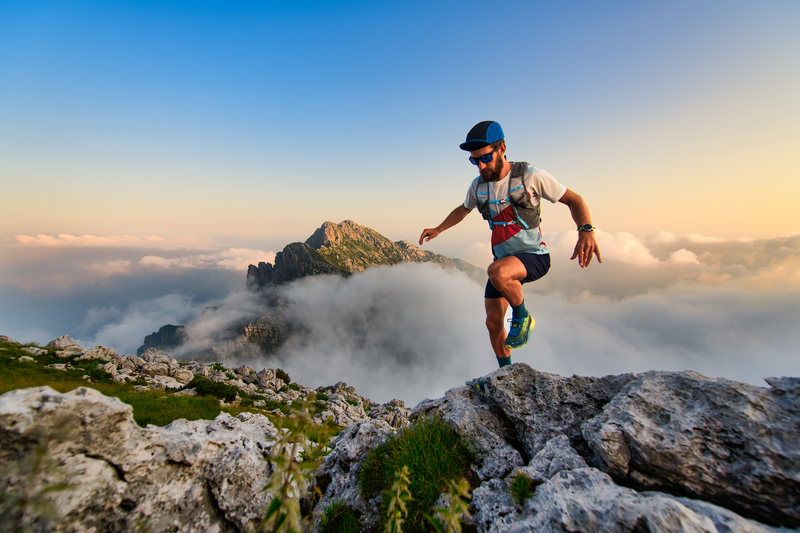
94% of researchers rate our articles as excellent or good
Learn more about the work of our research integrity team to safeguard the quality of each article we publish.
Find out more
ORIGINAL RESEARCH article
Front. Water , 08 February 2022
Sec. Water and Hydrocomplexity
Volume 4 - 2022 | https://doi.org/10.3389/frwa.2022.768898
This article is part of the Research Topic Modeling-based Approaches for Water Resources Problems View all 7 articles
Integrated Assessment Models (IAMs) were initially developed to inform decision processes relating to climate change and then extended to other natural resource management decisions, including issues around integrated water resources management. Despite their intention to support long-term planning decisions, model uptake has generally been limited, partly due to their unfulfilled capability to manage deep uncertainty issues and consider multiple perspectives and trade-offs involved when solving problems of interest. In recent years, more emphasis has been put on the need for existing models to evolve to be used for exploratory modeling and analysis to capture and manage deep uncertainty. Building new models is a solution but may face challenges in terms of feasibility and the conservation of knowledge assets. Integration and augmentation of existing models is another solution, but little guidance exists on how to realize model augmentation that addresses deep uncertainty and how to use such models for exploratory modeling purposes. To provide guidance on how to augment existing models to support decisions under deep uncertainty we present an approach for identifying minimum information requirements (MIRs) that consists of three steps: (1) invoking a decision support framework [here, Dynamic Adaptive Policy Pathways (DAPP)] to synthesize information requirements, (2) characterizing misalignment with an existing integrated model, (3) designing adjustable solutions that align model output with immediate information needs. We employ the Basin Futures model to set up the approach and illustrate outcomes in terms of its effectiveness to augment models for exploratory purposes, as well as its potential for supporting the design of adaptative pathways. The results are illustrated in the context of the Brahmani River Basin (BRB) system and discussed in terms of generalization and transferability of the approach to identifying MIRs. Future work directions include the refinement and evaluation of the approach in a planning context and testing of the approach with other models.
Supporting sustainable societies requires integrated approaches and informative decision support tools to manage the complexity and uncertainties associated with socio-ecological systems (SES). Among decision support tools, Integrated Assessment Models (IAMs) have been used as experimental laboratories to evaluate the response of existing systems to biophysical and anthropogenic changes (Loucks and Van Beek, 2017). They have been developed at various scales, from global scales that consider principally the effect of carbon emissions on climate change and its sectoral impacts, to local ones evaluating the costs and benefits of intervention options for managing a resource (e.g., Ackerman et al., 2009). At the river basin scale, they have been used to address water resources planning and management challenges (e.g., Jakeman and Letcher, 2003; Giupponi, 2006) and as experimental laboratories to “explore the linkages and feedbacks between different system components, including the social, economic, and ecological implications of different natural or anthropogenic factors” (Hamilton et al., 2015). It is that latter scale and use that is addressed here when considering IAMs.
Despite their intention to account holistically for multiple factors and optimally balance conflicting trade-offs associated with different uses of natural resources, model uptake has generally been limited (McIntosh et al., 2011). One reason may be their perceived limitations in providing relevant and actionable information to managers and decision makers, especially when considering long-term events, and misalignment with decision-maker perspectives (Van Voorn et al., 2016). An example of such limitations is illustrated by Balana et al. (2011) in their review of agri-environmental measures for implementation of the EU Water Framework Directive. Hence, expanding the capability of IAMs in the integration of diverse perspectives is expected to benefit the perceived quality of the information they provide and enhance their use (Bauler, 2012; Van Voorn et al., 2016).
Another challenge for IAMs is enhancing the consideration of deep uncertainty. Deep uncertainty has been defined as a situation in which various parties “do not know or could not agree on: a system and its boundaries; the outcomes of interest and their relative importance; the prior probability distribution of uncertain inputs to the system (Lempert et al., 2003; Walker et al., 2013). Moreover, deep uncertainty is also characterized by decisions that are made over time in dynamic interaction with the system and that cannot be considered independently (Hallegatte et al., 2012; Haasnoot et al., 2013).” In that situation, traditional approaches to planning, focused on identifying a best solution that maximizes identified beneficial objectives for a system, may fail to achieve their desirable outcomes. Conversely, an adaptive planning approach is considered more appropriate, aiming at providing more flexibility in planning decisions in the face of future uncertainties and at adapting alternatives along the planning horizon based on the monitoring of signals of changes (Loucks and Van Beek, 2017).
Different approaches to manage deep uncertainty are considered under the umbrella of Decision Making under Deep Uncertainty (DMDU) approaches, as described in Marchau et al. (2019). These approaches include: (1) robustness metrics that evaluate performance of decision choices across future conditions and/or scenario discovery to identify possible vulnerabilities, such as Robust Decision Making (RDM) (Lempert et al., 2006; Groves and Lempert, 2007), and Many Objective RDM (Kasprzyk, 2013). Decision Making under Deep Uncertainty approaches also emphasize: (2) methodologies to identify alternatives to prioritize under deep uncertainty, such as Info-Gap decision analysis (Ben-Haim, 2006), Engineering Options Analysis (De Neufville and Scholtes, 2011; Geltner and De Neufville, 2018); or with respect to easing the use of climate change projections for adaptation planning, Decision Scaling (Brown et al., 2012; Poff et al., 2016). Decision Making under Deep Uncertainty also involves: (3) tools to support the design of robust and adaptive plans, as seen in Adaptive Policy Making (Walker et al., 2001; Hamarat et al., 2013), and Dynamic Adaptive Policy Pathways (DAPP) (Haasnoot et al., 2013).
Dynamic Adaptive Policy Pathway is of particular interest in the context of this research, in providing guidance to design robust (i.e., able to remain effective under diverse future conditions) and adaptive plans through scenario exploration of adaptive pathways (i.e., sequences of actions including near term actions and long-term alternatives), making transparent path-dependencies between actions, and supporting the design of a monitoring system (Haasnoot et al., 2019). Additionally, DAPP has been applied to computational models to devise adaptation pathways (Kwakkel et al., 2015) and to support the design of a transdisciplinary modeling framework for model integration (Michas et al., 2020). Overall, the framework aims at managing different perspectives and uncertainties and supporting exploratory modeling approaches.
Methods to tackle deep uncertainty, including DAPP, tend to emphasize exploratory rather than consolidative modeling, that is, exploring assumptions and hypotheses rather than consolidating known facts (Moallemi, 2020). Exploratory models need to be able to explore multiple conflicting perspectives on a problem and possible solutions, and an existing model's vision may not align with those perspectives (Auping, 2018). Three approaches to consider multiple perspectives have been noted by Auping (2018): the definition of a “shared” and consensus representation for a system/problem of interest; the inclusion of all perspectives into a single conceptual representation; or the consideration of different models reflective of the diversity of perspectives to be explored. All approaches can also draw on participatory modeling (e.g., Voinov et al., 2018) and rapid prototyping (Soetaert and Meysman, 2012) in different ways. These approaches influence strategies for exploratory model design and integration of new features within modeling tools.
Auping (2018) delineated two model design strategies: developing a new model and reusing an existing model or its parts. The former approach leads to a single model with a broad scope to accommodate multiple perspectives whereas the latter is based on the consideration of a suite of models to represent different visions. Compared to developing a new model, model reuse is potentially more flexible to changes in worldviews in the context of composition of networks or workflows of models (Cetinkaya, 2010; Reichert and Weber, 2012), though this requires attention to interoperability of components in particular and orchestration of that task in general (Voinov and Shugart, 2013; Belete et al., 2017; Madni and Sievers, 2018). There is also a need to ensure sufficient model flexibility so as to adjust to user information needs (Cetinkaya, 2010; Reichert and Weber, 2012). Additionally, model reuse is expected to be more adaptable to the decision context and resource constraints, such as financial, expertise, analytical skills, and access to technology (Auping, 2018). It is also more inclined to preserve organizational knowledge assets (Chowdhury and Iqbal, 2004).
This research considers a third model design strategy, namely model augmentation. Compared to model reuse which implies repurposing or recomposition of models or model parts (Robinson et al., 2004), model augmentation builds on an existing model to add additional functionality according to information needs (Herlihy et al., 2019). This latter approach is grounded in ideas about augmentation of capabilities in autonomous systems. In a human augmentation paradigm, technologies, such as simulation modeling or system-thinking methodologies, augment human cognitive and learning performance, and associated boundaries of mental models, starting from an initial focus that places human actions at the center of changes in information systems (Sterman, 2006; Raisamo et al., 2019). Model augmentation continues this process by further augmenting those technologies to better meet the needs of those human actions.
Model augmentation can be seen as a hybrid between new model development and model reuse, which requires an orchestration framework to guide integration of new features into models (Belete et al., 2017). Building on Auping (2018), there is, however, still a need for guidance on how to augment existing models to support decisions under deep uncertainty. It appears that a framework like DAPP could be used to orchestrate deep uncertainty management and model integration and augmentation for exploratory modeling uses. Specifically, DAPP provides a stepwise process that prescribes both decision-making needs and required model capabilities, which may or may not be aligned with those of an existing model.
The purpose of this research is then to provide guidance on how to augment existing models to support decisions under deep uncertainty. It presents an approach for identifying minimum information requirements (MIRs) that consists of three steps: (1) invoking a decision support framework, DAPP here, to synthesize information requirements; (2) characterizing misalignment with an existing integrated model; and (3) designing adjustable solutions that align model output with immediate information needs. The values and contribution of this approach lie in its ability to consider and better integrate human-model perspectives and to provide guidance for augmenting existing models for exploratory modeling uses, which remains a major challenge for model integration and reuse (Auping, 2018). In effect, the novelty of this research lies in the use of a DMDU framework, DAPP, as an orchestration framework for model augmentation for exploratory modeling purposes.
We use the Basin Futures tool, a new IAM for surface water and water management policy assessment, as an example for setting the MIRs approach, and illustrate its effectiveness in augmenting the model for exploratory modeling purposes in an illustrative case study inspired from water resources management and development in the Brahmani River Basin (BRB). The case is described as “hypothetical” as it does not directly involve stakeholders in the BRB problem framing stage and evaluation of the adaptation pathways, the purpose of the research being to illustrate general principles for model augmentation.
The next section describes the methods and tools used. Results demonstrate key aspects of the approach and illustrate its ability to augment the Basin Futures software for exploratory modeling and the design of adaptation pathways. The generalizability of the approach to identify MIRs is then discussed in light of the results and consideration of possible future applications in a real-life decision-making context.
Basin Futures (BF) is a new cloud-based platform developed by CSIRO to holistically assess river basin surface water resources worldwide, with a major focus on food production and irrigation developments. Its user-friendly interface allows users to set up a basin model and create water resource development and management scenarios under population growth, historical climate conditions and climate change scenarios. Users can explore the impacts of water transfers, multi-purpose dams, environmental flows, irrigation developments, and changes in domestic and industrial (D&I) water demands, among others. Results are presented as average values, time-series, and visual representations, for variables such as irrigated crop incomes or mean monthly runoff timeseries and can be easily downloaded in spreadsheet format for further analytical uses. Basin Futures can be used to provide an initial picture of the state of water resources in a basin and summaries of changes to its water resources under different socioeconomic developments and management actions (mostly infrastructural). Finer analysis, such as considering more distributed modeling tools, field study or stakeholder engagement approaches, can then be realized prior to further investments and design of a plan. More details about the tool can be found in O'Sullivan et al. (2020) and Taylor et al. (2017, 2021).
Dynamic Adaptive Policy Pathways is a robust decision-making approach that combines features from Dynamic Adaptive Planning (Walker et al., 2001) and Adaptive Pathways (Haasnoot et al., 2013) methodologies. At the core of the approach is the identification of Adaptive Tipping Points (ATPs), which describe conditions (and expected timings) in which a solution may fail to achieve its purpose (Kwadijk et al., 2010). Additionally, the approach supports the on-going monitoring of adaptation pathways and their implementation based on the provision of signposts (indicators) and triggers (critical threshold values). These signposts and associated triggers aim to provide adaptivity to the plan by allowing for the consideration of fit-for-purpose adaptive actions (i.e., actions aiming at achieving specific performance objectives for a system), as well as mitigating or reducing to an acceptable level other risks for the plan using contingency actions (including provisions for the revision of the plan) (Hermans et al., 2017). Adaptation pathways therefore describe when and under what conditions actions need to be implemented, and help cope with future uncertainties by providing adaptive pathways that can change over time. Another interest of DAPP is its ability to support the active participation of stakeholders in problem framing, results analysis activities and decision making through the communication of adaptive pathways as “metro maps” visualizing opportunities to switch between actions over time. Along with the map, the performance of each adaptive pathway is summarized (in monetary and/or non-monetary units) in a scorecard (Lawrence et al., 2019). Broader details of the DAPP framework are provided in Haasnoot et al. (2019) and its main steps are summarized in Figure 1.
Figure 1. DAPP framework (adapted from Haasnoot et al., 2019).
The first step aims at defining the problem by specifying boundaries for the system of interest for analysis purposes, identifying vulnerabilities (exogenous and intrinsic to the system) and setting performance criteria for the system and conditions of success. This step is followed by the identification of the conditions of occurrence and expected timing of ATPs for the system and for plausible adaptive actions (steps 2 and 3). Next, adaptive pathways to achieve a primary objective for the system are designed and their impact on the performance of other objectives for the system is summarized in a scorecard (step 4). Pathways presenting acceptable results and allowing for plan flexibility are selected to constitute an adaptive plan (steps 5 and 6). A monitoring plan is designed to consider the timing for implementing adaptation actions (or not), based on the ongoing evaluation of signposts and triggers. Additionally, conditions to consider contingency actions or the re-evaluation of the plan are defined at that stage (step 7). For the present hypothetical case study, only steps 1–4 are within scope.
To identify information requirements specific to DAPP, we begin by comparing the steps and associated content of DAPP and its parent frameworks. This step highlights where DAPP differs from traditional systems approaches and provides the basis for us to emphasize the difference in our approach.
Next, the problem or system of interest, herein the BRB system (see Section Illustrative Case Study for Applying the DAPP-IAM Method—The Brahmani River Basin), is conceptualized from two perspectives: a user and the Basin Futures modeling tool; the latter perspective corresponds to the developers' or development team's vision for their tool. Here, we define stakeholders as both developers and users of models. Users could include data analysts, consultants, policy makers, local communities, as well as individuals and groups involved in the modeling design process. As for developers, they include programmers, and a development inclusive of developers and stakeholders involved in the modeling design process. As shorthand, we refer to the development team as developers. In the present research, we assume that the modeler's vision for the BRB system is representative of relevant users' vision for the basin. While this means that this illustrative case study does not meet the stakeholder engagement ideals of DAPP, we highlight that the study still involves a collaborative setting with interaction between developers and users of the original Basin Futures tool, where the users are responsible for the DAPP implementation and model augmentation task.
A comparison of conceptual representations from different perspectives is conducted that allows for the identification of information misalignments and, hence, the identification of salient (relevant) information to be represented in the tool. In addition, the comparative approach allows for the identification of information required to execute key steps of the DAPP framework in order to construct adaptation pathways. A heuristic approach is then used to identify solutions for representing the missing and/or partially represented information in the tool. Such a heuristic approach is based on the application of rules of thumb to systematically solve a problem of interest and identify plausible solutions (Smith, 1990). This approach therefore aims to address representation and technical gaps in the model to design adaptation pathways.
Considering different solutions implies an understanding of the tool structure and behavior and hence a collaborative setting. In this research context, that involved collaboration between modelers and developers, and was instrumental in the definition and setup of the approach. Involving broader stakeholder groups will be important in applied contexts, but for the purpose of evaluating our proof-of-concept, their contribution to the conceptual representation of BRB system is approximated based on our understanding of the literature and expert validation of the conceptual model.
The BRB is in Northeast India and covers about 39,268 km2 encompassing three State Governments: Odissa (57%), Jharkhand (39%), and Chhattisgard (4%). The climate is tropical, sub-humid with most of the precipitation occurring during the monsoon season from June to September. The Brahmani River is formed by the convergence of the Sankh and South Koel River systems in the upper region and flows into the Bay of Bengal. Ecologically, about a third of the basin is covered by forest, mostly located in the central region of the basin. Among protected areas, the Bhitakanika Mangrove ecosystem in the Deltaic region is recognized as a Ramsar wetland, and is of critical importance to support local livelihoods and biodiversity. Socio-economically, the population in the basin is mostly rural and relies on rainfed and irrigation crop productions, especially rice, for food and income. Rainfed agriculture is predominant in the basin, except in the deltaic region in the state of Odissa where irrigation plays a major role (Mainuddin et al., 2016). Additionally, the basin is rich in mineral resources which have contributed to its industrial and urban development, especially in the State of Odissa (ICID, 2005).
When considering issues in the basin, climate variability is influential on streamflow regimes and surface water availability. Flood hazards associated with the confluence of the Mahanadi, Baitarani, and Brahmani Rivers in the Deltaic region of the basin are threatening economic outcomes and livelihoods. Drought events are also a threat for water security and availability in the basin, especially under future climate change scenarios for which the frequency of low flows is expected to increase (Vandana et al., 2019; Swain et al., 2020). Water quality in the basin is also another source of concerns. Effluent discharges from urbanization, industrialization, and mining, and agricultural intensification are responsible for water pollution, endangering human, and ecosystems' health (ICID, 2005; Vandana et al., 2019). Finally, the development of major and medium storage projects, mostly for irrigation purposes, are modifying flow regimes and impacting riverine, estuarine, and coastal ecosystems. To support aquatic system needs, dam operations include water allocations for the environment corresponding to 30% of their inflow from June to September and 20% in the other months (Pollino et al., 2016). Among the main storages in the basin, the Rengali Dam in the middle catchment in the State of Odissa is critical to ensuring energy security, irrigation supply and providing flood protection to downstream regions (ICID, 2005).
The basin model setup using BF is presented in Figure 2. It includes seven reaches split partially or in their entirety between the three State Governments.
Figure 2. Representation of the BRB system and its seven reaches in Basin Futures. The numbers identify the reaches to parameterize and associated outputs from the tool. The upstream state of Jharkhand is represented by reaches 1–4; the downstream state of Odissa by reaches 5–7.
For simplification purposes, we assume that the State of Chhattisgarh, due to its small area within the catchment, would have little influence on the BRB's water developments, and that reaches in the model could be grouped with those in the State of Jharkhand (reaches 1–4) and the State of Orissa (reaches 5–7).
Information from the ICID (2005) and Pollino et al. (2016) were used to frame the problem, set a vision for the basin, and identify main vulnerabilities and plausible actions, as well as parameterize the exploratory scenarios to develop adaptation pathways.
As described in the method, the approach to the model augmentation adopted involved firstly identifying the information requirements of a framework (here, DAPP) for comparison against the capabilities of the existing model (here, BF). The DAPP framework follows a systems approach such that its information requirements include those similar to other systems approaches in addition to more distinctive requirements. Within a systems approach, DAPP emphasizes and makes explicit requirements for problem framing (Table 1), notably the definition of success/failure criteria and conditions for implementing actions (including lead-time).
Table 1. Summary of DAPP information requirements in the problem framing step, distinguishing additional requirements from those common to other systems approaches to planning.
The first step of DAPP aims at articulating a problem at stake to be able to conceptualize it. It implies defining a “shared” vision. For this study, this is “to support rural incomes and industrialization in the basin to exit poverty without hindering the population and ecological systems' health as well as ensuring population security from flood events in the Deltaic region.” Five objectives are considered: rural incomes, urban incomes, population health, ecological system health, and flood security. Conditions of success for the objectives are presented in the Supplementary Material together with their respective models (defined as mathematical formalisms mimicking the behavior of a feature or process of interest). As for external factors influential on the BRB system, they consist of climate change and socioeconomic uncertainties (increased global socioeconomic inequalities, impacting urbanization growth rate in addition to population growth in the basin, and associated demand for irrigated crop products). Such uncertainties are grouped into future scenarios to be analyzed simultaneously and are assumed to impact the two State Governments differently. For illustration purposes, we have focused on actions (levers) aiming to address the rural incomes objective, which are further described in the Supplementary Material, together with their conditions of implementation. They consist of infrastructural types (proposed medium and major dams or in-stream water storages), economic (irrigation developments), and demand management (on-farm water storages, effluent treatment by 20 or 50%, environmental flow increase, irrigation application efficiency improved to 80%).
Two conceptual representations of the BRB system were deduced: from a user perspective and the modeling tool's perspective. The comparison of the two representations allows for the identification of misalignments in perspectives and information gaps or partial representation in the tool (Figure 3; Supplementary Material).
Figure 3. Comparison of the conceptual representations of the BRB from a user perspective and the Basin Futures tool's perspective.
In addition to identifying information misalignments between user-model perspectives, the conceptual representation also allows one to identify variables that could be used as signposts to monitor ATPs, as directly or indirectly influenced by exogenous factors and levers. For example, surface water availability is indirectly sensitive to climate change and socioeconomic impacts on D&I and irrigation water demands, as well as on levers (infrastructural, economic, and demand management) aiming at achieving the rural incomes objective (see the associated model in the Supplementary Material). We assume that the supply reliability could therefore be used as a signpost to monitor ATPs and their expected timing of occurrence (see model in Supplementary Material).
Finally, the conceptual model also suggests what types of robust metrics/performance signposts to monitor when assessing the impact of plausible adaptation pathways on different objectives. For the rural incomes objective, we consider the average net benefit per rural inhabitant (US$/capita) as one of our performance indicators. The indicator implies that costs and benefits from rainfed, irrigated, and fisheries production need to be estimated. As BF does not consider costs, and only irrigation production benefits, additional information is required to monitor the performance of some objectives.
Following the identification of information gaps, the next step identifies solutions to re-align visions. Figure 4 presents our heuristic approach to guide reflection about “what” information is missing, “why” this information is important, and “how” to implement it within the modeling tool.
Figure 4. Heuristic approach to identifying minimum information requirements. Two types of solutions are considered, for the model (yellow) and for the plan (green). Black arrows are considered as solution identification paths to integrate in the model. Dashed arrows are indicative of solution pathways requiring additional attention when designing the adaptive plan, based on the consideration of extra-measures in situations where relevant information is partially, indirectly, or not represented at all.
The first step of the approach aims to answer the “what” question and is linked to the identification of information gaps in the model. This question is immediately followed by the “why” question (“Do I need this information?,” “why is it important?”), which aims to identify the level of salience of the missing information. In the illustrative case, this level is based on the modeler's perception of importance and on understanding of the BRB system. The next steps of the framework are associated with the “how” question and the methodologies/solutions that could be used to augment the model, both in terms of representation of the BRB system and ability to identify ATPs, design adaptation pathways, and monitor the performance of these pathways. Three major types of solutions could be considered, associated with different levels of abstraction and implications for the developers: assumption-based, calculation-based, and implementation-based. In addition, the choice of a solution is based on its (perceived) credibility (scientific soundness and validity) and legitimacy (ability to solve a problem at stake).
Assumption-based solutions (“Can it be extrapolated from the model's existing parameters and indicators?”) are considered to have the highest level of abstraction involving behavioral assumptions and new uses of primary features of the model (input parameters, existing datasets, indicators). The solution is non-intrusive as it does not involve direct changes to the existing model, with little implications for the developers. Validation of the solution is based on expert consultation (including developers) and its selection depends on user's assessment of whether it satisfies immediate information requirements to help address a problem. For example, to represent water treatment in the model, the use of the D&I water return parameter has been modified to account for water treatment. We assume that the proportion of water returned to surface water is representative of the proportion of treated D&I effluent only. For a treatment of D&I effluent by 20%, the water return parameter was set to 16%, considering that 80% of water extracted for D&I use is not consumed. In the case of no treatment, the parameter was set to 0%. The credibility of this assumption-based solution in this context was validated by experts, and considered sufficient to illustrate the effectiveness of the approach for model augmentation for exploring future uncertainties and to design adaptation pathways.
Calculation-based solutions (“Can it be extrapolated from the model data and timeseries?”) are considered to involve an intermediate level of abstraction; they attempt to more realistically represent a feature of interest and its associated behavior. Models deriving from this type of solution are loosely linked to the modeling tool, and in our case make use of the modeling tool's outputs (timeseries, data values, and estimates) as new inputs for these models. This type of solution was by far the most used in this research, to represent information perceived as necessary to address the illustrative case problem. The main information gaps were associated with missing components in the modeling tool (e.g., the definition of the rainfed agriculture model to estimate crop production based on mean monthly rainfall and evapotranspiration timeseries) and indicators to estimate the value of different objectives. To support exploratory modeling and the adaptation of DAPP, subcomponent models were developed that use BF results as inputs, e.g., Different demand and supply mean monthly timeseries feeding into a supply reliability component for each transient scenario to identify conditions of ATPs for different actions. Compared to assumption-based solutions, validation criteria are associated with peer literature and expert validation of the chosen indicator/method of estimation used. Additionally, expert feedback was necessary to ensure the proper definition of the model and setting.
Implementation-based solutions (“If requiring changes in the model, is it feasible for developers?”) are considered to have the lowest level of abstraction with direct changes to the model integrating a new component to represent a feature of interest and its behavior. On the other hand, this type of solution is highly dependent on availability and willingness of developers to integrate a new feature directly within the modeling tool. Validation is expected based on the developers' application of their validation and verification framework. As for legitimacy, it is based on both the user's necessity to have a finer representation of a feature of interest to solve a problem and the developers' perception of relevance for the tool, interest for its uses, and feasibility.
More than one of these solutions may be possible and could be chosen according to preferences and the feasibility of options. Also, a solution could include a mix of solutions. For example, for estimating the supply reliability indicator for identifying the expected timing of ATPs for different actions, climate change datasets have to be implemented in the tool (implementation-based solution), together with global socioeconomic changes associated with more global inequalities (assumption-based solution, using population number and urban growth rate as proxies) and livestock drinking requirements (calculation-based solution, based on the estimation of livestock growth rate and daily drinking requirements and their addition to existing monthly timeseries from the tool).
If no solutions can be found, the implications for the plan need to be weighed and the associated risk addressed by contingency actions. This stage is not considered in the present research, as we focus on model augmentation for designing and evaluating adaptation pathways. It is however acknowledged that the implications of solution choices need to be considered when designing an adaptive plan and associated monitoring system.
Examples of solution strategies used in the context of BF and the BRB system are presented in the Supplementary Material with their respective models.
We consider a planning horizon of 50 years for evaluation purposes, considered sufficient based on existing long-term planning simulations of 25 years in the BRB system (ICID, 2005). Each transient scenario represents a time step of 10 years, except for period 1 (from 1961 to 2005) and period 2 (from 2006 to 2010). This difference arises from the time periods of historical (1961–2005) and future (2006–2100) climate change datasets available in BF.
To identify ATPs for the system and alternatives (steps 2 and 3 in DAPP), we use a single signpost: water supply reliability (see Supplementary Material for details about the model used). We assumed that this signpost was sufficient to monitor ATPs and expected timing for all our actions in this context, considered either alone or in combination. The ATP signpost was considered as a proxy for rainfed agriculture and fisheries production. This reflects the assumptions that the available runoff is the remaining value after rainfed production uses, and that fisheries harvesting and irrigation will be equally impacted by D&I uses. Based on these assumptions, annual reliability values under an 85% threshold were considered unsatisfactory. Failure was considered to occur with more than 2 years under the acceptable threshold over 10 years. The expected timing of ATPs is linked to the time range associated with its respective transient scenario. Figure 5 presents some of the results obtained. The full table is provided in the Supplementary Material.
Figure 5. Examples of ATPs results and associated expected timing (black) for actions, alone or in combination. Results are presented at the basin scale and the states scale. Absence of failure are displayed in green. Two future scenarios are considered: under increased global socioeconomic inequalities and drier climate conditions (cc1) and similar global socioeconomic conditions and wetter climate (cc2). The mean annual runoff is provided under both future conditions. Highlighted in yellow are runoff conditions for which some actions did not fail. Numbers in brackets = time range for each transient scenario. Notations are as follows: hist, current situation; ofs, on-farm water storages; wwt20, treatment of 20% of D&I effluents; wwt50, treatment of 50% of D&I effluents; irr2, irrigation developments associated with the completion of ongoing and proposed dams; eflow, environmental flows; bau2, implementation of proposed dams.
When considering actions alone, only on-farm water storages (ofs) are robust enough under both future scenarios and our planning horizon. To ensure that the signpost used to monitor ATPs was sensitive enough, we consider other different combinations of actions, excluding ofs that are expected not to fail over our planning horizon. Over the resulting 548 possible combinations, only the ones systematically including both water treatment and the implementation of proposed dams were able to display different responses based on future scenarios.
Considering administrative scales highlights an uneven response to future uncertainties across our actions. Generally, the upstream state (Jharkhand) appears to benefit more from different combinations of actions, under both future scenarios, from 2021 onward. As for the state of Odissa, differences in the occurrence of ATPs are more visible, with conditions of failure alternating with conditions of success for some combination of actions, generally associated with climate conditions and a mean annual runoff above 480 mm/year. Such a variability in robustness is linked to the use of climate change datasets and non-monotonic fluctuations in value over time.
Following the identification of ATPs, the next step (step 4 of DAPP) consists of sequencing plausible actions into adaptation pathways. Sequencing rules that were considered are two-fold: (1) forbid scale back of some interventions, such as water treatment (e.g., shifting from 50–20% treatment), and (2) remove pathways that would be hardly or never reached (e.g., sequences without ofs and not including both water treatment and the implementation of proposed dams were excluded). Transitions from an action alone or a combination of actions to another alternative (consisting of new single action or a combination of actions) were based on the consideration of the time range associated with different transient scenarios.
In addition, selection rules for plausible adaptation pathways were based on achieving acceptable outcomes for rural incomes per capita, and for two out of four of the remaining objectives (except for urban density ratio). Acceptable values for the four objectives are: urban incomes (hydropower ratio > 0), ecological health (blue water scarcity including ecological requirements, bws > 0.4), population health (green blue water availability, gbws ≥ 1), and flood security (flood hazard index or fhi < value in period 1). The definition of such rules led to 5,000 plausible adaptation pathways. Figure 6 illustrates some of the pathways obtained and their performance.
Figure 6. Example of plausible adaptation pathways for the BRB system. (A) Metro map for selected adaptative pathways and their associated scorecards under the two future scenarios: global socioeconomic increase of inequalities under (B) drier climate (cc1) and (C) wetter climate (cc2). Adaptation pathways in the scorecards are symbolized by colored circles. Each color represents a corresponding action in the metro map. Numbers refer to the timing of transition and implementation (+ sign) of a solution. Basin and administrative scales are both represented. Sparklines depict the normalized score for each objective. Average values for the objective across the transient scenarios constitutive of a pathway are provided next to their corresponding sparkline. Yellow boxes identify the baseline situation in the absence of implementation of additional actions. Notations are as follows: bws, blue water scarcity index modified to include ecological water needs, and used as a proxy for aquatic ecosystems health; gbws, green blue water scarcity index, used as a proxy for population health; fhi, flood hazard index, used as proxy for flood security; urban density ratio and hydropower ratio are used as proxies for urban incomes—the former is indicative of urban poverty for positive values, and the latter is used as a proxy for industrial and services increased benefits based on energy supply and for positive values; bau1, current situation with ongoing dams completed in 2020; ofs, on-farm water storages; wwt20, treatment of 20% of D&I effluents; wwt50, treatment of 50% of D&I effluents; irr2, irrigation developments associated with the completion of ongoing and proposed dams; eflow, environmental flows; bau2, implementation of proposed dams.
Four adaptation pathways including ofs are displayed in the metro map. We considered two scorecards for each climate scenario separately due to their effect on ATPs. Performance of an adaptation pathway was calculated as the average across its constituent time periods. Fluctuations in each objective over time are visualized using sparklines. Finally, a baseline associated with the objectives' performance in the absence of changes compared to the current situation was added for contrast.
The first observation from the results is that pathways considering ofs alone were not sufficient to achieve our conditions of selection. In all our ofs-based pathways, improving irrigation application efficiency (eff ) to 80% was necessary in period 6. The next observation is the utility of sparklines to reflect on the implications of future trends for an objective, e.g., under cc1, the rural incomes objective appears to decrease starting from period 3, which is associated with reduced availability of suitable land. The sparklines also display direct or indirect effects associated with the implementation of adaptation pathways. For example, compared to the baseline, a change in shape in sparklines for fhi suggests a direct effect of the interventions on the objective. Also, a change in magnitude, such as for hydropower ratio, suggests potential direct or indirect effect of the adaptation pathways on the objective.
When comparing average values to the baseline and when considering different scales, uneven impacts of the pathways on objectives can be observed. For example, the implementation of these adaptation pathways appears to increase incomes in the upstream state of Jharkhand and to reduce them for the downstream one, with potential implications in terms of conflicts between the two states. Moreover, if no changes in average values compared to the baseline are observed, adaptation pathways may have no impact on an objective, as is the case for urban density ratio. As for the null value for hydropower ratio for the state of Jharkhand, it can be explained by the absence of hydropower projects.
It is noteworthy that in a model augmentation setting, the fitness for purpose of the indicators needs to be kept in mind when interpreting the results. For example, bws values increase with the implementation of adaptation pathways, which could be attributed to the pathways themselves, or to the proxy used to monitor aquatic ecosystem health which reflects increase in consumptive water use. If the proxy is no longer deemed sufficient, this would lead to another round of model augmentation, perhaps requiring greater investment to meet additional information requirements.
Finally, when comparing the average values between adaptation pathways for an objective, some of the results may not be significantly different, suggesting redundant pathways, as for pathways introducing effluent treatment (wwt20) at different time periods. This preliminary analysis could suggest simplifying the options considered—or discussion of impacts of treatment that need to be included in the next iteration.
The illustrative study of the BRB system showcases how different case-specific augmentations to BF have been able to meet requirements to monitor ATPs and design adaptation pathways, providing a demonstration of the MIRs approach to support model augmentation for exploratory modeling analysis.
The first steps in our analysis led to the identification of information requirements specific to DAPP and linked to the problem framing stage. In addition, other information requirements that are common to the frameworks underlying DAPP have implications in terms of information requirements to identify ATPs, build adaptation pathways, and design an adaptive plan and associated monitoring system (the latter point not being investigated in this research). These information requirements were critical to ensure the adaptation of the DAPP framework to the modeling tool for exploratory modeling purposes. In addition, specificities of DAPP associated with the problem framing stage have been emphasized while developing the approach and contributed to the identification of information misalignments between human-model perspectives and the definition of our heuristic approach to identify solutions. Lastly, the adaptation of solutions to BF allows for the evaluation of the robustness of various (combinations of) actions, design, and selection of different plausible adaptation pathways.
The following discussion considers our results and their potential for generalization, focusing on three main points: the choice of a robust and adaptive framework, our approach to identify misalignments and solutions, and our choice of signposts and decision rules when considering ATPs and plausible adaptation pathways.
Contrasting DAPP to the two frameworks at its origin and to traditional approaches to planning indicates that specificities of DAPP are to be found in its emphasis and clarification of the tasks to perform in order to adequately frame a problem. The strong emphasis on problem articulation supports the conceptualization of a system or problem of interest and clarification of the causal relationships between key components. In addition, DAPP more generally provides a stepwise approach to execute different tasks to design adaptation pathways. These characteristics of DAPP bestow some similarities with framework-based activities for orchestrating model integration (Belete et al., 2017), which was a reason for its selection.
Information requirements for DAPP are not expected to be generalizable—they remain specific to the framework and its main purpose to design adaptation pathways. If another DMDU framework was considered to orchestrate model augmentation for exploratory modeling purposes, it is expected that new MIRs would need to be defined specific to those frameworks. For example, when considering RDM approaches, information requirements in the problem framing stage emphasize the identification of vulnerabilities to be used to execute the scenario discovery process (Kwakkel et al., 2016). Information requirements for scenario discovery would involve: (1) data inputs from model simulations about uncertain factors and plausible actions to generate a database used to identify vulnerable scenarios; (2) the selection of appropriate scenario discovery algorithms (either statistical and/or data-mining algorithms) to iteratively identify vulnerable scenarios; and (3) diagnostic tools for evaluating the significance of the parameter constraints proposed by scenario discovery algorithms (Bryant and Lempert, 2010). Compared to DAPP, which uses the results from the exploratory model to design adaptation pathways, RDM may be more demanding in terms of information requirements as it relies on sufficient exploration of different model runs to allow for the discovery of vulnerable scenarios and evaluation of the robustness of actions.
Potentially, according to the framework(s) invoked, model and information complexity may limit the extent to which a model could be augmented and reused. According to (Voinov and Shugart, 2013), with increasing information requirements and the inclusion of more building blocks to the model, one major risk of model integration is that it increases the difficulty of analysis and interpretation of model outputs and propagation of uncertainty through the augmented model. One solution to integration complexity is to consider methodological approaches that ensure a proper balance between the levels of detail and resolution of information and model behavior and internal consistency, which could be achieved through collaborative modeling (Voinov and Shugart, 2013; Basco-Carrera et al., 2017). Such a collaborative modeling setting implies the opening of the “black box” of model development to the policy community and the development of “active, iterative, and inclusive” interactions between developers and users to address concerns and information needs (White et al., 2010).
The issue of integration complexity has been addressed to some extent in the present research. As mentioned by (Voinov and Shugart, 2013), it requires the identification of appropriate levels of abstraction and the consideration of different stakeholder perspectives. Two main steps are considered: the identification of information gaps or misalignments with the mental models of users, and the identification of solutions with an appropriate level of abstraction to bridge information gaps. Both approaches have implications for model credibility, salience, and legitimacy and the ability of boundary organizations to effectively manage iterative knowledge co-production between various actors to create purposeful IAM (White et al., 2010).
The identification of information gaps is based on contrasting two conceptual representations of a system of interest: a user one and a model one, the latter representative of developers' vision for their tool's purpose and its representation of a system. Contrasting representations aim at making explicit what is considered salient information for different actors and their degree of alignment. It implies the consideration of two learning loops: a single loop related to understanding a model architecture and behavior, and a second loop involving the clarification of mental models and questioning assumptions, cognitive rules and objectives (Pahl-Wostl, 2009). The single loop implies collaborative interactions between users and developers to “open the black box” of a modeling tool and clarify questions associated with model architecture and behavior. As for the second loop, it implies the use of communication tools, such as system-thinking diagrams, to translate concerns and information needs and reconcile mismatches between knowledge suppliers and users (White et al., 2010).
Based on the identification of mismatches, the next step involves the use of a heuristic approach to guide systematic reflection on effective means to represent information gaps in the tool. Three reflection stages are considered: (1) identifying information gaps (“what is missing?”); (2) considering the degree of salience of that information (“do I need that information?”, “why is it important?”); and (3) reflecting on the types of solutions that could be considered (“how could they be better represented?”). Answering the “how” question led to the consideration of four solutions, each of them having implications in terms of levels of abstraction (credibility, salience), and legitimacy.
Assumption-based solutions seek to virtually integrate a new feature, without leading to direct or indirect changes to the model. An advantage of this is the generality of the solutions (e.g., effluent treatment could involve infrastructural and/or ecological measures), thereby providing a creative window for users to identify relevant solutions, including non-conventional ones. This enhances the credibility of the information provided by models as well as on the inclusion of new knowledge as it becomes available (Etzioni, 2001).
For calculation-based solutions, we use a feed forward approach wherein the modeling tool's outputs act as input for loosely integrated models. Such an approach is considered less demanding in terms of information requirements to ensure the consistency and interoperability of loosely coupled models (Belete et al., 2017). As long as tight feedbacks do not need to be modeled, other advantages of loosely coupled models compared to tightly coupled ones include more flexibility, creation of new capabilities, and cost reduction, together with creating the illusion of an integrated system (Rothenberg, 2008). In addition, such a structure can better account for changing information requirement needs of users (Madni and Sievers, 2014). Coupling models generally implies the identification of a common core (e.g., Auping, 2018). In our case, the hydrological component of the modeling tool was the bridging factor for model component integration.
Central to integrating models, and in this case calculation-based solutions and associated models, validation and verification (V&V) are required to ensure the credibility of the augmented model. A qualitative V&V was considered sufficient in our case, based on expert feedback and peer review literature to identify solutions. Additionally, the observation of changing values for the model results in response to changing parameters was considered sufficient to validate the proper integration of the type of solutions (Madni and Sievers, 2018; Voinov, 2018). In a real-world planning context, a quantitative approach to evaluating the validity of the chosen solutions and proper implementation may be necessary depending on the stage of maturity of the planning process. However, in situations of deep uncertainty, especially when there is volatility in perspectives, traditional approaches to model structure and behavior V&V may be challenged. Such challenges and approaches to V&V are detailed in Auping (2018) in the context of model integration for exploratory modeling purposes based on the use of system dynamics models but these extend to other modeling paradigms. Future applications in real decision-making contexts of the approach could explore the implications of quantitative V&V in terms of information requirements and model augmentation for exploratory purposes.
Concern for V&V in the application process is expected to be lower in the case of implementation-based solutions as they are expected to be checked by developers during the development process. However, more rigidly coupled solutions tightly integrated in the existing model architecture may reduce model flexibility and adaptiveness (Madni and Sievers, 2014). In addition, limitations in terms of compatibility in codes or standards, among others, may occur in relation to the integration framework used to orchestrate the inclusion of new modules into the tool (Voinov and Shugart, 2013; Belete et al., 2017). Finally, rigidly coupled models may increase resource costs for the developers to integrate the new solution, test and validate it, and document changes, security, interfacing, among others (Madni and Sievers, 2014). With respect to legitimacy, the choice of implementation-based solutions implies a shared understanding and agreement between users and developers, and therefore a collaborative setting (Voinov and Shugart, 2013). In our case, this type of solution was considered in situations where the user could not easily apply the two other types of solutions and/or when needing the model to represent a feature of interest and associated behavior more realistically (e.g., climate change datasets to allow for model response to future climate and hence for future exploration). The fact that a collaborative setting was in place with the developers of BF has facilitated the communication of information needs, and discussion of constraints and advantages of representing that information in the tool, together with identifying acceptable solutions for both parties.
The residual uncertainty in meeting information requirements has implications in terms of risk management for a plan, which have not been addressed in the present research. Different approaches to communicate uncertainty could be considered in future applications, for example by making explicit residual uncertainties and their anticipated mitigation and impact (Guillaume et al., 2012), or considering a different set of visualization tools describing the uncertainty space of inputs (Woodruff et al., 2013) or of output uncertainty (Fu et al., 2015). Clear communication about uncertainty is expected to reduce issues of information quality and improve the uptake of models and associated outputs (Hudson-Doyle et al., 2018).
The proposed approach to reflecting on misalignments and identifying solutions or “areas of transformative changes,” can be considered to support a triple-loop learning process (Pahl-Wostl, 2009), feeding into the inner loops of learning about the system and actions to be taken. Such a process applied to model augmentation has received more consideration in recent years in the machine learning (ML) and artificial intelligence (AI) field. According to Seidel et al. (2019), there is a need for autonomous tools to reflect human–ML interaction processes, as the perception of the purposefulness of a tool will always be dependent on human “mental models.” They suggest the consideration of triple-loop learning approaches to achieve alignment of mental models and respective learning between humans and autonomous tools. Our heuristic approach involves similar steps as for human–ML triple learning loops and has similar implications in terms of considering a solution-driven approach to model augmentation and alignment with changing mental models (Seidel et al., 2019).
Overall, our heuristic approach to identify misalignments and solutions is expected to be generalizable, as it is based on common principles of model development and DMDU frameworks, notably problem framing and conceptualization, and formulation. In the context of model augmentation these tasks are tackled, respectively, by comparing user and model conceptualizations and identifying augmentation solutions to meet information needs.
The heuristic approach to model augmentation also provides the flexibility needed to enhance user learning and the capability to solve a problem of interest, by making incremental adjustments to account for different perspectives and associated levels of abstraction (levels of details) for the solutions (Auping, 2018). The assumption-based solutions are reflective of higher levels of abstraction and less detailed solutions, albeit still considered sufficient to solve a problem. Calculation-based solutions are more intermediate, with a higher level of detail but not to the point of directly representing a function or process (e.g., using proxies). As for implementation-based solutions, their choice is guided by the need to have a clear picture of the behavior of a process or function of interest. In that case, a high level of detail is considered, requiring agreement between users and developers on a solution that involves direct changes to the modeling tool.
The use of incremental solutions of varying complexity encourages an ordering of solutions that tries to meet user needs firstly by model reuse, progressing to more costly changes to model structure and behavior only when the need for the change is established. This is aligned with the idea of incremental learning, notably emphasized in the ML field, and identifying fit-for-purpose solutions for solving incremental learning tasks (Luo et al., 2020). Such incremental learning assumes on-going adaptation of models to learning (Luo et al., 2020) and allows for rapid feedback when evaluating planning assumptions and the identification of potential areas to explore prior to considering further investments (Fu et al., 2020; Zare et al., 2020). In addition, it assumes a flexible structure when considering solutions, with implications for model interoperability (Madni and Sievers, 2014), especially when considering implementation-based solutions.
This section investigates the transferability of MIRs for some components of DAPP, focusing on the signposts to monitor ATPs, robust metrics to evaluate the performance of adaptation pathways, decision rules to build such pathways and, to a lesser extent, communication of results.
Signpost identification for the construction of adaptation pathways is based on intrinsic variables or robust metrics determined based on conceptualizing a problem or system of interest. In the case of ATP signposts, we used the reliability of the water supply as a unique signpost to evaluate the robustness of different actions in achieving the rural incomes objective. The choice was considered sufficiently sensitive to levers and our exogenous factors. As a result, we observed that only ofs as a unique action was robust enough to avoid ATPs under both future scenarios. Two reasons may explain the result: either ofs is indeed robust against future conditions, or the chosen signpost may not allow us to observe conditions of failure.
To deal with the issue of detecting a signal, other signposts to complement the chosen one could have allowed for the identification of an ATP signal. Considering a combination of signposts implies handling issues of noise associated with the aggregation of signposts, which implies the consideration of more visual and statistical approaches to ensure the reliability (i.e., capacity to monitor a weak signal) and timeliness (i.e., capacity to support the anticipation of decisions) of signposts (Haasnoot et al., 2018). In addition, it involves the identification of a minimum number of signposts to observe and monitor critical uncertainties while avoiding cost of redundant signposts. Stochastic models coupled to exploratory ones could be used to ensure that principles of parsimony and completeness are achieved when considering the choice of a set of signposts (Raso et al., 2019b). Such additional approaches are expected to improve the technical soundness of signposts and to require a higher minimal level of information requirements.
Another limitation with the identification of ATPs is in relation to DAPP's assumption that signposts monotonically change over time (Kwakkel et al., 2016). We observed in the case of different combination of actions that ATP signals could vary according to runoff conditions. Such changes are explained by the non-monotonic behavior of the climate change datasets in BF, influencing water resources availability. In that case, other approaches could be considered such as exploring uncertain boundary conditions using a semi-probabilistic model to map conditions of failure and ATP occurrence (Raso et al., 2019a). Another solution could be to use “bricolage-type” approaches and various sets of visualization methods to acknowledge and map uncertainty (Fu et al., 2015, 2020). Future research will explore how these approaches could better contribute to the refinement of ATP conditions and their expected timing and implications for action robustness and associated adaptation pathways.
Another shortcoming of our approach is the limited number of uncertainties considered and the associated range of plausible values for each uncertain variable. These uncertainties were sufficient for our research purpose, but additional approaches will be required in more applied contexts to further explore the input uncertainty space, such as combining the DAPP framework with scenario discovery approaches (Kwakkel et al., 2016), which would alter MIRs, as discussed above.
Our choice of performance indicators and associated models is expected to influence the results and therefore the selection of plausible adaptation pathways (Kalra et al., 2014). For example, the rural incomes objective in the case study is estimated based on the sum of the net benefits from rainfed, irrigated, and fisheries productions from three sub models. As costs are not included in BF, associated constraints were inferred from physical variables. For rainfed and irrigated crop productions, costs were related to arable land suitability and availability. In this case, a small number of uncertainties with clear relationships meant that an exhaustive sampling approach was feasible. With a larger number of uncertainties, sampling (e.g. Monte Carlo, Latin Hypercube) or optimization approaches may be needed to adequately explore achievable performance. Scale of performance metrics can also be problematic (Jafino et al., 2021), and in our case was tackled by disaggregating results by state and using sparklines in addition to average values. This type of visualization tool is often advocated as an information dense representation of time series information but may be challenging to use without prior training in an applied context involving stakeholders.
The choice of robustness metrics is also influenced by the decision context, decision makers' preferred level of risk aversion and expected performance values (relative or absolute) for a system (McPhail et al., 2018). For example, the use of minimax metrics could reflect a decision makers' risk-averse attitude and attempt to minimize losses for a worst-case situation, and so could provide an additional dimension for evaluating the robustness of actions when considering the subjectivity of decisions associated with attitudes (Kalra et al., 2014). According to these authors, considering a set of metrics, including metrics reflecting decision makers' attitude, could help capture a broad set of possible assumptions. Another strategy to include the subjectivity behind decisions is to consider quantitative approaches, such as real-options analysis (Lawrence et al., 2019) to reduce the risks of under- or over-investments in actions. A broader set of signposts could be explored in future applications, including metrics capturing decision makers' attitude and behavior change (Giuliani and Castelletti, 2016).
More generally, the definition of the conditions of success/failure for signposts influences the identification of ATPs and the selection of plausible adaptation pathways. In our case, conditions of success were based on values found in the literature or from expert feedback. Other approaches could be used such as participatory ones, especially if considering more real-life planning situations (e.g., Bengston et al., 2020). Signposts and conditions of success/failure are not expected to be transferable beyond closely similar case studies. However, principles discussed and associated with the identification and use of signposts may be transferable, including criteria to select relevant signposts (Raso et al., 2019b), procedures to align with users' information requirements through reflection, discussion and deliberation (Bauler, 2012; Peppard and Campbell, 2014; Zare et al., 2020), consideration of different dimensions of robustness (Kalra et al., 2014; Lawrence et al., 2019), and approaches to handle iteration and emergent events (Peppard and Campbell, 2014; Fu et al., 2020).
The alignment of organizational structures would be critical to consider and monitor in regard to issues of uncertainty implementation (Peppard and Campbell, 2014). This was taken into account in the present study by considering administrative rather than basin boundaries and in comparing performance against baselines. This approach allows us to observe uneven impacts of adaptation pathways on the rural incomes objective, the downstream state seeing its rural incomes reduced compared to the upstream one, and potentially indicative of the emergence of inter-state tensions due to the upstream state water resources management strategy, which further work could follow up.
When considering the construction of adaptation pathways, two particular challenges need to be considered: (1) sequencing the (combination of) actions; and (2) selecting plausible adaptation pathways (Kwakkel et al., 2015). The first challenge involves the consideration of signposts and triggers to a change of action, while the second challenge implies the consideration of robustness metrics to measure the performance of adaptation pathways (Kwakkel et al., 2015).
Regarding sequencing rules, we use similar rules to Kwakkel et al. (2015), that is: (1) avoiding scale back of some interventions, and (2) removing pathways that would be rarely or never reached. Triggers to change of action were assumed to be represented by transient scenarios and their associated time range. In addition, assumptions in regard to the lead-time necessary to implement actions were considered beforehand when setting the parameterization of our scenarios. For simplification purposes, for example, we assumed that proposed dams need to be considered in period 1 to be able to be implemented in period 3. This involved the upfront parameterization of four scenarios (bau1, bau2, irr1, irr2) with different planting areas for irrigated and rainfed crops according to the available irrigation command area and irrigation growth rate. In different contexts of application, considering a more refined way to identify triggers could be based on expert opinions (Haasnoot et al., 2013), coupling with more detailed model analyses (Haasnoot et al., 2015), exploring vulnerabilities based on RDM approaches and iterative setting of the results to be considered or not (Kwakkel et al., 2016), or robust optimization (Hamarat et al., 2014). These approaches are expected to represent different levels of minimum information needs, with implications for what model augmentation solutions are feasible.
The selection of plausible adaptation pathways was based on the definition of conditions of success for our objectives and constraints in regard to the number of objectives with acceptable outcomes. Such definitions affect the number of plausible pathways that will be identified. For example, our initial constraint was that all objectives should achieve acceptable outcomes, which led to six plausible adaptation pathways, all including ofs in period 2. When relaxing that constraint to three out of five objectives, including rural incomes, and excluding urban density ratio, 5,000 pathways were identified, including ofs or not. Considering our integration approach, using a feed-forward rather than an iterative/feedback process also means that rapid prototyping and workflow execution was possible (less than a day) (Belete et al., 2017). Approaches such as ours with a short learning feedback loop—especially early in the planning design process—could help support discussions and the identification of information requirements to target specific information needs before considering further investments (Zare et al., 2020).
We also observed that some of our decision rules could have been constrained further, as some adaptation pathways appear redundant. For example, the inclusion of effluent treatment measures in different periods did not appear to change average values significantly when considering our scorecard results. Considering one of the four solutions could have been sufficient. On the other hand, exploiting redundancy could support a better approach to performing and coordinating tasks, as it considers more degrees of freedom (Todorov and Jordan, 2002), and could perhaps be exploited in the planning process.
We have presented a MIRs approach to guide and orchestrate model augmentation for exploratory modeling and analysis, employing the DAPP framework for the orchestration process. The Basin Futures software was used as a case study in the context of the BRB to illustrate details of the approach and associated results. The MIRs approach involves the identification of misalignments in perspectives between human and model visions, and formulation of different solutions reflective of different information requirements as well as conditions of collaboration and learning feedback loops for users and developers.
Despite its apparent simplicity, the MIRs approach allowed us to transform the use of a consolidative model for exploratory purposes through the identification of ATPs and the design of adaptation pathways. The approach could be used for rapid feedback early in the planning design process to raise awareness about potential issues to be explored prior to further investments. It could also benefit education and training of future natural resources managers to develop their critical thinking through a “learning-by-doing” process.
The paper raises many opportunities for future work. In terms of triple feedback loop learning there is promise in evaluating how a structured approach to misalignment could contribute to a more transformative impact, one that revolutionizes both users' and developers' conception of collaboration and that leads to the creation of a collaborative modeling culture and ongoing process throughout a model life cycle, from development to ongoing augmentation. Future work could also test different tools/mechanisms for exchanging and discussing information with and among stakeholders in the context of model augmentation.
There is potential for future work exploring real-world decision-making applications and transferability of the MIRs approach to other modeling tools beyond the Basin Futures tool considered here. Such applications have the potential to also explore issues around information redundancy, dynamic societal responses, alignments between different dimensions of robustness, and visualization tools to communicate uncertainty and support collaborative decision making.
The original contributions presented in the study are included in the article/Supplementary Material, further inquiries can be directed to the corresponding author/s.
This study was reviewed and approved by the Ethics Committee of the Second People’s Hospital of Wuhu. The patients/participants provided their written informed consent to participate in this study. Written informed consent was obtained from the individual(s) for the publication of any potentially identifiable images or data included in this article.
CR and JG contributed to the conception and design of the study. CR performed the analysis. PT and CP provided feedback about the Basin Futures tool and the BRB case study respectively. PT made changes to the basin futures to accommodate some aspects of the study. CR performed data analysis and wrote the first draft of the manuscript. All authors contributed to manuscript revision, read, and approved the submitted version.
JG received funding from an Australian Research Council Discovery Early Career Researcher Award (project no. DE190100317).
The authors declare that the research was conducted in the absence of any commercial or financial relationships that could be construed as a potential conflict of interest.
All claims expressed in this article are solely those of the authors and do not necessarily represent those of their affiliated organizations, or those of the publisher, the editors and the reviewers. Any product that may be evaluated in this article, or claim that may be made by its manufacturer, is not guaranteed or endorsed by the publisher.
We would like to thank the Basin Futures team for their support and assistance as well as the International Commission on Irrigation and Drainage (ICID) for their report used to calibrate and analyze water developments in the Brahmani River Basin, India. We also thank three reviewers for their useful feedback.
The Supplementary Material for this article can be found online at: https://www.frontiersin.org/articles/10.3389/frwa.2022.768898/full#supplementary-material
Ackerman, F., DeCanio, S. J., Howarth, R. B., and Sheeran, K. (2009). Limitations of integrated assessment models of climate change. Clim. Change 95, 297–315. doi: 10.1007/s10584-009-9570-x
Auping, W. L. (2018). Modeling Uncertainty: Developing and Using Simulation Models for Exploring the Consequences of Deep Uncertainty in Complex Problems. Doctoral thesis, Delft University of Technology, The Netherlands. doi: 10.4233/uuid:0e0da51a-e2c9-4aa0-80cc-d930b685fc53
Balana, B. B., Vinten, A., and Slee, B. (2011). A review on cost-effectiveness analysis of agri-environmental measures related to the EU WFD: key issues, methods, and applications. Ecol. Econ. 70, 1021–1031. doi: 10.1016/j.ecolecon.2010.12.020
Basco-Carrera, L., Warren, A., van Beek, E., Jonoski, A., and Giardino, A. (2017). Collaborative modeling or participatory modeling? A framework for water resources management. Environ. Model. Softw. 91, 95–110. doi: 10.1016/j.envsoft.2017.01.014
Bauler, T. (2012). An analytical framework to discuss the usability of (environmental) indicators for policy. Ecol. Indic. 17, 38–45. doi: 10.1016/j.ecolind.2011.05.013
Belete, G. F., Voinov, A., and Laniak, G. F. (2017). An overview of the model integration process: from pre-integration assessment to testing. Environ. Model. Softw. 87, 49–63. doi: 10.1016/j.envsoft.2016.10.013
Bengston, D. N., Westphal, L. M., and Dockry, M. J. (2020). Back from the future: the backcasting wheel for mapping a pathway to a preferred future. World Fut. Rev. 12, 270–278. doi: 10.1177/1946756720929724
Ben-Haim, Y. (2006). Info-Gap Decision Theory: Decisions under Severe Uncertainty. Oxford, UK: Elsevier.
Brown, C., Ghile, Y., Laverty, M., and Li, K. (2012). Decision scaling: linking bottom-up vulnerability analysis with climate projections in the water sector. Water Resour. Res. 48, W09537. doi: 10.1029/2011WR011212
Bryant, B. P., and Lempert, R. J. (2010). Thinking inside the box: a participatory, computer-assisted approach to scenario discovery. Technol. Forecast. Soc. Change 77, 34–49. doi: 10.1016/j.techfore.2009.08.002
Cetinkaya, D., Verbraeck, A., and Seck, M. D. (2010). “Applying a model driven approach to component based modeling and simulation,” in Proceedings of the 2010 Winter Simulation Conference (Baltimore, MD), 546–553. doi: 10.1109/WSC.2010.5679131
Chowdhury, M. W., and Iqbal, M. Z. (2004). “Integration of legacy systems in software architecture.” in SAVCBS 2004 Specification and Verification of Component-Based Systems (Newport Beach, CA), 110–113. Available online at: https://www.cs.ucf.edu/~leavens/SAVCBS/2004/savcbs04.pdf#page=122 (accessed December 2021).
De Neufville, R., and Scholtes, S. (2011). Flexibility in Engineering Design. Cambridge, MA: MIT Press.
Etzioni, A. (2001). “Humble decision making,” in Harvard Business Review on Decision Making, ed A. Etzioni, (Boston, MA: Harvard Business School Press), 45–57.
Fu, B., Guillaume, J. H. A., and Jakeman, A. J. (2015). An iterative method for discovering feasible management interventions and targets conjointly using uncertainty visualizations. Environ. Model. Softw. 71, 159–173. doi: 10.1016/j.envsoft.2015.05.017
Fu, B., Guillaume, J. H. A., Jakeman, A. J., and Asher, M. J. (2020). A bricolage-style exploratory scenario analysis to manage uncertainty in socio-environmental systems modeling: investigating integrated water management options. Soc. Environ. Syst. Model. 2, 16227. doi: 10.18174/sesmo.2020a16227
Geltner, D., and De Neufville, R. (2018). Flexibility and Real Estate Valuation under Uncertainty: A Practical Guide for Developers. Hoboken, NJ: John Wiley and Sons.
Giuliani, M., and Castelletti, A. (2016). Is robustness really robust? How different definitions of robustness impact decision-making under climate change. Clim. Change 135, 409–424. doi: 10.1007/s10584-015-1586-9
Giupponi, C., Jakeman, A. J., Karssenberg, D., and Hare, M. P. (2006). Sustainable Management of Water Resources: An Integrated Approach. Cheltenham: Edward Elgar Publishing.
Groves, D. G., and Lempert, R. J. (2007). A new analytic method for finding policy-relevant scenarios. Glob. Environ. Change 17, 73–85. doi: 10.1016/j.gloenvcha.2006.11.006
Guillaume, J. H. A., Qureshi, M. E., and Jakeman, A. J. (2012). A structured analysis of uncertainty surrounding modeled impacts of groundwater-extraction rules. Hydrogeol. J. 20, 915–932. doi: 10.1007/s10040-012-0864-0
Haasnoot, M., Kwakkel, J. H., Walker, W. E., and ter Maat, J. (2013). Dynamic adaptive policy pathways: a method for crafting robust decisions for a deeply uncertain world. Glob. Environ. Change 23, 485–498. doi: 10.1016/j.gloenvcha.2012.12.006
Haasnoot, M., Schellekens, J., Beersma, J. J., Middelkoop, H., and Kwadijk, J. C. J. (2015). Transient scenarios for robust climate change adaptation illustrated for water management in The Netherlands. Environ. Res. Lett. 10, 105008. doi: 10.1088/1748-9326/10/10/105008
Haasnoot, M., van't Klooster, S., and van Alphen, J. (2018). Designing a monitoring system to detect signals to adapt to uncertain climate change. Glob. Environ. Change 52, 273–285. doi: 10.1016/j.gloenvcha.2018.08.003
Haasnoot, M., Warren, A., and Kwakkel, J. H. (2019). “Dynamic adaptive policy pathways (DAPP).” in Decision Making under Deep Uncertainty, eds V. Marchau, W. Walker, P. Bloemen and S. Popper, (Cham: Springer)71–92.
Hallegatte, S., Shah, A., Brown, C., Lempert, R., and Gill, S. (2012). Investment Decision Making under Deep Uncertainty–Application to Climate Change. World Bank Policy Research Working Paper No. 6193. Available online at: https://ssrn.com/abstract=2143067 (accessed December 2021).
Hamarat, C., Kwakkel, J. H., and Pruyt, E. (2013). Adaptive robust design under deep uncertainty. Technol. Forecast. Soc. Change 80, 408–418. doi: 10.1016/j.techfore.2012.10.004
Hamarat, C., Kwakkel, J. H., Pruyt, E., and Loonen, E. T. (2014). An exploratory approach for adaptive policymaking by using multi-objective robust optimization. Simul. Modell. Pract. Theory 46, 25–39. doi: 10.1016/j.simpat.2014.02.008
Hamilton, S. H., ElSawah, S., Guillaume, J. H. A., Jakeman, A. J., and Pierce, S. A. (2015). Integrated assessment and modeling: overview and synthesis of salient dimensions. Environ. Model. Softw. 64, 215–229. doi: 10.1016/j.envsoft.2014.12.005
Herlihy, C., Cao, K., Reparti, S., Briscoe, E., and Fairbanks, J. (2019). “Semantic program analysis for scientific model augmentation,” in Modeling the World's Systems (Atlanta, GA: Georgia Tech Research Institute), 13-15. Available online at: http://jpfairbanks.com/doc/Fairbanks_MWS19.pdf
Hermans, L. M., Haasnoot, M., ter Maat, J., and Kwakkel, J. H. (2017). Designing monitoring arrangements for collaborative learning about adaptation pathways. Environ. Sci. Policy 69, 29–38. doi: 10.1016/j.envsci.2016.12.005
Hudson-Doyle, E. E., Paton, D., and Johnston, D. (2018). “Reflections on the communication of uncertainty: developing decision-relevant information,” in Proceedings of the ISCRAM Asia Pacific Conference (Wellington).
ICID (2005). Water Resources Assessment of Brahmani River Basin, India. A Document to Analyse the Future Scenarios of a Relatively Water-Rich Basin as Support to Country Water Policies. New Dehli: International Commission on Irrigation and Drainage.
Jafino, B. A., Kwakkel, J. H., and Taebi, B. (2021). Enabling assessment of distributive justice through models for climate change planning: a review of recent advances and a research agenda. WIREs Clim. Change. 12, e721. doi: 10.1002/wcc.721
Jakeman, A. J., and Letcher, R. A. (2003). Integrated assessment and modeling: features, principles and examples for catchment management. Environ. Modell. Softw. 18, 491–501. doi: 10.1016/S1364-8152(03)00024-0
Kalra, N., Hallegatte, S., Lempert, R., Brown, C., Fozzard, A., Gill, S., et al. (2014). Agreeing on Robust Decisions: New Processes for Decision Making under Deep Uncertainty. World Bank Policy Research Working Paper.
Kasprzyk, J. R. (2013). Many Objective Water Resources Planning and Management given Deep Uncertainties, Population Pressures, and Environmental Change. Doctoral thesis, The Pennsylvania State University, Pennsylvania, PA.
Kwadijk, J. C. J., Haasnoot, M., Mulder, J. P. M., Hoogvliet, M. M. C., Jeuken, A. B. M., van der Krogt, R. A. A., et al. (2010). Using adaptation tipping points to prepare for climate change and sea level rise: a case study in the Netherlands. WIREs Clim. Change 1, 729–740. doi: 10.1002/wcc.64
Kwakkel, J. H., Haasnoot, M., and Walker, W. E. (2015). Developing dynamic adaptive policy pathways: a computer-assisted approach for developing adaptive strategies for a deeply uncertain world. Clim. Change 132, 373–386. doi: 10.1007/s10584-014-1210-4
Kwakkel, J. H., Haasnoot, M., and Walker, W. E. (2016). Comparing robust decision-making and dynamic adaptive policy pathways for model-based decision support under deep uncertainty. Environ. Model. Softw. 86, 168–183. doi: 10.1016/j.envsoft.2016.09.017
Lawrence, J., Haasnoot, M., McKim, L., Atapattu, D., Campbell, G., and Stroombergen, A. (2019). “Dynamic adaptive policy pathways (DAPP): from theory to practice,” in Decision Making Under Deep Uncertainty, eds V. Marchau, W. Walker, P. Bloemen and S. Popper, (Cham: Springer) 187–99.
Lempert, R. J., Groves, D. G., Popper, S. W., and Bankes, S. C. (2006). A general, analytic method for generating robust strategies and narrative scenarios. Manage. Sci. 52, 514–528. doi: 10.1287/mnsc.1050.0472
Lempert, R. J., Popper, S. W., and Bankes, S. C. (2003). Shaping the Next One Hundred Years: New Methods for Quantitative, Long-Term Policy Analysis. Santa Monica, CA: RAND.
Loucks, D. P., and Van Beek, E. (2017). Water Resource Systems Planning and Management: An Introduction to Methods, Models, and Applications. Cham: Springer.
Luo, Y., Yin, L., Bai, W., and Mao, K. (2020). An appraisal of incremental learning methods. Entropy 22, 1190. doi: 10.3390/e22111190
Madni, A. M., and Sievers, M. (2014). Systems integration: key perspectives, experiences, and challenges. Syst. Eng. 17, 37–51. doi: 10.1002/sys.21249
Madni, A. M., and Sievers, M. (2018). Model-based systems engineering: motivation, current status, and research opportunities. Syst. Eng. 21, 172–190. doi: 10.1002/sys.21438
Mainuddin, M., Pollino, C. A., and Merrin, L. E. (2016). Agricultural Productivity in the Brahmani-Baitarni River Basin of India. CSIRO, Australia. doi: 10.4225/08/5877c43b8033a
Marchau, V. A. W. J., Walker, W. E., Bloemen, P. J. T. M., and Popper, S. W. (2019). Decision Making under Deep Uncertainty. Cham: Springer.
McIntosh, B. S., Ascough, J. C., Twery, M., Chew, J., Elmahdi, A., Haase, D., and Harou, J. J. (2011). Environmental decision support systems development - challenges and best practices. Environ. Model. Softw. 26, 1389–1402. doi: 10.1016/j.envsoft.2011.09.009
McPhail, C., Maier, H. R., Kwakkel, J. H., Giuliani, M., Castelletti, A., and Westra, S. (2018). Robustness metrics: how are they calculated, when should they be used and why do they give different results? Earths Fut. 6, 169–191. doi: 10.1002/2017EF000649
Michas, S., Stavrakas, V., Papadelis, S., and Flamos, A. (2020). A transdisciplinary modeling framework for the participatory design of dynamic adaptive policy pathways. Energy Policy 139, 111350. doi: 10.1016/j.enpol.2020.111350
Moallemi, E. A., Kwakkel, J., de Haan, F. J., and Bryan, B. A. (2020). Exploratory modeling for analyzing coupled human-natural systems under uncertainty. Glob. Environ. Change 65, 102186. doi: 10.1016/j.gloenvcha.2020.102186
O'Sullivan, J., Pollino, C., Taylor, P., Sengupta, A., and Parashar, A. (2020). An integrative framework for stakeholder engagement using the basin futures platform. Water 12, 2398. doi: 10.3390/w12092398
Pahl-Wostl, C. (2009). A conceptual framework for analysing adaptive capacity and multi-level learning processes in resource governance regimes. Glob. Environ. Change 19, 354–365. doi: 10.1016/j.gloenvcha.2009.06.001
Peppard, J., and Campbell, B. (2014). The co-evolution of business/information systems strategic alignment: an exploratory study. J. Inform. Technol. 1–51. Available online at: https://faculty-research.esmt.berlin/sites/faculty/files/full_text_upload/Coevolution%20of%20Strategic%20Alignment%20JIT2014%20revision%20v3-1.pdf (accessed December 2021).
Poff, N. L. R., Brown, C. M., Grantham, T. E., Matthews, J. H., Palmer, M. A., Spence, C. M., et al. (2016). Sustainable water management under future uncertainty with eco-engineering decision scaling. Nat. Clim. Chang. 6, 25–34. doi: 10.1038/nclimate2765
Pollino, C., Brown, A., Barma, D., Ahmad, M., Chen, Y., Cuddy, S., et al. (2016). Brahmani Model: Technical Description. Canberra, ACT: CSIRO. doi: 10.4225/08/58a1f46b0d642
Raisamo, R., Rakkolainen, I., Majaranta, P., Salminen, K., Rantala, J., and Farooq, A. (2019). Human augmentation: past, present and future. Int. J. Hum. Comp. Stud. 131, 131–43. doi: 10.1016/j.ijhcs.2019.05.008
Raso, L., Kwakkel, J., and Timmermans, J. (2019a). Assessing the capacity of adaptive policy pathways to adapt on time by mapping trigger values to their outcomes. Sustainability 11, 1716. doi: 10.3390/su11061716
Raso, L., Kwakkel, J., Timmermans, J., and Panthou, G. (2019b). How to evaluate a monitoring system for adaptive policies: criteria for signposts selection and their model-based evaluation. Clim. Change 153, 267–283. doi: 10.1007/s10584-018-2355-3
Reichert, M., and Weber, B. (2012). Enabling Flexibility in Process-Aware Information Systems: Challenges, Methods, Technologies. Cham: Springer Science and Business Media.
Robinson, S., Nance, R. E., Paul, R. J., Pidd, M., and Taylor, S. J. E. (2004). Simulation model reuse: definitions, benefits and obstacles. Simul. Operat. Res. 12, 479–494. doi: 10.1016/j.simpat.2003.11.006
Rothenberg, J. (2008). Interoperability as a Semantic Cross-Cutting Concern. Interoperabiliteit: Eerlijk Zullen We Alles Delen. Den Haag.
Seidel, S., Berente, N., Lindberg, A., Lyytinen, K., and Nickerson, J. V. (2019). Autonomous tools and design: a triple-loop approach to human-machine learning. Commun. ACM. 62, 50–57. doi: 10.1145/3210753
Smith, G. F. (1990). Heuristic methods for the analysis of managerial problems. Omega 18, 625–635. doi: 10.1016/0305-0483(90)90054-D
Soetaert, K., and Meysman, F. (2012). Reactive transport in aquatic ecosystems: rapid model prototyping in the open source software R. Environ. Model. Softw. 32, 49–60. doi: 10.1016/j.envsoft.2011.08.011
Sterman, J. D. (2006). Learning from evidence in a complex world. Am. J. Public Health. 96, 505–514. doi: 10.2105/AJPH.2005.066043
Swain, S. S., Mishra, A., Sahoo, B., and Chatterjee, C. (2020). Water scarcity-risk assessment in data-scarce river basins under decadal climate change using a hydrological modeling approach. J. Hydrol. 590, 125260. doi: 10.1016/j.jhydrol.2020.125260
Taylor, P., Rahman, J., O'Sullivan, J., Podger, G., Rosello, C., Parashar, A., et al. (2021). Basin futures, a novel cloud-based system for preliminary river basin modeling and planning. Environ. Model. Softw. 141, 105049. doi: 10.1016/j.envsoft.2021.105049
Taylor, P., Stewart, J., Rahman, J., Parashar, A., Pollino, C., and Podger, G. (2017). “Basin futures: supporting water planning in data poor basins.” in Proceedings of the 22nd International Congress on Modeling and Simulation (Hobart, TAS), 3–8.
Todorov, E., and Jordan, M. I. (2002). Optimal feedback control as a theory of motor coordination. Nat. Neurosci. 5, 1226–1235. doi: 10.1038/nn963
Van Voorn, G. A. K., Verburg, R. W., Kunseler, E.-M., Vader, J., and Janssen, P. H. M. (2016). A checklist for model credibility, salience, and legitimacy to improve information transfer in environmental policy assessments. Environ. Model. Softw. 83, 224–236. doi: 10.1016/j.envsoft.2016.06.003
Vandana, K., Islam, A., Sarthi, P. P., Sikka, A. K., and Kapil, H. (2019). Assessment of potential impact of climate change on streamflow: a case study of the Brahmani River Basin, India. J. Water Clim. Change 10, 624–641. doi: 10.2166/wcc.2018.129
Voinov, A. (2018). “Sensitivity, calibration, validation, verification.” in Encyclopedia of Ecology, (Oxford: Elsevier), 172–177. doi: 10.1016/B978-0-12-409548-9.11179-0
Voinov, A., Jenni, K., Gray, S., Kolagani, N., Glynn, P.D., and Bommel, P. (2018) Tools methods in participatory modeling: Selecting the right tool for the job. Environ. Model. Softw. 109 232–255. doi: 10.1016/j.envsoft.2018.08.028
Voinov, A., and Shugart, H. H. (2013). ‘Integronsters', integral and integrated modeling. Environ. Modell. Softw. 39, 149–158. doi: 10.1016/j.envsoft.2012.05.014
Walker, W. E., Lempert, R. J., and Kwakkel, J. H. (2013). “Deep uncertainty.” in Encyclopedia of Operations Research and Management Science, eds S. Gass, and M. Fu, (New York, NY: Springer), 395–402.
Walker, W. E., Rahman, S. A., and Cave, J. (2001). Adaptive policies, policy analysis, and policy-making. Compl. Soc. Probl. 128, 282–289. doi: 10.1016/S0377-2217(00)00071-0
White, D. D., Wutich, A., Larson, K. L., Gober, P., Lant, T., and Senneville, C. (2010). Credibility, salience, and legitimacy of boundary objects: water managers' assessment of a simulation model in an immersive decision theater. Sci. Publ. Policy 37, 219–232. doi: 10.3152/030234210X497726
Woodruff, M. J., Reed, P. M., and Simpson, T. W. (2013). Many objective visual analytics: rethinking the design of complex engineered systems. Struct. Multidiscipl. Optimiz. 48, 201–219. doi: 10.1007/s00158-013-0891-z
Keywords: deep uncertainty, adaptive planning, exploratory modeling, adaptation pathways, tipping point
Citation: Rosello C, Guillaume JHA, Taylor P, Cuddy S, Pollino C and Jakeman AJ (2022) Identifying Minimum Information Requirements to Improve Integrated Modeling Capabilities: Lessons Learned From Dynamic Adaptive Policy Pathways. Front. Water 4:768898. doi: 10.3389/frwa.2022.768898
Received: 01 September 2021; Accepted: 05 January 2022;
Published: 08 February 2022.
Edited by:
Maarten W. Saaltink, Universitat Politecnica de Catalunya, SpainReviewed by:
Francesca Pianosi, University of Bristol, United KingdomCopyright © 2022 Rosello, Guillaume, Taylor, Cuddy, Pollino and Jakeman. This is an open-access article distributed under the terms of the Creative Commons Attribution License (CC BY). The use, distribution or reproduction in other forums is permitted, provided the original author(s) and the copyright owner(s) are credited and that the original publication in this journal is cited, in accordance with accepted academic practice. No use, distribution or reproduction is permitted which does not comply with these terms.
*Correspondence: Caroline Rosello, Y2Fyb2xpbmUucm9zZWxsb0BhbnUuZWR1LmF1
Disclaimer: All claims expressed in this article are solely those of the authors and do not necessarily represent those of their affiliated organizations, or those of the publisher, the editors and the reviewers. Any product that may be evaluated in this article or claim that may be made by its manufacturer is not guaranteed or endorsed by the publisher.
Research integrity at Frontiers
Learn more about the work of our research integrity team to safeguard the quality of each article we publish.