- 1Department of Earth and Environmental Sciences, KU Leuven, Heverlee, Belgium
- 2European Space Agency Climate Office, ECSAT, Harwell Campus, Oxfordshire, Didcot, United Kingdom
- 3National Research Council, Research Institute for Geo-Hydrological Protection, Perugia, Italy
- 4CNRM, Université de Toulouse, Météo-France, CNRS, Toulouse, France
- 5Department of Meteorology and NCEO, University of Reading, Reading, United Kingdom
- 6Department of Physics and Astronomy “Augusto Righi”, University of Bologna, Bologna, Italy
- 7USDA-ARS Hydrology and Remote Sensing Laboratory, Beltsville, MD, United States
- 8European Centre for Medium-Range Weather Forecasts, Reading, United Kingdom
- 9School of Earth Sciences, Ohio State University, Columbus, OH, United States
- 10Byrd Polar and Climate Research Center, Ohio State University, Columbus, OH, United States
- 11Department of Civil and Environmental Engineering, University of Maryland, College Park, MD, United States
- 12Deutscher Wetterdienst, Data Assimilation and Predictability, Offenbach, Germany
- 13Environmental Science and Policy Management, University of California, Berkeley, Berkeley, CA, United States
- 14Forschungszentrum Jülich GmbH, Agrosphere (IBG-3), Jülich, Germany
- 15WSL Institute for Snow and Avalanche Research SLF, Davos, Switzerland
- 16NASA Goddard Space Flight Center, Hydrological Sciences Laboratory, Greenbelt, MD, United States
- 17Department of Environment, Ghent University, Ghent, Belgium
- 18School of Civil Engineering, Sun Yat-sen University, Guangzhou, China
- 19Guangdong Engineering Technology Research Center of Water Security Regulation and Control for Southern China, Sun Yat-sen University, Guangzhou, China
- 20Department of Civil Engineering, Monash University, Clayton, VIC, Australia
- 21NASA Goddard Space Flight Center, Global Modeling and Assimilation Office, Greenbelt, MD, United States
- 22Department of Geoscience and Remote Sensing, Delft University of Technology, Delft, Netherlands
The beginning of the 21st century is marked by a rapid growth of land surface satellite data and model sophistication. This offers new opportunities to estimate multiple components of the water cycle via satellite-based land data assimilation (DA) across multiple scales. By resolving more processes in land surface models and by coupling the land, the atmosphere, and other Earth system compartments, the observed information can be propagated to constrain additional unobserved variables. Furthermore, access to more satellite observations enables the direct constraint of more and more components of the water cycle that are of interest to end users. However, the finer level of detail in models and data is also often accompanied by an increase in dimensions, with more state variables, parameters, or boundary conditions to estimate, and more observations to assimilate. This requires advanced DA methods and efficient solutions. One solution is to target specific observations for assimilation based on a sensitivity study or coupling strength analysis, because not all observations are equally effective in improving subsequent forecasts of hydrological variables, weather, agricultural production, or hazards through DA. This paper offers a perspective on current and future land DA development, and suggestions to optimally exploit advances in observing and modeling systems.
Introduction
The distribution of water on Earth determines human livelihoods and is itself influenced by human activities. Estimating the water availability in various terrestrial compartments is essential for water resources management, agricultural monitoring, natural hazards and disaster risk assessment, biodiversity and planet health protection, numerical weather prediction (NWP), seasonal prediction, and climate change mitigation and adaptation. Currently, the most complete regional- to global-scale estimates of water-related variables are obtained by merging satellite data records into numerical models of Earth system processes through data assimilation (DA) (Asch et al., 2016). DA can combine the unprecedented amounts of satellite data with the steadily acquired process understanding of the past decades. Specifically, DA uses the satellite observations to correct errors in model simulations, including errors in unobserved variables. Thereby, DA adds value to the observations by inferring unobserved information, filling gaps and/or enhancing the spatial resolution of satellite data. In the geosciences, DA mostly refers to state estimation theory, but it more generally covers any technique that uses data to estimate the most accurate possible system state (Carrassi et al., 2018) and associated fluxes. Therefore, DA also encompasses model parameter optimization and the correction of boundary conditions, including meteorological forcings.
Land DA developments have been reviewed earlier (Reichle, 2008; Lahoz and De Lannoy, 2014; De Lannoy et al., 2016; Jin et al., 2018; Huang et al., 2019; Xia et al., 2019; Girotto et al., 2020; Baatz et al., 2021; Durand et al., 2021). In parallel to our paper, Kumar et al. (2022, in review) review and identify current community-agreed gaps and priorities for the future of state estimation via land DA. In this paper, we reflect on advances in observing, modeling and DA techniques, the associated opportunities and complexities of future land DA systems, and solutions to keep land DA efficient and effective, in the presence of rapid data growth and model sophistication in the first half of the 21st century. First, we summarize the state of the art of land DA for the estimation of water cycle variables (Section State of the art). Next, we offer a perspective on current observing, modeling and DA systems (Section Perspective on current observing, modeling and DA systems) and on the future goals of land DA (Section Perspective on future DA development). The focus will be on soil moisture, snow and vegetation estimation and how to extend the impact of satellite-based land DA to improved dynamic estimates of the atmosphere, vegetation, hydrological and biogeochemical cycles, as well as of natural hazards.
State of the art
The beginning of the 21st century has seen a sustained increase in remotely sensed data of the Earth system. Figure 1 shows the exponential growth in satellite missions, with about 4,800 active satellite platforms orbiting our Earth in 2021 (https://www.statista.com/statistics/897719/number-of-active-satellites-by-year/), but only 20–25% collect Earth observations, and fewer than 1% are regularly used for land DA. Gravity measurements from the Gravity Recovery and Climate Experiment (GRACE) and GRACE Follow-On missions directly sense changes in total water storage but at a very coarse scale. Optical sensors (onboard the Terra, Landsat, and Sentinel-2 missions, among others) measure fine-scale water content proxies, e.g., snow cover extent, open water extent, vegetation, and soil color or temperature. Microwave sensors (onboard the Soil Moisture Ocean Salinity -SMOS-, Soil Moisture Active Passive -SMAP- and Sentinel-1 missions, the Advanced Microwave Scanning Radiometer onboard Aqua, and the Advanced SCATterometer -ASCAT- onboard Metop, among many more) are used to retrieve water amounts in the soil, vegetation and snow. The passive radiometer sensors collect brightness temperature data at a coarse resolution (~40 km), whereas active synthetic aperture radar (SAR) instruments can collect backscatter data at finer resolutions (<1 km). Microwave sensors exploit the fact that the presence of water directly affects the dielectric properties of the soil, vegetation and snow, and it strongly influences the emission and scattering of microwave radiation (Ulaby et al., 2014). Insight into how radiation interacts with water in different land compartments is summarized in radiative transfer models, which can be used in two ways: (i) to invert the observed radiance into geophysical “retrieval” products (e.g., soil moisture, vegetation or snow water content), or (ii) as so-called observation operators to map simulated land surface variables to satellite-observed signals (e.g., brightness temperature or backscatter).
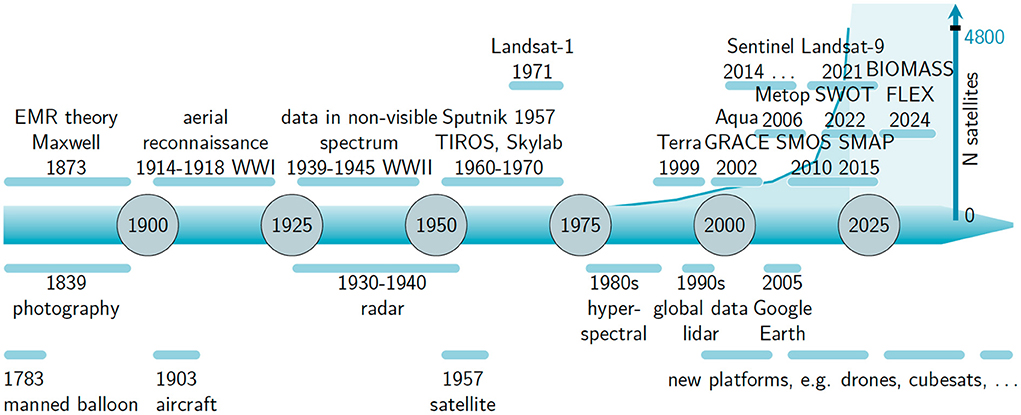
Figure 1. The 21st century within the history of remote sensing, which started with the understanding of electromagnetic radiation (EMR). Select satellite missions used for land DA are marked and discussed in the text.
Many land DA systems have used microwave observations to estimate surface and deeper soil moisture (de Rosnay et al., 2014; De Lannoy et al., 2016; Reichle et al., 2019), and related variables such as discharge (Lievens et al., 2015; De Santis et al., 2021), turbulent fluxes (Lu et al., 2020), and even groundwater in peatlands (Bechtold et al., 2020). With the activation of dynamic vegetation models, the assimilation of optical vegetation indices (e.g., leaf area index) and microwave vegetation optical depth (Fairbairn et al., 2017; Kumar et al., 2020; Mucia et al., 2022) has gained interest, including to improve evapotranspiration (ET) and runoff. DA of thermal satellite data has also been popular for ET and soil moisture estimation (Crow et al., 2008), but studies on the intersection between the water and energy cycle will not be further discussed, to keep the focus on water cycle variables. At the finer scale, optical and radar satellite data have been assimilated in crop models to update canopy or soil state variables and ultimately estimate transpiration, agricultural biomass and yield (Jin et al., 2018; Lu et al., 2022). Under frozen conditions, the assimilation of optical snow cover fraction or microwave-based snow depth has been explored (Helmert et al., 2018; Girotto et al., 2021).
In practice, land DA systems are developed by merging the theoretical insights in DA, which provide a portfolio of algorithms, with the operational and physical constraints of land surface observations and modeling. An overview of regional and global land DA systems is given by Xia et al. (2019). The observations consist either of satellite retrieval products (soil moisture: Dharssi et al., 2011; Liu et al., 2011; Rodríguez-Fernández et al., 2019; vegetation: Albergel et al., 2017; Kumar et al., 2020; snow: De Lannoy et al., 2010) or direct satellite signals (related to soil moisture: De Lannoy and Reichle, 2016; Lievens et al., 2017; Muñoz-Sabater et al., 2019; Reichle et al., 2019; snow: Larue et al., 2018; Xue et al., 2018), and most land DA systems consider far fewer observations than state variables (this characterizes DA in the geosciences at large). For example, one surface soil moisture retrieval every few days can update soil moisture in multiple soil layers and possibly vegetation, or one weekly snow cover fraction observation can update the water amount in different snow layers, while the model state evolves at sub-hourly time steps. Furthermore, most land DA systems are one-dimensional, i.e., they update each soil-vegetation-snow column (grid cell) independently and the analysis update is strictly limited to the observed columns. This formulation does not exploit the capability of many DA approaches to propagate information across the model domain from observed to unobserved areas. If communication among different columns is made possible via the physics-based model or via spatial error correlations, thus making the DA system spatially distributed, then state variables in neighboring (observed or unobserved) columns within the influence radius of a given observation are analyzed together (Reichle and Koster, 2003; De Lannoy et al., 2010; Magnusson et al., 2014; Reichle et al., 2019).
The above studies all aim at state estimation via particle or Kalman filtering variants (other DA methods such as variational DA or direct insertion have also been used) to correct the land surface state for short-term and interannual errors in the meteorological forcings (in offline systems, i.e., not coupled to an atmospheric model) or other unmodeled temporary deviations in some water compartments. In this process, only a few DA systems effectively assign the DA corrections to the source of errors, such as for example snowfall or precipitation input to obtain good snow depth or total water storage estimates (Winstral et al., 2019; Girotto et al., 2021). Most DA systems do not conserve mass, unless the water budget is explicitly constrained (Pan et al., 2012).
To correct land surface estimates for longer-term or systematic deviations, and to minimize water budget imbalances, satellite data can be used more effectively for parameter estimation. These parameters can be part of the prognostic model (Han et al., 2014; Kolassa et al., 2020), the diagnostic radiative transfer model (De Lannoy et al., 2013; Rains et al., 2022), or represent a bias factor for meteorological input (Wrzesien et al., 2022). Long-term model calibration could be seen as a form of long-term DA or history matching. Alternatively, DA for sequential parameter updating (with or without simultaneous state updating) allows to account for time-varying parameters (Montzka et al., 2011; Magnusson et al., 2016).
Perspective on current observing, modeling and DA systems
Observations
The spaceborne observations of many water cycle variables have been improving in radiometric, spatial, and temporal resolution, but dedicated missions are not yet available for all parts of the water budget. Soil moisture is now routinely measured at a coarse resolution by dedicated L-band satellite missions (SMOS, SMAP, Kerr et al., 2010; Entekhabi et al., 2014), and can also be inferred from shorter wavelength C-band sensors onboard meteorological satellite missions (ASCAT, Figa-Saldaña et al., 2002). Finer-scale estimates can be obtained from current C-band SAR or optical sensors, and the upcoming NASA-ISRO L-band SAR (NISAR, Rosen and Kumar, 2021) and ESA High Priority Candidate Mission Radar Observation System for Europe in L-band (ROSE-L, Pierdicca et al., 2019) are expected to improve fine-scale soil moisture estimates.
There is currently no mission devoted to SWE, but various passive microwave sensors have been combined to produce coarse-scale SWE products (Luojus et al., 2021). The complexity of snow itself and its presence in complex terrain require more insight on how different types of radiation interact with snow to support the development of a dedicated mission (e.g., Ku and X-band) for fine-scale SWE observation. Multi-frequency missions such as the planned Copernicus Imager Microwave Radiometer (CIMR) will become relevant for SWE remote sensing in the future. Meanwhile, existing sensors have been used in an opportunistic way (e.g., snow depth from Sentinel-1 radar, Lievens et al., 2022), and upcoming missions such as NISAR and ROSE-L will further help to estimate high resolution SWE.
The water stored in vegetation is also not yet fully observed from space. Several optical vegetation indices (e.g., leaf area index) approximate the vegetation health and transpiration (Bannari et al., 2009). More recently, the microwave-based vegetation optical depth (VOD) products have shown promise to represent biomass, vegetation structure and water (Steele-Dunne et al., 2017; Chaubell et al., 2020; Wigneron et al., 2021). The upcoming BIOMASS Earth Explorer mission (Quegan et al., 2019) promises to explore long wavelength (P-band) measurements to estimate the total biomass in whole forest layers. Recent studies also aim at the estimation of plant transpiration from novel solar induced fluorescence (SIF) retrievals (Maes et al., 2020). The upcoming FLEX Earth Explorer mission (Drusch et al., 2017) will collect SIF data to serve agricultural purposes. Ultimately, advancing VOD and SIF-based retrievals and gaining insights in how vegetation affects microwave radiation or fluorescence will lead to better estimates of the water, carbon and energy cycle when combined with dynamic vegetation and crop yield modeling.
Spaceborne observation of water fluxes such as total ET and discharge remains a challenge. Intermittent satellite-based discharge estimates can be derived from optical and altimeter data (Abdalla et al., 2021; Tarpanelli et al., 2021). The Surface Water and Ocean Topography (SWOT) mission will soon enable frequent spaceborne observations of river stage for large rivers to allow inference of discharge (Biancamaria et al., 2016; Frasson et al., 2021). Currently, no mission is specifically dedicated to ET measurements (Fisher et al., 2017), and ET is most typically inferred from satellite-observed surface or skin temperature (related to sensible heat) as the residual of a simple energy balance model (Anderson et al., 2021), or indirectly obtained via soil moisture and VOD DA in a land surface model (Martens et al., 2017). Most high-resolution ET methods based on optical sensors suffer from low coverage (clear-sky conditions, low revisit times) and from large discrepancies among the various products. The ECOsystem Spaceborne Thermal Radiometer Experiment on Space Station (ECOSTRESS, Fisher et al., 2020) mission helped evaluating the use of thermal infrared observations at fine spatial and temporal resolutions to define future ET mission requirements. The future Land Surface Temperature Monitoring (LSTM, or Sentinel-8) and Thermal infraRed Imaging Satellite for High-Resolution Natural resource Assessment (TRISHNA, Roujean et al., 2021) missions promise to advance ET measurements in the coming decade. The quantification of other water fluxes, such as irrigation fluxes, from satellite observations is still in its infancy (Kumar et al., 2015; Massari et al., 2021; Dari et al., 2022).
Most DA systems use satellite observations that are directly related to land surface state variables (e.g., soil moisture, temperature, vegetation, snow) to improve subsequent state and flux forecasts. Conversely, the assimilation of satellite-based flux observations (e.g., ET, runoff, irrigation) is relatively less explored and limited to regional applications (Hartanto et al., 2017; Gavahi et al., 2020), because only a few global flux products are available (mainly ET) and they heavily depend on model background which might be inconsistent with the assimilation model. Furthermore, the diagnostic flux DA requires a careful design to link flux observations to prognostic state or parameter updates that are memorized in the system for improved forecasts. The latter can be achieved e.g., via selecting particles with a self-consistent combination of parameter, state and flux values in particle filters or via an adequate observation operator in Kalman filter-based techniques (Pauwels and De Lannoy, 2006).
New sensor technologies have not only helped to observe more variables, but also increased the resolution of data. For example, SAR data can regularly monitor soil moisture and surface water at km-scale resolution, albeit with more noise, longer revisit times or smaller coverage than coarser-scale data. Even if some (mainly commercial) sensors are indeed able to measure with high levels of detail, observations with meter-scale resolution are unlikely to make it into equally fine-scale land DA systems for global applications any time soon (Section Increased dimensions of future land DA: challenges and opportunities).
The level of satellite observation processing desirable for land DA is the subject of a debate that should strengthen the collaboration between geophysical retrieval and DA communities in the future. Land DA uses satellite observations either in the form of gridded radiances collected by the sensor or as the associated geophysical retrievals. Just like retrieval DA, radiance DA has been used to update the land surface state (examples below) and parameters in the land surface or radiative transfer model (Han et al., 2014). Radiance DA requires a forward model to relate the land surface state (soil moisture, temperature, snow, vegetation water content) and parameters (clay fraction, vegetation scattering albedo) to the satellite radiance signals as part of the observation operator (Reichle et al., 2014). The observation operator can also deal with the difference in spatial support of the observations and simulations in a multiscale DA system, e.g., to downscale coarse-scale observations to a finer resolution. Some studies report little DA skill difference between radiance and geophysical retrieval assimilation (De Lannoy and Reichle, 2016; Aires et al., 2021), and other studies show that radiance DA can circumvent biases associated with retrievals. For example, for deep mountain snow, SWE retrievals can be significantly biased (e.g., Wrzesien et al., 2017), but microwave radiance DA allowed both Li et al. (2017) and Kim et al. (2019) to achieve unbiased SWE estimates. Furthermore, DA of radiances facilitates the simultaneous updating of multiple state variables (e.g., soil moisture, temperature and vegetation) more elegantly than DA of the various associated individual retrieval products with cross-correlated errors. Radiance DA is also physically more self-consistent than retrieval DA, because retrievals are constrained by background information that may deviate from that of the model. E.g., soil moisture retrieval may use temperature information, and soil or vegetation parameters from data sources that are different from those of the model. The physical consistency makes radiance DA particularly attractive for coupled land-atmosphere DA (de Rosnay et al., 2022). Finally, the observation error characterization is more traceable for radiance DA. In the realm of DA algorithms, the use of (non-linear) observation operators enables solving DA as a non-linear optimization problem, without (or with limited) relying on linearity assumptions. In short, satellite observations should be provided along with good observation operators that can support land DA.
The spatio-temporal characterization of the observation error (that is, retrieval or instrument error, plus representativeness error) is a key element to successful DA systems. New sensor developments would thus ideally be preceded by a synthetic observing system simulation experiment (Crow et al., 2005) to quantify the tolerable levels of uncertainty for efficient DA. Furthermore, observations and model estimates typically have distinct biases, which are ideally resolved, explained, or removed prior to state updating (see Section DA methods and Land DA goals of the future: priorities). This requires that satellite missions span enough years to quantify climatological biases in observation space, and this has so far limited the use of short-lived exploratory missions onboard new platforms (e.g., drones, cubesats) in DA systems.
Models
The beauty of nature is that it is intelligible and can be captured in general physical laws, despite its complexity in the details. This knowledge is indispensable to add value to observations, and to inter- and extrapolate them to unobserved variables. In the last decades, a slow but steady increase in sophistication of large-scale land surface modeling and DA systems has been achieved (Fisher and Koven, 2020) by (i) improving model parameterizations (Balsamo et al., 2009) and resolving multiscale processes (Figure 2A), (ii) improving prognostic representations of hydrological processes, such as e.g., lateral subsurface flows in aquifers (Shrestha et al., 2014), snow processes (Bartelt and Lehning, 2002; Deschamps-Berger et al., 2022), peatland-specific processes (Bechtold et al., 2020), (iii) improving prognostic representations of vegetation (Clark et al., 2011), biogeochemical cycles including the nitrogen cycle (Oleson et al., 2013) and phosphorus cycle (Goll et al., 2017), (iv) activating anthropogenic processes, such as irrigation (Lawston et al., 2017), or by (v) land-atmosphere coupling (Figure 2B).
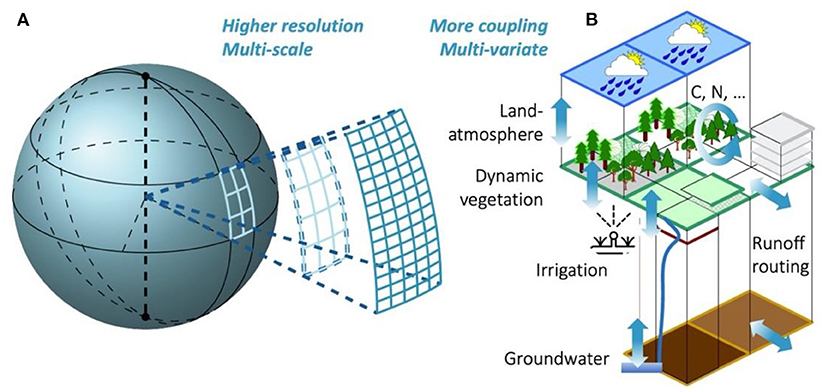
Figure 2. Land surface model sophistication increased in the 21st century toward (A) higher resolutions, by resolving multi-scale hydrological processes and improving model parameterizations, and (B) coupling of more processes, by replacing simplistic parameterizations and including more interactions between variables in multiple compartments.
By shifting from parameterized to physically resolved modeling (e.g., static parameterized to prognostic dynamic vegetation) and by coupling more processes, the DA impact of a single observation can reach more unobserved, but model-resolved, compartments. For example, snow depth DA can improve discharge and low-level atmospheric estimates (Griessinger et al., 2019; Rudisill et al., 2021; Lahmers et al., 2022), and backscatter DA can update dynamic vegetation and soil moisture, to eventually update irrigation (Modanesi et al., 2022). Efforts are ongoing to advance land DA in coupled land-atmosphere models (de Rosnay et al., 2014; Boussetta et al., 2015; Carrera et al., 2019; Reichle et al., 2021b) to make good on the promise to improve NWP and subseasonal to seasonal predictions (Kumar et al., 2022). As a matter of fact, the use of physics-based models has also been pivotal to the success of atmospheric DA in NWP to propagate information to unobserved areas (Kalnay, 2002). At the same time, several studies with current state-of-the-art land surface models also reported limited success (Crow et al., 2020; Hung et al., 2022) in propagating information from one compartment to another, which suggests that the modeling (parameterization) of the coupling and fluxes between land compartments as well as DA strategies need further research.
Surprisingly few new prognostic physics-based models (or model components) have been developed in response to the growing number of satellite data. This might be because we have reached the maximal desired structural complexity for large-scale applications, or because the coarse resolution of many satellite data integrates too much spatial variability, complicating a clean local physical interpretation of processes. As both model simulations and satellite observations become available at higher resolutions and for longer time spans, more spatial and temporal scales get resolved (Figure 2A). This might possibly deepen our process understanding, limit parameterizations and ultimately help hyper-resolution modeling (Wood et al., 2011) and DA.
Alternative ways of model development are emerging, which in fact have the potential to use the growing amount of (possibly coarse-scale) data and artificial intelligence rather than our human intelligence to build a model. Specifically, machine learning (ML) holds promise (Nearing et al., 2020) to develop models for multiple variables directly from multiple types of observations. e.g., ML can be used to diagnose how satellite-observed signals can be related to a set of land surface variables via complex interactions. Especially for microwave-based observation operators (Xue et al., 2018; Shan et al., 2022), ML might currently be more efficient than trying to fully understand and parameterize all radiation interactions. It is however unclear if ML is capable of entirely replacing prognostic land surface models in Earth system models, given that ML is not well-suited for non-stationary systems (e.g., under climate change), or to support the inference of unobserved land variables, because ML typically employs supervised learning that requires the existence of observations prior to training. More pragmatically and potentially more successfully, ML might complement physically based descriptions in a hybrid fashion (Reichstein et al., 2019). Note that in this subsection, ML is presented as a tool for model development. The following section discusses how ML can be used for DA.
Ideally, models offer a framework to propagate observations to unobserved variables, but models are imperfect, and their uncertainties originate from errors in the numerical schemes, unresolved scales, parameters, initial conditions, meteorological input (in offline systems) or missing processes. Via DA, the model state, parameters or forcing inputs will be updated to correct the model trajectory. If parameterizations are replaced with physically resolved process descriptions and the associated parameters would become physically measurable, then the need to update parameters should reduce in favor of more state updating. Similarly, when offline forcing inputs are replaced with coupled land-atmosphere modeling and constrained by atmospheric observations, then the need to update meteorological input in land surface models should reduce in favor of more state updating.
DA methods
The choice of DA method for a given application is arguably often driven by the research group's repository of source code, and it is rarely optimal in a mathematical sense (Carrassi et al., 2018). However, the discontinuity (e.g., via activation thresholds) and non-differentiability of land surface processes (including prognostic soil-water-vegetation-snow and diagnostic radiation interactions) is a valid reason to favor ensemble Kalman or particle-based techniques (Evensen et al., 2022), instead of variational methods that require model adjoints, which are difficult to obtain and maintain. Furthermore, ensemble- and particle-based DA can diagnose flow-dependent forecast error estimates for non-linear land surface models. Like in other areas of climate science, filters dominate operational land DA systems because they naturally support the sequential inclusion of satellite observations, provided they are available to describe an optimal current state for subsequent forecasts. For longer-term re-analysis solutions, or for slowly varying variables, smoothers (Dunne and Entekhabi, 2006; Margulis et al., 2015) gather observations over a sliding retrospective time window to obtain the best historical solution.
The key to any DA method is in the treatment of the forecast and observation errors. State estimation assumes random errors. In the ensemble Kalman filter (EnKF) or particle filter (PF), the distribution of the random forecast error accumulated between assimilation time steps is diagnosed from an ensemble of realizations, and ensemble generation is an art by itself (choice of perturbations, variable transformations to obtain Gaussianity, covariance inflation, localization; Carrassi et al., 2018). The observation errors are typically set to a constant standard deviation parameter that reflects the instrument or retrieval error, increased by the representativeness error that also includes observation operator error (Tijana et al., 2018). The forecast and observation error estimates are typically hyperparameters optimized by manual tuning of DA diagnostics (Reichle et al., 2017), because automated adaptive filtering (Crow and Reichle, 2008; De Lannoy et al., 2009) remains too inefficient. Most DA methods rely on the assumption of unbiased sources of information, and thus biases are typically removed prior to DA via, e.g., cumulative distribution function matching between the assimilated observations and the model simulations (Reichle and Koster, 2004; Kumar et al., 2012); consequently, DA analyses are consistent with the (potentially erroneous) model climatology. Ideally, biases are disentangled to estimate (De Lannoy et al., 2007; Pauwels et al., 2013) and possibly remove forecast or observation bias, or perhaps to identify the impact of water management or other human activity (e.g., unmodeled groundwater pumping and irrigation; Kumar et al., 2015; Girotto et al., 2017).
The nature of the errors associated with different land variables is very different. Figure 3 illustrates how soil moisture has a more bounded error growth than snow or vegetation, and that a single DA update reduces the forecast error for a longer time in variables with longer error autocorrelation lengths. More research is needed on how to best address these various types of errors for different variables via different DA methods and different bias treatments. For example, state-only updating without observation bias correction was advantageous to correct the accumulated snow and the associated river discharge in Smyth et al. (2019) and Lahmers et al. (2022), but in other studies, snow observation bias correction (De Lannoy et al., 2012; Liu et al., 2013) or bias correction to snowfall (Magnusson et al., 2016) was preferred. Similarly, Albergel et al. (2017) and Kumar et al. (2020) used a bias-blind filter for vegetation updating, but omitting bias correction for vegetation observations can possibly cause undesirable sawtooth timeseries and inferior ET and runoff estimates when assimilating intermittent observations, when the model is pushed out of its statistical equilibrium. The need for observation bias correction might depend on the boundedness of variables and the coupling between variables in different models, i.e., whether there is strong circular coupling equilibrium (vegetation-transpiration-soil moisture-vegetation) or rather a dominant one-way coupling (snowpack-discharge).
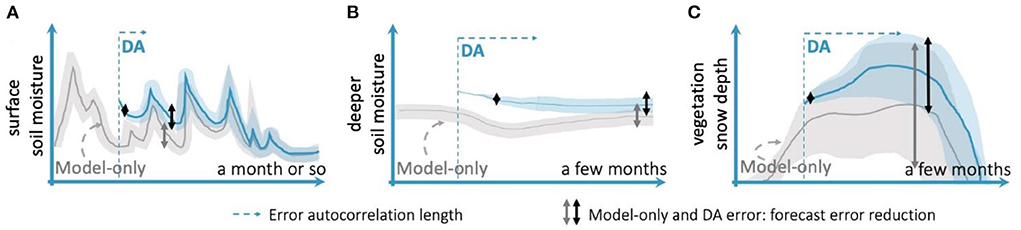
Figure 3. Different error characteristics of (A) surface soil moisture, (B) deeper soil moisture, and (C) vegetation or snow depth, for (gray) model-only and (blue) DA simulations. The semi-transparent plumes represent ensemble uncertainties.
Finally, most of the above considerations hold for DA in the traditional sense of merging physics-based model variables with satellite observations. New, data-driven methods such as ML offer an alternative to DA and can in some ways be similar to four-dimensional variational DA (by including the time dimension as in smoothers). Like DA (Geer, 2021), ML can be used to obtain better state estimates, bias estimates (Pan et al., 2021) or parameter estimates (Mudunuru et al., 2022). Novel hybrid DA-ML methods (Bonavita and Laloyaux, 2020) are showing success in discovering and emulating unresolved-scale processes, whenever a chronic lack of data makes the task extremely difficult for pure ML. In the context of coupled atmosphere-ocean modeling, DA-ML has shown promising results (Brajard et al., 2021) and its future use in a land-atmosphere context could be attractive.
Perspective on future DA development
Land DA goals of the future: Priorities
From its origin in atmospheric and ocean sciences, DA for state updating provides the best-possible initial conditions for subsequent forecasts. Properly estimating the initial state is critically important in chaotic systems (Carrassi et al., 2022), where small errors can grow exponentially in time and where the characteristics of such growth are themselves unpredictable. By contrast, land systems are usually asymptotically stable. Therefore, initial errors are typically internalized in the state (memory) until the system reaches an equilibrium after some time. Nevertheless, in a coupled land-atmosphere system, a small land initialization error could result in exponential error growth in the atmosphere. Seemingly small improvements obtained via land DA are therefore critical for NWP and seasonal predictions, provided the coupling mechanisms for long-term predictability are well represented. In land-only applications, state updating is essential to reset cumulative vegetation or snow variables for seasonal-scale yield or discharge forecasts, or to adjust soil moisture or input forcings for more accurate short-term hazard predictions of landslides (Felsberg et al., 2021), fires (Jensen et al., 2018), floods (Massari et al., 2018), and droughts (Li et al., 2019).
However, DA is not equally effective in all circumstances. For example, soil moisture updating can generally improve streamflow predictions (Mahanama et al., 2012; Reichle et al., 2021a), but might not be effective to reduce errors in the fast runoff component which are dominated by rainfall errors (Mao et al., 2020). Similarly, the influence of soil moisture on ET depends on the seasons, the coupling strength between soil moisture and ET in different climate regimes (Dong et al., 2020) and the ability of the assimilation model to accurately capture that coupling (Crow et al., 2020). To use data and resources most efficiently in a century when ever more data are becoming available (Section Increased dimensions of future land DA: challenges and opportunities), one should wonder which specific type of observations at what time and location has the largest impact on land DA analyses and beyond. A suggestion for future research is thus to explore targeted land DA. This requires that we first determine which type of observations are most useful to improve the forecast skill of particular land or atmosphere variables under the given circumstances, via sensitivity studies, forecast sensitivity-based observation impact studies, or coupling strength analyses. The land DA community can learn from the NWP community, which already has a strong grasp on how much various observations contribute to forecast skill (Eyre, 2021). Thereafter, we can efficiently assimilate those observations that likely have the most impact. The limitation is that satellite observations are collected in fixed orbits and not necessarily at the strategically most optimal location or time, so the main goal of targeting observations is a careful selection of the available observations.
Apart from state updating, satellite DA should be further explored for parameter estimation to (i) improve inherited static global soil and vegetation parameter databases that served older model generations, and to (ii) assign values to newly resolved parameters that will emerge from the sophistication of land surface models, e.g., to parameterize dynamic vegetation growth or water table dynamics. Parameter estimation is in principle possible using the same DA framework used for state estimation. Nevertheless, the success of parameter updating depends on the model sensitivity to that specific parameter and to its correlation with the observed quantities. The latter could be automatically estimated via the ensemble (for the EnKF) or the particles (for the PF), thus further promoting the use of this family of methods. Recently, hybrid EnKF-PF methods have been developed precisely to use the EnKF for the “more linear” state update and the PF for the “more non-linear” parameter updates (van Leeuwen et al., 2019). Those methods could prove effective in land DA as well. Finally, parameter updating is particularly relevant for long-term applications, because DA frameworks for state-only updating rely on the assumption of the system being autonomous and stationary and are thus not theoretically suitable if a system is subject to climate change or human activities that cause changes in the system's equilibrium. This is a broader issue for DA and goes well beyond the realm of land DA. Usually, the assumption is that by sequentially updating the system state and parameters we drive the conditional posterior probability toward the new equilibrium, yet rigorous mathematical results along these lines are still missing.
DA can be used to correct the state, parameters or forcings for unmodeled or poorly modeled processes, such as e.g., human activities. For example, Saharan dust deposited on snow should result in a sudden update of the parameterized or simulated albedo to ensure correct snow melt estimates. A forest fire, deforestation, land use change, or crop rotation within or across years (Boas et al., 2021) all require updates of vegetation model parameters or states. Such events will be followed by a gradual adjustment to a new soil moisture equilibrium both in the model and reality, but the transition time might differ, because some (unobserved) model parameters that determine the transition time are not in line with reality. The same is true for land systems in the presence of climate change, which might necessitate gradually changing model parameters. How to combine long-term updates for poorly modeled processes via parameter updating with short-term state updating should be explored in the future.
DA diagnostics of observation-minus-forecast and analysis-minus-forecast residuals allow an evaluation of the optimality of the DA system (Desroziers et al., 2005; Reichle et al., 2017). These diagnostics could in the future also help to identify (and improve) times and locations of poorly modeled processes, or system transitions from steady state to a new equilibrium.
DA aims at blending multiple sources of information seamlessly. However, in one-dimensional DA systems, no horizontal information propagation is achieved, which can result in artificial spatial patterns (e.g., swath edges or cloud screening imprinted in the DA analysis). When the land DA is coupled to an atmospheric model, such spatial discontinuities could lead to undesirable triggering of turbulence (Alapaty et al., 1997). Furthermore, only a part of the model variables might be included in the DA state vector. E.g., only a few soil moisture layers might be updated out of all soil-vegetation variables, or only the land variables and no atmospheric variables might be updated in a coupled DA system. To avoid unphysical discontinuities at the border between domains (e.g., land vs. atmosphere, or observed vs. non-observed land) or at the interface between variables, spatially distributed and multivariate DA methods are recommended, where multiple state variables of the land surface and coupled processes are updated.
As an extension of multivariate DA, the use of coupled DA is seen as another key area of desired DA development (de Rosnay et al., 2022). Strongly-coupled DA intends to inform one component of the climate system (e.g., the land) by using observations of the other (e.g., the atmosphere) and vice-versa (Penny and Hamill, 2017). This contrasts with the so called “weakly-coupled DA” in which the analysis update only affects the model compartment where data are collected, but then a coupled model is used in the forecast step. The model usually acts as a dynamical way of propagating information from the observed to the unobserved component, and weakly-coupled DA is usually developed first toward the ultimate goal of strongly-coupled DA. The spatio-temporal difference between processes in the coupled media (e.g., land-atmosphere) make it extremely difficult to construct a suitable error covariance across them (Tondeur et al., 2020). The sophistication of DA techniques will need to grow with a stronger coupling of the simulated water, energy and biogeochemical cycles (Baatz et al., 2021) in land surface, terrestrial ecosystem and atmospheric modeling and with the use of multivariate constraints across the various compartments of these coupled systems.
Increased dimensions of future land DA: Challenges and opportunities
Most visions for future land DA include multisensor DA (Durand et al., 2021), multivariate DA (Kumar et al., 2022), and multi-scale DA with a push toward finer resolutions. Our priorities above should be viewed against the backdrop of these foreseen developments, and here we highlight some associated opportunities. A multisensor approach is recommended to constrain more water cycle variables (Girotto et al., 2019) and obtain finer spatial and temporal resolutions, e.g., to benefit from the higher accuracy of coarse-scale observations and from the spatial detail in fine-scale observations (De Lannoy et al., 2012; Lievens et al., 2017). The use of multiple independent observations also has the potential to mitigate equifinality problems, i.e., to identify the state variable, input or parameter correction, or combination thereof, that results in the most effective constraint (e.g., particle selection). As discussed above, multivariate DA is needed for physical consistency and to reach more unobserved variables in more sophisticated systems. Higher-resolution (km-scale) DA systems promise to better resolve local land details for improved NWP and land-atmosphere reanalysis products. Higher resolutions for coupled land surface-subsurface models also better represent runoff processes at the hillslope scale, and narrow valleys with underlying groundwater bodies, which affect the simulation of ET (Shrestha et al., 2018). Furthermore, high-resolution estimates are needed for agricultural applications and hazard estimation.
From the viewpoint of system theory, these desires for high-resolution multivariate and multisensor DA translate into larger dimensions of state and observation error covariances, which necessitates practical and computationally affordable solutions. Larger updated state vectors require larger ensemble sizes to mitigate the sampling error in the ensemble-based error covariances, or beg for alternative solutions to partition the state into less-dependent groups of correlated variables that can be updated sequentially (thus making the ensemble covariance essentially block-diagonal). Indeed, the latter grouping of state variables conceptually mimics the idea of localization to filter out spurious error correlations in spatially distributed DA systems, and is also the essence of weakly-coupled DA systems.
Assimilating more observations from multiple sensors, multiple products, or high-resolution datasets increases the dimensions of the observation error covariance matrix. Spatially neighboring observations, or joint soil moisture and vegetation retrievals from the same microwave sensor, cannot be assimilated independently due to associated error correlations. Solutions can be found in directly assimilating radiances rather than multiple derived retrieval products, targeting only those observations that have most impact on the forecast (Section Land DA goals of the future: priorities), and thinning the observations (Waller et al., 2018). A second problem with assimilating multiple observations is that they each might have their own bias, represent something different than the model variables, and/or might cause contradicting updates (Girotto et al., 2019). Appropriate uncertainty estimates and bias removal partly solve this problem, and allow DA to update the temporal variability, while preserving the model's climatological water distribution as a strong constraint (Pan et al., 2012). Ideally, when observation biases get resolved and we find adequate ways to relate modeled and observed land estimates in absolute terms, then the multitude of observations should be used to also correct the modeled terrestrial water partitioning, and thereby create the correct climatological land conditions to support the correct coupling regimes.
Finally, using models, observations and DA at ever finer resolutions inevitably requires advanced computational infrastructure, more background information, e.g., on land surface processes, soil and land use parameters, high-resolution meteorological information (for off-line land simulations), and a DA method that can address the problem complexity (Carrassi et al., 2018). Furthermore, fine-scale estimates are by their nature more uncertain than the aggregated counterparts. In the future, we will have to balance the advantages of resolving more detail against the curse of dimensionality.
Conclusion
Satellite-based land DA is an interdisciplinary field of research that yields the most complete and consistent estimates of terrestrial water cycle variables. The growing amount of satellite data and the sophistication of modeling systems in the 21st century require efficient land DA systems to fuse observations and models into meaningful information for end users. Land DA can convert the intermittent swaths of satellite signals into temporally and spatially complete, gridded fields of soil moisture, snow or vegetation estimates and related variables, including land surface fluxes such as ET and runoff. By coupling the land with atmosphere or groundwater processes, and by resolving vegetation or snow parameterization schemes with physics-based processes, observations have the potential to update more unobserved variables, and to have an impact beyond the land surface. This is especially the case for NWP, crop monitoring, hazard (landslides, fires, floods, droughts) assessment, and carbon management.
Large, dynamical modeling systems that include more resolved or coupled processes require sophisticated DA techniques (perhaps supplemented with ML) to optimally distribute the observed information into improved estimates of the multivariate state, parameters, or boundary conditions. The exponential growth of satellite data will support improved constraints of the advanced modeling frameworks, but the growing dimensions in land DA will also necessitate the development of efficient DA algorithms. It will thus become increasingly important to select the most suitable levels of observation processing and the most impactful observations for assimilation, because not all observations are equally efficient all the time in DA systems. We can curb the growth of state and observation dimensions in the DA problem by considering targeted DA, rather than a mass integration of all data.
Author contributions
GDL developed the paper structure and wrote the initial draft. All co-authors contributed to the shaping of the paper contents and to the writing and editing of the paper. All authors contributed to the article and approved the submitted version.
Funding
This research is supported by Belspo EODAHR (SR/00/376), the European Commission, Horizon 2020 SHui (773903), FWO CONSOLIDATION (G0A7320N), ESA 4D-MED (4000136272/21/I-EF) and KU Leuven C1 (C14/21/057).
Acknowledgments
The authors thank editor Alex Sun and three reviewers for their constructive feedback.
Conflict of interest
All authors declare that the research was conducted in the absence of any commercial or financial relationships that could be construed as a potential conflict of interest.
The reviewer GB declared a past co-authorship with the author PR to the handling editor.
Publisher's note
All claims expressed in this article are solely those of the authors and do not necessarily represent those of their affiliated organizations, or those of the publisher, the editors and the reviewers. Any product that may be evaluated in this article, or claim that may be made by its manufacturer, is not guaranteed or endorsed by the publisher.
References
Abdalla, S., Kolahchi, A. A., Ablain, M., Adusumilli, S., Bhowmick, S. A., Alou-Font, E., et al. (2021). Altimetry for the future: building on 25 years of progress. Adv. Space Res. 68, 319–363. doi: 10.1016/j.asr.2021.01.022
Aires, F., Weston, P., de Rosnay, P., and Fairbairn, D. (2021). Statistical approaches to assimilate ASCAT soil moisture information: methodologies and first assessment. QJRMS 147, 1823–1852. doi: 10.1002/qj.3997
Alapaty, K., Raman, S., and Niyogi, D. (1997). Uncertainty in the specification of surface characteristics: a study of prediction errors in the boundary layer. Boundary-Layer Meteorol. 82, 475–502. doi: 10.1023/A:1017166907476
Albergel, C., Munier, S., Leroux, D. J., Dewaele, H., Fairbairn, D., Barbu, A. L., et al. (2017). Sequential assimilation of satellite-derived vegetation and soil moisture products using SURFEX v8.0: LDAS-Monde assessment over the Euro-Mediterranean area. Geosci. Model Dev. 10, 3889–3912. doi: 10.5194/gmd-10-3889-2017
Anderson, M. C., Yang, Y., Xue, J., Knipper, K. R., Yang, Y., Gao, F., et al. (2021). Interoperability of ECOSTRESS and Landsat for mapping evapotranspiration time series at sub-field scales. Remote Sens. Environ. 252, 112189. doi: 10.1016/j.rse.2020.112189
Asch, M., Bocquet, M., and Nodet, M. (2016). “Data assimilation: methods, algorithms, and applications,” in Society for Industrial and Applied Mathematics. p. 295. doi: 10.1137/1.9781611974546
Baatz, R., Hendricks-Franssen, H., Euskirchen, E., Sihi, D., Dietze, M., Ciavatta, S., et al. (2021). Reanalysis in earth system science: toward terrestrial ecosystem reanalysis. Rev. Geophys., 59, e2020RG000715. doi: 10.1029/2020RG000715
Balsamo, G., Viterbo, P., Beljaars, A. C. M., van den Hurk, B. J. J. M., Hirschi, M., Betts, A. K., et al. (2009). A revised hydrology for the ECMWF model: verification from field site to terrestrial water storage and impact in the Integrated Forecast System. J. Hydrometeorol. 10, 623–643. doi: 10.1175/2008JHM1068.1
Bannari, A., Morin, D., Bonn, F., and Huete, A. (2009). A review of vegetation indices. Remote Sens. Rev. 13, 95–120. doi: 10.1080/02757259509532298
Bartelt, P., and Lehning, M. (2002). A physical SNOWPACK model for the Swiss avalanche warning: Part I: numerical model. Cold Regions Sci. Technol. 35, 123–145. doi: 10.1016/S0165-232X(02)00074-5
Bechtold, M., De Lannoy, G., Reichle, R., Roose, D., Balliston, N., Burdun, I., et al. (2020). Improved groundwater table and L-band brightness temperature estimates for northern hemisphere peatlands using new model physics and SMOS observations in a global data assimilation framework. Remote Sens. Environ. 246, 111805. doi: 10.1016/j.rse.2020.111805
Biancamaria, S., Lettenmaier, D. P., and Pavelsky, T. M. (2016). The SWOT mission and its capabilities for land hydrology. Surv. Geophys. 37, 307–337. doi: 10.1007/s10712-015-9346-y
Boas, T., Bogena, H., Grunwald, T., Heinesch, B., Ryu, D., Schmidt, M., et al. (2021). Improving the representation of cropland sites in the Community Land Model (CLM) version 5.0. Geosci. Model Dev. 14, 573–601 doi: 10.5194/gmd-14-573-2021
Bonavita, M., and Laloyaux, P. (2020). Machine learning for model error inference and correction. Earth Space Sci. Open Archive 12, 36. doi: 10.1029/2020MS002232
Boussetta, S., Balsamo, G., Dutra, E., Beljaars, A., and Albergel, C. (2015). Assimilation of surface albedo and vegetation states from satellite observations and their impact on numerical weather prediction. Remote Sens. Environ. 163, 111–126. doi: 10.1016/j.rse.2015.03.009
Brajard, J., Carrassi, A., Bocquet, M., and Bertino, L. (2021). Combining data assimilation and machine learning to infer unresolved scale parametrization. Philosophical Trans. Royal Soc. A379, 20200086. doi: 10.1098/rsta.2020.0086
Carrassi, A., Bocquet, M., Bertino, L., and Evensen, G. (2018). Data assimilation in the geosciences: an overview of methods, issues, and perspectives. WIREs Clim. Change 9, e535. doi: 10.1002/wcc.535
Carrassi, A., Bocquet, M., Demaeyer, J., Grudzien, C., Raanes, P., and Vannitsem, S. (2022). Data assimilation for chaotic dynamics. Data Assimilation for Atmospheric, Oceanic and Hydrologic Applications IV, 1–42. doi: 10.1007/978-3-030-77722-7_1
Carrera, M., Belair, S., and Bilodeau, B. (2019). Assimilation of passive L-band microwave brightness temperatures in the Canadian land data assimilation system: Impacts on short-range warm season numerical weather prediction. J. Hydrometeorol. 20, 1053–1079. doi: 10.1175/JHM-D-18-0133.1
Chaubell, M. J., Yueh, S. H., Dunbar, R. S., Colliander, A., Chen, F., and Chan, S. (2020). Improved SMAP dual-channel algorithm for the retrieval of soil moisture. IEEE Trans. Geosci. Remote Sens. 58, 3894–3905. doi: 10.1109/TGRS.2019.2959239
Clark, D. B., Mercado, L. M., Sitch, S., Jones, C. D., Gedney, N., Best, M. J., et al. (2011). The Joint UK Land Environment Simulator (JULES), model description – Part 2: Carbon fluxes and vegetation dynamics. GMD 4, 701–722. doi: 10.5194/gmd-4-701-2011
Crow, W., Kustas, W., and Prueger, J. (2008). Monitoring root-zone soil moisture through the assimilation of a thermal remote sensing-based soil moisture proxy into a water balance model. Remote Sens. Environ. 112, 1268–1281. doi: 10.1016/j.rse.2006.11.033
Crow, W. T., Arroyo-Gomez, C., Muñoz-Sabater, J., Holmes, T., Hain, C. R., Lei, F., et al. (2020). Soil moisture/evapotranspiration over-coupling and L-band brightness temperature assimilation: sources and forecast implications. J. Hydrometeorol. 21, 2359–2374. doi: 10.1175/JHM-D-20-0088.1
Crow, W. T., Chan, S. T. K., Entekhabi, D., Houser, P. R., Hsu, A. Y., Jackson, T. J., et al. (2005). An observing system simulation experiment for Hydros radiometer-only soil moisture products. IEEE Trans. Geosci. Remote Sens. 43, 1289–1303. doi: 10.1109/TGRS.2005.845645
Crow, W. T., and Reichle, R. H. (2008). Comparison of adaptive filtering techniques for land surface data assimilation. Water Resour. Res. 44, W08423. doi: 10.1029/2008WR006883
Dari, J., Quintana-Seguí, P., Morbidelli, R., Saltalippi, C., Flammini, A., Giugliarelli, E., et al. (2022). Irrigation estimates from space: implementation of different approaches to model the evapotranspiration contribution within a soil-moisture-based inversion algorithm. Agric. Water Manag. 265, 107537. doi: 10.1016/j.agwat.2022.107537
De Lannoy, G., and Reichle, R. (2016). Assimilation of SMOS brightness temperatures or soil moisture retrievals into a land surface model. Hydrol. Earth Sys. Sci. 20, 4895–4911. doi: 10.5194/hess-20-4895-2016
De Lannoy, G., Reichle, R., Arsenault, K., Houser, P., Kumar, S., Verhoest, N., et al. (2012). Multiscale assimilation of advanced microwave scanning radiometer-EOS snow water equivalent and moderate resolution imaging spectroradiometer snow cover fraction observations in northern Colorado. Water Resour. Res. 48, W01522. doi: 10.1029/2011WR010588
De Lannoy, G., Reichle, R., and Pauwels, V. (2013). Global calibration of the GEOS-5 L-band microwave radiative transfer model over non-frozen land using SMOS observations. J. Hydrometeorol. 14, 765–785. doi: 10.1175/JHM-D-12-092.1
De Lannoy, G. J. M., de Rosnay, P., and Reichle, R. H. (2016). “Soil moisture data assimilation,” in Handbook of Hydrometeorological Ensemble Forecasting, eds Q. Duan, F. Pappenberger, J. Thielen, A. Wood, H. Cloke, and J. Schaake (Switzerland: Springer Verlag), 1–43. doi: 10.1007/978-3-642-40457-3_32-1
De Lannoy, G. J. M., Houser, P. R., Verhoest, N. E. C., and Pauwels, V. R. N. (2009). Adaptive soil moisture profile filtering for horizontal information propagation in the independent column-based CLM2.0. J. Hydrometeorol. 10, 766–779. doi: 10.1175/2008JHM1037.1
De Lannoy, G. J. M., Reichle, R. H., Houser, P. R., Arsenault, K. R., Verhoest, N. E. C., and Pauwels, V. R. N. (2010). Satellite-scale snow water equivalent assimilation into a high-resolution land surface model. J. Hydrometeorol. 11, 352–369. doi: 10.1175/2009JHM1192.1
De Lannoy, G. J. M., Reichle, R. H., Houser, P. R., Pauwels, V. R. N., and Verhoest, N. E. C. (2007). Correcting for forecast bias in soil moisture assimilation with the ensemble Kalman filter. Water Resour. Res. 43, W09410. doi: 10.1029/2006WR005449
de Rosnay, P., Balsamo, G., Albergel, C., Muñoz-Sabater, J., and Isaksen, L. (2014). Initialisation of land surface variables for numerical weather prediction. Surv. Geophys. 35, 607–621. doi: 10.1007/s10712-012-9207-x
de Rosnay, P., Browne, P., de Boisséson, E., Fairbairn, D., Hirahara, Y., Ochi, K., et al. (2022). Coupled assimilation at ECMWF: current status, challenges and future developments. QJRMS. 148, 2672–2702. doi: 10.1002/qj.4330
De Santis, D., Biondi, S., Crow, W., Camici, S., Modanesi, S., Brocca, L., et al. (2021). Assimilation of satellite soil moisture products for river ow prediction: an extensive experiment in over 700 catchments throughout Europe. Water Resour. Res. 57, e2021WR029643. doi: 10.1029/2021WR029643
Deschamps-Berger, C., Cluzet, B., Dumont, M., Lafaysse, M., Berthier, E., Fanise, P., et al. (2022). Improving the spatial distribution of snow cover simulations by assimilation of satellite stereoscopic imagery. Water Resour. Res. 58, e2021WR030271. doi: 10.1029/2021WR030271
Desroziers, G., Berre, J., Chapnik, B., and Poli, P. (2005). Diagnosis of observation, background and analysis-error statistics in observation space. QJRMS 131, 3385–3396. doi: 10.1256/qj.05.108
Dharssi, I., Bovis, K. J., Macpherson, B., and Jones, C. P. (2011). Operational assimilation of ASCAT surface soil wetness at the MetOfice. Hydrol. Earth Sys. Sci. 15, 2729–2746. doi: 10.5194/hess-15-2729-2011
Dong, J., Dirmeyer, P. A., Lei, F., Anderson, M. C., Holmes, T. R. H., Hain, C., et al. (2020). Soil evaporation stress determines soil moisture-evapotranspiration coupling strength in land surface modeling. Geophys. Res. Lett. 47, e2020GL090391. doi: 10.1029/2020GL090391
Drusch, M., Moreno, J., Del Bello, U., Franco, R., Goulas, Y., and Huth, A. (2017). The FLuorescence Explorer Mission Concept – ESA's Earth Explorer 8. IEEE Trans. GRS 55, 1273–1284. doi: 10.1109/TGRS.2016.2621820
Dunne, S., and Entekhabi, D. (2006). Land surface state and flux estimation using the ensemble Kalman smoother during the Southern Great Plains 1997 field experiment. Water Resour. Res. 42, 1–15. doi: 10.1029/2005WR004334
Durand, M., Barros, A., Dozier, J., Adler, R., Cooley, S., Entekhabi, D., et al. (2021). Achieving breakthroughs in global hydrologic science by unlocking the power of multisensor, multidisciplinary earth observations. AGU Adv. 2, e2021AV000455. doi: 10.1029/2021AV000455
Entekhabi, D., Yueh, S., O'Neill, P., and Kellogg, K. (2014). SMAP Handbook. Pasadena, CA, USA: JPL, 400–1567.
Evensen, G., Vossepoel, F., and van Leeuwen, P. J. (2022). Data Assimilation Fundamentals. (Switzerland: Springer). p. 243. doi: 10.1007/978-3-030-96709-3
Eyre, J. (2021). Observation impact metrics in NWP: a theoretical study. Part I: optimal systems. QJRMS 147, 3180–3200. doi: 10.1002/qj.4123
Fairbairn, D., Barbu, A., Napoly, A., Albergel, C., Mahfouf, J., and Calvet, J. (2017). The effect of satellite-derived surface soil moisture and leaf area index land data assimilation on streamflow simulations over France. Hydrol. Earth Sys. Sci. 21, 2015–2033. doi: 10.5194/hess-21-2015-2017
Felsberg, A., De Lannoy, G., Girotto, M., Poesen, J., Reichle, R., and Stanley, T. (2021). Global soil water estimates as landslide predictor: the efiectiveness of SMOS, SMAP and GRACE observations, land surface simulations and data assimilation. J. Hydrometeorol. 22, 1065–1084. doi: 10.1175/JHM-D-20-0228.1
Figa-Saldaña, J., Wilson, J. J. W., Attema, E., Gelsthorpe, R., Drinkwater, M. R., and Stoffelen, A. (2002). The advanced scatterometer (ASCAT) on the meteorological operational (MetOp) platform: a follow on for European wind scatterometers. Can. J. Remote Sens. 28, 404–412. doi: 10.5589/m02-035
Fisher, J. B., Lee, B., Purdy, A. J., Halverson, G. H., Dohlen, M. B., Cawse-Nicholson, K, et al. (2020). ECOSTRESS: NASA's next generation mission to measure evapotranspiration form the International Space Station. Water Resour. Res. 56, e2019WR026058. doi: 10.1029/2019WR026058
Fisher, J. B., Melton, F., Middleton, E., Hain, C., Anderson, M., Allen, R., et al. (2017). The future of evapotranspiration: global requirements for ecosystem functioning, carbon and climate feedbacks, agricultural management, and water resources. Water Resour. Res. 53, 2618–2626. doi: 10.1002/2016WR020175
Fisher, R., and Koven, C. (2020). Perspectives on the future of land surface models and challenges of representing complex terrestiral systems. JAMES 12, e2018MS001453. doi: 10.1029/2018MS001453
Frasson, R. P., de, M., Durand, M. T., Larnier, K., Gleason, C., Andreadis, K. M., Hagemann, M., et al. (2021). Exploring the factors controlling the error characteristics of the surface water and ocean topography mission discharge estimates Water Resour. Res. 57:e2020WR028519. doi: 10.1029/2020WR028519
Gavahi, K., Abbaszadeh, P., Moradkhani, H., Zhan, X., and Hain, C. (2020). Multivariate assimilation of remotely sensed soil moisture and evapotranspiration for drought monitoring. J. Hydrometeorol. 21, 2293–2308. doi: 10.1175/JHM-D-20-0057.1
Geer, A. (2021). Learning earth system models from observations: machine learning or data assimilation? Philosophical Trans. Royal Soc. A 379, 2194. doi: 10.1098/rsta.2020.0089
Girotto, M., De Lannoy, G., Reichle, R., Rodell, M., Draper, C., Banja, S., et al. (2017). Benefits and pitfalls of GRACE data assimilation: a case study of terrestrial water storage depletion in India. Geo. Res. Lett. 44, 4107–4115. doi: 10.1002/2017GL072994
Girotto, M., Musselman, K., and Essery, R. (2020). Data assimilation improves estimates of climate-sensitive seasonal snow. Curr. Clim. Change Rep. 6, 81–94. doi: 10.1007/s40641-020-00159-7
Girotto, M., Reichle, R., Rodell, M., Liu, Q., Mahanama, S., and De Lannoy, G. (2019). Multi-sensor assimilation of SMOS brightness temperature and GRACE terrestrial water storage observations for soil moisture and shallow groundwater estimation. Remote Sens. Environ. 227, 12–27. doi: 10.1016/j.rse.2019.04.001
Girotto, M., Reichle, R., Rodell, M., and Maggioni, V. (2021). Data assimilation of terrestrial water storage observations to estimate precipitation fluxes: a synthetic experiment. Remote Sens. 13, 1223. doi: 10.3390/rs13061223
Goll, D. S., Vuichard, N., Maignan, F., Jornet-Puig, A., Sardans, J., Violette, A., et al. (2017). A representation of the phosphorus cycle for ORCHIDEE (revision 4520). Geosci. Model Dev. 10, 3745–3770. doi: 10.5194/gmd-10-3745-2017
Griessinger, N, Schirmer, M., Helbig, N., Winstral, A., Michel, A., and Jonas, T. (2019). Implications of observation-enhanced energy-balance snowmelt simulations for runoff modeling of Alpine catchments. Adv. Water Resour. 133, 103410. doi: 10.1016/j.advwatres.2019.103410
Han, X., Hendricks Franssen, H. J., Montzka, C., and Vereecken, H. (2014). Soil moisture and soil properties estimation in the Community Land Model with synthetic brightness temperature data. Water Resour. Res. 50, 6081–6105. doi: 10.1002/2013WR014586
Hartanto, I. M., van der Kwast, J., Alexandridis, T., Almeida, W., Song, Y., van Andel, S., et al. (2017). Data assimilation of satellite-based actual evapotranspiration in a distributed hydrological model of a controlled water system. Int. J. Appl. Earth Observ. Geoinform. 57, 123–135. doi: 10.1016/j.jag.2016.12.015
Helmert, J., Sorman, A. S., Montero, R. A., Michele, C. D., de Rosnay, P., Dumont, M., et al. (2018). Review of snow data assimilation methods for hydrological, land surface, meteorological and climate models: results from a COST HarmoSnow Survey. Geosciences 8, 489. doi: 10.3390/geosciences8120489
Huang, J., Gomez-Dans, J. L., Huang, H., Ma, H., Wu, Q., Lewis, P. E., et al. (2019). Assimilation of remote sensing into crop growth models: current status and perspectives. Agric. Forest Meteorol. 276, 107609. doi: 10.1016/j.agrformet.2019.06.008
Hung, C. P., Schalge, B., Baroni, G., Vereecken, H., and Hendricks Franssen, H.-J. (2022). Assimilation of groundwater level and soil moisture data in an integrated land surface-subsurface model for southwestern Germany. Water Resour. Res. 58, e2021WR031549. doi: 10.1029/2021WR031549
Jensen, D., Reager, J. T., Zajic, B., Rousseau, N., Rodell, M., and Hinkley, E. (2018). The sensitivity of US wildfire occurrence to pre-seasonsoil moisture conditions across ecosystems. Environ. Res. Lett. 13, 014021. doi: 10.1088/1748-9326/aa9853
Jin, X., Kumar, L., Li, Z., Feng, H., Xu, X., Yang, G., et al. (2018). A review of data assimilation of remote sensing and crop models. Eur. J. Agronomy 92, 141–152. doi: 10.1016/j.eja.2017.11.002
Kalnay, E. (2002). Atmospheric Modeling, Data Assimilation and Predictability. UMD, 368. doi: 10.1017/CBO9780511802270
Kerr, Y., Waldteufel, P., Wigneron, J.-P., Delwart, S., Cabot, F., Boutin, J., et al. (2010). The SMOS mission: new tool for monitoring key elements of the global water cycle. IEEE 98, 666–687. doi: 10.1109/JPROC.2010.2043032
Kim, R. S., Durand, M., Li, D., Baldo, E., Margulis, S. A., Dumont, M., et al. (2019). Estimating alpine snow depth by combining multifrequency passive radiance observations with ensemble snowpack modeling. Remote Sens. Environ. 226, 1–15. doi: 10.1016/j.rse.2019.03.016
Kolassa, J., Reichle, R. H., Koster, R. D., Liu, Q., and Mahanama, S. (2020). An observation driven approach to improve vegetation phenology in a global land surface model. J. Adv. Model. Earth Sys. 12, e2020MS002083. doi: 10.1029/2020MS002083
Kumar, S., and Kolassa, J.. (2022). co-authors An agenda for land data assimilation priorities: Realizing the promise of terrestrial water, energy, and vegetation observations from space. JAMES (in review).
Kumar, S., Peters-Lidard, C., Santanello, J., Reichle, R., Draper, C., Koster, R., et al. (2015). Evaluating the utility of satellite soil moisture retrievals over irrigated areas and the ability of land data assimilation methods to correct for unmodeled processes. HESS 19, 4463–4478. doi: 10.5194/hess-19-4463-2015
Kumar, S., Reichle, R., Harrison, K., Peters-Lidard, C., Yatheendradas, S., and Santanello, J. (2012). A comparison of methods for a priori bias correction in soil moisture data assimilation. Water Resour. Res. 48, W03515. doi: 10.1029/2010WR010261
Kumar, S. V., Holmes, T. R., Bindlish, R., de Jeu, R., and Peters-Lidard, C. (2020). Assimilation of vegetation optical depth retrievals from passive microwave radiometry. Hydrol. Earth Sys. Sci. 24, 3431–3450. doi: 10.5194/hess-24-3431-2020
Lahmers, T. M., Kumar, S. V., Rosen, D., Dugger, A., Gochis, D. J., Santanello, J. A., et al. (2022). Assimilation of NASA's airborne snow observatory snow measurements for improved hydrological modeling: a case study enabled by the coupled LIS/WRF-Hydro system. Water Resour. Res. 57, e2021WR029867. doi: 10.1029/2021WR029867
Lahoz, W., and De Lannoy, G. (2014). Closing the gaps in our knowledge of the hydrological cycle over land: conceptual problems. Surv. Geophys. 35, 577–606. doi: 10.1007/s10712-013-9221-7
Larue, F., Royer, A., Seve, D. D., Roy, A., and Cosme, E. (2018). Assimilation of passive microwave AMSR-2 satellite observations in a snowpack evolution model over northeastern Canada. Hydrol. Earth Syst. Sci. 22, 5711–5734. doi: 10.5194/hess-22-5711-2018
Lawston, P. M., Santanello, J. A., Franz, T. E., and Rodell, M. (2017). Assessment of irrigation physics in a land surface modeling framework using non-traditional and human-practice datasets. Hydrol. Earth Syst. Sciences 21, 2953–2966. doi: 10.5194/hess-21-2953-2017
Li, B., Rodell, M., Kumar, S., Beaudoing, H. K., Getirana, A., Zaitchik, B. F., et al. (2019). Global GRACE data assimilation for groundwater and drought monitoring: advances and challenges. Water Resour. Res. 55, 7564–7586. doi: 10.1029/2018WR024618
Li, D., Durand, M., and Margulis, S. A. (2017). Estimating snow water equivalent in a Sierra Nevada watershed via spaceborne radiance data assimilation. Water Resour Res 53, 647–671. doi: 10.1002/2016WR018878
Lievens, H., Brangers, I., Marshall, H.-P., Jonas, T., Olefs, M., and De Lannoy, G. (2022). Sentinel-1 snow depth retrieval at sub-kilometer resolution over the European alps. The Cryosphere 16, 159–177. doi: 10.5194/tc-16-159-2022
Lievens, H., Reichle, R. H., Liu, Q., De Lannoy, G. J. M., Dunbar, R. S., Kim, S. R., et al. (2017). Joint Sentinel-1 and SMAP data assimilation to improve soil moisture estimates. Geophys. Res. Lett. 44, 6145–6153. doi: 10.1002/2017GL073904
Lievens, H., Tomer, S., Al Bitar, A., De Lannoy, G., Drusch, M., Dumedah, G., et al. (2015). SMOS soil moisture assimilation for improved hydrologic simulation in the Murray Darling Basin, Australia. Remote Sens. Environ. 168, 146–162. doi: 10.1016/j.rse.2015.06.025
Liu, Q., Reichle, R. H., Bindlish, R., Cosh, M. H., Crow, W. T., de Jeu, R., et al. (2011). The contributions of precipitation and soil moisture observations to the skill of soil moisture estimates in a land data assimilation system. J. Hydrometeorol. 12, 750–765. doi: 10.1175/JHM-D-10-05000.1
Liu, Y., Peters-Lidard, C. D., Kumar, S., Foster, J. L., Shaw, M., Tian, Y., et al. (2013). Assimilating satellite-based snow depth and snow cover products for improving snow predictions in Alaska. Adv. Water Resour. 54, 208–227. doi: 10.1016/j.advwatres.2013.02.005
Lu, Y., Steele-Dunne, S., and De Lannoy, G. J. M. (2020). Improving surface heat flux estimates by assimilation of SMAP brightness temperature and GOES land surface temperature data. J. Hydrometeorol. 21, 186–203. doi: 10.1175/JHM-D-19-0130.1
Lu, Y., Wei, C., McCabe, M., and Sheffield, J. (2022). Multi-variable assimilation into a modified AquaCrop model for improved maize simulation without management or crop phenology information. Agric. Water Manag. 266, 107576. doi: 10.1016/j.agwat.2022.107576
Luojus, K., Pulliainen, J., Takala, M., Lemmetyinen, J., Mortimer, C., Derksen, C., et al. (2021). GlobSnow v3.0 northern hemisphere snow water equivalent dataset. Nat. Sci. Data 8, 163. doi: 10.1038/s41597-021-00939-2
Maes, W., Pagan, B., Martens, B., Gentined, P., Guanter, L., Steppe, K., et al. (2020). Sun-induced fluorescence closely linked to ecosystem transpiration as evidenced by satellite data and radiative transfer models. Remote Sens. Environ. 249, 112030. doi: 10.1016/j.rse.2020.112030
Magnusson, J., Gustafsson, D., Husler, F., and Jonas1, T. (2014). Assimilation of point swe data into a distributed snow cover model comparing two contrasting methods. Water Resour. Res., 50, 10.1002/2014WR015302. doi: 10.1002/2014WR015302
Magnusson, J., Winstral, A., Stordal, A., Essery, R., and Jonas1, T. (2016). Improving physically based snow simulations by assimilating snow depths using the particle filter. Water Resour. Res. 53, WR019092. doi: 10.1002/2016WR019092
Mahanama, S., Livneh, B., Koster, R., Lettenmaier, D., and Reichle, R. (2012). Soil moisture, snow, and seasonal streamflow forecasts in the United States. J. Hydrometeorol. 13, 189–203. doi: 10.1175/JHM-D-11-046.1
Mao, Y., Crow, W., and Nijssen, B. (2020). Dual state/rainfall correction via soil moisture assimilation for improved streamflow simulation: evaluation of a large-scale implementation with Soil Moisture Active Passive (SMAP) satellite data. Hydrol. Earth Syst. Sci. 24, 615–631. doi: 10.5194/hess-24-615-2020
Margulis, S., Girotto, M., Cortes, G., and Durand, M. (2015). A particle batch smoother approach to snow water equivalent estimation. J. Hydrometeorol. 16, 1752–1772. doi: 10.1175/JHM-D-14-0177.1
Martens, B., Miralles, D. G., Lievens, H., van der Schalie, R., de Jeu, R. A. M., Frnandez-Prieto, D., et al. (2017). GLEAM v3: satellite-based land evaporation and root-zone soil moisture. Geosci. Model Dev. 10, 1903–1925. doi: 10.5194/gmd-10-1903-2017
Massari, C., Camici, S., Ciabatta, L., and Brocca, L. (2018). Exploiting satellite-based surface soil moisture for flood forecasting in the mediterranean area: state update versus rainfall correction. Remote Sens. 10, 292. doi: 10.3390/rs10020292
Massari, C., Modanesi, S., Dari, J., Gruber, A., De Lannoy, G., Girotto, M., et al. (2021). A review of irrigation information retrievals from space and their utility for users. Remote Sens. 13, 4112. doi: 10.3390/rs13204112
Modanesi, S., Massari, C., Bechtold, M., Lievens, H., Tarpanelli, A., Brocca, L., et al. (2022). Challenges and benefits of quantifying irrigation through the assimilation of sentinel-1 backscatter observations into noah-mp. Hydrol. Earth Sys. Sci. 2, 1–21. doi: 10.5194/hess-2022-61
Montzka, C., Moradkhani, H., Weiheermuller, L., Franssen, H.-J. H., Canty, M., and Vereecken, H. (2011). Hydraulic parameter estimation by remotely-sensed top soil moisture observations with the particle filter. J. Hydrol. 399, 410–421. doi: 10.1016/j.jhydrol.2011.01.020
Mucia, A., Bonan, B., Albergel, C., Zheng, Y., and Calvet, J.-C. (2022). Assimilation of passive microwave vegetation optical depth in LDAS-Monde: a case study over the continental USA. Biogeosciences 19, 2557–2581. doi: 10.5194/bg-19-2557-2022
Mudunuru, M., Cromwell, E., Wang, H., and Chen, X. (2022). Deep learning to estimate permeability using geophysical data. Adv. Water Resour. 167, 104272. doi: 10.1016/j.advwatres.2022.104272
Muñoz-Sabater, J., Lawrence, H., Albergel, C., de Rosnay, P., Isaksen, L., Mecklenburg, S., et al. (2019). Assimilation of smos brightness temperatures in the ecmwf integrated forecasting system. Q. J. R. Meteorol. Soc. 145, 2524–2548. doi: 10.1002/qj.3577
Nearing, G., Kratzert, F., Sampson, A., Pelissier, C., Klotz, D., Frame, J., et al. (2020). What role does hydrological science play in the age of machine learning. Water Resour. Res. 57, e2020WR028091. doi: 10.1029/2020WR028091
Oleson, K., Lawrence, D., Bonan, G., Drewniak, B., Huang, M., Koven, C., et al. (2013). Technical Description of Version 4.5 of the Community Land Model (CLM). NCAR Tech Rep. Note NCAR/TN-5031 STR, 422 pp., Natl. Cent. for Atmos. Res., Boulder, Colo.
Pan, B., Anderson, G., Gonclaves, A., Lucas, D., Bonfils, C., Lee, J., et al. (2021). Learning to correct climate projection biases. JAMES 13, e2021MS002509. doi: 10.1029/2021MS002509
Pan, M., Sahoo, A., Troy, T., Vinukollu, R., Shefield, J., and Wood, E. F. (2012). Multisource estimation of long-term terrestrial water budget for majorglobal river basins. J. Clim. 25, 3191–3206. doi: 10.1175/JCLI-D-11-00300.1
Pauwels, V. R. N., and De Lannoy, G. J. M. (2006). Improvement of modeled soil wetness conditions and turbulent fluxes through the assimilation of observed discharge. J. Hydrometeorol. 7, 458–477. doi: 10.1175/JHM490.1
Pauwels, V. R. N., De Lannoy, G. J. M., Hendricks Franssen, H.-J., and Vereecken, H. (2013). Simultaneous estimation of model state variables and observation and forecast biases using a two-stage hybrid Kalman filter. Hydrol. Earth Syst. Sci. 17, 3499–3521. doi: 10.5194/hess-17-3499-2013
Penny, S., and Hamill, T. (2017). Coupled data assimilation for integrated Earth system analysis and prediction. BAMS 98, ES169–ES172. doi: 10.1175/BAMS-D-17-0036.1
Pierdicca, N., Davidson, M., Chini, M., Dierking, W., Djavidnia, S., Haarpaintner, J., et al. (2019). The Copernicus L-band SAR mission ROSE-L (Radar Observing System for Europe) (Conference Presentation), Proc. SPIE 11154, Active and Passive Microwave Remote Sensing for Environmental Monitoring III, 111540E, doi: 10.1117/12.2534743
Quegan, S., Le Toan, T., Chave, J., Dall, J., Exbrayat, J. F., Ho Tong Minh, D., et al. (2019). The European Space Agency BIOMASS mission: measuring forest above-ground biomass from space, Remote Sens. Environ. 227, 40–60. doi: 10.1016/j.rse.2019.03.032
Rains, D., Lievens, H., De Lannoy, G., McCabe, M., de Jeu, R., and Miralles, D. (2022). Sentinel-1 backscatter data assimilation using a support vector regression and water cloud model at European sites. IEEE Geosci. Remote Sens. Lett. 19, 1–5. doi: 10.1109/LGRS.2021.3073484
Reichle, R., De Lannoy, G., Forman, B., Draper, C., and Liu, Q. (2014). Connecting satellite observations with water cycle variables through land data assimilation: Examples using the NASA GEOS-5 LDAS. Surveys of Geophysics 35, 577–606. doi: 10.1007/s10712-013-9220-8
Reichle, R., Liu, Q., Koster, R. D., Crow, W. T., De Lannoy, G. J. M., Kimball, J. S., et al. (2019). Version 4 of the SMAP level-4 soil moisture algorithm and data product. JAMES 11, 3106–3130. doi: 10.1029/2019MS001729
Reichle, R., Zhang, S., Liu, Q., Kolassa, J., and Todling, R. (2021b). Assimilation of SMAP brightness temperature observations in the GEOS land-atmosphere data assimilation system. IEEE J. Select. Topics Appl. Earth Observ. Remote Sens. 14, 10628–10643. doi: 10.1109/JSTARS.2021.3118595
Reichle, R. H. (2008). Data assimilation methods in the earth sciences. Adv. Water Resour. 31, 1411–1418. doi: 10.1016/j.advwatres.2008.01.001
Reichle, R. H., De Lannoy, G. J., Liu, Q., Koster, R. D., Kimball, J. S., Crow, W. T., et al. (2017). Global assessment of the SMAP level-4 surface and root-zone soil moisture product using assimilation diagnostics. J. Hydrometeorol. 18, 3217–3237. doi: 10.1175/JHM-D-17-0130.1
Reichle, R. H., and Koster, R. (2003). Assessing the impact of horizontal error correlations in background fields on soil moisture estimation. J. Hydrometeorol. 4, 1229–1242. doi: 10.1175/1525-7541(2003)004<1229:ATIOHE>2.0.CO;2
Reichle, R. H., and Koster, R. (2004). Bias reduction in short records of satellite soil moisture. Geophys. Res. Lett. 31, L19501.1–L19501.4. doi: 10.1029/2004GL020938
Reichle, R. H., Liu, Q., Ardizzone, J., Crow, W., De Lannoy, G. J. M., Dong, J., et al. (2021a). The contributions of gauge-based precipitation and SMAP brightness temperature observations to the skill of the SMAP Level-4 soil moisture product. J. Hydrometeorol. 22, 405–424. doi: 10.1175/JHM-D-20-0217.1
Reichstein, M., Camps-Valls, G., Stevens, B., Jung, M., Denzler, J., Carvalhais, N., et al. (2019). Deep learning and process understanding for data-driven earth system science. Nature 566, 195–204. doi: 10.1038/s41586-019-0912-1
Rodríguez-Fernández, N., de Rosnay, P., Albergel, C., Richaume, P., Aires, F., Prigent, C., et al. (2019). SMOS neural network soil moisture data assimilation in a land surface model and atmospheric impact. Remote Sens. 11, 1334. doi: 10.3390/rs11111334
Rosen, P., and Kumar, R. (2021). NASA0ISRA SAR (NISAR) mission status. IEEE Radar Conference. doi: 10.1109/RadarConf2147009.2021.9455211
Roujean, J. L., Bhattacharya, B., Gamet, P., Pandya, M. R., Boulet, G., Olioso, A., et al. (2021). TRISHNA: An Indo-French Space Mission to Study the Thermography of the Earth at Fine Spatio-Temporal Resolution, 2021 IEEE International India Geoscience and Remote Sensing Symposium (InGARSS), 49–52. doi: 10.1109/InGARSS51564.2021.9791925
Rudisill, W., FLores, A., and McNamara, J. (2021). The impact of initial snow conditions on the numerical weather simulation of a northern rockies atmospheric river. J. Hydrometeorol. 22, 155–167. doi: 10.1175/JHM-D-20-0018.1
Shan, X., Steele-Dunne, S. C., Huber, M., Hahn, S., Wagner, W., Bonan, B., et al. (2022). Towards constraining soil and vegetation dynamics in land surface models: modeling ASCAT backscatter incidence-angle dependence with a deep neural network. Remote Sens. Environ. 279, 113116. doi: 10.1016/j.rse.2022.113116
Shrestha, P., Sulis, M., Masbou, M., Kollet, S., and Simmer, C. (2014). A scale-consistent terrestrial systems modeling platform based on COSMO, CLM, and ParFlow. Monthly Weather Rev. 142, 3466–3483. doi: 10.1175/MWR-D-14-00029.1
Shrestha, P., Sulis, M., Simmer, C., and Kollet, S. (2018). Effects of horizontal grid resolution on evapotranspiration partitioning using TerrSysMP. J. Hydrol. 557, 910–915. doi: 10.1016/j.jhydrol.2018.01.024
Smyth, E. J., Raleigh, M. S., and Small, E. E. (2019). Particle filter data assimilation of monthly snow depth observations improves estimation of snow density and SWE. Water Resour. Res. 55, 1296–1311. doi: 10.1029/2018WR023400
Steele-Dunne, S. C., McNairn, H., Monsivais-Huertero, A., Judge, J., Liu, P.-W., and Papathanassiou, K. (2017). Radar remote sensing of agricultural canopies: A review. IEEE J. Select. Top. Appl. Earth Obs. Remote Sens. 10, 2249–2273. doi: 10.1109/JSTARS.2016.2639043
Tarpanelli, A., Camici, S., Nielsen, K., Brocca, L., Moramarco, T., and Beneviste, J. (2021). Potentials and limitations of Sentinel-3 for river discharge assessment. Adv. Space Res. 68, 595–606. doi: 10.1016/j.asr.2019.08.005
Tijana, J., Bormann, N., Bocquet, M., Carton, J. A., Cohn, S. E., Dance, S. L., et al. (2018). On the representation error in data assimilation. Q. J. R. Meteorol. Soc. 144, 1257–1278. doi: 10.1002/qj.3130
Tondeur, M., Carrassi, A., Vannitsem, S., and Bocquet, M. (2020). On temporal scale separation in coupled data assimilation with the ensemble kalman filter. J. Stat. Phys. 179, 1161–1185. doi: 10.1007/s10955-020-02525-z
Ulaby, F. T., Long, D. G., Blackwell, W. J., Elachi, C., Fung, A. K., Ruf, C., et al. (2014). Microwave Radar and Radiometric Remote Sensing. Michigan: University of Michigan Press. doi: 10.3998/0472119356
van Leeuwen, P. J., Kunsch, H., Nerger, L., Potthast, R., and Reich, S. (2019). Particle filters for high-dimensional geoscience applications: a review. QJRMS 145, 2335–2365. doi: 10.1002/qj.3551
Waller, J. A., García-Pintado, J., Mason, D. C., Dance, S. L., and Nichols, N. K. (2018). Technical note: Assessment of observation quality for data assimilation in flood models. Hydrol. Earth Syst. Sci. 22, 3983–3992. doi: 10.5194/hess-22-3983-2018
Wigneron, J.-P., Li, X., Frappart, F., Fan, L., Al Yaari, A., De Lannoy, G., et al. (2021). SMOS-IC data record of soil moisture and L-VOD: historical development, applications and perspectives. Remote Sens. Environ. 254, 1–20. doi: 10.1016/j.rse.2020.112238
Winstral, A., Magnusson, J., Schirmer, M., and Jonas, T. (2019). The bias-detecting ensemble: a new and efficient technique for dynamically incorporating observations into physics-based, multilayer snow models. Water Resour. Res. 55, 613–631. doi: 10.1029/2018WR024521
Wood, E., Roundy, J., Trou, T., van Beek, L., Bierkens, M., Blyth, E., et al. (2011). Hyperresolution global land surface modeling: meeting a grand challenge for monitoring earth's terrestrial water. Water Resour. Res. 47, W05301. doi: 10.1029/2010WR010090
Wrzesien, M., Kumar, S. V., Vuyovich, C. M., Gutmann, E., Kim, R., Forman, B., et al. (2022). Development of a nature run for observing system simulation experiments (OSSEs) for snow mission development. J. Hydrometeorol. 23, 351–375.
Wrzesien, M. L., Durand, M. T., Pavelsky, T. M., Howat, I. M., Margulis, S. A., and Huning, L. S. (2017). Comparison of methods to estimate snow water equivalent at the mountain range scale: a case study of the California Sierra Nevada. J. Hydrometeorol. 18, 1101–1119. doi: 10.1175/JHM-D-16-0246.1
Xia, Y., Hao, Z., Shi, C., Li, Y., Meng, J., Xu, T., et al. (2019). Regional and global land data assimilation systems: innovations, challenges, and prospects. J. Meteorol. Res. 33, 159–189. doi: 10.1007/s13351-019-8172-4
Xue, Y., Forman, B., and Reichle, R. (2018). Estimating snow mass in north America through assimilation of advanced microwave scanning radiometer brightness temperature observations using the catchment land surface model and support vector machines. Water Resour. Res. 54, 6488–6509. doi: 10.1029/2017WR022219
Keywords: data assimilation, soil moisture, snow, vegetation, microwave remote sensing, land surface modeling, targeted observations
Citation: De Lannoy GJM, Bechtold M, Albergel C, Brocca L, Calvet J-C, Carrassi A, Crow WT, de Rosnay P, Durand M, Forman B, Geppert G, Girotto M, Hendricks Franssen H-J, Jonas T, Kumar S, Lievens H, Lu Y, Massari C, Pauwels VRN, Reichle RH and Steele-Dunne S (2022) Perspective on satellite-based land data assimilation to estimate water cycle components in an era of advanced data availability and model sophistication. Front. Water 4:981745. doi: 10.3389/frwa.2022.981745
Received: 29 June 2022; Accepted: 24 August 2022;
Published: 16 September 2022.
Edited by:
Alex Sun, University of Texas at Austin, United StatesReviewed by:
Amen AL-YAARI, Université Paris-Sorbonne, FranceGianpaolo Balsamo, European Centre for Medium-Range Weather Forecasts, United Kingdom
Peishi Jiang, Pacific Northwest National Laboratory (DOE), United States
Copyright © 2022 De Lannoy, Bechtold, Albergel, Brocca, Calvet, Carrassi, Crow, de Rosnay, Durand, Forman, Geppert, Girotto, Hendricks Franssen, Jonas, Kumar, Lievens, Lu, Massari, Pauwels, Reichle and Steele-Dunne. This is an open-access article distributed under the terms of the Creative Commons Attribution License (CC BY). The use, distribution or reproduction in other forums is permitted, provided the original author(s) and the copyright owner(s) are credited and that the original publication in this journal is cited, in accordance with accepted academic practice. No use, distribution or reproduction is permitted which does not comply with these terms.
*Correspondence: Gabriëlle J. M. De Lannoy, Z2FicmllbGxlLmRlbGFubm95QGt1bGV1dmVuLmJl