- 1Natural Systems Design, Seattle, WA, United States
- 2Civil and Environmental Engineering, University of Washington, Seattle, WA, United States
- 3The Nature Conservancy of Washington, Seattle, WA, United States
- 4Seattle Public Utilities, Seattle, WA, United States
Forest thinning and gap creation are being implemented across the western United States of America (USA) to reduce wildfire and forest mortality risk as the climate warms. The Eastern Cascades in Washington, USA, is in a transitional zone between maritime and continental climate conditions and represents a data gap in observations describing the relationship between forest density and snowpack. We collected 3 years of snow observations across a range of forest densities to characterize how forest management efforts in this region may influence the magnitude and duration of snow storage. Observations indicate that peak snow storage magnitude in small gaps ranges from the same to over twice that observed in unburned forest plots in the Eastern Cascades. However, differences in snow duration are generally small. Across all Eastern Cascade sites and years, we observed a median difference of snow storage lasting 7 days longer in gaps as compared to nearby forest plots. A notable exception to this pattern occurred at one north-facing site, where snow lasted 30 days longer in the gap. These observations of similar snow storage duration in the Eastern Cascades are attributed to minimal differences in canopy snow interception processes between forests and gaps at some sites, and to higher ablation rates that counterbalance the higher snow accumulation in the gaps at other sites. At the north-facing site, more snow accumulated in the gap, and ablation rates in the open gap were similar to the shaded forest due to the aspect of the site. Thus, snow storage duration was much longer in the gap. Together, these data suggest that prescriptions to reduce forest density through thinning and creating gaps may increase the overall amount of snow storage by reducing loss due to sublimation and melting of canopy-intercepted snow. However, reducing forest density in the Eastern Cascades is unlikely to buffer climate-induced shortening of snow storage duration, with the possible exception of gap creation in north-facing forests. Lastly, these observations fill a spatial and climatic data gap and can be used to support hydrological modeling at spatial and temporal scales that are relevant to forest management decisions.
Introduction
Across the western USA forest management actions to reduce forest density, such as gap creation, thinning, and prescribed burning, are becoming widely implemented to address forest vulnerability to wildfire, drought, and insect damage (Kane et al., 2011; Churchill et al., 2013; Prichard et al., 2021; Knight et al., 2022). These stressors are amplified in overstocked, even-aged forests, which reflect a legacy of Indigenous depopulation, timber harvest, timber regeneration, and fire suppression (Hessburg et al., 2000; Churchill et al., 2013; Haugo et al., 2015). These stressors are also amplified by climate change, particularly declines in the amount and duration of mountain snow storage (Mote et al., 2005, 2018; Westerling, 2006; Halofsky et al., 2020). Thus, forest management actions to reduce forest density are also being planned and implemented as a buffer against climate impacts. Reducing forest density typically increases the overall magnitude of snow storage due to reduced interception by the forest canopy (Church, 1912; Golding and Swanson, 1986; Varhola et al., 2010; Lundquist et al., 2013), except in locations subject to high wind speeds (Hiemstra et al., 2002; Dickerson-Lange et al., 2017). However, in some regions it is unknown whether forest management actions will have the unintended consequence of decreasing the duration of snow stored on the landscape. Reducing forest density can have opposite effects on the duration of snow storage by either delaying or accelerating snow disappearance timing in open areas relative to continuous forest (Varhola et al., 2010; Lundquist et al., 2013). As mountain snow storage diminishes due to climate warming, understanding where reducing forest density causes an overall shortening versus lengthening of snow storage duration will determine where these management efforts amplify vs. buffer climate impacts on water availability.
Previous studies in the western USA (open symbols in Figure 1) and across the globe have demonstrated that the net effect of forest density on snow storage duration changes direction between maritime and continental climate conditions (Lundquist et al., 2013). Within warmer and wetter maritime climates, such as western Washington, Oregon, and California (USA), peak snow storage magnitude is up to 3x greater and snow storage duration is 2-13 weeks longer in sheltered open areas, such as small forest gaps, as compared to higher density forests with continuous forest canopy (Storck et al., 2002; Dickerson-Lange et al., 2015a, 2017; Roth and Nolin, 2019). In comparison, within colder and drier continental climates, such as the Rocky Mountains, USA, peak snow storage magnitude only ranges from 0.9 to 1.9x greater in open areas compared to forested areas (LaMalfa and Ryle, 2008; Harpold et al., 2015). However, even where snow storage magnitude is greater in open areas, snow storage duration can switch to snow lasting up to 20 days longer in the forest (Rutter et al., 2009; Lundquist et al., 2013). This shift in the directionality is the result of combining a complex suite of snow accumulation and ablation processes, each of which are modified by forests to different extents depending on climate, wind exposure, elevation, latitude, slope, aspect, forest characteristics, and fire history (e.g., Marks et al., 1998; Strasser et al., 2011; Gleason et al., 2013; Lundquist et al., 2013; Seyednasrollah et al., 2013; Broxton et al., 2015, 2020). These forest modifications of key individual processes are reviewed further below (See also recent reviews: Goeking and Tarboton, 2020; Dickerson-Lange et al., 2021; Lundquist et al., 2021). Although the forest density characteristics of the comparisons made in previous studies are highly variable, a meta-analysis of previous work suggested that mean winter temperature > −1°C (vertical dashed line in Figure 1) is a first-order predictor of where snow storge duration is lengthened by decreasing forest density or gap creation (Lundquist et al., 2013). However, this threshold is based on a synthesis of studies in which most observations were collected where winter temperatures are substantially warmer or colder than −1°C (Figure 1).
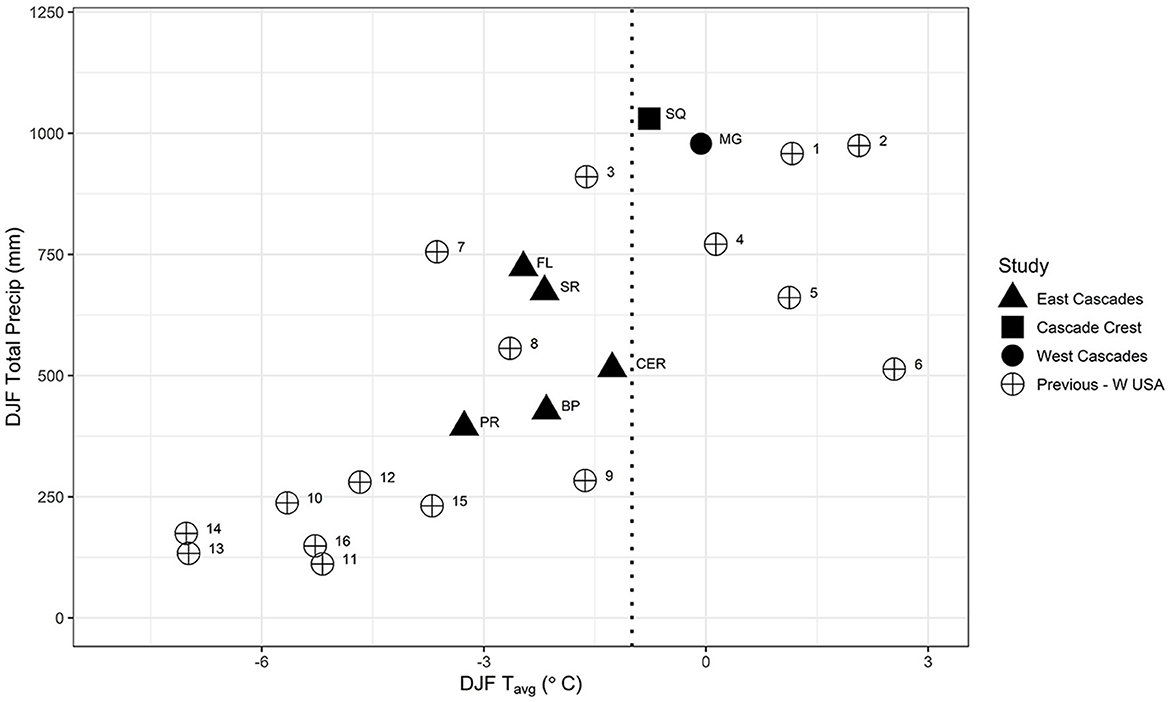
Figure 1. Mean total winter [inclusive of December, January, and February (DJF)] precipitation versus mean DJF temperature, extracted from 800 m gridded 30-year normals [1981–2010; (PRISM Climate Group, 2015)] for the sites included in this study (filled symbols) and previous studies in the western USA [open circles using same reference numbering as in Figure 3 of Dickerson-Lange et al. (2021)]. The dotted vertical line indicates the −1°C threshold proposed by Lundquist et al. (2013) as a climate-based indicator of where snow storage duration switches from longer in open areas (>-1°C) to snow lasting longer in forests (< -1°C). Note the sparse coverage of previous observations in the western USA at sites located where mean DJF temperature is between −3 and 0°C and where DJF total precipitation is >300 mm.
Field observations of forest effects on snow storage are sparse within transitional climate zones that are drier and colder than maritime conditions, yet warmer than continental conditions. This data gap is particularly problematic for forest management initiatives in the Eastern Cascades in Washington (WA), where there are no forest-snow observations and where state and federal management initiatives aim to reduce forest density across 1.25 million acres over the next 20 years to restore forest health and increase wildfire resilience (Washington State Department of Natural Resources, 2018). The region is critically dependent on snowpack storage for up to 75% of its water supply (Donley et al., 2012; Li et al., 2017), which supports Tribal Treaty Rights resources, such as threatened and endangered anadromous fish species, a multi-billion dollar agricultural industry, and downstream communities. However, little attention has focused on the impacts of forest treatments on snowpack retention and subsequent effects on hydrologic resilience.
Previous forest-snow studies in this transitional climate zone include 1–3 years of observations comparing snow at: open canopy positions to closed-canopy positions in the southern Sierra Nevada range, California (Bales et al., 2011; Harpold et al., 2015; point 7 on Figure 1), 10–20 ha clearcuts to continuous forest in northern Idaho (Hubbart et al., 2015; Dickerson-Lange et al., 2017; point 8 on Figure 1); and a 40-m gap to the surrounding forest in central Idaho (Carson, 2010; Dickerson-Lange et al., 2017; point 9 on Figure 1). These studies reported snow storage duration differences that ranged from 1 day longer in the forest to 18 days longer in the open, with median values of 5, 0, and 6 days longer in the open, respectively. These limited observations are insufficient to characterize whether snow storage magnitude and duration differences across the Eastern Cascades (filled triangles in Figure 1) are likely to be similar to maritime or continental climates, or whether forest-snow interactions in this climate zone fall somewhere in between.
A hierarchy of forest-snow processes (Figure 2) proposed by Dickerson-Lange et al. (2021) provides a conceptual model to classify and map zones based on hypothesized forest effects on snow storage (Figure 3A). However, field observations comparing snow storage in small open gaps (defined here as gap diameters of approximately 1–3x the surrounding tree height) vs. dense forests and across a range of forest densities are needed to test these hypotheses.
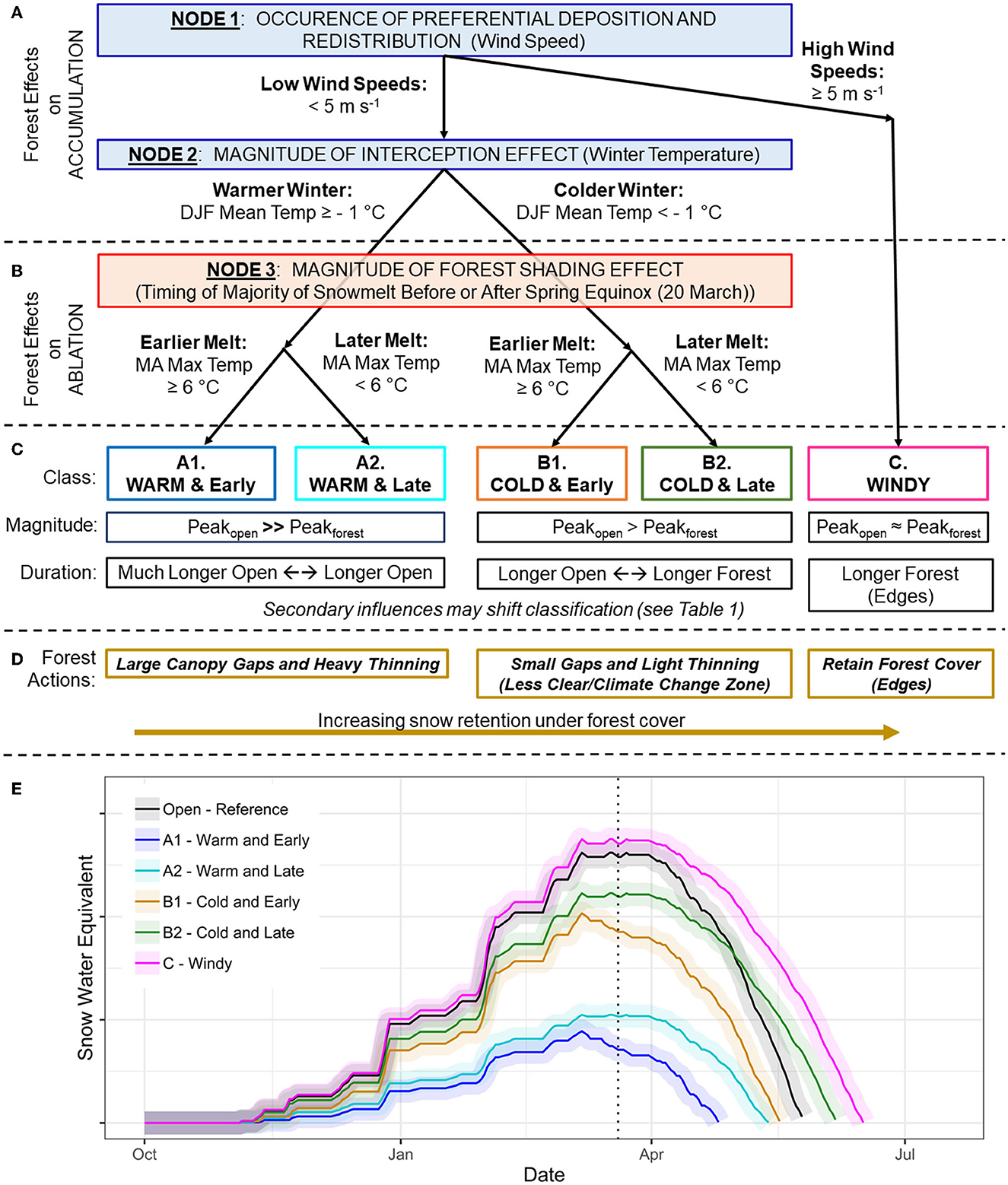
Figure 2. Reproduced from Dickerson-Lange et al. (2021). Hypothesized hierarchy of processes presented as a decision tree framework. (A) Nodes 1 (amount of preferential deposition and redistribution) and 2 (amount of interception) reflect the relative effect of forest cover on snow accumulation rates. (B) Node 3 (amount of shading) reflects the relative effect of forest cover on snow ablation rates. (C) Five forest–snow classes relate to the difference in snow storage magnitude and duration between forest and open, which (D) relate to potential forest actions to maximize the snow storage on the landscape. (E) Conceptual relation between a reference open location (black) and adjacent forest in the five forest–snow classes [colors and letters are defined in (C)]. Note that the open reference snow depth time series (black line) is drawn without a sharp peak, to facilitate comparison of all forest sites to the same open reference site; however, the timing of peak snow is likely to be similar at the adjacent forest and open sites. The shaded area around each line is a conceptual representation of the spectrum of effects within a single class, determined by secondary influences.
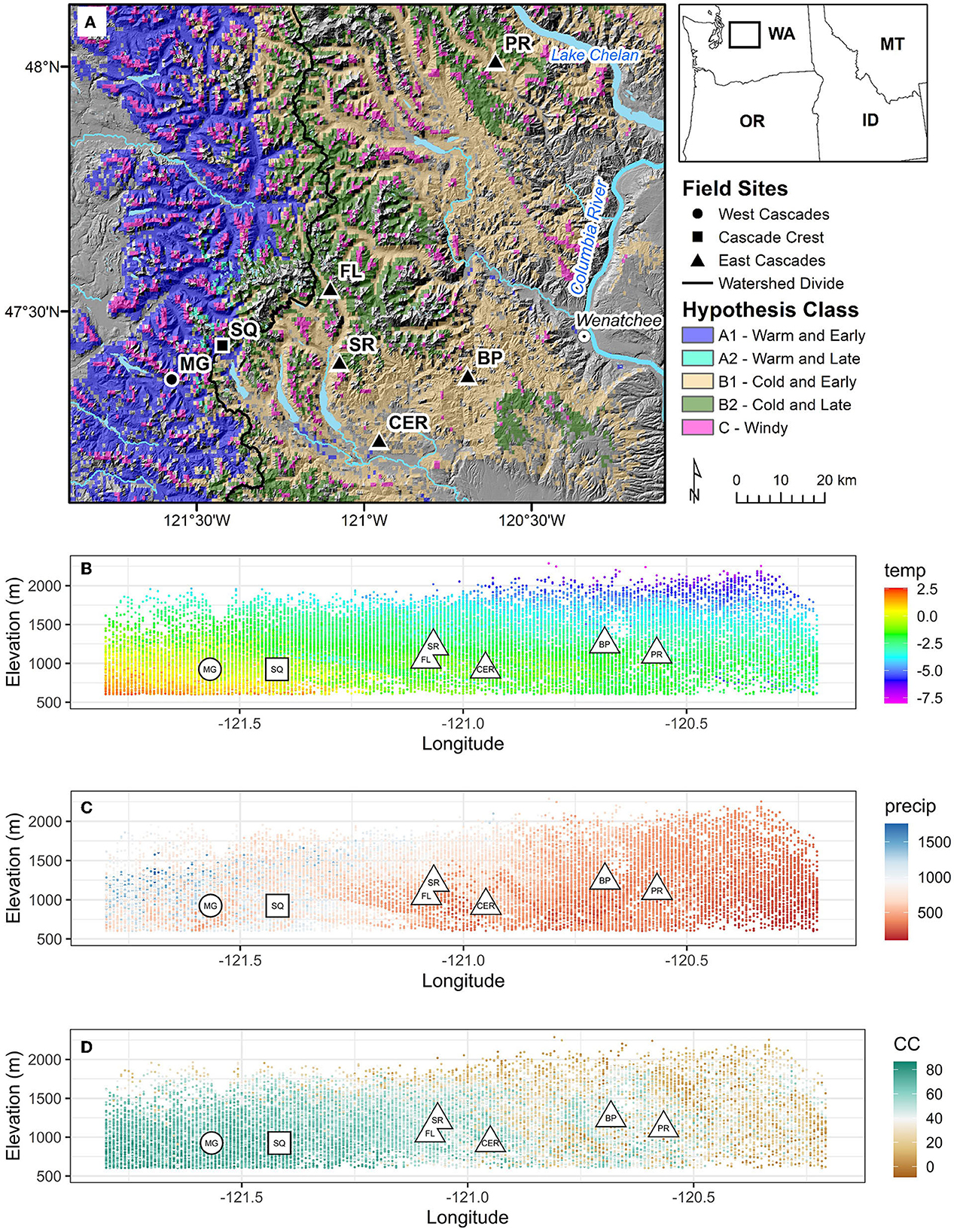
Figure 3. (A) Map of field sites across hypothesized forest-snow classes proposed in Dickerson-Lange et al. (2021); inset shows domain of map (dark box). (B) Longitude and elevation of field sites (open symbols with field site abbreviation in center) plotted in front of mean DJF temperature (°C) across the region, plotted by longitude and elevation. DJF temperature values are extracted from a 0.5-degree latitude swath of panel (A), and aggregated from 800 m gridded 30-year normals [1981–2010; (PRISM Climate Group, 2015)]. (C) Same as in panel (B), but for mean total DJF precipitation (mm). (D) Same as in panel (B), but for canopy cover (CC; %) extracted and aggregated from the 30 m National Land Cover Database (Dewitz, 2019).
The model proposes that forest influences on snow accumulation processes are more important than forest influences on snow ablation processes for determining the net effect of forest density on snow storage magnitude and duration (Dickerson-Lange et al., 2021). With respect to snow accumulation processes, forests reduce wind speed and control snow deposition patterns (Essery and Pomeroy, 2004; Hiemstra et al., 2006; Trujillo et al., 2009). Forest canopies also reduce the accumulation of under-canopy snowpack through canopy snow interception (Hedstrom and Pomeroy, 1998; Storck et al., 2002; Martin et al., 2013). A portion of the snow captured and stored by a forest canopy subsequently sublimates or melts and evaporates without reaching the forest floor (Ellis et al., 2010; Roth and Nolin, 2017; Lumbrazo et al., 2022). The remaining portion of canopy-intercepted snow either melts and drips to the under-canopy snowpack, or falls to the ground due to sliding or wind and joins the under-canopy snowpack in solid form (Roesch et al., 2001; Lumbrazo et al., 2022). Forest modifications of snow accumulation rates control the difference in peak snow storage magnitude between forests and open areas (Nodes 1 and 2 in Figure 2). Differences in the subsequent duration of snow storage are strongly influenced by these initial differences in peak snow storage magnitude, along with forest modifications of under-canopy ablation rates.
With respect to snow ablation processes, the degree to which snow ablation rates are lower under a forest canopy compared to open gaps controls whether snow storage duration becomes longer within a forest even after accumulating less snow than an open area (Node 3 in Figure 2). Forest canopies can slow ablation rates by shading snow from solar radiation (Link et al., 2004; Musselman et al., 2008, 2015; Ellis et al., 2011; Lawler and Link, 2011) and sheltering snow from wind (Marks et al., 1998; Wayand et al., 2015). In some cases, the under-canopy ablation rate is sufficiently reduced relative to open areas to compensate for much-higher accumulation rates in the open, thus setting up longer snow storage duration in the forest. However, snow storage duration is longer in the open in the following cases: (1) sites where the magnitude of increased snow storage in the open is large enough to not be balanced by increased ablation rates (Dickerson-Lange et al., 2017); (2) sites where the under-canopy ablation rate is only moderately reduced relative to the open because of topographic or forest characteristics, such as north-facing aspects or low density forests (Lundquist and Flint, 2006; Ellis and Pomeroy, 2007); (3) sites where the under-canopy ablation rate is only moderately reduced relative to the open because solar elevation is low at the time snowmelt occurs, such as at higher latitudes or lower elevations (Essery et al., 2008; Strasser et al., 2011; Musselman et al., 2015; Seyednasrollah and Kumar, 2019); and (4) sites where the under-canopy ablation rate is enhanced relative to the open due to increased under-canopy longwave radiation, which can occur with increasing air temperatures or forest density (Lundquist et al., 2013; Musselman and Pomeroy, 2017).
In this study, we applied the Dickerson-Lange et al. (2021) model framework to sites where wind exposure is low (Node 1 of Figure 2) and mean winter temperatures span a gradient from warmer than −1 °C in the Western Cascades, to colder than −1 °C in the Eastern Cascades (Node 2 of Figure 2). We used the framework to develop hypotheses to test with field observations comparing snow storage magnitude and duration under the forest to that in small forest gaps. We hypothesized that:
1) The magnitude of snow storage would be higher in forest gaps and thinned plots due to increased canopy snow interception in denser forests;
2) Differences in peak snow storage magnitude in the colder Eastern Cascades would be less than in the warmer climate of the Western Cascades due to lower snow cohesion and therefore lower canopy interception rates (Shidei et al., 1952; Satterlund and Haupt, 1967; Kobayashi, 1987; Pfister and Schneebeli, 1999; Martin et al., 2013);
3) Ablation rates in the Eastern Cascades sites would be only moderately higher in forest gaps relative to denser forest due to relatively early timing of the onset of ablation (Node 3 in Figure 2); and
4) Topographic position (aspect) would contribute to variability in snow storage differences between forest and gap plots but remain secondary to climate variables for determining relative snow storage.
With respect to hypothesis 3, in locations where ablation occurs earlier in the year due to warmer spring temperatures, the magnitude of incoming solar radiation is much less, and therefore forest shading matters less (Strasser et al., 2011; Seyednasrollah and Kumar, 2014). Thus, increased snow accumulation balances increased snow ablation, resulting in similar snow storage duration between forest gaps and dense forest. In the proposed classification, this balance occurs within the “Cold and Early” class (Figure 2). Lastly, we aimed to further characterize how forest species composition may contribute to variability in snow storage differences.
Testing the hypothesized effects of forest density on snow storage and characterizing the potential hydrologic effects of forest management in the Eastern Cascades is of interest to municipal, agricultural, tribal, and fisheries stakeholders (e.g., Wigmosta et al., 2015; Povak et al., 2022). The magnitude and duration of snow storage affects upland hydrologic and ecological processes, like soil water availability (Harpold et al., 2015) and plant phenology (Ford et al., 2013), along with contributing to integrated effects such as the magnitude and timing of streamflow (Lundquist et al., 2005; Stewart et al., 2005; Clark et al., 2011) and stream temperature (Leach and Moore, 2014; Cline et al., 2020; Lee et al., 2020). Hydrologic modeling has been implemented to characterize forest thinning effects on snow storage and streamflow in this region (Wigmosta et al., 2015; Sun et al., 2018; Currier et al., 2022) and throughout the western USA (Saksa et al., 2017, 2020; Harpold et al., 2020; Krogh et al., 2020; Sun et al., 2022). The Eastern Cascades modeling studies suggest that adding canopy gaps (Sun et al., 2018), creating east-west oriented open strips to maximize north-facing edges (Currier et al., 2022), or thinning (Wigmosta et al., 2015) could increase late-season flow by as much as 20 to 43%. However, these modeling studies currently lack field observations to validate simulated under-canopy snowpack, which is particularly relevant given that model representations of forest-snow processes are subject to considerable uncertainty (Essery et al., 2009; Rutter et al., 2009) and may lack transferability across climate zones (Clark et al., 2015; Lundquist et al., 2021).
This study therefore aims to test the effects of forest density on snow storage magnitude and duration through field observations across the Eastern Cascades in Washington. In this study we (1) present three years of field-based snow observations to quantify the magnitude and duration of snow storage within a range of forest densities, and across a gradient of climate and forest types where under-canopy snow observations are sparse (i.e., filled triangles in Figure 1); and (2) synthesize the results to provide guidance for regional forest management initiatives that have multiple objectives related to wildfire risk, forest health, and water resources.
Methods
Site locations and context
To characterize the magnitude and duration of snow storage across a range of climates, forest types, and forest canopy densities, we collected field observations at eight sites arranged in an approximate west-to-east transect from the western slopes of the Cascade Range to almost as far east as the Columbia River in WA (Figure 3A; Table 1). The eight sites were selected to sample a gradient of climate conditions across the North Cascades EPA Level III ecoregion. The sites extend from the warmer and wetter western slopes of the Cascade Range across the eastern slopes of the Cascade Range, where the climate begins to transition to the colder continental conditions of the Rocky Mountains to the east (Figures 3B, C).
The transect of sites also samples the diverse assemblage of forest species compositions and densities that follows the gradient of climate conditions across the Cascade Range (Franklin and Dyrness, 1973; Figure 3D). With spatially continuous warm temperatures and abundant precipitation, Western Cascades forests grow at high density, and species composition varies primarily with elevation. These forests are dominated by relatively few conifer species, including western hemlock (Tsuga heterophylla), Douglas-fir (Pseudotsuga menziesii), and Pacific silver fir (Abies amabilis), and forest structure is strongly influenced by previous or active timber harvest. In the Eastern Cascades, less precipitation and clearer sky conditions result in site conditions that vary more with elevation, topographic position, and longitude, and results in greater species diversity and variable forest productivity (Agee, 2003; Table 1). Eastern Cascade forests range from cold, wet forests dominated by subalpine fir (Abies lasiocarpa) and Engelmann spruce (Picea engelmannii) at higher elevations, to Grand fir (Abies grandis) and Douglas-fir forests at mid-elevations, and Ponderosa pine (Pinus ponderosa) forests at lower elevations, south-facing slopes, and eastern longitudes. Forest structure in the Eastern Cascades is also influenced by the natural, mixted-severity fire regime (Perry et al., 2011), along with a legacy of fire suppression and the resulting shift to lower frequency and higher severity fires (Everett et al., 2000).
The eight study sites include one site located on the western slopes of the Cascades [Mount Gardner (MG)] and one at the Cascade Crest [Snoqualmie Pass (SQ)]. Observations at these sites were intended primarily for comparison to previous field studies at these sites, particularly MG, where snow storage differences between a forest gap and the adjacent higher density forest (hereafter, relative snow storage) is well-characterized (Dickerson-Lange et al., 2015b, 2017). Six additional sites are the focus of this investigation and are located across the Eastern Cascades: Fish Lake (FL), Sasse Ridge (SR), Cle Elum Ridge North (CER-N), Cle Elum Ridge South (CER-S), Blewett Pass (BP), and Pope Ridge (PR). The two sites at Cle Elum Ridge are located <500 m from each other on north- and south-facing slopes at similar elevations and were selected to observe relative snow storage differences based on aspect. Topographic position and heat load index (McCune and Keon, 2002) were characterized from 10 m digital elevation models (DEMs) from the United States Geological Survey (USGS) National Elevation Dataset (NED; http://nationalmap.gov/elevation.html) to aid in site selection. Although some sites were located on a hillslope, plots were generally flat to gently sloping, except for the FL forest plot, which was moderately sloped but also topographically shaded near the toe of the valley wall, and the CER-N and CER-S plots which were intended to characterize aspect differences (Table 1). Six out of eight sites are co-located with either a National Resource Conservation Service (NRCS) SNOw TELemetry (SNOTEL) or a Northwest Avalanche Center (NWAC) meteorological and snow observation station. Snow depth observations from acoustic sensors were used from the SNOTEL and NWAC stations, along with snow water equivalent (SWE) from snow pillow observations at SNOTEL sites.
Within each of the eight sites, we designated 2–3 plots, each approximately 15 m × 15 m (0.03 ha), based on types of forest canopy densities at each site (Table 1). The plots were selected to represent the range of forest densities present at each site, including from highest to lowest canopy density: un-thinned forest, thinned forest, burned forest, and forest gaps (Figure 4). Gap plots encompassed circular to elliptical open areas with a ratio of gap diameter to surrounding tree height ranging from 0.5 to 2.8. Snow observations within gap plots were located inside the boundaries of a gap. Each plot was initially designated based on qualitative field observations of canopy density; forest species composition, stem density, and canopy density were then quantified from field- and lidar-based metrics. Plots were selected to maximize proximity to one another within the site, and to maximize similarity in elevation, slope, and aspect across the site.
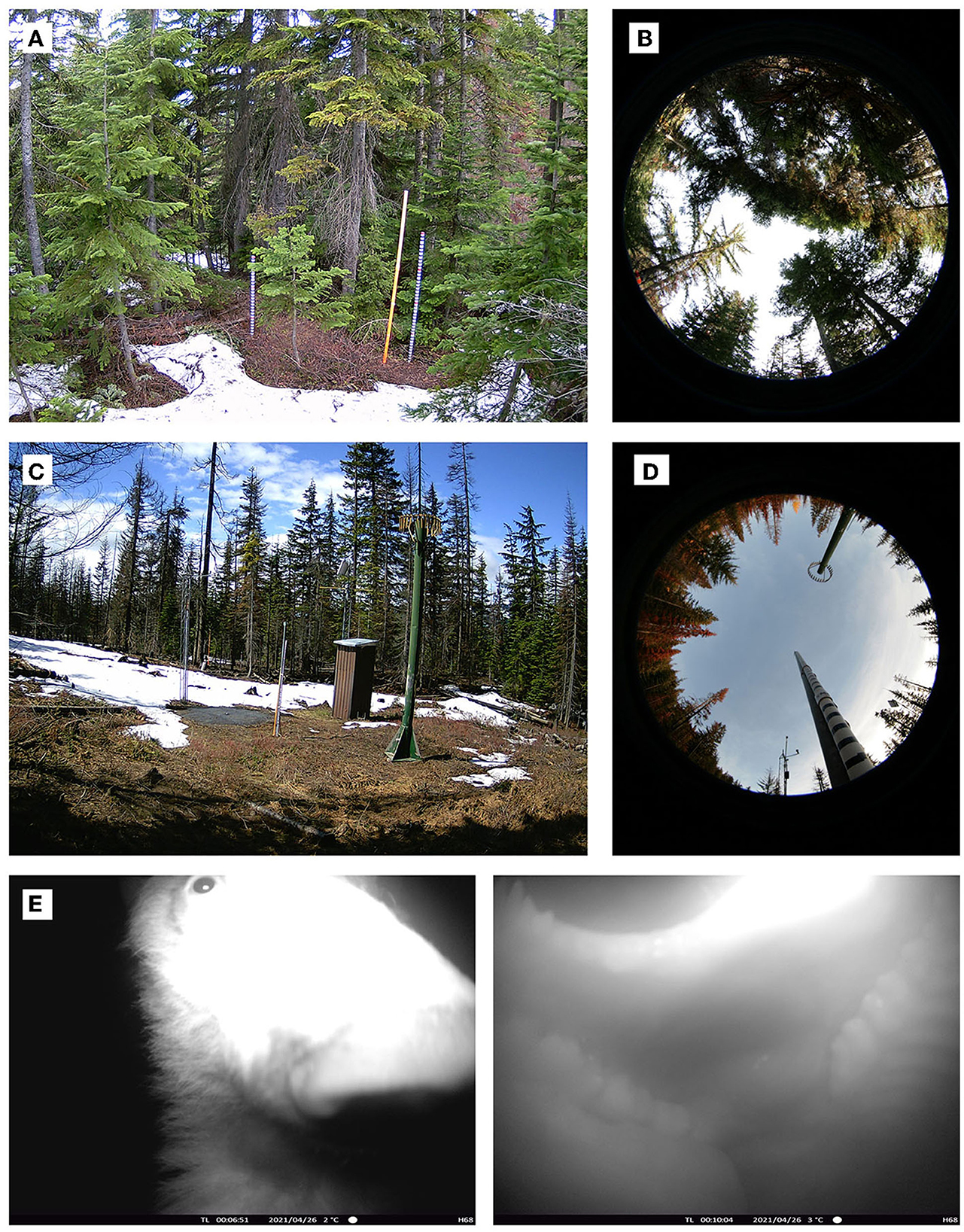
Figure 4. Examples of plots and methods, including (A) time lapse photograph at the SR forest plot (SR-F) taken on 19 May 2020 facing north, (B) hemispherical photo at SR-F with north oriented to top of photograph, (C) time lapse photograph at the SR gap plot (SR-G) taken on 19 May 2020 facing northwest, and (D) hemispherical photo at SR-G with north oriented to top of photograph. Some snow depth time series include periods of missing data due to camera malfunction, snow over-topping the poles or cameras, or (E) damage from bears.
Field-based snow observations
The overall design of the study was to observe and compare snow storage magnitude and duration across a transect of eight sites and between 2 and 3 plots located at each site. We quantified snow storage magnitude via observations of peak snow depth, and quantified snow storage duration via observations of snow disappearance timing. Comparisons across the transect of sites were used to characterize variability associated with climate, topographic position, and longitude. Comparisons between plots at the same site were used to isolate the local effect of forest canopy density on snow storage. The decision to use snow depth rather than SWE to quantify snow storage magnitude was based on limited winter access to the sites, previous work that indicates that snow density is less spatially variable than snow depth (Elder et al., 1998; Sturm et al., 2010; López-Moreno et al., 2013), and sensitivity testing that indicates snow depth is robust for relative comparisons of snow storage across forest densities (see supporting information in Dickerson-Lange et al., 2017).
At each plot we deployed remote instrumentation to observe a time series of snow depth, snow presence, and air temperature. With eight sites that span a west-to-east distance of 80 km, we used low-cost instrumentation to characterize overall patterns of relative snow storage at a plot scale. Snow depth was measured using time lapse cameras recording sub-daily images of PVC poles, which were marked at 5 cm increments (Figure 4). Three or more poles were deployed at each plot within the field of view of the cameras, except for gap plots, which were co-located with SNOTEL or NWAC stations.
Placement of the poles was determined by qualitative assessment of the canopy distribution and an aim to represent the conditions present. At most gap plots, a single pole was deployed to supplement the acoustic snow depth sensor, and snow depth values reflect the center of the gap rather than at the edges. At the CER gap plots, the poles were deployed in an approximate east-west transect of the middle of the gap. Previous work indicates that observations at the center of the gap are likely to reflect maximum snow storage magnitude (Mazzotti et al., 2019). We assume that observations from the center represent the median snow storage duration, between the range of earlier snow disappearance timing near the north edge and later timing near the south edge, but previous high-resolution observations and modeling also highlight substantial spatial variability associated with forest edges, aspect, and wind conditions (Currier and Lundquist, 2018). For a given forest plot, typically one pole was deployed directly under a canopy opening, one pole was deployed under canopy but away from a tree trunk, and one pole was deployed adjacent to a tree trunk. For each pole, a daily time series of snow depth, determined to the nearest 5 cm, was extracted via visual processing (Figure 5). Snow depth values from the poles were aggregated to a daily time series of plot-scale median snow depth, from which the maximum value was used to identify the timing and magnitude of peak snow depth (PSD) for the plot. Snow disappearance day (SDD) was determined as the day when the plot-scale median snow depth reached zero.
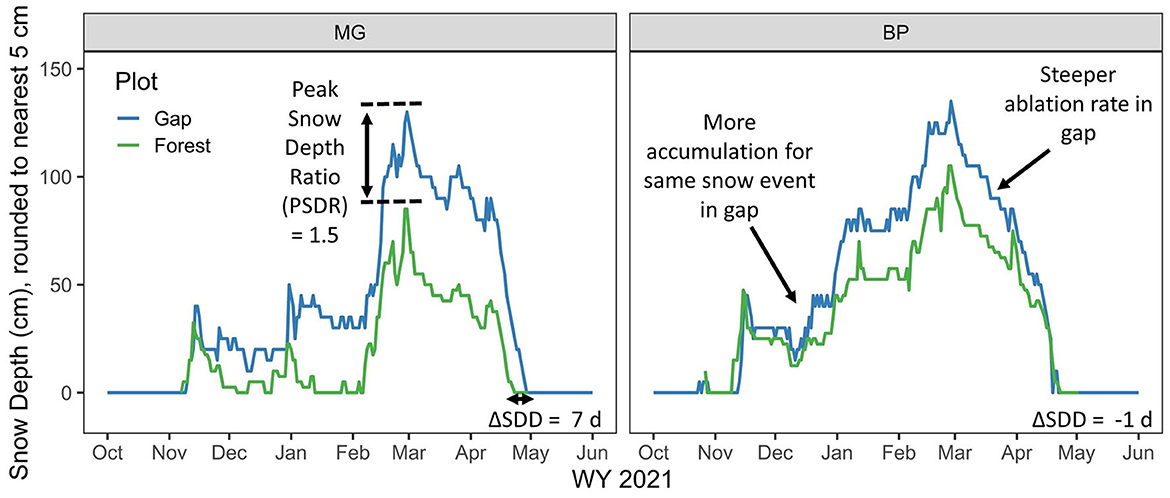
Figure 5. Example comparison of snow depth observed in a gap plot (blue line) and a forest plot (green line) at two sites: Mount Gardner (MG) in the Western Cascades and Blewett Pass (BP) in the Eastern Cascades. Metrics that were used to quantify relative snow storage differences between plots include Peak Snow Depth Ratio (PSDR) to compare snow storage magnitude and ΔSDD to compare snow storage duration. Example differences in accumulation and ablation rates between the gap and forest plots are also annotated.
Since the measurement poles sample snow depth at only a few points within a given plot, we additionally deployed ground temperature sensors in an evenly spaced grid at each plot to quantify snow duration by inferring snow presence or absence based on ground temperature (Lundquist and Lott, 2008). Measurement poles were also used for determining snow duration at each point and additional extraction of distributed SDD is possible via image analysis (e.g., Raleigh et al., 2013). However, we implemented the ground temperature approach to provide redundancy for inevitable field instrumentation challenges and to cover a larger spatial footprint than is visible in the field of view of a camera, particularly in dense forest. We used waterproof temperature sensors with onboard dataloggers (Onset Hobo 64K Pendant Temperature Data Logger, # UA-001-64) buried 1–2 cm below the ground surface. Three rows of 6–9 sensors were deployed and marked with stakes in each plot, with 5 m spacing based on previous findings of maximum spacing to observe plot-scale snow duration in diverse forest types (Dickerson-Lange et al., 2015b). We inferred snow presence at a daily time scale based on 3 criteria: diurnal temperature range below 1.5°C, maximum daily temperature below 1.5°C, and a minimum of 48 continuous hours where both temperature criteria are met (Lundquist and Lott, 2008; Raleigh et al., 2013). Snow disappearance day (SDD) was identified from each sensor location as the last day with snow in a given season, excluding any isolated late season snow accumulation events that occur after the perennial snow has disappeared from the site. Plot-scale SDD were computed as the median values across all the sensors at the plot.
For gap plots that were co-located at SNOTEL or NWAC sites (e.g., Figure 4C), poles and timelapse cameras were deployed and processed only for verification of snow depth methods, and ground temperature sensors were not deployed. Snow depth derived from the time lapse cameras was compared to snow depth from the acoustic snow depth sensors to verify that the camera method produced similar results. SDD in those gap plots was determined from snow depth from acoustic instruments and cross-checked against the time series of snow water equivalent from snow pillows.
Using snow depth and snow disappearance timing, we compared absolute snow storage magnitude and duration across sites and between plots. Across sites, focusing on the gap plot at each site only, we compared the peak snow depth (PSD) and SDD to quantify differences in absolute snow storage magnitude and duration associated with climate, topographic position, and longitude.
We compared relative snow storage magnitude and duration between plots at the same site to isolate differences associated with variable forest canopy density. We quantified relative differences in snow storage magnitude via the peak snow depth ratio (PSDR), given as:
where peak snow depth (PSD) in the gap plot is divided by PSD in each of the forest plots, including un-thinned, thinned, and burned forest plots (Figure 5). Gap plots represent the lowest forest density of any of the plots and are the equivalent of the “open” plots presented in Lundquist et al. (2013) and Dickerson-Lange et al. (2017). We quantified relative differences in snow storage duration by comparing the difference SDD (ΔSDD), given as:
where the SDD in each of the forest plots, including un-thinned, thinned, and burned forest plots, is subtracted from SDD in the gap plot (Figure 5).
At most plots, we deployed one air temperature sensor (same HOBO model) hanging from a conifer tree at a height of approximately 2 m using an inverted funnel with holes as a radiation shield (Lundquist and Huggett, 2010). These observations were used to assess site-scale temperature variability and to check for plot-scale differences in air temperature due to cold air pooling that may contribute to the snow storage differences that would otherwise be attributed to forest type.
Forest characterization
At each plot we characterized forest structure and composition of tree species. We recorded tree species, stem diameter at 1.4 m above ground, and tree height for all trees taller than 1.4 m that were located within the sampling domain at each plot. From these observations we calculated forest metrics that include density [trees per hectare and basal area (m2ha−1)], mean stem diameter, and mean tree height. Tree species were ranked by their contribution to total plot basal area.
Hemispherical photography and digital image analysis were used to quantify canopy gap fraction at the location of each individual observation (Figures 4B, D). Photographs were taken facing vertically upward at each of the 6–9 ground temperature sensor locations within each plot and at each snow pole location. Photographs were taken during the first installation of plot instrumentation, which occurred during the autumn season, using a Nikon 60D DSLR camera mounted with a Sigma 4.5 mm f2.8 hemispherical lens attachment. The camera was leveled using a tripod and bubble levels with the top of the photo facing north (azimuth of 0°). Five photos were taken across a range of camera exposure settings (−2, −1, 0, 1, 2) to reduce effects of sun angle and varying sky conditions, and because automatic exposure has been demonstrated to prevent accurate estimates of gap fraction (Chen et al., 1991). Due to the remoteness of the field sites and limited field time, photographs were taken opportunistically under sky conditions that ranged from sunny to overcast rather than the ideal scenarios of overcast sky conditions or during dawn or dusk. Hemispherical images were analyzed to quantify gap fraction using ImageJ v. 1.48 software (http://imagej.nih.gov/ij/), along with the Hemispherical_2.0 macro plugin (Beckschafer, 2015). The plugin registers and crops the images to remove the black frame surrounding the image margin, splits out the blue color frame to obtain the largest contrast between vegetation and sky (Brusa et al., 2014), binarizes the image and applies a thresholding algorithm to classify vegetation and sky (Glatthorn and Beckschäfer, 2014), and reports the total gap fraction (%).
Plot-scale forest metrics were also derived from 1 m lidar-derived digital surface models (DSM) and DEMs, where available via the WA Department of Natural Resources (https://lidarportal.dnr.wa.gov/). Tree height was calculated as the difference between the DSM, which represents the highest lidar return value and therefore the highest vegetation in each pixel, and the DEM, which represents the lowest lidar return value or the bare earth topographic surface. Canopy cover was estimated as the proportion of 1 m pixels with vegetation height >2 m to the total number of pixels in each plot.
Results
Absolute snow storage differences between sites
Absolute snow storage magnitude and duration varied between sites and years and is characterized here by comparing observations at the gap plots across all sites. The greatest snow storage magnitude and longest duration snow storage was observed at SQ, at the Cascade Crest, and FL and SR, in the Eastern Cascades in WY 2019–2021 (Figure 6, Table 2). Peak snow depths in the gap plots at these sites ranged from 190 to 360 cm, and SDD occurred in May or early June (Table 2). Moderate snow storage magnitude and duration was observed at MG, in the Western Cascades, and CER-N, BP, and PR, in the Eastern Cascades. Peak snow depths were similar at these sites, with values that ranged from 90 to 145 cm in the gap plots. In all years, snow disappearance at MG, CER-N, BP, and PR occurred in mid- to late-April, which was approximately 3 to 4 weeks earlier than at SQ, FL, and SR (Figure 6). One site, CER-S, was an outlier with the lowest snow storage magnitude and duration. At this south-facing site located in the Eastern Cascades near CER-N, peak snow depth that ranged from 55 to 60 cm and snow disappearance occurred in late February and early March (Figure 7).
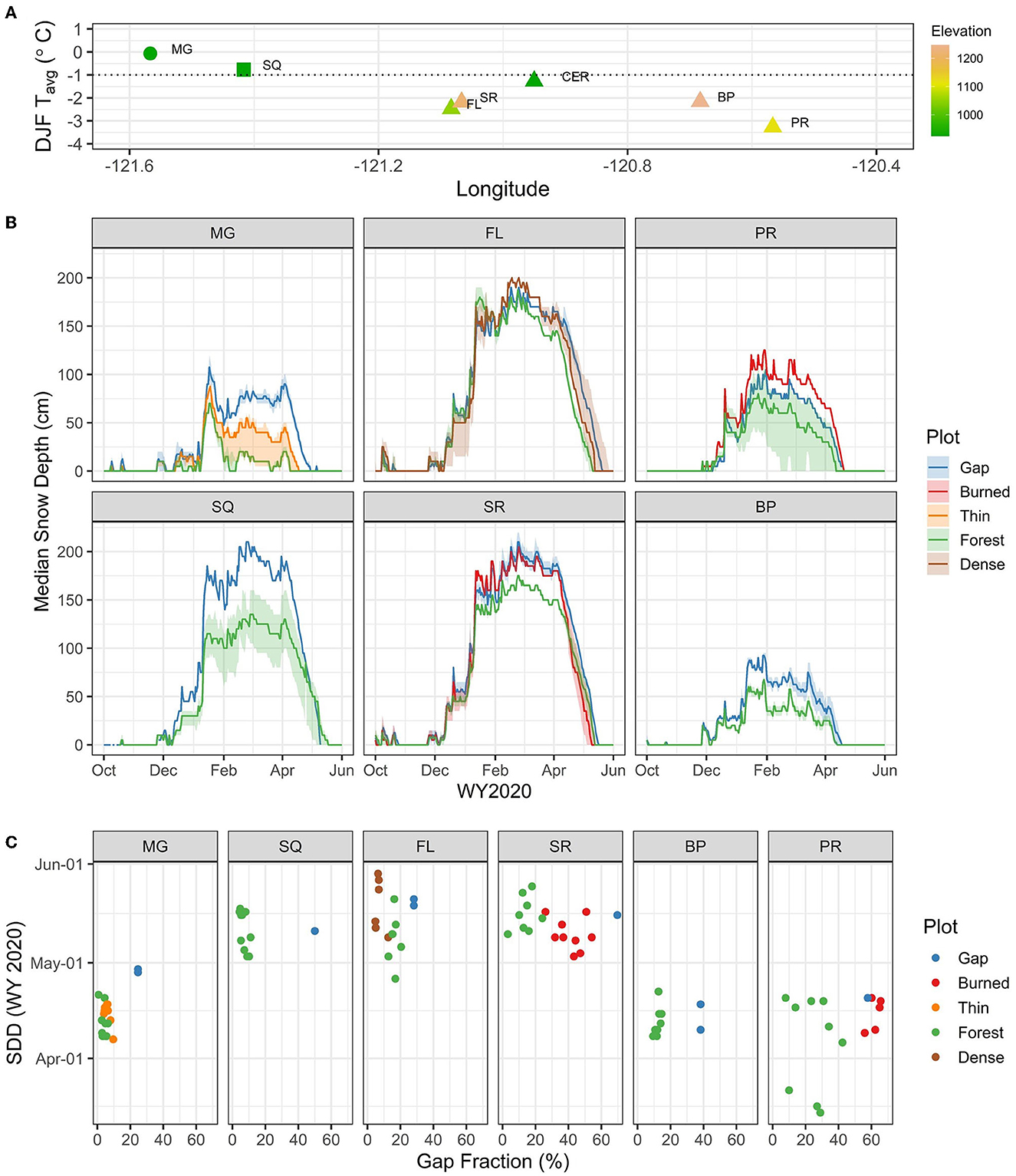
Figure 6. (A) Longitude and DJF mean temperature of the sites included in this study, with color showing elevation. (B) Snow depth during WY2020 from timelapse cameras and stations, arranged left to right approximately by longitude and excluding the two CER sites which are presented separately in Figure 7; lines reflect median values for each plot and colored envelope indicates range (see Table 2 for number of observations at peak depth and at SDD for each plot). (C) Snow disappearance day (SDD) during WY 2020 as a function of gap fraction over each observational location, at each site (excluding CER), arranged left to right by longitude, with colors indicating plot type.
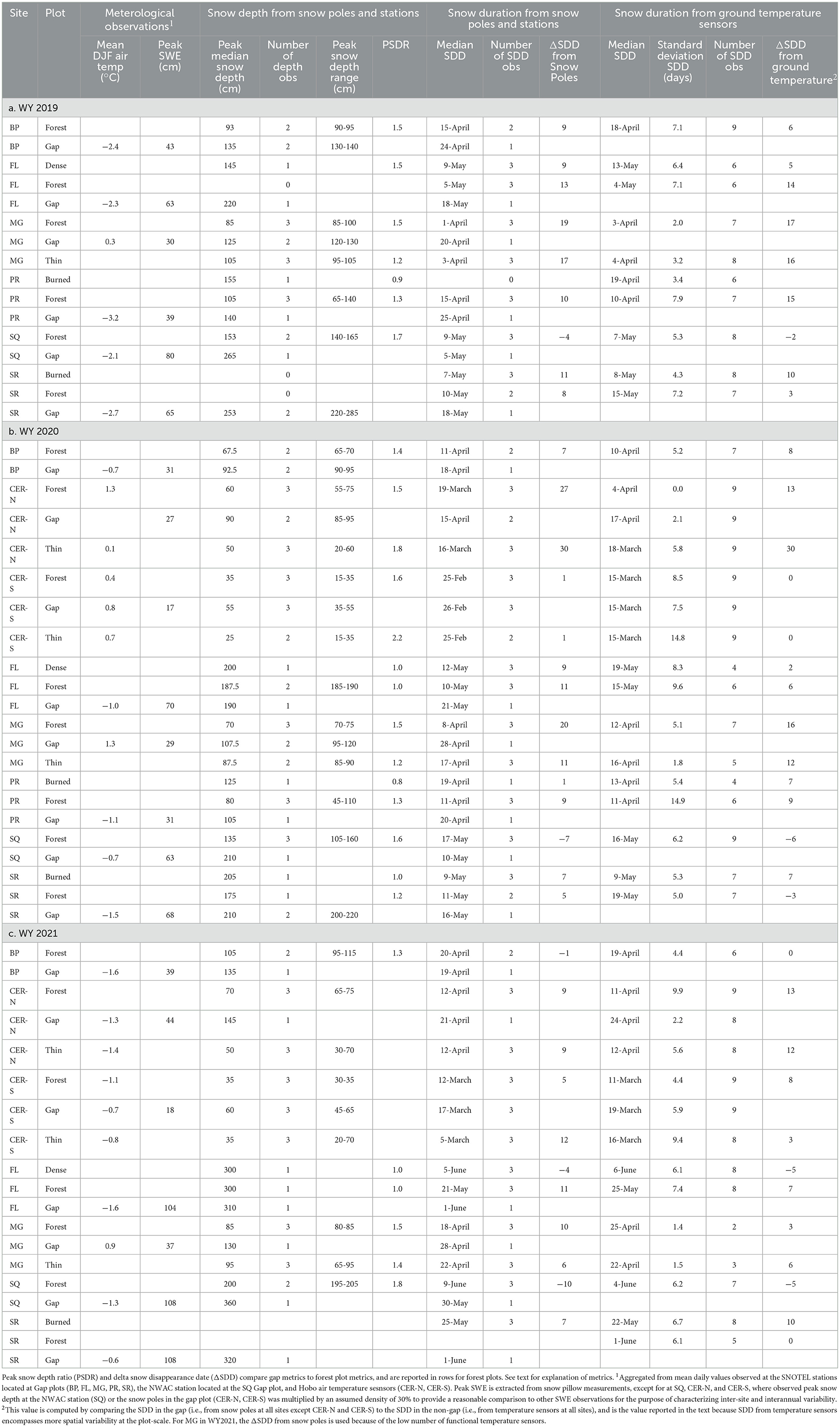
Table 2. Snow depth and snow duration from snow poles, snow stations, and ground temperature sensors for WY 2019-2021.
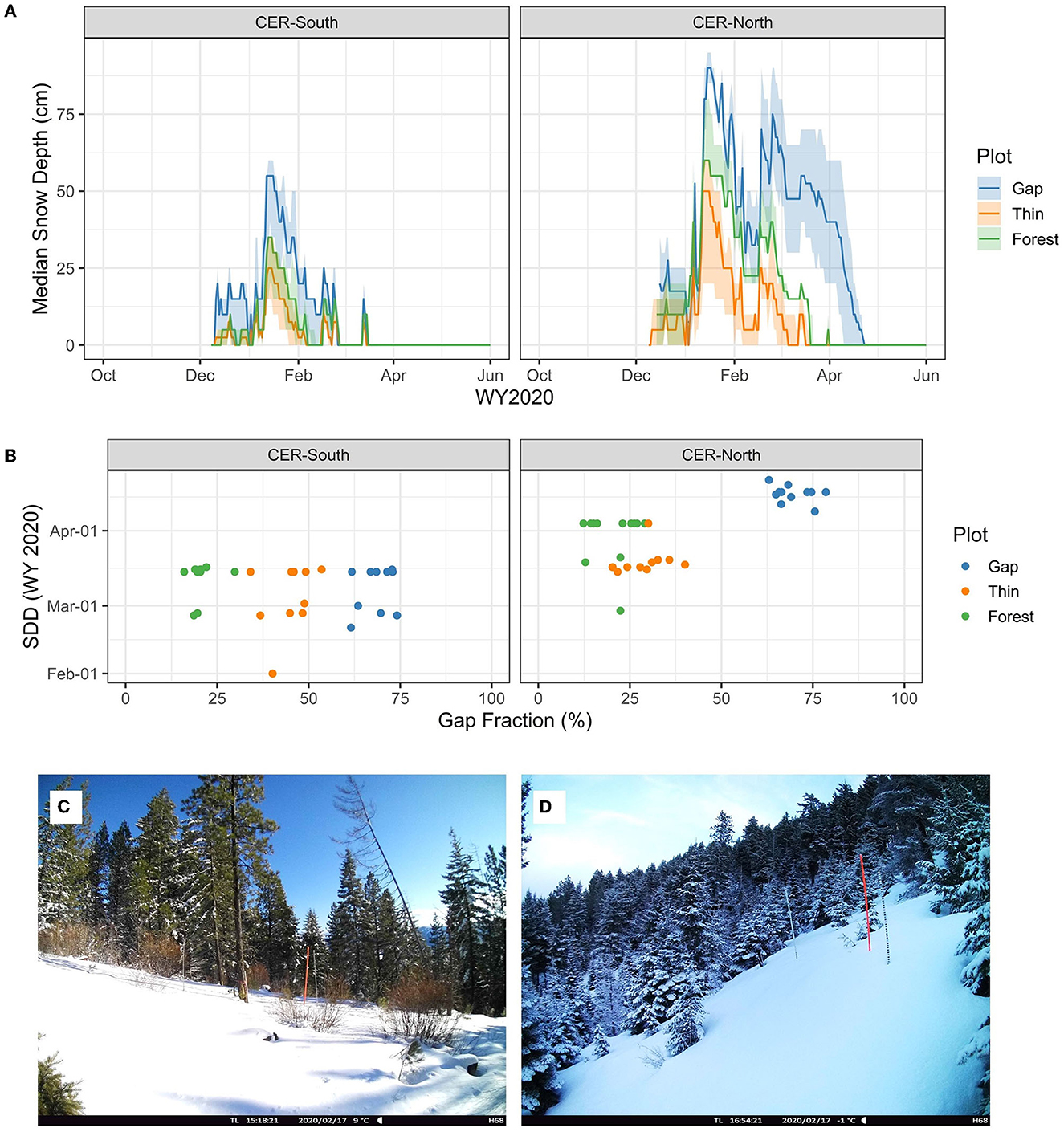
Figure 7. Snow observations during WY 2020 at the Cle Elum Ridge (CER) sites, each of which includes a forest, thinned forest, and gap plot, including snow depth (A), snow disappearance timing as a function of gap fraction (B), and afternoon photographs from 17 February 2020, which was the day after a storm, at the south-facing gap plot (C), and the north-facing gap plot (D).
Relative snow storage differences between plots at the same site
Relative differences in snow storage between plots at the same site, which are subject to similar climate conditions and incoming radiation, demonstrate the effect of forest density. Relative differences varied by site, and we present results as three groups of sites: (1) Western Cascades and Cascade Crest (MG and SQ), (2) Eastern Cascades (FL, SR, BP, and PR), and (3) Cle Elum Ridge (CER-N and CER-S). The two sites at Cle Elum Ridge are treated separately because of the contrast in aspect. Key results are summarized, and all observed values for snow storage magnitude and duration are reported in Table 2, with missing data at some plots and years due to instrument malfunction (e.g., battery failure, bear interference (Figure 4), snow overtopping poles). With 2–3 years of observations at all sites, interannual variability of weather contributes to variability in results, but overall patterns of relative snow storage were consistent; meteorological observations to characterize each winter are given in Table 2.
Western Cascades and Cascade Crest (MG and SQ)
Relative snow storage magnitude and duration was greater at the MG gap plot than either of the MG forest plots in all 3 years. The Peak Snow Depth Ratio (PSDR) indicated there was 1.5x greater snow storage in the gap plot as compared to the forest plot and 1.2 to 1.4x greater snow storage in the gap plot as compared to the thinned plot (Figure 6). Snow storage duration was 10–17 days longer in the gap than the un-thinned forest and 6–16 days longer in the gap than the thinned forest. These observations of relative snow storage are consistent with previous work at the MG site and in the western Cascades (Dickerson-Lange et al., 2015a,b). The longer snow duration in the gap is attributed to greater peak snow storage, due to higher snow accumulation rates, combined with higher rates of ablation in the forest plots during mid-winter melt events (Figure 6; e.g., note steeper declines in snow depth in the forest plot during January to early February melt event).
At the SQ site, located at a pass on the crest of the Cascades, peak snow storage was 1.6 to 1.8x greater in the gap, but snow storage duration was slightly longer in the forest in all years, with ΔSDD values −6 to −2. The longer snow duration in the forest is explained by a steeper ablation rate in the gap that counterbalances the higher accumulation rate in the gap (Figure 6). This relative difference between the forest and gap plots at SQ contrasts with previous observations of a PSDR of 2.9 and snow storage duration as 28 days longer in the gap (Dickerson-Lange et al., 2017); however, those observations were collected during the warmest winter on record in the Cascades (WY 2015) when DJF mean air temperature at SQ was 0.5°C.
Eastern Cascades (FL, SR, BP, and PR)
In the Eastern Cascades, relative differences in both snow storage magnitude and duration were small between forest and gap plots at FL and SR, which are located within 20 km of the Cascade Crest (Table 2). At both sites, PSDR ranged from 1.0 to 1.5, with a median of 1.0 across the two sites and three winters. Relative differences in snow storage duration as quantified by ΔSDD ranged from −5 to 14 days, with a median value of 3 days longer in the gap as compared to the forest plots.
Farther to the east, at BP and PR we observed greater relative snow storage magnitude in the gap plots but similar snow storage duration between gap and forest plots. The PSDR ranged from 1.3 to 1.5, with a median of 1.3, and ΔSDD ranged from −3 to 8 days, with a median value of 3 days longer in the gap as compared to the forested plots.
These results reflect two scenarios for which the combined effects of forest on snow processes resulted in similar relative snow storage duration between forest and gap plots. In the first scenario, illustrated by the PSDR ratios along with the time series of snow depth at the three plots at FL (Figure 6), snow accumulation rates, and therefore snow depth, are similar through the season, with minimal reductions due to canopy snow interception. In WY 2021 (not shown), the ablation rate became steeper in the forest plot as compared to the gap plot in the early spring, which resulted in slightly reduced snow duration in the forest plot. In general, however, both accumulation rates and ablation rates were similar between the forest and gap plots at FL. Similar rates resulted in similar snow storage magnitude and duration. In the second scenario, illustrated by observations at SR, BP, and PR, snow accumulation rates were moderately higher in the gap plots but snow ablation rates were also higher. Forest effects on accumulation and ablation rates balanced each other, which resulted in moderately more snow storage magnitude and similar snow storage duration.
Two eastern Cascade sites, SR and PR, included a burned forest plot, in addition to the unburned forest and gap plots, to further characterize the spectrum of forest conditions that are present on a landscape. Relative snow storage magnitude was similar or greater in the burned plots relative to the gap plots; PSDR values ranged from 0.8 to 1.0. Despite having higher snow storage magnitude, snow storage duration was shorter in the burned plots as compared to the gap plots. Values for ΔSDD ranged from 7 to 10, indicating longer duration in the gap plots. These observations are consistent with previous work that indicates that canopy snow interception is reduced within burned forests, resulting in similar snow accumulation rates, but that snow ablation rates are enhanced due to black carbon deposition and the resulting increase in net radiation (Burles and Boon, 2011; Gleason et al., 2013, 2019). Fires occurred in 2017 at SR and 2014 at PR (Table 1), and previous remote sensing analysis by Gleason et al. (2019) indicates that post-fire reductions in snow storage duration can persist for at least 10 years.
Eastern Cascades - Cle Elum Ridge (CER-N and CER-S)
There were substantial differences in absolute snow storage on either side of Cle Elum Ridge, with approximately 2–3x more snow in the CER-N gap as compared to the CER-S gap, but relative snow storage duration between the forest, thinned forest, and gap plots located on each side of the ridge was also markedly different (Figure 7; Table 2). At both the north-facing and south-facing sites, there was greater snow storage magnitude in the gap plots as compared to the forest and thinned plots. Values for PSDR ranged from 1.5 to 2.9 (median of 2.0). However, on the north side of the ridge (CER-N), relative snow storage duration was longer in the gap plot as compared to the forest and thinned forest plots, and ΔSDD values ranged from 12 to 30 days (median of 13). In contrast, on the south side of the ridge (CER-S), relative snow storage duration was similar among forest and gap plots and values for ΔSDD ranged from 0 to 8 days (median of 2).
The differences in both absolute and relative snow storage between the two topographic positions are likely related to heat load differences. At the south-facing site, air temperature was similar or slightly higher during the winter (DJF) and consistently higher in the spring (MA). Mean DJF maximum daily temperature was 0.6 and −0.9°C at the south-facing site in WY 2020 and 2021, respectively, as compared to 0.7 and −1.3°C at the north-facing site. Mean MA maximum daily temperature was 16.9 and 19.5°C at the south-facing site vs. 13.8 and 16.6°C at the north-facing site. The observed warmer air temperatures may be partially explained by enhanced direct radiation on the sensors at the CER-S plots, despite using radiation shields and installing them within the branches of conifer trees to minimize direct radiation to the sensors. However, even within the forest plots with a more closed canopy and minimal direct radiation to the sensors, we observed consistently warmer air temperatures at the south-facing site, which is likely due to enhanced solar heating and the resulting enhanced longwave radiation from trees and non-snow covered ground surfaces (Pomeroy et al., 2009; Webster et al., 2016). The increased heat load on the south-facing site likely contributes to warming of the ground surface prior to snow accumulation and to increased ablation of the ground snowpack (Seyfried et al., 2021).
In addition, warmer air temperatures, more direct solar radiation, and more solar-enhanced longwave radiation on the south side of the ridge would result in more melting of canopy-stored snow, rather than the snow blowing or sliding off and becoming part of the ground snowpack (Lumbrazo et al., 2022). Photographic evidence from time-lapse cameras supports the difference in canopy snow storage duration between CER-N and CER-S and suggests that canopy snow melts rapidly on the south-facing site (Figure 7). Overall, relative snow storage on the south side of the ridge is likely dominated by effects of high heat load, which results in enhanced ablation rates of both canopy-stored snow and ground snowpack. In contrast, relative snow storage on the north side of the ridge is dominated by the effects of canopy snow interception, with enhanced accumulation rates in the gap, along with topographic shading that diminishes differences in ablation rates between the gap and the forest plots.
Discussion
Comparing observations to hypotheses based on conceptual model
The relative snow storage comparisons quantified by these data fill a spatial data gap within the transitional climate zone of the Eastern Cascades. These data provide an observational comparison to hypotheses for how forest-snow processes combine to influence snow storage. The Western Cascades sites, including MG and SQ, were previously classified within the framework based on low wind exposure, warm winter temperatures (i.e., ≥ −1°C), and early melt timing (i.e., majority of melt before the spring equinox). Thus, we expected that snow storage magnitude would be much greater and snow storage duration would be much longer in gaps as compared to forests at these two sites. Observations from the MG site are aligned with this classification, and increased snow accumulation rates in the gap combined with reduced ablation rates in the gap relative to the forest during warm, mid-winter melt events resulted in snow storage that was approximately 2 weeks longer in the gap. However, at SQ, which is located at the Cascade Crest, large increases in snow accumulation rates in the gap as compared to the forest were more than counterbalanced by enhanced ablation rates in the gap, which resulted in snow storage duration that was longer in the forest. These results run counter to one winter of previous observations of longer snow storage duration in the gap plot at this site (Dickerson-Lange et al., 2017). The net effect of longer snow storage duration in the forest has previously been observed in windy environments and in colder, continental climates, but was not expected at SQ. We speculate that the possible explanations for this dynamic could include three factors that may have been misrepresented by the data used for the initial classification: (1) greater influence of wind exposure on snow deposition patterns than the topographic curvature at the site suggests; (2) later melt timing, which enhances the role of forest shading; or (3) variable local weather patterns at the pass, which alternate between warmer and wetter air masses moving from the west, and colder and drier air masses from the east (Wayand et al., 2016, 2017).
Relative snow storage at the Eastern Cascades sites was generally consistent with the hypothesis that snow storage magnitude would be moderately higher in the gaps as compared to the forest plots, but that snow storage duration would be approximately equal. This hypothesis is based on the balanced effect that forests have on snow processes in colder climate conditions. Under-canopy accumulation rates are reduced, but not as drastically as in maritime climates where snow cohesion and therefore canopy snow interception rates are very high. Lower under-canopy accumulation rates are coupled with reduced under-canopy ablation rates, which results in approximately equal snow storage duration between gap and forest plots. At all of the sites across the Eastern Cascades except for CER-N, which is north facing, we observed relative snow storage duration differences of a few days to up to two weeks between gap and forest plots. At one site, FL, similar values for snow storage duration appear to be the result of almost identical accumulation and ablation rates between all plots, but at all other sites we observed enhanced snow accumulation rates balanced by enhanced snow ablation rates in the gap plots.
Observations from the CER-N site conflict with the hypothesis of similar relative snow storage duration between gap and forest plots, and also with the hypothesis that aspect is secondary to climate variables for determining relative snow storage. We initially considered aspect as a secondary influence because previous studies have demonstrated that the timing of the ablation season, and the associated solar elevation, are more dominant influences than slope aspect on the difference in net radiation between a forested and open plot (Strasser et al., 2011; Seyednasrollah and Kumar, 2014). Because our study sites experience cold winter temperatures and relatively early timing of ablation, we hypothesized that observed forest-snow storage relationships would match the hypothesized “cold and early” class, and that topographic position would secondarily contribute variability to relative snow storage metrics. In the “cold and early” classification, snow storage magnitude is greater in gap plots, but snow storage duration is equal between gap and forest plots. In contrast, we observed that relative snow storage at CER-N was fundamentally different than at CER-S and the other Eastern Cascade sites. As expected, snow accumulation rates were enhanced in the CER-N gap plot relative to the CER-N forest plot. However, topographic shading reduced incoming solar radiation to both plots, thereby reducing the effect of forest shading on ablation rates. The result was similar ablation rates at the gap and forest plots. Because the gap plot accumulated more snow overall, snow storage duration was 2–4 weeks longer in the gap as compared to the forest. These results match hypothesized relative snow storage dynamics in warmer maritime climates, such as the western Cascades and western Sierra Nevada.
The hypothesis that aspect exerts a secondary influence on relative snow storage was also based on previous work across the Pacific Northwest that suggested that forest modifications of snow accumulation processes are more influential than modifications of ablation processes for determining relative snow storage (Dickerson-Lange et al., 2017, 2021). This hierarchy is well-supported by previous work in the warmer, higher latitude, and cloudier conditions of the Pacific Northwest region (USA). However, previous work also suggests that aspect has a strong influence on relative snow storage in the lower latitudes of the western USA, where higher solar elevations and frequent clear sky conditions enhance the role of forest shading for modifying snow ablation processes (Anderson, 1963; Musselman et al., 2008; Harpold et al., 2015; Broxton et al., 2020). In a study in Arizona, Broxton et al. (2020) demonstrated that snow storage magnitude is highest on north-facing slopes in forests with 30-50% canopy cover as compared to openings and denser forests. They also found that at nearby south-facing slopes, snow storage declines with increasing forest density, which they attribute to the domination of reduced accumulation and enhanced longwave radiation over reduced shortwave radiation. Recent high resolution modeling demonstrates that forest-influenced patterns of accumulation can be overridden by ablation patterns on south-facing slopes (Mazzotti et al., 2022). Thus, we suggest that the relative snow storage scenario observed at CER-N is the result of forest-modified accumulation processes dominating over forest-modified ablation processes, which is the case for the maritime climate of the Western Cascades. Snow depth observations at CER-N show substantially higher snow accumulation rates and slightly higher snow ablation rates in the gap relative to the forest or thinned plots (Figure 6). Given that the two CER sites are at the lowest elevations and are subject to the warmest winter temperatures among the Eastern Cascades study sites, additional observations are needed to fully understand how landscape-scale forest management initiatives will impact hydrologic resilience, particularly in the lower elevation lands that are included in the wildland-urban interfaces. Field observations or remotely sensed measurements that cover more elevations and gap sizes across a range of topographic positions are needed to characterize the influence of both aspect and forest structure on relative snow storage across this climate zone.
Lastly, we observed variability in forest species composition and structure, which co-varied with climate, to be a secondary influence on relative snow storage difference between forest and gap plots. The Eastern Cascades sites sample a range of forest types (Table 1) and canopy densities (Figures 6C, 7B), but the overall patterns of relative snow storage are similar across all years of observations at the Eastern Cascades sites, with the exception of CER-N, discussed above. One example is the similarity in relative snow storage between BP and PR during WY 2019 (not shown) and WY 2020 (Figure 6). The BP forest plot is dominated by Grand fir with gap fraction values at observation points that range from 5 to 15%, whereas the PR forest plot is dominated by Ponderosa pine with gap fraction values at observation points that range from 8 to 42% (Figure 6C). Even with this level of variability in forest type and canopy density, the PDSR was 1.3–1.5 at both sites over 2 years and ΔSDD was 6–8 days and 9–15 days at BP and PR, respectively. Although this study is not exhaustive in sampling different forest characteristics, these results provide limited evidence that forest characteristics are secondary to climate in influencing relative snow storage differences in this climate zone.
Management applications and future work
This study was largely motivated by a need to characterize the hydrologic effects of reducing fire fuels across dry forest ecosystems in the Eastern Cascades in WA, but the findings are applicable to multi-objective forest management strategies across the western USA. Both thinning and canopy gap-creation are used to create structural variability on the landscape and create different conditions in terms of productivity, regeneration, and wildfire fuels (e.g., Churchill et al., 2013). These observations provide a first step toward directly quantifying the effects of forest density on both snow storage magnitude and duration in this climate zone. Two key findings are relevant to current forest management considerations: (1) reducing forest density in this climate zone via thinning or canopy gap creation may have a greater effect on overall water balance (i.e., water quantity) and a lesser effect on the timing of water availability; and (2) thinning and canopy gap-creation in north-facing forests may be the best opportunity for extending snow storage duration.
In the Eastern Cascades, we find that relative snow storage magnitude ranges from equivalent to greater in gaps as compared to forest plots. Thus, uneven thinning that creates small gaps, along with targeted canopy gap creation in this climate zone, may increase local water availability by increasing snow accumulation rates. However, with little to no difference in snow disappearance timing between gap and forest plots, these observations suggest that reductions in forest density are unlikely to extend snow storage duration except in areas dominated by north facing slopes.
An increase in snow storage magnitude alone may increase the snowmelt-driven hydrograph peak and thus extend water availability later in the season through an elongated recession curve. However, snow at all of our sites disappeared by early June, and thus, late season streamflow likely depends more strongly on summer weather, subsurface water storage, and evapotranspiration. Previous modeling of forest gaps, forest strips, or thinning in the region has suggested that reducing forest density could increase both water yield and late-summer streamflow (Wigmosta et al., 2015; Sun et al., 2018; Currier et al., 2022). These studies partially attribute the effects to snow storage duration, and therefore our observations point to the need for testing against observations and for additional observations and analysis related to hydrologic partitioning across the range of climate, forest, and topographic conditions in the Eastern Cascades.
Aside from effects on streamflow, snow storage also affects the amount and timing of soil moisture availability. Forest management to increase snow storage magnitude may thus increase forest resilience regardless of its effect on snow storage duration. Soil moisture deficits at depth play a critical role in water supply to forests during drought conditions (Belmonte et al., 2022), and may be more affected by snow storage magnitude and evaporative demand than by snow storage duration. Soil water deficits during recent droughts have been shown to end transpiration in Ponderosa pine forests (Sankey and Tatum, 2022), shutting down carbon assimilation and possibly leading to foliar damage. Management to reduce water stress is therefore becoming increasingly important with increased drought conditions, which may ultimately change forest composition and distribution in a changing climate (Grant et al., 2013). In the Eastern Cascades, reducing canopy density via thinning or gap creation is likely both to reduce competition for soil water among trees and also to increase available soil water.
Leveraging advances in both remote sensing and modeling will continue to be critical to extrapolate field-based observations to spatial and temporal scales that are relevant to management planning and implementation. The observations presented herein provide evidence for the spatial variability in plot-scale relative snow storage and also document the full time series of how differences evolved through a snow season. Snow-on lidar acquired at key points in a snow season, such as the timing of peak snow storage, could provide insight into the spatial drivers of differences in relative snow storage magnitude and duration, such as elevation and aspect. Lidar-based analysis at finer spatial scales, rather than plot-scale aggregations, could be used to characterize plot-scale variations in snow depth that are neglected with only 1–3 point observations. High resolution snow observations could also be used to develop predictive relations for topographic position and forest characteristics and structure, such as gap size and canopy density, across this climate zone. Additional years of high resolution observations would also improve understanding of forest-snow interactions across interannual and intra-annual variations in weather, including considerations of the sequencing of storm events that affect canopy snow interception (Shidei et al., 1952; Miller, 1964) and the occurrence of warm Chinook winds in the Eastern Cascades that affect ablation (Swanson, 1980). Lastly, further development of recent work to leverage remote sensing and machine learning to estimate snow density and SWE at landscape scales (Broxton et al., 2019) could be applied to address uncertainty in relative snow storage magnitude that is introduced by neglecting potential differences in snow density between gap and forest plots (Veatch et al., 2009; Bonner et al., 2022).
The observed difference in relative snow storage in north-facing versus south-facing topographic positions suggests that gap creation on north-facing slopes may be the best opportunity to increase the amount and duration of water storage in the Eastern Cascades. Additional empirical data across more elevations, topographic positions, and gap sizes are needed, but these results indicate that managing forests with small canopy gaps may improve local soil water availability. A north-aspect management approach could also reduce risk of high severity wildfire (Povak et al., 2022) and support the development and maintenance of climate refugia, since many species of wildlife already seek north-facing slopes for temperature regulation (Krosby et al., 2018). In addition, extension of snow storage duration by a few days to weeks may be especially important in the Eastern Cascades, where temperature, quantity, and timing of water in streams has direct impacts on the survival and recovery of salmon (Kock et al., 2016; Cline et al., 2020). Interestingly, these proposed actions contradict general recommendations for north-facing forests, where less intensive thinning is needed to mimic the historical landscape and vegetation structure (Hessburg et al., 2021). However, rapidly changing climate conditions may require creatively managing for the needs of both people and nature, and possibly embracing novel ecosystem patterns (Schlaepfer and Lawler, 2022), which could include departing from historically denser forests on north-facing slope aspects in order to manage for future water availability and climate refugia.
Conclusions
Through field-based observations, we quantified the effect of forest density on the magnitude and duration of snow storage in the Eastern Cascades, WA. This field effort included sites along a transect spanning the Western to Eastern Cascades, but focused on the Eastern Cascades because this region (1) represents a critical spatial and climatic data gap for forest-snow observations; (2) is heavily dependent on snowpack for its water supply; and (3) is a hotspot of forest management activity due to increasing wildfire risk and decreasing snow and soil water storage due to climate warming. Our data show that the effect of forest density on snow storage is spatially variable. Snow storage magnitude is greater in forest gaps as compared to under forest canopies, and snow storage duration is similar in gap and forest conditions on the eastern slopes of the Cascade Mountain range. This study thus indicates that reducing forest density in the Eastern Cascades may increase the overall water balance (i.e., water quantity), but will have minimal effect on the timing of water availability. Snow disappearance date was similar among gap and forest plots at most sites, which reflects the balance between forest modifications of snow accumulation and snow ablation processes. Overall, these findings are largely consistent with a previously proposed hierarchy for forest-snow processes (Dickerson-Lange et al., 2021). A key exception, however, occurs at one low elevation, north-facing site where snow storage duration was substantially longer in the forest gap as compared to the dense forest. This indicates that aspect may be a primary driver of snow storage magnitude and duration differences across forest densities in the transitional climate zone. North-aspect slopes thus represent the best opportunity for influencing the timing of water availability via forest-snow processes. Overall, these results suggest that forest thinning prescriptions intended to improve forest health and wildfire risk are unlikely to exacerbate the hydrologic impacts of climate change. Lastly, these data can be used in support of modeling hydrological effects of forest management at spatial and temporal scales that are relevant to management decisions.
Data availability statement
The datasets presented in this study can be found in online repositories. The names of the repository/repositories and accession number(s) can be found at: CUAHSI HydroShare; http://www.hydroshare.org/resource/6127902b82674b8097ec3c372f14514f.
Author contributions
SD-L, EH, RG, and JL contributed to the conception and design of the investigation. KP led the field instrumentation, data management, and GIS mapping efforts. SD-L, EH, RG, and KP collected field data. SD-L and JL led the analysis and interpretation of the snow data. RG and EH led the analysis and interpretation of the forest data. SD-L, EH, and RG wrote the first draft of the manuscript. SD-L created the figures. JL provided feedback to shape the direction of revisions. All authors contributed to manuscript revision and approved the submitted version.
Funding
Funding was provided by the Tieton River Conservation Area Fund at the Yakima Valley Community Foundation with approval by the Tapash Forest Collaborative board, the Washington Department of Natural Resources, and The Nature Conservancy of Washington. Funding for open access publication fees was provided by Natural Systems Design.
Acknowledgments
We are grateful to editor Ning Sun, and to Timothy Link and Joel Biederman for constructive review comments that substantially improved this manuscript. We thank the many people who contributed time, expertise, and resources to allow us to conceive of, execute, and learn from a complex field investigation. In particular, we thank Scott Pattee at the NRCS for continuing support of our studies at SNOTEL stations; Jamie Robertson and Michael Case for lending a hand in the field; Ryan Currier, Cassie Wells Lumbrazo, and other members of the UW Mountain Hydrology Research Group for helping us find and use extra equipment; Amir Harding and Ian Shiach for contributions to timelapse photograph processing; Julia Jay for support with data processing and visualization; Phil Levin for administrative support; and Paul Hessburg, Derek Churchill, and Mark Wigmosta for contributing to early discussions on the motivation and experimental design of this study. We are additionally appreciative of the partners, grandparents, teachers, and childcare professionals who made it possible for scientists who are parents to also complete field research and data analysis, particularly during a global pandemic. Lastly, thanks to the bear that ate our CER-S forest plot camera (but left the SD card intact!) in 2021 for keeping data processing interesting.
Conflict of interest
The authors declare that the research was conducted in the absence of any commercial or financial relationships that could be construed as a potential conflict of interest.
Publisher's note
All claims expressed in this article are solely those of the authors and do not necessarily represent those of their affiliated organizations, or those of the publisher, the editors and the reviewers. Any product that may be evaluated in this article, or claim that may be made by its manufacturer, is not guaranteed or endorsed by the publisher.
References
Agee, J. K. (2003). Historical range of variability in eastern Cascades forests, Washington, USA. Landscape Ecol. 18, 725–740. doi: 10.1023/B:LAND.0000014474.49803.f9
Anderson, H. W. (1963). Managing California's Snow Zone Lands for Water - USFS Research Paper PSW- 6 1963. Berkeley, California.
Bales, R. C., Hopmans, J. W., O'Geen, A. T., Meadows, M., Hartsough, P. C., Kirchner, P., et al. (2011). Soil moisture response to snowmelt and rainfall in a sierra nevada mixed-conifer forest. Vadose Zone J. 10, 786. doi: 10.2136/vzj2011.0001
Beckschafer, P. (2015). Hemispherical 2, 0.- Batch processing hemispherical and canopy photographs with ImageJ- User Manual. Gottingen: Chair of Forest Inventory and Remote Sensing, Georg-August-Universitat Gottingen.
Belmonte, A., Ts. Sankey, T., Biederman, J., Bradford, J. B., and Kolb, T. (2022). Soil moisture response to seasonal drought conditions and post-thinning forest structure. Ecohydrology 15, 2406. doi: 10.1002/eco.2406
Bonner, H. M., Raleigh, M. S., and Small, E. E. (2022). Isolating forest process effects on modelled snowpack density and snow water equivalent. Hydrol. Proc. 36, 14475. doi: 10.1002/hyp.14475
Broxton, P. D., Harpold, A. A., Biederman, J. A., Troch, P. A., Molotch, N. P., Brooks, P. D., et al. (2015). Quantifying the effects of vegetation structure on snow accumulation and ablation in mixed-conifer forests. Ecohydrology 8, 1073–1094. doi: 10.1002/eco.1565
Broxton, P. D., Leeuwen, W. J. D., and Biederman, J. A. (2019). Improving snow water equivalent maps with machine learning of snow survey and lidar measurements. Water Res. Res. 55, 3739–3757. doi: 10.1029/2018WR024146
Broxton, P. D., Leeuwen, W. J. D., and Biederman, J. A. (2020). Forest cover and topography regulate the thin, ephemeral snowpacks of the semiarid Southwest United States. Ecohydrology 13, 2202. doi: 10.1002/eco.2202
Brusa, D., Bunker, D. D. E., Brusa, A., and Bunker, D. D. E. (2014). Increasing the precision of canopy closure estimates from hemispherical photography: blue channel analysis and under-exposure. Agric. Forest Meteorol. 102–107, 195–196. doi: 10.1016/j.agrformet.2014.05.001
Burles, K., and Boon, S. (2011). Snowmelt energy balance in a burned forest plot, crowsnest pass, Alberta, Canada. Hydrol. Proc. 25, 3012–3029. doi: 10.1002/hyp.8067
Carson, D. R. (2010). Quantification of Snow Pack Mass and Energy Dyamics Across a Canopy Discontinuity. Idaho: University of Idaho.
Chen, J. M., Black, T. A., and Adams, R. S. (1991). Evaluation of hemispherical photography for determining plant area index and geometry of a forest stand. Agric. Forest Meteorol. 56, 129–143. doi: 10.1016/0168-1923(91)90108-3
Church, J. E. (1912). The conservation of snow. Sci. Am. Suppl. 4, 152–155. doi: 10.1038/scientificamerican09071912-152supp
Churchill, D. J., Larson, A. J., Dahlgreen, M. C., Franklin, J. F., Hessburg, P. F., and Lutz, J. (2013). Restoring forest resilience: From reference spatial patterns to silvicultural prescriptions and monitoring. Forest Ecol. Manage. 291, 442–457. doi: 10.1016/j.foreco.2012.11.007
Clark, M. P., Hendrikx, J., Slater, A. G., Kavetski, D., Anderson, B., Cullen, N. J., et al. (2011). Representing spatial variability of snow water equivalent in hydrologic and land-surface models: a review. Water Res. Res. 47, W07539. doi: 10.1029/2011WR010745
Clark, M. P., Nijssen, B., Lundquist, J. D., Kavetski, D., Rupp, D. E., Woods, R. A., et al. (2015). A unified approach for process-based hydrologic modeling: 1. Modeling concept. Water Res. Res. 51, 1–17. doi: 10.1002/2015WR017198
Cline, T. J., Schindler, D. E., Walsworth, T. E., French, D. W., and Lisi, P. J. (2020). Low snowpack reduces thermal response diversity among streams across a landscape. Limnol. Oceanograph. Lett. 5, 254–263. doi: 10.1002/lol2.10148
Currier, W. R., and Lundquist, J. D. (2018). snow depth variability at the forest edge in multiple climates in the western United States. Water Res. Res. 54, 8756–8773. doi: 10.1029/2018WR022553
Currier, W. R., Sun, N., Wigmosta, M., Cristea, N., and Lundquist, J. D. (2022). The impact of forest-controlled snow variability on late-season streamflow varies by climatic region and forest structure. Hydrol. Proc. 36, e14614. doi: 10.1002/hyp.14614
Dewitz, J. (2019). National Land Cover Database (NLCD) 2016 Products (ver. 2.0, July 2020): U.S. Geological Survey Data Release. doi: 10.5066/P96HHBIE
Dickerson-Lange, S. E., Gersonde, R. F., Hubbart, J. A., Link, T. E., Nolin, A. W., Perry, G. H., et al. (2017). Snow disappearance timing is dominated by forest effects on snow accumulation in warm winter climates of the Pacific Northwest, United States. Hydrol. Proc. 31, 1846–1862. doi: 10.1002/hyp.11144
Dickerson-Lange, S. E., Gersonde, R. F., Vano, J. A., and Lundquist, J. D. (2021). Ranking forest effects on snow storage: a decision tool for forest management. Water Res. Res. 57, e2020. doi: 10.1029/2020WR027926
Dickerson-Lange, S. E., Lutz, J. A., Gersonde, R., Martin, K. A., Forsyth, J. E., Lundquist, J. D., et al. (2015b). Observations of distributed snow depth and snow duration within diverse forest structures in a maritime mountain watershed. Water Res. Res. 51, 9353–9366. doi: 10.1002/2015WR017873
Dickerson-Lange, S. E., Lutz, J. A., Martin, K. A., Raleigh, M. S., Gersonde, R., Lundquist, J. D., et al. (2015a). Evaluating observational methods to quantify snow duration under diverse forest canopies. Water Res. Res. 51, 1203–1224. doi: 10.1002/2014WR015744
Donley, E. E., Naiman, R. J., and Marineau, M. D. (2012). Strategic planning for instream flow restoration: a case study of potential climate change impacts in the central Columbia River basin. Global Change Biol. 18, 3071–3086. doi: 10.1111/j.1365-2486.2012.02773.x
Elder, K., Rosenthal, W., and Davis, R. E. (1998). Estimating the spatial distribution of snow water equivalence in a montane watershed\r. Hydrol. Proc.12, 1793–1808. doi: 10.1002/(SICI)1099-1085(199808/09)12:10/11<1793::AID-HYP695>3.0.CO;2-K
Ellis, C. R., and Pomeroy, J. W. (2007). Estimating sub-canopy shortwave irradiance to melting snow on forested slopes. Hydrol. Proc. 21, 2581–2593. doi: 10.1002/hyp.6794
Ellis, C. R., Pomeroy, J. W., Brown, T., and MacDonald, J. (2010). Simulation of snow accumulation and melt in needleleaf forest environments. Hydrol. Earth System Sci. 14, 925–940. doi: 10.5194/hess-14-925-2010
Ellis, C. R., Pomeroy, J. W., Essery, R. L. H., and Link, T. E. (2011). Effects of needleleaf forest cover on radiation and snowmelt dynamics in the Canadian Rocky Mountains. Can. J. Forest Res. 41, 608–620. doi: 10.1139/X10-227
Essery, R., and Pomeroy, J. (2004). Vegetation and topographic control of wind-blown snow distributions in distributed and aggregated simulations for an arctic tundra basin. J. Hydrometeorol. 5, 735–744. doi: 10.1175/1525-7541(2004)005<0735:VATCOW>2.0.CO;2
Essery, R., Pomeroy, J., Ellis, C., and Link, T. (2008). Modelling longwave radiation to snow beneath forest canopies using hemispherical photography or linear regression. Hydrol. Pro. 22, 2788–2800. doi: 10.1002/hyp.6930
Essery, R., Rutter, N., Pomeroy, J., Baxter, R., Stähli, M., Gustafsson, D., et al. (2009). SNOWMIP2: an evaluation of forest snow process simulations. Bulletin Am. Meteorol. Soc. 90, 1120–1135. doi: 10.1175/2009BAMS2629.1
Everett, R. L., Schellhaas, R., Keenum, D., Spurbeck, D., and Ohlson, P. (2000). Fire history in the ponderosa pine/Douglas-fir forests on the east slope of the Washington Cascades. Forest Ecol. Manage. 129, 207–225. doi: 10.1016/S0378-1127(99)00168-1
Ford, K. R., Ettinger, A. K., Lundquist, J. D., Raleigh, M. S., and Hille Ris Lambers, J. (2013). Spatial heterogeneity in ecologically important climate variables at coarse and fine scales in a high-snow mountain landscape. PloS ONE 8, e65008. doi: 10.1371/journal.pone.0065008
Franklin, J. F., and Dyrness, C. T. (1973). Natural Vegetation of Oregon and Washington. Washington, DC: US Government Printing Office.
Glatthorn, J., and Beckschäfer, P. (2014). Standardizing the protocol for hemispherical photographs: accuracy assessment of binarization algorithms. PloS ONE 9, e111924. doi: 10.1371/journal.pone.0111924
Gleason, K. E., McConnell, J. R., Arienzo, M. M., Chellman, N., and Calvin, W. M. (2019). Four-fold increase in solar forcing on snow in western U.S. burned forests since 1999. Nat. Commun. 10,2026. doi: 10.1038/s41467-019-09935-y
Gleason, K. E., Nolin, A. W., and Roth, T. R. (2013). Charred forests increase snowmelt: effects of burned woody debris and incoming solar radiation on snow ablation. Geophys. Res. Letters 40, 4654–4661. doi: 10.1002/grl.50896
Goeking, S. A., and Tarboton, D. G. (2020). Forests and water yield: a synthesis of disturbance effects on streamflow and snowpack in western coniferous forests. J. Forestry 118, 172–192. doi: 10.1093/jofore/fvz069
Golding, D. L., and Swanson, R. H. (1986). Snow distribution patterns in clearings and adjacent forest. Water Res. Res. 22, 1931–1940. doi: 10.1029/WR022i013p01931
Grant, G. E., Tague, C. L., and Allen, C. D. (2013). Watering the forest for the trees: an emerging priority for managing water in forest landscapes. Front. Ecol. Environ. 11, 314–321. doi: 10.1890/120209
Halofsky, J. E., Peterson, D. L., and Harvey, B. J. (2020). Changing wildfire, changing forests: the effects of climate change on fire regimes and vegetation in the Pacific Northwest, USA. Fire Ecol. 16, 1–26. doi: 10.1186/s42408-019-0062-8
Harpold, A. A., Krogh, S. A., Kohler, M., Eckberg, D., Greenberg, J., Sterle, G., et al. (2020). Increasing the efficacy of forest thinning for snow using high-resolution modeling: a proof of concept in the Lake Tahoe Basin, California, USA. Ecohydrology 13, 2203. doi: 10.1002/eco.2203
Harpold, A. A., Molotch, N. P., Musselman, K. N., Bales, R. C., Kirchner, P. B., Litvak, M., et al. (2015). Soil moisture response to snowmelt timing in mixed-conifer subalpine forests. Hydrol. Processes 29, 2782–2798. doi: 10.1002/hyp.10400
Haugo, R., Zanger, C., DeMeo, T., Ringo, C., Shlisky, A., Blankenship, K., et al. (2015). A new approach to evaluate forest structure restoration needs across Oregon and Washington, USA. Forest Ecol. Manage. 335, 37–50. doi: 10.1016/j.foreco.2014.09.014
Hedstrom, N. R., and Pomeroy, J. W. (1998). Measurements and modelling of snow interception in the boreal forest. Hydrol. Proc. 12, 1611–1625. doi: 10.1002/(SICI)1099-1085(199808/09)12:10/11<1611::AID-HYP684>3.0.CO;2-4
Hessburg, P. F., Prichard, S. J., Hagmann, R. K., Povak, N. A., and Lake, F. K. (2021). Wildfire and climate change adaptation of western North American forests: a case for intentional management. Ecol. Appl. 31, 2432. doi: 10.1002/eap.2432
Hessburg, P. F., Smith, B. G., Salter, R. B., Ottmar, R. D., and Alvarado, E. (2000). Recent changes (1930s−1990s) in spatial patterns of interior northwest forests, USA. Forest Ecol. Manage. 136, 53–83. doi: 10.1016/S0378-1127(99)00263-7
Hiemstra, C. A., Liston, G. E., and Reiners, W. A. (2002). Snow redistribution by wind and interactions with vegetation at upper treeline in the medicine bow mountains, wyoming, U.S.A. Arctic Antarctic Alpine Res. 34, 262. doi: 10.1080/15230430.2002.12003493
Hiemstra, C. A., Liston, G. E., and Reiners, W. A. (2006). Observing, modelling, and validating snow redistribution by wind in a Wyoming upper treeline landscape. Ecol. Modell. 197, 35–51. doi: 10.1016/j.ecolmodel.2006.03.005
Hubbart, J. A., Link, T. E., and Gravelle, J. A. (2015). Forest canopy reduction and snowpack dynamics in a northern Idaho satershed of the continental-maritime region, United States. Forest Sci. 61, 882–894. doi: 10.5849/forsci.14-025
Kane, V. R., Gersonde, R. F., Lutz, J. A., McGaughey, R. J., Bakker, J. D., and Franklin, J. F. (2011). Patch dynamics and the development of structural and spatial heterogeneity in Pacific Northwest forests. Can. J. For. Res. 41, 2276–2291. doi: 10.1139/x11-128
Knight, C. A., Anderson, L., Bunting, M. J., Champagne, M., Clayburn, R. M., Crawford, J. N., et al. (2022). Land management explains major trends in forest structure and composition over the last millennium in California's Klamath Mountains. Proc. Natl. Acad. Sci. 119, e2116264119. doi: 10.1073/pnas.2116264119
Kobayashi, D. (1987). Snow accumulation on a narrow board. Cold Reg. Sci. Technol. 13, 239–245. doi: 10.1016/0165-232X(87)90005-X
Kock, T. J., Perry, R. W., and Hansen, A. C. (2016). Survival of juvenile Chinook salmon and coho salmon in the Roza Dam fish bypass and in downstream reaches of the Yakima River, Washington, (ver. 1.1, April 2017). US Geol. Surv. Open-File Report 1210, 32. doi: 10.3133/ofr20161210
Krogh, S. A., Broxton, P. D., Manley, P. N., and Harpold, A. A. (2020). Using process based snow modeling and lidar to predict the effects of forest thinning on the northern sierra nevada snowpack. Front. Forests Global Change 3, 21. doi: 10.3389/ffgc.2020.00021
Krosby, M., Theobald, D. M., Norheim, R., and McRae, B. H. (2018). Identifying riparian climate corridors to inform climate adaptation planning. PLoS ONE 13, e0205156. doi: 10.1371/journal.pone.0205156
LaMalfa, E. M., and Ryle, R. (2008). Differential snowpack accumulation and water dynamics in aspen and conifer communities: implications for water yield and ecosystem function. Ecosystems 11, 569–581. doi: 10.1007/s10021-008-9143-2
Lawler, R. R., and Link, T. E. (2011). Quantification of incoming all-wave radiation in discontinuous forest canopies with application to snowmelt prediction. Hydrol. Processes 25, 3322–3331. doi: 10.1002/hyp.8150
Leach, J. A., and Moore, R. D. (2014). Winter stream temperature in the rain-on-snow zone of the Pacific Northwest: influences of hillslope runoff and transient snow cover. Hydrol. Earth Syst. Scie. 18, 819–838. doi: 10.5194/hess-18-819-2014
Lee, S. Y., Fullerton, A. H., Sun, N., and Torgersen, C. E. (2020). Projecting spatiotemporally explicit effects of climate change on stream temperature: a model comparison and implications for coldwater fishes. J. Hydrol. 588, 125066. doi: 10.1016/j.jhydrol.2020.125066
Li, D., Wrzesien, M. L., Durand, M., Adam, J., and Lettenmaier, D. P. (2017). How much runoff originates as snow in the western United States, and how will that change in the future? Geophys. Res. Lett. 44, 6163–6172. doi: 10.1002/2017GL073551
Link, T. E., Marks, D., and Hardy, J. P. (2004). A deterministic method to characterize canopy radiative transfer properties. Hydrol. Proc. 18, 3583–3594. doi: 10.1002/hyp.5793
López-Moreno, J. I., Fassnacht, S. R., Heath, J. T., Musselman, K. N., Revuelto, J., Latron, J., et al. (2013). Small scale spatial variability of snow density and depth over complex alpine terrain: implications for estimating snow water equivalent. Adv. Water Res. 55, 40–52. doi: 10.1016/j.advwatres.2012.08.010
Lumbrazo, C., Bennett, A., Currier, W. R., Nijssen, B., and Lundquist, J. (2022). Evaluating multiple canopy-snow unloading parameterizations in SUMMA with time-lapse photography characterized by citizen scientists. Water Res. Res. 58, e2021WR. doi: 10.1029/2021WR030852
Lundquist, J. D., Dettinger, M. D., and Cayan, D. R. (2005). Snow-fed streamflow timing at different basin scales: case study of the Tuolumne River above Hetch Hetchy, Yosemite, California. Wat. Res. Res. 41, W07005. doi: 10.1029/2004WR003933
Lundquist, J. D., Dickerson-Lange, S., Gutmann, E., Jonas, T., Lumbrazo, C., and Reynolds, D. (2021). Snow interception modelling: Isolated observations have led to many land surface models lacking appropriate temperature sensitivities. Hydrol. Proc. 35, 274. doi: 10.1002/hyp.14274
Lundquist, J. D., Dickerson-Lange, S. E., Lutz, J. A., and Cristea, N. C. (2013). Lower forest density enhances snow retention in regions with warmer winters: a global framework developed from plot-scale observations and modeling. Wat. Res. Res. 49, 6356–6370. doi: 10.1002/wrcr.20504
Lundquist, J. D., and Flint, A. L. (2006). Onset of snowmelt and streamflow in 2004 in the western United States: how shading may affect spring streamflow timing in a warmer world. J. Hydrometeorol. 7, 1199–1217. doi: 10.1175/JHM539.1
Lundquist, J. D., and Huggett, B. (2010). Evergreen trees as inexpensive radiation shields for temperature sensors. Water Res. Res. 46, W00D. doi: 10.1029/2008WR006979
Lundquist, J. D., and Lott, F. (2008). Using inexpensive temperature sensors to monitor the duration and heterogeneity of snow-covered areas. Wat. Res. Res. 44, W00D.16. doi: 10.1029/2008WR007035
Marks, D., Kimball, J., Tingey, D., and Link, T. (1998). The sensitivity of snowmelt processes to climate conditions and forest cover during rain-on-snow: a case study of the 1996 Pacific Northwest flood. Hydrolo. Proc. 12, 1569–1587. doi: 10.1002/(SICI)1099-1085(199808/09)12:10/11andlt;1569::AID-HYP682andgt;3.0.CO;2-L
Martin, K. A., Van Stan, J. T., Dickerson-Lange, S. E., Lutz, J. A., Berman, J. W., Gersonde, R., et al. (2013). Development and testing of a snow interceptometer to quantify canopy water storage and interception processes in the rain/snow transition zone of the North Cascades, Washington, USA. Wat. Res. Res. 49, 3243–3256. doi: 10.1002/wrcr.20271
Mazzotti, G., Currier, W. R., Deems, J. S., Pflug, J. M., Lundquist, J. D., Jonas, T., et al. (2019). Revisiting snow cover variability and canopy structure within forest stands: insights from airborne Lidar Data. Wat. Res. Res. 2019, WR024898. doi: 10.1029/2019WR024898
Mazzotti, G., Webster, C., Quéno, L., Cluzet, B., and Jonas, T. (2022). Canopy structure, topography and weather are equally important drivers of small-scale snow cover dynamics in sub-alpine forests. Hydrol. Earth Syst. Sci. 31, 1–32. doi: 10.5194/hess-2022-273
McCune, B., and Keon, D. (2002). Equations for potential annual direct incident radiation and heat load. J. Veg. Sci. 13, 603–606. doi: 10.1111/j.1654-1103.2002.tb02087.x
Miller, D. H. (1964). Interception processes during snowstorms. In U.S. Forest Service Research Paper PSW – 18 (p. 24). Berkeley, CA: Pacific Southwest Forest and Range Experiment Station.
Mote, P. W., Hamlet, A. F., Clark, M. P., and Lettenmaier, D. P. (2005). Declining mountain snowpack in western North America*. Bullet. Am. Meteorol. Soc. 86, 39–49. doi: 10.1175/BAMS-86-1-39
Mote, P. W., Li, S., Lettenmaier, D. P., Xiao, M., and Engel, R. (2018). (2018). Dramatic declines in snowpack in the western US. NPJ Climate Atmos. Science 1, 1–6. doi: 10.1038/s41612-018-0012-1
Musselman, K. N., Molotch, N. P., and Brooks, P. D. (2008). Effects of vegetation on snow accumulation and ablation in a mid-latitude sub-alpine forest. Hydrol. Proc. 22, 2767–2776. doi: 10.1002/hyp.7050
Musselman, K. N., and Pomeroy, J. W. (2017). Estimation of needleleaf canopy and trunk temperatures and longwave contribution to melting snow. J. Hydrometeorol. 18, 555–572. doi: 10.1175/JHM-D-16-0111.1
Musselman, K. N., Pomeroy, J. W., and Link, T. E. (2015). Variability in shortwave irradiance caused by forest gaps: measurements, modelling, and implications for snow energetics. Agric. Forest Meteorol. 207, 69–82. doi: 10.1016/j.agrformet.2015.03.014
Perry, D. A., Hessburg, P. F., Skinner, C. N., Spies, T. A., Stephens, S. L., Taylor, A. H., et al. (2011). The ecology of mixed severity fire regimes in Washington, Oregon, and Northern California. Forest Ecol. Manage. 262, 703–717. doi: 10.1016/j.foreco.2011.05.004
Pfister, R., and Schneebeli, M. (1999). Snow accumulation on boards of different sizes and shapes. Hydrol. Proc. 13, 2345–2355. doi: 10.1002/(SICI)1099-1085(199910)13:14/153.0.CO;2-N
Pomeroy, J. W., Marks, D., Link, T., Ellis, C., Hardy, J., Rowlands, A., et al. (2009). The impact of coniferous forest temperature on incoming longwave radiation to melting snow. Hydrol. Proc. 23, 2513–2525. doi: 10.1002/hyp.7325
Povak, N. A., Furniss, T. J., Hessburg, P. F., Salter, R. B., Wigmosta, M. S., Duan, Z., et al. (2022). Evaluating basin-scale forest adaptation scenarios: wildfire, streamflow, biomass, and economic recovery synergies and trade-offs. Front. Forests Global Change 5, 805179. doi: 10.3389/ffgc.2022.805179
Prichard, S. J., Hessburg, P. F., Hagmann, R. K., Povak, N. A., Dobrowski, S. Z., Hurteau, M. D., et al. (2021). Adapting western North American forests to climate change and wildfires: 10 common questions. Ecol. Appl. 31, e02433. doi: 10.1002/eap.2433
PRISM Climate Group (2015). Oregon State University, Available online at: https://prism.oregonstate.edu (accessed January 21, 2015).
Raleigh, M. S., Rittger, K., Moore, C. E., Henn, B., Lutz, J. A., Lundquist, J. D., et al. (2013). Ground-based testing of MODIS fractional snow cover in subalpine meadows and forests of the Sierra Nevada. Remote Sens. Environ. 128, 44–57. doi: 10.1016/j.rse.2012.09.016
Roesch, A., Wild, M., Gilgen, H., and Ohmura, A. (2001). A new snow cover fraction parametrization for the ECHAM4 GCM. Clim. Dyn. 17, 933–946. doi: 10.1007/s003820100153
Roth, T. R., and Nolin, A. W. (2017). Forest impacts on snow accumulation and ablation across an elevation gradient in a temperate montane environment. Hydrol. Earth Syst. Sci. 21, 5427–5442. doi: 10.5194/hess-21-5427-2017
Roth, T. R., and Nolin, A. W. (2019). Characterizing maritime snow canopy interception in forested mountains. Wat. Res. Res. 55, 2018. doi: 10.1029/2018WR024089
Rutter, N., Essery, R., Pomeroy, J., Altimir, N., Andreadis, K., Baker, I., et al. (2009). Evaluation of forest snow processes models (SnowMIP2). J. Geophys. Res. 114, 11063. doi: 10.1029/2008JD011063
Saksa, P. C., Conklin, M. H., Battles, J. J., Tague, C. L., and Bales, R. C. (2017). Forest thinning impacts on the water balance of Sierra Nevada mixed-conifer headwater basins. Wat. Res. Res. 53, 5364–5381. doi: 10.1002/2016WR019240
Saksa, P. C., Conklin, M. H., Tague, C. L., and Bales, R. C. (2020). Hydrologic response of sierra nevada mixed-conifer headwater catchments to vegetation treatments and wildfire in a warming climate. Front. Forests Global Change 3. doi: 10.3389/ffgc.2020.539429
Sankey, T., and Tatum, J. (2022). Thinning increases forest resiliency during unprecedented drought. Sci. Rep. 12, 9041. doi: 10.1038/s41598-022-12982-z
Satterlund, D. R., and Haupt, H. F. (1967). Snow catch by Conifer Crowns. Wat. Res. Res. 3, 1035–1039. doi: 10.1029/WR003i004p01035
Schlaepfer, M. A., and Lawler, J. J. (2022). Conserving biodiversity in the face of rapid climate change requires a shift in priorities. WIREs Climate Change 14, e798. doi: 10.1002/wcc.798
Seyednasrollah, B., and Kumar, M. (2014). Net radiation in a snow-covered discontinuous forest gap for a range of gap sizes and topographic configurations. J. Geophys. Res. Atmos. 119, 323–342. doi: 10.1002/2014JD021809
Seyednasrollah, B., and Kumar, M. (2019). How surface radiation on forested snowpack changes across a latitudinal gradient. Hydrology 6, 62. doi: 10.3390/hydrology6030062
Seyednasrollah, B., Kumar, M., and Link, T. E. (2013). On the role of vegetation density on net snow cover radiation at the forest floor. J. Geophys. Res. Atmosp. 118, 8359–8374. doi: 10.1002/jgrd.50575
Seyfried, M., Flerchinger, G., Bryden, S., Link, T., Marks, D., McNamara, J., et al. (2021). Slope and aspect controls on soil climate: field documentation and implications for large-scale simulation of critical zone processes. Vadose Zone J. 20, 158. doi: 10.1002/vzj2.20158
Shidei, T., Takahashi, T., Takahashi, K., and Kataoka, K. (1952). Study of the fallen snow on the forest trees. Bull. Gov. For. Exp. Stn. 54, 115-164.
Stewart, I. T., Cayan, D. R., and Dettinger, M. D. (2005). Changes toward earlier streamflow timing across western North America. J. Climate 18, 1136–1155. doi: 10.1175/JCLI3321.1
Storck, P., Lettenmaier, D. P., and Bolton, S. M. (2002). Measurement of snow interception and canopy effects on snow accumulation and melt in a mountainous maritime climate, Oregon, United States. Wat. Res. Res. 38, 1123. doi: 10.1029/2002WR001281
Strasser, U., Warscher, M., and Liston, G. E. (2011). Modeling snow–canopy processes on an idealized mountain. J. Hydrometeorol. 12, 663–677. doi: 10.1175/2011JHM1344.1
Sturm, M., Taras, B., Liston, G. E., Derksen, C., Jonas, T., Lea, J., et al. (2010). Estimating snow water equivalent using snow depth data and climate classes. J. of Hydrometeorol. 11, 1380–1394. doi: 10.1175/2010JHM1202.1
Sun, N., Wigmosta, M., Zhou, T., Lundquist, J., Dickerson-Lange, S., Cristea, N., et al. (2018). Evaluating the functionality and streamflow impacts of explicitly modelling forest-snow interactions and canopy gaps in a distributed hydrologic model. Hydrol. Processes. 32, 2128–2140. doi: 10.1002/hyp.13150
Sun, N., Yan, H., Wigmosta, M. S., Lundquist, J., Dickerson-Lange, S., Zhou, T., et al. (2022). Forest canopy density effects on snowpack across the climate gradients of the western United States Mountain ranges. Wat. Res. Res. 58, 2020WR029194. doi: 10.1029/2020WR029194
Swanson, R. H. (1980). “Surface wind structure in forest clearings during a chinook,” in 48th Annual Western Snow Conference, Laramie, WY.
Trujillo, E., Ramírez, J. A., and Elder, K. J. (2009). Scaling properties and spatial organization of snow depth fields in sub-alpine forest and alpine tundra. Hydrol. Proc. 23, 1575–1590. doi: 10.1002/hyp.7270
Varhola, A., Coops, N. C., Weiler, M., and Moore, R. D. (2010). Forest canopy effects on snow accumulation and ablation: an integrative review of empirical results. J. Hydrol. 392, 219–233. doi: 10.1016/j.jhydrol.2010.08.009
Veatch, W., Brooks, P., Gustafson, J., and Molotch, N. (2009). Quantifying the effects of forest canopy cover on net snow accumulation at a continental, mid-latitude site. Ecohydrology 2, 115–128. doi: 10.1002/eco.45
Washington State Department of Natural Resources (2018). 20.-Year Forest Health Strategic Plan. Washington, DC: Olympia, WA.
Wayand, N. E., Clark, M. P., and Lundquist, J. D. (2017). Diagnosing snow accumulation errors in a rain-snow transitional environment with snow board observations. Hydrol. Proc. 31, 349–363. doi: 10.1002/hyp.11002
Wayand, N. E., Lundquist, J. D., and Clark, M. P. (2015). Modeling the influence of hypsometry, vegetation, and storm energy on snowmelt contributions to basins during rain-on-snow floods. Water Res. Res. 51, 8551–8569. doi: 10.1002/2014WR016576
Wayand, N. E., Stimberis, J., Zagrodnik, J. P., Mass, C. F., and Lundquist, J. D. (2016). Improving simulations of precipitation phase and snowpack at a site subject to cold air intrusions: Snoqualmie Pass, WA. J. Geophys. Res. Atmos. 121, 9929–9942. doi: 10.1002/2016JD025387
Webster, C., Rutter, N., Zahner, F., and Jonas, T. (2016). Modeling subcanopy incoming longwave radiation to seasonal snow using air and tree trunk temperatures. J. Geophys. Res. Atmos. 121, 1220–1235. doi: 10.1002/2015JD024099
Westerling, A. L. (2006). Warming and earlier spring increase western U.S. forest wildfire activity. Science 313, 940–943. doi: 10.1126/science.1128834
Keywords: snow - vegetation interactions, forest management, snow duration, forest gap, transitional climate zone, Eastern Cascade Range, fire fuel reduction, hydrological resilience
Citation: Dickerson-Lange SE, Howe ER, Patrick K, Gersonde R and Lundquist JD (2023) Forest gap effects on snow storage in the transitional climate of the Eastern Cascade Range, Washington, United States. Front. Water 5:1115264. doi: 10.3389/frwa.2023.1115264
Received: 03 December 2022; Accepted: 06 June 2023;
Published: 20 July 2023.
Edited by:
Ning Sun, Pacific Northwest National Laboratory (DOE), United StatesReviewed by:
Joel Biederman, Agricultural Research Service (USDA), United StatesTimothy Link, University of Idaho, United States
Copyright © 2023 Dickerson-Lange, Howe, Patrick, Gersonde and Lundquist. This is an open-access article distributed under the terms of the Creative Commons Attribution License (CC BY). The use, distribution or reproduction in other forums is permitted, provided the original author(s) and the copyright owner(s) are credited and that the original publication in this journal is cited, in accordance with accepted academic practice. No use, distribution or reproduction is permitted which does not comply with these terms.
*Correspondence: Susan E. Dickerson-Lange, c3VzYW4mI3gwMDA0MDtuYXR1cmFsZGVzLmNvbQ==