- 1Department of Microbiology, Oregon State University, Corvallis, OR, United States
- 2Grupo de Ecología de Sistemas Acuáticos a Escala de Paisaje (GESAP), INIBIOMA-CONICET Universidad Nacional del Comahue, Bariloche, Argentina
- 3Department of Earth and Environmental Sciences, Michigan State University, East Lansing, MI, United States
- 4Department of Microbiology and Molecular Genetics, Michigan State University, East Lansing, MI, United States
- 5Marine and Coastal Research Laboratory, Pacific Northwest National Laboratory, Sequim, WA, United States
- 6School of Oceanography, University of Washington, Seattle, WA, United States
- 7Department of Marine Sciences, University of Georgia, Athens, GA, United States
- 8Environmental Sciences Division, Oak Ridge National Laboratory, Oak Ridge, TN, United States
- 9Ecosystems and Global Change Group, Department of Plant Sciences, University of Cambridge, Cambridge, United Kingdom
- 10Section for Applied Marine Ecology and Modelling, Department of Ecoscience, Aarhus University, Roskilde, Denmark
Inland waters are hotspots for biogeochemical activity, but the environmental and biological factors that govern the transformation of organic matter (OM) flowing through them are still poorly constrained. Here we evaluate data from a crowdsourced sampling campaign led by the Worldwide Hydrobiogeochemistry Observation Network for Dynamic River Systems (WHONDRS) consortium to investigate broad continental-scale trends in OM composition compared to localized events that influence biogeochemical transformations. Samples from two different OM compartments, sediments and surface water, were collected from 97 streams throughout the Northern Hemisphere and analyzed to identify differences in biogeochemical processes involved in OM transformations. By using dimensional reduction techniques, we identified that putative biogeochemical transformations and microbial respiration rates vary across sediment and surface water along river continua independent of latitude (18°N−68°N). In contrast, we reveal small- and large-scale patterns in OM composition related to local (sediment vs. water column) and reach (stream order, latitude) characteristics. These patterns lay the foundation to modeling the linkage between ecological processes and biogeochemical signals. We further showed how spatial, physical, and biogeochemical factors influence the reactivity of the two OM pools in local reaches yet find emergent broad-scale patterns between OM concentrations and stream order. OM processing will likely change as hydrologic flow regimes shift and vertical mixing occurs on different spatial and temporal scales. As our planet continues to warm and the timing and magnitude of surface and subsurface flows shift, understanding changes in OM cycling across hydrologic systems is critical, given the unknown broad-scale responses and consequences for riverine OM.
Introduction
Global rivers and streams export about 0.95 ± 0.15 Pg C yr−1 of inorganic and organic carbon from land to the ocean, linking terrestrial and aquatic systems as an important component of the global carbon budget (Catalán et al., 2016; Regnier et al., 2022). A substantial fraction of this carbon (up to 25%, depending on several factors; Cole et al., 2007; Aufdenkampe et al., 2011) is in the form of dissolved organic matter (DOM), and during the movement from land to the ocean, DOM is modified by a complex array of physical, chemical, and biological processes that can alter their concentrations, composition, and reactivity (Regier et al., 2020). These DOM transformation processes also support high carbon dioxide emissions from inland waters to the atmosphere (Raymond et al., 2013; Ward et al., 2017; Drake et al., 2018) and are therefore critical for understanding how river biogeochemical cycles are altered by anthropogenic climate change.
Biogeochemical transformations of DOM are shaped by its reactivity and transport, which are controlled by biotic and abiotic processes in localized habitats and along a watershed (Stegen et al., 2022). During riverine transport, aromatic DOM compounds are especially susceptible to photochemical modification and degradation, producing carbon dioxide and new inorganic and organic compounds (Stubbins et al., 2010). Biotic degradation of DOM (e.g., bacterial metabolism) can transform part of the pool into new biomass and carbon dioxide, simultaneously producing diverse DOM molecules (Guillemette and del Giorgio, 2012; Cory et al., 2013). Both autochthonous (produced within a system) and allochthonous (produced outside a system) sources of DOM are biologically and photochemically reactive in rivers (Seidel et al., 2016), and interactive effects (e.g., priming) stimulate DOM bioavailability and degradation (Ward et al., 2016).
River DOM chemical composition shapes aquatic ecosystem function (Benner, 2002; Aiken, 2014). Previous studies have characterized the composition of DOM pools at a range of detail spanning from bulk elemental carbon, nitrogen, and phosphorus ratios, using advanced mass spectrometry (i.e., Fourier transform ion cyclotron resonance mass spectrometry, or FT-ICR-MS) techniques (Repeta, 2015; McCallister et al., 2018). In particular, FT-ICR-MS, which can resolve hundreds to thousands of individual molecular formulae from a single sample (McCallister et al., 2018), has been increasingly applied over the past decade to uncover the diversity of molecules present in the DOM pool (Kellerman et al., 2018; Cooper et al., 2020; Holt et al., 2021). This technique has clear limitations as it cannot capture the whole DOM pool as commonly used extraction methods such as solid phase are chemically selective (Chen et al., 2016; Baltar et al., 2021). However, despite these limitations, the FT-ICR-MS technique is a high-throughput, and non-destructive method that has provided valuable results, including sources and processes affecting different DOM molecules (Koch and Dittmar, 2006; Geer Wallace and McCord, 2020), revealing high-resolution pool compositions (Zark and Dittmar, 2018) and providing novel ecological analysis tools (Breitling et al., 2006; Danczak et al., 2020). Among biotic processes, river sediments can be biogeochemical “hot spots” which hold diverse microbial communities potentially capable of processing complex DOM molecules.
Using FT-ICR-MS data to identify putative microbial metabolic activity is possible by calculating the mass difference between metabolite m/z peaks, and comparing to known mass differences for metabolic transformation, known as ab initio prediction of metabolic networks (Breitling et al., 2006). These methods generate new insights by establishing putative biogeochemical transformations (PBT) from which we may draw novel hypotheses on the links between microbial biogeochemistry and metabolomics.
In rivers, spatial variation in DOM composition is related to heterogeneity in the catchments. Spatial patterns can, in some cases, be used to track the transformation or persistence of specific organic molecules (Kellerman et al., 2018); for example, greater DOM chemo-diversity has been observed in low-order streams compared to higher order systems (Mosher et al., 2015). With increasing stream order, the DOM pool appears to progress toward a state of chemostasis, diminishing the variation in concentration and composition of DOM (Creed et al., 2015), which is consistent with principles of the original river continuum concept (Vannote et al., 1980) and emerging theories such as the pulse-shunt concept (Raymond et al., 2016). The original river continuum concept suggests that communities downstream are adapted to use organic matter (OM) not consumed upstream and that DOM is less diverse as stream order increases (Vannote et al., 1980). The pulse shut concept suggests that lower order rivers act as “passive pipes” during high discharge and OM is shunted downstream to higher order rivers where it can be processed (Raymond et al., 2016).
In this study we use the unique large-scale dataset obtained from the framework of the Worldwide Hydrobiogeochemistry Observation Network for Dynamic River Systems (WHONDRS) consortium, to investigate how DOM pools and putative biogeochemical transformations vary across river sediment and surface water environments and how this may influence the reactivity of this pool. Data collected by the WHONDRS consortium was used to test the hypothesis that river characteristics such as stream order, sediment type, and carbon concentrations are primary drivers of DOM composition and reactivity across river gradients. We identified catchment-wide patterns including stream order, latitude, and sample type (sediment versus surface water) that influence the composition of the DOM pool. Specifically, heterogeneous and deterministic selection of protein-class DOM is observed across stream order and sample type, yet emergent patterns of increased stochasticity in surface water compared to the sediment. Furthermore, the addition of lipid-class DOM shifts assemblage toward stochastic as stream order increases, suggesting different DOM processing regimes exist, related to vertical mixing, compound availability, microbial activity, and terrestrial input.
Materials and methods
Dataset description
The dataset was obtained from a North Hemisphere survey of surface river water and sediment samples generated as part of the WHONDRS consortia (Stegen and Goldman, 2018). Briefly, in 2019 a total of 97 sites were surveyed across eight countries within a 6-week period from 29 July to 19 September (Toyoda et al., 2020). All water samples were filtered through 0.22 μm sterivex cartridge filters (EMD Millipore), while 125 ml of surface (1–3 cm) sediment samples were collected using a sterilized stainless-steel scoopula (Garayburu-Caruso et al., 2020; Goldman et al., 2020). Samples were collected following the protocol of the National Ecological Observatory Network (NEON) program (NEON.DOC.001193; Jensen, 2022) and shipped within 24 hours on blue ice packs to the Pacific Northwest National Laboratory's Environmental Molecular Sciences Laboratory, USA. Surface water samples were frozen at −20°C upon arrival and sediments were sieved (<2 mm) and subsampled and stored at −20°C. Before analyses, all samples were thawed in the dark at 4°C for 72 hours. A FT-ICR-MS (12 Tesla Bruker SolariX, resolution was 220 K at 481.185 m/z) was used to collect ultrahigh-resolution mass spectra of both the surface water and sediment samples (Garayburu-Caruso et al., 2020). Biological respiration rates using incubated sediment samples was calculated as the slope of the linear regression between dissolved oxygen (DO) concentration and incubation time (~50–150 mins; Goldman et al., 2020). In addition, surface water samples were measured for dissolved organic carbon (DOC), stable water isotopes [oxygen (O) and hydrogen (H)], specific conductivity, total nitrogen (TN), chloride (Cl−), sulfate (), nitrate (), nitrite (), and fluorine (F−); details for these measurements can be found in Toyoda et al. (2020). Sediment samples were measured for non-purgeable organic carbon as sediment water extractable organic carbon (WEOC), respiration rates, and X-ray fluorescence; details for these measurements can be found in Goldman et al. (2020). More specific information on WHONDRS and methods used can be found in https://whondrs.pnnl.gov.
Statistical analyses
To understand what types of OM are present and how compositions change throughout the WHONDRS watersheds, we constructed van Krevelen plots in R (v. 4.1.2). Pairwise monotonic relationships between DOM and physiochemical features were evaluated by calculating Spearman rank correlation coefficients using the rcorr function in the “Hmisc” package (v. 4.6-0; Harrell Jr, 2021). As respiration rates are generally indicative of microbial activity, we sought to investigate how respiration rates relate to landscape patterns including stream order, estimated percent macrophyte and algal mat coverage, and sediment type (general grain size). Using R (v. 1.1.456) package “ggpubr” (v. 0.4.0; Kassambara, 2020) we conducted non-parametric statistical tests to assess pairwise comparisons (kruskal.test and wilcox.test). The p-values were adjusted using Holm-Bonferroni corrections. To identify significance between the metabolome assemblage and abiotic parameters, we used permutational multivariate analysis of variance (PERMANOVA, reps = 999).
We constructed measurement models, i.e., the link between riverine abiotic variables and their respective latent variables, in R using the package “lavaan” (v. 0.6-12; Rosseel, 2012) to calculate multivariate regressions of predictor and latent variables, as defined by:
Where x = vector of x-side indicators, τx = vector of q intercepts for x-side indicators, Λx = matrix loadings (q x n) corresponding to the latent exogenous variable, ξ = vector of n latent exogenous variables, and δ = vector of residuals for x-side indicators. The latent exogenous variable ξ represented abiotic factors and was defined by the x-side indicators DO, surface water pH, surface water temperature, and stream order.
Endogenous measurement models were constructed to fit riverine biogeochemistry, where the latent endogenous variable remains the same as the above measurement model, except the parameters are now y-side variables;
Where y = vector of y-side indicators, τy = vector of p intercepts for y-side indicators, Λy = matrix loadings (m x q) corresponding to the latent endogenous variables, η = vector of m latent endogenous variables, ϵ = vector of residuals for y-side indicators. The latent endogenous variable η represented biogeochemically active molecules in the sediment or the surface water and was defined by the y-side indicators DOC, WEOC, TN, and .
Measurement models were further analyzed using structural regression modeling with two endogenous variables (surface water and sediment biogeochemistry) and one exogenous variable (abiotic factors), where the endogenous variables may predict each other. Similar to the equations above, we modeled the relationships of the exogenous variable to the endogenous variables, yet the addition of the matrix enables the specification of the relationship between the two endogenous variables.
Where η = vector of m latent endogenous variables, α = vector of p intercepts, γ = vector (1 × q) of regression coefficients where q is the total number of exogenous variables, ξ = vector of n latent exogenous variables, β = matrix of regression coefficients (p × p) of endogenous to endogenous variables whose i-th row indicates the source variable and the j-th column indicates the target variable, and ς = vector of residuals. The latent exogenous variable ξ represented abiotic factors, as defined by the x-side indicators of surface water temperature, DO, and stream order. The first latent endogenous variable η represented surface water biogeochemistry defined by the y-side indicators , TN, and DOC, while the second latent endogenous variable represented sediment biogeochemistry as defined by WEOC and microbial respiration rates.
For all models, fit was assessed using confirmatory factor indices (CFI), Tucker Lewis indices (TLI), and root mean square error approximation (RMSEA). To achieve acceptable values of CFI (>0.95), TLI (>0.90), and RMSEA (<0.05), model fit was altered using modification indices values as predicted by the “lavaan” package (modindices). Once the fit was acceptable by these three metrics, exogenous and endogenous relationships were assessed by averages between the marker method (“lavaan” output Estimate) and the variance standard method (“lavaan” output std.lv), where acceptable variance/covariance ranges between −1 and 1.
To analyze inferred biogeochemical associations and identify core metabolic processes, we inferred putative biogeochemical transformations (PBT) of the WHONDRS FT-ICR-MS dataset, as described by Garayburu-Caruso et al. (2020). The PBT metric is a way to estimate the gain or loss of specific molecules (e.g., amino acids such as valine, and sugars such as glucose) between sample locations/types based on the mass difference between m/z peaks within each sample compared to a reference database of common metabolic transformations (Bailey et al., 2017). While FT-ICR-MS analyses provide mass and elemental stoichiometric information, PBT analyses also include inferred compound structural information. We may from this draw conclusions on links between the structure of organic compounds and their chemically transformed species as defined by their mass differences. The PBT dataset generation was calculated as pairwise mass differences between every peak per sample and compared to known biogeochemical transformations (reference list containing 1,255 associated masses). Emergent patterns in PBT across Northern Hemisphere river corridors were analyzed using Bray-Curtis dissimilarity matrices, constructed and visualized using nonmetric multidimensional scaling (NMDS) plots in R (v. 1.1.456) and the package “vegan” (v. 2.5-6; metaMDS; Oksanen et al., 2020). When ecologically relevant, associated metadata were fitted to the NMDS ordination as environmental vectors (envfit), and p-values of the multiple testing were adjusted using Bonferroni correction (p.adjust). Significance between-location PBT compared to abiotic parameters was determined using permutational multivariate analysis of similarities (PERMANOVA; adonis, permutations = 999) and similarity percentage analysis (SIMPER; simper, permutations = 999).
Further analysis of potential ecological drivers was evaluated using meta-metabolome ecology methods as described by Danczak et al. (2020) and Stegen et al. (2022). Briefly, to quantify deterministic versus stochastic processes responsible for driving putative biochemistry across stream orders and sample type, we applied mean nearest taxon distance (MNTD; ses.mntd; R “picante” package v. 1.8.2; Kembel et al., 2010) and nearest taxon index for sample beta diversity (βNTI; comdistn) to a subset of the FT-ICR-MS dataset (Stegen et al., 2012, 2013; Danczak et al., 2020), specifically analyzing the elemental groups categorized as “protein” and “protein and lipid” with the identified molecular formula and elemental atoms present per metabolite, as identified through molecular formula assignment described in Danczak et al. (2020). Selected protein- and lipid-class compounds were verified to contain both nitrogen and sulfur atoms, to distinguish between potential misclassified lipids. These protein-class compounds were chosen to provide a high-level analysis of biogeochemically active molecules across stream orders and sample type. Given protein-class compound assemblage's ability to be linked to microbial processes and biochemically active metabolites, we may draw preliminary conclusions on amino acid-like transformations (Fudyma et al., 2021). Furthermore, lipids may represent microbial biomass, indicative to the metabolic and structural diversity between samples (Bailey et al., 2017). To calculate βMNTD and βNTI, a dendrogram representative of molecular characteristics of FT-ICR-MS masses of protein class compounds was created. The molecular class dendrogram (MCD) is generated by comparing derived statistics such as double-bond equivalents, modified aromaticity index, and elemental composition, to the identified molecular formulae from the FT-ICR-MS dataset. The UPGMA hierarchical clustering analysis on the molecular characteristic differences matrix then generates the MCD.
Using the MCD, we calculated β diversity through null modeling and calculating βNTI. Briefly, βNTI is the observed distribution of pairwise phylogenetic distances compared to a null model, calculated by βMNTD. The βMNTD quantified the phylogenetic distance between two mass differences in different communities,
Where fik = the relative abundance of the mass difference i in sample k, nk = is the number of mass differences in sample k, min(Δikjm) is the calculated minimum distance between mass difference i in sample k and all mass distances j in sample m. A mass difference null distribution of βMNTDobs is calculated by randomizing mass differences across the sample and recalculated 999 times to find βMNTDnull. While βNTI is the number of standard deviations between βMNTDobs and βMNTDnull,
where βMNTDsd = the standard deviation of βMNTDnull values.
To assess metabolome assemblage processes (deterministic or stochastic) across watersheds, pairwise comparisons between βMNTD values are used to calculate the βNTI of metabolome diversity. The βNTI is the observed deviation of a null βMNTD model where −2 > βNTI values > +2 values represent a metabolome experiencing deterministic selection (> +2 signifies variable selective pressures while < −2 is homogenizing selective pressures) and |βNTI| values < +2 represents a stochastically assembled metabolome. All βNTI values were then compared to abiotic factors using multiple linear regression (“lavaan”). By identifying the underlying environmental processes driving putative biochemistry, we can further link ecological systems to their molecular processes. As described by Danczak et al. (2020), a deterministic assemblage is constructed by any factors that alter the production and degradation rates of molecules, persistent low oxidation states in anaerobic environments, or ongoing degradation. Conversely, dispersal patterns and mass effects govern metabolomes through stochastic assemblage, akin to a lack of temporal or spatial variation where unstructured compositional deviations occur in metabolite assemblages. Furthermore, pairwise metabolome assemblage that were not governed by deterministic processes (|βNTI| < +2) can be further classified as the stochastic processes drift, dispersal limitation and drift, or homogenizing dispersal by calculating Bray-Curtis based Raup-Crick indices (RCBray) as developed by Stegen et al. (2013). The RCBray extends the Raup-Crick dissimilarity metric to include relative abundance of each metabolite as opposed to presence/absence by adding Bray-Curtis dissimilarities to quantify compositional turnover during each iteration (permutations = 999) when constructing null models. Probabilistically assembled metabolomes can then be interpreted based on an individual metabolite's presence and its relative abundance across each sample, where the standard deviation between Bray-Curtis values and the null distribution may range between −1 and +1. Here RCBray values > +0.95 or < −0.95 are considered significant departures from the expected degree of metabolite turnover related to ecological drift, where |RCBray| values > +0.95 indicates drift acting alone. Conversely, RCBray values < −0.95 indicate homogenizing dispersal (high dispersal rates have a larger impact than selective pressures) and RCBray values > +0.95 indicates combined pressures of dispersal limitation and drift (Doherty et al., 2020).
Results
Delineation of watershed-wide characteristics
Previous research on the WHONDRS dataset by Garayburu-Caruso et al. (2020) calculated descriptive metrics pertaining to the metabolome (Supplementary Table 1), finding the surface water metabolome has increased unsaturation, aromaticity, oxidation, and variation when compared to the sediment, and that sediment metabolomes are more spatially variable. To analyze the DOM compositional data, we visualized and characterized the assigned molecular formulas using van Krevelen plots (Supplementary Figure 1). When coupled by stream order ranks, the H:C and O:C atomic ratios revealed overlap between surface water and sediment samples (Supplementary Figure 1). The van Krevelen plots reveal no unique molecular formula pattern with stream order, although in low order streams we observed a wider distribution of formulae in sediment samples while having more peaks observed (3,953) when compared to the surface water (3,653). In surface water samples, Kendrick mass defect (KMD), Kendrick Mass, double bond equivalent (DBE), , and were strongly positively correlated (rho > 0.9, p-value < 0.05). In contrast, strong negative correlations (rho < −0.9, p-value < 0.05) appeared among variables such as nominal oxidation state carbon (NOSC) and Gibbs Free Energy (GFE), as GFE is calculated from NOSC (Supplementary Figure 2A). In the case of sediment samples, strong positive correlations (rho > 0.9) between aromaticity (AI) and NOSC were found, meanwhile negative correlations (rho > −0.9) similar to the surface water samples were found between aromaticity index (AI mod), NOSC, and GFE (Supplementary Figure 2B).
Patterns of peak diversity between surface water and sediment sample types were significant when compared to all available metadata [Bray-Curtis PERMANOVA p-values = 0.001 (, stream order, , TN, NPOC, pH, water temperature, DO), 0.002 (F−), and 0.03 (Cl−); Supplementary Figure 3], reflecting the biogeochemical complexity between abiotic factors and metabolome structure. Additionally, the PBT metabolome structure was also significant to all abiotic variables (Bray-Curtis PERMANOVA p-values = 0.001 (stream order, NPOC, , TN, pH, water temperature), 0.002 (), and 0.04 (general grain size, rate of respiration, DO); Supplementary Figure 3), save Cl− (p-value = 0.128). Comparatively, the PBT dataset was less significant overall with lower F-statistics (1.4–9.7) when compared to the original FT-ICR-MS data (2.0–13.6; Supplementary Figure 3).
Biogeochemical parameters link to stream characteristics
To assess abiotic factors contributing to varying sediment respiration rates, we explored the influence of stream characteristics, stream order, algal mat coverage, and sediment type. Across low to mid-order streams, respiration rates increased (stream order 1 average: 6.75 mg L−1 h−1 to stream order 5 average: 21.6 mg L−1 h−1), but were lowest in large order streams (stream orders 6 through 8 average: 4.53 mg L−1 h−1), suggesting that stream order plays an important, but non-linear role in watershed respiration dynamics. Mid-stream orders (3–5) and stream order 7 were significantly different when compared to all other stream orders, likely due to higher respiration rates in mid-stream orders, and low rates in high stream orders (Kruskal-Wallis, p-value < 0.001; Figure 1A). We observed that higher algal mat and macrophyte coverage are linked to significantly higher respiration rates (Kruskal-Wallis p-value = 0.018; Kruskal-Wallis p-value = 0.01; Figure 1B). Likewise, we found that stream bed sediment particle size was also a significant factor influencing respiration rates (Kruskal-Wallis p-value < 0.001; Figure 1C), which increased as particle sizes decreased (i.e., lowest for gravel and highest for silt).
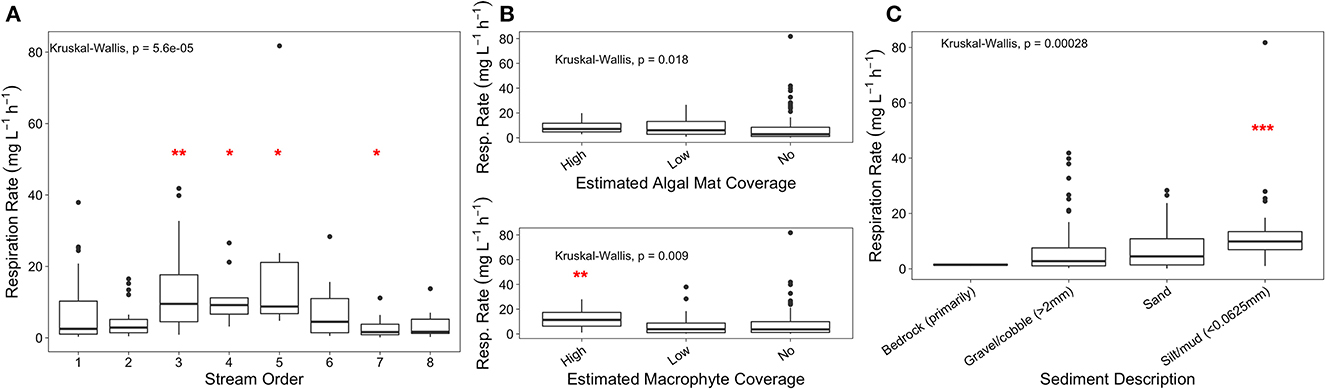
Figure 1. Respiration rates of sediment samples ordered by (A) stream order, (B) algal mat and macrophyte coverage, and (C) sediment type. Wilcoxon pairwise comparison significance denoted by red asterisks.
We calculated measurement models of exogenous and endogenous latent variables to show the relationships between abiotic and biotic factors across all watersheds sampled. These models were separated into exogeneous abiotic latent variables (“Abiotic”) and endogenous biogeochemical latent variables (“Water Biogeochemistry” and “Sediment Biogeochemistry”). The exogenous model, “Abiotic,” represents measured abiotic parameters across all sampled watersheds (df = 6, CFI = 1, TLI = 1, RMSEA = 0). This model showed significant variance between the latent variable and stream order, surface water temperature, and pH (standardized variance = 0.51, 0.61, and 0.3, respectively; Supplementary Figure 4A), where significant covariance occurred between pH and DO (standardized variance = 0.32). The first endogenous model, “Water Biogeochemistry” represents potentially biogeochemically active molecules across all sampled watersheds (df = 6, CFI = 1, TLI = 1, RMSEA = 0). We found significant variance between the latent variable and all endogenous indicators (standardized variance = 0.22–0.94), and covariance between endogenous indicators NPOC and yielded a significant variance of 0.25 (Supplementary Figure 4B). Finally, the second exogenous model “Sediment Biogeochemistry” represents sediment biogeochemistry modeled with abiotic variables and respiration rates (df = 9, CFI = 0.99, TLI = 0.97, RMSEA = 0.05). Significant variance was found only with WEOC concentrations (0.19) while all other covariances were significant (values ranging from 0.34 to 0.53; Supplementary Figure 4C).
Using the measurement models, we resolved the three latent variables into a single structural regression which revealed relationships between ecosystem parameters and biogeochemical activity (df = 11, RMSEA = 0.027, CFI = 0.993, and TLI = 0.982; Figure 2). Several significant variances were found, specifically between the latent variable “Water” and TN (standardized variance = 0.072, p-value = 0.05) and DOC (standardized variance = 0.260, p-value = 0.003). The latent variable “Sed” significantly varied between respiration rates (standardized variance = 0.637, p-value = 0.008), yet the latent variable “Abiotic” showed no significant variance between its defined x-side indicators. Significant covariance occurred between surface water temperature and sediment WEOC (standardized variance = −0.169, p-value < 0.001), and DOC (standardized variance = 0.269, p-value = 0.03), DO and respiration rate (standardized variance = 0.223, p-value < 0.001), and DO and DOC (standardized variance = −0.577, p-value < 0.001). Negative standardized variance represents an inverse relationship between the exogenous and endogenous variables (e.g., an increase in surface water temperature will likely result in a decrease of sediment WEOC).
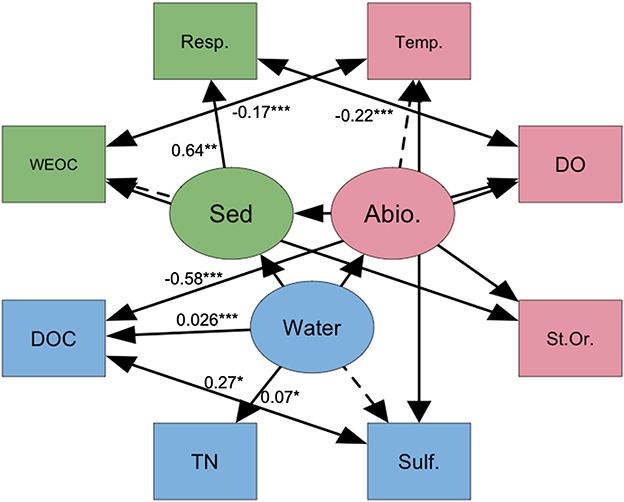
Figure 2. Structural regression model of latent variables “Abiotic” (abiotic lotic variables), “Sed” (sediment biogeochemistry) and “Water” (surface water biogeochemistry) respectively. Values represent significant (p-value < 0.05) variance (single sided arrow) or covariance (double sided arrow). Rectangles represent indicator variables while circles represent latent variables. Dashed lines represent fixed loadings. Resp., microbial respiration rate; Temp., surface water temperature; DO, dissolved oxygen; St.Or., stream order; sulf., ; TN, total N; DOC, dissolved organic carbon; WEOC, water-extractable organic carbon. The asterisks *** indicates the significance levels of given [co]variance within the model.
PBT shaped by stream order and DOC
Patterns of PBT diversity were visualized using NMDS plots of Bray-Curtis dissimilarity (Figure 3). Sediment PBT are significantly different from surface water PBT (PERMANOVA p-value < 0.001), yet there are a few surface water samples grouped with the sediment (with differences in DOC concentrations likely driving the observed difference between sediment and surface water samples; Figure 3A; PERMANOVA p-value < 0.001), as sediment samples contain higher WEOC concentrations on average (sediment = 14.7 ± 8.7 mg L−1, surface water = 2.8 ± 3.7 mg L−1). Likewise, stream order plays a significant role in the sample dissimilarity (PERMANOVA p-value < 0.001). Conversely, latitude and longitude are not significant in driving PBT dissimilarities (PERMANOVA p-value = 0.74, 0.35, respectively), and no variables measured in this study account for the observed spread of surface water samples (Figure 3A).
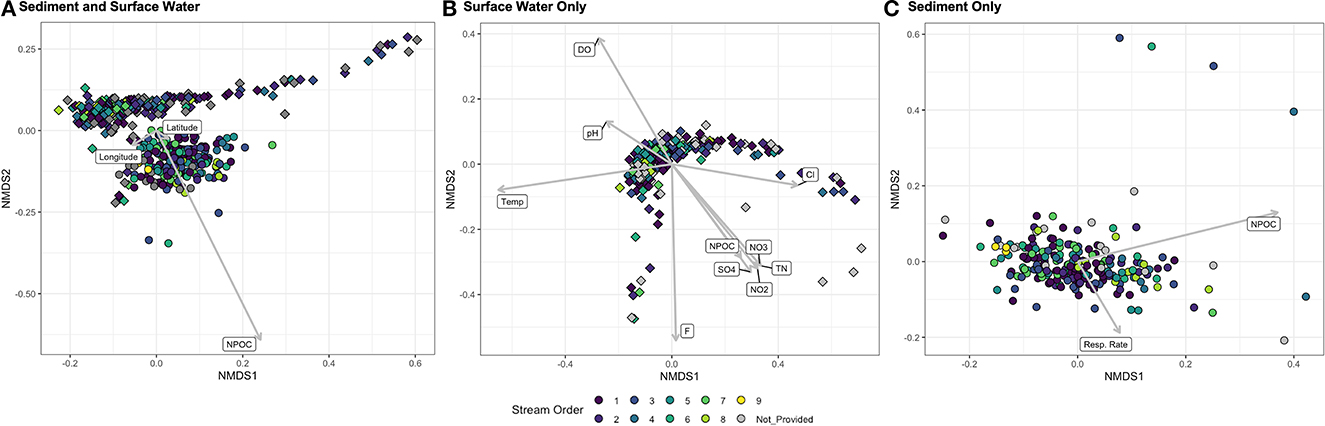
Figure 3. Non-metric multidimensional scaling plots (NMDS) based on Bray-Curtis dissimilarities between putative biogeochemical transformations across (A) all sediment (circle) and surface water (diamond) sample (stress = 0.04), (B) surface water samples only (stress = 0.03), and (C) sediment sample only (stress = 0.05). All plots are colored on the basis of stream order (1–9).
In an independent NMDS analysis of surface water samples and relevant metadata, we found that stream order significantly influenced the compound dissimilarities observed between all surface water samples (PERMANOVA p-value < 0.001; Figure 3B). Temperature, DO, pH, DOC, , , , F−, and Cl− were all insignificant environmental vectors (NMDS vector p-value range from 0.14 to 0.77) yet retain explanatory and suggestive significance for emergent dissimilarity patterns (Figure 3B), and are significant in PERMANOVA geometric partitioning of variation (Supplementary Figure 3). Additionally, when assessing the sediment samples and relevant metadata only, we found that WEOC concentrations (NMDS vector p-value < 0.001) and sediment respiration rates (NMDS vector p-value = 0.05) significantly contribute to the dissimilarity across samples (Figure 3C). Sediment PBT composition differs across stream order and reported sediment type (PERMANOVA p-value < 0.001), while neither latitude (PERMANOVA p-value = 0.5) nor longitude (PERMANOVA p-value = 0.1) were significant in explaining the distribution of dissimilarities. Outliers are likely influenced by differences in DOC concentrations (NMDS vector p-value < 0.001). The PBT molecule explaining the highest degrees of dissimilarity between sediment and surface water was C35H56 (tetraprenyl-beta-curcumene), a triterpene (SIMPER p-value = 0.019, cumulative contribution to overall dissimilarity = 0.2%).
In order to assess the relationships between PBT and stream order ranks, samples were grouped by stream order in additional NMDS analyses (Supplementary Figures 5A–C). Patterns in dissimilarity were similar across all groups, being that DOC concentrations were a significant environmental vector in every case (p-value < 0.001) and sample types remained a determining factor in sample dispersion (PERMANOVA p-value < 0.001). As expected, stream order was not significant in any grouping (0.6 < p-value < 0.12).
Spatial drivers of riverine metabolome assemblage
Using Mantel tests based on Spearman's Correlation, we sought to understand to what extent the environment is “selecting” for specific PBT assemblage across all watersheds. Across both surface water and sediment samples, DOC concentrations (Mantel Statistic R = 0.15, p-value < 0.001, Mantel Statistic R = 0.08, p-value = 0.02, respectively) and geographic separation as Haversine distance (Mantel Statistic R = 0.16, p-value < 0.001, Mantel Statistic R = 0.14, p-value < 0.001, respectively) both had a significant relationship with the PBT assemblage. However, in sediment PBT assemblages, respiration rates and DO concentrations were not significantly correlated (R = −0.01, p-value = 0.6; R = 0.04, p-value = 0.19, respectively). Likewise, cumulative environmental factors (TN, Cl−, , , , and F−) had no relationship with surface water PBT (Mantel Statistic R = −0.03, p-value = 0.7). These correlations support a connection between environmental variables and PBT assembly, which are further dissected using null modeling to understand the relative influences of deterministic versus stochastic influences.
Molecular characteristics dendrogram (MCD) of the FT-ICR-MS peaks associated with protein-class compounds revealed surface water and sediment assemblies across stream orders 1 and 2 all exhibited mean βNTI average values of 2.7 in surface water and 3.8 in sediment samples (Figure 4A). This indicates that deterministic processes govern these protein-class compound assemblages. Heterogeneous selection dominated across surface water (50.4% of comparisons) and sediment (60.2% of comparisons) samples in low-order streams. Surface water protein-class compound assemblages show a secondary influence of stochastic forces (~22.7% of all comparisons), while the sediment assemblage had a lower influence (~38.7% of comparisons). Homogenizing selection was in 26.9% in the surface water assemblages and was consistently low in sediment assemblages (1.1%; Figure 4A). Multiple linear regression with low stream order βNTI values correlated significantly with both WEOC (standardized variance = 0.023, p-value < 0.001) and respiration rates (standardized variance = −0.006, p-value = 0.01; Supplementary Figure 6C).
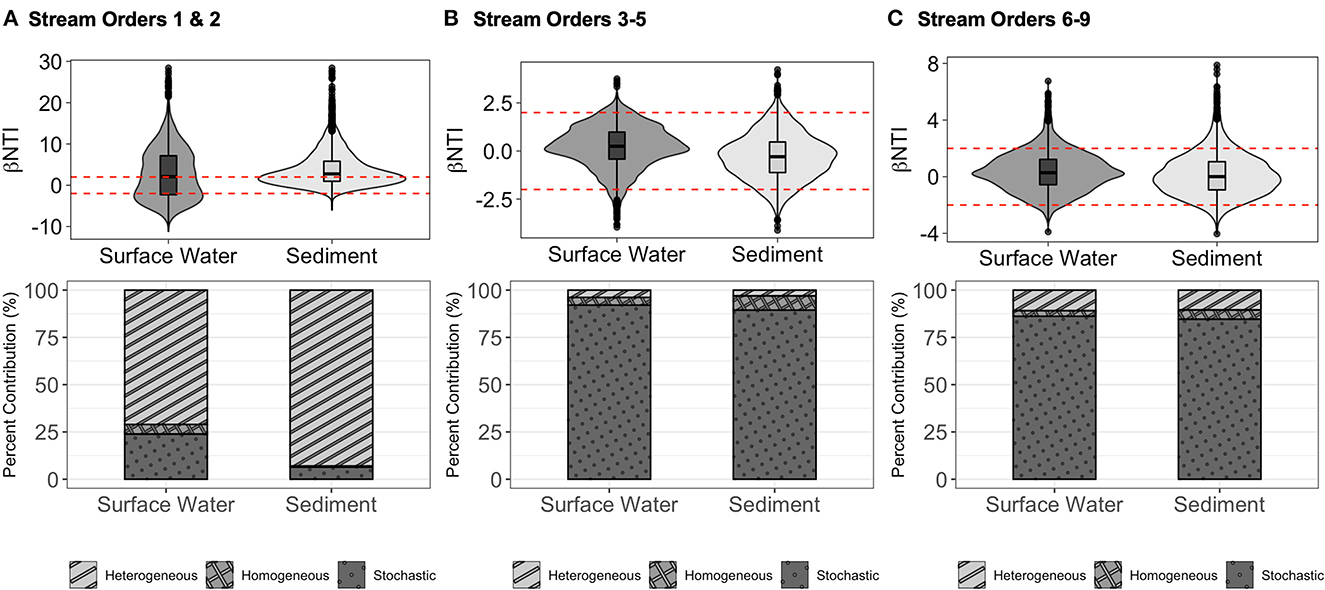
Figure 4. β-Nearest Taxon Index (βNTI) and percent contribution of ecological processes of protein-class compounds sans lipids in sediment and surface water samples of stream orders 1 and 2 (A), 3–5 (B) and 6–9 (C); note the difference in y-axis scales. Dashed red lines indicate βNTI values > +2 (deterministic, heterogeneous assemblage) and < −2 (deterministic, homogeneous assemblage). |βNTI| values < +2 represent stochastic assemblage. Barcharts below represent the percent contribution of a given assemblage process to each sample.
Protein-class compounds in mid-order streams (3, 4, and 5) are likely assembled by stochastic patterns (surface water and sediment mean βNTI values were 0.2 and −0.3, respectively). In 89.4–92.7% of all comparisons in the surface water and sediment these were stochastic, with minimal secondary influences by homogeneous selection (surface water = 4.7%, sediment = 7.5%) and tertiary influences by heterogeneous selection (surface water = 3.9%, sediment = 3.7%; Figure 4B). Surface water and sediment protein-class compound assemblages in high order streams (6, 7, 8, and 9) exhibited very strongly stochastic patterns (βNTI values averaging 0.4 in surface water and 0.1 in sediment samples; Figure 4C), where heterogeneous selection of protein class compounds constitute 10.9% in surface water and 10.5% in sediment in high order streams. To a lesser extent, homogeneous selection is responsible for <5% of comparisons in surface water protein-class compound assemblages. Stochastic forces contributed to 84.6–86.7% of compound selection (Figure 4C). High stream order βNTI values compared to respiration showed a significant inverse relationship (standardized variance = −0.094, p-value = 0.003), while βNTI and WEOC concentrations were not significantly related (standardized variance = 0.006, p-value = 0.7; Supplementary Figure 6D). The WEOC concentrations and respiration rates shared a significant covariance within these models (standardized variance = 0.96, p-value < 0.001). When compared to DOC and DO, βNTI significantly and inversely correlated with DO concentrations (standardized variance = −0.96, p-value < 0.001), while it was not significantly related with DOC (standardized variance = 0.006, p-value = 0.7; Supplementary Figure 6D). The RCBray analyses of the stochastic assemblages revealed that drift (|RCBray | < +0.95) governed mid order (average surface water = −0.2, average sediment = −0.3) and high order (average surface water = −0.1, average sediment = −0.2) streams.
Conversely, protein- and lipid-class compounds revealed deterministic assemblages across low (surface water = 5.2, sediment = 5.9) and high (surface water = 8.7, sediment = 11.0) stream orders (Supplementary Figures 6A, B). Heterogeneous selection comprised 57–63% of comparisons in low stream order surface water, and 72–76% in sediments. Similarly, high stream orders had very strongly heterogeneous selection (>80%) in the surface water and sediment. Stochastic and homogeneous selection were both limited in all comparisons (≤27%; Supplementary Figure 6).
Discussion
DOM heterogeneity is shaped by local ecological patterns
Inland water ecosystems significantly contribute to the global carbon cycle by exporting, transforming, and sequestering carbon (Butman et al., 2016), with respiration contributing notably to carbon emissions (Hotchkiss et al., 2015). In particular, low order streams with relatively short water residence time shunt labile OM to higher order streams along the hydrologic network (Vannote et al., 1980; Raymond et al., 2016). When compared to higher (6–9) stream orders, we found that low order streams (1–5) generally have higher and more variable respiration rates (average range from 4.85 to 21.6 mg L−1 h−1). The source of this variability likely relates to several factors including climate, land use, anthropogenic influence, residence time, longitudinal slope, watershed area, and river discharge. Latitude and longitude did not significantly control PBT compositions, yet when assessing the original molecular compounds (without putative transformations), mid-latitude rivers (30°N−50°N) showed a broader variation of hydrogenation, perhaps due to pyrogenic carbon that has been oxidized and became more soluble (Kim et al., 2004).
The obtained results did not show a clear pattern in OM molecular formulae, despite variation in the catchments across all stream orders (Supplementary Figure 1). A possible explanation is that the samples were collected once with high heterogeneity, potentially explained by factors such as the pulse-shunt concept, in which terrestrial DOM is exported downstream. Furthermore, the DOM heterogeneity observed in low-order streams may be linked to differences in residence times of DOM or sediment particle size. However, higher respiration rates are generally found in systems with greater primary production which likely provide labile sources of autochthonous OM to the sediments (Ellis et al., 2012; Ward et al., 2016, 2018), an observation consistent with higher algal mat coverage in this study. Interestingly, the main identified difference in surface water versus sediment PBT is differential triterpene abundances, which serve as steroid precursors and are produced by macrophytes and eukaryotic microorganisms actively performing primary metabolism. This relationship likely reflects higher activity by algae and macrophytes in the surface water compared to the sediment (Ghosh, 2017; Santana-Molina et al., 2020). This finding is further supported by the observed relationship between respiration rates, WEOC concentrations, and surface water pH, with an increase in available DOM being significantly linked to circumneutral pH (6.7) and increased sediment respiration rates. We therefore hypothesize that this observed correlation is linked to a higher abundance of autotrophs due to the availability of solar radiation (Tanabe et al., 2019; Savoy and Harvey, 2021), which may also contribute to photodegradation of DOM and link abiotic and biotic processing.
Water biogeochemistry of river corridors in this study was driven primarily by DOC given the general role of DOC in redox reactions as a nutrient and micronutrient vector, and as a source of energy for microbial communities (Figure 2). Though nutrients such as TN were important in vegetation-rich climates in shaping water biogeochemistry, the main driver was DOC and its links to and DO. This finding supports the relationships between DOC (electron source) and electron acceptors ( and DO). We observed that DOC had an inverse relationship with DO, implying rates of DOC consumption were increased with DO, while rates decreased with as the electron acceptor. In contrast to the river water, sediment biogeochemistry showed that DO negatively impacts respiration rates, suggesting the sediments are primarily anaerobic. Therefore, DOC is likely processed within the sediments at varying rates depending on DO availability, shaping the DOM pool through heterogeneous deterministic selection. Additionally, the sediment WEOC concentrations were inversely related to surface water temperature, suggesting a link between climate and OM concentrations, likely originating from allochthonous inputs (e.g., macrophytes). Thus, the contribution of OM as a driver of biogeochemical patterns varies depending on the environment.
Putative biogeochemical transformations across watersheds
Stream order was a key driver of PBT composition in both the surface water and sediment. Terrestrial linkage to watershed functionality is apparent in low stream orders as lateral inputs of DOM from terrigenous sources affect the structure and function of aquatic ecosystems (Buffam et al., 2021; Estévez et al., 2021). Additionally, DO correlated with stream order (Diamond et al., 2021), creating a dynamic system of environmental drivers of respiration rates and PBT (Figure 4). However, despite the explanatory power of stream order, there are no overarching characteristics across all watersheds to fully explain the drivers of the PBT composition.
Surface water biogeochemistry
The separation of surface water and sediment DOC compositions has been observed previously (Garayburu-Caruso et al., 2020), while we in this study also found significant differences in the PBT of surface water samples (when visualized using NMDS dimensional reduction) and a clear coupling of the biological processes on surface water PBT and DOC, , , and . As expected, all these biologically active data are inversely related to DO and pH, evidenced by opposite-facing vectors (Figure 3A). This relationship is likely described by microbial thermodynamics as and , are alternative electron acceptors in the absence of DO. Surface water PBT profiles are related to DO and pH variations (Figure 3), implying biological processing occurring in toxic conditions, yielding more available energy and occurring at an increased rate compared to anoxic conditions (Bethke et al., 2011). The majority of surface water samples had DO levels (average 7.3 mg L−1) above hypoxic levels (<2–3 mg L−1) and likely the coupling of DOC and alternative electron acceptors do not have a direct effect on the observed PBT, which supports our hypothesis that oxygen is the primary electron acceptor in the water column. Conversely, in samples with lower DO (<5 mg L−1) DOC was partially processed using alternative metabolisms (e.g., reduction; Roden and Jin, 2011), leaving a diversity of metabolites available to move downstream to higher order ecosystems (Vannote et al., 1980).
The identified relationships between surface water PBT and DO, DOC, and alternative electron acceptors hints at a disconnect between DOC and microbial access likely due to spatial isolation (Bailey et al., 2017). For example, particle-associated microbes may have greater access to DOC suspended in the water column compared to free-living microbes, which rely on hydrologic flow and chemotaxis to access substrate (Liu et al., 2020). Rivers with greater suspended sediment loads may therefore reveal closer correlations between microbial processing (high turnover of PBT) and increased DOC concentrations (Crump et al., 1998; Kieft et al., 2020). Similarly, studies in the lower Amazon river revealed that surface water respiration rates increased with river flow velocity, likely due to interactions among particle-bound communities and dissolved substrates (Ward et al., 2018, 2019). A lack of access to DOC would be reflected in the PBT assemblage as shaped by stochastic forces (Danczak et al., 2020), and are explored in detail in the following sections.
Anions associated with mineral weathering likely influenced surface water PBT composition. In particular, F− concentrations were related to high levels of PBT in several surface water samples. These samples originated from locations in Western (Colorado) and Eastern (Massachusetts to Alabama, excluding Florida) North America and approximately overlay with igneous bedrock, which is a known source of F− (Berger et al., 2016; The North America Tapestry of Time and Terrain, 2022). There are no clear patterns of F− concentrations in these samples (0.03–0.19 mg L−1), yet all these samples were characterized by gravel/cobble (>2 mm) sediments which may speak to the balance tradeoffs between residence time in the hyporheic zone, available DO, and increased activity with particle associated microbial assemblages. It is likely that stream beds with coarser particles have increased hyporheic flux and lower mean residence time (Boano et al., 2014), both resulting from the higher hydraulic conductivity of the coarser particles relative to finer, silty material. Compound assemblages might then be more deterministic as water interacts with the diverse particle-bound assemblage and the shorter residence time, preventing the water from becoming hypoxic or anoxic. However, when the βNTI values of molecular characteristics of protein-class compounds were compared by grain size, there was no significant difference in the mean pairwise distances (Supplementary Figure 7). These results illustrate the complexity of lotic systems and that there is no single driver, rather the result of the balance of fluxes, residence times, and reaction rates among all interacting components.
Increasing Cl− concentrations may be indicative of groundwater flow and solute flushing into the water column as concentration-discharge relationships are altered (Leibundgut and Seibert, 2011; Winnick et al., 2017), yet as these samples are discrete, Cl− more generally reflects ultramafic rock weathering, proximity to brackish or saline environments or the anthropogenic influences, due to residual road salt (Kuroda and Sandell, 1953; Graedel and Keene, 1996; Corsi et al., 2015; Kupka et al., 2021). Interestingly, high Cl− concentrations (295 mg L−1 to 3964 mg L−1) in brackish samples near Chesapeake Bay and the Everglades, USA did not exhibit PBT compositions correlated to Cl−, rather samples low in Cl− (0.2 mg L−1 to 3.3 mg L−1) were correlated. However, in this study the PBT of only a handful of samples low in Cl− correlated directly with measured concentrations, suggesting there are additional environmental factors which have a larger impact on shaping riverine PBT, such as climate, evaporation, and residence times.
Sediment biogeochemistry
Regardless of seasonal hyporheic flow and groundwater discharge, permanently saturated sediments are continuously influenced by solute flushing and hyporheic mixing. During upwelling events—as seen during low flow events—hyporheic zones oxygen availability is limited, driving the chemical and microbial community toward a more reduced environment which likely has a major influence on the carbon processing rate and products (Danczak et al., 2016; Saup et al., 2019; Buser-Young et al., 2021). Sediment PBT were not strongly correlated to surface water DO concentrations or the sediment respiration rates, suggesting groundwater is dominating leading to a reduced environment (Nelson et al., 2019). In addition, it is important to note that sediment respiration rates do not necessarily only represent biotic processes, but abiotic mineral-associated redox processes also consume oxygen, potentially confounding relationships between microorganisms and DOC. To that end, the high levels of aerobic respiration measured in vitro suggest the sediment microbiome are stimulated by mixing events, such as hyporheic upwelling (low-flow, anoxic) and downwelling (high-flow, oxic) as also found previously (De Falco et al., 2018). Our results suggest that microbial respiration rates, and resultant PBT composition, are linked to downwelling events and increasing DO concentrations (Reeder et al., 2018). The observed disconnect in sediment PBT and respiration rates suggests a strong biogeochemical connection with riverine oxygen patterns across all stream orders and serves to highlight the importance of future sampling efforts to understand biogeochemical transformations. Similarly, sediment grain size was significantly correlated to sediment PBT composition. Grain size and hyporheic exchange are intimately connected as porosity is a key control on the microbial access to DOC and other solutes and reflects subsurface flow regimes (Li et al., 2020). Changes in DOC and anions through surface-subsurface exchange drive and link the sediment biogeochemical transformations to surface water activities. Given these results, we hypothesize that these patterns of DO, DOC, and PBT exist across large scales, likely demonstrating the importance of hyporheic exchange and its link to carbon cycling. These findings highlight the importance of local reach characteristics (macrophyte coverage, sediment type, algal mat presence, etc.) in determining biogeochemical transformations. Future studies should consider anthropogenic climatic impacts on transport and processing of DOM in light of major hydrologic events (Lu and Liu, 2019), peak glacial melt (Buser-Young et al., 2022), and other extreme weather events (Zhang et al., 2016; Olmedo et al., 2022).
Ecological control of riverine protein assemblages
Our study revealed that the environmental protein-class compound assemblage was reliant upon stream order classifications and associated ecological relationships (DO, DOC, etc.). By measuring trait-like relational information shared between protein-class compounds using molecular characteristics (Danczak et al., 2020). Essentially, protein-class compounds were organized into a molecular characteristics dendrogram (MCD) which integrated elemental composition and derived statistics, including AI, DBE, and NOSC, to resolve potential relationships which are independent of the PBT previously discussed. Protein class compounds were chosen for preliminary analysis due to their link to biotic processes and to allow exploration of broad patterns of amino acid-like transformation across surface water and sediment (Fudyma et al., 2021). Briefly, as determined by the MCD analyses, the degree of protein-class compound assemblage can be linked to microbial processes and local environmental factors to determine underlying biogeochemistry to curate understanding and generate hypotheses. Heterogeneous selection of protein-class compounds likely represents biochemically active metabolites and will therefore exhibit deterministic assemblage evidenced by high positive values in null modeling techniques. However, the nuances of heterogeneous selection, homogeneous selection, and stochastic processes revealed by protein-class compound analyses strengthens our understanding of the biogeochemical activity. Here we conducted βNTI analyses to determine surface water and sediment protein-class assemblage characteristics across low (1, 2), mid (3–5), and high stream orders (6–9).
Using metacommunity ecology approaches (βNTI, mantel tests) we found that low stream orders primarily exhibit deterministic protein-class compound assemblages in both surface water and sediment samples. Deterministic assemblage is most likely indicative of biochemical transformations and potentially active metabolites (Danczak et al., 2020). However, low stream order is likely governed by high rates of advective hydrologic transport or vector movements further driving stochasticity (Figure 5) due to significant mixing and subsequent randomization of compound assemblage. Low stream orders such as headwater streams have highly heterogeneous DOM compositions, mirroring the variability in land cover, increased terrigenous input, and fewer biogeochemical transformations (Garcia et al., 2015; Estévez et al., 2021). Riverine DOM is a mixture of autochthonous and allochthonous OM (Fudyma et al., 2021) and previous research has found that DOM composition is remarkably similar across catchments, reflecting most closely the terrestrial environment (Jaffé et al., 2012; Hawkes et al., 2018). In this study, low stream order protein-class compounds were primarily governed by deterministic selection where other processes have lower influence on assemblage, while surface water samples retain apparent advective impacts but to a lesser extent than when compared to high stream orders. High stream order compound assemblage likely becomes more homogeneous in their structural diversity across the surface water and sediment, which is linked to constant microbial degradation and longer residence time. Across high stream orders, stochasticity is higher in surface water and sediment environments as increased hydrologic transport homogenizes the compound assemblage. Similarly, free-living and particle-associated microorganisms in the water column have variable access to DOM (Chen et al., 2021) due to selective pressures (i.e., photodegradation) which further contribute to higher homogeneous and stochastic selection of the protein-class compounds in the water column. Additionally, persistence of anaerobic environments within sediments drives preferential consumption of labile compounds, likely leading to protein-class assemblages that are undominated by, or reflecting of, no singular assemblage process. Under these circumstances, selection and dispersal of compounds is likely weak, hinting at a relatively heterogeneous protein-class pool in surface water and sediment. Summarily, a lack of temporally or spatially consistent factors drives metabolite drift and low exchange rates. Single time point and discrete sampling campaigns provide critical information on a snapshot of the metabolome, yet more in depth or continuous sampling would further identify consistent factors of variation that drive metabolite assemblages on short and long terms. High stream orders were more generally multi-channeled or cover a large floodplain which has a great effect on DOM export and biogeochemical activity shaped by peak flows (Lynch et al., 2019).
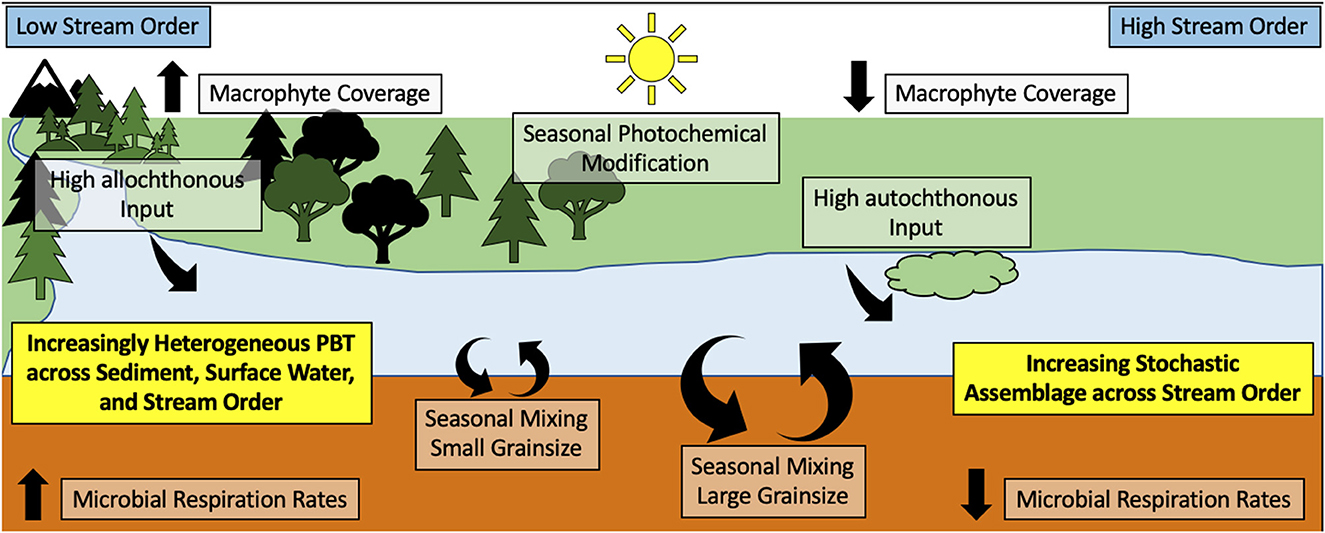
Figure 5. Conceptual overview of the proposed organic matter transformations in WHONDRS lotic environments (18°N−68°N). The PBT is an abbreviation of putative biogeochemical transformations.
We found that stream order significantly influences the PBT dissimilarities observed between surface water and sediment samples when protein-class compounds are expanded to include lipids, a likely proxy for microbial biomass (Bailey et al., 2017). When scrutinizing the molecular character of the protein-class compounds, there was a general similarity across stream orders and ecological assemblage processes. As found by Garayburu-Caruso et al. (2020), the distinct shift from stochastic to deterministic processes when proteins and lipids are analyzed (as opposed to just proteins) could represent increased microbial biomass and their relative influence on metabolome assemblage. These contrasting relationships suggest that stream orders have different PBT compositions yet retain similar patterns of biogeochemical activity. For example, low stream orders may be dominated by terrestrial inputs and macrophyte coverage, while high stream orders contain more autochthonous primary production and residual DOM from upstream, shaping a PBT composition reflecting unique, regional compounds. Despite the differences in PBT compositions, both stream order locations are undergoing rapid biogeochemical activity, evidenced by deterministic assembly processes. This pattern has also been observed previously (Danczak et al., 2021), an occurrence termed “thermodynamic redundancy,” and we expand it here to encompass the WHONDRS watersheds.
Overall our results offer a glimpse into biochemical assemblage of environmental metabolomes and clearly demonstrate a need to further assess the entire WHONDRS environmental metabolomes (e.g., using transformation dendrograms) for a complete biogeochemical picture. Despite these limitations, the MCD assemblage and PBT composition results presented here are consistent with previous research regarding surface water and sediment biotic and abiotic transformations (Stegen et al., 2022). Regardless of stream order, seasonal variation likely has a predominant effect on the environmental metabolome assemblage (Guarch-Ribot and Butturini, 2016; Coble et al., 2019), evidenced by similar assemblage patterns across low and high stream orders. Given our results above regarding the correlations between PBT assemblage, DO, and sediment grain size, we hypothesize that a strong, variable, and seasonal selection such as hyporheic flushing events lead to a divergence in protein-class compound assemblage in the sediment (and to a lesser extent in the surface water). Future research should therefore encompass samples representing spring melt or other such downwelling events, as we predict that surface water and sediment compositions would be less deterministically structured as advective forces increase and solutes are mixed. Additional analyses may address the contributing environmental factors to stochastic assemblage in riverine water columns to determine the degree to which drift, dispersal limitation, and homogenizing dispersal drive assemblage.
Conclusion
In order to understand putative biogeochemical transformations (PBT) across 97 watersheds sampled by the WHONDRS consortium, we explored the relationships between DOC concentrations, OM pools (determined using FT-ICR-MS), sediment respiration rates, and molecular diversity patterns linked to biochemical transformations. We found a strong variation of PBT across studied rivers, yet stream order was a main driver of respiration rates and PBT compositional dissimilarity, regardless of latitude. Despite high heterogeneity across watershed characteristics, emergent patterns in PBT were largely linked to stream order and, to a greater extent, sample type (surface water versus sediment) where mid-stream orders and sediment samples showcase the highest biochemical activity. Protein-class molecular assemblage across stream orders was strongly deterministic within sediments versus surface waters, where the surface water assemblages secondarily reflect flow regimes, vertical mixing, and terrestrial input through stochastic drivers. However, addition of lipid-class compounds revealed that along stream order assemblages became strongly stochastic, showing that no single process is able to dominate the DOM assemblage of bioactive molecules. We modeled relationships between DOM and electron acceptors across stream orders and sample types, finding evidence that sediments are generally limited in DO, driving anaerobic processes and a heterogeneous DOM assemblage. Broad-scale biogeochemical processes capable of shaping DOM assemblages—and therefore carbon cycling—change in response to seasonal processes and local landscape patterns. As lotic systems experience shifting temperature regimes and altered DOM transport and processing, improved understanding of DOM dynamics and drivers in these systems is critical. In this regard this work provides a foundation for further characterization of global watersheds and how they participate in biogeochemical transformations.
Data availability statement
The datasets presented in this study can be found in online repositories. The names of the repository/repositories and accession number(s) can be found below: https://github.com/WHONDRS-Crowdsourced-Manuscript-Effort/Topic3.
Author contributions
Data analysis: JB-Y, PR, SB, and KB. Conceptualization and methodology: JB-Y, PG, MS, PR, and NW. Writing and original version: JB-Y and PG. Writing and revisions: JB-Y, PG, MS, PR, NW, SB, and CL. All authors contributed to the article and approved the submitted version.
Funding
PR and NW were supported by COMPASS-FME, a multi-institutional project funded by the U.S. Department of Energy, Office of Science, Biological and Environmental Research as part of the Environmental System Science Program. MS was supported by the NOAA Michigan Sea Grant (R/ET-2) program. CL was supported by the Independent Research Fund Denmark (Grant: 1127-00033B). PG was a CONICET researcher.
Acknowledgments
Oak Ridge National Laboratory was managed by UT-Battelle, LLC for the US Department of Energy under contract no. DE-AC05-00OR22725. The Pacific Northwest National Laboratory is operated for DOE by Battelle Memorial Institute under contract DE-AC05-76RL01830. The authors would like to thank the WHONDRS consortium for their volunteer sample collection. We are grateful to the reviewers who provided helpful suggestions that improved the manuscript.
Conflict of interest
The authors declare that the research was conducted in the absence of any commercial or financial relationships that could be construed as a potential conflict of interest.
Publisher's note
All claims expressed in this article are solely those of the authors and do not necessarily represent those of their affiliated organizations, or those of the publisher, the editors and the reviewers. Any product that may be evaluated in this article, or claim that may be made by its manufacturer, is not guaranteed or endorsed by the publisher.
Supplementary material
The Supplementary Material for this article can be found online at: https://www.frontiersin.org/articles/10.3389/frwa.2023.1005792/full#supplementary-material
References
Aiken, G. (2014). “Fluorescence and dissolved organic matter: a chemist's perspective,” in Aquatic Organic Matter Fluorescence. Cambridge Environmental Chemistry Series, eds. A. Baker, D. M. Reynolds, J. Lead, P. G. Coble, and R. G. M. Spencer (Cambridge: Cambridge University Press), 35–74. doi: 10.1017/CBO9781139045452.005
Aufdenkampe, A. K., Mayorga, E., Raymond, P. A., Melack, J. M., Doney, S. C., Alin, S. R., et al. (2011). Riverine coupling of biogeochemical cycles between land, oceans, and atmosphere. Front. Ecol. Environ. 9, 53–60. doi: 10.1890/100014
Bailey, V. L., Smith, A. P., Tfaily, M., Fansler, S. J., and Bond-Lamberty, B. (2017). Differences in soluble organic carbon chemistry in pore waters sampled from different pore size domains. Soil Biol. Biochem. 107, 133–143. doi: 10.1016/j.soilbio.2016.11.025
Baltar, F., Alvarez-Salgado, X. A., Arístegui, J., Benner, R., Hansell, D. A., Herndl, G. J., et al. (2021). What is refractory organic matter in the ocean? Front. Mar. Sci. 8, 642637. doi: 10.3389/fmars.2021.642637
Benner, R. (2002). “Chapter 3 - chemical composition and reactivity,” in Biogeochemistry of Marine Dissolved Organic Matter, eds. D. A. Hansell and C. A. Carlson (San Diego: Academic Press), 59–90. doi: 10.1016/B978-012323841-2/50005-1
Berger, T., Mathurin, F. A., Drake, H., and Åström, M. E. (2016). Fluoride abundance and controls in fresh groundwater in Quaternary deposits and bedrock fractures in an area with fluorine-rich granitoid rocks. Sci. Total Environ. 569–570, 948–960. doi: 10.1016/j.scitotenv.2016.06.002
Bethke, C. M., Sanford, R. A., Kirk, M. F., Jin, Q., and Flynn, T. M. (2011). The thermodynamic ladder in geomicrobiology. Am. J. Sci. 311, 183–210. doi: 10.2475/03.2011.01
Boano, F., Harvey, J. W., Marion, A., Packman, A. I., Revelli, R., Ridolfi, L., et al. (2014). Hyporheic flow and transport processes: mechanisms, models, and biogeochemical implications. Rev. Geophys. 52, 603–679. doi: 10.1002/2012RG000417
Breitling, R., Ritchie, S., Goodenowe, D., Stewart, M. L., and Barrett, M. P. (2006). Ab initio prediction of metabolic networks using Fourier transform mass spectrometry data. Metabolomics 2, 155–164. doi: 10.1007/s11306-006-0029-z
Buffam, I., Bishop, K., and Laudon, H. (2021). Influence of the landscape template on chemical and physical habitat for brown trout within a Boreal Stream network. Front. Water 3, 683070. doi: 10.3389/frwa.2021.683070
Buser-Young, J., Lapham, L., Thurber, A. R., Williams, K. H., and Colwell, F. (2021). Hidden processes during seasonal isolation of a high-altitude watershed. Front. Earth Sci. 9, 666819. doi: 10.3389/feart.2021.666819
Buser-Young, J., Peck, E. K., Chace, P., Lapham, L. L., Vizza, C., and Colwell, F. S. (2022). Biogeochemical dynamics of a glaciated high-latitude wetland. J. Geophys. Res. Biogeosci. 127, e2021JG006584. doi: 10.1029/2021JG006584
Butman, D., Stackpoole, S., Stets, E., McDonald, C. P., Clow, D. W., and Striegl, R. G. (2016). Aquatic carbon cycling in the conterminous United States and implications for terrestrial carbon accounting. Proc. Natl. Acad. Sci. USA 113, 58–63. doi: 10.1073/pnas.1512651112
Catalán, N., Marcé, R., Kothawala, D. N., and Tranvik, L. J. (2016). Organic carbon decomposition rates controlled by water retention time across inland waters. Nat. Geosci. 9, 501–504. doi: 10.1038/ngeo2720
Chen, M., Kim, S., Park, J.-E., Jung, H.-J., and Hur, J. (2016). Structural and compositional changes of dissolved organic matter upon solid-phase extraction tracked by multiple analytical tools. Anal. Bioanal. Chem. 408, 6249–6258. doi: 10.1007/s00216-016-9728-0
Chen, Y.-J., Leung, P. M., Cook, P. L. M., Wong, W. W., Hutchinson, T., Eate, V., et al. (2021). Hydrodynamic disturbance controls microbial community assembly and biogeochemical processes in coastal sediments. ISME J. 16, 752–763. doi: 10.1038/s41396-021-01111-9
Coble, A. A., Koenig, L. E., Potter, J. D., Parham, L. M., and McDowell, W. H. (2019). Homogenization of dissolved organic matter within a river network occurs in the smallest headwaters. Biogeochemistry 143, 85–104. doi: 10.1007/s10533-019-00551-y
Cole, J. J., Prairie, Y. T., Caraco, N. F., McDowell, W. H., Tranvik, L. J., Striegl, R. G., et al. (2007). Plumbing the global carbon cycle: integrating inland waters into the terrestrial carbon budget. Ecosystems 10, 172–185. doi: 10.1007/s10021-006-9013-8
Cooper, W. T., Chanton, J. C., D'Andrilli, J., Hodgkins, S. B., Podgorski, D. C., Stenson, A. C., et al. (2020). A history of molecular level analysis of natural organic matter by FTICR mass spectrometry and the paradigm shift in organic geochemistry. Mass Spectrom. Rev. 41, 21663. doi: 10.1002/mas.21663
Corsi, S. R., De Cicco, L. A., Lutz, M. A., and Hirsch, R. M. (2015). River chloride trends in snow-affected urban watersheds: increasing concentrations outpace urban growth rate and are common among all seasons. Sci. Total Environ. 508, 488–497. doi: 10.1016/j.scitotenv.2014.12.012
Cory, R. M., Crump, B. C., Dobkowski, J. A., and Kling, G. W. (2013). Surface exposure to sunlight stimulates CO2 release from permafrost soil carbon in the Arctic. Proc. Natl. Acad. Sci. USA 110, 3429–3434. doi: 10.1073/pnas.1214104110
Creed, I. F., McKnight, D. M., Pellerin, B. A., Green, M. B., Bergamaschi, B. A., Aiken, G. R., et al. (2015). The river as a chemostat: fresh perspectives on dissolved organic matter flowing down the river continuum. Can. J. Fish. Aquat. Sci. 72, 1272–1285. doi: 10.1139/cjfas-2014-0400
Crump, B., Baross, J., and Simenstad, C. (1998). Dominance of particle-attached bacteria in the Columbia River Estuary, USA. Aquat. Microb. Ecol. 14, 7–18. doi: 10.3354/ame014007
Danczak, R. E., Chu, R. K., Fansler, S. J., Goldman, A. E., Graham, E. B., Tfaily, M. M., et al. (2020). Using metacommunity ecology to understand environmental metabolomes. Nat. Commun. 11, 6369. doi: 10.1038/s41467-020-19989-y
Danczak, R. E., Goldman, A. E., Chu, R. K., Toyoda, J. G., Garayburu-Caruso, V. A., Tolić, N., et al. (2021). Ecological theory applied to environmental metabolomes reveals compositional divergence despite conserved molecular properties. Sci. Total Environ. 788, 147409. doi: 10.1016/j.scitotenv.2021.147409
Danczak, R. E., Sawyer, A. H., Williams, K. H., Stegen, J. C., Hobson, C., and Wilkins, M. J. (2016). Seasonal hyporheic dynamics control coupled microbiology and geochemistry in Colorado River sediments. J. Geophys. Res. Biogeosci. 121, 2976–2987. doi: 10.1002/2016JG003527
De Falco, N., Boano, F., Bogler, A., Bar-Zeev, E., and Arnon, S. (2018). Influence of stream-subsurface exchange flux and bacterial biofilms on oxygen consumption under nutrient-rich conditions. J. Geophys. Res. Biogeosci. 123, 2021–2034. doi: 10.1029/2017JG004372
Diamond, J. S., Bernal, S., Boukra, A., Cohen, M. J., Lewis, D., Masson, M., et al. (2021). Stream network variation in dissolved oxygen: metabolism proxies and biogeochemical controls. Ecol. Indic. 131, 108233. doi: 10.1016/j.ecolind.2021.108233
Doherty, S. J., Barbato, R. A., Grandy, A. S., Thomas, W. K., Monteux, S., Dorrepaal, E., et al. (2020). The transition from stochastic to deterministic bacterial community assembly during permafrost thaw succession. Front. Microbiol. 11, 596589. doi: 10.3389/fmicb.2020.596589
Drake, T. W., Raymond, P. A., and Spencer, R. G. M. (2018). Terrestrial carbon inputs to inland waters: a current synthesis of estimates and uncertainty. Limnol. Oceanogr. Lett. 3, 132–142. doi: 10.1002/lol2.10055
Ellis, E. E., Richey, J. E., Aufdenkampe, A. K., Krusche, A. V., Quay, P. D., Salimon, C., et al. (2012). Factors controlling water-column respiration in rivers of the central and southwestern Amazon Basin. Limnol. Oceanogr. 57, 527–540. doi: 10.4319/lo.2012.57.2.0527
Estévez, E., Álvarez-Martínez, J. M., Dittmar, T., Barquín, J., and Singer, G. (2021). When forests take over after land abandonment: dissolved organic matter response in headwater mountain streams. Front. Water 3, 682608. doi: 10.3389/frwa.2021.682608
Fudyma, J. D., Chu, R. K., Graf Grachet, N., Stegen, J. C., and Tfaily, M. M. (2021). Coupled biotic-abiotic processes control biogeochemical cycling of dissolved organic matter in the columbia river hyporheic zone. Front. Water. 2, 574692. doi: 10.3389/frwa.2020.574692
Garayburu-Caruso, V., Danczak, R., Stegen, J., Renteria, L., McCall, M., Goldman, A., et al. (2020). Using community science to reveal the global chemogeography of river metabolomes. Metabolites 10, 518. doi: 10.3390/metabo10120518
Garcia, R. D., Reissig, M., Queimaliños, C. P., Garcia, P. E., and Dieguez, M. C. (2015). Climate-driven terrestrial inputs in ultraoligotrophic mountain streams of Andean Patagonia revealed through chromophoric and fluorescent dissolved organic matter. Sci. Total Environ. 521–522, 280–292. doi: 10.1016/j.scitotenv.2015.03.102
Geer Wallace, M. A., and McCord, J. P. (2020). “Chapter 16—High-resolution mass spectrometry,” in Breathborne Biomarkers and the Human Volatilome (Second Edition), eds. J. Beauchamp, C. Davis, and J. Pleil (Boston: Elsevier), 253–270. doi: 10.1016/B978-0-12-819967-1.00016-5
Ghosh, S. (2017). Triterpene structural diversification by plant cytochrome P450 enzymes. Front. Plant Sci. 8, 1886. doi: 10.3389/fpls.2017.01886
Goldman, A. E., Chu, R. K., Danczak, R. E., Daly, R. A., Fansler, S., Garayburu-Caruso, V. A., et al. (2020). WHONDRS Summer 2019 Sampling Campaign: Global River Corridor Sediment FTICR-MS, NPOC, and Aerobic Respiration. Environmental System Science Data Infrastructure for a Virtual Ecosystem (ESS-DIVE) (United States); River Corridor and Watershed Biogeochemistry SFA Worldwide Hydrobiogeochemistry Observation Network for Dynamic River Systems (WHONDRS). doi: 10.15485/1729719
Graedel, T. E., and Keene, W. C. (1996). The budget and cycle of earth's natural chlorine. Pure Appl. Chem. 68, 1689–1697. doi: 10.1351/pac199668091689
Guarch-Ribot, A., and Butturini, A. (2016). Hydrological conditions regulate dissolved organic matter quality in an intermittent headwater stream. From drought to storm analysis. Sci. Total Environ. 571, 1358–1369. doi: 10.1016/j.scitotenv.2016.07.060
Guillemette, F., and del Giorgio, P. A. (2012). Simultaneous consumption and production of fluorescent dissolved organic matter by lake bacterioplankton. Environ. Microbiol. 14, 1432–1443. doi: 10.1111/j.1462-2920.2012.02728.x
Harrell, F. Jr (2021). Hmisc: Harrell Miscellaneous. R package version 4.6-0. Available online at: https://CRAN.R-project.org/package=Hmisc (accessed February 8, 2023).
Hawkes, J. A., Radoman, N., Bergquist, J., Wallin, M. B., Tranvik, L. J., and Löfgren, S. (2018). Regional diversity of complex dissolved organic matter across forested hemiboreal headwater streams. Sci. Rep. 8, 16060. doi: 10.1038/s41598-018-34272-3
Holt, A. D., Fellman, J., Hood, E., Kellerman, A. M., Raymond, P., Stubbins, A., et al. (2021). The evolution of stream dissolved organic matter composition following glacier retreat in coastal watersheds of southeast Alaska. Biogeochemistry. 2021, 1–18. doi: 10.1007/s10533-021-00815-6
Hotchkiss, E. R., Hall, R. O. Jr, Sponseller, R. A., Butman, D., Klaminder, J., Laudon, H., et al. (2015). Sources of and processes controlling CO2 emissions change with the size of streams and rivers. Nat. Geosci. 8, 696–699. doi: 10.1038/ngeo2507
Jaffé, R., Yamashita, Y., Maie, N., Cooper, W. T., Dittmar, T., Dodds, W. K., et al. (2012). Dissolved organic matter in headwater streams: compositional variability across climatic regions of North America. Geochim. Cosmochim. Acta 94, 95–108. doi: 10.1016/j.gca.2012.06.031
Jensen, B. (2022). AOS Protocol and Procedure: Sediment Chemistry Sampling in Wadeable Streams (NEON.DOC.001193). Available online at: http://data.neonscience.org/documents (accessed on 1 June 2022).
Kassambara, A. (2020). ggpubr: 'ggplot2' Based Publication Ready Plots. R package version 0.4.0. Available online at: https://CRAN.R-project.org/package=ggpubr
Kellerman, A. M., Guillemette, F., Podgorski, D. C., Aiken, G. R., Butler, K. D., and Spencer, R. G. M. (2018). Unifying concepts linking dissolved organic matter composition to persistence in aquatic ecosystems. Environ. Sci. Technol. 52, 2538–2548. doi: 10.1021/acs.est.7b05513
Kembel, S. W., Cowan, P. D., Helmus, M. R., Cornwell, W. K., Morlon, H., Ackerly, D. D., et al. (2010). Picante: R tools for integrating phylogenies and ecology. Bioinformatics 26, 1463–1464. doi: 10.1093/bioinformatics/btq166
Kieft, B., Crump, B., White, A. E., Goñi, M., and Mueller, R. S. (2020). Winter river plumes shape community composition and activity of heterotrophic microorganisms on the Oregon Coast. Aquat. Microb. Ecol. 84, 15–29. doi: 10.3354/ame01922
Kim, S., Kaplan, L. A., Benner, R., and Hatcher, P. G. (2004). Hydrogen-deficient molecules in natural riverine water samples—evidence for the existence of black carbon in DOM. Mar. Chem. 92, 225–234. doi: 10.1016/j.marchem.2004.06.042
Koch, B. P., and Dittmar, T. (2006). From mass to structure: an aromaticity index for high-resolution mass data of natural organic matter. Rapid Commun. Mass Spectrom. 20, 926–932. doi: 10.1002/rcm.2386
Kupka, D., Kania, M., and Gruba, P. (2021). The proximity of a highway increases CO2 respiration in forest soil and decreases the stability of soil organic matter. Sci. Rep. 11, 21605. doi: 10.1038/s41598-021-00971-7
Kuroda, P. K., and Sandell, E. B. (1953). Chlorine in igneous rocks: some aspects of the geochemistry of chlorine. GSA Bull. 64, 879–896. doi: 10.1130/0016-7606(1953)64[879:CIIR]2.0.CO;2
Li, Y., Zhu, J., Wang, L., Gao, Y., Zhang, W., Zhang, H., et al. (2020). Grain size tunes microbial community assembly and nitrogen transformation activity under frequent hyporheic exchange: a column experiment. Water Res. 182, 116040. doi: 10.1016/j.watres.2020.116040
Liu, Y., Lin, Q., Feng, J., Yang, F., Du, H., Hu, Z., et al. (2020). Differences in metabolic potential between particle-associated and free-living bacteria along Pearl River Estuary. Sci. Total Environ. 728, 138856. doi: 10.1016/j.scitotenv.2020.138856
Lu, K., and Liu, Z. (2019). Molecular level analysis reveals changes in chemical composition of dissolved organic matter from south Texas Rivers after high flow events. Front. Mar. Sci. 6, 673. doi: 10.3389/fmars.2019.00673
Lynch, L. M., Sutfin, N. A., Fegel, T. S., Boot, C. M., Covino, T. P., and Wallenstein, M. D. (2019). River channel connectivity shifts metabolite composition and dissolved organic matter chemistry. Nat. Commun. 10, 459. doi: 10.1038/s41467-019-08406-8
McCallister, S. L., Ishikawa, N. F., and Kothawala, D. N. (2018). Biogeochemical tools for characterizing organic carbon in inland aquatic ecosystems. Limnol. Oceanogr. Lett. 3, 444–457. doi: 10.1002/lol2.10097
Mosher, J. J., Kaplan, L. A., Podgorski, D. C., McKenna, A. M., and Marshall, A. G. (2015). Longitudinal shifts in dissolved organic matter chemogeography and chemodiversity within headwater streams: a river continuum reprise. Biogeochemistry 124, 371–385. doi: 10.1007/s10533-015-0103-6
Nelson, A. R., Sawyer, A. H., Gabor, R. S., Saup, C. M., Bryant, S. R., Harris, K. D., et al. (2019). Heterogeneity in hyporheic flow, pore water chemistry, and microbial community composition in an alpine streambed. J. Geophys. Res. Biogeosci. 124, 3465–3478. doi: 10.1029/2019JG005226
Oksanen, J., Blanchet, F. G., Friendly, M., Kindt, R., Legendre, P., McGlinn, D., et al. (2020). vegan: Community Ecology Package. R package version 2.5-7. Available online at: https://CRAN.R-project.org/package=vegan (acessed January 27, 2022).
Olmedo, E., Turiel, A., González-Gambau, V., González-Haro, C., García-Espriu, A., Gabarró, C., et al. (2022). Increasing stratification as observed by satellite sea surface salinity measurements. Sci. Rep. 12, 6279. doi: 10.1038/s41598-022-10265-1
Raymond, P. A., Hartmann, J., Lauerwald, R., Sobek, S., McDonald, C., Hoover, M., et al. (2013). Global carbon dioxide emissions from inland waters. Nature 503, 355–359.
Raymond, P. A., Saiers, J. E., and Sobczak, W. V. (2016). Hydrological and biogeochemical controls on watershed dissolved organic matter transport: pulse-shunt concept. Ecology 97, 5–16. doi: 10.1890/14-1684.1
Reeder, W. J., Quick, A. M., Farrell, T. B., Benner, S. G., Feris, K. P., and Tonina, D. (2018). Spatial and temporal dynamics of dissolved oxygen concentrations and bioactivity in the hyporheic zone. Water Resour. Res. 54, 2112–2128. doi: 10.1002/2017WR021388
Regier, P., Larsen, L., Cawley, K., and Jaffé, R. (2020). Linking hydrology and dissolved organic matter characteristics in a subtropical wetland: a long-term study of the florida everglades. Glob. Biogeochem. Cycles 34, e2020GB006648. doi: 10.1029/2020GB006648
Regnier, P., Resplandy, L., Najjar, R. G., and Ciais, P. (2022). The land-to-ocean loops of the global carbon cycle. Nature 603, 401–410. doi: 10.1038/s41586-021-04339-9
Repeta, D. J. (2015). “Chapter 2 - Chemical characterization and cycling of dissolved organic matter,” in Biogeochemistry of Marine Dissolved Organic Matter (Second Edition), eds. D. A. Hansell and C. A. Carlson (Boston: Academic Press), 21–63. doi: 10.1016/B978-0-12-405940-5.00002-9
Roden, E. E., and Jin, Q. (2011). Thermodynamics of microbial growth coupled to metabolism of glucose, ethanol, short-chain organic acids, and hydrogen. Appl. Environ. Microbiol. 77, 1907–1909. doi: 10.1128/AEM.02425-10
Rosseel, Y. (2012). lavaan: an R package for structural equation modeling. J. Stat. Softw. 48, 1–36. doi: 10.18637/jss.v048.i02
Santana-Molina, C., Rivas-Marin, E., Rojas, A. M., and Devos, D. P. (2020). Origin and evolution of polycyclic triterpene synthesis. Mol. Biol. Evol. 37, 1925–1941. doi: 10.1093/molbev/msaa054
Saup, C. M., Bryant, S. R., Nelson, A. R., Harris, K. D., Sawyer, A. H., Christensen, J. N., et al. (2019). Hyporheic zone microbiome assembly is linked to dynamic water mixing patterns in snowmelt-dominated headwater catchments. J. Geophys. Res. Biogeosci. 124, 3269–3280. doi: 10.1029/2019JG005189
Savoy, P., and Harvey, J. W. (2021). Predicting light regime controls on primary productivity across CONUS river networks. Geophys. Res. Lett. 48, e2020GL092149. doi: 10.1029/2020GL092149
Seidel, M., Dittmar, T., Ward, N. D., Krusche, A. V., Richey, J. E., Yager, P. L., et al. (2016). Seasonal and spatial variability of dissolved organic matter composition in the lower Amazon River. Biogeochemistry 131, 281–302. doi: 10.1007/s10533-016-0279-4
Stegen, J. C., Fansler, S. J., Tfaily, M. M., Garayburu-Caruso, V. A., Goldman, A. E., Danczak, R. E., et al. (2022). Organic matter transformations are disconnected between surface water and the hyporheic zone. Biogeosciences 19, 3099–3110. doi: 10.5194/bg-19-3099-2022
Stegen, J. C., and Goldman, A. E. (2018). WHONDRS: a community resource for studying dynamic river corridors. mSystems 3, e00151–e00118. doi: 10.1128/mSystems.00151-18
Stegen, J. C., Lin, X., Fredrickson, J. K., Chen, X., Kennedy, D. W., Murray, C. J., et al. (2013). Quantifying community assembly processes and identifying features that impose them. ISME J. 7, 2069–2079. doi: 10.1038/ismej.2013.93
Stegen, J. C., Lin, X., Konopka, A. E., and Fredrickson, J. K. (2012). Stochastic and deterministic assembly processes in subsurface microbial communities. ISME J. 6, 1653–1664. doi: 10.1038/ismej.2012.22
Stubbins, A., Spencer, R. G. M., Chen, H., Hatcher, P. G., Mopper, K., Hernes, P. J., et al. (2010). Illuminated darkness: molecular signatures of Congo River dissolved organic matter and its photochemical alteration as revealed by ultrahigh precision mass spectrometry. Limnol. Oceanogr. 55, 1467–1477. doi: 10.4319/lo.2010.55.4.1467
Tanabe, Y., Hori, M., Mizuno, A. N., Osono, T., Uchida, M., Kudoh, S., et al. (2019). Light quality determines primary production in nutrient-poor small lakes. Sci. Rep. 9, 4639. doi: 10.1038/s41598-019-41003-9
The North America Tapestry of Time and Terrain (2022). USGS I-map 2781. Available online at: https://pubs.usgs.gov/imap/i2781/ (accessed July 7, 2022).
Toyoda, J. G., Goldman, A. E., Chu, R. K., Danczak, R. E., Daly, R. A., Garayburu-Caruso, V. A., et al. (2020). WHONDRS Summer 2019 Sampling Campaign: Global River Corridor Surface Water FTICR-MS and Stable Isotopes. Environmental System Science Data Infrastructure for a Virtual Ecosystem (ESS-DIVE) (United States); Worldwide Hydrobiogeochemistry Observation Network for Dynamic River Systems (WHONDRS). doi: 10.15485/1603775
Vannote, R. L., Minshall, W. G., Cummins, K. W., Sedell, J. R., and Cushing, C. E. (1980). The river continuum concept. Can. J. Fish. Aquat. Sci. 37, 130–137. doi: 10.1139/f80-017
Ward, N. D., Bianchi, T. S., Medeiros, P. M., Seidel, M., Richey, J. E., Keil, R. G., et al. (2017). Where carbon goes when water flows: carbon cycling across the aquatic continuum. Front. Mar. Sci. 4. doi: 10.3389/fmars.2017.00007
Ward, N. D., Bianchi, T. S., Sawakuchi, H. O., Gagne-Maynard, W., Cunha, A. C., Brito, D. C., et al. (2016). The reactivity of plant-derived organic matter and the potential importance of priming effects along the lower Amazon River. J. Geophys. Res. Biogeosciences 121, 1522–1539. doi: 10.1002/2016JG003342
Ward, N. D., Sawakuchi, H. O., Neu, V., Less, D. F. S., Valerio, A. M., Cunha, A. C., et al. (2018). Velocity-amplified microbial respiration rates in the lower Amazon River. Limnol. Oceanogr. Lett. 3, 265–274. doi: 10.1002/lol2.10062
Ward, N. D., Sawakuchi, H. O., Richey, J. E., Keil, R. G., and Bianchi, T. S. (2019). Enhanced aquatic respiration associated with mixing of clearwater tributary and turbid amazon river waters. Front. Earth Sci. 7, 101. doi: 10.3389/feart.2019.00101
Winnick, M. J., Carroll, R. W. H., Williams, K. H., Maxwell, R. M., Dong, W., and Maher, K. (2017). Snowmelt controls on concentration-discharge relationships and the balance of oxidative and acid-base weathering fluxes in an alpine catchment, East River, Colorado. Water Resour. Res. 53, 2507–2523. doi: 10.1002/2016WR019724
Zark, M., and Dittmar, T. (2018). Universal molecular structures in natural dissolved organic matter. Nat. Commun. 9, 3178. doi: 10.1038/s41467-018-05665-9
Keywords: organic matter, FT-ICR-MS, biogeochemical transformations, crowdsourced science, rivers
Citation: Buser-Young JZ, Garcia PE, Schrenk MO, Regier PJ, Ward ND, Biçe K, Brooks SC, Freeman EC and Lønborg C (2023) Determining the biogeochemical transformations of organic matter composition in rivers using molecular signatures. Front. Water 5:1005792. doi: 10.3389/frwa.2023.1005792
Received: 28 July 2022; Accepted: 06 March 2023;
Published: 21 March 2023.
Edited by:
Sarah Collins, University of Wyoming, United StatesReviewed by:
Meilian Chen, Western Carolina University, United StatesMalak M. Tfaily, University of Arizona, United States
Copyright © 2023 Buser-Young, Garcia, Schrenk, Regier, Ward, Biçe, Brooks, Freeman and Lønborg. This is an open-access article distributed under the terms of the Creative Commons Attribution License (CC BY). The use, distribution or reproduction in other forums is permitted, provided the original author(s) and the copyright owner(s) are credited and that the original publication in this journal is cited, in accordance with accepted academic practice. No use, distribution or reproduction is permitted which does not comply with these terms.
*Correspondence: Patricia E. Garcia, Z2FyY2lhcGVAY29tYWh1ZS1jb25pY2V0LmdvYi5hcg==