- 1LEESU, Ecole des Ponts, Univ Paris Est Creteil, Marne-la-Vallée, France
- 2LHSV, Ecole des Ponts, EDF R&D, Chatou, France
- 3Syndicat Marne Vive, Saint-Maur-des-Fossés, France
- 4Prolog Ingenierie, Paris, France
Dissolved organic matter (DOM) plays a crucial role in freshwater ecosystem function. Monitoring of DOM in aquatic environments can be achieved by using fluorescence spectroscopy. Particularly, DOM fluorescence can constitute a signature of microbiological contamination with a potential for high frequency monitoring. However, limited data are available regarding urban waterbodies. This study considers fluorescence data from field campaigns conducted in the Paris metropolitan region: two watercourses (La Villette basin and the river Marne), two stormwater network outlets (SO), and a wastewater treatment plant effluent (WWTP-O). The objectives of the study were to characterize the major fluorescence components in the studied sites, to investigate the impact of local rainfall in such components and to identify a potential fluorescence signature of local microbiological contamination. The components of a PARAFAC model (C1-C7), corresponding to a couple of excitation (ex) and emission (em) wavelengths, and the fluorescence indices HIX and BIX were used for DOM characterization. In parallel, fecal indicator bacteria (FIB) were measured in selected samples. The PARAFAC protein-like components, C6 (ex/em of 280/352 nm) and C7 (ex/em of 305/340 nm), were identified as markers of microbial contamination in the studied sites. In the La Villette basin, where samplings covered a period of more than 2 years, which also included similar numbers of wet and dry weather samples, the protein-like components were significantly higher in wet weather in comparison to dry weather. A positive relationship was obtained between C6 and FIB. In urban rivers, the high frequency monitoring of C6 levels would support the fecal contamination detection in rivers. In addition, it could help targeting specific field campaigns to collect comprehensive dataset of microbiological contamination episodes.
1 Introduction
In freshwaters, dissolved organic matter (DOM) plays a key role in ecosystem functioning. Part of the DOM pool is associated to bacterial degradation and nutrient availability (Wetzel, 1992; Boavida and Wetzel, 1998), acting as a supplier of biologically accessible organic substances that sustains heterotrophic aquatic organisms. DOM may also attenuate light intensity (Morris et al., 1995; Markager and Vincent, 2000), mobilize and transport contaminants (Polubesova et al., 2007; Mladenov et al., 2010), and affect the concentration of dissolved oxygen in the water (McCabe et al., 2021). Changes in DOM composition may reflect the variation of the status of an ecosystem caused by land use and climate change (Wilson and Xenopoulos, 2009; Williams et al., 2010).
In urban areas, water sports and recreative activities in open waters are increasingly popular. However, these activities can expose users to waterborne diseases when the water quality is poor due to fecal pollution. The microbiological quality of water bodies is assessed through microorganism indicators of fecal contamination. In the United States, the Clean Water Act (USEPA, 1977), and in Europe, the European Bathing Water Directive (BWD, 2006/7/EC) (EU, 2006), require the monitoring of fecal contamination through fecal indicator bacteria (FIB). Escherichia coli (E. coli) and intestinal enterococci (IE) are well established indicators and are recommended for freshwater microbiological quality assessment (USEPA, 1986). In fecal contaminated waters, E. coli is present in greater concentrations than IE. Edberg et al. (2000) recommended to use E. coli as the best indicator, with IE as a complementary one. However, the standard methods of FIB enumeration require more than 24 h to provide results. For a reactive contamination detection, a fast-monitoring technique is required.
Fluorescence spectrophotometry has been widely used for characterizing DOM in surface waters (Cumberland et al., 2012; Romero González-Quijano et al., 2022), ground waters (Frank et al., 2018; Sorensen et al., 2020), marine waters (Parlanti et al., 2000; Kim et al., 2020), and for microbial quality assessment (Baker et al., 2015; Sciscenko et al., 2022). It can be used to assess DOM composition, DOM source, type, and microbiological activity (Coble, 1996).
Fluorescent dissolved organic matter (FDOM) contains molecular groups called fluorophores which, when excited by specific UV wavelengths emit fluorescence (Fellman et al., 2010). The fluorophores identified in freshwaters are associated with different types of dissolved organic matter and can be mostly attributed to humic-like (peaks A, C and M) and protein-like (peak T) components. Peak A is associated with terrestrial components. Peak C is often linked to anthropogenic activities such as agriculture. Peak M is associated with marine humic-like components. Peak T may indicate microbial activity (Coble, 1996).
Fluorescence intensity ratios can be used to assess the origin and transformation degree of DOM. Three fluorescence indices are commonly used: BIX, HIX and FI. The biological index (BIX) was introduced by Huguet et al. (2009), based on the freshness index (ratio β:α) from Parlanti et al. (2000). BIX is an indicator of the contribution of recently autochthonous produced DOM. It represents more recently derived DOM, including those freshly transferred from algae to the DOM pool, and the more decomposed DOM.
The humification index (HIX) is related to the extent of humification of organic matter. It was introduced by Zsolnay et al. (1999), denoted HIXZsolnay, who assumed that humification is associated with an increase of molecular weight. The emission spectra of these more condensed fluorescing molecules tend to shift to longer emission wavelengths. It was used to estimate the degree of DOM aromatization in soil, and firstly applied to aquatic systems by Huguet et al. (2009). It is the ratio of the sum of the fluorescence intensities between the emission wavelengths of 435 nm and 480 nm and the sum of the intensities emitted between 300 nm and 345 nm, at excitation wavelength of 254 nm.
Ohno (2002) proposed a modified HIX calculation, denoted HIXOhno, introducing a second inner-filter effect correction. With this new ratio, HIX ranges from 0 to 1, also increasing with the increase of DOM aromatization degree. Since HIX values from Ohno’s expression are independent of the concentrations of humic-like substances, it facilitates the comparison of results across different studies. In this paper, both HIX expressions were compared.
The fluorescence index (FI) was first introduced by McKnight et al. (2001). It is also known as f450/f500 index, used by Huguet et al. (2009), and later modified by Cory and McKnight (2005). It allows to determine the source of DOM either microbial (≥1.8) or terrestrial (≤1.4) (Fellman et al., 2010).
Independent fluorescence components, which can be associated to different origins of DOM, can be obtained through the application of the parallel factor (PARAFAC) method to fluorescence excitation-emission matrices (EEMs). PARAFAC is a powerful tool on the characterization and quantification of DOM in different aquatic environments (Stedmon and Bro, 2008).
In urban waterbodies, during rain events, wastewater discharge and runoff affect the water quality and change the DOM composition (Xenopoulos et al., 2021). Humic-like components are associated with terrestrial organic matter such as run-off water, and protein-like components are associated with a high level of microbial activity, such as wastewater. However, few field data are available in urban waters.
The main objective of this study was to use the fluorescence spectroscopy for the characterization of DOM in urban waters with contrasting origins and FIB levels. The variation of fluorescence indices and PARAFAC components was analyzed in an EEM dataset of 414 samples. The components from PARAFAC model, specific to our dataset, were associated to the reference fluorophores from Coble (1996), peaks T, M, C and A, for characterization.
Fluorescence data were collected in waterbodies located in the Paris metropolitan region, France. The sampling sites are as follows: two watercourses, La Villette basin and the river Marne; two stormwater network outlets (SO); one wastewater treatment plant outlet (WWTP-O). Samples were collected during dry and wet weather. In the La Villette basin, regular field campaigns were conducted for 29 months (October 2020–March 2023), in three sampling points. In the river Marne, samples were collected in 19 different points along a stretch of around 30 km for one summer (2022).
In the samples from SO and WWTP-O, and in the samples from one sampling point from La Villette basin and one from the river Marne, the enumeration of fecal indicator bacteria (FIB) E. coli and intestinal enterococci (IE) were conducted. The fluorescence intensities of all samples were normalized in Raman Units and the fluorescence indices were computed.
The specific objectives of the study were:
i. Identifying the major fluorescence components in different investigated urban waters.
ii. Examining spatial variation of FDOM components and the impact of local rainfall episodes in these components.
iii. Exploring the information provided by FDOM about microbiological water quality for monitoring purposes and identifying a potential fluorescence signature of local contamination due to SO and WWTP effluents or runoff during rainfall events.
2 Materials and methods
2.1 Sampling sites
The study sites are located in the metropolitan area of Paris, France. Two watercourses, the river Marne and La Villette basin, two SO and one WWTP-O were sampled (Figure 1; Supplementary Table S1).
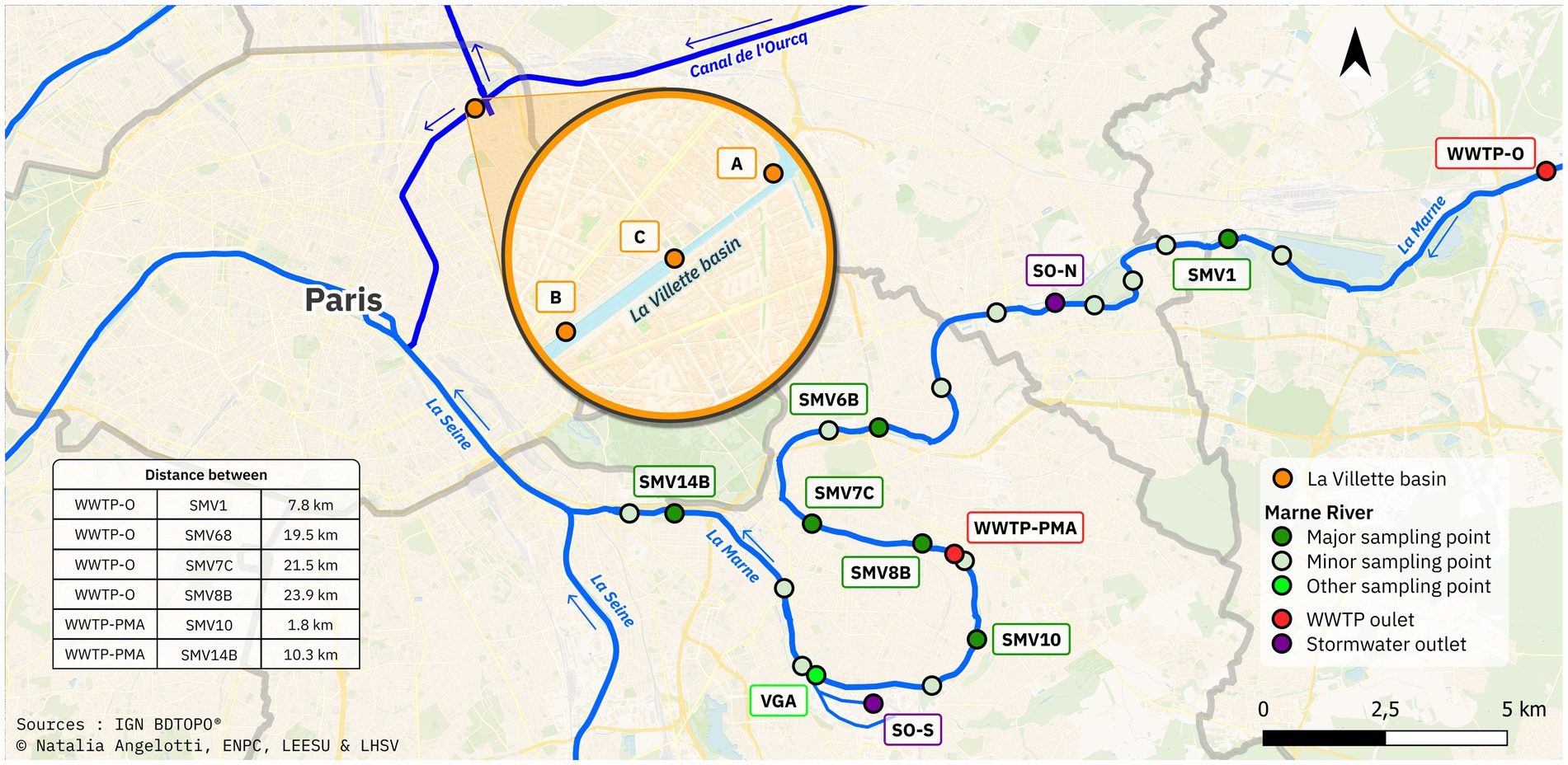
Figure 1. Sampling points. La Villette basin (orange), the river Marne (SMV in dark green; VGA in light green), stormwater outlets (SO-N and SO-S in purple), and WWTP outlet (WWTP-O in red).
2.1.1 Watercourses
La Villette waterbody, Paris (48.8918 N, 2.3855 E), is 1,500 m long and 3 m deep with a mean flowrate of 2 m3s−1. A bathing area is located downstream during summer since 2017. Three sampling points, A, B and C were located at the inlet of the canal (A), 1,000 m downstream, in the bathing area (B) and C between points A and B (Figure 1). The point A is 9.7 km downstream a SO and 20 km downstream the WWTP of Villeparisis, which has a mean discharge of 0.13 m3s−1 (Portail Assainissement Collectif, 2021). The samples were collected from October 2020 to March 2023, twice a month in average (85 campaigns in total).
The studied stretch of the river Marne is around 30 km long (48.8758 N 2.6721 E upstream, 48.8163 N 2.4414 E downstream). During the studied period (15/06 to 20/10/2022) the mean flowrate was around 36 m3s−1 (HydroPortail, 2022). The samples were collected, in 19 sampling points along the river stretch. The point VGA is located in Saint-Maur-des-Fossés (VGA sailing club). The other 18 points are part of Syndicat Marne Vive monitoring program (points SMV). Among the SMV points, 6 correspond to cities candidates to open bathing sites as a legacy of the Paris Olympic Games in 2024. They were considered as “main points” due to a three times higher sampling frequency than the other 12 minor sampling points (Figure 1).
In this river stretch, two outlets of wastewater treatment plants (WWTP) are located. The most upstream outlet is the WWTP St-Thibault-des-Vignes (hereafter WWTP-O), with 44,000 m3 wastewater treated per day (0.46 m3s−1) (Portail Assainissement Collectif, 2021). It discharges at 7.8 km upstream point SMV1. The second outlet is WWTP Paris Marne Aval (WWTP-PMA), with 35,000 m3 wastewater treated per day (0.41 m3s−1) (Portail Assainissement Collectif, 2021). It is located in Noisy-le-Grand, but it discharges at 1.8 km upstream point SMV10, in Saint-Maur-des-Fossés, on the second half of the studied stretch of the river Marne (Figure 1).
The land use data of the river Marne sampling sites was obtained from the open data platform from Institut Paris Region (2022). An area of 1 km2 immediately upstream the sampling points was considered to study the influence of their immediate surroundings. Due to the lack of samples from the SMV minor sampling points (n = 4 at each point), only the 6 major points were explored (Supplementary Figure S1). In the Marne SMV main sampling points, the main land use was impervious area, with average of 65%.
The distance between the sampling points (Supplementary Table S1) and the proportional land-use areas (Supplementary Figure S2; Supplementary Table S2) were calculated using an open-source geographic information system software (QGIS 3.30).
The sampling weather conditions were obtained from Météo-France stations: for La Villette, it was the Le Bourget weather station (48.96722 N, 2.42778 E, 9 km to the north), and for the Marne, the Torcy weather station (48.86361 N, 2.65111 E, 5 km to the east from SMV1).
2.1.2 Stormwater network and WWTP outlets
Two stormwater outlets (points SO-N and SO-S, Figure 1) were sampled. In both catchments, with a surface area, respectively, around 500 ha and 1,400 ha, the drainage network is separated and discharges into the river Marne. The sampling was conducted at the outlet of their respective catchment. At SO-N, the water is directly released into the river Marne. At SO-S, downstream the sampling point, the water is screened before going through two settling tanks and being released into the river Marne.
Samples of the treated water of WWTP St-Thibault were collected before its release into the river Marne (point WWTP-O in Figure 1). This WWTP is approximately 8 km upstream the SMV1 point with no intermediate WWTP.
2.2 Water sampling
Samples were collected during dry and wet weather. An overview of the water sampling is summarized in Supplementary Table S3. Wet weather is defined as a rainfall episode with precipitation height higher than 5 mm.day−1 over 3 days. Two types of sample collection were used: grab samples and 24-h integrated samples. The grab samples were collected at a punctual moment in time. The integrated samples were collected for 24 h and mixed, representing a mean sample of the collected period.
For the grab sampling, 100 mL of water were manually collected at 30 cm below the water surface at a pre-established schedule, covering wet and dry weather, in HDPE (high density polyethylene) flasks. The flasks were triple rinsed with the water from the site before sampling. At La Villette, grab samples were collected at points A and B, twice a month from October 2020 to March 2023 (n = 85 in each point). At point C, four grab samples were collected: one in 22/06/2021 and three in 16/12/2021. At the river Marne (SMV), grab samples were collected during summer 2022, three times a week in average (n = 189). Six of the SMV sampling points formed a main group, where sampling collection was 3 times a week. A total of 4 cruises were conducted in all 18 SMV points on the same day.
The 24-h samples were collected by an automatic sampler, 30 cm below the surface, at an hourly time step (1 L each), during wet and dry weather. All the samples were stored at 5°C and manually mixed on a 25 L container before being submitted to the lab analysis. 24-h samples were collected at La Villette point C (n = 10), the river Marne point VGA (n = 18), the stormwater outlet points SO-N (n = 9) and SO-S (n = 8) and the WWTP outlet WWTP-O (n = 5).
Summarizing all sampling sites, a total of 414 samples were collected (Supplementary Table S3). All samples were covered in aluminum foil for sunlight protection and stored at 4°C until being submitted to analysis in the laboratory within 24 h. Only the turbidity of samples from the studied SO (points SO-N and SO-S) were higher than 10 NTU. In order to avoid interference on fluorescence and absorbance measurements (Bedell et al., 2022), the samples were filtered with a 0.45 μm glass microfiber filter (Whatman® Cat No 1825 047). Due to high concentration of FDOM, samples from points SO-N, SO-S and WWTP-O were diluted with ultra-pure water with dilution factor of 1/5.
2.2.1 Fecal indicator bacteria measurement
FIB are represented by E. coli and IE. Sample analysis included E. coli and IE measurement of the 24-h integrated samples from Villette C (n = 10), Marne VGA (n = 18), SO-N (n = 9), SO-S (n = 8) and WWTP-O (n = 5), within 8 h.
From the 50 integrated samples, E. coli and IE were measured according to ISO-Norms ISO (1998b) and ISO (1998a), respectively. The results are expressed as the most probable number per 100 mL (MPN.100 mL−1).
2.3 Fluorescence measurements and fluorescence indices
Fluorescence measurements of all samples were performed on a Cary Eclipse Fluorescence Spectrophotometer (Agilent Technologies) with a 10 mm quartz cell, at the laboratory temperature (around 20°C). A simultaneous scan of excitation wavelength (ex) from 200 to 450 nm with 5-nm intervals and emission wavelength (em) from 250 to 550 nm with 2-nm intervals was performed, with a scan rate of 1,200 nm.min−1.
The absorbance was obtained with a Lambda 35 spectrophotometer (PerkinElmer). The samples were analyzed in a 10 cm quartz cell and scanned from 200 to 750 nm with 1-nm intervals, at a scan speed of 240 nm.min−1. In both fluorescence and absorbance analysis, an ultra-pure water sample blank was analyzed in the beginning of each series of measurements. The cell was tripled-rinsed with ultra-pure water and with the water sample between measurements.
The processing of the Excitation-Emission Matrix (EEM) spectra was conducted according to Murphy et al. (2013), using the drEEM toolbox for MATLAB® R2022b (MathWorks®, United States). The absorbance was used for inner filter effect correction (Parker and Barnes, 1957). The values were normalized to Raman Units (RU) according to Lawaetz and Stedmon (2009). The sample EEMs were subtracted by the corresponding blank EEM, removing Rayleigh and Raman scatters. The fluorescence intensities were linearly interpolated to a resolution of 1 nm in excitation and emission wavelengths to calculate and extract peaks and indices. Subsequently, they were smoothed with the Savitzky–Golay method, with a span of 21 data points and polynomial of degree 2.
From the corrected EEMs, the intensities of the fluorophores of interest (Coble, 1996) were obtained. Peak T is the intensity at wavelength excitation (ex) 275 nm and wavelength emission (em) 340 nm. Peak M is the maximum intensity at ex 312 nm and in the interval em 380–420 nm. Peak C is the maximum intensity in the intervals ex 320–360 nm and em 420–460 nm. Peak A is the maximum at ex 260 nm and em 400–460 nm.
In addition, several spectral indices were computed and used to describe DOM composition. The biological index (BIX) was computed at an excitation wavelength of 310 nm (Equation 1), according to Huguet et al. (2009).
Where and are the fluorescence intensities at 380 nm and 430 nm emission wavelength, respectively.
The humification index (HIX) was calculated at an excitation wavelength of 254 nm (Equation 2), according to Ohno (2002).
Where is the sum of the fluorescence intensity between 435 nm and 480 nm of emission wavelength, and is the sum of the fluorescence intensity between 300 nm and 345 nm emission wavelength. This index was compared with the HIX expression given by Zsolnay et al. (1999) (Equation 3). For brevity, when not specified, HIX will correspond to HIXOhno.
The fluorescence index (FI) was calculated as the ratio between the fluorescence intensity at excitation wavelength of 370 nm (Equation 4), according to Fellman et al. (2010).
Where and are the fluorescence intensities at 470 nm and 520 nm emission wavelength, respectively.
The locations of the fluorophores of interest (peaks T, M, C and A) and of the terms used to obtain the fluorescence indices BIX, HIX and FI on a fluorescence EEM are indicated in Supplementary Figure S3.
2.4 PARAFAC model
PARAFAC (PARAllel FACtor analysis) is a statistic tool applicable to data arranged in three (or more) arrays. The method is based on a generalization of Principal Component Analysis (PCA) to higher order arrays (Murphy et al., 2013). It can be used to decompose a EEM dataset into independent and distinct components that can be overlapped and combined to form the original dataset. The data signal is decomposed into a set of trilinear terms and a residual array, as in Equation 5.
where xijk is the fluorescence intensity of the ith sample at the jth emission mode and at the kth excitation mode; is named “score” and is directly proportional to the concentration of the fth analyte of sample i; is a scaled estimate of the emission spectrum of the fth analyte at emission wavelength j; is linearly proportional to the specific absorption coefficient of the fth analyte at excitation wavelength k; and is the residual noise that represents the variability not accounted for by the model (Murphy et al., 2013).
The PARAFAC model was run and validated using drEEM toolbox in MATLAB® R2022b (MathWorks®, United States), following the recommendations of Murphy et al. (2013) and Stedmon and Bro (2008). A final step of the dataset preprocessing is needed to run PARAFAC. The addition signal treatment to smooth the EEM dataset is detailed in Supplementary material S1A.
The PARAFAC model was developed with a total dataset of 414 samples. Thirty-one samples presented high residual values and were identified as outliers: 27 from La Villette basin, three from the Marne VGA and one from SO-N. The total rejection rate of 7.5% is similar for all study sites. After their removal, the final dataset contained 383 samples (n = 158 La Villette, n = 204 the Marne, n = 8 SO-N, n = 8 SO-S and n = 5 WWTP-O). Since PARAFAC modeling was used to identify the characteristic components of the dataset, the outliers must be removed.
PARAFAC models with F = 4 to 8 components (see Equation 5) were fitted to find the best number of components. The 7-component PARAFAC model was validated through a split-half analysis, a validation with multiple split-half tests to confirm if the model is identically produced in independent subsample groups of the final dataset.
The validation method was an alternating ‘S4C6T3’. This means that four split groups (S) were created. Each sample was alternately assigned to one of the four splits. The splits were assembled in six different combinations (C) to produce three split-half comparison tests (T).
The obtained components were compared to the data in the OpenFluor database1 (Murphy et al., 2014). Each component was related to one type of DOM (humic-like, protein-like) and associated with components from the open database for qualitative comparisons.
The fluorescence intensity at maximum loadings of emission (bif) and excitation (cif) modes (Supplementary material S2A) was used to quantify components in a sample, denoted Cif. For a given sample i and a component f, Cif is given by Equation 6.
The relative percentage of each component f was calculated by dividing the correspondent Cf value by the sum of Cf of each sample i (Equation 7). Cf represents its correspondent PARAFAC component f. Hereafter, the component and its fluorescence intensity will both be denoted Cf.
According to Murphy et al. (2013), PARAFAC modeling assumes that the fluorescence intensity increases approximately linearly with the concentrations of their corresponding component. Therefore, the relative percentage could be associated with the relative abundance of each component. However, this assumption must be carefully considered.
2.5 Data analysis
The fluorescence data was analyzed in five main steps. First, the fluorescence indices of all samples were compared. Second, the PARAFAC components of all samples were compared and associated with fluorophores from Coble (1996). Third, fluorescence indices from the river Marne samples collected at the same day were used to investigate the variation of DOM composition with land cover in river Marne. Fourth, fluorescence indices from La Villette basin samples were compared to assess the temporal variation of DOM composition, comparing samples from dry and wet weathers. Fifth, the relationship between FIB and protein-like PARAFAC components was obtained through a linear regression model.
Differences between the studied sample groups were determined by a Kruskal-Wallis test, a non-parametric one-way ANOVA test. This multiple comparison test among more than two groups determinates which group differs from the others by using average ranks of the medians of the groups (Helsel et al., 2020). For La Villette samples, Kruskal-Wallis test was also performed to investigate the variability of fluorescence indices between dry (n = 85) and wet (n = 98) weather samples.
Principal components analysis (PCA) was applied to reduce the dimensionality of fluorescence data (PARAFAC components and indices) and visualize the correlated variables among water types and among sampling sites.
3 Results
This section will successively set forth the results concerning: (1) the sampling weather conditions in La Villette and the river Marne; (2) the fluorescence indices of all sampling sites; (3) the PARAFAC model results; (4) the DOM composition among water types; (5) the impact of land-use in the Marne FDOM; (6) the time variation of FDOM in La Villette; (7) the relationship between PARAFAC components and FIB.
3.1 Sampling weather conditions in La Villette and in the river Marne
In La Villette basin, samples were collected over 29 months (October 2020 to March 2023). From the totality of the samples (n = 183), 54% (n = 98) were collected in wet weather, according to the definition of wet weather given in paragraph 2.2, and 46% (n = 85) in dry weather.
In the river Marne, a total of 34 sampling campaigns were conducted in 4 months (June to September 2022): 18 in VGA (4 in dry and 14 in wet weather) and 19 in SMV points (11 in dry and 5 in wet weather). The campaigns were predominantly conducted during dry weather (78% of the totality of samples).
The rainfall events associated to wet weather samples have different distribution of intensities in both watercourses, due to the difference in the length of the sampling periods. In La Villette, 24% of the wet weather samples were collected after rainfall between 8 and 10 mm in 72 h. The observed maximum intensity was of 48 mm in 72 h (Supplementary Figure S4A). In the river Marne, 27% of the wet weather samples were collected after rainfall between 18 and 20 mm in 72 h. The observed maximum intensity was of 26 mm in 72 h (Supplementary Figure S4B).
3.2 Fluorescence indices for DOM characterization
3.2.1 Comparison of HIX values
Huguet et al. (2009) associated DOM characteristics with a range of values calculated according to Zsolnay et al. (1999). To find the correspondent range values for Ohno’s expression (2002), which provides values in the range [0,1], better adapted to comparison between different water bodies, HIX was calculated with both expressions for all samples (Equation 8). The curve linking both expressions (Supplementary Figure S5) can be used for the conversion between HIXOhno and HIXZsolnay. This helps to compare the values found in different waterbodies from the literature.
The HIX ranges from Huguet et al. (2009) and their DOM characteristics were then associated with new HIX ranges from Ohno (2002). For comparative purposes, DOM characteristics for HIX and BIX ranges defined by Huguet et al. (2009) are grouped in Table 1 (shown in section 4.1.1).

Table 1. DOM characteristics associated with HIX values calculated according to Zsolnay et al. (1999) and Ohno (2002) expressions.
3.2.2 DOM characteristics and origin
The characteristics and origin of DOM can be estimated with the indices HIX and BIX (Figure 2; Supplementary Table S4).
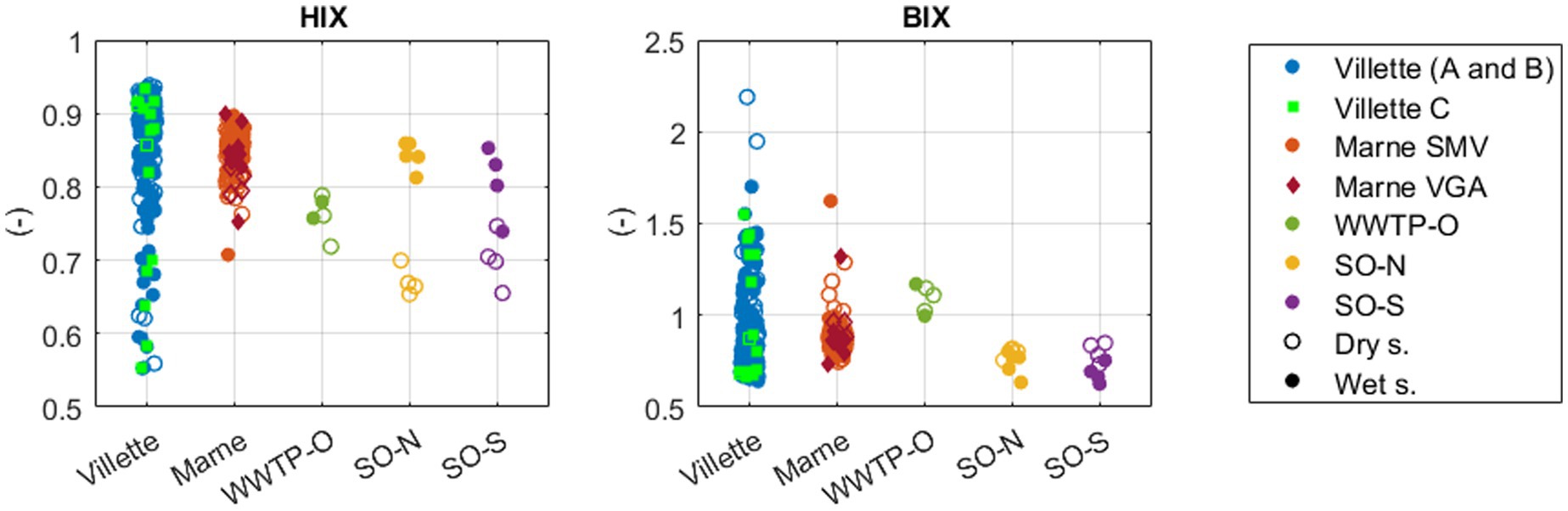
Figure 2. HIX and BIX values in La Villette basin at points (squares), the Marne SMV (o) and VGA (diamond), SO-N, SO-S and WWTP-O. Dry weather samples are open markers and wet weather samples are filled markers.
In La Villette (n = 183), HIX covers a range of [0.55–0.94], with an average of 0.86 ± 0.08. BIX covers a range of [0.64–2.19], with an average of 0.90 ± 0.26. In the river Marne (n = 207), HIX covers a range of [0.71–0.90], with an average of 0.85 ± 0.03. BIX covers a range of [0.73–1.62], with average of 0.87 ± 0.09. All the values were very similar along the stretch. They showed less variation than in La Villette.
In WWTP-O effluent (n = 5), a low variation of indices was observed. HIX was close to 0.80 and BIX was higher or equal to 1. This indicates a predominant biological origin of DOM. In SO-N (n = 9) and SO-S (n = 8), the ranges of HIX and BIX values were similar. Higher variations of HIX and BIX than in La Villette and the Marne were observed. HIX lower values in dry weather suggested lower humic character. BIX was lower than 1.0 at both sampling points.
According to Huguet et al. (2009), the combination of HIX and BIX values can be used to synthetize the DOM characteristics of the studied sites (mean with one-standard-deviation confidence ellipses are set forth in Figure 3). The river Marne samples showed weak humic character and an important biological activity. La Villette samples showed weak to strong humic character and low to high biological activity. WWTP-O effluents showed very low humic character and high biological origin. The HIX and BIX values of both SO samples are close. The humic character and the biological origin were relatively low. The samples of La Villette, the Marne and WWTP-O matched the classification. Differently, the SO samples were located outside of the expected categories, suggesting that the classification could be extended to different urban waters categories.
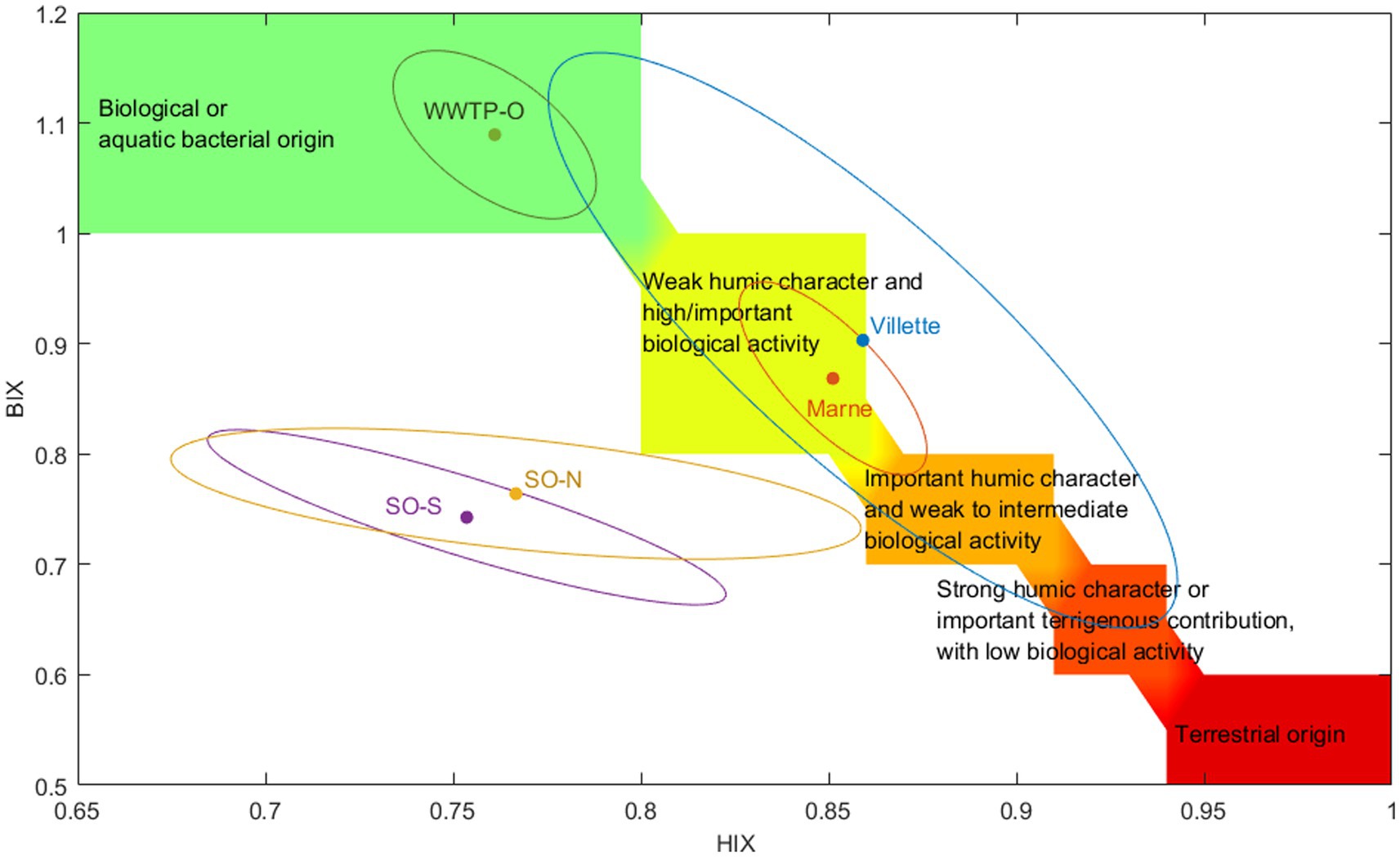
Figure 3. DOM characteristics associated with HIX and BIX according to Huguet et al. (2009); mean (filled circles) and one standard deviation (confidence ellipse) of the site samples.
3.3 PARAFAC model results
3.3.1 PARAFAC function
The first result was to define a function which could be applied, by using the identified PARAFAC components, to obtain the corresponding PARAFAC values for future samples.
The creation of a robust PARAFAC model requires a large number of EEMs with great diversity of DOM sources of diverse chemical quality. However, once the DOM fluorescence signal is decomposed, new EEMs from the same or similar study site, should not interfere in the obtained PARAFAC model. Therefore, these new EEMs should fit well in the existing model (Fellman et al., 2009; Murphy et al., 2011).
The defined function provides the new PARAFAC scores, and therefore Cf, on future samples from the same sampling sites. With the emission ( ) and excitation ( ) modes obtained from the original PARAFAC model, it was possible to obtain the score and compute Cf values of new EEMs (Fellman et al., 2009). To this end, the values of to obtain the minimum squared error εi in the sample i are computed by Equation 9.
3.3.2 PARAFAC components
A model with 7 components was validated by PARAFAC analysis (C1-C7, Supplementary Figure S6). Each component was associated with similar components from OpenFluor Database (Supplementary Table S5). The similarity score (Tucker congruence coefficient) of all the components was >0.95 for emission and excitation spectra loading, with global score > 0.90.
Components C1 to C5 were identified as humic-like components. C1, C2, C3 and C5 were identified as terrestrial humic-like components. The maximum loadings of C1, C2 and C5 were associated to peak A. C2 displayed a greater emission wavelength (red-shifted). The maximum loading of C3 was similar to peak C.
C4 was associated to humic-like substances produced in-situ by phytoplankton and microbial activity. The maximum loading of C4 was in lower emission wavelengths (blue-shifted) than peak C and in greater excitation wavelengths than peak M.
Components C6 and C7 were identified as protein-like, close to tryptophan-like fluorescence (peak T). The maximum loading of component C7 has greater excitation wavelength than peak T.
The components C1-C7 were represented by Cf, the fluorescence intensity at the maximum loading of excitation and emission modes for each component f. The components were grouped in terrestrial humic-like (C1, C2, C3 and C5), “low” humic-like (C4), with lower molecular weight, and protein-like (C6 and C7).
The fluorescence intensities (Supplementary Figure S7) and the relative percentage of PARAFAC components from all the sampling points in the five water bodies was estimated. The mean relative percentage of each component f (%Cf) was calculated for the five water bodies (Figure 4; Supplementary Table S6). The relative percentage of each component contributes to the signatures of SO and WWTP-O and of the watercourses La Villette and the Marne.
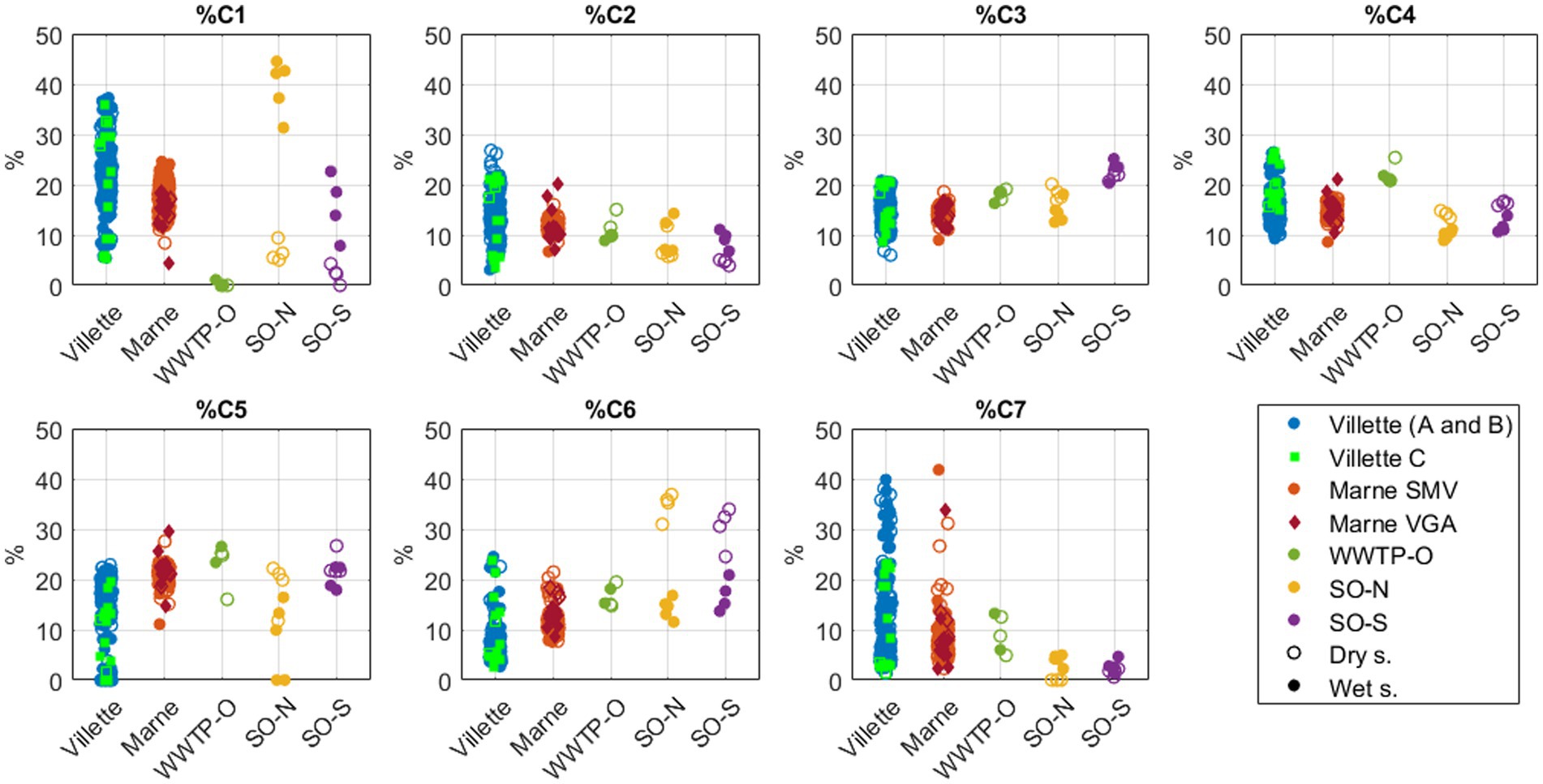
Figure 4. Relative percentage of PARAFAC components (%) in La Villette basin points A (blue), B (blue) and C (bright green), in the river Marne points SMV (orange) and VGA (dark red), in the outlet of WWTO-O (dark green) and the outlets from stormwater networks SO-N.
In La Villette, terrestrial humic-like composition was predominant on average (66.0%). The predominant terrestrial humic-like component and with higher variability was C1 (22.4% ± 6.6%), followed by C5 (15.0% ± 6.5%). Protein-like components represent 18.5%. The predominant protein-like component was C7 (11.7% ± 9.6%). It is more abundant and more variable than C6 (6.8% ± 4.0%). The highest variations of C1 and C7 occurred mostly during wet weather (Supplementary Figure S8).
In the river Marne, terrestrial humic-like composition was predominant (65.1%). The predominant humic-like component was C5 (20.8% ± 1.6%). C1 has the highest variability (17.8% ± 2.7%). The protein-like components represent 20.0%. The predominant protein-like component was C6 (11.8% ± 2.3%). The higher variability was associated to C7 (8.2% ± 4.4%) (Supplementary Figure S8).
In WWTP-O (Supplementary Figure S10), terrestrial humic-like components were the most abundant (52.3%). Protein-like components represented 25.6%. The predominant humic-like components were C5 (23.2% ± 4.2%), terrestrial humic-like, and C4 (21.9% ± 2.0%), “low” humic-like. The predominant protein-like component was C6 (16.5% ± 2.1%). The variability of the components was very low, as evidenced by their standard deviations (ranging from 0.5 to 4.2%) and narrow %Cf ranges [(0.0–26.5%)].
In both SO-N and SO-S (Supplementary Figure S10), terrestrial humic-like components were the most abundant (62.5 and 60.1%, respectively). Protein-like components represented 25.7 and 25.8%, respectively. The predominant terrestrial humic-like component in SO-N was C1 (24.9% ± 17.8), and in SO-S, C3 (22.5% ± 1.6%). The dominant protein-like component was C6 in both SO-N (23.4% ± 11.0%) and SO-S (23.6% ± 8.0%). These values are the highest measured in all samples. The components with the highest variability were C1 and C6. Higher values of %C1 and lower %C6 were obtained in wet weather, suggesting that they could be potentially used as signature of local precipitation.
In La Villette and the Marne, the average of relative percentage of all terrestrial humic-like components was around 66%, of “low” humic-like component was 15%, and of protein-like components, 19%. In SO, terrestrial humic-like components corresponded to 61%, “low” humic-like components to 13%, and protein-like components to 26%. In WWTP-O, terrestrial humic-like components represented 52% of components abundance, “low” humic-like component, 22%, and protein-like component, 26% (Figure 5). In SO and WWTP-O, protein-like components are more abundant than in La Villette and the river Marne. In WWTP-O “low” humic-like component was more abundant than in SO.
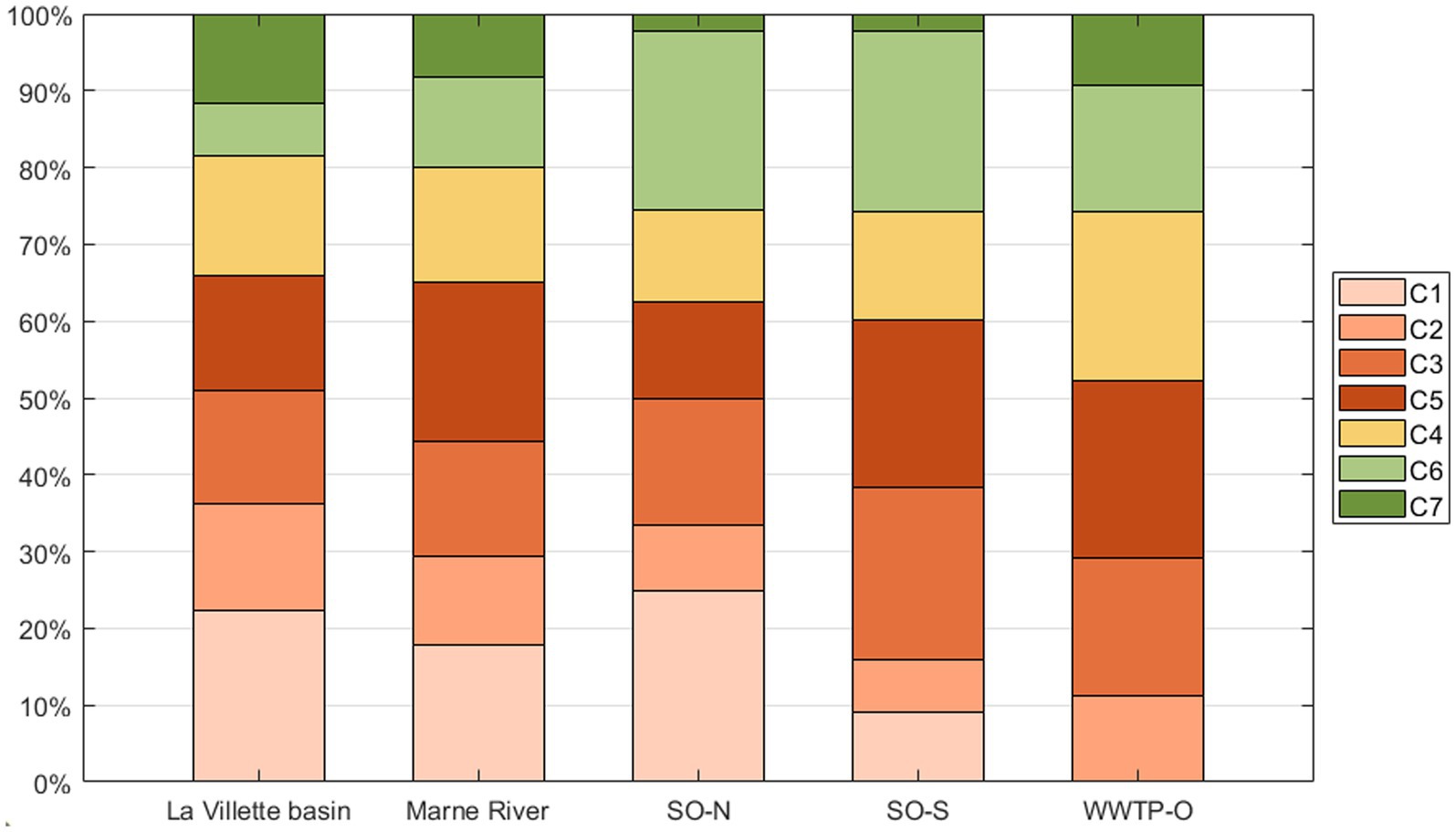
Figure 5. Relative abundance of the PARAFAC components. Components were gathered in three groups: terrestrial humic-like (orange), “low” humic-like (yellow) and protein-like (green).
3.4 Dissolved organic matter composition among water types
The distribution of the relative abundance of PARAFAC components among the different water types was compared through Kruskal-Wallis test. The average ranks of the median of each component for each water type was computed. The statistical similarity of each component among the water types is presented in Supplementary Figure S11.
Overall, the contribution of all PARAFAC components were very similar in SO and WWTP-O. The main difference between these groups were the low humic-like component C4 and the protein-like component C7. Both %C4 and %C7 were higher in WWTP-O and significantly different from the SO. In WWTP-O, %C4 was significantly higher from the other water types and %C7 was similar to the watercourses.
The highest %C6 values were measured in SO and WWTP-O. In contrast, %C6 in the river Marne was greater than in La Villette. This may suggest that the outlets which discharge in the river Marne could be the main source of C6 in the river. Higher variability in the composition of protein-like components could contribute to the detection of change in microbial DOM. In the watercourses, the protein-like component with higher standard deviation and wider range was C7. In SO and WWTP-O, it was C6 (Supplementary Table S6). This suggests that %C7 could be an indicator of microbial DOM change in La Villette and the river Marne.
The median HIX of La Villette (0.89) was greater than in the Marne (0.86). The median BIX of La Villette (0.80) was lower than in the Marne (0.86). La Villette and the Marne HIX medians were significantly different (Supplementary Figure S11), indicating a potentially higher humification level in La Villette. However, HIX and BIX median values between both watercourses were close enough to be part of the same category, indicating a DOM composition with an important humic character and an intermediate biological activity. Among the samples from SO and WWTP-O, HIX was very similar. WWTP-O samples presented the highest mean BIX values, being significantly similar from the river Marne samples.
The differences between the variances in La Villette and the Marne was likely due to the different characteristics of the sampling periods of each site. In La Villette, samples were collected for more than 2 years (October 2020 to March 2023), covering all seasons, and including 38 rainfall events of intensities from [2.6–48.2 mm] in 72 h. In the Marne, samples were collected only during Summer 2022, in scheduled dates regardless the weather conditions.
A PCA analysis was performed to analyze the patterns of PARAFAC components and fluorescence indices HIX and BIX among the different water types. The two first axis explained 76.4% of the total variance (Figure 6). In the plan defined by axis PC1 and PC2, the watercourses (La Villette and the Marne river) were well separated from SO and WWTP-O.
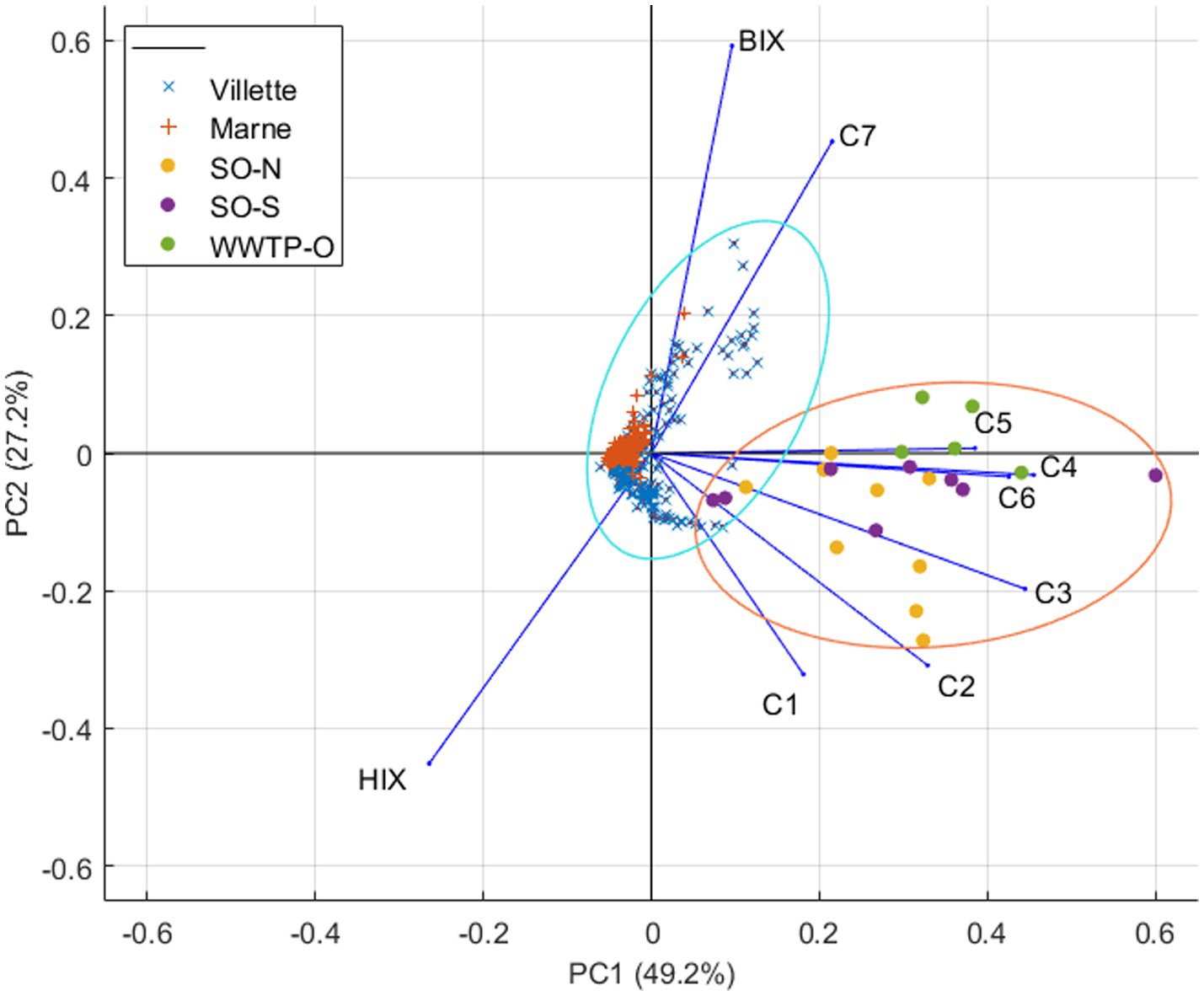
Figure 6. PCA of PARAFAC components, HIX and BIX, with dataset of La Villette basin (blue, n = 183), Marne (orange, n = 207), SO-N (yellow, n = 9), SO-S (purple, n = 8), and WWTP-O (green, n = 5). Blue ellipse groups watercourses samples (La Villette basin and the river Marne) and orange ellipse the SO and WWTP-O.
The first axis, PC1, explained 49.2% of total variance. PC1 was defined positively by C4, C5, and C6. SO and WWTP-O are distributed along PC1, close to C1 to C6. The second axis, PC2, explained 27.2% of total variation. It was defined positively by C7 and BIX, and negatively by HIX. La Villette data spread along PC2, mainly driven by C7 and BIX. The dispersion of La Villette samples was higher than the Marne samples. This could be due to the longer studied period in La Villette, covering more than 2 years (29 months) instead of one summer in the Marne.
3.5 Impact of land-use in the river Marne
In the river Marne, the influence of land-use on fluorescence components and their variation along the river stretch, upstream to downstream, were investigated. Samples collected at the same day were compared. A total of 15 sampling campaigns were conducted in the main 6 SMV points (SMV1, SMV6B, SMV7C, SMV8B, SMV10 and SMV14B).
Two WWTP outlets are located in the studied stretch. The most upstream, WWTP-O, is located 7.8 km upstream SMV1. The second, WWTP-PMA, is 1.8 km upstream SMV10. The distance between the sampling points and their nearest WWTP outlet at upstream was considered (DW): the distance between WWTP-O and SMV1, SMV6B, SMV7C, SMV8B; and the distance between WWTP-PMA and SMV10, SMV14B (Supplementary Table S7).
The mean values of the PARAFAC component proportion and the fluorescence indices were very close among all 6 SMV points, from upstream to downstream (Figure 7A). Among the terrestrial humic-like components, C1 and C5 have the highest mean values, respectively of 18 and 21%.
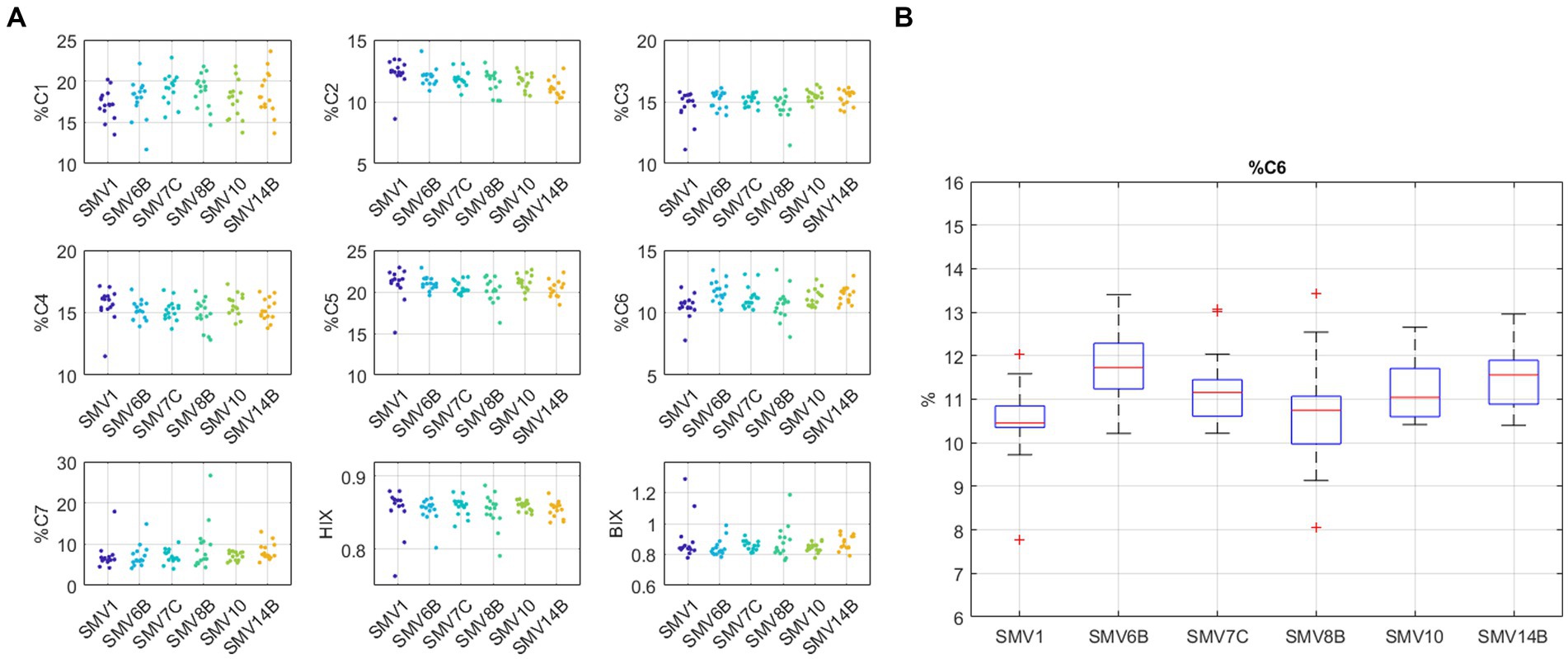
Figure 7. In the 6 main SMV sampling points (river Marne): (A) Swarm charts of %C1 to %C7, HIX and BIX and (B) Boxplot of %C6.
Regarding the statistical similarity of the protein-like components, some differences appeared in C6 (Figure 7B). At SMV6B, %C6 presented the highest median (11.7) and the highest 3rd quartile (13.4%). Between SMV1 and SMV6B, the 12 km-stretch contains 26 stormwater network outlets, including SO-N. The high %C6 level at SMV6B could be due to large outlet loading. %C6 was also slightly higher at SMV10 than SMV8B. Between SMV8B and SMV10 is located the outlet of WWTP-PMA, suggesting an impact of the WWTP on the protein-like DOM composition.
A Principal Component Analysis (PCA) was computed including the seven PARAFAC components, the distance from the closest upstream WWTP outlet (DW) and the land cover in the 1km2 upstream each sampling point, grouped in “urban green area,” “natural green area,” “impervious area” and “water area” (Figure 8). The first two axis, PC1 and PC2, explained 53.8% of total variance of the dataset. PC1 explained 34.5% of the total variance. It was predominantly defined by the positive loadings of the C1 to C6, and the negative loadings of DW. PC2 explained 19.3% of total variance. It was positively defined by BIX, C7 and the impervious area land-use, and negatively defined by urban green area and HIX.
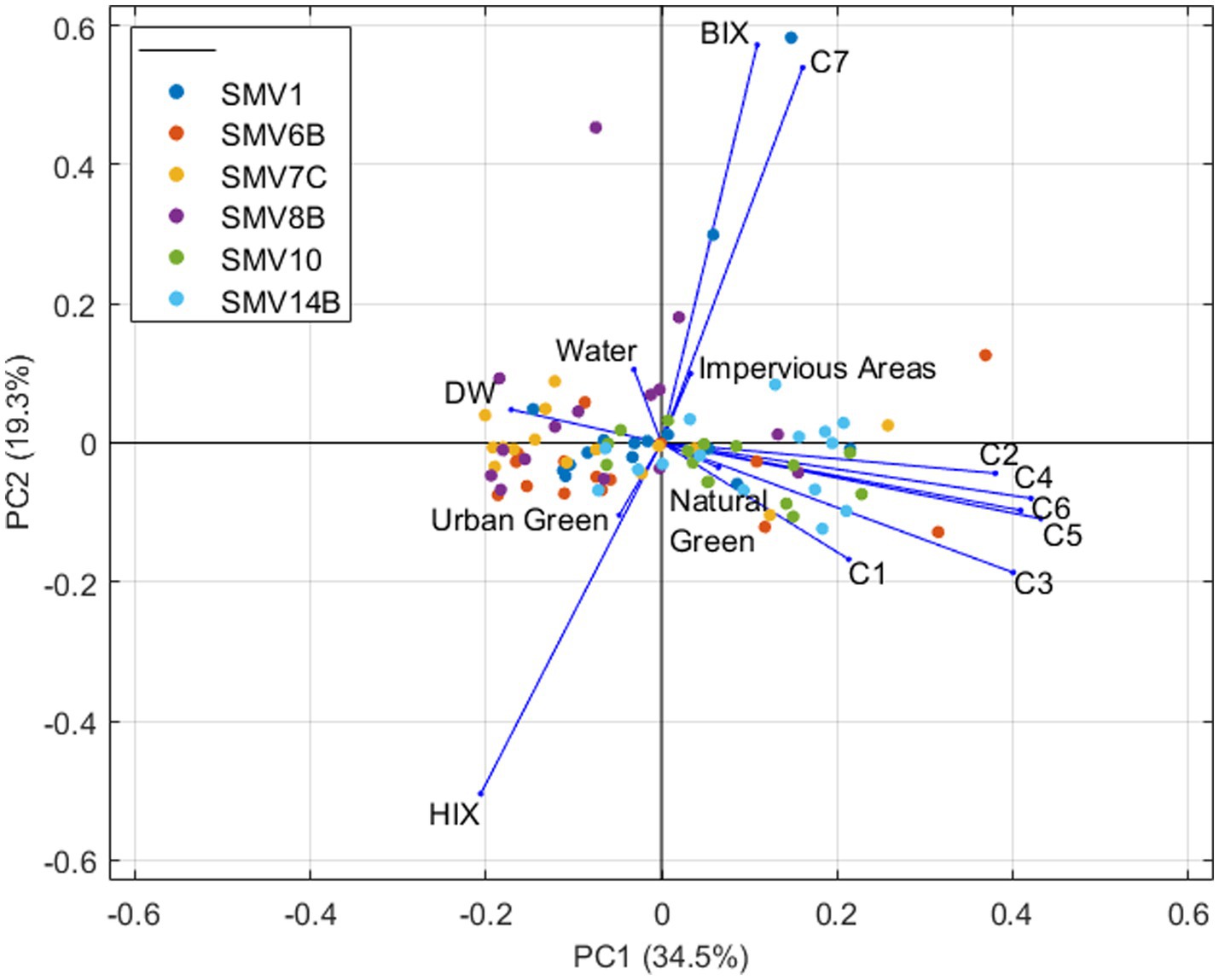
Figure 8. PCA of the main group of 6 SMV river Marne points with C1-C7, land-use and distance from the nearest WWTP outlet upstream (DW).
The values were mainly distributed along PC1. The components C1 to C6 were closely distributed and orthogonally to C7. C1 to C6 were inversely related to the distance from a WWTP outlet upstream, suggesting that the lower the distance, the higher the loadings of these components.
A slight influence of the land use was observed. Impervious areas appeared to be more related to BIX and C7; they may contribute to higher microbial loading. Urban green areas appeared to be more related to HIX; they may have higher DOM humification level.
3.6 Rainfall impact on DOM composition in La Villette
The impact of rainfall on fluorescent DOM in La Villette was explored. Samples were collected during dry (85 samples) and wet weather (98 samples), over 2.5 years (October 2020 to March 2023). A Kruskal-Wallis test was performed for assessing the differences between the samples.
The relative contribution of the PARAFAC components, HIX and BIX were compared to identify a potential signature of local contamination due to stormwater network or WWTP outlets or runoff during rainfall events. No significant differences were observed between dry and wet weather for %C3, %C5, %C7 and BIX (Figure 9).
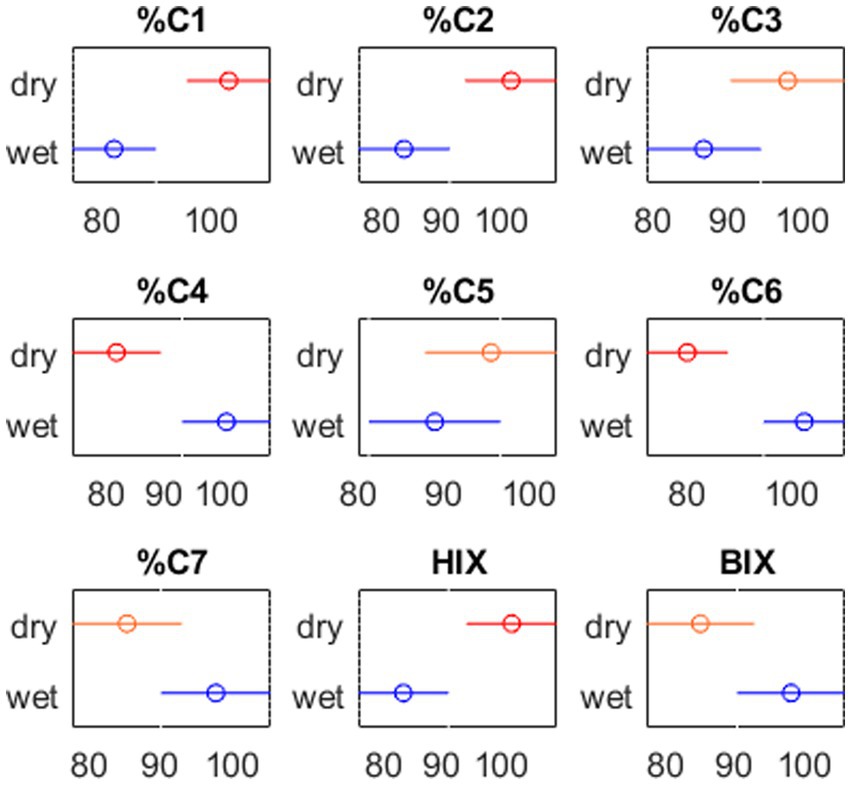
Figure 9. Mean ranks of Kruskal-Wallis test of relative PARAFAC components, HIX and BIX, for comparison between dry (n = 85, red) and wet (n = 98, blue) weather La Villette samples.
Significant differences were identified in %C1, %C2, %C4, %C6 and HIX. In wet weather, the humic-like components %C1, %C2 and HIX were lower than in dry weather. The values of %C4, a component related to microbial activity (Galletti et al., 2019), and %C6, a protein-like component, were significantly higher. The increase of the components C6 and C4 suggests an increase of microbial loadings during rainy events.
3.7 Relationship of FIB and PARAFAC components
The enumeration of FIB, E. coli and IE in MPN.100 mL−1, was performed in samples from all water types. The sampling points were La Villette point C (n = 10), the Marne VGA (n = 18), WWTP-O (n = 5), SO-N (n = 9) and SO-S (n = 8). The same sampling protocol was applied (sub-section 2.2).
The log10-transformed values (in MPN.100 mL−1) are presented in Figure 10. The order of magnitude of the FIB varied among the sampling sites. For E. coli, in La Villette, it varied by a factor between 2 and 3, in the Marne VGA, between 2 and 4, in WWTP-O, between 4 and 5, in SO-N, between 5 and 6, and in SO-S, between 5 and 7. The order of magnitude of IE, in La Villette varied by a factor between 0 and 2, in the Marne VGA, between 0 and 3, in WWTP-O, between 3 and 4, in SO-N, between 4 and 5, in SO-S, between 4 and 6.
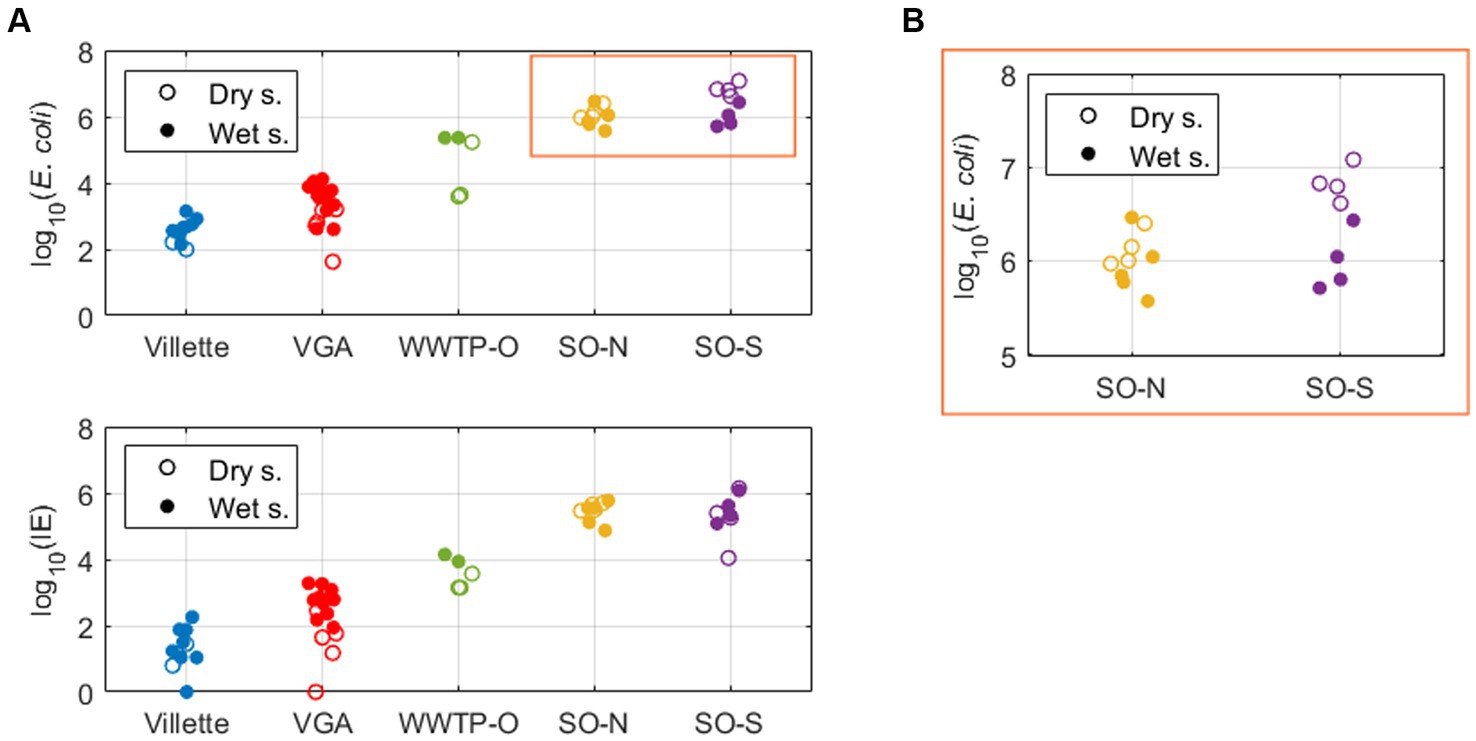
Figure 10. (A) log-10 transformed FIB dataset in MPN.100mL-1 of the samples from La Villette point C, the Marne VGA, WWTP-O, SO-N and SO-S; (B) zoom in SO-N and SO-S E. coli data. Dry samples (Dry s.) were collected in dry weather and wet samples (Wet s.) were collected in wet weather.
Interestingly, in the stormwater network outlets SO-N and SO-S, during dry weather, higher E. coli values were observed in comparison to wet weather. This unexpected result suggests a constant contamination on the network by sewage infiltration or misconnection of the sewage network. During rain events, the microbiological contaminants are probably diluted by drainage water.
Among all samples, E. coli and IE had a strong relationship (R2 = 0.88, p-value<0.001; Supplementary Figure S12). This result reinforced the use of FIB as indicators of fecal contamination.
The correlation between log10-transformed FIB and PARAFAC components was explored using all FIB data (n = 50). A good relationship with the protein-like components, C6 or C7, was expected. A significant relationship with log10(C6) with both FIB, E. coli (R2 = 0.72, Figure 11A, Equation 10) and IE (R2 = 0.61, Figures 11B), was obtained (p < 0.001).
Where E. coli is in MPN.100 mL−1 and C6 in RU. According to this relationship, considering the E. coli threshold for sufficient bathing water quality (900 MPN.100 mL−1), an indicative warning level could be based on C6 < 0.13 RU.
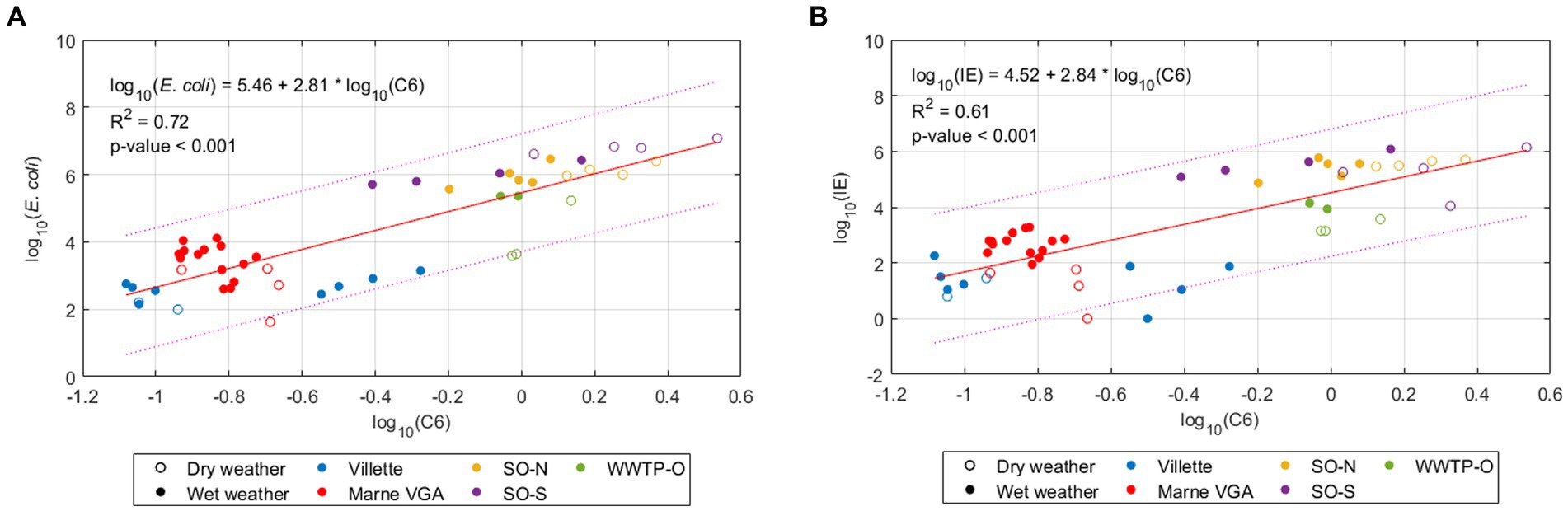
Figure 11. Linear relationships between: (A) log10(C6) and log10(E. coli) (n = 50) and (B) log10(C6) and log10(IE) (n = 50).
The relationships between C7 and both FIB were not significant (p > 0.5). Therefore, they were not considered.
In 2023, samples were collected in the river Marne at an additional point, point IMP, close to SMV8B. The samples (n = 16) were collected from 25/05–03/07/2023, following the same sampling protocol as in point VGA (24-h integrated samples). C6 values were obtained from the PARAFAC function (Equation 9 from 3.3.1). The relationship between E. coli and C6 was applied for E. coli estimation (Figure 12A).
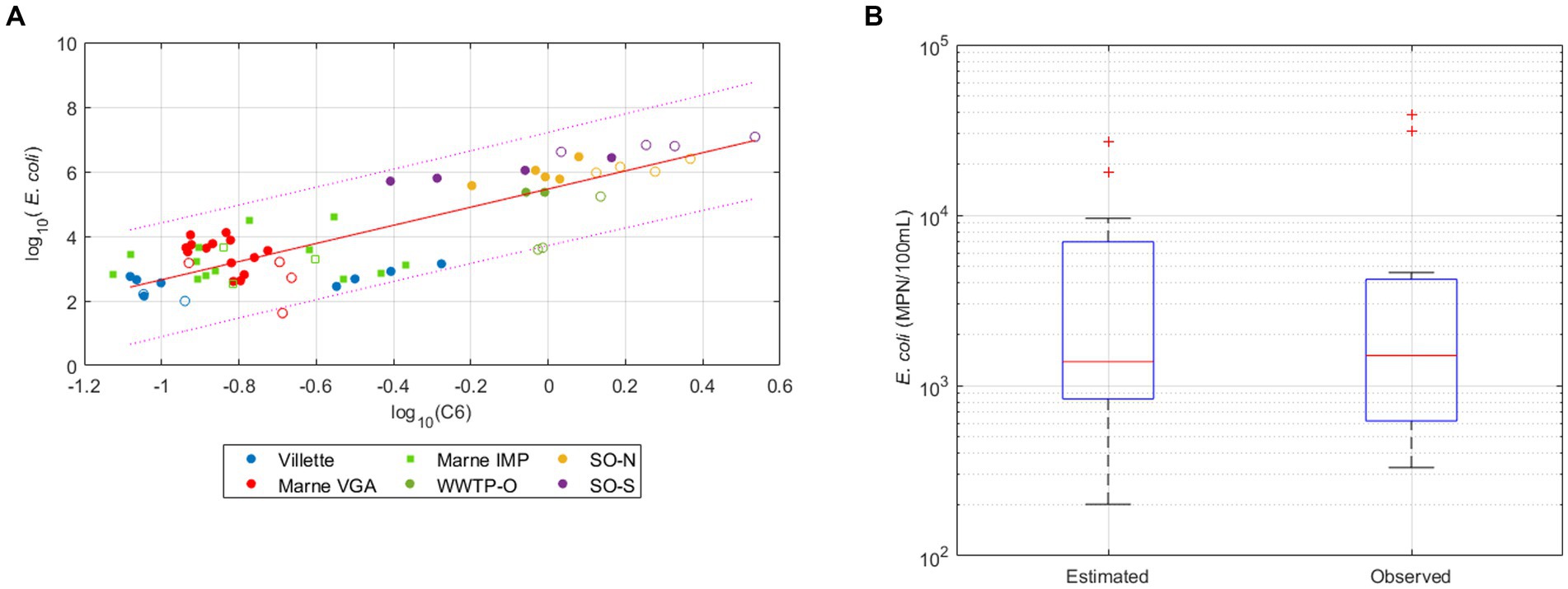
Figure 12. (A) E. coli and C6 relationship with 2023 additional values superimposed (Marne IMP, green squares). Wet weather in filled markers and dry weather in empty markers; (B) Estimated E. coli from C6 values and observed E. coli from additional samples from Marne IMP (n = 16).
The median of E. coli in the IMP samples (2023) was 1,500 MPN.100 mL−1. The estimated E. coli median from Equation 10 was 1,378 MPN.100 mL−1, very close to the observed value. The distribution of observed and estimated E. coli was very similar (Figure 12B). This result contributes to the validation of Equation 10.
The observed E. coli values can be used to determine the overpassing of the bathing threshold (900 MPN.100 mL−1). From 25/05 to 03/07/2023, 11 out 16 occurrences (68%) matched the observations, either higher or lower than the threshold. Of the five incorrect estimations, three overestimations and two underestimations were found.
4 Discussion
Hereafter, the results concerning the fluorescence indices and the PARAFAC components in the studied sites are discussed and compared to other results in similar waterbodies. The land cover in the river Marne and the variation of fluorescence components in La Villette are also discussed, as well as the relationship between E. coli and protein-like components.
4.1 Fluorescent dissolved organic matter patterns in different urban water types
4.1.1 Fluorescence indices HIX, BIX and FI
HIX computed according to Zsolnay et al. (1999) or to Ohno (2002) has different scales. The proposed conversion between these scales allows us to compare the values in a unified way (Table 1). BIX is associated to the biological activity from first stages (around 2 days) of organic matter decomposition. BIX and HIX values are related to the DOM characteristics: they are complementary and expected to be inversely proportional to each other. Samples with high bacterial activity should have high BIX and present low humic character, associated to low HIX, and vice versa (Huguet et al., 2009).
The samples of La Villette and the river Marne matched well the typology proposed by Huguet et al. (2009). However, the stormwater network outlets (SO-N and SO-S) do not fit within this typology (see Figure 3). Therefore, such type of urban water probably holds a different FDOM signature, which could be used to extend the classification of DOM characteristics.
Previous HIX and BIX data from the Seine and the Marne were obtained in September 2012 by Nguyen (2014). The mean HIX values from La Villette (0.86) and the Marne (0.85) are similar to 2012 values found in the river Marne (0.88) in the same stretch as ours. In the Seine, downstream Paris, close values (0.87) from La Villette were found. The mean BIX values from La Villette (0.90) and the Marne (0.87) were greater than in the Seine downstream Paris (0.75) and in the Marne (0.67) in September 2012.
HIX values in La Villette and the Marne were compared to 9 rivers and 9 streams, in Berlin area, including two heavily polluted rivers due to WWTP effluents (Romero González-Quijano et al., 2022). HIX in La Villette and in the Marne were similar to the Berlin streams (0.86) and rivers (0.85).
FI was introduced by McKnight et al. (2001) for the source assessment of humic substances. Low FI values (~1.2) indicate terrestrial derived DOM and high FI (~1.8), microbial derived DOM (Fellman et al., 2010). In this study, FI was higher than 1.8 in all samples. In La Villette and in the Marne, FI was close, 1.97 and 2.13, respectively. In the SO and WWTP effluent, FI was higher, around 2.4 (Supplementary Table S4). In Berlin rivers, FI was 1.66, but in the two highly polluted rivers, FI was higher (~1.8) (Romero González-Quijano et al., 2022). In an anthropized watershed in Spain (Marcé et al., 2021), FI was 1.4 in the Ter River but it was higher, 1.6, in the impaired streams and WWTP effluent. Based in high FI values, a dominant microbial contribution on DOM composition cannot be concluded. However, high FI values may indicate a highly urbanized watershed with limited terrestrial DOM components.
4.1.2 PARAFAC components
As the position of the characteristic fluorescence peaks, such as peak T, can be slightly shifted according to the water matrix, PARAFAC model achieves a more accurate location of the fluorescence peaks.
In the obtained 7-component PARAFAC model, the protein-like components were C6, close to peak T, and C7. C6 was similar to the main protein-like fluorophore in WWTP effluents (Cohen et al., 2014). C6 displayed a high correlation with biogeochemical parameters, such as organic carbon, phosphates and fecal bacteria (Tedetti et al., 2012; Cohen et al., 2014).
The comparison of the PARAFAC components among different studies is not straightforward. Similar water types may be represented by different PARAFAC models with different characteristic components (Meng et al., 2013; Lambert et al., 2017; Marcé et al., 2021; Romero González-Quijano et al., 2022). To overcome this limitation, we considered two fractions: protein-like components and humic-like components.
In the Berlin region, Romero González-Quijano et al. (2022) obtained a 7-component PARAFAC model for a dataset including rivers, streams, ponds and lakes. They obtained two protein-like components. In the rivers, the average of protein-like components was 16.0%, slightly lower than La Villette (18.5%) and the Marne (20.0%). In the two highly polluted rivers, the protein-like components were 20.5%, similar to the values found in WWTP-O (25.6%). A percentage of protein-like components higher than 20% might indicate a microbiological pollution such as WWTP effluents.
Among our study sites, the tryptophan-like component (C6) was high in WWTP-O effluent. Based on the OpenFluor database, C6 was found very similar to a component found by Cohen et al. (2014) in four WWTPs (Israel). C6 was also a dominant component in other WWTP studies (Li et al., 2020; Sciscenko et al., 2022). C6 might be considered as a signature of a microbial activity in a WWTP effluent.
A high percentage of C6 was observed in the stormwater outlets in dry weather (Figure 4). It was higher than in wet weather, contrary to what is expected in a stormwater network. A probable wastewater contamination may occur in the SO during dry weather. This was corroborated by higher E. coli (Figure 10B) in dry than in wet weather samples.
In lakes, tryptophan-like component can also be considered as a potential indicator of eutrophication. In Chinese lakes, a component close to peak T and to our C6 was found (Ren et al., 2021). It was associated to pollutant-degrading activity of microalgae, including cyanobacteria. Therefore, in future studies, it will be interesting to explore the sensitivity of C6 to eutrophication indices.
4.2 Land cover around the river Marne study sites
In the river Marne, according to the PCA results, impervious areas may be associated to BIX and the protein-like component C7. Urban green areas may be associated to HIX, suggesting higher humification level of the FDOM. These results are consistent with previous studies. Urbanized areas have a high microbial organic matter composition, resulting in higher BIX values, and less plant–soil-derived character, with lower HIX (Lambert et al., 2017). The relationship between microbial activity (BIX and C7) and impervious areas may origin from surface runoff, composed by different sources of pollution, and from WWTP effluents (Romero González-Quijano et al., 2022).
4.3 Rainfall impact in fluorescence components in La Villette basin
In general, humic-like fluorescence is strongly controlled by hydrological processes. Protein-like fluorescence is tightly linked with biological processes, depending of water temperature, sunlight intensity, water turbidity, etc. (Parlanti et al., 2000; Fellman et al., 2010; Meng et al., 2013). Higher humic character was found during high-water than in low-water periods in the catchments of the Seine (France) (Nguyen, 2014) and in a river in the United States (Jaffé et al., 2008).
In urban catchments, microbiological contamination peaks occur following rainfall episode (Burnet et al., 2019). Therefore, rainfall may have a significant impact in the protein-like components. In La Villette, the protein-like components C6 and C7 in wet weather samples increased more than the humic-like components. Therefore, the relative contribution of protein-like components was higher. The wet weather samples presented lower humic character (lower HIX and lower terrestrial humic-like components) and higher microbial loading (higher protein-like components) than the dry weather samples. These results were consistent with the values obtained in an urban river in China (Yuan et al., 2023).
It must be highlighted that La Villette is a highly controlled hydraulic system, with many sluices upstream and one sluice downstream. The variation of discharge is very limited, even during heavy rainfall episodes, reducing their impact on humic-like components. Protein-like components from direct runoff and stormwater networks are dominant. The protein-like components can be used as a fingerprint of rainy events. An improved assessment of rain impact on FDOM in La Villette, would require sampling before, during and after rainy events (for example, every 5 h during 4 days).
4.4 Escherichia coli and protein-like component relationship
The link between E. coli and tryptophan-like component has been explored for several years (Cumberland et al., 2012; Baker et al., 2015; Frank et al., 2018; Nowicki et al., 2019; Bedell et al., 2020). The obtained relationships were very variable according to the study sites (Table 2), probably because the water matrix composition changes with the catchment hydrogeological characteristics.
Similar relationships between E. coli and peak T were obtained in South Africa and in the United Kingdom. In South Africa, the relationship (n = 136, r = 0.75) was obtained from the samples of six rivers (Baker et al., 2015). In the United Kingdom, the relationship (n = 46, r = 0.87) was established from the samples from urban rivers and a sewage treatment plant effluent (Cumberland et al., 2012).
A relationship (Equation 10) between C6 and E. coli was obtained with our study site samples (n = 50; r = 0.85). More data of C6 and FIB covering a wider range of FIB values, will allow to have a statistically stronger relationship. Measurement of turbidity and dissolved organic carbon may also provide additional information to improve the relationship between FIB and FDOM.
This relationship was applied to additional samples from the Marne, collected in 2023. The distribution of estimated and observed E. coli were close. This result opens the way to the application of Equation 10 to determine, from FDOM measurements, the overpassing of the E. coli bathing threshold (900 MPN.100 mL−1).
5 Conclusion
The origin, terrestrial or biological, of the main components of the studied urban waters was identified through the fluorescence of dissolved organic matter (FDOM). The continuum of the water types, from WWTP effluent, stormwater outlet, urban river, to a very urbanized basin, cover a wide range of FDOM fingerprints, extending the classification of surface water FDOM.
The validated 7-component PARAFAC model was effective in characterizing the different urban water types. Components C6 and C7, as well as BIX, were identified as fingerprints of microbial contamination. These protein-like components may serve as valuable markers for the assessment of microbial contamination in urban water samples.
In La Villette, the impact of rainfall on water microbiological quality was observed. The FDOM values demonstrated a significant increase in microbial activity following rainy periods. FDOM analysis can provide a sensitive technique for detecting changes in water quality after rainfall events.
In SO and WWTP-O effluent, component C6 exhibited high values. In agreement with previous studies, protein-like fluorescence components, such as C6, could be used as effective indicators for monitoring microbiological water quality. An increase of C6 level would suggest a microbial contamination and deserves further attention for managing water resources.
Urban waterways are highly dynamic systems, with fluctuating microbiological quality depending on the hydrometeorology and sewer accidents. For different uses of water resources, such as bathing, the implementation of an active management has been recommended by the World Health Organization (2021) and the Bathing Water Directive (BWD, 2006/7/EC). A real time monitoring of FDOM could be coupled with a numerical modeling in such a warning system aimed to support the management decision-making.
Data availability statement
The original contributions presented in the study are publicly available. This data can be found here: https://openfluor.lablicate.com/of/measurement/15067.
Author contributions
NP: Conceptualization, Data curation, Formal analysis, Investigation, Methodology, Writing – original draft, Writing – review & editing. RC: Conceptualization, Formal analysis, Methodology, Project administration, Writing – original draft, Writing – review & editing. AG: Formal analysis, Investigation, Writing – review & editing, Visualization. FL: Funding acquisition, Investigation, Writing – review & editing. CT: Writing – review & editing, Resources, Investigation. MN: Investigation, Writing – review & editing. AJ: Writing – review & editing, Resources. FP: Investigation, Writing – review & editing. MS: Investigation, Writing – review & editing. PD: Writing – review & editing, Resources. BV-L: Conceptualization, Data curation, Formal analysis, Funding acquisition, Methodology, Project administration, Supervision, Writing – original draft, Writing – review & editing.
Funding
The author(s) declare that financial support was received for the research, authorship, and/or publication of this article. This work was supported by Ecole des Ponts ParisTech. It is part of the OPUR project and of the Eurostars project (E115737-FORBATH). NP was granted a doctoral fellowship from Ecole des Ponts ParisTech.
Acknowledgments
The authors are grateful to Ville de Paris (Service des Canaux and Service Technique de l’Eau et de l’Assainissement), Eau de Paris, Syndicat Marne Vive, Syndicat Intercommunal d’Assainissement de Marne-la-Vallée (SIAM), CD93 (Direction de l’Eau et de l’Assainissement), VGA sailing club, Guinguette de l’Ile du Martin-Pêcheur and Marin d’Eau Douce for the support and access to the sampling points. We thank CD94 DSEA (Direction des Services de l’Environnement et de l’Assainissement) and Prolog Ingénierie for the support in the field work in the river Marne. We thank the interns for the sampling and laboratory analysis: Vincent Thiercelin, Ana Carolina Pires, Clélia Rancinangue, Romero Lacerda and Anna Luong-Dihn-Giap. We also thank the interns Amanda Tolentino Mendes and Lorena Araújo for the fluorescence data treatment and analysis.
Conflict of interest
The authors declare that the research was conducted in the absence of any commercial or financial relationships that could be construed as a potential conflict of interest.
Publisher’s note
All claims expressed in this article are solely those of the authors and do not necessarily represent those of their affiliated organizations, or those of the publisher, the editors and the reviewers. Any product that may be evaluated in this article, or claim that may be made by its manufacturer, is not guaranteed or endorsed by the publisher.
Supplementary material
The Supplementary material for this article can be found online at: https://www.frontiersin.org/articles/10.3389/frwa.2024.1358483/full#supplementary-material
Footnotes
References
Baker, A., Cumberland, S. A., Bradley, C., Buckley, C., and Bridgeman, J. (2015). To what extent can portable fluorescence spectroscopy be used in the real-time assessment of microbial water quality? Sci. Total Environ. 532, 14–19. doi: 10.1016/j.scitotenv.2015.05.114
Bedell, E., Harmon, O., Fankhauser, K., Shivers, Z., and Thomas, E. (2022). A continuous, in-situ, near-time fluorescence sensor coupled with a machine learning model for detection of fecal contamination risk in drinking water: design, characterization and field validation. Water Res. 220:118644. doi: 10.1016/j.watres.2022.118644
Bedell, E., Sharpe, T., Purvis, T., Brown, J., and Thomas, E. (2020). Demonstration of tryptophan-like fluorescence sensor concepts for fecal exposure detection in drinking water in remote and resource constrained settings. Sustain. For. 12:3768. doi: 10.3390/su12093768
Boavida, M.-J., and Wetzel, R. G. (1998). Inhibition of phosphatase activity by dissolved humic substances and hydrolytic reactivation by natural ultraviolet light. Freshw. Biol. 40, 285–293. doi: 10.1046/j.1365-2427.1998.00349.x
Burnet, J.-B., Sylvestre, É., Jalbert, J., Imbeault, S., Servais, P., Prévost, M., et al. (2019). Tracking the contribution of multiple raw and treated wastewater discharges at an urban drinking water supply using near real-time monitoring of β-d-glucuronidase activity. Water Res. 164:114869. doi: 10.1016/j.watres.2019.114869
Coble, P. G. (1996). Characterization of marine and terrestrial DOM in seawater using excitation-emission matrix spectroscopy. Mar. Chem. 51, 325–346. doi: 10.1016/0304-4203(95)00062-3
Cohen, E., Levy, G. J., and Borisover, M. (2014). Fluorescent components of organic matter in wastewater: efficacy and selectivity of the water treatment. Water Res. 55, 323–334. doi: 10.1016/j.watres.2014.02.040
Cory, R. M., and McKnight, D. M. (2005). Fluorescence spectroscopy reveals ubiquitous presence of oxidized and reduced Quinones in dissolved organic matter. Environ. Sci. Technol. 39, 8142–8149. doi: 10.1021/es0506962
Cumberland, S., Bridgeman, J., Baker, A., Sterling, M., and Ward, D. (2012). Fluorescence spectroscopy as a tool for determining microbial quality in potable water applications. Environ. Technol. 33, 687–693. doi: 10.1080/09593330.2011.588401
Edberg, S. C., Rice, E. W., Karlin, R. J., and Allen, M. J. (2000). Escherichia coli: the best biological drinking water indicator for public health protection. J. Appl. Microbiol. 88, 106S–116S. doi: 10.1111/j.1365-2672.2000.tb05338.x
EU. (2006). Directive 2006/7/EC of the European Parliament and of the Council of 15 February 2006 concerning the management of bathing water quality and repealing Directive 76/160/EEC. Available at: https://eur-lex.europa.eu/legal-content/GA/TXT/?uri=CELEX:32006L0007 (Accessed November 16, 2020).
Fellman, J. B., Hood, E., and Spencer, R. G. M. (2010). Fluorescence spectroscopy opens new windows into dissolved organic matter dynamics in freshwater ecosystems: a review. Limnol. Oceanogr. 55, 2452–2462. doi: 10.4319/lo.2010.55.6.2452
Fellman, J. B., Miller, M. P., Cory, R. M., D’Amore, D. V., and White, D. (2009). Characterizing dissolved organic matter using PARAFAC modeling of fluorescence spectroscopy: a comparison of two models. Environ. Sci. Technol. 43, 6228–6234. doi: 10.1021/es900143g
Frank, S., Goeppert, N., and Goldscheider, N. (2018). Fluorescence-based multi-parameter approach to characterize dynamics of organic carbon, faecal bacteria and particles at alpine karst springs. Sci. Total Environ. 615, 1446–1459. doi: 10.1016/j.scitotenv.2017.09.095
Galletti, Y., Gonnelli, M., Retelletti Brogi, S., Vestri, S., and Santinelli, C. (2019). DOM dynamics in open waters of the Mediterranean Sea: New insights from optical properties. Deep Sea Research Part I: Oceanographic Research Papers 144, 95–114. doi: 10.1016/j.dsr.2019.01.007
Helsel, D. R., Hirsch, R. M., Ryberg, K. R., Archfield, S. A., and Gilroy, E. J. (2020). Statistical methods in water resources. Reston, VA: U.S. Geological Survey.
Huguet, A., Vacher, L., Relexans, S., Saubusse, S., Froidefond, J. M., and Parlanti, E. (2009). Properties of fluorescent dissolved organic matter in the Gironde estuary. Org. Geochem. 40, 706–719. doi: 10.1016/j.orggeochem.2009.03.002
HydroPortail, E. (2022). Station hydrométrique - F664 0001 04: La Marne à Gournay-sur-Marne - Pont - Fiche de synthèse - Données hydrologiques de synthèse | SCHAPI - HydroPortail. HydroPortail. Available at: https://www.hydro.eaufrance.fr/stationhydro/F664000104/synthese (Accessed November 29, 2022).
Institut Paris Region. (2022). Occupation du sol 2021 en 11 postes de légende d’Île-de-France. Available at: https://data-iau-idf.opendata.arcgis.com/datasets/iau-idf::occupation-du-sol-2021-en-11-postes-de-l%C3%A9gende-d%C3%AEle-de-france/about (Accessed March 14, 2023).
ISO. (1998a). ISO 7899-1:1998 - Water quality — Detection and enumeration of intestinal enterococci — Part 1: Miniaturized method (Most Probable Number) for surface and waste water. Available at: https://www.iso.org/standard/14852.html (Accessed February 22, 2022).
ISO. (1998b). ISO 9308-3:1998 - Water quality — Detection and enumeration of Escherichia coli and coliform bacteria–Part 3: Miniaturized method (Most Probable Number) for the detection and enumeration of E. coli in surface and waste water. ISO. Available at: https://www.iso.org/cms/render/live/en/sites/isoorg/contents/data/standard/02/08/20878.html (Accessed June 15, 2020).
Jaffé, R., McKnight, D., Maie, N., Cory, R., McDowell, W. H., and Campbell, J. L. (2008). Spatial and temporal variations in DOM composition in ecosystems: the importance of long-term monitoring of optical properties. J. Geophys. Res. Biogeo. 113:683. doi: 10.1029/2008JG000683
Kim, J., Kim, Y., Kang, H.-W., Kim, S. H., Rho, T., and Kang, D.-J. (2020). Tracing water mass fractions in the deep western Indian Ocean using fluorescent dissolved organic matter. Mar. Chem. 218:103720. doi: 10.1016/j.marchem.2019.103720
Lambert, T., Bouillon, S., Darchambeau, F., Morana, C., Roland, F. A. E., Descy, J.-P., et al. (2017). Effects of human land use on the terrestrial and aquatic sources of fluvial organic matter in a temperate river basin (the Meuse River, Belgium). Biogeochemistry 136, 191–211. doi: 10.1007/s10533-017-0387-9
Lawaetz, A. J., and Stedmon, C. A. (2009). Fluorescence intensity calibration using the Raman scatter peak of water. Appl. Spectrosc. 63, 936–940. doi: 10.1366/000370209788964548
Li, J., Wang, L., Geng, J., Li, S., Yu, Q., Xu, K., et al. (2020). Distribution and removal of fluorescent dissolved organic matter in 15 municipal wastewater treatment plants in China. Chemosphere 251:126375. doi: 10.1016/j.chemosphere.2020.126375
Marcé, R., Verdura, L., and Leung, N. (2021). Dissolved organic matter spectroscopy reveals a hot spot of organic matter changes at the river–reservoir boundary. Aquat. Sci. 83:67. doi: 10.1007/s00027-021-00823-6
Markager, S., and Vincent, W. F. (2000). Spectral light attenuation and the absorption of UV and blue light in natural waters. Limnol. Oceanogr. 45, 642–650. doi: 10.4319/lo.2000.45.3.0642
McCabe, K. M., Smith, E. M., Lang, S. Q., Osburn, C. L., and Benitez-Nelson, C. R. (2021). Particulate and dissolved organic matter in Stormwater runoff influences oxygen demand in urbanized headwater catchments. Environ. Sci. Technol. 55, 952–961. doi: 10.1021/acs.est.0c04502
McKnight, D. M., Boyer, E. W., Westerhoff, P. K., Doran, P. T., Kulbe, T., and Andersen, D. T. (2001). Spectrofluorometric characterization of dissolved organic matter for indication of precursor organic material and aromaticity. Limnol. Oceanogr. 46, 38–48. doi: 10.4319/lo.2001.46.1.0038
Meng, F., Huang, G., Yang, X., Li, Z., Li, J., Cao, J., et al. (2013). Identifying the sources and fate of anthropogenically impacted dissolved organic matter (DOM) in urbanized rivers. Water Res. 47, 5027–5039. doi: 10.1016/j.watres.2013.05.043
Mladenov, N., Zheng, Y., Miller, M. P., Nemergut, D. R., Legg, T., Simone, B., et al. (2010). Dissolved organic matter sources and consequences for Iron and arsenic mobilization in Bangladesh aquifers. Environ. Sci. Technol. 44, 123–128. doi: 10.1021/es901472g
Morris, D. P., Zagarese, H., Williamson, C. E., Balseiro, E. G., Hargreaves, B. R., Modenutti, B., et al. (1995). The attenuation of solar UV radiation in lakes and the role of dissolved organic carbon. Limnol. Oceanogr. 40, 1381–1391. doi: 10.4319/lo.1995.40.8.1381
Murphy, K. R., Hambly, A., Singh, S., Henderson, R. K., Baker, A., Stuetz, R., et al. (2011). Organic matter fluorescence in municipal water recycling schemes: toward a unified PARAFAC model. Environ. Sci. Technol. 45, 2909–2916. doi: 10.1021/es103015e
Murphy, K. R., Stedmon, C. A., Graeber, D., and Bro, R. (2013). Fluorescence spectroscopy and multi-way techniques. PARAFAC. Anal. Methods 5:6557. doi: 10.1039/c3ay41160e
Murphy, K. R., Stedmon, C. A., Wenig, P., and Bro, R. (2014). OpenFluor– an online spectral library of auto-fluorescence by organic compounds in the environment. Anal. Methods 6, 658–661. doi: 10.1039/C3AY41935E
Nguyen, P. T. (2014). Study of the aquatic dissolved organic matter from the Seine River catchment (France) by optical spectroscopy combined to asymmetrical flow field-flow fractionation. Université de Bordeaux. Available at: https://theses.hal.science/tel-01170629 (Accessed March 21, 2023).
Nowicki, S., Lapworth, D. J., Ward, J. S. T., Thomson, P., and Charles, K. (2019). Tryptophan-like fluorescence as a measure of microbial contamination risk in groundwater. Sci. Total Environ. 646, 782–791. doi: 10.1016/j.scitotenv.2018.07.274
Ohno, T. (2002). Fluorescence inner-filtering correction for determining the Humification index of dissolved organic matter. Environ. Sci. Technol. 36, 742–746. doi: 10.1021/es0155276
Parker, C. A., and Barnes, W. J. (1957). Some experiments with spectrofluorimeters and filter fluorimeters. Analyst 82, 606–618. doi: 10.1039/AN9578200606
Parlanti, E., Wörz, K., Geoffroy, L., and Lamotte, M. (2000). Dissolved organic matter fluorescence spectroscopy as a tool to estimate biological activity in a coastal zone submitted to anthropogenic inputs. Org. Geochem. 31, 1765–1781. doi: 10.1016/S0146-6380(00)00124-8
Polubesova, T., Sherman-Nakache, M., and Chefetz, B. (2007). Binding of pyrene to hydrophobic fractions of dissolved organic matter: effect of polyvalent metal complexation. Environ. Sci. Technol. 41, 5389–5394. doi: 10.1021/es070722r
Portail Assainissement Collectif. (2021). Stations de traitement des eaux usées. Portail assainissement collectif. Available at: https://www.assainissement.developpement-durable.gouv.fr/PortailAC/data (Accessed March 14, 2023).
Ren, W., Wu, X., Ge, X., Lin, G., Zhou, M., Long, Z., et al. (2021). Characteristics of dissolved organic matter in lakes with different eutrophic levels in southeastern Hubei Province, China. J Ocean Limnol 39, 1256–1276. doi: 10.1007/s00343-020-0102-x
Romero González-Quijano, C., Herrero Ortega, S., Casper, P., Gessner, M., and Singer, G. (2022). Dissolved organic matter signatures in urban surface waters: Spatio-temporal patterns and drivers. Biogeochemistry 19:340. doi: 10.5194/bg-2021-340
Sciscenko, I., Arques, A., Micó, P., Mora, M., and García-Ballesteros, S. (2022). Emerging applications of EEM-PARAFAC for water treatment: a concise review. Chem. Eng. J. Adv. 10:100286. doi: 10.1016/j.ceja.2022.100286
Sorensen, J. P. R., Carr, A. F., Nayebare, J., Diongue, D. M. L., Pouye, A., Roffo, R., et al. (2020). Tryptophan-like and humic-like fluorophores are extracellular in groundwater: implications as real-time faecal indicators. Sci. Rep. 10:15379. doi: 10.1038/s41598-020-72258-2
Stedmon, C. A., and Bro, R. (2008). Characterizing dissolved organic matter fluorescence with parallel factor analysis: a tutorial. Limnol. Oceanogr. Methods 6, 572–579. doi: 10.4319/lom.2008.6.572
Tedetti, M., Longhitano, R., Garcia, N., Guigue, C., Ferretto, N., and Goutx, M. (2012). Fluorescence properties of dissolved organic matter in coastal Mediterranean waters influenced by a municipal sewage effluent (bay of Marseilles, France). Environ. Chem. 9:438. doi: 10.1071/EN12081
USEPA. (1977). Clean Water Act of 1977. Available at: https://www.regulations.gov/document/EPA-HQ-OW-2021-0602-0076 (Accessed November 27, 2023).
USEPA. (1986). Ambient water quality criteria for Bacteria - 1986. Washington, DC: Office of Water. Available at: https://nepis.epa.gov/ (Accessed November 13, 2023).
Wetzel, R. G. (1992). Gradient-dominated ecosystems: sources and regulatory functions of dissolved organic matter in freshwater ecosystems. Hydrobiologia 229, 181–198. doi: 10.1007/BF00007000
Williams, C. J., Yamashita, Y., Wilson, H. F., Jaffé, R., and Xenopoulos, M. A. (2010). Unraveling the role of land use and microbial activity in shaping dissolved organic matter characteristics in stream ecosystems. Limnol. Oceanogr. 55, 1159–1171. doi: 10.4319/lo.2010.55.3.1159
Wilson, H. F., and Xenopoulos, M. A. (2009). Effects of agricultural land use on the composition of fluvial dissolved organic matter. Nat. Geosci. 2, 37–41. doi: 10.1038/ngeo391
World Health Organization. (2021). Guidelines on recreational water quality: volume 1 coastal and fresh waters. In: Guidelines on recreational water quality: Volume 1 coastal and fresh waters water, sanitation, hygiene and health. World Health Organization, No. 164. Available at: https://www.who.int/publications-detail-redirect/9789240031302 (Accessed November 30, 2023).
Xenopoulos, M. A., Barnes, R. T., Boodoo, K. S., Butman, D., Catalán, N., D’Amario, S. C., et al. (2021). How humans alter dissolved organic matter composition in freshwater: relevance for the Earth’s biogeochemistry. Biogeochemistry 154, 323–348. doi: 10.1007/s10533-021-00753-3
Yuan, K., Wan, Q., Chai, B., Lei, X., Kang, A., Chen, J., et al. (2023). Characterizing the effects of stormwater runoff on dissolved organic matter in an urban river (Jiujiang, Jiangxi province, China) using spectral analysis. Environ. Sci. Pollut. Res. 30, 50649–50660. doi: 10.1007/s11356-023-25933-6
Keywords: fluorescent dissolved organic matter, parallel factor analysis, DOM signature, urban waters, urban swimming, fecal indicator bacteria
Citation: Angelotti de Ponte Rodrigues N, Carmigniani R, Guillot-Le Goff A, Lucas FS, Therial C, Naloufi M, Janne A, Piccioni F, Saad M, Dubois P and Vinçon-Leite B (2024) Fluorescence spectroscopy for tracking microbiological contamination in urban waterbodies. Front. Water. 6:1358483. doi: 10.3389/frwa.2024.1358483
Edited by:
Amin Mojiri, Arizona State University, United StatesReviewed by:
Qinghui Huang, Tongji University, ChinaIrene Esteban Cuesta, Ludwig Maximilian University of Munich, Germany
Copyright © 2024 Angelotti de Ponte Rodrigues, Carmigniani, Guillot-Le Goff, Lucas, Therial, Naloufi, Janne, Piccioni, Saad, Dubois and Vinçon-Leite. This is an open-access article distributed under the terms of the Creative Commons Attribution License (CC BY). The use, distribution or reproduction in other forums is permitted, provided the original author(s) and the copyright owner(s) are credited and that the original publication in this journal is cited, in accordance with accepted academic practice. No use, distribution or reproduction is permitted which does not comply with these terms.
*Correspondence: Natália Angelotti de Ponte Rodrigues, bmF0YWxpYS5hbmdlbG90dGktZGUtcG9udGUtcm9kcmlndWVzQGVucGMuZnI=; Brigitte Vinçon-Leite, Yi52aW5jb24tbGVpdGVAZW5wYy5mcg==
†PRESENT ADDRESS
Aurélie Janne, Agence Eau Seine Normandie, Courbevoie, France