaining the geometric and hydraulic configuration. Secondly, it offers alternative scenario development, crucial for investigating the impacts of individual or combined flood drivers. This empowers the development of current and future flood predictions, paving the way for effective mitigation strategies from the simulation results.
To implement this approach, each identified driver is projected for the desired future timeframe. Projections for the flood drivers were based on established methodologies outlined in the literature. The data are then converted into the preferred format for use within the hydrology-hydraulic model and/or ArcMap of ArcGIS. Subsequently, the potential interactions and combinations of these drivers are assessed and loaded into the models as well. Simulations are conducted using the hydrology-hydraulic model, incorporating current baseline parameters alongside the projected future changes for each driver. ArcMap is used to visually represent these changes on a map. This approach offers significant flexibility. It allows for the selection of future timeframes based on planned developments and the anticipated needs of managers and policymakers. Additionally, it provides the ability to simulate flooded areas at various points in time, enabling a comparative analysis of potential flood risks. This multi-time step approach is crucial for informed decision-making. Furthermore, the simulations can be superimposed/overlaid allowing visual comparison pond water levels and variations in inundated areas. The holistic approach not only clarifies how flooding unfolds based on the identified drivers, but also provides clear pathways for decision-making by offering a range of potential outcomes.
Bonita Bay, located in Southwest Florida, is within the City of Bonita Springs, United States which has a population of about 56,000. This area is highly valued for its scenic beauty, featuring nature preserves, lakes, and manicured landscaping (Phillips, 2010; Missimer, 1992). Bonita Bay is well developed and has a total area of 10.1 km2 that extends from Palmesta to Pelican Landing (Figure 2). There are 1,529 residents in the development, experiencing the access of comfortable lifestyle of the area. However, like most of the Florida communities, Bonita Bay Communities have no choice but to adapt to more extreme flooding of the area (Wang et al., 2024; Tenali and McManus, 2022). The major threats of flooding are meteorological events including the increased frequency, intensity, and duration of heavy rainfall events in the region, especially during the wet season. As a result, intense downpours can overwhelm drainage systems, leading to flash floods. Hurricanes also occur as the most extreme events submerging all the low-laying lands and causing several damages to the built environment as seen in 2022 (Wang et al., 2024).
Figure 2. Location map of Bonita Bay Community with LiDAR DEM and study area boundary Location map of the study area: (A) Global location of Florida and US on National Geographic World map (B) Location of Bonita Bay area in Florida (C) Model boundary superimposed in the Bonita Bay area boundary and LiDAR DEM.
Bonita Bay Community is known for its well-established infrastructure, including well-designed drainage and interconnected pond systems for flood protection. However, because of the proximity to tidal water bodies, as SLR, the storm surge associated with hurricanes will have a greater impact that might cause saltwater intrusion into coastal aquifers, pushing freshwater upwards (Wang et al., 2024; Sweet et al., 2022). This leads to higher water table positions and increased saturation of the ground, making it more susceptible to flooding. This vulnerability is exacerbated by the higher base water levels in bays and canals, which hinder the effectiveness of drainage systems during heavy rainfall (Missimer & Associates, Inc., 1981). In addition, land use changes due to increasing demand for urban expansion create additional impervious areas which increase stormwater runoff and result in increased flooding.
Some areas in Bonita Bay are low-lying, making them vulnerable to flooding from hurricanes like Ian, which can produce strong storm surges. Expansion of urban area increased the impervious surface, making the area more susceptible to flooding during heavy rainfall. This situation was exacerbated by rising sea and groundwater levels as result of the region’s proximity to the sea and low elevation (Wang et al., 2024). Figure 2 displays the boundary of the Bonita Bay Community alongside LiDAR Digital Elevation Model (DEM) and the study are maps. The study area boundary is delineated by the major contributing areas that drain water to the stream on the south side and the wetlands crossing the community. Areas on the west and northwest sides of the community have intentionally been excluded from the project boundary as they drain directly into the bay. Despite the robust infrastructure in place, the community faces increasing risks due to land use and climate change, including a combination of hurricanes with high winds and storm surges, SLR, and groundwater level rise (Wang et al., 2024). Continued monitoring and adaptive management strategies will be crucial in maintaining the safety and resilience of Bonita Bay in the face of environmental challenges (Tenali and McManus, 2022).
Datasets required to model for the developed approach were obtained from readily available sources without field data collection. First, an inventory of the existing drainage structures (pipes, drop structure, weirs, ponds) was provided by the Water Management District (SFWMD, 2024). Through the inventory about 671 Pipes and 813 Structures (Curb Inlets, Grate Inlets, Manholes, Mitered Ends, and Projecting Ends) were mapped.
The study focused on two major scenarios that integrated three key flood drivers: rainfall intensity, land use changes, SLR, and the resulting groundwater rise (GWR). These scenarios, outlined in Table 1, encompass current (Year 2024) and projected (Year 2,100) conditions. Each scenario incorporates four different design storms tailored to specific land use, SLR, and associated groundwater levels. This approach led to the simulation of four distinct conditions for each scenario, resulting in a total of eight sub-scenarios in this study.
The four design storm events were simulated across different scenarios to encompass a range of rainfall intensities in the area. These storms include the mean annual (2.33-year), 10-year, 25-year, and 100-year, 24-h design storm. These design storms are specific to the environmental permitting requirements for typical developments in Southwest Florida, ensuring effective stormwater management. Table 2 provides a summary of the design storms along with their respective total rainfall amounts. The rainfall accumulations associated with each design storm were obtained from the National Oceanographic and Atmospheric Agency (NOAA) Precipitation Frequency Data Server (PFDS) (NOAA, 2024).
The summary of data, spatial and temporal resolution of the data, the sources of the data are tabulated in Table 3. The projected SLR for 2,100 scenarios was obtained from NOAA (Sweet et al., 2022). Whereas the initial groundwater level was established using a stabilization simulation with the StormWise model which held the boundary at the projected tidal elevation along the boundary and each pond within the model region at its control elevation. The model was simulated until the groundwater elevations reached steady-state condition. The stabilized groundwater surface was exported to provide the initial groundwater surface for each simulation.
Land use/land cover data was obtained from the Geospatial Services Unit of the South Florida Water Management District Information Technology Bureau (SFWMD, 2024). The data consists of 2016 GIS land-use coverage based on the Florida Land Use and Cover Classification System (FLUCCS).
Topography determines the rate and direction of surface water flow as well as quantifies storage volume within each individual subbasin. The digital terrain model (DEM) used for this analysis was obtained from NOAA Digital Coast, Data Access Viewer website (NOAA, 2018) and was developed using LIDAR data collected in 2018. The LiDAR data are in NAVD88 Horizontal Datum are referenced to the Florida State Plane Coordinate System, West Zone (0902), North American Datum of 1983 HARN 920070. The DEM provided a two and three-dimensional representation of the watershed.
The soils coverage of the study area was obtained from the United States Department of Agriculture–Natural Resource Conservation Service (formerly USDA/SCS) Soil Survey Geodatabase (SSURGO) (SSURGO, 2024). The soil data were collected as a GIS shapefile (polygons) with the associated Map Unit Key (MUKEY). The MUKEY is used to relate each unique soil type to the soil characterization in the SURGO database, which is used to define the associated hydrologic parameters. More specifically, The SURGO database was used to extract hydraulic conductivity rates, porosity, and various moisture contents.
The hydrology-hydraulic model setup involves configuring computational algorithms, hydrologic processes, hydraulic simulations, and utilizes the GIS spatial data on the train/digital elevation model (DEM), soil data, land use/cover, spatial–temporal hydroclimatic and hydraulic infrastructure data of the area of interest using ArcGIS to develop water flow simulations. While the spatial data are primary inputs, governing equations, assumptions, and mathematical processes are critical to understand the surface runoff processes such as the effect of rainfall pattern, infiltration, evapotranspiration, and runoff conditions.
Integrating hydrological and hydraulic simulations with GIS capabilities offers numerous benefits. Spatial data is prepared and visualized using ArcGIS, facilitating user-friendly input and output and enabling data visualization in desired formats ensuring a detailed representation of the reality (Peker et al., 2024; Goodchild and Haining, 2004). Key spatial data, including the digital elevation model (DEM), soil data, land use/cover, and hydraulic data, are organized within ArcGIS to accurately represent the area of interest, i.e., Figure 1. Preparing the spatial data on GIS provides essential information for identifying areas susceptible to flooding there by leading to better representation of flood-prone areas (Zhou and Li, 2020). For example, DEMs help determine the topography and slope, which influence water flow and accumulation. Land use/cover maps reveal impervious surfaces that can increase runoff, while soil maps help assess infiltration rates and drainage patterns. Integrating GIS is not only crucial for data preparation but also indispensable for visualizations, flood map interpretation, and understanding the complex interplay of flood-related factors.
While many existing models focus solely on either hydrology (the rainfall-runoff process) or hydraulics (groundwater and surface-water flow behaviors). This often necessitates setting up two separate models, which can be cumbersome, time-consuming, and can compound errors. A combined surface water- groundwater model, e.g., StormWise, developed by Streamline Technology (Streamline Technology, 2018), provides a more holistic and flexible view of flooding. This allowed a simulation of a wider range of scenarios to test various proactive management strategies, ultimately leading to more effective flood control and preparedness.
The StromWise model is a powerful hydrologic–hydraulic modeling approved by Federal Emergency Management Agency (FEMA). It excels at simulating both surface water runoff and groundwater flow and has been extensively validated for Florida watersheds and applied in several other watersheds across the United States (Saksena et al., 2019, 2021). The StormWise model setup consists of defining the hydrological boundaries of the catchment areas contributing to runoff, identifying existing conveyance infrastructures such as pipes, drop structures, weir, pond, outlet location, defining roughness coefficients, and starting water elevations (Figure 3). Loading all the available spatial data into the model is mandatory.
The integration of spatial data, such as DEM, soil, and land use, is crucial for the moded ability to predict flooding and provides essential inputs for accurately assessing flood risk. Given that this study involves a combined scenario that includes land use change and groundwater fluctuation, integrating spatial data is vital to support the flexible framework for multi-scenario analysis presented in Method Section 2.3 and the StormWise-GIS modeling approach illustrated in Figure 3. The scenarios considered incorporate four different design storms, predicted land use, SLR, and associated groundwater levels. The spatial data,including DEM, soil, land use, rain zones, and drainage infrastructure—were pre-processed using ArcMap and integrated into the StormWise model for flood prediction. This integration enables multiple scenario simulations, allowing for a comprehensive assessment of flood risk under varying conditions.
To setup the StormWise model, a digital elevation model (DEM), stormwater inventory data, and aerial imagery were used. These data inputs allowed identification of significant surface water storage areas such as ponds, wetlands, depressions, and hydraulic conveyance features within the study area. The storage areas (115 identified) were incorporated as point features (stage-area nodes) within the StormWise Node point shapefile (Figure 4). Each node allows the model to store and attenuate surface water before routing it through connected hydraulic features. The hydraulic features identified from the stormwater inventory, 54 drop structures and 39 pipes, were added as line features within the StormWise Link polyline shapefile. Using the DEM, a 115 subbasin polygon feature was delineated in the StormWise Basins polygon feature shapefile for each stage-area node. This drainage basin allowed the model to define the hydrologic characteristics of the area draining to each node and calculate the resulting runoff rates which are routed to each node.
The GIS Model Features consisted of land cover, and soils data were imported into StormWise to allow for pre-precessing and model characterization. A stage-area table was then developed for each storage node. The data were extracted at 0.64 cm intervals using the DEM and StormWise stage storage extraction tool. A breakdown of the unique soil/land use area within each subbasin was developed using the StormWise basin characterization tool. The stage-area node starting water levels was parametrized afterwards for each stage area node, using the DEM and aerial imagery to interpolate a normal water level for each storage area. The parametrization was also performed for hydraulic features. This step included developing invert elevations and dimensions for each modeled pipe and control structure. The stormwater inventory obtained as part of the initial data collection effort showed the location, structure type, and pipe size of each feature within the stormwater conveyance system. However, invert elevations and weir dimensions were not available so estimates had to be made using the following assumptions: pipe Inverts were set to elevation 0.0 which is below the normal water levels in the area. This assumption is reasonable since the pipes will generally be fully submerged so the flow rate will be driven by water level differential rather than physical slopes. For control structure elevations, dimensions and attenuation weir vertical slot invert was set at the estimated normal water level for each node, attenuation weir vertical slot size was set based on discharge pipe size (38.1 cm Pipe = 61 cm Slot, 45.7–75 cm Pipe = 91.4 cm Slot, and 91.4–137.2 cm Pipe = 121.9 Slot) (15” Pipe = 24” Slot, 18″-30” Pipe = 36” Slot, and 36″ – 54” Pipe = 48″ slot). The overflow horizontal grate weir invert was set 61 cm above the estimated slot invert, and the overflow horizontal grate weir dimensions were set based on discharge pipe size (38.1 cm Pipe = FDOT Type C Grate, 45.7–76.2 cm Pipe = FDOT Type D Grate, and 91.4–137.2 Pipe = FDOT Type H Grate) (15” Pipe = FDOT Type C Grate, 18″-30” Pipe = FDOT Type D Grate, and 36″ – 54” Pipe = FDOT Type H Grate).
For the hydrologic calculations, the Green-Ampt hydrologic method (Rawls et al., 1983) was used within StormWise to calculate runoff rates and volumes. The model requires soil conductivity, various moisture content, bubble pressure, and pore size to utilize the green-ampt method. These parameters were developed using the SSURGO soil survey in combination with values provided by Rawls et al. (1982). Along with this, the percentages of impervious area were developed based on aerial interpolation which was required for each unique land cover type to determine the percentage of each subbasin that is allowed to infiltrate stormwater into the soil column.
To allow surface water staging within each storage area a pond control volume was delineated using 61 m sides to encompass the ponding area for each stage-area node (Figure 4B). Within each pond, control volume breakpoints were set at 15.2 m spacings to allow StormWise to delineate a honeycomb grid. Each honeycomb acts as a straw to calculate the rate and volume of flow from surface water ponding above the ground to the groundwater model component. This also includes the ability to simulate water levels and flows in the unconfined aquifer using a 2-D groundwater model that is connected to the 1-D surface water model. The groundwater components consist of a triangular network which uses the spatial soil coverage to determine horizontal conductivity and porosity to calculate flow rates through the network.
Boundary conditions were established using current year 2024 – elevation that is −3.81 cm, and projected year 2,100 – elevation that is 78.7 of NOAA tidal data/datum level. Due to variation of tidal elevation for each scenario and the potential of each elevation to influence adjacent groundwater levels, the model needed to simulate an initial groundwater surface raster. A raster was created for each scenario by setting the boundary condition at the estimated tidal elevation and each pond was held at its normal water level. The model was then run until the groundwater levels reached steady-statethroughout the model domain. The resulting surface was then used as the starting groundwater level for each scenario.
Finally, each scenario was simulated using the appropriate rainfall accumulation for the 2.33, 10, 25 and 100-year/24-h design storms. The simulations were run for 60 h to ensure each node reached a peak stage and began to recede. The peak stage for each node was extracted for each scenario/simulation. The process of delineating sub-basins for each pond node involves a detailed analysis using the Digital Elevation Model (DEM) alongside careful consideration of pond interconnections and the placement of control structures. Using ArcGIS Raster Calculator tool and the DEM the ground surface below the peak stage within each subbasin was identified to delineate an area of inundation for each node. Figure 4 shows model setup, flow contributing areas, drainage structures and simulated pond initial water levels.
An integrated surface and groundwater model was developed for the entire study area to analyse the impact of rainfall, SLR, groundwater rise, and land use. However, only four sites (two ponds and two wetlands) were used to present the outcomes in this study. The selections were made to represent both lowland and elevated areas of the study area. Accordingly, the results for the two wetlands (Storage 96 at low elevation and Storage 90 at high elevation) and two ponds (Storage 1 at high elevation and Storage 66 at low elevation) are presented in the next section.
The study investigated the impact of SLR on groundwater levels and its subsequent effects on pond storage and flooding. After reaching steady-state groundwater condition in StormWise, the hourly groundwater elevations were generated to assess the median groundwater table elevation for the current 2024 condition and future 2,100 conditions. Figures 5A,B, the discrepancy displays the groundwater elevation raster from 25-year/24-h design storm for Bonita Bay. A notable change between the current and the future groundwater conditions were observed, with difference in groundwater elevation extending up to an increase of 0.91 m. Another significant finding illustrated is that in the future scenario, the groundwater elevation rises above the surface level for most of the ponds throughout the simulation period. Rising groundwater levels are a consequence of SLR associated with climate change (Davtalab et al., 2020; Shrestha et al., 2023). Besides, infiltration rates from large storms may significantly impact groundwater levels, causing it to rise in both locations and over time in given area (Machusick et al., 2011).
Figure 5 illustrates the dynamic impact of SLR on groundwater levels throughout the study area. It provides a representation of spatial variations in groundwater levels influenced by SLR, highlighting potential risks such as increased flooding. Understanding these dynamics is crucial for developing effective adaptation strategies in water resource management and coastal planning. The study analyzes the response of two ponds (Ponds 1 and 66) and two wetlands (Wetlands 90 and 96) depicted in Figure 6. These findings are discussed in detail in the following section, emphasizing the need for adaptive measures to mitigate the effects of SLR on water storage capacities.
Figure 6. Pond and wetland water level variation for the 2024 and 2,100 scenarios: (A) Pond, storage 1, (B) pond storage 66, (C) wetland storage 90, and (D) Wetland storage 96.
The response of ponds in urban areas is affected by the rainfall intensity, soil type, land use, groundwater and their initial available storage. Ponds in close proximity of the coast are affected by the SLR and its impact on the ground-water fluctuation. The simulation results for the four stormwater systems within the study are presented to demonstrate ponds and wetlands responses (discussed as stage) with respect to SLR, land use and groundwater. Figure 6 a through d shows variations in stage levels for the two ponds (Ponds 1 and 66) and two wetlands (Wetlands 90 and 96) across four design storms, considering both current and future scenarios.
The response of storage 66, 90, and 96 is better compared to storage 1, despite being situated in areas with high groundwater levels. Storage 1 is located at a higher elevation, resulting in relatively lower groundwater depth compared to the other three storage areas. There is no difference in the initial pond stage between the 2024 and 2,100 scenarios because Storage 1 is far from the coast, and the groundwater mound due to the 2,100 SLR scenario is not significant. However, under the worst-case scenario for 2,100, the initial pond stage increases from 2 m (6.5 ft) to 2.4 (8 ft). This increase is attributed to changes in land use and rainfall intensity. The greater area of impervious surfaces reduces the infiltration rate of rainwater, leading to faster runoff and flooding. Also, greater storm intensity and duration coupled with the impervious areas aggravates flooding (Breinl et al., 2021; Viglione and Blöschl, 2009). The ponds behave differently one from the other influenced by factors such as their capacity, geographical location, and proximity to the sea. These findings are consistent with previous research on reliability of stormwater retention ponds in southwest Florida (Davtalab et al., 2020).
Storage 66, 90, and 96 experienced significant loss of initial storage due to the SLR-driven groundwater mound. As the catchments for these storages are located in less developed parts of the community, the increase in water stage is less pronounced compared to Storage 1. Wetlands, represented by storage 90 and 96, exhibit unique behavior during the simulation period. Water levels show significant differences, reaching depths of up to 0.61 m (2 ft) in storage area 90 (Figure 6C) and 0.76 m (2.5 ft) in storage 96 (Figure 6D). Storage 90 responds uniquely because it is situated near the coast and at the outlet of the study area. The elevated water stages observed in Figure 6D suggest the wetlands in storage area 96 accumulate more water for the 2,100 scenario. Consequently, the wetland becomes submerged due to SLR and its influence on the groundwater mound, thereby functioning as surface-water storage. The storage elevation does not fluctuate significantly because the subsurface water table in the area remains high (Messerli et al., 2000).
As sea levels rise, they exert an impact on coastal aquifers, causing groundwater levels to increase. This rising groundwater can contribute to flooding in low-lying areas, as saturated soils struggle to absorb additional rainfall. The combination of elevated sea and groundwater levels increase the risk of coastal flooding, particularly during heavy storms or high tides. The pond and wetland systems in the Bonita Bay communities exemplify effective stormwater management within the community. Strategically designed and integrated into the landscape, these systems serve as vital components for managing runoff.
Figure 7 indicates the flooding areas in Bonita Bay, considering factors such storm severity, rising sea level, groundwater fluctuation, and land use changes. The simulation showed flooding increases steadily with stronger storms. The flooded area increased from 12.3% for an average annual storm to 14, 16, and 18.4% for storms with 10, 25, and 100-years return periods, respectively. This consistent increase of 2% per severity level shows the storm severity has significant impact in changing the flooded areas. Other studies also suggested that storm severity is likely the most significant factor influencing urban catchments flooding (Viglione and Blöschl, 2009). Furthermore, efficiently designed ponds in this well-developed project effectively capture excess floodwater which is in line with other findings conducted in southwest Florida (Davtalab et al., 2020; Duke et al., 2024). Figure 6 demonstrates this critical role, with pond levels rising to eight feet during the event.
Figure 7. Current (2024) and future (2100) flood maps considering the existing land use, colors are overlaid one on top of the other to separate 2024 and 2,100.
While specific flooded areas may differ (Figure 7 a-d), the overall percentage of inundated land in Bonita Bay is projected to rise by 2,100. The data predicts an average increase of 5% compared to today (2024), indicating an additional 5% of Bonita Bay will be susceptible to flooding. This translates to a progressive rise, with flooding areas reaching 19.6, 20.8, and 22.5% for 10, 25, and 100-years/24-h design storms, respectively. The current extent of flooding underscores the effectiveness of the community’s well-developed infrastructure and sustainable drainage practices. As the flood drivers are exacerbated by human induced change, flooding in Florida is expected to increase significantly in the future (Sweet et al., 2022).
The study provides valuable insight to the growing concerns of flooding due to the combined effects is critical. It demonstrated the interaction between various flood drivers—specifically rainfall intensity, land use changes, SLR, and groundwater rise—through comprehensive scenario simulations. It highlighted how increased impervious surfaces from land use changes can exacerbate flooding, especially during intense storms. The simulations showed that flooding areas significantly increased with higher storm severities, illustrating the cumulative impact of these drivers. Additionally, rising groundwater levels from SLR can lead to higher pond storage levels and contribute to surface flooding. By comparing current conditions to projected scenarios for 2,100, the study provided clear evidence of these interactions, particularly in hydrologically sensitive areas like Bonita Bay.
Most coastal areas face similar challenges, including land use changes, rising sea levels, groundwater fluctuations, and varying rainfall patterns. These factors contribute to complex flood dynamics that necessitate effective management strategies. The modeling approach presented in this study is designed to be replicable in coastal regions worldwide. Its adaptable framework integrates GIS, hydrological, and hydraulic models, allowing customization based on local topography, land use, and hydrological conditions. By utilizing widely available datasets and scenario-based simulations, this interdisciplinary approach enhances the understanding of flood dynamics. This methodology not only yields valuable scientific insights but also provides practical applications for flood risk management, making it applicable to various coastal areas facing similar challenges.
The insights from the outcomes can also be applied in other regions. For example, the spatial maps depicted in Figure 7 are helpful for identifying evacuation routes, locating impacted critical infrastructure, and effectively planning and allocating resources. These maps play a crucial role in communicating flood risks to stakeholders, policymakers, and the public, ultimately empowering informed decision-making to safeguard communities from flood hazards. These insights can inform future flood mitigation strategies by emphasizing the need for integrated management approaches that consider multiple flood drivers. Policymakers and planners can use this information to design adaptive infrastructure, enhance stormwater management, and implement land use regulations that address the cumulative impacts of climate change and urban development on flood risk.
The developed method is applied to the Bonita Bay area, Florida, and predicted current and future flooding risks. However, some coastal areas, like Bonita Bay, face a significant threat of flooding due to a combination of hurricanes, extreme meteorological events, rising sea and groundwater levels, and land-use changes associated with urban expansion. While we have quantified the combined effects of design storms, rising sea and groundwater levels, and land-use change, the impact of Hurricanes associated with high winds and storm surges remains uncertain. Further development of the method may help address this gap and offer valuable insights for coastal areas facing the impacts of hurricanes. Additionally, the proposed modeling approach lacks uncertainty analysis. It mainly relies on regional climate models to predict a range of possible deterministic future scenarios. Future refinements of the method and model could benefit from incorporating uncertainty modeling and sensitivity analysis, which would strengthen the robustness of flood risk assessments.
Managing flood risk is a global challenge due to the complex interaction between various flood drivers. This study developed and tested a comprehensive method integrating GIS and hydrology-hydraulic modeling approaches to assess the individual and combined impacts of flooding from precipitation, land use change, groundwater fluctuations, and SLR. The integration of GIS and hydrology-hydraulic model offers a flexible approach for evaluating multiple drivers with minimal change much to model setups. The synergy between GIS and the hydrology-hydraulic model facilitates an iterative process of scenario development enabling assessment of multiple options. This approach provides two significant advantages. It quantifies flood inundation areas for specific drivers by maintaining the geometric and hydraulic configuration. It offers flexibility in scenario development to visualize the impacts of individual and combined flood drivers. These features are crucial for the evaluation of current and future flood predictions and effective mitigation strategies.
To test the method applicability, the StormWise-GIS modeling approach was developed and applied to the Bonita Bay incorporating four flood drivers that are design storm, land use change, sea level and associated groundwater level rises. Spatial data including DEM, soil, land use, rain zone, and drainage infrastructure were analyzed using ArcMap and integrated into the StormWise model. After incorporating all the input data and developing the hydrologic and hydraulic processes, simulations were conducted. The result shows realistic flood maps that reflect the combined effect of the drivers. The sea and groundwater level rise increases the initial water storage, while runoff from the design storm is exacerbated by the land use. The model predicts a 2% increase of flooded area due to more intense design storm, and approximately 5% increase by 2,100 compared to current condition. While the method effectively considers multiple flood drivers, further investigation is needed to understand the impact of Atlantic Hurricanes on Bonita Bay flooding patterns.
This study serves as a foundational step toward assessing a large combination of drivers and creating more accurate predictions of flooding. It enables water engineers, planners, communities, and policymakers to make informed decisions regarding flood preparedness and insurance. Furthermore, understanding combined flood risk zones through these maps enables communities to proactively develop tailored mitigation strategies, enhancing their resilience to future flood events.
The original contributions presented in the study are included in the article/supplementary material, further inquiries can be directed to the corresponding author/s.
SNT: Conceptualization, Data curation, Investigation, Methodology, Project administration, Supervision, Writing – original draft, Writing – review & editing. MK: Formal analysis, Methodology, Validation, Visualization, Writing – original draft, Writing – review & editing. KA: Formal analysis, Software, Writing – review & editing, Validation. TM: Methodology, Writing – review & editing. SRT: Methodology, Writing – review & editing. AE: Methodology, Writing – review & editing.
The author(s) declare that financial support was received for the research, authorship, and/or publication of this article. The first and last authors were partially supported by the U.A. Whitaker College of Engineering Head-Start Program, Backe Chair Foundation, U.S. Environmental Protection Agency (EPA) Award Number AWD-03D09024, and Bonita Bay Community Association.
We extend our sincere gratitude to Mr. Thomas Singleton for his insightful advice based on his practical real-world experience and to Mr. Pete Singhofen, Streamline Technologies, for generously providing access to StormWise Software and invaluable guidance on integrated simulation. This support was pivotal in conducting a comprehensive analysis of the combined effect of SLR, precipitation, groundwater dynamics, and land use impacts in the study area.
The authors declare that the research was conducted in the absence of any commercial or financial relationships that could be construed as a potential conflict of interest.
All claims expressed in this article are solely those of the authors and do not necessarily represent those of their affiliated organizations, or those of the publisher, the editors and the reviewers. Any product that may be evaluated in this article, or claim that may be made by its manufacturer, is not guaranteed or endorsed by the publisher.
Aguiar, C. R., Nuernberg, J. K., and Leonardi, T. C. (2020). Multicriteria GIS-based approach in priority areas analysis for sustainable urban drainage practices: a case study of Pato Branco, Brazil. Eng 1, 96–111. doi: 10.3390/eng1020006
Andersen, T. K., and Marshall Shepherd, J. (2013). Floods in a changing climate. Geogr. Compass 7, 95–115. doi: 10.1111/gec3.12025
Bosserelle, A. L., Morgan, L. K., and Hughes, M. W. (2022). Groundwater rise and associated flooding in coastal settlements due to sea-level rise: a review of processes and methods. Earth’s. Future 10:2580. doi: 10.1029/2021EF002580
Breinl, K., Lun, D., Müller-Thomy, H., and Blöschl, G. (2021). Understanding the relationship between rainfall and flood probabilities through combined intensity-duration-frequency analysis. J. Hydrol. 602:126759. doi: 10.1016/j.jhydrol.2021.126759
Davtalab, R., Mirchi, A., Harris, R. J., Troilo, M. X., and Madani, K. (2020). Sea level rise effect on groundwater rise and stormwater retention pond reliability. Water 12:1129. doi: 10.3390/w12041129
De Sherbinin, A., Schiller, A., and Pulsipher, A. (2012). “The vulnerability of global cities to climate hazards” in Adapting cities to climate change (New York, USA: Routledge), 129–157.
Duke, L. D., Mullen, M. N., Unger, K. E., Rotz, R., and Thomas, S. (2024). Flood mitigation: regulatory and hydrologic effectiveness of multicomponent runoff detention at a Southwest Florida site. JAWRA 60, 189–210. doi: 10.1111/1752-1688.13157
Fang, J., Wahl, T., Fang, J., Sun, X., Kong, F., and Liu, M. (2021). Compound flooding potential from storm surge and heavy precipitation in coastal China: dependence, drivers, and impacts. Hydrol. Earth Syst. Sci. 25, 4403–4416. doi: 10.5194/hess-25-4403-2021
Fuller, R. A., and Irvine, K. N. (2010). Interactions between people and nature in urban environments. (ed. Gaston K.J.) (UK: Cambridge University Press), 134–171.
Goodchild, M. F., and Haining, R. P. (2004). GIS and spatial data analysis: converging perspectives. Fifty Years Reg. Sci.. 83, 363–385. doi: 10.1007/978-3-662-07223-3_16
Howland, M. D., and Thompson, V. D. (2024). Modeling the potential impact of storm surge and sea level rise on coastal archaeological heritage: a case study from Georgia. PlosOne 19:e0297178. doi: 10.1371/journal.pone.0297178
Jonkman, S. N. (2005). Global perspectives on loss of human life caused by floods. Nat. Hazards 34, 151–175.
Kumar, V., Ranjan, D., and Verma, K. (2021). “Global climate change: the loop between cause and impact” in Global climate change (Elsevier, Amsterdam, Netherlands: Elsevier), 187–211.
Long, Z.-Y., and Gao, L. (2023). Estimating the combined risks of sea level rise and storm surges using a numerical model: application to Macao. J. Clean. Prod. 407:137155. doi: 10.1016/j.jclepro.2023.137155
Machusick, M., Welker, A., and Traver, R. (2011). Groundwater mounding at a storm-water infiltration BMP. J. Irrig. Drain. Eng. 137, 154–160. doi: 10.1061/(ASCE)IR.1943-4774.0000184
McLennan, M. (2022). The global risks report 2022 17th edition. Available at: http://tatsigroup.com/fa/wp-content/uploads/2022/02/the-global-risks-report-2022.pdf (Accessed June 15, 2024).
Messerli, B., Grosjean, M., Hofer, T., Nunez, L., and Pfister, C. (2000). From nature-dominated to human-dominated environmental changes. Quat. Sci. Rev. 19, 459–479. doi: 10.1016/S0277-3791(99)00075-X
Missimer, T. M. (1992). Stratigraphic relationships of sediment facies within the Tamiami formation of Southwest Florida: proposed intraformational correlations. Plio-Pleistocene Stratigraphy Paleontol. South. Florida 36, 63–92.
Missimer & Associates, Inc., (1981). Groundwater resources of the Bonita Bay Development, vols. 1 and 2. Consultants report to David B. Shakarian & Associates, Inc. Cape Cora, Florida, USA.
NOAA. (2018). NOAA: Data Access Viewer, Geospatial data discovery and access tool with custom download options for elevation (lidar), land cover, imagery, and other data. Available at: https://www.coast.noaa.gov/dataviewer/#/lidar/search/ (Accessed May 17, 2024).
NOAA. (2024). National Oceanic and Atmospheric Administration. Available at: https://www.noaa.gov/ (Accessed May 17, 2024).
Peker, İ. B., Gülbaz, S., Demir, V., Orhan, O., and Beden, N. (2024). Integration of HEC-RAS and HEC-HMS with GIS in flood modeling and flood hazard mapping. Sustain. For. 16:1226. doi: 10.3390/su16031226
Peter Sheng, Y., Paramygin, V. A., Yang, K., and Rivera-Nieves, A. A. (2022). A sensitivity study of rising compound coastal inundation over large flood plains in a changing climate. Sci. Rep. 12:3403. doi: 10.1038/s41598-022-07010-z
Rahimi, R., Tavakol-Davani, H., Graves, C., Gomez, A., and Fazel Valipour, M. (2020). Compound inundation impacts of coastal climate change: sea-level rise, groundwater rise, and coastal precipitation. Water 12:2776. doi: 10.3390/w12102776
Rawls, W. J., Brakensiek, D. L., and Miller, N. (1983). Green-ampt infiltration parameters from soils data. J. Hydraul. Eng. 109, 62–70. doi: 10.1061/(ASCE)0733-9429(1983)109:1(62)
Rawls, W. J., Brakensiek, D. L., and Saxtonn, K. E. (1982). Estimation of soil water properties. Transact. ASAE 25, 1316–1320. doi: 10.13031/2013.33720
Saksena, S., Merwade, V., and Singhofen, P. J. (2019). Flood inundation modeling and mapping by integrating surface and subsurface hydrology with river hydrodynamics. J. Hydrol. 575, 1155–1177. doi: 10.1016/j.jhydrol.2019.06.024
Saksena, S., Merwade, V., and Singhofen, P. J. (2021). An alternative approach for improving prediction of integrated hydrologic-hydraulic models by assessing the impact of intrinsic spatial scales. Water Resour. Res. 57:e2020WR027702. doi: 10.1029/2020WR027702
Schroeder, D. W., Tsegaye, S., Singleton, T. L., and Albrecht, K. K. (2022). GIS- and ICPR-based approach to sustainable urban drainage practices: case study of a development site in Florida. Water 14:1557. doi: 10.3390/w14101557
Sempewo, J. I., Kyeyune, J., Nyenje, P. M., Nkwasa, A., Mugume, S. N., Tsegaye, S., et al. (2024). Distinct and combined impacts of future climate and land use change on the flow of river Rwizi in Uganda, East Africa. J. Water Climate Change 15, 1667–1692. doi: 10.2166/wcc.2024.542
SFWMD. (2016). South Florida water Management District, information Technology bureau geospatial services unit, land cover land use 2014–2016. Available at: https://geo-sfwmd.hub.arcgis.com/datasets/sfwmd::sfwmd-land-cover-land-use-2014-2016/about (Accessed March 12, 2024).
SFWMD. (2024). South Florida water Management District, open data, LWC Hydrogeologic unit mapping—Contours by aquifer (arcgis.com), South Florida water Management District, West Palm Beach, FL, USA. Available at: https://hub.arcgis.com/datasets/43ec9df314f94996bd3f847a61b4e96e_0 (Accessed March 12, 2024).
SFWMD. (2024). South Florida water Management District, surface water management data on the Bonita Bay development. South Florida Water Management District, West Palm Beach, FL, USA, 2024. https://www.sfwmd.gov/
Shrestha, A., Howland, G. J., and Chini, C. M. (2023). A review of climate change-induced flood impacts and adaptation of coastal infrastructure systems in the United States. Environ. Res. 3:042001. doi: 10.1088/2634-4505/ad097b
SSURGO. (2024). Soil Survey Geographic Database (SSURGO) | Natural Resources Conservation Service. Available at: https://www.nrcs.usda.gov/resources/data-and-reports/soil-survey-geographic-database-ssurgo
Streamline Technology. (2018). Streamline technologies: ICPR4 technical reference manual—Google scholar. Available at: https://scholar.google.com/scholar_lookup?title=ICPR4%20Technical%20Reference%20Manual&author=Streamline%20Technologies&publication_year=2018 (Accessed February 26, 2024).
Su, F., Kaplan, D., Li, L., Li, H., Song, F., and Liu, H. (2017). Identifying and classifying pollution hotspots to guide watershed management in a large multiuse watershed. Int. J. Environ. Res. Public Health 14:260. doi: 10.3390/ijerph14030260
Sukop, M. C., Rogers, M., Guannel, G., Infanti, J. M., and Hagemann, K. (2018). High temporal resolution modeling of the impact of rain, tides, and sea level rise on water table flooding in the Arch Creek basin, Miami-Dade County Florida USA. Sci. Total Environ. 616-617, 1668–1688. doi: 10.1016/j.scitotenv.2017.10.170
Sweet, W. V., Hamlington, B. D., Kopp, R. E., Weaver, C. P., Barnard, P. L., Bekaert, D., et al. (2022). Global and regional sea level rise scenarios for the United States: Updated mean projections and extreme water level probabilities along US coastlines. Silver Spring, Maryland, USA: National Oceanic and Atmospheric Administration.
Tenali, S., and McManus, P. (2022). Climate change acknowledgment to promote sustainable development: a critical discourse analysis of local action plans in coastal Florida. Sustain. Dev. 30, 1072–1085. doi: 10.1002/sd.2301
Viglione, A., and Blöschl, G. (2009). On the role of storm duration in the mapping of rainfall to flood return periods. Hydrol. Earth Syst. Sci. 13, 205–216. doi: 10.5194/hess-13-205-2009
Wang, P., Royer, E. L., Jackson, K., and Gutierrez, S. (2024). Impacts of hurricane Ian along the low-lying Southwest Florida coast (USA) in 2022: lessons learned. J. Coast. Res. doi: 10.1007/978-3-031-63300-3
Keywords: combined factor flooding assessment, sea level rise, groundwater table rise, impervious surfaces, StormWise and GIS modeling, hydrology-hydraulic modeling
Citation: Tsegaye S, Kebedew MG, Albrecht KK, Missimer TM, Thomas S and Elshall AS (2024) Integrated GIS-hydrologic-hydraulic modeling to assess combined flood drivers in coastal regions: a case study of Bonita Bay, Florida. Front. Water. 6:1468354. doi: 10.3389/frwa.2024.1468354
Received: 21 July 2024; Accepted: 08 October 2024;
Published: 25 October 2024.
Edited by:
Akhouri Pramod Krishna, Birla Institute of Technology, Mesra, IndiaReviewed by:
Maryem Ismaili, Université Sultan Moulay Slimane, MoroccoCopyright © 2024 Tsegaye, Kebedew, Albrecht, Missimer, Thomas and Elshall. This is an open-access article distributed under the terms of the Creative Commons Attribution License (CC BY). The use, distribution or reproduction in other forums is permitted, provided the original author(s) and the copyright owner(s) are credited and that the original publication in this journal is cited, in accordance with accepted academic practice. No use, distribution or reproduction is permitted which does not comply with these terms.
*Correspondence: Seneshaw Tsegaye, c3RzZWdheWVAZmdjdS5lZHU=
Disclaimer: All claims expressed in this article are solely those of the authors and do not necessarily represent those of their affiliated organizations, or those of the publisher, the editors and the reviewers. Any product that may be evaluated in this article or claim that may be made by its manufacturer is not guaranteed or endorsed by the publisher.
Research integrity at Frontiers
Learn more about the work of our research integrity team to safeguard the quality of each article we publish.