- 1Institute of Geography, University of Augsburg, Augsburg, Germany
- 2Institute of Meteorology and Climate Research (IMK-IFU), Karlsruhe Institute of Technology, Garmisch-Partenkirchen, Germany
- 3Agrosphere (IBG-3), Forschungszentrum Jülich GmbH, Jülich, Germany
Cosmic-Ray Neutron Sensing (CRNS) offers a non-invasive method for estimating soil moisture at the field scale, in our case a few tens of hectares. The current study uses the Ensemble Adjustment Kalman Filter (EAKF) to assimilate neutron counts observed at four locations within a 655 km2 pre-alpine river catchment into the Noah-MP land surface model (LSM) to improve soil moisture simulations and to optimize model parameters. The model runs with 100 m spatial resolution and uses the EU-SoilHydroGrids soil map along with the Mualem–van Genuchten soil water retention functions. Using the state estimation (ST) and joint state–parameter estimation (STP) technique, soil moisture states and model parameters controlling infiltration and evaporation rates were optimized, respectively. The added value of assimilation was evaluated for local and regional impacts using independent root zone soil moisture observations. The results show that during the assimilation period both ST and STP significantly improved the simulated soil moisture around the neutron sensors locations with improvements of the root mean square errors between 60 and 62% for ST and 55–66% for STP. STP could further enhance the model performance for the validation period at assimilation locations, mainly by reducing the Bias. Nevertheless, due to a lack of convergence of calculated parameters and a shorter evaluation period, performance during the validation phase degraded at a site further away from the assimilation locations. The comparison of modeled soil moisture with field-scale spatial patterns of a dense network of CRNS observations showed that STP helped to improve the average wetness conditions (reduction of spatial Bias from –0.038 cm3 cm−3 to –0.012 cm3 cm−3) for the validation period. However, the assimilation of neutron counts from only four stations showed limited success in enhancing the field-scale soil moisture patterns.
1. Introduction
The amount of water present in the root zone of the soil is an essential climate variable and a common link between the carbon, water, and energy cycles. Soil water content (SWC) influences multiple processes like runoff generation, evapotranspiration, sensible and latent heat fluxes, groundwater recharge, plant water stress and vegetation development (Mishra et al., 2014; McColl et al., 2017). It acts as a regulator of the hydrological cycle and the global radiation budget (Small and Kurc, 2003; Brocca et al., 2017). Therefore, SWC constitutes a key state variable of land surface models and governs the lower boundary of atmospheric models. Consequently, the past two decades have witnessed significant efforts to observe and assimilate SWC states into hydrological (Moradkhani et al., 2005; Chen et al., 2011), and land surface models (Kumar et al., 2008; De Lannoy and Reichle, 2016) to adjust the model states toward the real-world conditions. The well-established point measurement techniques for estimating SWC are thermo-gravimetry, time/frequency domain reflectometry, thermal sensors and electrical conductivity sensors (Johnson, 1962; Walker et al., 2004). These approaches are labor-intensive and time consuming. However, there are automated systems (Bogena et al., 2007) that link multiple sensors to estimate spatially representative soil moisture estimates over a longer timeframe. These sensor networks need regular maintenance and are expensive. Therefore, it is difficult to use them effectively in catchment scale land surface modeling and data assimilation applications which requires spatially representative soil moisture estimates (Entin et al., 2000). Among the spatially distributed soil moisture estimation techniques, active and passive microwave remote sensing methods use the dielectric properties of soils to estimate the SWC. The thermal microwave radiation of a surface is highly influenced by the dielectric properties of a substance. The difference between the thermal microwave emissions of dry soil (dielectric constant of ~3.5) and that of water (dielectric constant of ~80) is significant and can be detected with a high signal to noise ratio within 1–5 GHz of sensing frequency. This variation in the brightness temperature is used for the estimation of SWC at the land surface. The soil moisture estimation from remote platforms possess a coarse spatial footprint (9 to 25 km) and it contains information from the top few centimeters of soil (0 to 5 cm). Good quality soil moisture estimates from remote sensing techniques are limited to the regions with bare soil and low to moderate vegetation cover (Njoku et al., 2003; Zribi and Dechambre, 2003; Kerr et al., 2012). However, most of the land surface, crop and hydrology models operate at finer spatial resolution (100 m–1 km) and they rely on root zone soil moisture information to efficiently evaluate water and energy balances.
Recent developments of the Cosmic-Ray Neutron Sensing (CRNS) soil moisture estimation method (Zreda et al., 2008; Andreasen et al., 2017; Fersch et al., 2018; Schrön et al., 2018) are promising for overcoming the limitations of point- and remote sensing-based techniques of SWC quantification. Water sensitive neutrons are being generated when high energy primary cosmic radiation (~1 GeV) makes its way through the Earth's magnetosphere, thereby generating cascades of secondary cosmic particles. These secondary cosmic particles further collide with atmospheric gases (oxygen and nitrogen nuclei) and water molecules to produce the so called epithermal or fast neutrons (with energy levels in the range of 1–105 eV) which are omnipresent near the Earth's surface. The CRNS method relies on the density variations of fast neutrons close to the land surface as the moderation of fast neutron is highly sensitive to the presence of hydrogen atoms below and at the land surface due to the similar mass of hydrogen nuclei (Desilets and Zreda, 2013; Köhli et al., 2015). In addition to soil water, other hydrogen pools like, e.g., vegetation can influence the amount of available fast neutrons (Desilets et al., 2010; Zreda et al., 2012). The cosmic-ray neutron probes (CRNPs) count these ambient fast neutrons concentrations over certain time intervals which can be then related to soil moisture due to the strong inverse relationship. When a CRNP is placed 2 m above ground, the footprint of the estimated SWC is typically in the range of 130–240 m radius, depending on the SWC itself, air humidity and vegetation conditions (Köhli et al., 2015). Similarily, depending on the soil dryness, the penetration or origination depth of the measured neutrons varies from ~15 to 80 cm directly below the sensor and decreases exponentially with the horizontal distance. Thus, the spatial footprint of several hectares is analogous to typical grid resolutions of current generation land surface models. Therefore, the CRNS based SWC estimates can be effectively used in hydrological and land surface modeling applications by means of data assimilation.
To facilitate the assimilation of neutron counts from CRNPs Shuttleworth et al. (2013) developed the COsmic-ray Soil Moisture Interaction Code (COSMIC). COSMIC is a forward operator which relates the depth averaged SWC from multiple soil layers from a land surface model to the expected ambient concentration of fast neutrons. COSMIC can be effectively used for ensemble based sequential assimilation. In the past, very few studies (specifically, Rosolem et al., 2014; Han et al., 2015; Baatz et al., 2017) have assimilated CRNS information to estimate SWC within land surface models. It should be emphasized that the past study by Baatz et al. (2017) was the only one to deploy the spatially distributed hydrological modeling for updating the soil moisture states across the catchment using CRNS information. Baatz et al. (2017) used the soil moisture derived from nine CRNP's from a widely spaced observation network and estimated SWC and soil hydraulic parameters of the Community Land Model (CLM) using the FAO soil map, the BK50 soil map and falsified soil data as input information. The study confirmed the positive results of data assimilation by a jackknife validation experiment. Furthermore, they found that the use of more accurate soil type information can lead to better soil moisture predictions, hence data assimilation has limited scope to further improve the soil moisture states during the validation period. In this study the COSMIC operator was utilized to inversely estimate the average profile soil moisture from measured neutron counts prior to assimilation into the land surface model. However, within an ensemble-based data assimilation framework, the COSMIC operator can be used to predict expected neutron counts information in order to optimally update the spatially and vertically varying soil moisture states. Rosolem et al. (2014) found that the Noah LSM can perform exceptionally good to produce "true" profile soil moisture estimates when deploying the COSMIC operator for first predicting neutron counts from model soil moisture in the data assimilation algorithm. As a result, the COSMIC operator should be used to predict neutron counts inside the data assimilation framework (as studied by Rosolem et al., 2014) to fully analyze the benefits of the CRNS technique for enhancing soil moisture characterization at the catchment scale.
The work of Tóth et al. (2017) has provided soil hydraulic information with high spatial resolution (250 m) for the European Union. This raises the question if the assimilation of CRNS data still holds potential for improvements in land surface model simulations while using such high spatial resolution soil type data sets. Further, the observational data sets from a dense CRNS sensor network as presented by Fersch et al. (2020a) opens up new possibilities to asses the value of CRNS data assimilation from more sparsely distributed CRNPs. These data sets are collected at the TERENO observation site in Fendt using 24 CRNPs over an area of just 1 km2, providing soil moisture variability at finer spatial scales. This data set along with other independent sensor observations from the study region can be used to rigorously test the improved skill of the land surface model at a larger spatial scale by assimilation of CRNS neutron counts from a sparse observation network.
Parameter estimation is an important aspect of data assimilation applications where model parameters can be estimated along with model states using observed information. This approach deviates from the assumption of time invariance of model parameters and helps to accommodate them as time varying quantities to adapt to changes in catchment characteristics. Also, it can be useful to improve model performance during non-assimilation periods. Multiple parameter estimation approaches have been proposed in literature such as the augmented state vector approach (Hendricks Franssen and Kinzelbach, 2008; Xie and Zhang, 2010) and the dual correction approach (Moradkhani et al., 2005; Pathiraja et al., 2016). Past studies have shown their importance in estimating soil hydraulic properties (Pauwels et al., 2009; Baatz et al., 2017) and land surface modeling (Yang et al., 2016; Baatz et al., 2017). Cuntz et al. (2016) revealed the impact of traditional and hard-coded model parameters on the hydrological fluxes in the Noah-MP land surface model and found that the hard coded parameter of soil surface resistance for direct evaporation was the most sensitive one. Further, they showed that the calibration of only soil hydraulic parameters is not enough for a realistic model setup. Therefore, it is important to study the possible application of data assimilation techniques to improve model simulations by estimating a combination of conventional and hard-coded parameters in land surface models.
To address these research gaps, the key focus of the present study is to evaluate the potential of sparsely distributed CRNS data to improve the soil moisture states and dynamics of a mesoscale land surface model. This is achieved by using the best quality available input data sets of model forcing, soil and land use type information. Field scale soil moisture information from four sparsely distributed CRNPs is assimilated into the Noah-MP land surface model for a meso-scale river catchment in southern Germany, using the Ensemble Adjustment Kalman Filter (EAKF) method to simultaneously update soil moisture and model parameters (without any soil hydraulic parameters). The resulting soil moisture fields are analyzed using independent observation data sets of root zone soil moisture measurements from a distributed soil sensor network, point profile measurements at several locations within the study domain and in addition spatially distributed field scale soil moisture information from a dense temporary CRNS network as presented by Fersch et al. (2020a). In contrast to previous studies by Rosolem et al. (2014), Han et al. (2015), and Baatz et al. (2017), this study assimilates neutron counts from four CRNPs to improve 2D soil moisture characterization for a catchment of 655 km2. The high spatial resolution (250 m) EU-SoilHydroGrids data set, as well as the Mualem–van Genuchten soil water retention model, were implemented to accommodate the best quality soil type information within the existing Noah-MP setup. Furthermore, the COSMIC operator (Shuttleworth et al., 2013; Rosolem et al., 2014) was utilized, using a more process-oriented approach within the data assimilation framework, and the evaluation was based on independent soil moisture observations from multiple sources (point, sensor network CRNS).
In the following, section 2 first discusses the study area and data sets, followed by the description of the model, forward operator and data assimilation technique. Then the ensemble generation methods and experimental setup are given. In section 3 the results are presented and discussed. Finally, the summary and conclusions are given in section 4.
2. Methodology
2.1. Study Area and Data
This study is carried out at the combined Rott and Ammer catchment (Figure 1) located in southern Bavaria, Germany. The catchment comprises the German Terrestrial Environmental Observatories (TERENO) program's Pre-Alpine observatory (Kiese et al., 2018). At the location Peißenberg-Fendt, the observatory is equipped with a wireless underground sensor network (SoilNet) to measure spatially distributed soil moisture and soil temperature profiles at 55 locations and 5, 20, and 50 cm depths below the surface. The SoilNet spreads across 6 ha and the sampling rate is 15 min (see also Fersch et al., 2018). Additional soil moisture profile observations are available in the vicinity of climate stations at the TERENO Pre-Alpine sites Graswang and Rottenbuch (Figure 1). These soil moisture sensors are located at 2, 6, 12, 25, 35, and 50 cm depths in three profiles that are displaced by about 1 m each and records available every 15 min. These observations are used in the present study for model evaluation purpose. The Fendt observatory is further equipped with a permanent CRNP (Table 1). In addition, a joint field campaign was carried out by the Cosmic Sense research unit from 16 May to 22 July 2019. During that time, a dense network of 24 CRNPs was installed around the Fendt site, distributed over 1 km2 within the headwater catchment of the upper Rott river. For the calibration of the CRNS network derived SWC, in situ root zone SWC observations were collected on June 25 and 26, 2019. They comprise 23 thermo-gravimetric and 139 FDR soil profiles. The CRNPs were placed with overlapping footprints to facilitate spatial maps of field scale SWC dynamics for the headwater catchment area (Fersch et al., 2020a).
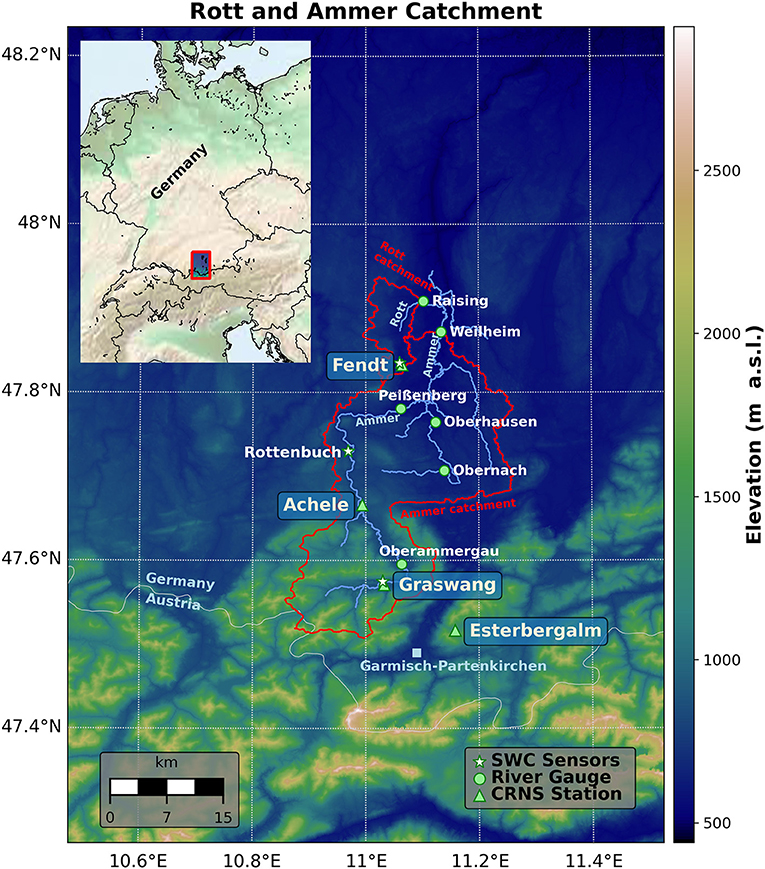
Figure 1. Location of the study area, the TERENO Pre-Alpine Observatory sites and river gauges. The elevation map was derived from the SRTM 30 meter DEM.

Table 1. CRNS station information on operational month, soil type, elevation (m.a.s.l.), and parameters used in the COSMIC forward operator, background cosmic radiation N (-), soil bulk density ρs (g/cm3), efficiency of fast neutron creation α (-), and shape parameter L3 (g/cm3).
The present study uses 4 CRNPs, operated within the boundaries of the model domain, for data assimilation purpose. The station's properties are listed in Table 1. The Esterbergalm station is located outside the study catchment but still within the model domain and therefore included in the analysis. The CRNS observations exhibit artifacts due to external influences, such as variations of the incoming neutron flux density, atmospheric water vapor, and barometric pressure. Thus, all the CRNS observations were corrected according to Zreda et al. (2012) using local pressure observations and incoming neutron variations measured at the Jungfraujoch, Switzerland (JUNG, https://www.nmdb.eu/) and according to Rosolem et al. (2013) for water vapor, also based on local observations.
To force the land surface model, the climatologically corrected DWD radar-based precipitation product RADOLAN-YW (Winterrath et al., 2018) was used. These data are available at 5 min interval, with 1 × 1 km resolution. Due to the lack of suitable observations in the study region, all other model forcing variables (temperature, wind speed, relative humidity, radiation, and surface pressure) were derived with the Weather Research and Forecasting modeling system (WRF Skamarock and Klemp, 2008), driven by the ERA-Interim reanalysis (Berrisford et al., 2011). The WRF model setup closely follows Fersch et al. (2020b).
The spatial variability and temporal dynamics of soil moisture within the land surface model framework is highly dependent on soil type information. The present study uses the high resolution 3D soil hydraulic EU SoilHydroGrids data set (v 1.0) of Tóth et al. (2017). This database provides soil water content at saturation, field capacity, and wilting point, soil saturated hydraulic conductivity, and Mualem–van Genuchten parameters for the description of the unsaturated hydraulic conductivity curves. All these soil hydraulic parameters are available at 250 and 1,000 m spatial resolution and for 6 layers below the surface (0–5, 5–15, 15–30, 30–60, 60–100, and 100–200 cm). The additionally required soil parameters, namely quartz, and saturated matric potential were obtained from Dai et al. (2019).
2.2. Land Surface Model
The Noah Multi-Parameterization (Noah-MP) land surface model (Niu et al., 2011) was selected to simulate the water and energy balance of the land surface. The Noah-MP land surface model is the successor of the Noah LSM (Chen and Dudhia, 2001) and used as an inherent land surface scheme in WRF. Noah-MP calculates a closed energy balance coupled with the water balance. The multi-parameterization options can be used to activate different formulations for interactive processes like the radiative transfer model, stomatal conductance, soil parameterization, snow dynamics, and runoff generation.
For the present study, a 100 m spatial discretization of Noah-MP was set-up over the entire spatial domain as shown in Figure 1. The resampled elevation map at 100 m resolution was derived using the 30 m global digital elevation model (GDEM) from the Advanced Spaceborne Thermal Emission and Reflection radiometer mission (ASTER, NASA, 2009). The land cover information was derived reclassifying the CORINE Land Cover (CLC) data set (Bttner, 2014) to the closest USGS land use class. The standard Noah-MP configuration possesses one canopy layer, two snow layers and 4 soil layers. In this study, the 4 soil layer parameterization was extended to 6 soil layers for compatibility with the EU-SoilHydroGrids data set. As this data set is based on the Mualem–van Genuchten soil water retention model, the Clapp and Hornberger (Clapp and Hornberger, 1978) scheme in standard Noah-MP configuration was complemented by an implementation of the Mualem–van Genuchten equations (Mualem, 1976; Van Genuchten, 1980). In the present study, all model simulations were carried out at hourly time step. The formulation for soil water estimation and parameterization relevant in the present study is briefly described here. A detailed description of Noah-MP can be found in Niu et al. (2011).
Table 2 lists the physical options that were chosen for the simulations with Noah-MP.
We selected the infiltration excess surface runoff scheme with gravitational free drainage of Schaake et al. (1996). The maximum infiltrated water over a given time step is computed as,
Where, P is the precipitation rate (mm s−1), Imax is the infiltration rate (mm s−1), δt is the model time step (days), D is the liquid soil moisture deficit of the modeled soil column (m) and given as , kdt is a shape parameter and given as kdt = kdtref(Ksat/Kref). Ksat is the saturated hydraulic conductivity (m s−1). Kref is the reference saturated hydraulic conductivity (m s−1) and commonly referred to as REFDK. In the standard Noah-MP configuration, REFDK is kept constant with a value of 2 × 10−6 m s−1 for the silt-clay-loam soil texture. kdtref is an infiltration capacity scaling parameter, commonly referred to as REFKDT and has a standard value of 3. Studies of Cuntz et al. (2016) and Rummler et al. (2019) showed that REFDK and REFKDT are sensitive to the surface runoff computation. As the quantity kdt in Equation (1) above is depending on both these parameters their sensitivity in computing Imax is interlinked (Cuntz et al., 2016). Therefore, in the present work REFDK is kept constant and spatially distributed values of REFKDT are updated based on the land-cover classification of the study domain. Figure 2 shows the spatial distribution of REFKDT where smaller values of REFKDT are mapped to areas with higher runoff potential (e.g., urban areas, barren land). The transport of liquid water within the soil column is simulated using the diffusive form of the Richards equation (Koren et al., 1999),
where, θ is the liquid soil water content (m3 m−3), K is the hydraulic conductivity (m s−1), D is the water diffusivity (m2 s−1) and S is the water storage. In the present study the functions K(θ) and D(θ) for unsaturated soils were calculated using the Mualem–van Genuchten soil water retention function,
where, Se is the effective saturation (0–1), α n, and m = 1 − (1/n) are shape parameters provided in 3D by the soil hydraulic database of Tóth et al. (2017). The effect of vegetation on evapotranspiration is accounted for by choosing the dynamic vegetation model of Yang and Niu (2003) and the Ball-Woodrow-Berry stomatal resistance method (Ball et al., 1987).
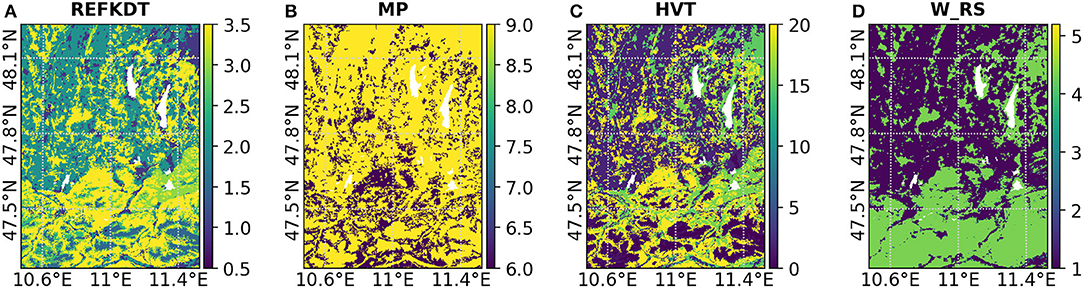
Figure 2. Spatial plots of parameters estimated during the data assimilation experiment (A) REFKDT (infiltration capacity scaling parameter), (B) MP (slope of Ball-Woodrow-Berry stomatal conductance model), (C) HVT (vegetation height in meters), and (D) W_RS (control soil surface layer resistance to evaporation).
2.3. Cosmic Forward Operator
To compare the observed neutron counts with model simulated soil moisture within the data assimilation framework a forward model is required. The Monte Carlo N-Particle eXtended (MCNPX) model is a software to compute the neutron counts of modeled soil moisture, however, due to its complexity it does not qualify for ensemble data assimilation applications. Therefore, a simple and analytical model, the COsmic-ray Soil Moisture Interaction Code (COSMIC), introduced by Shuttleworth et al. (2013) was used as forward operator in this work. The COSMIC method relies on three key assumptions. First it assumes that the available high energy neutrons to produce fast neutrons reduce exponentially with the soil depth. Secondly, the moderation of high energy neutrons to fast neutrons is isotropic at each soil layer. Finally, there is an exponential reduction of detected fast neutrons with respect to the distance from their origin to the detector. Based on these assumptions, the number of fast neutrons detected by the CRNS sensor can be derived using a single integral equation as,
where,
Here, N is number of high energy neutrons at soil surface, ρs is soil bulk density (g cm−3), ρw is the soil water density (g cm−3), z denotes soil depth (m), ms(z) and mw(z) are integrated mass per unit area of dry soil and water, respectively, (g cm−2), γ is the angle between the vertical below detector and each point in the measurement plane. Parameter α represents the relative efficiency of fast neutron creation by the soil and L1, L2, L3, and L4 (in g cm−3) are length constants related to the local soil properties. Shuttleworth et al. (2013) have calibrated the COSMIC operator against MCNPX model simulations at 42 CRNS sites distributed over the contiguous USA. In their analysis the parameters L1, L2, and L4 are found constant as 129.1 g cm−3, 162.0 g cm−3 and 3.16 g cm−3, respectively. Also, the calibrated values of the parameters alpha and L3 are correlated with the soil bulk density and their relation is given as,
The value of N in Equation (5) depends strongly on the site specific soil chemistry, vegetation cover and sensor characteristics. Therefore, it needs to be calibrated at the observation site using observed soil moisture information. In the present study, N was calibrated at the Fendt site, incorporating observations from 12 SoilNet profiles, radially distributed around the CRNS sensor in ~40 and ~80 m radius. The SoilNet sensor observations (placed at 5, 20, and 50 cm depths) were first depth averaged and later area weighted averaged to represent the average soil moisture for the sensor footprint. For the Achele, Graswang and Esterbergalm CRNS stations the soil moisture profiles were taken on July 4, August 22, and July 19, 2017, respectively. The soil profiles were spatially distributed at annulus distances of 2, 45, and 125 m for Achele and 2, 30, and 100 m for Graswang and Estbergalm. All measurements were taken at intervals of 5 cm depths from surface to 30 cm. After scaling the parameter N, using calibration data-sets at all 4 CRNS stations, the final parameters for the COSMIC operator at all CRNS stations are summarized in Table 1.
To depict the soil moisture for a CRNS sensor centered at a 100 m spatial model grid, the simulated soil moisture from Noah-MP was first spatially interpolated from four neighboring model grids of 100 m spatial resolution using an area weighted averaging method. After that, COSMIC was used to compute expected neutron counts at the soil surface using this spatially interpolated soil moisture at 6 Noah-MP soil layers (up to 2 meter depth). COSMIC first linearly interpolates the 6 soil layers information to 200 soil layers with 1 cm thickness, then computes the contribution of each soil layer to the total neutron flux at the surface to compute the expected neutron count. Although the COSMIC used soil moisture from all 6 modeled soil layers (up to 2 meter depth), only the top 60 to 80 cm soil depth contributed to the neutron count calculation as CNRPs can only measure up to 80 cm deep in dry soil conditions (Rosolem et al., 2013).
2.4. Data Assimilation Algorithm
The present work employs the Data Assimilation Research Testbed (DART) (Anderson et al., 2009; Rosolem et al., 2014) for the assimilation of CRNS neutron counts in the Noah-MP LSM. DART is an open-source framework developed by the National Center for Atmospheric Research and provides efficient data assimilation tools to implement with large-scale geophysical models. DART provides the capabilities for multiple data assimilation specific configurations for, e.g., inflation, localization, choice of data assimilation algorithms and a set of tools to effectively test assimilation performance. Another advantage of using DART is that it improves reproducibility by eliminating user-specific implementation differences. The present study uses the Ensemble Adjustment Kalman Filter (EAKF Anderson, 2001) as an assimilation algorithm. For the application of EAKF, the first step is to convert the model state to model predicted observation state as,
Where x is an ensemble of model states or augmented states and parameters, y is an ensemble of model predicted observations, H is a non-linear forward operator which maps the model state to the observations. In our case the COSMIC operator was used to compute expected neutron counts as a function of modeled soil moisture as introduced before. ψ represents the process noise with a zero mean multivariate normal distribution with covariance Q. The increment for each ensemble member is computed in observation space as,
where, Δy is the adjustment to each ensemble member in the observation space. The superscript a and f represent analysis and forecast, respectively, and subscript i represents ensemble member. The parameter R is observation variance and Qf is the variance of the model predicted observation. In this way, without calculating full error co-variances the model analysis is calculated with a minimum computational burden. Once the Δy are calculated, the final state update is computed using local linear regression. Multiple observations are processed sequentially in EAKF, where the analyzed state after assimilating the first observation is considered as the background state for the second observation. This ensures that the mean and covariance of the updated ensemble are consistent with theoretical analysis with the minimum computational requirement.
2.5. Experimental setup
2.5.1. Ensemble Generation
The uncertainties in the model input forcing were represented by stochastic realizations of precipitation and temperature data sets. The precipitation data was perturbed using log-normally distributed multiplicative noise with 0 mean and 0.2 mm mm−1 standard deviation (20% errors). The air temperature was perturbed using normally distributed additive noise with 0 mean and 2 K standard deviation. Both forcing variables were applied with spatially correlated noise derived using fast Fourier transform (Chabot et al., 2015; Han et al., 2015) with 10 km radius of influence. The model parameters REFKDT, MP, HVT and W_RS (as shown in Figure 2) were perturbed to represent model physics uncertainty. All four parameters were selected for their sensitivity based on Cuntz et al. (2016). The perturbation applied to a particular parameter value was spatially homogeneous. This setup was selected to avoid a spurious evolution of parameters over the space during assimilation. All four parameters were perturbed using log-normally distributed multiplicative noise with 0 mean and 0.1 standard deviation (10% errors). Furthermore, initial soil moisture was perturbed using normally distributed additive noise with 0 mean and 0.05 cm3 cm−3 standard deviation to have a satisfactory ensemble spread of model states at the start of the ensemble simulations. The measured neutron count rate follows Poisson statistics. It can be approximated by a normal distribution for a large number of events per unit time (>30) (Zreda et al., 2012; Schrön, 2016). Therefore, the observation error standard deviation is kept as , where Nobs is the observed neutron count. In this work, an ensemble size of 64 realizations was used. All the error terms used are based on literature (Kumar et al., 2009; Reichle et al., 2010; Han et al., 2015; Cuntz et al., 2016) and also a few trial model runs. To maintain sufficient ensemble spread during the data assimilation experiment, the spatially-varying Gaussian inflation (adaptive) by Anderson (2009) was used.
2.5.2. Simulation Setup
The main objective of the present study was to understand the potential of the field scale soil moisture estimates from CRNS sensors to improve model parameterization and the catchment scale soil moisture. To evaluate this, the present study used three model simulations namely open loop (OL), state estimation (ST), and state–parameter estimation (STP). The OL run serves as a baseline run without any data assimilation. During the ST run, soil moisture at each soil layer was updated by assimilating neutron counts from all four CRNS stations (Table 1). Similarly, during the STP run, soil moisture states and four input parameters (REFKDT, MP, HVT and W_RS; Figure 2) were updated. As a result, for the ST experiment, the state vector x in Equation (9) was comprised of soil moisture states at all soil layers across the catchment. For the STP experiment, x was comprised of soil moisture states and four selected model parameters across the catchment. To initialize the model states for all the members, ensemble model runs with perturbed parameters and input forcing were performed from 15 April, 2016 to 1 July 2017. This initialization run produced the model's initial conditions on 1st July 2017 for all three simulation experiments. The hourly neutron counts were assimilated once per day (at 0 H UTC) during both assimilation runs (ST and STP). This was done to prevent the ensemble spread from collapsing due to frequent data assimilation. The assimilation and model performance assessment was carried out during non-snow conditions (April 15–October 30) for the years 2017–2019. However, the model simulations were run with updated states (and parameters) over the winter season to preserve continuity (from 30th October until 15th April of next year).
Considering the availability of data at all 4 CRNS stations (Table 1), the assimilation was started on 1 July 2017 for both ST and STP runs and continued until 9 May 2019. The evaluation was carried out from 10 May to 30 August 2019. During the evaluation period, the respective initial conditions (model states and parameters) for ST and STP runs on 10 May 2019 were used to run the model without assimilation. The ST run during the evaluation period helps in understanding the effect of updated soil moisture states on the subsequent model simulations in absence of data assimilation. Similarly, the evaluation period during the STP run helps in understanding the effect of parameter estimation along with soil moisture initial condition on model simulations beyond the assimilation period.
The data assimilation performance was evaluated using depth weighted root zone soil moisture derived from SoilNet observations at the Fendt site (see section 2) and the SWC observations available in the vicinity of the climate stations at Graswang and Rottenbuch (Figure 1). The depth weighted soil moisture from sensor observations was computed by taking the mean of linearly interpolated SWC estimates at 1 cm thick soil layers within the root zone (0–60 cm). The Rottenbuch site is not equipped with a CRNP and therefore the modeled soil moisture evaluation at this site gives insights for assimilation benefits from sparsely located CRNS stations on catchment scale soil moisture simulations. To evaluate the improvements by assimilation beyond the footprint of a single CRNS observation, additional analysis was carried out using data from the dense temporary network of CRNPs (section 2). The statistical evaluation of simulated and observed soil moisture during all three model runs was carried out with Root Mean Square Error (RMSE) and Bias statistics.
3. Results and Discussion
3.1. Soil Moisture Evaluation
From Figure 3, it can be seen that for the open loop (OL) simulation the SWC states at Fendt and Graswang correlate well with the observations, nevertheless they were considerable drier, as indicated by the Bias statistics for OL (Table 3). Fendt and Graswang had a strong negative bias (–0.135 cm3 cm−3 and –0.123 cm3 cm−3), whereas Rottenbuch had only a small deviation (–0.024 cm3 cm−3). Likewise the RMSE values for the OL run (Table 3) were high for Fendt (0.143 cm3 cm−3), and Graswang (0.133 cm3 cm−3) and low for Rottenbuch (0.046 cm3 cm−3).
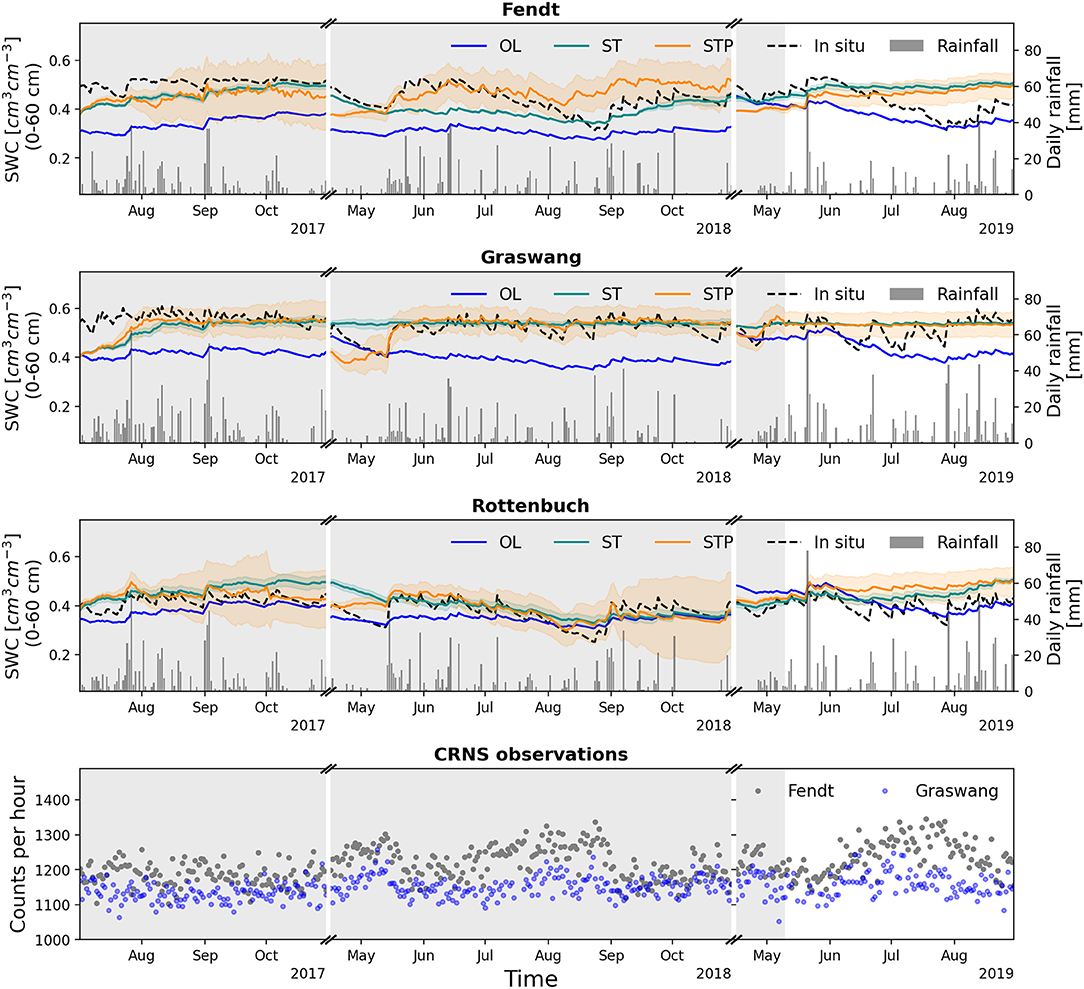
Figure 3. Temporal evolution of simulated soil moisture for the root zone (0–60 cm) from the open loop (OL), state estimation (ST) and state parameter estimation (STP) model runs during the assimilation (1 July 2017–09 May 2019) and evaluation (10 May 2019–30 Aug 2019) period at Fendt, Graswang and Rottenbuch observation sites along with observed (in-situ) soil moisture and Rainfall. The temporal evolution of observed CRNS neutron counts at Fendt and Graswang site are also shown as gray and blue dots, respectively. The aggregated SWC estimates were obtained by depth weighted averaging from all soil layers within the root zone depth.
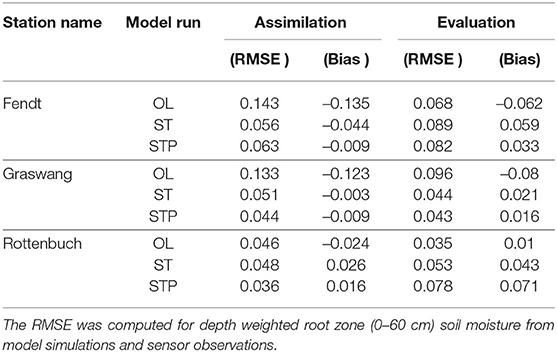
Table 3. RMSE (cm3 cm−3) and mean Bias (cm3 cm−3) for root zone soil moisture at three observation sites for OL, ST, and STP model runs for assimilation (1 July 2017–09 May 2019) and evaluation (10 May 2019–30 Aug 2019) period.
During the assimilation period (1 July 2017–9 May 2019), the simulated root zone SWC matches better with observations at Fendt and Graswang for both the state estimation (ST) and the state–parameter estimation (STP) assimilation runs (Figure 3). Among the assimilation runs, STP has resulted in relatively better performance for most of the simulation time for Graswang and Rottenbuch. For the STP run. the improvements of the RMSE were 67 and 21% of their OL value, respectively, for Graswang and Rottenbuch.
On the contrary, for Rottenbuch, the course of SWC for the ST run showed relatively wetter characteristics than observations, resulting in slightly higher RMSE compared to OL run (increase of RMSE by 4%). The wetter SWC updates for Rottenbuch during the ST run were mainly caused by the updates at the surface (0–5 cm and 5–15 cm) soil layers (see Figure A3). One of the reasons for slightly degraded SWC updates at the Rottenbuch site was the relatively low spatial correlation with observations from distant CRNPs. Another reason could be that during the OL simulation, the SWC predictions had small RMS errors at Rottenbuch, leaving little opportunity for improvement after assimilation. Despite this, the parameter estimation during the STP assimilation experiment enhanced the spatial correlations of SWC at the CRNP's and Rottenbuch site and improved the RMSE by 21%.
It is observed that during OL (mainly for Graswang and Rottenbuch), the variability of simulated SWC (corresponding to the soil water depletion rate) was much lower than the observed SWC (Figure 3). This variability in observation was mainly due to the high drying rate in the surface layer SWC (0–15 cm) at Graswang and Rottenbuch (Figures A2, A3). One of the reasons behind this was that the Graswang and Rottenbuch observation sites exhibit higher clay content for the upper soil layers (0–30 cm depth). Also, the use of the Mualem–van Genuchten (Van Genuchten, 1980) parameterisation in the present study has shown slower soil water movement within soil layers during OL as compared to observed SWC conditions. The SWC changes on a short time scale are better simulated during STP than during ST, as shown in the Figure 3. During STP assimilation, this helped to enhance the RMSE statistics at all observation sites.
During the evaluation period (10 May 2019–30 Aug 2019), the ST and STP assimilation runs yielded significantly wetter SWC characteristics at all observation sites. For both assimilation runs, the simulated SWC of the evaluation period does not resemble the temporal dynamics of the observations. Nonetheless, the SWC characteristics at the Graswang were clearly improved during the evaluation period. This is also reflected in the improvement in RMSE (55% reduction for ST and STP) and Bias (74% reduction for ST and 80% for STP) for Graswang (Table 3). The Bias statistics at Fendt site were improved (by 4% for ST and 46% for STP) during the evaluation period but the RMSE was increased by 30% compared to the OL run. The simulated SWC at the Rottenbuch site deviated larger from the observations than the OL for evaluation period. One of the reasons behind wetter SWC conditions at all three evaluation sites were the updates in the REFKDT parameter (discussed in section 3.2). REFKDT for Fendt was updated to a higher value (more than 5) at the end of the assimilation experiment. Further, it was found that the observation sites Fendt, Graswang and Rottenbuch share the same value for REFKDT. The updated REFKDT has increased the soil infiltration and therefore induced the positive bias in simulated SWC (wet soil water conditions) at all evaluation sites. In the present study, the observations from 4 CRNPs were assimilated to update the states and model parameters. However, all possible model parameter configurations were not covered by the locations of these 4 stations. Therefore, in order to estimate the associated parameters, it is necessary to have CRNPs in the catchment sufficiently covering all land use and soil types. This would help in efficiently improving the parameter estimation procedure and help in improving model performance for SWC estimation over the entire catchment. Another cause for the varying success in soil moisture characterization among assimilation studies at different sites is the comparatively short time (113 days) of the evaluation experiment when compared to the assimilation period (346 days). Longer duration of assessment can help reduce the fluctuations in the performance of simulated SWC induced by seasonal changes. Furthermore, the errors adopted for perturbing the initial conditions and model forcing were based on previous studies and a few trial simulations, and adaptive inflation by Anderson (2009) was employed to maintain enough ensemble spread during data assimilation. The observational errors, on the other hand, were derived based on past study by Schrön (2016) and were not explicitly evaluated. As a result, there could be more room to tune the forcing and observational errors in order to improve the assimilation performance. Additional research is required to optimize the forecast and observational errors in the data assimilation system.
3.2. Parameter Estimation
The temporal evolution for parameters REFKDT, MP, HVT and W_RS during the STP assimilation run is shown in Figure 4. The standard Noah-MP configuration uses a constant value (rk3.0) for REFKDT (Eq 1). At simulation initialization, the values of REFKDT are spatially varied from 0.5 to 3.5, based on the land use type information as shown in Figure 2. The temporal evolution of the individual REFKDT values during the STP assimilation mode is represented by rk0.5–rk3.5 (Figure 4). The spatially distributed parameter W_RS (Figure 2) is initially varied from 1 to 5.01 and its temporal evolution is shown as wr1.0 to wr5.01 in Figure 4. Similarly, the parameters MP and HVT are based on USGS land use categories and their temporal evolution is represented by mp6.0 and mp9.0 for MP and from hvt0.5 to hvt20.0 for HVT in Figure 4. For reference, the parameters representing the CRNP location at Fendt are printed as dashed red line.
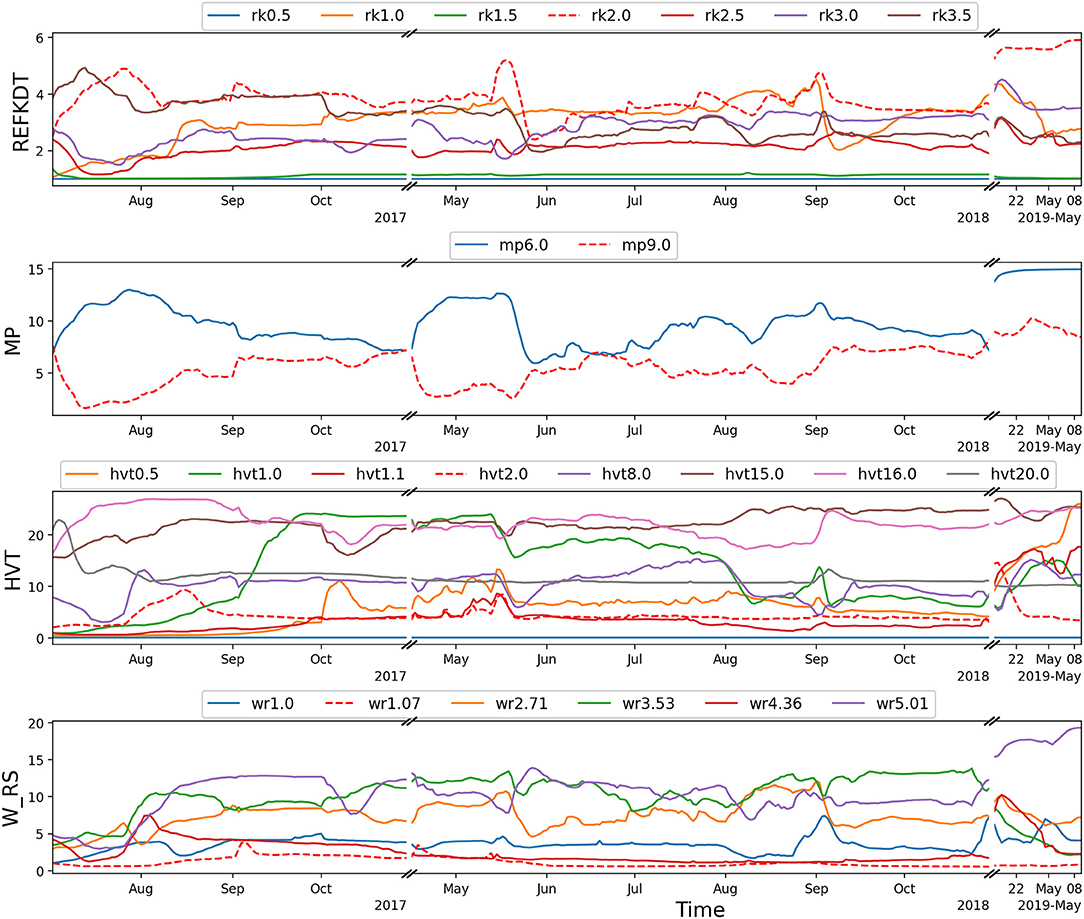
Figure 4. Parameter updates for REFKDT, MP, HVT, and W_RS during the STP assimilation run. The parameters representing the Fendt CRNP are printed as dashed red line.
From Figure 4, it is observed that the estimated parameters did not converge to constant values during the STP experiment. Among the parameters, REFKDT varies between 0.5 and 6 for the entire assimilation period which is also within the assumed physically meaningful range. Smaller values of REFKDT represent soils with lesser infiltration and higher runoff potential. During the STP run, REFKDT for Fendt (rk2.0) was increased (close to 6) thus increasing the soil infiltration and therefore compensating the negative Bias in simulated SWC as well as causing wet soil moisture conditions at all three observation sites (Fendt, Graswang, Rottenbuch) for the evaluation period (discussed in section 3.1). Similar patterns for REFKDT were found for the Estbergalm site (rk2.5). Here, relatively drier soil moisture conditions were observed from Apr 2018 to July 2018 (not shown) and rk2.5 evolved to compensate for the dry soil conditions by allowing more infiltration. According to Cuntz et al. (2016), the MP parameter has the highest sensitivity for transpiration computations. During STP, MP varied between 1 and 15 from initial values of 9 and 6, respectively. It was found that all four CRNP locations represented the same value for MP (mp9.0). During parameter estimation, mp9.0 evolved to the value 15, therefore yielded an transpiration increase at all three observation sites.
The parameter HVT (maximum height of vegetation canopy) which had initial values between 0–22 m evolved and 0–25 m over the entire assimilation period. For evergreen and deciduous forest (hvt16.0 and hvt20.0) the values were consistently above 20 m and finally updated to 24 and 25 m, respectively, at the end of assimilation period (on 9 May 2019). The parameters MP and HVT can have an interlinked feedback with respect to soil moisture and evapotranspiration calculations. Therefore, the estimated values of these two parameters may depend on each other. The parameter W_RS controls how fast water can be removed from the surface soil layer by evaporation and it is the most sensitive hard coded parameter for evaporation computation (Cuntz et al., 2016). In this study, wr5.0 is evolving within a broad range of 5 to 20 and all other courses of W_RS were optimized within the range of 1–6 toward the end of the STP run, thereby reducing the soil evaporation effectively for large parts of the catchment.
The evolution of the four parameters in Figure 4 indicates that updates in all sets of parameters over the assimilation period were dynamic and they did not converge toward a consistent value. In particular, modifications of the parameter values were noticeable near the end of the assimilation experiment throughout 2019. This could have adversely affected modeled SWC estimations throughout the STP assimilation cycle validation phase (as indicated in section 3.1). The temporally unstable parameter values therefore imply the possibility of various optimal seasonal parameter values. The selected parameters in the present study may not be the only error sources in the soil moisture estimation. For example, the assumption of a uniform root distribution for the removal of water from subsurface layers adds additional uncertainties. Such kind of uncertainties can also affect the performance of the parameter estimation. In addition, the current framework is not looking at the soil hydraulic parameters for calibration, because of the tremendous parameter space in the 3D EUSoilHydroGrids data sets. All of these factors may have an influence on the convergence of parameter values. In the end, only a much more rigorous multivariate assimilation study with a higher density of CRNPs (as opposed to 4 stations over 655 km2 in this study) and appropriate validation data sets would be able to address these shortcomings in the future.
Depending on the observation location, the parameters of the COSMIC operator are subject to different uncertainties which add to those of the parameters estimated during the data assimilation experiment. In particular the variation in bulk density (ρs) of soil within the CRNS footprint can lead to errors in estimation of parameter L3 and α. Furthermore, for the Achele, Graswang, and Esterbergalm sites, the scaling of parameter N in the COSMIC operator (Equation 5) was based on only 1 day of observed soil moisture information in the vicinity of these CRNPs. The performance of both the ST and STP experiments might have been affected as a consequence of these uncertainties.
3.3. Spatial Evaluation of Field Scale SWC
In the following it is analyzed, if the assimilation of cosmogenic neutron counts can improve the simulated SWC in the proximity of a CRNP. To that end the simulation results are evaluated with observations from the dense CRNS network experiment of Fersch et al. (2020a) for the 1 km2 Rott headwater catchment at Fendt (Figure 5). The root zone SWC estimates (0–60 cm) of the evaluation period (10 May 2019–30 Aug 2019), are compared against the derived soil moisture estimates from the dense CRNS sensor network on 6 different days (Figure 5), for the OL, ST, and STP assimilation runs. The CRNS based SWC estimates illustrated in Figure 5 were derived following the approach of Desilets et al. (2010). The depth weighted SWC from the model was computed with the depth distribution obtained from the COSMIC operator at the permanent CRNP location. The temporal evolution of spatial mean, spatial standard deviation of all four SWC estimates (CRNS, OL, ST and STP), spatial mean Bias, and spatial RMSE of the three model runs is visualized in Figure 6.
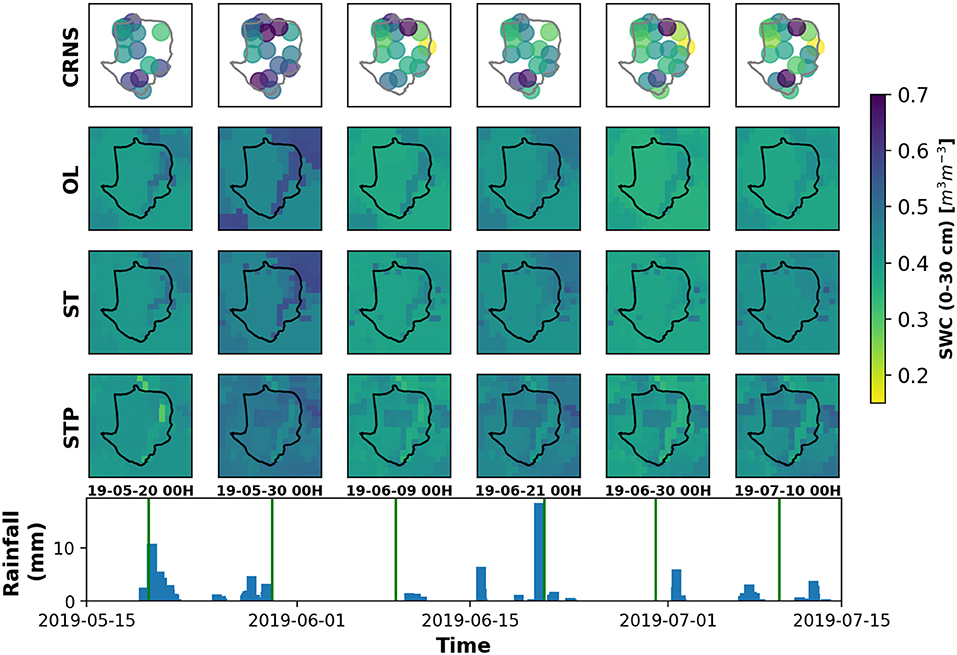
Figure 5. Rainfall and spatial distribution of field scale soil moisture on 20 May 19, 30 May 19, 9 June 19, 21 June 19, 30 June 19, and 10 July 19 at the Fendt study site from dense CRNS sensor network (Fersch et al., 2020a) and its comparison with simulated root zone soil moisture (0–60 cm) from OL, ST, and STP model runs for the evaluation period. The black line in the spatial soil moisture plots shows the boundary of the field campaign site and the vertical lines on the rainfall time series represent the selected time steps for the spatial plots.
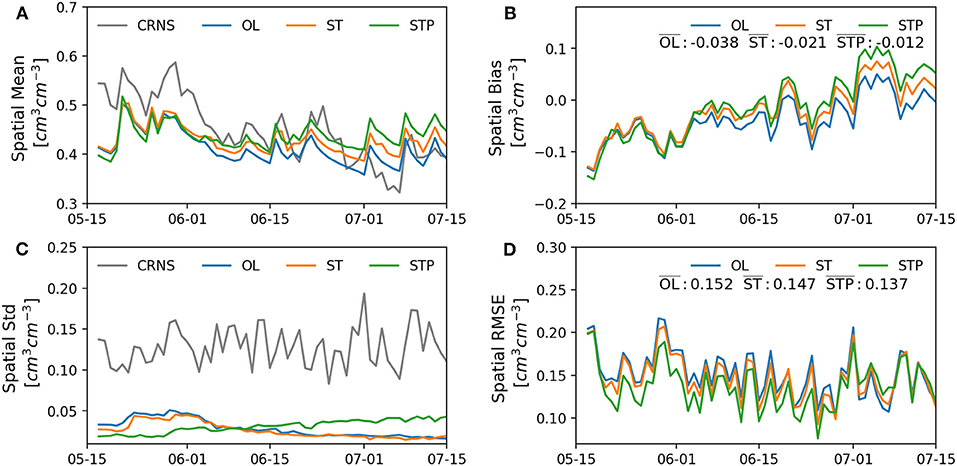
Figure 6. Temporal evolution of (A) spatial mean and (C) spatial standard deviation, respectively, for root zone SWC derived using CRNS and open loop (OL), state estimation (ST) and state–parameter estimation (STP) model runs. Similarly (B,D) represents the spatial Bias and spatial RMSE, respectively, during OL, ST, and STP model runs. The the statistics RMSE and Bias were computed with respect to CRNS derived SWC estimates at the Fendt study site from 15 May 19 to 16 July 19. Both the assimilation runs (ST and STP) soil moisture estimates belongs to the evaluation period (10 May 19–30 Aug 19).
In Figure 5, the CRNS derived SWCs are represented by circles with 150 m ground radius. High intensity rainfall events on May 20–21, May 29 and June 19 have resulted in wet soil moisture conditions over the entire field. Accordingly, the spatial SWC patterns on May 20, May 30, and June 21 show wet conditions across space. The spatial variability of the soil moisture observed by CRNS is largely dominated by the topography. The soil moisture condition around the watershed outlet in the north of the observation field shows wetter soil moisture conditions during the entire field campaign. This part of the catchment is characterized by higher water tables where capillary rise keeps the root zone SWC close to saturation. Due to the topography and land use patterns, SWC from CRNS exhibits a high spatial variability across the observation site (Figure 5). From Figure 6C it is observed that the spatial standard deviation of CRNS derived SWC ranges from 0.08 to 0.18 (cm3 cm−3).
The temporal evolution of spatial mean SWC from all three model runs correlates well with the CRNS derived SWC (Figure 6A). The simulated SWC maintained some negative Bias from 15 May to 5 June (Figure 6B). Nonetheless, the high variability of SWC states across space as observed from CRNS stations is not well reflected by all three model runs. As shown in Figures 5, 6C, OL and ST have very low spatial variability, while ST has a similar SWC progression to OL (Figure 3). This is due to the updated SWC states at the beginning of the evaluation period (on May 10th 2019) which had little influence to alter the spatial distribution of SWC for Fendt. Because of modified model parameters the SWC estimates for the STP run have a different spatial distribution than the OL run. The parameter estimation assisted in improving the spatial mean of simulated SWC (Figure 6A) and reducing the spatial Bias from –0.033 (cm3 cm−3) to –0.013 (cm3 cm−3) (Figure 6B). However, when compared to CRNS based SWC (Figure 6C) for the STP model run the overall spatial standard deviation increased slightly after 15 July 2019 (Figure 6C) and the soil moisture fields shows some variability (Figure 5). Therefore, the spatial RMSE improved from 0.152 (cm3 cm−3) in the OL simulation to 0.137 (cm3 cm−3) in the STP experiment.
The lack of spatial variability in model simulated SWC can have multiple causes. Noah-MP does not consider the lateral interaction of soil water between grid cells. Also, the assumption of the free drainage lower boundary in the model does not consider upward groundwater flow in the lowest soil layer. Therefore, the spatial variability in soil moisture due to lateral movement of water and high water table may not be well represented in model simulations. The obtained spatial heterogeneity in the simulated soil moisture for all three model runs (OL, ST and STP) is mainly governed by spatially variable forcing, land cover, and soil type parameterization. However, the rainfall and other forcing data sets may not be substantially variable over the 1 km2 observation site as both the WRF simulations and the gridded RADOLAN precipitation product have 1 km2 resolution. Another factor can be the presence of high clay contents in the soils at Fendt. The Mualem–van Genuchten soil parameterization is known to be relatively less accurate in clay type soils. Similar observations of the low spatial variance of simulated soil moisture in clay soil types are reported by Poltoradnev et al. (2018). In general, the average field soil moisture conditions are better represented by all model runs with improved simulations by the STP run due to updated model parameters. However, the assimilation of CRNS neutron counts at one observation site can not improve the characterization of the smaller-scale SWC variability for domains.
4. Summary and Conclusions
The presented study investigates the real world application of the CRNS technique for improving SWC states and parameter values for a Noah-MP land surface model configuration that features high resolution soil information and land use maps. Count rates of cosmogenic water sensitive neutrons were assimilated with an EAKF and the COSMIC forward operator. The study was conducted for the 655 km2 pre-alpine Ammer and Rott catchments with a spatial resolution of the land surface model of 100 m. The high resolution 3D soil hydraulic EU SoilHydroGrids data set of Tóth et al. (2017) was used along with the Mualem–van Genuchten (Van Genuchten, 1980) scheme to describe the vertical water transport in the soil. Soil water content dynamics and four sensitive model parameters controlling the infiltration and evaporation rates were estimated using the joint state–parameter estimation technique. Separate evaluations were made to assess the added value at assimilation locations, distant location from CRNS stations, and at a small catchment within the vicinity of the assimilation location.
The joint state—parameter estimation technique (STP) allowed to update the model parameter values within physically realistic ranges without any discrepancies in the underlying model physics and improved the SWC estimates locally, for the assimilation period. The parameter estimation helped mainly to reduce the error and Bias in model simulated SWC. The RMSE of simulated SWC after assimilation was improved by 60 and 55% for Fendt and 62 and 66% for Graswang during state estimation ST and STP assimilation runs, respectively. For the ST experiment, the SWC estimation at locations remote to the assimilation sites (Rottenbuch) was affected by imperfect forecast error co-variances and resulted in erroneous SWC estimates compared to OL simulation. The joint state—parameter estimation experiment helped to improve the forecast errors, therefore, helped to minimize the impact of drier SWC estimates at this location during assimilation experiment. During the assimilation period of the STP experiment, small temporal variations of the simulated SWC were better represented. These temporal changes in the simulated SWC were not effectively reflected during the evaluation period. Therefore, during the evaluation experiment with ST and STP assimilation runs, the performance of model simulated SWC exhibited mixed results. For all assimilation studies, the SWC characterization at Fendt and Graswang showed a reduced Bias compared to the OL run, however the reduction in RMSE was only detected at Graswang. The comparison of spatial SWC states with a dense network of CRNS stations at one of the observation sites shows that the joint state—parameter estimation experiment helped to improve the average SWC with the reduction of spatial Bias from –0.038 (cm3 cm−3) to –0.012 (cm3 cm−3). However, the assimilation of neutron counts at a single station showed partial success in altering the field-scale spatial patterns of estimated SWC (only during STP run) in the present assimilation framework. The limitations in improving the spatial SWC patterns can be attributed to the existing Noah-MP model physics which do not consider the 2D lateral soil water movement between grid cells, therefore, limiting the influence of topographic effects on SWC. Also, the assumptions of free drainage from lower soil layers was not valid at the evaluation site. Another important factor that needs to be considered is that the present study used the best quality available detests for meteorological forcing, soil type and land use information. Therefore, the data assimilation experiments resulted in minimal or no further improvements in SWC estimations at the locations where open-loop SWC states were reasonably accurate (e.g., for Rottenbuch). However, clear improvements were found for Bias and errors in simulated SWC across the catchment.
When compared to previous research that used microwave remote sensing-based soil moisture data assimilation to improve catchment scale SWC prediction, the CRNS technique clearly demonstrates several advantages. Information from CRNS observations greatly enhanced root zone soil moisture estimates at the observation sites, whereas improving the root zone estimates using surface soil moisture information from remote sensing products depends on model physics and needs special attention (Patil and Ramsankaran, 2018; Ju et al., 2020). When using CRNS data, improved soil moisture estimation at locations distant from the observation site can be achieved through parameter estimation and using cross-correlation between soil moisture states, whereas the spatial soil moisture patterns of the surface soil layer (0–5 cm) can be better represented when assimilating remote sensing-based products (Han et al., 2012). Furthermore, the root zone information on soil moisture from the CRNS technique provides the opportunity to optimize the parameters of multiple model processes ranging from soil infiltration to evapotranspiration. In future research, it would be desirable to incorporate the benefits of both CRNS and microwave remote sensing data products in land surface data assimilation applications.
In this study, only four stations were used to assimilate the neutron count information into the land surface model. The existing configuration may not be sufficient for considering the large variety in soil types, land use classes and slope patterns in the study area. Therefore, more CRNS stations are desirable to enhance the parameter estimation during the data assimilation. The present study did not update any parameters related to soil types due to the vast parameter space and to maintain consistency in the parameter sets. However, future work needs to be done to estimate the parameters relevant to the soil water routing method by optimizing the soil pedotransfer functions within the data assimilation framework. In future, the spatial patterns in model states can be improved by assimilation of mobile CRNPs that are suited to scan larger areas, even with diverse land cover properties while the stationarity assumption for the observed SWC still holds true.
Data Availability Statement
The raw data supporting the conclusions of this article will be made available by the authors, without undue reservation.
Author Contributions
HK, H-JH, and BF contributed to the conception and design of the study. BF contributed to the preparation of the input data sets and implemented the EU-SoilHydroGrids data set in Noah-MP LSM. AP prepared the DART-Noah-MP assimilation setup, carried out the ensemble data assimilation, and drafted the manuscript. AP and BF performed the analysis. All authors revised and approved the submitted version.
Funding
This work was supported by the Deutsche Forschungsgemeinschaft (grant no. FOR 2694), and the Helmholtz Association initiatives Terrestrial Environmental Observatories (TERENO), Modular Observation Solutions for Earth Systems (MOSES), and Advanced Earth System Model Capacity (ESM).
Conflict of Interest
The authors declare that the research was conducted in the absence of any commercial or financial relationships that could be construed as a potential conflict of interest.
Publisher's Note
All claims expressed in this article are solely those of the authors and do not necessarily represent those of their affiliated organizations, or those of the publisher, the editors and the reviewers. Any product that may be evaluated in this article, or claim that may be made by its manufacturer, is not guaranteed or endorsed by the publisher.
Acknowledgments
The authors gratefully acknowledge the Gauss Centre for Supercomputing e.V. (www.gauss-centre.eu) for funding this project by providing computing time on the GCS Supercomputer SuperMUC at the Leibniz Supercomputing Centre (www.lrz.de). The authors also acknowledge and thank to the Cosmic Sense research unit for freely sharing the high-quality CRNS observations.
Supplementary Material
The Supplementary Material for this article can be found online at: https://www.frontiersin.org/articles/10.3389/frwa.2021.729592/full#supplementary-material
References
Anderson, J. (2009). Spatially and temporally varying adaptive covariance inflation for ensemble filters. Tellus A 61, 72–83. doi: 10.1111/j.1600-0870.2008.00361.x
Anderson, J., Hoar, T., Raeder, K., Liu, H., Collins, N., Torn, R., et al. (2009). The data assimilation research testbed: a community facility. Bull. Am. Meteorol. Soc. 90, 1283–1296. doi: 10.1175/2009BAMS2618.1
Anderson, J. L. (2001). An ensemble adjustment Kalman filter for data assimilation. Mon. Weather Rev. 129, 2884–2903. doi: 10.1175/1520-0493(2001)129andlt;2884:AEAKFFandgt;2.0.CO;2
Andreasen, M., Jensen, K. H., Desilets, D., Franz, T. E., Zreda, M., Bogena, H. R., et al. (2017). Status and perspectives on the cosmic-ray neutron method for soil moisture estimation and other environmental science applications. Vadose Zone J. 16, 1–11. doi: 10.2136/vzj2017.04.0086
Baatz, R., Hendricks Franssen, H.-J., Han, X., Hoar, T., Bogena, H. R., and Vereecken, H. (2017). Evaluation of a cosmic-ray neutron sensor network for improved land surface model prediction. Hydrol. Earth Syst. Sci. 21, 2509–2530. doi: 10.5194/hess-21-2509-2017
Ball, J. T., Woodrow, I. E., and Berry, J. A. (1987). “A model predicting stomatal conductance and its contribution to the control of photosynthesis under different environmental conditions,” in Progress in Photosynthesis Research (Dordrecht: Springer), 221–224.
Berrisford, P., Dee, D., Poli, P., Brugge, R., Fielding, M., Fuentes, M., et al. (2011). The Era-Interim Archive Version 2.0. Technical Report 1, ECMWF, Shinfield Park, Reading.
Bogena, H. R., Huisman, J. A., Oberdörster, C., and Vereecken, H. (2007). Evaluation of a low-cost soil water content sensor for wireless network applications. J. Hydrol. 344, 32–42. doi: 10.1016/j.jhydrol.2007.06.032
Brocca, L., Ciabatta, L., Massari, C., Camici, S., and Tarpanelli, A. (2017). Soil moisture for hydrological applications: open questions and new opportunities. Water 9, 140. doi: 10.3390/w9020140
Chabot, V., Nodet, M., Papadakis, N., and Vidard, A. (2015). Accounting for observation errors in image data assimilation. Tellus A 67, 23629. doi: 10.3402/tellusa.v67.23629
Chen, F., Crow, W. T., Starks, P. J., and Moriasi, D. N. (2011). Improving hydrologic predictions of a catchment model via assimilation of surface soil moisture. Adv. Water Resour. 34, 526–536. doi: 10.1016/j.advwatres.2011.01.011
Chen, F., and Dudhia, J. (2001). Coupling an advanced land surface-hydrology model with the Penn State-NCAR MM5 modeling system. Part I: Model implementation and sensitivity. Mon. Weather Rev. 129, 569–585. doi: 10.1175/1520-0493(2001)129andlt;0569:CAALSHandgt;2.0.CO;2
Clapp, R. B., and Hornberger, G. M. (1978). Empirical equations for some soil hydraulic properties. Water Resour. Res. 14, 601–604. doi: 10.1029/WR014i004p00601
Cuntz, M., Mai, J., Samaniego, L., Clark, M., Wulfmeyer, V., Branch, O., et al. (2016). The impact of standard and hard-coded parameters on the hydrologic fluxes in the Noah-MP land surface model. J. Geophys. Res. Atmospheres 121, 10–676. doi: 10.1002/2016JD025097
Dai, Y., Xin, Q., Wei, N., Zhang, Y., Shangguan, W., Yuan, H., et al. (2019). A global high-resolution data set of soil hydraulic and thermal properties for land surface modeling. J. Adv. Model. Earth Syst. 11, 2996–3023. doi: 10.1029/2019MS001784
De Lannoy, G. J., and Reichle, R. H. (2016). Assimilation of SMOS brightness temperatures or soil moisture retrievals into a land surface model. Hydrol. Earth Syst. Sci. 20, 4895–4911. doi: 10.5194/hess-20-4895-2016
Desilets, D., and Zreda, M. (2013). Footprint diameter for a cosmic-ray soil moisture probe: theory and monte carlo simulations. Water Resour. Res. 49, 3566–3575. doi: 10.1002/wrcr.20187
Desilets, D., Zreda, M., and Ferré, T. P. (2010). Nature's neutron probe: Land surface hydrology at an elusive scale with cosmic rays. Water Resour. Res. 46:W11505. doi: 10.1029/2009WR008726
Dickinson, R. E., Shaikh, M., Bryant, R., and Graumlich, L. (1998). Interactive canopies for a climate model. J. Climate 11, 2823–2836. doi: 10.1175/1520-0442(1998)011andlt;2823:ICFACMandgt;2.0.CO;2
Entin, J. K., Robock, A., Vinnikov, K. Y., Hollinger, S. E., Liu, S., and Namkhai, A. (2000). Temporal and spatial scales of observed soil moisture variations in the extratropics. J. Geophys. Res. Atmospheres 105, 11865–11877. doi: 10.1029/2000JD900051
Fersch, B., Francke, T., Heistermann, M., Schrön, M., Döpper, V., Jakobi, J., et al. (2020a). A dense network of cosmic-ray neutron sensors for soil moisture observation in a highly instrumented pre-Alpine headwater catchment in Germany. Earth Syst. Sci. Data 12, 2289–2309. doi: 10.5194/essd-12-2289-2020
Fersch, B., Jagdhuber, T., Schrön, M., Völksch, I., and Jäger, M. (2018). Synergies for soil moisture retrieval across scales from airborne polarimetric SAR, cosmic ray neutron roving, and an in situ sensor network. Water Resour. Res. 54, 9364–9383. doi: 10.1029/2018WR023337
Fersch, B., Senatore, A., Adler, B., Arnault, J., Mauder, M., Schneider, K., et al. (2020b). High-resolution fully coupled atmospheric-hydrological modeling: a cross-compartment regional water and energy cycle evaluation. Hydrol. Earth Syst. Sci. 24, 2457–2481. doi: 10.5194/hess-24-2457-2020
Han, X., Franssen, H.-J., Rosolem, R., Jin, R., Li, X., and Vereecken, H. (2015). Correction of systematic model forcing bias of CLM using assimilation of cosmic-ray Neutrons and land surface temperature: a study in the Heihe Catchment, China. Hydrol. Earth Syst. Sci. 19, 615–629. doi: 10.5194/hess-19-615-2015
Han, X., Li, X., Hendricks Franssen, H., Vereecken, H., and Montzka, C. (2012). Spatial horizontal correlation characteristics in the land data assimilation of soil moisture. Hydrol. Earth Syst. Sci. 16, 1349–1363. doi: 10.5194/hess-16-1349-2012
Hendricks Franssen, H., and Kinzelbach, W. (2008). Real-time groundwater flow modeling with the ensemble Kalman filter: joint estimation of states and parameters and the filter inbreeding problem. Water Resour. Res. 44:W09408. doi: 10.1029/2007WR006505
Johnson, A. I. (1962). Methods of Measuring Soil Moisture in the Field. US Department of the Interior, US Geological Survey.
Ju, F., An, R., Yang, Z., Huang, L., and Sun, Y. (2020). Assimilating smos brightness temperature for hydrologic model parameters and soil moisture estimation with an immune evolutionary strategy. Remote Sens. 12:1556. doi: 10.3390/rs12101556
Kerr, Y. H., Waldteufel, P., Richaume, P., Wigneron, J. P., Ferrazzoli, P., Mahmoodi, A., et al. (2012). The smos soil moisture retrieval algorithm. IEEE Trans. Geosci. Remote Sens. 50, 1384–1403. doi: 10.1109/TGRS.2012.2184548
Kiese, R., Fersch, B., Bassler, C., Brosy, C., Butterbach-Bahlc, K., Chwala, C., et al. (2018). The TERENO Pre-Alpine observatory: integrating meteorological, hydrological, and biogeochemical measurements and modeling. Vadose Zone J. 17, 1–17. doi: 10.2136/vzj2018.03.0060
Köhli, M., Schrön, M., Zreda, M., Schmidt, U., Dietrich, P., and Zacharias, S. (2015). Footprint characteristics revised for field-scale soil moisture monitoring with cosmic-ray neutrons. Water Resour. Res. 51, 5772–5790. doi: 10.1002/2015WR017169
Koren, V., Schaake, J., Mitchell, K., Duan, Q.-Y., Chen, F., and Baker, J. (1999). A parameterization of snowpack and frozen ground intended for NCEP weather and climate models. J. Geophys. Res. Atmospheres 104, 19569–19585. doi: 10.1029/1999JD900232
Kumar, S. V., Reichle, R. H., Koster, R. D., Crow, W. T., and Peters-Lidard, C. D. (2009). Role of subsurface physics in the assimilation of surface soil moisture observations. J. Hydrometeorol. 10, 1534–1547. doi: 10.1175/2009JHM1134.1
Kumar, S. V., Reichle, R. H., Peters-Lidard, C. D., Koster, R. D., Zhan, X., Crow, W. T., et al. (2008). A land surface data assimilation framework using the land information system: description and applications. Adv. Water Resour. 31, 1419–1432. doi: 10.1016/j.advwatres.2008.01.013
McColl, K. A., Alemohammad, S. H., Akbar, R., Konings, A. G., Yueh, S., and Entekhabi, D. (2017). The global distribution and dynamics of surface soil moisture. Nat. Geosci. 10, 100–104. doi: 10.1038/ngeo2868
Mishra, V., Shah, R., and Thrasher, B. (2014). Soil moisture droughts under the retrospective and projected climate in India. J. Hydrometeorol. 15, 2267–2292. doi: 10.1175/JHM-D-13-0177.1
Moradkhani, H., Sorooshian, S., Gupta, H. V., and Houser, P. R. (2005). Dual state-parameter estimation of hydrological models using ensemble Kalman filter. Adv. Water Resour. 28, 135–147. doi: 10.1016/j.advwatres.2004.09.002
Mualem, Y. (1976). A new model for predicting the hydraulic conductivity of unsaturated porous media. Water Resour. Res. 12, 513–522. doi: 10.1029/WR012i003p.00513
NASA, M. (2009). AIST, Japan Space Systems, and US/Japan ASTER Science Team (2009). ASTER Global Digital Elevation Model 3.
Niu, G.-Y., Yang, Z.-L., Mitchell, K. E., Chen, F., Ek, M. B., Barlage, M., et al. (2011). The community Noah land surface model with multiparameterization options (Noah-MP): 1. Model description and evaluation with local-scale measurements. J. Geophys. Res. Atmospheres 116:D12109. doi: 10.1029/2010JD015139
Njoku, E. G., Jackson, T. J., Lakshmi, V., Chan, T. K., and Nghiem, S. V. (2003). Soil moisture retrieval from amsr-e. IEEE Trans. Geosci. Remote Sens. 41, 215–229. doi: 10.1109/TGRS.2002.808243
Pathiraja, S., Marshall, L., Sharma, A., and Moradkhani, H. (2016). Hydrologic modeling in dynamic catchments: a data assimilation approach. Water Resour. Res. 52, 3350–3372. doi: 10.1002/2015WR017192
Patil, A., and Ramsankaran, R. (2018). Improved streamflow simulations by coupling soil moisture analytical relationship in enkf based hydrological data assimilation framework. Adv Water Resour. 121:173–188. doi: 10.1016/j.advwatres.2018.08.010
Pauwels, V. R., Balenzano, A., Satalino, G., Skriver, H., Verhoest, N. E., and Mattia, F. (2009). Optimization of soil hydraulic model parameters using synthetic aperture radar data: an integrated multidisciplinary approach. IEEE Trans. Geosci. Remote Sens. 47, 455–467. doi: 10.1109/TGRS.2008.2007849
Poltoradnev, M., Ingwersen, J., Imukova, K., Högy, P., Wizemann, H.-D., and Streck, T. (2018). How well does Noah-MP simulate the regional mean and spatial variability of topsoil water content in two agricultural landscapes in southwest Germany? J. Hydrometeorol. 19, 555–573. doi: 10.1175/JHM-D-17-0169.1
Reichle, R. H., Kumar, S. V., Mahanama, S. P., Koster, R. D., and Liu, Q. (2010). Assimilation of satellite-derived skin temperature observations into land surface models. J. Hydrometeorol. 11, 1103–1122. doi: 10.1175/2010JHM1262.1
Rosolem, R., Hoar, T., Arellano, A., Anderson, J. L., Shuttleworth, W. J., Zeng, X., et al. (2014). Translating aboveground cosmic-ray neutron intensity to high-frequency soil moisture profiles at sub-kilometer scale. Hydrol. Earth Syst. Sci. 18, 4363–4379. doi: 10.5194/hess-18-4363-2014
Rosolem, R., Shuttleworth, W. J., Zreda, M., Franz, T., Zeng, X., and Kurc, S. (2013). The effect of atmospheric water vapor on neutron count in the cosmic-ray soil moisture observing system. J. Hydrometeorol. 14, 1659–1671. doi: 10.1175/JHM-D-12-0120.1
Rummler, T., Arnault, J., Gochis, D., and Kunstmann, H. (2019). Role of lateral terrestrial water flow on the regional water cycle in a complex terrain region: Investigation with a fully coupled model system. J. Geophys. Res. Atmospheres 124, 507–529. doi: 10.1029/2018JD029004
Sakaguchi, K., and Zeng, X. (2009). Effects of soil wetness, plant litter, and under-canopy atmospheric stability on ground evaporation in the Community Land Model (CLM3. 5). J. Geophys. Res. Atmospheres, 114. doi: 10.1029/2008JD010834
Schaake, J. C., Koren, V. I., Duan, Q.-Y., Mitchell, K., and Chen, F. (1996). Simple water balance model for estimating runoff at different spatial and temporal scales. J. Geophys. Res. Atmospheres 101, 7461–7475. doi: 10.1029/95JD02892
Schrön, M. (2016). Cosmic-Ray Neutron Sensing and Its Applications to Soil and Land Surface Hydrology: On Neutron Physics, Method Development, and soil Moisture Estimation Across Scales. Universität Potsdam.
Schrön, M., Rosolem, R., Köhli, M., Piussi, L., Schröter, I., Iwema, J., et al. (2018). Cosmic-Ray Neutron Rover surveys of field soil moisture and the influence of roads. Water Resour. Res. 54, 6441–6459. doi: 10.1029/2017WR021719
Shuttleworth, J., Rosolem, R., Zreda, M., and Franz, T. (2013). The COsmic-ray soil moisture interaction code (COSMIC) for use in data assimilation. Hydrol. Earth Syst. Sci. 17, 3205–3217. doi: 10.5194/hess-17-3205-2013
Skamarock, W. C., and Klemp, J. B. (2008). A time-split nonhydrostatic atmospheric model for weather research and forecasting applications. J. Comput. Phys. 227, 3465–3485. doi: 10.1016/j.jcp.2007.01.037
Small, E. E., and Kurc, S. A. (2003). Tight coupling between soil moisture and the surface radiation budget in semiarid environments: implications for land-atmosphere interactions. Water Resour. Res. 39:SWC41-SWC414. doi: 10.1029/2002WR001297
Tóth, B., Weynants, M., Pásztor, L., and Hengl, T. (2017). 3D soil hydraulic database of Europe at 250 m resolution. Hydrol. Process 31, 2662–2666. doi: 10.1002/hyp.11203
Van Genuchten, M. T. (1980). A closed-form equation for predicting the hydraulic conductivity of unsaturated soils. Soil Sci. Soc. Am. J. 44, 892–898. doi: 10.2136/sssaj1980.03615995004400050002x
Walker, J. P., Willgoose, G. R., and Kalma, J. D. (2004). In situ measurement of soil moisture: a comparison of techniques. J. Hydrol. 293, 85–99. doi: 10.1016/j.jhydrol.2004.01.008
Winterrath, T., Brendel, C., Hafer, M., Junghnel, T., Klameth, A., Lengfeld, K., et al. (2018). RADKLIM Version 2017.002: Reprocessed quasi gauge-adjusted radar data, 5-minute precipitation sums (YW). doi: 10.5676/DWD/RADKLIM_YW_V2017.002
Xie, X., and Zhang, D. (2010). Data assimilation for distributed hydrological catchment modeling via ensemble Kalman filter. Adv. Water Resour. 33, 678–690. doi: 10.1016/j.advwatres.2010.03.012
Yang, K., Zhu, L., Chen, Y., Zhao, L., Qin, J., Lu, H., et al. (2016). Land surface model calibration through microwave data assimilation for improving soil moisture simulations. J. Hydrol. 533, 266–276. doi: 10.1016/j.jhydrol.2015.12.018
Yang, Z.-L., and Niu, G.-Y. (2003). The versatile integrator of surface and atmosphere processes: Part 1. Model description. Glob. Planetary Change 38, 175–189. doi: 10.1016/S0921-8181(03)00028-6
Zreda, M., Desilets, D., Ferré, T., and Scott, R. L. (2008). Measuring soil moisture content non-invasively at intermediate spatial scale using cosmic-ray neutrons. Geophys. Res. Lett. 35:D01107. doi: 10.1029/2008GL035655
Zreda, M., Shuttleworth, W., Zeng, X., Zweck, C., Desilets, D., Franz, T., et al. (2012). COSMOS: The cosmic-ray soil moisture observing system. Hydrol. Earth Syst. Sci. 16, 4079–4099. doi: 10.5194/hess-16-4079-2012
Keywords: cosmic-ray neutron sensing, ensemble adjustment Kalman filter, DART, soil moisture, data assimilation, land surface modeling
Citation: Patil A, Fersch B, Hendricks Franssen H-J and Kunstmann H (2021) Assimilation of Cosmogenic Neutron Counts for Improved Soil Moisture Prediction in a Distributed Land Surface Model. Front. Water 3:729592. doi: 10.3389/frwa.2021.729592
Received: 23 June 2021; Accepted: 19 August 2021;
Published: 16 September 2021.
Edited by:
Yanjun Gan, University of Texas at Arlington, United StatesCopyright © 2021 Patil, Fersch, Hendricks Franssen and Kunstmann. This is an open-access article distributed under the terms of the Creative Commons Attribution License (CC BY). The use, distribution or reproduction in other forums is permitted, provided the original author(s) and the copyright owner(s) are credited and that the original publication in this journal is cited, in accordance with accepted academic practice. No use, distribution or reproduction is permitted which does not comply with these terms.
*Correspondence: Harald Kunstmann, harald.kunstmann@kit.edu