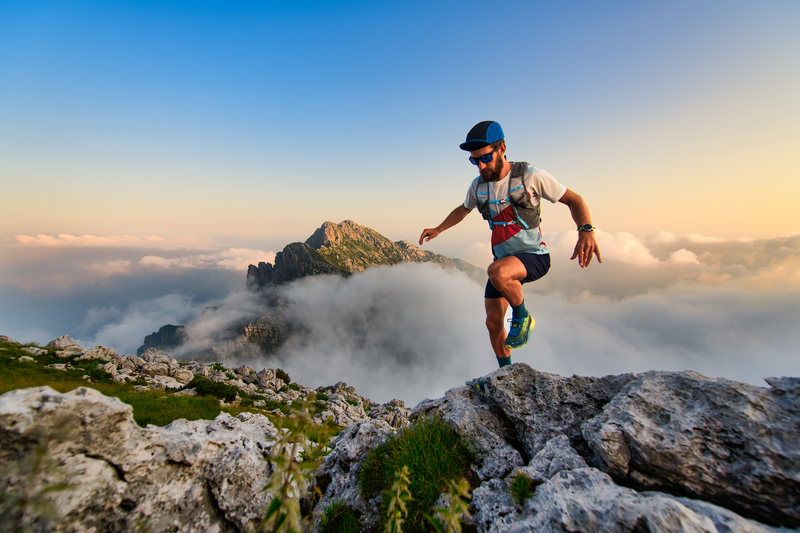
95% of researchers rate our articles as excellent or good
Learn more about the work of our research integrity team to safeguard the quality of each article we publish.
Find out more
ORIGINAL RESEARCH article
Front. Water , 11 December 2024
Sec. Water and Climate
Volume 6 - 2024 | https://doi.org/10.3389/frwa.2024.1442023
This article is part of the Research Topic Economic Resilience through Water Resilience: Managing and Evaluating Economies for Uncertainty and Change View all articles
A broad set of tools, frameworks, and guidance documents are available for water resources project planning, design, evaluation, and implementation in an ever-evolving world. The principles underlying most of these resources aim to advance the practice of water systems engineering under uncertainty, preserve and enhance project benefits, and achieve investment goals. Approaches to financial and economic evaluation under climate uncertainty in civil infrastructure investments, in particular, are currently being reviewed by academics and practitioners in the field to assess their ability to deliver resilience, sustainability, and equity. In climate-sensitive projects, adaptation measures that help mitigate the adverse effects of climate change and preserve project benefits are required, and stakeholder willingness-to-pay (WTP) for these must be assessed. Typically, stakeholders and decision-makers utilize the outcomes of economic assessment methods such as cost–benefit analysis (CBA) to justify large capital investments. Synthesizing previous advancements in water resources planning and evaluation, this study illustrates how a CBA framework can be augmented by applying a Climate-informed Robustness Index (CRI). The analytics underpinning the CRI, as well as the summary metric itself, help characterize project climate vulnerability, while conducting CBA with and without potential adaptation measures can be used to estimate WTP of investors for adaptation to the identified climate vulnerabilities. The case study of a planned irrigated agriculture project in Lesotho highlights critical climate conditions for which adaptation measures such as integrated catchment management (ICM) plans can be introduced to safeguard project robustness.
Modern water resources planning draws on centuries of historical efforts to provide guidelines, outline principles, and develop reproducible methods for project planning, assessment, evaluation, and implementation. Maass et al. (1962) presented techniques for relating economic objectives, engineering analysis, and government planning in the design of water resources systems. This study set the stage for structured, analytical approaches to water resource project planning and evaluation in practice. The acceleration of global climate change (Dangendorf et al., 2019) and an improved understanding of water project risks under climate uncertainty (Ray and Brown, 2015; Kundzewicz et al., 2018; Borgomeo et al., 2018; Ray et al., 2019; Tsakiris and Loucks, 2023) have motivated additional attention to the study of economic assessment, in particular cost–benefit analysis (CBA), under climate uncertainty.
CBA is a systematic approach to evaluating proposed investments in terms of benefits provided relative to costs incurred so as to promote efficient and effective allocation of financial resources, rank alternatives, or report the expected net benefit of a single project (FAO, 2018). A project for which the benefits exceed the costs represents a potentially Pareto-improving1 investment, in the sense that while it may create winners and losers, the overall value created would be sufficient for the winners to fully compensate those who are losing and still remain better off (Bakkoury et al., 2004). Metrics from CBA evaluation such as the economic rate of return (ERR2) or net present value (NPV) compare the costs and benefits of investments to facilitate decision support. The ERR is the discount rate at which the economic costs and benefits of a project are equal. The NPV is the difference between the present value of discounted investment costs and benefits. While the NPV and ERR are commonly used in practice for project appraisal, the present value (PV) of investment benefits (independent of costs) provides information on the current value of a future sum of projected benefits and therefore implies a willingness-to-pay (WTP) for the benefits provided by the project, or for incremental design adaptations.
An individual’s WTP is defined as the maximum amount a fully informed buyer would pay to acquire a defined good or service (Gall-Ely, 2009). However, for public projects, the sum of societal members’ WTP provides key information about whether a project is worth executing. For incremental investment adaptation elements of climate-sensitive projects like water resources infrastructure, the WTP is the maximum additional amount (beyond baseline capital costs) that stakeholders would be willing to invest in the project (including improvement to the original design) to promote robust performance, preserve projected benefits, and achieve overall investment goals throughout a range of uncertain potential future climate conditions. In a climate change planning paradigm, estimating an economically consistent WTP as the PV of benefits becomes expedient for projects, which are identified as vulnerable, and can be used to compare against the cost of adaptations.
The need to identify WTP for adaptations to a base design can be informed by summarizing the robustness of the project performance across a range of climate futures. The robustness index (RI), a generalized metric ranging between 0 and 1, represents the fraction of potential futures in which a project achieves an acceptable level of performance, thus providing a decision-relevant indicator as part of uncertainty characterization in CBA (Moody and Brown, 2013). The Climate-informed Robustness Index (CRI) goes a step further than uniform RI (which assumes equal likelihood of alternative scenarios) by attaching likelihood information regarding climate futures, e.g., Taner et al. (2019), from general circulation models (GCMs) (Wilby and Wigley, 1997), as weights to the RI. The CRI and other approaches to climate-investment vulnerability assessment such as climate risk-informed decision-making (CRIDA) (Jeuken et al., 2016; Mendoza et al., 2018), decision scaling (Brown et al., 2012), and robust decision-making (RDM) (Lempert et al., 2010; Lempert, 2019) identify project vulnerabilities for which adaptations are necessary, and adequate budgets must be prepared.
When the CRI indicates sufficient vulnerability to investigate adaptations, WTP estimates can support more accurate budget allocations for contingency funds in project planning under climate uncertainty. If accurately calculated, WTP estimates indicate an upper bound on the incremental expenditures that should be considered when redesigning the project or implementing adaptation solutions such as integrated catchment management (ICM) measures (Batchelor, 1999). Consequently, the CRI enables stakeholders to make timely decisions now that ensure acceptable project performance over a wide range of possible future conditions. In cases where engineering or political conditions limit potential for significant adjustments or additional investments during the operation phase (as is the situation for our case study), improving robustness in the initial design is likely more appropriate than other methods such as real options analysis (ROA) (Wreford et al., 2020) and adaptation pathways (AP) (Buurman and Babovic, 2016) that emphasize flexibility over robustness and are dependent on future “tipping points,” which could redefine the approach to adaptation.
While it is conceptually straightforward to generate evaluation metrics such as NPV and ERR, in practice, uncertainty about future climatic conditions that may affect project performance poses challenges when trying to utilize them to inform decisions (Lempert et al., 2004; De Boer et al., 2010). Water projects are sensitive to prevailing climate conditions (Kazemi Garajeh et al., 2024a) and weather extremes (e.g., floods, droughts, heat waves), and estimation of their costs and benefits for economic evaluation is contingent on the assumptions made about future climate conditions. This introduces a limitation to the application of the ERR or NPV for project appraisal, or the use of the PV of benefits as an estimate of WTP (Arndt et al., 2011; Chambwera and Stage, 2010). Without a proactive stance toward the integration of vulnerability assessment using methods like the CRI into the economic assessment of climate-sensitive infrastructure projects, investment decisions may be based on a weak or insufficient understanding of project uncertainties, and approved projects could be under- or over-designed, or worse, maladaptive to climate hazards.
To address this, the methodology of study synthesizes previous advancements in water resources planning and evaluation and pairs a CRI approach to a two-step workflow for CBA under uncertainty. The CRI provides an indication of whether a base project design has sufficient climate vulnerability to merit design adaptations for climate robustness or whether it is sufficiently climate-robust. If merited, the performance of potential adaptation is evaluated under a wide range of climate futures, and the difference in expected performance provides the PV of the investment benefits, in effect imputing the stakeholders’ WTP for the adaptation measure being evaluated. The methodology is applied to a case study of irrigation investment in Lesotho. Initial assessment of the baseline (business as usual) scenario using the CRI showed that the investment is vulnerable to climate change in all the 28 climate scenarios analyzed and so requires some form of adaptation to preserve projected benefit streams. By examining an integrated catchment management (ICM) scenario, results show that adaptations could lead to expected incremental benefits of $0.03 million and up to $0.13 million of benefits depending on which of the climate scenarios examined is realized. Due to limitations in estimation costs, choice of the discount rate, and modeling assumptions carefully outlined in the paper, our analysis is not definitive in its conclusions about the proposed investment. However, the case study illustrates a complete workflow in a real project context that demonstrates the integration of climate uncertainty analysis into multi-model, economic evaluation frameworks, highlighting value, feasibility, and challenges related to this underutilized mode of analysis. The rest of the paper reviews the literature on economic evaluation under climate change in water resources and then presents the methodology followed by the case study and results. Afterward, we discuss the results, highlight limitations to the approach of study, and recommend further research specifically in the area of distributional equity.
In CBA, a hurdle rate for the ERR is typically set as a break-even condition (typically 6–12% in the context of international development) to appraise the efficiency of resource allocation (Brigham, 1975; Poterba and Summers, 1995; Meier and Tarhan, 2007). This break-even condition is equivalent to having a positive NPV when the discount rate is set equal to the hurdle rate. However, while the ERR and other threshold-informing CBA metrics can be calculated with ranges or statistical distributions, they are often reported to decision-makers as point estimates. This collapsing of information masks the influence of uncertainties such as climate change that affect water budgets used in modeling and quantifying water project costs and benefits (World Bank Independent Evaluation Group 2010; Wegner and Pascual, 2011). When these metrics are presented as point estimates, decision-makers are left without an understanding of the variance of the chosen financial metric across a range of possible future climate conditions.
Well-known analytical methods of uncertainty characterization have been introduced to CBA studies to address these concerns (Table 1). Sensitivity analysis and scenario analysis focus on preliminary identification and characterization of sources of uncertainty. For instance, Hallegatte (2006) used sensitivity analysis to assess the uncertainty added by climate change to infrastructure design, which could increase the probability of either under-adaptation (and increased risk) or over-adaptation (and sunk costs) in their CBA study of the New Orleans Flood Protection System. Denver Water used scenario analysis in the development of its infrastructure investment program by identifying three key uncertain variables: climate warming, economic growth, and urban versus suburban preference, which were combined to create five easy-to-interpret scenarios for performance evaluation (Denver Water, 2018). Other methods, such as Monte Carlo analysis, are used in combination with sensitivity analysis and scenario analysis to explore uncertainty levels, propagate uncertainty through models, or trace and rank sources of uncertainty. Asplund and Eliasson (2016) used Monte Carlo Analysis to manage the uncertainty in transport infrastructure investment CBA. In this study, sensitivity analysis identified investment costs and transport demands as the key risk parameters, while Monte Carlo Analysis investigated the range of uncertainty presented by the risk parameters using probability density functions. The results showed that uncertainties associated with investment costs and transport demands could lead to a reduction of 5–15% of net benefits. The range of net benefits calculated by Asplund and Eliasson (2016) provides the decision-maker with a better understanding of climate-related risks to the proposed investment; however, the rigorous application of sensitivity analysis, scenario analysis, and/or Monte Carlo Analysis to characterize climate change-related risks to infrastructure investments is often lacking (or insufficient) in practice.
For more confident decisions, methods that characterize and propagate uncertainty through the workflow and, when possible, use results to reduce the sensitivity of a project to uncertainty are recommended (Refsgaard et al., 2007). Typical foreign aid development agencies like the World Bank, Asian Development Bank (ADB), Millennium Challenge Corporation (MCC), and United States Agency for International Development (USAID) use these uncertainty characterization methods while appraising proposed projects. A survey of the CBA spreadsheets of 82 projects from closed MCC compacts executed between 2005 and 2020 (see Supplementary material) showed that sensitivity analysis was conducted on project costs and benefits, and other project-specific uncertainties such as water demand, hectares of cropland cultivated and storage capacity of planned infrastructure. With-project and without-project scenarios (storylines) were also analyzed in line with standard economic practice. However, none of the 82 projects explored the consideration of alternate climate scenarios as part of scenario analysis; rather, they used scenario analysis to evaluate the impact on local economic outcomes of interest using with-project and without-project storylines. Furthermore, only 50% of the surveyed projects from MCC compacts indicate that a Monte Carlo analysis, in addition to sensitivity analysis, was conducted on CBA metrics such as the ERR, NPV, and PV to assess the robustness of investment under uncertainty.
Like the MCC, the ADB recommends the conventional approaches of sensitivity analysis and probabilistic/risk analysis (Monte Carlo analysis) as well as alternative approaches such as scenario analysis, real options analysis (Wang et al., 2019), and robust decision-making (RDM) (Lempert et al., 2010; Lempert, 2019) for uncertainty characterization. The World Bank, USAID, and other agencies like the United States Army of Corp Engineers (USACE) and the National Institute of Standards and Technology (NIST) all use conventional sensitivity analysis for preliminary risk assessment in CBA (Hallegatte et al., 2012, 2021; Gilbert et al., 2015) and recommend Monte Carlo Analysis as an additional form of risk analysis. Synthesizing from this survey of compact evaluation, sensitivity analysis is widely adopted in assessments of the potential future performance of water resources projects, but in practice, there is generally limited exploration of alternative climate scenarios as part of scenario analysis, and Monte Carlo analysis although recommended, is rarely applied.
Project vulnerability to characterized uncertainties can be understood through graphical summaries, as well as metrics like the RI, that summarize overall performance across the range of uncertainties examined (Moody and Brown, 2013). Using a range of 0 to 1, representing never meeting the criteria or perfectly acceptable performance, respectively, the RI quantifies project robustness under uncertainty and aids comparison of alternative adaptation strategies. The metric is calculated as a ratio of the sum of acceptably performing scenarios to the total number of scenarios in the uncertainty space (effectively assuming equal likelihood for all scenarios or uniform distribution in a continuous uncertainty space). This concept has been extended to sources of climate uncertainty associated with CBA studies to provide insight into the level of project performance under climate (and other) uncertainties (Moody and Brown, 2013; Whateley et al., 2014; Taner et al., 2017; Ray et al., 2018). For instance, in conventional CBA, the RI is computed from sensitivity analysis and Monte Carlo analysis based on uncertainties in costs, benefits, or their drivers which may be project-specific. The CRI further evaluates project performance under a wide range of plausible climate scenarios and weighs the RI with likelihoods of those climate scenarios: that is, it incorporates a variety of likelihood information (e.g., Taner et al., 2019) in its translation of a scenario-neutral robustness measure (RI) into a climate-informed robustness measure. The CRI has been applied to the economic evaluation approaches of institutions such as the World Bank (Ray and Brown, 2015; Ray et al., 2019; Rodríguez et al., 2021), UNESCO (Mendoza et al., 2018), the California Department of Water Resources (Schwarz et al., 2018; Ray et al., 2020), and the International Hydropower Association (2019).
However, uptake of the CRI in decision-making has been limited, particularly within the CBA sphere. In the MCC projects surveyed, the RI concept (not even considering climate elements) was referenced in 12% of the sampled project CBAs from prior to 2020, although updated guidance published in 2021 requires the use of the RI. While the use of confidence intervals rather than point estimates has been encouraged by some of the development institutions surveyed, ranges of CBA metrics resulting from uncertainty characterization, and robustness metrics computed from such ranges, are often not reported to stakeholders alongside the expected value for holistic decision-making under uncertainty. Our examination of publicly available spreadsheets of CBA studies found that they usually do not include uncertainty ranges or robustness metrics like the RI on their summary pages. This paper aims to demonstrate the process of estimating a CRI as well as the utilization of climate-informed likelihoods to estimate the value of incremental investments to improve project performance in the face of uncertain climate change.
To compute a CRI from CBA results, we integrate decision scaling principles (Brown et al., 2012) including decision framing, the climate stress test, and estimation of climate-informed risks, into the standard economic evaluation process. Decision scaling utilizes a decision-analytic framework to first identify the climate conditions to which the project performance is vulnerable, and then supplement the scenario-neutral vulnerability assessment with likelihood information derived from a study of the most up-to-date climate science (and projections), analysis of historical patterns and trends, and local expert opinions (Brown et al., 2012). Specifically, with the climate stress test, an analyst develops a two-dimensional representation of project performance under different climate futures, which can then be used to inform specific climate vulnerabilities and associated design adaptations. By introducing these principles into the workflow of conventional economic assessment methods, we provide a climate-informed basis for uncertainty characterization and investment vulnerability assessment and subsequently improved decisions in water project planning and management.
This paper defines project vulnerabilities using the CRI and contributes to literature by mapping out a novel, targeted process by which stakeholders’ WTP for robustness-improving adaptations can be estimated. The methodology presented in the next section covers techniques of uncertainty characterization used in typical CBA studies and adopts a framework that connects hydrologic/hydraulic models, climate vulnerability assessments, and economic evaluation techniques that typical development agencies utilize during compact development.
Figure 1 presents the study methodology. In Step 1, we screen for climate hazards using mostly qualitative and categorical mechanisms. In Step 2, we identify the modeling tools and algorithmic workflows needed to conduct a climate stress test for our particular case. In Step 3, the climate stress test is executed, and visualizations of the results are developed. In Step 4, relevant thresholds that define acceptable performance are selected and the CRI, along with other distribution information on CBA metrics, is calculated using likelihood information from GCMs (Wilby and Wigley, 1997). In Step 5, we estimate the WTP for a potential adaptation under a range of climate scenarios generated by the stress test using the PV of benefits from CBA calculations. Brown et al. (2024) present a comprehensive description of these steps in their guidance document for achieving robust project design through iterative climate-informed performance assessment, developed under a partnership with the Millennium Challenge Corporation.
The climate hazard screening is conducted in collaboration with stakeholders, using readily accessible screening tools such as the World Bank’s resilience booster tool (Ospina and Rigaud, 2021) and climate and disaster risk screening tool (Kull et al., 2016), the USAID’s climate risk screening and management tools (Hammill and Tanner, 2011), and the International Institute for Sustainable Development’s CRiSTAL tool (Keller et al., 2013; Zamudio, 2016). This step identifies potential climatic and non-climatic threats to project performance (Phan et al., 2018; Xiong et al., 2022; Kazemi Garajeh et al., 2024b), taking into consideration stakeholder-defined performance indicators, acceptability thresholds, project location, and useful lifespan, among other factors. In climate-sensitive projects, screening for hazards by considering changes to variables such as temperature, precipitation, sea level rise, and recurrent intervals of extreme weather events helps identify threats to the project over its expected lifespan that require formal attention in project design and assessment of project performance.
Based on identified climate hazards in Section 3.1, a modeling framework is developed. The requirement for climate-responsive modeling is an integrated model, or set of linked models, that accepts climate-affected variables as inputs and quantifies their impacts on key project performance metrics, including CBA metrics such as the ERR and NPV. While described succinctly here, adapting a pre-existing model to be functionally climate-responsive can be a significant effort. The models should be able to relate climate variables to the outcomes of interest. In the context of this study, costs and benefits are treated as a function of climate variables (precipitation and temperature), thereby linking climate to the outcomes that are relevant to project design, economic evaluation, and decision-making. The modeling framework developed for this study is discussed further in the Case Study section.
The third step makes use of a “climate stress test” to input a range of possible climate scenarios (futures) to the model chain developed in Section 3.2. This way, the models are used to simulate the effects of different climate futures on the key metrics of project, thereby quantitatively evaluating the conditions under which the project is robust, and those under which it is vulnerable. Scenarios for the stress test are typically generated to include the range of plausible changes in mean climate that capture the uncertainty introduced by unknown future anthropogenic activities and several realizations of natural variability. The recommended approach is to create a weather generator (Wilks and Wilby, 1999) designed to generate a large set of climate scenarios that comprehensively explores climate variability and climate change. While the level of sophistication varies, this can be done through the following steps (Steinschneider and Brown, 2013; Steinschneider et al., 2019).
a. Define the range of climate change to be explored in the analysis. The range is selected to ensure all plausible climate changes are included, and this judgment can be based on available climate projections for the region of interest. For context, when looking 30–40 years into the future, varying precipitation from −30 to +30% of the average annual value is often adequate, while the range is typically 0°C to +5°C of warming for temperature.
b. Define the incremental changes in the climate variables to be applied. Typical increments are changes of 10% of the annual average for precipitation and 1°C for temperature. This step and the previous step effectively define a grid over which project performance is sampled.
c. Create scenarios of stochastic climate variability based on the observed historical variability and apply them as inputs for each point in the grid developed above. This involves statistical modeling of the observed variability and Monte Carlo sampling from the resulting autoregressive moving average (ARMA) models of seasonal or interannual variability, Markov chains of daily/weekly transition probabilities, and distribution functions representative of the likelihoods of events of various magnitudes.
The climate vulnerability stress test carefully integrates the methods of uncertainty characterization used in typical economic assessment. To understand the value-add of the stress test in uncertainty characterization, the distinction between epistemic and stochastic (or ontological) uncertainty as defined by Walker et al. (2003) needs to be highlighted. Epistemic uncertainty results from imperfect knowledge of a system and may be reduced by additional studies, for example more data collection and further research as explored by van der Pol et al. (2017). However, stochastic uncertainty, also referred to as aleatory uncertainty by Hallegatte et al. (2012), results from inherent or intrinsic variability in a system and this is the case with climate uncertainty. The weather generator for the climate stress test examines intrinsic variability within a climate scenario by sampling the historical time series using Monte Carlo, thereby identifying historical trends of variability that can inform the projection of future climate for the area of study. The two-way sensitivity analysis and scenario analysis, on the other hand, adopted in generating the alternate scenarios’ temperature and precipitation grid (steps (a) and (b) above), characterize variability among or between plausible climate futures. This way, the weather generator considers a wide range of alternative climate futures informed by known variability in historical climate and based on incremental changes in temperature and precipitation from the specified range to account for likely unknown changes in future climate. The climate stress test is not a substitute for existing methods of uncertainty characterization but rather a more thorough approach to climate uncertainty characterization.
Step 4 characterizes, using likelihood information, the climate conditions under which the project performs acceptably or otherwise using the results of the climate stress test in Section 3.3. To do this, a benchmark for acceptable performance is set; for instance, a minimum amount of streamflow required for irrigation or industrial production, the hurdle rate for investment decision-making, or a specified (net) present value of the investment. Mathematically, following Moody and Brown (2013), the investment is judged to perform acceptably in a given climate future using the binary performance function, ˄(d, X), as in Equation 1. This function computes to 0 for unacceptable performance and 1 for acceptable performance.
where, d is the decision. X is a vector of (climate) uncertainty variables representing a future state, that is, one of the possible climate scenarios generated from the stress test. ˄ (d, X) is the value of the performance metric, in our case the ERR, NPV, or PV of the investment under a given climate scenario.
Uniform RI (Equation 2) is calculated as the integral of the binary performance function, ˄ (d, X), divided by the integral of the (climate) uncertainty space considered, resulting in a fraction between 0 (vulnerable investment) and 1 (robust investment over the entire range of climate scenarios considered) (Moody and Brown, 2013).
where, dxi represents the climate variables—in our case, temperature and precipitation. This can be expanded to accommodate the appropriate number of climate variables for specific studies.
However, Equation 2 assumes an equal likelihood of all the climate scenarios considered in the stress test, which effectively overweighs more extreme scenarios. Attaching likelihood information to climate scenarios to calculate the CRI helps analyze more accurately the investment’s vulnerability to uncertainty under climate change. For instance, climate scenarios from the CMIP6 projections (output of the 6th Coupled Model Intercomparison Project (CMIP6) of the global circulation models (GCMs)) can be used to assess the likelihood of the climate scenarios generated from the stress test and calculate the CRI as in Equation 3.
where, Pr (X) is the probability of a given climate scenario.
In addition, following Moody and Brown (2013) the study used the multivariate normal distribution (parameters of mean, μ and covariance, Ʃ) to model the distribution of climate variables, as shown in Equation 4.
where, X and μ are k-length vectors of climate variables and climate variable means, respectively. Ʃ is the symmetric, positive definite k x k covariance matrix.
The CRI is defined in a decision-focused manner and, when adopted in an economic evaluation of water resources projects, provides a robust approach to uncertainty characterization and investment vulnerability assessment under a changing climate. It also gives sufficient flexibility to update information as the project progresses and new information on uncertainties is observed (Moody and Brown, 2013; Whateley et al., 2014). Importantly, alternative investment options that have CBA metrics of similar expected values but different robustness to climate change can be compared using the CRI. Depending on how the analytical framework is defined, flexibility-emphasizing methods such as real options analysis (ROA) can accommodate climate likelihood information, when the need for climate vulnerability assessment becomes obvious at tipping points. However, by using the CRI, project robustness can be evaluated upfront, thereby facilitating effective adaptation planning.
If the CRI calculated in Section 3.4 reveals unacceptable vulnerability in project performance, then decision-makers will likely wish to consider adaptation measures to increase the robustness of the project. Adaptations include adjustments to systems, processes, practices, and structures in response to actual or expected climatic stimuli or their effects, which moderate damages or exploit benefits (Adger et al., 2005; Berrang-Ford et al., 2011). Proposed methods of adaptation for vulnerability reduction could range from relatively small adjustments to existing project elements such as increasing road culvert size or changing construction material, to significant design changes, such as the inclusion of nature-based solutions (NbS) (Nesshöver et al., 2017; Jeuken et al., 2023) or ICM measures.
For long-term adaptation planning, information on the costs and benefits of adaptation is required (Estrada et al., 2017). As Stern (2007) notes, the gross benefits of adaptations are in the damage avoided while the net benefit of adaptation is the damage avoided less the cost of adaptation, but few adaptation projects have been subjected to robust economic analysis that weighs tradeoffs in investment options (World Bank, 2010). Having identified possible design adaptations, their incremental benefits (relative to the base design) need to be assessed, in effect estimating stakeholders’ WTP for the adaptations beyond the base design costs of the project. This is the additional amount that investors are willing to pay to sustain robust project performance under a changing climate (Freeman et al., 2020). Using the PV of benefits from CBA, the WTP for adaptations can be estimated under each climate scenario of the stress test. This is calculated using two storylines: without adaptations and with adaptations, referred to as the Baseline (business as usual) scenario and ICM scenario, respectively, in the case study. The stakeholders’ WTP is calculated as the difference in PV of benefits between the ICM scenario and the baseline scenario as in Equations 5, 6.
where, PVICM—Present value of investment benefits with adaptations. PVBase—Present value of investment benefits without adaptations. future benefits—projected future benefits of investments. i—discount rate. n—project time horizon.
Lesotho, located between latitudes 28 °S and 31 °S, and longitudes 27 °E and 30 °E, is a mountainous, landlocked country, with a population of approximately 2.3 million and a land area of just over 30,000 m2. High elevations make Lesotho (entirely above 1,000 m), relatively cold with the wet summer season extending from October to April, providing a long-term annual precipitation average of 761.2 mm. Despite having vast water resources, periodic droughts have been part of Lesotho’s recent history (Belle and Hlalele, 2015; Kamara et al., 2019), leading to food insecurity (Verschuur et al., 2007) and disrupting industrial activity.
The MCC signed its second compact with Lesotho in May 2022 (MCC, 2022). This compact consists of three projects, one of which is the Market Driven Irrigation Horticulture (MDIH) project used as our case study. The MDIH project aims to increase rural incomes and fight food insecurity by investing in climate-smart irrigation infrastructure, and attracting commercial farmers to collaborate with local, small-holder farmers to produce high-value crops and build strong value chains (MCC, 2022). Four areas in the Lesotho Lowlands were shortlisted for implementation of the MDIH scheme: Tsoili-Tsoili, Manka, and Likhakeng in the Leribe area which forms a part of the little Caledon River basin; and Phamong (Lithipeng), which is part of the large Orange-Senqu River basin. In each location, the key infrastructure is a small irrigation dam designed to supply water to demarcated cultivated areas. ICM interventions, such as check dams, hill regrading (contours), and waterways, have also been proposed for implementation upstream of the dams to reduce sedimentation and subsequently preserve live dam storage volume for irrigation purposes (AECOM, 2022a). The study focuses on the Phamong irrigation scheme area shown in Figure 2 (one of four), and the analysis is conducted under the Baseline and the ICM scenarios. The latter examines ICM as a possible adaptation strategy that improves project performance and investment robustness in order to estimate WTP of stakeholders.
Figure 2. Phamong sub-basin showing the location of the proposed dam and planned irrigated perimeters (inset A shows the location of Lesotho in Africa, while inset B shows the location of the Phamong Basin in Lesotho).
Figure 3 presents the climate-responsive modeling framework developed for the study, and the following is a summary of the key features of each component of the framework: the hydrologic model, the reservoir model, the agronomy model, and the economic model.
A SWAT (Soil and Water Assessment Tool) hydrologic model (Gassman et al., 2014) was built for the Phamong MDIH sub-basin (Figure 2) using input data recorded in Supplementary material. The small size of the sub-basin meant that pre-existing data sources did not allow for differentiated rainfall patterns, land use (rangeland—grasses), and soil type (clay loam with hydrologic group C), and available water capacity (AWC) = 0.122). The SWAT model disaggregated the sub-basin into 30 unique hydrologic response units (HRUs) based on elevation and slope differences. Due to unavailability of streamflow gages at the sub-basin level, a drainage-area ratio method was used to estimate observed streamflow at the Phamong sub-basin outlet point by applying an area factor of 0.042 to streamflow data from the available stream gage. Inset B in Figure 2 shows the relative size of the study sub-basin (≈ 83 km2) relative to the area drained by the available gage (19,875 km2). The SWAT model was calibrated to the estimated flows at the Phamong sub-basin outlet using 2,000 runs on a monthly time step, and calibration results are presented in Figure 4 (see Supplementary material for calibration parameters). Although the study also addresses sedimentation, sediment data were unavailable for calibration, so sediment estimates from previous feasibility studies have been adopted. Proposed ICM plans were also implemented, and alternative climate scenarios were run through the model.
a. ICM Implementation: To assess the investment with adaptations, the ICM measures in Table 2 were implemented in SWAT (detailed information in Supplementary material). The targeted hydrologic impact of these measures was increased infiltration within, and reduced runoff from the sub-basin, which subsequently reduces the flow of sediments into the reservoir downstream. The 2-fold ICM benefits projected for the erosion-prone study area are reduced dam siltation leading to preserved live storage volume, and increased soil water retention which has the benefits of supplementing crop water demand within the cultivated areas and possible community water supply benefits from deep aquifer recharge.
b. Stochastic Weather Generator: For the climate stress test (Figure 1), 28 alternative scenarios were generated using a stochastic weather generator built in the R programming language following the approach outlined in Section 3.3. For our analysis, a range of −30 to +30% of the average annual value for precipitation and a temperature range of 0°C to +3°C of warming was sufficient, and increments of 10% of annual average precipitation and 1°C for temperature were adopted. These ranges ensure we explore an uncertainty space greater than that encapsulated in the GCM ensemble over the planning period. This was evaluated from the full ensemble of GCMs, plotted on the climate response surface as a “GCM cloud.” Daily time series from the two available precipitation and temperature stations were sampled (Supplementary material) to capture interannual variability, and monthly means were adjusted to ensure total monthly precipitation was maintained when the Markov Chain was adjusted. Ten trials of the weather generator were run, and an ensemble of 69 years of data was generated to match the length of available precipitation and temperature data records. Refer to Supplementary material for monthly statistical characteristics of the weather generator precipitation traces.
The reservoir model was built using the R programming language, beginning with modifying the res_supply function in the Reservoir package (Turner and Galelli, 2016) for flexibility of reservoir release rules optimization under the different climate scenarios, and to specify release targets based on the irrigation water demand. The reservoir optimization aimed to minimize the deviation between monthly reservoir allocations to crops, At, and monthly crop irrigation water demand, Dt. A specified weight, wt, was applied to penalize months in which reservoir allocation falls below the crop water demand. Equation 7 is the optimization function for the reservoir. Optimized reservoir allocations under each climate scenario were specified for the simulation of reservoir crop allocation following the workflow in Figure 3. In simulating the reservoir releases, environmental flow requirements were calculated as 13.4% of historical mean monthly runoff based on hydrological similarities of the Phamong dam sub-basin to the Metolong dam location (ORASECOM, 2014; AECOM, 2022b).
where, t—current month. n—total number of months. wt—penalizing weight. At—reservoir allocation to crops. Dt—crop water demand.
The irrigation water demand assessment conducted during the project’s feasibility studies (AECOM, 2022c) made use of the SAPWAT model (Crosby and Crosby, 1999) and followed guidelines from FAO Paper No. 56 (Allen et al., 2000) with generic crop coefficients modified to suit local climate conditions (Van Heerden and Walker, 2016) as shown in Supplementary material. The agronomic model for the MDIH scheme is a simple mathematical model, looped with the reservoir simulation model in R programming language, which estimates the number of monthly hectares that can be sustainably irrigated under each climate scenario using the optimized reservoir release rules. The gross irrigation requirement (GIR) was combined with the proposed cropping patterns (Supplementary material) according to Equation 8 to calculate the target number of hectares to be irrigated each month. From a total scheme area of 70 hectares marked out for irrigation by the dam, the number of hectares to be irrigated monthly, and their corresponding irrigation water requirement is presented in Supplementary material (AECOM, 2022c). In each climate scenario, the actual number of hectares irrigated monthly is calculated using Equation 9.
where, i—specific crop in the cropping pattern. n—total number of crops in the cropping patterns. GIRi—gross irrigation requirement (crop water requirement x efficiency of irrigation system) for crop i. mi—number of total hectares allocated to crop i. mon—month of the year. Htarget,mon—hectares of crops irrigated in a given month. Htotal—total hectares of the MDIH scheme to be irrigated by the dam (70 ha).
where, A—amount of water allocated to crops in a given month. Hactual,mon—actual hectares of crops irrigated in a given month.
The economic model is a CBA model looped into the model workflow (Figure 3) with the reservoir and crop models in the R programming language. A discount rate of 7% was adopted in the case study. The key benefit stream for the project is revenue from the hectares cultivated each month, and more hectares cultivated based on sufficient water availability lead to higher benefits. Stakeholders desire to have a measure of confidence in their investments under climate change and typically have varying levels of risk tolerance (Kunreuther et al., 2013; Aengenheyster et al., 2018; Ainia and Lutfi, 2019). In the economic model, we illustrate stakeholders’ risk tolerance by calculating benefits from the 80th percentile of monthly hectares cultivated during the study time horizon, where the area cultivated accounts for variability in water flows within and across climate scenarios. Two sources facilitate irrigation of the cultivated hectares in the study.
a. In the baseline (business as usual) scenario, the Phamong dam is relied on to meet all the irrigation demand and
b. In the ICM (adaptations) scenario, the irrigation demand is supported by both the dam and additional moisture retained within the irrigated perimeters and available to the crops.
Additionally, small quantities of deep aquifer recharge were observed in the ICM scenario, which suggests that community water supply benefits could be an added benefit stream with adaptations. Recognizing the importance of this observation in the holistic consideration of adaptation benefits, an attempt has been made to quantify and include this benefit stream in the CBA evaluation, using ranges of groundwater values estimated in literature (Bann and Wood, 2012; Bierkens et al., 2019; Charalambous, 2020). From post-2000 studies examined, the value of groundwater (adjusted to 2021 prices) ranges from $0.27/m3 to $0.67/m3 for agriculture and associated rural domestic supply. A summary of the literature review on groundwater valuation conducted for the study can be accessed in Supplementary material.
Table 3 summarizes the CBA metrics calculated for the baseline scenario, and from the highest NPV, we observe that approximately $0.82 million of investment costs (PV) could be lost over the design life of the project in the best-case climate simulated by the stress test (30% more precipitation and no change to historical average temperature). This means that even the maximum amount of water available under the baseline scenario, when managed according to assumed reservoir operations, does not irrigate sufficient hectares to cover the assumed investment costs. The maximum ERR calculated across the 28 climate scenarios was 2.08%, which is much lower than the 7% analysis discount rate. It is, however, important to note that while the modeling is rigorous in many respects as described in Section 4 (Case Study) and informed by available local data, certain cost allocation and operational assumptions were made for illustrative purposes, and therefore the absolute value of these findings should not be interpreted as definitive assessments of the performance of actual MCC project. However, taking these results at face value, the baseline investment would be judged vulnerable to changing climate with an RI (and CRI) of 0 as all ERRs calculated across the stress test scenarios fall below the discount rate and the NPV is entirely negative. Projects with zero RI cannot be expected to perform acceptably and require adaptation measures. ICM has been recommended as an adaptation option in our case study. The following section illustrates the potential of adaptations in preserving the project benefit streams.
The Phamong irrigation dam is susceptible to high sedimentation due to land degradation and high erosion rates in the sub-basin. These high sedimentation rates threaten projected benefit streams as they could reduce dam storage capacity and limit the number of hectares that can be sustainably irrigated over time. From previous feasibility studies (AECOM, 2022b), a reservoir storage loss of 0.11 MCM is projected to occur over 50 years of operating the dam. This means that the Phamong irrigation dam could lose 24% of its original storage capacity in approximately 40 years, which translates to a potential $0.28 million investment benefit loss. Figure 5 illustrates the potential of ICM measures in preserving dam storage capacity as modeled in our study. Over a period of 44 years, the projected loss of benefits due to sedimentation is only $0.09 million when ICM measures are implemented. We estimate that ICM implementation could potentially preserve $0.19 million of crop revenue benefits in the Phamong scheme. If we extended this analysis to the entire MDIH scheme, the investment benefits preserved would be much more significant. Furthermore, adaptation measures like ICM often provide co-benefits such as carbon sequestration (Smith, 2004; Lal, 2008), which if valued could augment the value of the total benefits. Some studies have highlighted the climate change adaptation and mitigation benefits associated with carbon sequestration (Lal, 2004; Ussiri and Lal, 2017; Villa and Bernal, 2018). The valuation of these co-benefit streams is beyond the scope of the study but has been highlighted for future study.
Figure 5. Potential benefit of ICM in preserving dam storage volume over a period of 44 years (2025–2068).
Although check dams were designated as the priority ICM for the Phamong area in the feasibility studies, implementation of all recommended ICM measures (check dams, contours and waterways, and fencing) in combination was found to be the most effective in preventing excessive dam siltation. Our analysis results showed that contours and grassed waterways are the most effective individual ICM measures for preservation of dam storage, then checking dams, and then fencing off degraded areas. This is consistent with results from Shrestha et al. (2021) who found terracing to be the best catchment-level management option which reduced both magnitude and loss of reservoir capacity and cost of sediment management in the Mekong River Basin in Southern Laos, China. Vogl et al. (2017) also found improved soil retention, reduced in-stream sediment, and lowered erosion rates, while exploring the economic benefits of investments in sustainable land use practices, including terracing, on ecosystem services in the Upper Tana basin, Kenya. Despite the poor performance of ERR and NPV metrics in our case study, the PV of benefits is still beneficial in illustrating stakeholders’ WTP for ICM adaptations that preserve project benefits. The discussion from this point forward focuses on the PV of benefits to compare the ICM and baseline scenarios and illustrate the recommended approach to investment decision-making using the CRI, and estimate WTP.
Likelihood information from GCMs can assist in identifying which of the stress test-generated climate scenarios are more likely to occur, leading to a more robust consideration of investment vulnerability. Average monthly precipitation and temperature from SSP2-4.5 and SSP5-8.5 scenarios of CMIP6 projections (historical baseline period: 2000–2020 and future analysis period: 2040–2060; resolution 0.25 × 0.25) were obtained from NASA Earth Exchange Global Daily Downscaled Projections and used to calculate the CRI. The SSP2-4.5 projection represents a low to medium greenhouse gas (GHG) emission scenario with medium challenges to mitigation and adaptation, while the SSP5-8.5 projection represents a high GHG emissions scenario with low challenges to adaptation but high challenges to mitigation (Tebaldi et al., 2006; O'Neill et al., 2016).
To evaluate the fit of the GCMs, we compared seasonality (long-term average per month) and average annual depth of precipitation in the study area with historical GCM runs and observed data (from the Princeton dataset) between 1950 and 2011. After this preliminary skill assessment, some GCM models were selected for the analysis, and others excluded as shown in Table 4 (also see Supplementary material). While there is no strict threshold recommendation for evaluating GCM fits, our analysis uses a correlation threshold of 0.7 to select the final suite of GCMs to include in the analysis. In addition, for illustration purposes, an arbitrary analysis threshold of $1 million was adopted for the PV of benefits; that is, the study assumes that the Phamong irrigation scheme performs acceptably if the PV of benefits is at least $1 million. Table 5 summarizes the expected values and CRIs of PV under the baseline and ICM scenarios. From the expected values calculated, the ICM scenario performs slightly better than the baseline (by $0.03 million). However, this metric does not offer any information on the added robustness of the investment due to adaptations, and so it forms only part of the picture of project performance.
Additional information for robust decision-making was gained by calculating the uniform RI. Assuming equal likelihood for all climate scenarios, however, led to misleading results in the baseline scenario. Uniform RI was calculated as 42.4% in the baseline scenario, and the project may have been judged as moderately robust to climate uncertainties. More information on the robustness of investment was gained from the CRI by using the multivariate normal distribution (mean precipitation change = 0.89 mm, mean temperature change = 1.69°C, and covariance matrix, ) to assess the investment’s vulnerability to possible changes in historical average climate. With likelihood information, the results found that baseline investment robustness is much lower: approximately 16 percentage points less than the uniform RI indicated (CRI = 26.5%), suggesting a higher need for climate adaptation than is revealed by the RI alone. This adds a layer of confidence to the benefits of adaptations already hinted at by higher expected value in the ICM scenario. The investment was found to be more robust to climate change when adaptations are implemented, using both the uniform RI and CRI. With uniform RI, the added robustness from ICM appears negligible (an increase of 7.2%), but the CRI clearly shows that investment robustness is almost double in the ICM scenario compared to the baseline. The CRI confirms that the robustness of the irrigation investment is improved with ICM, and stakeholders should be willing to explore adaptation options. A recent study by François et al. (2024) illustrates practically this added benefit of the CRI in investment decision-making.
The CDFs in Figure 6 visualize the robustness information presented in Table 5. Without the consideration of likelihood information (Figure 6A), at the 50th percentile (dotted gray lines), the ICM scenario is closer to the PV threshold than the baseline scenario. This, like the expected value, identifies the better performance of the ICM scenario. With likelihood information (Figure 6B), the robust performance of the ICM scenario relative to the baseline is more appreciable. The ICM scenario is the clear winner in more than 90% of the climate scenarios considered as seen in Figure 6B where the red ICM line emerges above the blue baseline scenario line before the 10th percentile. The red ICM line emerges above the blue baseline scenario line only after the 30th percentile when information from GCMs regarding the likelihood of potential future climate states is ignored (Figure 6A), masking the benefits of ICM.
Figure 6. Cumulative density functions of PV of benefits without (A) and with (B) likelihood information (solid gray line is the PV analysis threshold of $1 million, and the circles mark the points at which the ICM scenario begins to outperform the baseline scenario).
Based on the vulnerability assessment results, the study proposes the adoption of the recommended ICM options to preserve the benefit streams of the MDIH investment under climate change. The WTP for such adaptations under climate change is often a concern in project development. For instance, Mandri-Perrott and Bisbey (2016) highlight financial risk, that is, the likelihood that investors will not (continue) to provide sufficient funding for project development (including adaptation planning) as a key risk to irrigation schemes. Stakeholders and decision makers require justification for additional investment and need to understand whether adaptations contribute to overall investment goals, or lead to more loss of investment benefits due to uncertainty in future climate. Figure 7 presents the difference in the PV of investment benefits in the ICM and baseline scenarios under the 28 climate storylines generated from the stress test and Table 6 presents the summary statistics of the PV benefits. Higher PV values are observed in the ICM scenario, especially in high precipitation scenarios (blue portion of Figure 7), and this shows the effectiveness of check dams, contours, and waterways, in upstream water retention and reduction of sediment flow into the dam.
Figure 7. Difference in PV of investment benefits (estimated WTP) (The solid GCM cloud shows the 95th percentile of GCMs with correlation >0.7 after the preliminary skill assessment while the dotted GCM cloud presents fifth percentile of GCMs with correlation >0.7).
The red portion of Figure 7 suggests that adaptation measures are not always beneficial to investments. In low-precipitation scenarios, the results show that investing in ICM measures could lead to more losses. In the worst-case scenario (20% less precipitation and ≥ +2°C temperature), approximately $0.18 million could be lost compared to proceeding with the baseline investment without adaptations. For our case study, such losses are traceable to the counter-effect of the ICM measures when there is low-precipitation and/or high temperature. For example, check dams function by reducing the flow rate of runoff, thereby intercepting sediments that would have flowed directly into the reservoir downstream. When natural water budgets are low and temperatures are high, the flow held upstream is mostly evaporated before it either infiltrates and travels downstream through sub-surface channels, is taken up by growing crops, or percolates to recharge deep aquifers. The irrigation infrastructure could end up redundant and non-functional in drought periods since water is held upstream and evaporated at accelerated rates. The same mechanism is observed in the case of contours, grassed waterways, and fencing to revegetate degraded areas with increased rates of water loss through evapotranspiration. Martin et al. (2021) similarly found high evapotranspiration associated with extreme climate scenarios and noted that nature-based solutions (NbS) may not be sufficient adaptation to intense climate change. Rather than adaptations, if such low-precipitation scenarios are more likely to occur, a redesign of the project in terms of scope, for example, reducing the number of hectares planned for irrigation, can be explored.
Considering the position of the GCM cloud in Figure 7, the negative returns associated with investing in ICM measures in drier climate scenarios appear to be low probability. This has been confirmed by the vulnerability assessment results presented using the CRI in the previous section. In other individual climate futures, the incremental benefit of ICM implementation could potentially reach up to $0.13 million on adaptations (Table 6). The PV of benefits of adaptations under climate change is illustrated in Figure 7. This is the maximum amount investors should spend on implementing adaptations now to secure acceptable future performance of the investment, and preservation of projected benefits under climate change. This estimate finds application in the drafting of contingency fund budgets for climate-sensitive projects and guards against uninformed investment in adaptation options under climate change. From our case study results, investing in ICM adaptation would lead to expected incremental benefits of $0.03 million, though they could range from negative $0.18 million to $0.13 million depending on which climate future is realized.
Estimating what stakeholders should be willing to pay for adaptations using the presented methodology contributes further to existing guidance for the planning and management of water resources projects which make up a large portion of climate-sensitive investments. This is at the forefront of climate change adaptation planning globally (Derkzen et al., 2017; O'Garra and Mourato, 2016; Badura et al., 2021; Kruse and Atkinson, 2022). For instance, Tang et al. (2022) examined factors influencing the WTP for agricultural irrigation water in Sichuan, China, using an empirical double-hurdle model based on survey data from farming households. Our study presents a climate-informed and economically defensible basis for estimating stakeholders’ WTP for adaptations using the PV of adaptation benefits. While other factors such as affordability, political acceptability, and market forces will eventually contribute to the choice of adaptations (if any) and estimating the actual WTP for them, the methodology presented still finds useful application in water projects planning, budgeting, design, and implementation under climate change.
The study has presented a step-by-step approach to the assessment of investment vulnerability and estimation of the stakeholders’ WTP for adaptations under climate change. The strengths, applications, and limitations of conventional methods of uncertainty characterization like sensitivity analysis, scenario analysis, and Monte Carlo analysis have been examined. The proposed methodology carefully integrates all these methods in vulnerability assessment using the CRI. By introducing bottom-up decision scaling principles (Brown et al., 2012), such as decision framing, the climate stress test, and estimation of climate-informed risks into standard economic evaluation process using CBA, we present a robust approach to economic evaluation and decision-making in climate-sensitive projects such as water resources projects (Figure 1).
The methodology was demonstrated using a case study of an irrigation scheme in the Phamong area of Lesotho (Figure 2). Results of the study show the value of the CRI in investment vulnerability assessment and decision-making under climate change. In addition, we use our results to estimate the investors’ WTP for adaptations that secure projected benefits under a changing climate. The selected case study was one of four demarcated irrigation schemes in a sub-activity of the planned Lesotho 2 compact (see Case Study), and results did not yield sufficient economic benefits to be evaluated using standard ERR hurdle rates and the NPV. Initial assessment of the baseline (business as usual) scenario using a discount rate of 7% showed that the investment is vulnerable to climate change with NPV lying entirely below 0 in all the 28 climate scenarios analyzed.
However, the PV of the investment benefits was a sufficient basis for discussing investors’ WTP for adaptations and comparing the performance of baseline and ICM scenarios. With PV benefits threshold of $1 million, and benchmark RI = 50%, the ICM scenario performed better in terms of both expected value and RI. With adaptations, $0.03million of expected incremental benefits was calculated, and the uniform RI improved by 7.2% relative to the baseline design. Including likelihood information from CMIP6 projections for the Lesotho area provided more insight into the robustness of investment to climate change. The CRI under the ICM scenario was 50.6%, almost double the CRI of 26.5% under the baseline scenario. While noting the limitation of the PV in standard economic decision-making, the difference in PV of benefits between the ICM and baseline scenarios puts in current value perspective the maximum amount investors should be willing to spend on adaptation measures that preserve investment robustness.
Our results showed that in very low-precipitation scenarios, investing in ICM measures could lead to up to $0.18 million in incremental losses. Such losses are traceable to the counter-effect of extreme evaporation associated with ICM measures when there is low precipitation and/or high temperature. However, from the vulnerability assessment, such extreme low-precipitation scenarios are unlikely to occur. More benefits can be gained from ICM implementation if investors are willing to spend up to $0.13 million (PV) on adaptations, depending on which climate future is realized. This WTP estimate is useful for contingency budget preparation for the planned project, with sufficient justification from the CRI results, which equips project planners with a demonstrated approach for contingency budget defense. We also provide direction for improving climate adaptation policies by illustrating the value-add of climate vulnerability assessment and WTP estimates in climate-sensitive investment decision-making. For the successful implementation of our methodology in policymaking, we recommend the policy integration (Biesbroek, 2021) and policy diffusion (Schoenefeld et al., 2022) approaches. The former enables continuous improvement to climate adaptation policies as more knowledge is gained and novel analytical methods are developed, while the latter facilitates interdependent policymaking among several stakeholders.
In our study, there are limitations associated with analyzing part of a sub-activity in a development compact as a standalone investment, especially with respect to estimated costs and choice of discount rate. Assumptions associated with modeling the ICM measures and their sediment capture efficiency (Table 2), as well as inadequate data for hydrologic model calibration, are additional sources of limitation to the study. Therefore, our analysis is not definitive in its conclusions about the proposed Lesotho MDIH scheme. The MDIH scheme is also centralized and benefits from the scheme and implementation of adaptations are unlikely to be equitably distributed within the Phamong community. According to Fletcher et al. (2022), to achieve equitable outcomes in water resources management, it is critical that equity be included in the decision-making process. While several studies have investigated matters of distributional equity in water resources, there remains a disconnect in translating theoretical recommendations into practice (Jafino et al., 2021; Seigerman et al., 2023; Odunola et al., 2023a,b).
This study has contributed on one hand to the practical demonstration of robust investment decision-making using results of climate vulnerability assessment and by estimating stakeholders’ WTP for adaptations under climate change. In future study, we will contribute further by integrating the assessment of spatial and distributional equity into the standard economic evaluation and decision-making processes under climate change. By carefully illustrating the place of recognitional (involving diverse stakeholder input), procedural (improved modeling frameworks and assumptions), and distributional equity (allocation of resources, costs, and benefits) in the various phases of water project planning (Seigerman et al., 2023), including modeling and financial assessment, we aim to propose a reproducible approach to robust and equitable economic evaluation under changing climate.
The original contributions presented in the study are included in the article/Supplementary material, further inquiries can be directed to the corresponding author.
TO: Data curation, Formal analysis, Investigation, Methodology, Software, Visualization, Writing – original draft, Writing – review & editing. BeB: Conceptualization, Methodology, Project administration, Supervision, Writing – review & editing, Writing – original draft, Validation. PeR: Formal analysis, Writing – review & editing, Data curation. SZ: Data curation, Formal analysis, Visualization, Writing – original draft. JR: Methodology, Writing – original draft. BrB: Conceptualization, Methodology, Writing – original draft. DC: Methodology, Writing – original draft. KS: Methodology, Writing – original draft. EM: Data curation, Writing – review & editing. CB: Conceptualization, Funding acquisition, Investigation, Methodology, Supervision, Validation, Writing – original draft, Project administration. PaR: Conceptualization, Funding acquisition, Investigation, Methodology, Resources, Supervision, Validation, Writing – original draft, Writing – review & editing.
The author(s) declare that financial support was received for the research, authorship, and/or publication of this article. This study was funded by the Millennium Challenge Corporation (MCC) as part of a partnership agreement focused on improving methods for considering climate change in the development and evaluation of its international projects (Federal Award: 9332420T0002). See also Brown et al. (2024).
In addition to the funding source, the authors would like to thank members of the Water Systems Analysis Research Group at the University of Cincinnati who assisted with the hydrologic analysis. We also appreciate Benjamin Campbell, Stefan Osborne, Sarah Martiny, and other Lesotho II team members and economists at the Millennium Challenge Contribution for providing insight on compact project development and economic evaluation at MCC.
BeB and EM were employed by Millennium Challenge Corporation. BrB, DC, and KS were employed by Industrial Economics, Incorporated (IEc).
The remaining authors declare that the research was conducted in the absence of any commercial or financial relationships that could be construed as a potential conflict of interest.
All claims expressed in this article are solely those of the authors and do not necessarily represent those of their affiliated organizations, or those of the publisher, the editors and the reviewers. Any product that may be evaluated in this article, or claim that may be made by its manufacturer, is not guaranteed or endorsed by the publisher.
The Supplementary material for this article can be found online at: https://www.frontiersin.org/articles/10.3389/frwa.2024.1442023/full#supplementary-material
ADB, Asian Development Bank; AECOM, Architecture, Engineering, Construction, Operations, and Management (a global infrastructure firm); CBA, Cost Benefit Analysis; CMIP, Coupled Model Intercomparison Project; CRI, Climate-informed Robustness Index; CRIDA, Climate Risk-Informed Decision Analysis; CRiSTAL, Community-based Risk Screening Tool – Adaptation and Livelihoods; ERR, Economic Rate of Return; FAO, Food and Agriculture Organization of the United Nations; GCMs, Global Circulation Models; GHG, Greenhouse Gas; ICM, Integrated Catchment Management; MCC, Millennium Challenge Corporation; MCM, Million Cubic Meters; MDIH, Market Driven Irrigation Horticulture; NbS, Nature-based Solutions; NPV, Net Present Value; PV, Present Value; RI, Robustness Index; SAPWAT, South African Procedure for estimating irrigation WATer requirements; SSP, Shared Socio-economic Pathways; SWAT, Soil and Water Assessment Tool; USAID, United States Agency for International Development; WTP, Willingness-to-pay.
1. ^Also known as the Kaldor-Hicks compensation test (Brown, 2022).
2. ^The ERR is simply the Internal Rate of Return formula applied to costs and benefits that have been calculated in economic (social) terms, rather than with respect to the finance of an investor or private entity. It is sometimes also referred to as the Economic Internal Rate of Return (EIRR).
Adger, W. N., Arnell, N. W., and Tompkins, E. L. (2005). Successful adaptation to climate change across scales. Glob. Environ. Chang. 15, 77–86. doi: 10.1016/j.gloenvcha.2004.12.005
AECOM. (2022a). Lesotho: Feasibility study on potential Investments in Irrigated Agriculture and Associated Agronomic, engineering, economic, environmental, and social assessments: Provisional environmental and social management framework.
AECOM. (2022b). Lesotho: Feasibility study on potential Investments in Irrigated Agriculture and Associated Agronomic, engineering, economic, environmental, and social assessments: Water resources assessment report.
AECOM. (2022c). Lesotho: Feasibility study on potential Investments in Irrigated Agriculture and Associated Agronomic, engineering, economic, environmental, and social Assessments: Irrigation Water Demand Assessment Report.
Aengenheyster, M., Feng, Q. Y., Van Der Ploeg, F., and Dijkstra, H. A. (2018). The point of no return for climate action: effects of climate uncertainty and risk tolerance. Earth Syst. Dynam. 9, 1085–1095. doi: 10.5194/esd-9-1085-2018
Ainia, N. S. N., and Lutfi, L. (2019). The influence of risk perception, risk tolerance, overconfidence, and loss aversion towards investment decision making. J. Econ. Bus. Account. Ventura 21, 401–413. doi: 10.14414/jebav.v21i3.1663
Allen, R. G., Smith, M., Pereira, L. S., Raes, D., and Wright, J. L. (2000). Revised FAO procedures for calculating evapotranspiration: irrigation and drainage paper no. 56 with testing in Idaho. Watershed Management and Operations Management (pp. 1–10)
Arndt, C., Strzepeck, K., Tarp, F., Thurlow, J., Fant, C., and Wright, L. (2011). Adapting to climate change: an integrated biophysical and economic assessment for Mozambique. Sustain. Sci. 6, 7–20. doi: 10.1007/s11625-010-0118-9
Asplund, D., and Eliasson, J. (2016). Does uncertainty make cost-benefit analyses pointless? Transp. Res. A Policy Pract. 92, 195–205. doi: 10.1016/j.tra.2016.08.002
Badura, T., Lorencová, E. K., Ferrini, S., and Vačkářová, D. (2021). Public support for urban climate adaptation policy through nature-based solutions in Prague. Landsc. Urban Plan. 215:104215. doi: 10.1016/j.landurbplan.2021.104215
Bakkoury, Z., Ouazar, D., and Walters, G. (2004). EVAPRO: economic and financial evaluation of water supply projects. J. Hydroinf. 6, 109–121. doi: 10.2166/hydro.2004.0010
Bann, C., and Wood, S. C. (2012). Valuing groundwater: a practical approach for integrating groundwater economic values into decision making–a case study in Namibia, southern Africa. Water SA 38, 461–466. doi: 10.4314/wsa.v38i3.12
Batchelor, C. (1999). Improving water use efficiency as part of integrated catchment management. Agric. Water Manag. 40, 249–263. doi: 10.1016/S0378-3774(98)00125-5
Belle, J. A., and Hlalele, M. B. (2015). Vulnerability assessment of agricultural drought Hazard: a case of KotiSe-Phola community council, Thabana Morena, Mafeteng District in Lesotho. J. Geogr. Nat. Disast. 5:143. doi: 10.4172/2167-0587.1000143
Berrang-Ford, L., Ford, J. D., and Paterson, J. (2011). Are we adapting to climate change? Glob. Environ. Chang. 21, 25–33. doi: 10.1016/j.gloenvcha.2010.09.012
Bierkens, M. F., Reinhard, S., de Bruijn, J. A., Veninga, W., and Wada, Y. (2019). The shadow price of irrigation water in major groundwater-depleting countries. Water Resour. Res. 55, 4266–4287. doi: 10.1029/2018WR023086
Biesbroek, R. (2021). Policy integration and climate change adaptation. Curr. Opin. Environ. Sustain. 52, 75–81. doi: 10.1016/j.cosust.2021.07.003
Borgomeo, E., Mortazavi-Naeini, M., Hall, J. W., and Guillod, B. P. (2018). Risk, robustness and water resources planning under uncertainty. Earth's Future 6, 468–487. doi: 10.1002/2017EF000730
Brigham, E. F. (1975). Hurdle rates for screening capital expenditure proposals. Financ. Manag. 4, 17–26. doi: 10.2307/3665186
Brown, Z. S. (2022). Distributional policy impacts, WTP-WTA disparities, and the Kaldor-Hicks tests in benefit-cost analysis. J. Environ. Econ. Manag. 113:102654. doi: 10.1016/j.jeem.2022.102654
Brown, C., Ghile, Y., Laverty, M., and Li, K. (2012). Decision scaling: linking bottom-up vulnerability analysis with climate projections in the water sector. Water Resour. Res. 48, W09537. doi: 10.1029/2011WR011212
Brown, C., Ray, P., Bernstein, A., Castillo, D., Boehlert, B., Strzepek, K., et al. (2024). Achieving robust project design through iterative climate-informed performance assessment. Millennium Challenge Corporation Cooperative Agreement Product. Available at: https://www.mcc.gov/resources/doc/guidance-achieving-robust-project-design-through-climate-assessment/
Buurman, J., and Babovic, V. (2016). Adaptation pathways and real options analysis: an approach to deep uncertainty in climate change adaptation policies. Polic. Soc. 35, 137–150. doi: 10.1016/j.polsoc.2016.05.002
Chambwera, M., and Stage, J. (2010). Climate change adaptation in developing countries: Issues and perspectives for economic analysis : International Institute for Environment and Development. London, United Kingdom.
Charalambous, A. N. (2020). Groundwater and its economic nature. Q. J. Eng. Geol. Hydrogeol. 53, 176–188. doi: 10.1144/qjegh2019-068
Crosby, C. T., and Crosby, C. P. (1999). A computer program for establishing irrigation requirements and scheduling strategies in South Africa. WRC Report.
Dangendorf, S., Hay, C., Calafat, F. M., Marcos, M., Piecuch, C. G., Berk, K., et al. (2019). Persistent acceleration in global sea-level rise since the 1960s. Nat. Clim. Chang. 9, 705–710. doi: 10.1038/s41558-019-0531-8
De Boer, J., Wardekker, J. A., and Van der Sluijs, J. P. (2010). Frame-based guide to situated decision-making on climate change. Glob. Environ. Chang. 20, 502–510. doi: 10.1016/j.gloenvcha.2010.03.003
Derkzen, M. L., Van Teeffelen, A. J., and Verburg, P. H. (2017). Green infrastructure for urban climate adaptation: how do residents’ views on climate impacts and green infrastructure shape adaptation preferences? Landsc. Urban Plan. 157, 106–130. doi: 10.1016/j.landurbplan.2016.05.027
Estrada, F., Botzen, W. J. W., and Tol, R. S. J. (2017). A global economic assessment of city policies to reduce climate change impacts. Nat. Clim. Chang. 7, 403–406. doi: 10.1038/nclimate3301
FAO (2018). Cost-benefit analysis for climate change adaptation policies and investments in the agriculture sectors. A Briefing Note.
Fletcher, S. M., Hadjimichael, A., Quinn, J., Osman, K., Giuliani, M., Gold, D., et al. (2022). Equity in Water resources planning: A path forward for decision support modelers. J. Water Resour. Plan. Manag. 148:02522005. doi: 10.1061/(ASCE)WR.1943-5452.0001573
François, B., Dufour, A., Nguyen, T. N. K., Bruce, A., Park, D. K., and Brown, C. (2024). From many futures to one: climate-informed planning scenario analysis for resource-efficient deep climate uncertainty analysis. Clim. Chang. 177, 1–23. doi: 10.1007/s10584-024-03772-9
Freeman, S. S. G., Brown, C., Cañada, H., Martínez, V., Nava, A. P., Ray, P., et al. (2020). Resilience by design in Mexico City: a participatory human-hydrologic systems approach. Water Security 9:100053. doi: 10.1016/j.wasec.2019.100053
Gall-Ely, M. L. (2009). Definition, measurement and determinants of the consumer's willingness to pay: a critical synthesis and avenues for further research. Rech. Appl. Mark. 24, 91–113. doi: 10.1177/076737010902400205
Gassman, P. W., Sadeghi, A. M., and Srinivasan, R. (2014). Applications of the SWAT model special section: overview and insights. J. Environ. Qual. 43, 1–8. doi: 10.2134/jeq2013.11.0466
Gilbert, S. W., Butry, D. T., Helgeson, J. F., and Chapman, R. E. (2015). Community resilience economic decision guide for buildings and infrastructure systems. NIST Special Publication, 1197, 1–69.
Hallegatte, S. (2006). A cost-benefit analysis of the New Orleans flood protection system. AEI-Brookings joint center. Regulatory Analysis, 06-02.
Hallegatte, S., Anjum, R., Avner, P., Shariq, A., Winglee, M., and Knudsen, C. (2021). Integrating climate change and natural disasters in the economic analysis of projects a disaster and climate risk stress test methodology. (No. 35751). The World Bank Group, doi: 10.1596/35751
Hallegatte, S., Shah, A., Brown, C., Lempert, R., and Gill, S. (2012). Investment decision making under deep uncertainty--application to climate change. World Bank Policy Research Working Paper (6193), doi: 10.1596/1813-9450-6193
Hammill, A., and Tanner, T. (2011). Harmonising climate risk management: adaptation screening and assessment tools for development co-operation. OECD Environment Working Papers, (36), 0_1.
International Hydropower Association (2019). Hydropower sector climate resilience guide. International Hydropower Association: London, UK.
Jafino, B. A., Kwakkel, J. H., and Taebi, B. (2021). Enabling assessment of distributive justice through models for climate change planning: a review of recent advances and a research agenda. Wiley Interdiscip. Rev. Clim. Change 12:e721–n/a. doi: 10.1002/wcc.721
Jeuken, A., Mendoza, G., Matthews, J., Ray, P., Haasnoot, M., Gilroy, K., et al. (2016). Climate risk informed decision analysis (CRIDA): a novel practical guidance for climate resilient investments and planning. Paper presented at the EPSC2016–8797. Available at: https://ui.adsabs.harvard.edu/abs/2016EGUGA..18.8797J
Jeuken, A., Ray, P., Penning, E., Bouaziz, L., Tracy, J., Wi, S., et al. (2023). Challenges for upscaling hydrological effectiveness of nature-based solution for adaptation to climate change in watersheds. Aquat. Ecosyst. Health Manage. 26, 19–32. doi: 10.14321/aehm.026.02.019
Kamara, J. K., Agho, K., and Renzaho, A. M. N. (2019). Understanding disaster resilience in communities affected by recurrent drought in Lesotho and Swaziland—a qualitative study. PLoS 14:e0212994. doi: 10.1371/journal.pone.0212994
Kazemi Garajeh, M., Akbari, R., Aghaei Chaleshtori, S., Shenavaei Abbasi, M., Tramutoli, V., Lim, S., et al. (2024b). A comprehensive assessment of climate change and anthropogenic effects on surface Water resources in the Lake Urmia Basin, Iran. Remote Sens. 16:1960. doi: 10.3390/rs16111960
Kazemi Garajeh, M., Haji, F., Tohidfar, M., Sadeqi, A., Ahmadi, R., and Kariminejad, N. (2024a). Spatiotemporal monitoring of climate change impacts on water resources using an integrated approach of remote sensing and Google earth engine. Sci. Rep. 14:5469. doi: 10.1038/s41598-024-56160-9
Keller, M., Swanson, D., Bizikova, L., Hammill, A., Zamudio, A., Guevara, R., et al. (2013). Climate resilience and food security. A framework for planning and monitoring. Winnipeg: International Institute for Sustainable Development (IISD).
Kruse, T., and Atkinson, G. (2022). Understanding public support for international climate adaptation payments: evidence from a choice experiment. Ecol. Econ. 194:107321. doi: 10.1016/j.ecolecon.2021.107321
Kull, D., Gitay, H., Bettencourt, S., Reid, R., Simpson, A., and McCall, K. (2016). “Building resilience: World Bank Group experience in climate and disaster resilient development” in Climate Change Adaptation Strategies–An Upstream-Downstream Perspective. eds. N. Salzmann, C. Huggel, S. Nussbaumer, and G. Ziervogel (Cham: Springer), 255–270.
Kundzewicz, Z. W., Krysanova, V., Benestad, R. E., Hov, Ø., Piniewski, M., and Otto, I. M. (2018). Uncertainty in climate change impacts on water resources. Environ. Sci. Pol. 79, 1–8. doi: 10.1016/j.envsci.2017.10.008
Kunreuther, H., Heal, G., Allen, M., Edenhofer, O., Field, C. B., and Yohe, G. (2013). Risk management and climate change. Nat. Clim. Chang. 3, 447–450. doi: 10.1038/nclimate1740
Lal, R. (2004). Soil carbon sequestration to mitigate climate change. Geoderma 123, 1–22. doi: 10.1016/j.geoderma.2004.01.032
Lal, R. (2008). Carbon sequestration. Philos. Trans. R. Soc. B Biol. Sci. 363, 815–830. doi: 10.1098/rstb.2007.2185
Lempert, R. J. (2019). “Robust decision making (RDM)” in Decision making under deep uncertainty: From theory to practice. eds. V. A. W. J. Marchau, W. E. Walker, P. J. T. M. Bloemen, and S. W. Popper (Switzerland: Springer International Publishing), 23–51.
Lempert, R., Nakicenovic, N., Sarewitz, D., and Schlesinger, M. (2004). Characterizing climate-change uncertainties for decision-makers. Clim. Chang. 65, 1–9. doi: 10.1023/B:CLIM.0000037561.75281.b3
Lempert, R. J., Popper, S. W., and Bankes, S. C. (2010). Robust decision making: coping with uncertainty. The Futurist 44:47.
Maass, A., Hufschmidt, M. M., Dorfman, R., Thomas, J., Harold, A., Marglin, S. A., et al. (1962). Design of water-resource systems: New techniques for relating economic objectives, engineering analysis, and governmental planning. Cambridge, MA: Harvard University Press.
Mandri-Perrott, C., and Bisbey, J. (2016). How to develop sustainable irrigation projects with private sector participation. Washington, DC: World Bank.
Martin, E. G., Costa, M. M., Egerer, S., and Schneider, U. A. (2021). Assessing the long-term effectiveness of nature-based solutions under different climate change scenarios. Sci. Total Environ. 794:148515. doi: 10.1016/j.scitotenv.2021.148515
Meier, I., and Tarhan, V. (2007). Corporate investment decision practices and the hurdle rate premium puzzle. Available at SSRN 960161, doi: 10.2139/ssrn.960161
Mendoza, G., Jeuken, A., Matthews, J. H., Stakhiv, E., Kucharski, J., and Gilroy, K. (2018). Climate risk informed decision analysis (CRIDA): Collaborative water resources planning for an uncertain future. Paris: UNESCO Publishing.
Moody, P., and Brown, C. (2013). Robustness indicators for evaluation under climate change: application to the upper Great Lakes. Water Resour. Res. 49, 3576–3588. doi: 10.1002/wrcr.20228
Nesshöver, C., Assmuth, T., Irvine, K. N., Rusch, G. M., Waylen, K. A., Delbaere, B., et al. (2017). The science, policy and practice of nature-based solutions: an interdisciplinary perspective. Sci. Total Environ. 579, 1215–1227. doi: 10.1016/j.scitotenv.2016.11.106
Odunola, T. O., Ray, P., Brown, C., Boehlert, B., Strzepek, K., Richardson, J., et al. (2023a). Practical steps for achieving equity in Water resources system planning: Lesotho irrigation investment under climate change. Authorea Preprints.
Odunola, T., Ray, P., Brown, C., Boehlert, B., Strzepek, K., Richardson, J., et al. (2023b). Economic evaluation of Lesotho investment under climate change. Paper presented at the World Environmental and Water Resources Congress 2023, 534–552.
O'Garra, T., and Mourato, S. (2016). Are we willing to give what it takes? Willingness to pay for climate change adaptation in developing countries. J. Environ. Econ. Policy 5, 249–264. doi: 10.1080/21606544.2015.1100560
O'Neill, B. C., Tebaldi, C., Van Vuuren, D. P., Eyring, V., Friedlingstein, P., Hurtt, G., et al. (2016). The scenario model intercomparison project (ScenarioMIP) for CMIP6. Geosci. Model Dev. 9, 3461–3482. doi: 10.5194/gmd-9-3461-2016
ORASECOM. (2014). Water demand projections and synthesis of planned infrastructure investments: Integrated Water resources management plan for the Orange-Senqu River Basin.
Ospina, A. V., and Rigaud, K. K. (2021). Integrating resilience attributes into operations: A note for practitioners. Washington, DC: World Bank.
Phan, T. D., Smart, J. C., Sahin, O., Capon, S. J., and Hadwen, W. L. (2018). Assessment of the vulnerability of a coastal freshwater system to climatic and non-climatic changes: a system dynamics approach. J. Clean. Prod. 183, 940–955. doi: 10.1016/j.jclepro.2018.02.169
Poterba, J. M., and Summers, L. H. (1995). A CEO survey of US companies' time horizons and hurdle rates. MIT Sloan Manag. Rev. 37:43.
Ray, P. A., Bonzanigo, L., Wi, S., Yang, Y. E., Karki, P., Garcia, L. E., et al. (2018). Multidimensional stress test for hydropower investments facing climate, geophysical and financial uncertainty. Glob. Environ. Chang. 48, 168–181. doi: 10.1016/j.gloenvcha.2017.11.013
Ray, P. A., and Brown, C. M. (2015). Confronting climate uncertainty in water resources planning and project design: The decision tree framework. Washington, DC: World Bank Publications.
Ray, P. A., Taner, M. Ü., Schlef, K. E., Wi, S., Khan, H. F., Freeman, S. S. G., et al. (2019). Growth of the decision tree: advances in bottom-up climate change risk management. J. Am. Water Resour. Assoc. 55, 920–937. doi: 10.1111/1752-1688.12701
Ray, P., Wi, S., Schwarz, A., Correa, M., He, M., and Brown, C. (2020). Vulnerability and risk: climate change and water supply from California’s Central Valley water system. Clim. Chang. 161, 177–199. doi: 10.1007/s10584-020-02655-z
Refsgaard, J. C., van der Sluijs, J. P., Højberg, A. L., and Vanrolleghem, P. A. (2007). Uncertainty in the environmental modelling process–a framework and guidance. Environ. Model Softw. 22, 1543–1556. doi: 10.1016/j.envsoft.2007.02.004
Rodríguez, D. J., Paltán, H. A., García, L. E., Ray, P., and St. George Freeman, S. (2021). Water-related infrastructure investments in a changing environment: a perspective from the World Bank. Water Policy 23, 31–53. doi: 10.2166/wp.2021.265
Schoenefeld, J. J., Schulze, K., and Bruch, N. (2022). The diffusion of climate change adaptation policy. Wiley Interdiscip. Rev. Clim. Chang. 13:e775. doi: 10.1002/wcc.775
Schwarz, A., Ray, P., Wi, S., Brown, C., He, M., and Correa, M. (2018). Climate change risks faced by the California Central Valley water resource system. California’s Fourth Climate Change Assessment. Publication Number: CCCA4-EXT-2018-001.
Seigerman, C. K., McKay, S. K., Basilio, R., Biesel, S. A., Hallemeier, J., Mansur, A. V., et al. (2023). Operationalizing equity for integrated water resources management. J. Am. Water Resourc. Assoc. 59, 281–298. doi: 10.1111/1752-1688.13086
Shrestha, B., Cochrane, T. A., Caruso, B. S., Arias, M. E., and Wild, T. B. (2021). Sediment management for reservoir sustainability and cost implications under land use/land cover change uncertainty. Water Resour. Res. 57:e2020WR028351. doi: 10.1029/2020WR028351
Smith, P. (2004). Carbon sequestration in croplands: the potential in Europe and the global context. Eur. J. Agron. 20, 229–236. doi: 10.1016/j.eja.2003.08.002
Steinschneider, S., and Brown, C. (2013). A semiparametric multivariate, multisite weather generator with low-frequency variability for use in climate risk assessments. Water Resour. Res. 49, 7205–7220. doi: 10.1002/wrcr.20528
Steinschneider, S., Ray, P., Rahat, S. H., and Kucharski, J. (2019). A weather-regime-based stochastic weather generator for climate vulnerability assessments of Water systems in the western United States. Water Resour. Res. 55, 6923–6945. doi: 10.1029/2018WR024446
Taner, M. Ü., Ray, P., and Brown, C. (2017). Robustness-based evaluation of hydropower infrastructure design under climate change. Clim. Risk Manag. 18, 34–50. doi: 10.1016/j.crm.2017.08.002
Taner, M. Ü., Ray, P., and Brown, C. (2019). Incorporating multidimensional probabilistic information into robustness-based water systems planning. Water Resour. Res. 55, 3659–3679. doi: 10.1029/2018WR022909
Tang, H., Yang, Z., Guo, Z., Yang, C., Huang, F., and Ran, R. (2022). The willingness to pay for agricultural irrigation water and the influencing factors in the Dujiangyan irrigation area: an empirical double-hurdle model analysis. Front. Environ. Sci. 10:906400. doi: 10.3389/fenvs.2022.906400
Tebaldi, C., Hayhoe, K., Arblaster, J. M., and Meehl, G. A. (2006). Going to the extremes: an intercomparison of model-simulated historical and future changes in extreme events. Clim. Chang. 79, 185–211. doi: 10.1007/s10584-006-9051-4
Tsakiris, G. P., and Loucks, D. P. (2023). Adaptive water resources management under climate change: an introduction. Water Resour. Manag. 37, 2221–2233. doi: 10.1007/s11269-023-03518-9
Turner, S. W. D., and Galelli, S. (2016). Water supply sensitivity to climate change: an R package for implementing reservoir storage analysis in global and regional impact studies. Environ. Model Softw. 76, 13–19. doi: 10.1016/j.envsoft.2015.11.007
Ussiri, D. A., and Lal, R. (2017). Carbon sequestration for climate change mitigation and adaptation. Cham, Switzerland: Springer International Publishing, 287–325.
van der Pol, T. D., van Ierland, E. C., and Gabbert, S. (2017). Economic analysis of adaptive strategies for flood risk management under climate change. Mitig. Adapt. Strateg. Glob. Chang. 22, 267–285. doi: 10.1007/s11027-015-9637-0
Van Heerden, P. S., and Walker, S. (2016). Upgrading of SAPWAT3 as a management tool to estimate the irrigation Water use of crops: Revised Edition: SAPWAT4: Executive Summary. Water Research Commission.
Verschuur, J., Li, S., Wolski, P., and Otto, F. E. L. (2007). Climate change as a driver of food insecurity in the 2007 Lesotho-South Africa drought. Sci. Rep. 11:3852. doi: 10.1038/s41598-021-83375-x
Villa, J. A., and Bernal, B. (2018). Carbon sequestration in wetlands, from science to practice: an overview of the biogeochemical process, measurement methods, and policy framework. Ecol. Eng. 114, 115–128. doi: 10.1016/j.ecoleng.2017.06.037
Vogl, A. L., Bryant, B. P., Hunink, J. E., Wolny, S., Apse, C., and Droogers, P. (2017). Valuing investments in sustainable land management in the Upper Tana River basin, Kenya. J. Environ Manage, 195, 78–91.
Walker, W. E., Harremoës, P., Rotmans, J., van der Sluijs, J. P., van Asselt, M. B. A., Janssen, P., et al. (2003). Defining uncertainty: a conceptual basis for uncertainty Management in Model-Based Decision Support. Integr. Assess. 4, 5–17. doi: 10.1076/iaij.4.1.5.16466
Wang, T., Liu, B., Zhang, J., and Li, G. (2019). A real options-based decision-making model for infrastructure investment to prevent rainstorm disasters. Prod. Oper. Manag. 28, 2699–2715. doi: 10.1111/poms.13074
Wegner, G., and Pascual, U. (2011). Cost-benefit analysis in the context of ecosystem services for human well-being: a multidisciplinary critique. Glob. Environ. Chang. 21, 492–504. doi: 10.1016/j.gloenvcha.2010.12.008
Whateley, S., Steinschneider, S., and Brown, C. (2014). A climate change range-based method for estimating robustness for water resources supply. Water Resour. Res. 50, 8944–8961. doi: 10.1002/2014WR015956
Wilby, R. L., and Wigley, T. M. (1997). Downscaling general circulation model output: a review of methods and limitations. Prog. Phys. Geogr. 21, 530–548. doi: 10.1177/030913339702100403
Wilks, D. S., and Wilby, R. L. (1999). The weather generation game: a review of stochastic weather models. Prog. Phys. Geogr. 23, 329–357. doi: 10.1177/030913339902300302
World Bank (2010). Economic evaluation of climate change adaptation projects: Approaches for the agricultural sector and beyond. Washington, DC: World Bank.
World Bank Independent Evaluation Group (2010). Cost-benefit analysis in World Bank projects. Washington, DC: World Bank.
Wreford, A., Dittrich, R., and van Der Pol, T. D. (2020). The added value of real options analysis for climate change adaptation. Wiley Interdiscip. Rev. Clim. Chang. 11:e642. doi: 10.1002/wcc.642
Xiong, J., Guo, S., Chen, D., Zhong, Y., Liu, B., and Yin, J. (2022). Past and future terrestrial water storage changes in the lower Mekong River basin: the influences of climatic and non-climatic factors. J. Hydrol. 612:128275. doi: 10.1016/j.jhydrol.2022.128275
Keywords: willingness-to-pay, adaptations, Lesotho irrigation, climate change uncertainty, robust water investments, cost benefit analysis
Citation: Odunola T, Bryant B, Rowcroft P, Zhilyaev S, Richardson J, Boehlert B, Castillo D, Strzepek K, Myer E, Brown C and Ray P (2024) Estimating willingness-to-pay for adaptations to climate change in water resources projects: demonstration for Lesotho irrigation investment. Front. Water. 6:1442023. doi: 10.3389/frwa.2024.1442023
Received: 01 June 2024; Accepted: 22 November 2024;
Published: 11 December 2024.
Edited by:
Jos Timmerman, Waterframes, NetherlandsReviewed by:
Ismael Aguilar-Barajas, Monterrey Institute of Technology and Higher Education (ITESM), MexicoCopyright © 2024 Odunola, Bryant, Rowcroft, Zhilyaev, Richardson, Boehlert, Castillo, Strzepek, Myer, Brown and Ray. This is an open-access article distributed under the terms of the Creative Commons Attribution License (CC BY). The use, distribution or reproduction in other forums is permitted, provided the original author(s) and the copyright owner(s) are credited and that the original publication in this journal is cited, in accordance with accepted academic practice. No use, distribution or reproduction is permitted which does not comply with these terms.
*Correspondence: Tolulope Odunola, b2R1bm9sdG9AbWFpbC51Yy5lZHU=
Disclaimer: All claims expressed in this article are solely those of the authors and do not necessarily represent those of their affiliated organizations, or those of the publisher, the editors and the reviewers. Any product that may be evaluated in this article or claim that may be made by its manufacturer is not guaranteed or endorsed by the publisher.
Research integrity at Frontiers
Learn more about the work of our research integrity team to safeguard the quality of each article we publish.