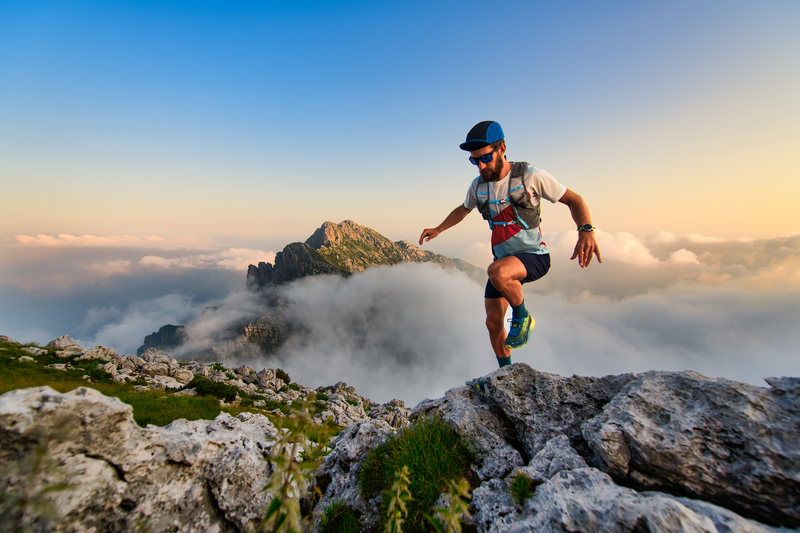
94% of researchers rate our articles as excellent or good
Learn more about the work of our research integrity team to safeguard the quality of each article we publish.
Find out more
REVIEW article
Front. Water , 05 October 2021
Sec. Water and Hydrocomplexity
Volume 3 - 2021 | https://doi.org/10.3389/frwa.2021.728156
This article is part of the Research Topic Water Resources of Mountainous Basins in a Changing Climate View all 4 articles
Climate-influenced changes in hydrology affect water-food-energy security that may impact up to two billion people downstream of the High Mountain Asia (HMA) region. Changes in water supply affect energy, industry, transportation, and ecosystems (agriculture, fisheries) and as a result, also affect the region's social, environmental, and economic fabrics. Sustaining the highly interconnected food-energy-water nexus (FEWN) will be a fundamental and increasing challenge under a changing climate regime. High variability in topography and distribution of glaciated and snow-covered areas in the HMA region, and scarcity of high resolution (in-situ) data make it difficult to model and project climate change impacts on individual watersheds. We lack basic understanding of the spatial and temporal variations in climate, surface impurities in snow and ice such as black carbon and dust that alter surface albedo, and glacier mass balance and dynamics. These knowledge gaps create challenges in predicting where and when the impact of changes in river flow will be the most significant economically and ecologically. In response to these challenges, the United States National Aeronautics and Space Administration (NASA) established the High Mountain Asia Team (HiMAT) in 2016 to conduct research to address knowledge gaps. This paper summarizes some of the advances HiMAT made over the past 5 years, highlights the scientific challenges in improving our understanding of the hydrology of the HMA region, and introduces an integrated assessment framework to assess the impacts of climate changes on the FEWN for the HMA region. The framework, developed under a NASA HMA project, links climate models, hydrology, hydropower, fish biology, and economic analysis. The framework could be applied to develop scientific understanding of spatio-temporal variability in water availability and the resultant downstream impacts on the FEWN to support water resource management under a changing climate regime.
The World Economic Forum identifies water crises, energy price fluctuations, and climate change as the top three risks the world faces in the next decade. These environmental risks have been gaining prominence with the rise in carbon dioxide emissions (World Economic Forum., 2019) and global annual temperature and radiative forcing (Hawkins et al., 2017; Kramer et al., 2021). High Mountain Asia (HMA, Figure 1), extending from the Hindu Kush and Tien Shan in the northwest to eastern Himalaya in the southeast (Bolch et al., 2012; Guo et al., 2015), is particularly vulnerable to these changes in climate. The region has extensive snow and glacier cover responsible for regulating downstream water supply, with impacts on water-food-energy security (Viviroli et al., 2011; Lutz et al., 2014; Biemans et al., 2019; Immerzeel et al., 2020; Mishra et al., 2020). Changes in HMA hydrology may impact up to two billion people downstream (Akhtar et al., 2008; Biemans et al., 2019), affecting agriculture, ecosystems, industry, transportation, energy, fisheries, and recreation, and as a result, the social, environmental and economic fabrics of HMA (Barnett et al., 2005; Viviroli et al., 2007; Moors et al., 2011). Sustainability of the highly interconnected Food-Energy-Water Nexus (FEWN) will be a fundamental and increasing challenge in a changing regional climate regime (D'Odorico et al., 2018). The grand challenge for HMA is in understanding how climate change will impact the FEWN and identifying water resource management plans that can mitigate pending risks and foster Sustainable Development Goals (SDGs) in these vulnerable nations.
Figure 1. Terrain (grayscale basemap), glaciers (dark blue), snow cover days (coral), rivers (cyan lines), and hydrological basins (black lines) of High Mountain Asia and the surrounding regions. Major glacierized basins are labeled for reference. Data sources: OpenStreetMap, RGI v 6.0 (Pfeffer et al., 2014), STC-MODSCAG snow cover days (Rittger et al., 2020), Global HydroSHEDS (Lehner et al., 2008).
The highly variable topography and distribution of glaciated and snow covered areas in HMA, coupled with an overall lack of ground observations, makes it difficult to predict the response of individual watersheds to projected changes in climate. As a consequence, we lack a basic understanding of the relative impact of key physical drivers on HMA river flow. These drivers include spatial and temporal variations in climate, surface impurities such as black carbon and dust that alter surface albedo, and glacier mass balance and dynamics. These knowledge gaps create challenges in predicting where and when the impact of change in river flow will be the most significant economically and ecologically. In response to these challenges, the United States National Aeronautics and Space Administration (NASA) initiated the High Mountain Asia Team (HiMAT) in 2016.
This paper summarizes the scientific challenges in improving our understanding of the hydrology of the HMA region. We highlight findings from HiMAT that focus primarily on the use of remote sensing data, models and reanalysis products to assess historical and project future variations in water resource availability (Arendt et al., 2020). The important measurement, monitoring, and/or modeling components to consider include the cryosphere (snow, glaciers, and permafrost), topography, land use and land cover, meteorological observations, stream-gauge data, and groundwater level observations. We focus on the major challenges of high resolution hydrologic modeling in HMA in the context of FEWN security and vulnerability, particularly in (1) understanding the significant physical drivers of hydrological processes at sub-basin levels; (2) aggregating individual streamflow contributors into a comprehensive assessment of total water budgets for every catchment; (3) projection of hydrologic flow under various climate scenarios, and (4) translating the impacts of changed hydrology into food and energy production, as well as in the maintenance of biodiversity at sub-basin levels. We summarize the remotely sensed and reanalysis products available at high temporal and spatial resolution that could be used to force and calibrate various components of hydrological system models at high resolution to reduce the uncertainties in projected hydrologic changes under various likely climate scenarios. Finally, we discuss the associated challenges in predicting changes in river flow at sub-basin level where decisions made affect FEWN security.
HMA rivers receive water from glacier and snow melt, rainfall, surface water, baseflow, and groundwater flow. The timing and magnitude of river discharge depend on an integrated assessment of these runoff sources, each of which varies with climatic and geographic location (Immerzeel and Bierkens, 2012). For example, stream flow in the upper Ganges Basin is dominated by rainfall runoff due to heavy monsoon precipitation, whereas streamflow in the Karakoram subbasins of the Upper Indus watershed is dominated by glacier melt water (Lutz et al., 2014, 2016). Each runoff source also impacts river flow over distinct time spans, with precipitation often making instantaneous contributions to river flow, while glaciers and snow store water temporarily and contribute to runoff later. Glaciers provide a vital renewable water resource to sustain downstream watersheds, especially during periods of drought (Pritchard, 2019). Human activity also plays a key role in altering river dynamics through irrigation and the building of reservoirs, as well as in assessing water scarcity through hydroelectric and water resource demands.
Measurements of streamflow across the HMA region are extremely sparse, creating considerable challenges in calibrating physical or statistical models of river runoff. Therefore, discharge is often assessed through a combined estimation of runoff sources. In this section, we discuss the challenges associated with the quantification of precipitation, glacier mass balance, snow cover/water equivalent, lakes, and groundwater for predicting streamflow at the sub-basin scale. We highlight the role of reanalysis products, modeling and assimilation, and remote sensing in assessing the physical drivers of HMA hydrology in the absence of in-situ observations.
HMA has complex meteorological conditions in part due to its highly variable topography. The region has a mean elevation exceeding 4,0.000 m (Yao et al., 2012) and forms a topographic barrier to mid-latitude westerlies, causing alterations in atmospheric dynamics with impacts on global scale circulation patterns (Bothe et al., 2011; Wu et al., 2012). In the eastern and central Himalayas and their southern foothills, most precipitation falls from June to September as part of the South Asia Summer Monsoon (SASM). SASM onset in HMA follows an east to west pattern, and its total precipitation amount also decreases from east to west. Condensation of moisture-laden air from the Indian Ocean rising toward the Himalayas results in a precipitation maximum at around 2,000 m elevation. For example, weather stations in Nepal show mean annual precipitation of around 1,400 mm for elevations below 1 km, increasing to above 2,000 mm at an elevation of 1.6-2.4 km and decreasing to under 1,000 mm by 4 km (Krakauer et al., 2013). The north side of the Himalayas is in a rain shadow and has annual precipitation of only around 50–200 mm (International Centre for Integrated Mountain Development, 2005; Riley et al., 2021). Winter storms that propagate from the Atlantic-Mediterranean sector are a significant portion of annual precipitation in the western HMA, and are critical contributors to snowpack at high elevations further east (Lang and Barros, 2004; Riley et al., 2021).
While the general, large-scale patterns in precipitation are fairly well-constrained, the details requisite for understanding precipitation at scales relevant to water resources are less well-defined. The remote and rugged terrain of the HMA region has uneven and sparse station coverage of direct precipitation measurement with stations many mountain valleys and tens or hundreds of kilometers apart and many of these in-situ observation records are too short temporally to robustly assess trends and variability (Yatagai et al., 2012). Ice core (Duan et al., 2002, 2006; He et al., 2003) and tree ring (Brauning, 2006; Schwab et al., 2016; Gaire et al., 2017, 2018) measurements in HMA have been used to fill in some temporal gaps; however, they are still spatially limited. Therefore, spatially continuous gridded precipitation products cannot be well-constrained with ground measurements. Remote sensing products are available but suffer from retrieval uncertainties by as much as 100–140% in individual seasons, along with enhanced biases in retrieval over mountainous and snowy regions (Tian and Peters-Lidard, 2010; Liu and Allan, 2012). As a result, quantifying regional precipitation climatologies and trends in the current climate is challenging and requires careful assessment of measurement biases.
We addressed these challenges by comparing gridded precipitation products with available station observations in the Indus basin and then analyzed these for basinwide precipitation trends (Krakauer et al., 2019). We found evidence for an increasing trend in basinwide precipitation from station records, which was not significant in satellite and reanalysis datasets that covered shorter periods. This work highlighted the potential utility of remote sensing products in regions with sparse station data and showed substantial biases in reanalysis products relative to station observations. Moreover, it was concluded that both satellite and reanalysis data need to be compared against available ground observations to obtain confidence in their reliability, particularly in remote and topographically complex areas.
To evaluate gridded product uncertainty, we developed a dynamic linear model for precipitation that integrates information from existing gridded climate products (Christensen et al., 2019). The integrated precipitation product is modeled as a combination of the four products' probabilistic shared strength and structure, spatially- and temporally-dependent discrepancies between the products, and Gaussian noise. The new product provides a “consensus” precipitation product with objectively quantified uncertainties. We also used this new, integrated precipitation product to assess the discrepancies between four gridded climate products. The results show a significant dry bias in MERRA-2 and slight wet bias in ERA5 (Liu and Margulis, 2019). Remote sensing observations were also used to evaluate reanalysis products. For example, gravity observations from the Gravity Recovery and Climate Experiment/Follow On (GRACE/GRACE-FO) missions, in combination with observations of river discharge, were used to evaluate and validate Precipitation-Evaporation from several reanalysis and climate model products through the closure of the water budget in the upper Indus basin. In another study, we showed that systematic and random land surface model output errors were minimized when forced by precipitation products that incorporate information from ground observations (Yoon et al., 2019).
Snowmelt is a critical component of the water budget in most HMA watersheds (Smith and Bookhagen, 2018), accounting in some cases for the majority of the annual water flow (Bookhagen and Burbank, 2010; Shrestha et al., 2015). Precipitation falling as snow is temporarily stored during colder days/seasons and released during warmer days/seasons as melt, shifting the seasonality of water resources relative to the seasonality of precipitation. Snow is also highly reflective of shortwave radiation and therefore plays a critical role in affecting HMA climate and surface processes (Skiles et al., 2018). Despite its importance in the HMA water budget, regional assessment of seasonal snow is difficult due to snowpack heterogeneity, limited in-situ observations, and challenges in measuring snow-water equivalent (SWE) remotely (Bormann et al., 2018).
Snow cover fraction (SCF) or snow covered area (SCA) refers to the fraction of the earth's surface covered by snow and is one of the primary ways to assess HMA snow distribution. Satellite measurements of SCF began in the 1990s using Landsat TM (Rosenthal and Dozier, 1996). Soon after the launch of MODIS in 2000, these methods were further developed using data from the Airborne Visible/Infrared Imaging Spectrometer (AVIRIS) with a method called spectral mixture analysis (Painter et al., 2003), allowing the estimate of snow grain size in addition to snow cover fraction. Continued algorithm and filter development has enabled determination of SCF in forested areas (Raleigh et al., 2013) and under cloud cover (Rittger et al., 2020) while also accounting for off-nadir satellite view angles (Dozier et al., 2008). We show that SCF from newer sensors like VIIRS are as accurate as MODIS (Rittger et al., 2021) and will provide data well into the future with multiple instruments launched by NASA and NOAA. SCF also plays an important role in understanding land-atmosphere feedbacks as they impact snow-derived water resources. For example, studies show the strength of the Indian monsoon is negatively related to the spring SCF in the Himalaya and positively related to the rate of snowmelt, implying that a smaller SCF and fast snowmelt is conducive for more intense summer monsoon due to land-atmosphere feedbacks (Kripalani et al., 2003; Senan et al., 2016).
SWE refers to the total amount of water stored in a snowpack and is much more difficult than SCF to measure remotely. We explored several approaches for estimating SWE, including atmospheric modeling, offline land surface modeling, and reconstruction/reanalysis. Within the context of these approaches, we discuss the use of remote sensing for validation and assimilation. An essential defining feature of any modeled SWE value is whether precipitation is generated internally by the model (atmospheric modeling) or forced externally by independent precipitation datasets (offline land surface models and reconstruction/reanalysis). In both cases, precipitation (snowfall) uncertainty is generally high, and this propagates directly to SWE estimate uncertainty. To reduce this inherent uncertainty, an additional defining feature in SWE estimation schemes is whether the approach relies on external constraints from remote sensing observations (reconstruction/reanalysis).
In atmospheric models, the SWE is estimated directly from the amount and type of precipitation simulated on the 3D model grid. The models are initialized with the data describing the initial atmospheric state and then driven by chosen boundary conditions. As described in the precipitation section above, we generated a variety of WRF simulations for HMA that include SWE estimates (Sarangi et al., 2020; Riley et al., 2021). Land surface models (LSM's) are often coupled with these atmospheric models to provide surface heat and moisture fluxes at the ground. The LSM model computes snow accumulation, melting, and runoff in a simplified fashion. Land surface models can be improved through assimilation of remotely-sensed snow, ice, freeze/thaw, soil moisture and surface temperature observations in Land Data Assimilation Systems (LDASs). As described in section Glaciers below, LDASs are uncoupled land surface modeling schemes that are forced primarily by observations that started being developed in the late 1990s. We developed a 1-km HMA-LDAS reanalysis using the NOAH-MP, forced by physically downscaled surface meteorology, parameterized by remotely sensed topography and vegetation, and constrained by remotely sensed snow, Freeze/Thaw, temperature, and glacier observations (Xue et al., 2019). The HMA-LDAS surface atmospheric forcing dataset is based on a downscaled numerical weather forecast analysis/reanalysis first guess, integrated with satellite observed precipitation, radiation, and temperature. In an LDAS, the model-generated states are corrected toward the observational estimate. For the HMA snow, freeze/thaw, and glaciers are the most critical states for assimilation into the HMA-LDAS. Therefore, the HMA-LDAS snow fields (snow depth, density, and water equivalent) were physically modeled reactions to atmospheric forcings and its errors, constrained by MODIS snow cover fraction observations, microwave snow observations, and freeze/thaw observations. HMA-LDAS uses both a machine-learning technique to assimilate satellite microwave data and a rule-based updating scheme to assimilate snow cover, freeze/thaw, and glacier extent observations (Ahmad et al., 2019; Kwon et al., 2019). These fields were evaluated using the few snow observations available over the HiMAT region and show significant improvement with respect to the open-loop model. We applied the snow reanalysis approach over the joint Landsat-MODIS era by generating an ensemble of forward model realizations driven by MERRA-2 forcing inputs and constrained by Landsat- and MODIS-derived fSCA. The newly generated HiMAT dataset (Liu et al., 2021) provides posterior estimates of SWE (and other snow variables) over 2000–2017, which can be used to assess seasonal snow storage over HMA and verify or constrain other HiMAT-generated or other data products.
Historical snow reanalysis approaches, like those above, provide an estimate of SWE by combining observations (both satellite and ground-based) with numerical (a priori) snow model estimates through the process of data assimilation. Alternatively, snow reconstruction approaches (Bair et al., 2016; Rittger et al., 2016) combine remote sensing observations with snow energy balance models applied backward in time from melt-out to peak SWE forced by reanalysis data. An advantage of this method is that it does not rely on uncertain estimates of precipitation and does not require in-situ observations. Both retrospective methods take advantage of information in the depletion time series of remotely sensed fractional snow covered area (fSCA) as a constraint on estimating SWE. The snow reconstruction approach also uses remotely sensed snow albedo, which is more accurate than snow aging schemes typically used in snow models (Bair et al., 2019). Our reconstruction method for HMA did not use precipitation inputs but relied solely on an energy balance model (ParBal) and remotely-sensed fSCA and albedo measurements to work backward from melt-out to peak SWE. Reconstructed melt is constrained to be ≥0, so large storms after peak SWE will cause an overestimate of peak SWE. The energy balance model requires (non-precipitation) forcings, including CERES estimates of incoming shortwave and longwave fluxes and near-surface air temperature, humidity, and winds from GLDAS.
The major uncertainty associated with snowmelt in current large-scale models is the parameterization of snow albedo, and consequently, the amount of solar radiation being absorbed and the rate of melt. Snow albedo parameterizations, such as those used in GFDL AM4/LM4 (Zhao et al., 2018) or ECMWF (Beljaars et al., 2018), are often oversimplified and neglect the influence of snow microstructure, the presence of Light Absorbing Particles (LAPs), such as black carbon, mineral dust, and microbial growth, and their mixing state. Most models also generalize impurity type, leading to a degree of uncertainty dependent on specific optical absorption properties of the snow and ice surface impurities (Casey et al., 2017). Even for albedo parameterizations that accounted for specific LAPs, there are still uncertainties related to quantifying the optical properties of the LAPs, especially for microbial and organic compounds. The assumption of a clean (impurity-free) snowpack with spherical grains can lead to an overestimation of surface albedo by more than 50% (He et al., 2018). The melt rate is 5 times faster if the snow albedo is reduced from 0.9 to 0.5, given the incoming radiative fluxes and turbulent fluxes and snow density. The errors due to LAPs can be significant, especially across HMA, which is surrounded by urban pollution sources and affected by dust transported from both local and distant deserts. For example, a recent study found a 30% reduction in LAP affected the timing of 6.6 km3 of runoff from the Indus River basin (Bair et al., 2021).
We used multiple satellite products and coupled atmosphere-chemistry-snow models to demonstrate a robust physical association between the elevated dust layer in the atmosphere and dust-induced snow darkening (Sarangi et al., 2020). Our results revealed a signature of increasing dust-induced snow darkening effect with elevation over HMA that peaks around 4,500 m. The dust-induced snow darkening effect is greater than that of black carbon above 4,000 m, suggesting a discernable role of dust in observed spatial heterogeneity of snowmelt and snowline trends over HMA and an increasing contribution of dust to snowmelt as the snowline rises with warming. Advances in remote sensing of snow properties may provide important constraints and calibration data to improve snowmelt models. We have utilized satellite observations of snow properties (Painter et al., 2009, 2012; Bair et al., 2019; Sarangi et al., 2020) to validate global to regional scale models to better understand regional dust and carbon impacts on snowmelt (Painter et al., 2010; Sarangi et al., 2019). We have also used satellite radar to map diurnal cycles in wet snow, which can be leveraged for model calibration and validation (Lund et al., 2020). Snow properties have also been used to partition the snow melt from the glacier melt across large river basins of High Mountain Asia (Armstrong et al., 2018).
Watersheds containing glaciers contribute more runoff to streamflow than similarly sized, non-glacierized basins (O'Neel et al., 2014). Glacier runoff includes meltwater derived both from seasonal and annual mass balance contributions (Cogley et al., 2011), underscoring the unique capacity of glaciers to contribute water derived from long-term storage. Mass balance variations drive changes in glacier area (primarily through terminus advance or retreat), surface elevation and ice dynamics. Therefore, an integrated assessment of glacier response to climate ideally includes direct mass balance observations coupled with inventories of ice area, volume, and surface velocity. The HMA region contains the largest concentration of glaciers outside the polar regions (Pfeffer et al., 2014). HMA glaciers are located in rugged and tectonically active terrain that deposit debris on about 11% of glacier surfaces (Kraaijenbrink et al., 2017), complicating efforts to assess surface energy balance (Scherler et al., 2018). HMA glaciers also span steep climatological gradients both spatially and with elevation, creating unique challenges for monitoring and modeling.
Direct field observations of HMA glacier mass balances provide useful information for specific watersheds (Yao et al., 2012; Wagnon et al., 2013) but are limited due to complex terrain and severe weather (Racoviteanu et al., 2014). Remote sensing data provide a more comprehensive picture of the regional mass balance (Bolch et al., 2012; Azam et al., 2018). For example, the Global Land Ice Measurement from Space (GLIMS) and the Randolph Glacier Inventory (Pfeffer et al., 2014; RGI Consortium, 2017) significantly improved maps of HMA glacier surface area via digitization of 15 to 30 m optical multispectral satellite images from ASTER (Advanced space-borne thermal emission and reflection radiometer) and Landsat. We contributed to extending the HMA mapping inventory back to the 1960s and 1970s, using declassified Corona (0.6–1.2 m) and Hexagon (6–9 m) imagery (Maurer et al., 2019), placing recent glacier changes into historical context (Dehecq et al., 2019).
In addition to providing information on glacier extent, remote sensing offers new tools for measuring glacier mass and volume change. Stereo optical imagery has been used to generate Digital Elevation Models (DEMs), which, over time, can provide measurements of changes in volume. We used this approach to generate DEMs from declassified Hexagon and from ASTER satellite imagery to show that the rate of Himalaya glacier mass loss has roughly doubled between 1975–2000 and 2000–2016 (Maurer et al., 2019). In another study, we combined DEMs from very high-resolution commercial imagery (WorldView-1/2/3 and GeoEye-1) medium-resolution ASTER stereo imagery to calculate a mass balance estimate of −0.19 ± 0.03 m w.e. yr−1 for 99% of HMA glaciers between 2000 and 2018 (Shean et al., 2020). This compared well with earlier estimates from Brun et al. (2017), who used ASTER optical satellite stereo pairs to estimate the mass balance of HMA glaciers to be −0.18 ± 0.04 m w.e. yr−1, during 2000–2016.
Data from the GRACE can also provide estimates of mountain glacier mass balance at the regional scale. We used GRACE/GRACE-FO observations to evaluate the mass balance of all HMA glaciers, and four major subregions for the period 2002–2019 (Ciracı̀ et al., 2020). We used an ad-hoc solution to extract the signal of the glaciers to estimate the mass loss. We calculated an HMA mass balance of −28.8 ± 12 Gt yr−1. during 2002–2019. Most of the glacier mass loss was from the Tien Shan, Himalaya, South and East Tibet, and Hengduan Shan. Some of the acceleration in mass loss was driven by a large interannual variability in precipitation which reflects an influx of solid precipitation from the Mediterranean Sea during the winter season (Yao et al., 2012). While GRACE is limited in spatial resolution, given proper corrections, it provides estimates of mass change that are useful for evaluating surface mass balance estimates from re-analysis and regional atmospheric climate models.
Both mass balance and flow speeds of glaciers are significantly influenced by the presence of debris cover. Many glaciers in the HMA are heavily debris-covered, mostly in their lower parts (Rounce et al., 2021). In principle, the presence of a thick debris layer reduces the melt of the ice beneath it due to insulating effect, but thinner debris enhances melt due to lower albedo. Despite the significant influence of debris on glacier surface mass balance, HMA debris-covered glaciers have been losing mass at rates similar to debris-free glaciers (Kääb et al., 2012; Maurer et al., 2019; Buri et al., 2021). Ablation of ice cliffs, sub-aqueous melt of supraglacial ponds, and internal ablation due to englacial hydrology can substantially enhance mass losses in debris-covered glaciers and may explain the similarity in mass balance between debris-covered and debris-free glaciers. However, we showed that the primary cause of the similar thinning rates on debris-covered and debris-free glaciers can be attributed to differences in ice dynamics (Rounce et al., 2021). At the same time, we showed reduced surface melting on debris may allow glaciers to flow to lower elevations and build larger ablation areas, resulting in a more negative mass budget relative to clean glaciers (Bhushan et al., 2018).
The hydrograph of glacier runoff is often similar to that for snow surfaces surrounding a glacier in the earlier ablation season, making it difficult to partition water from these two sources. To explore this, we compared snow and glacier melt estimated using the distributed Glacio-hydrological Degree-day Model (GDM) compared with remotely sensed Advanced Scatterometer (ASCAT) data and found much similarity in areas of snow and ice melt (Kayastha et al., 2020). That was the first step in examining how remote sensing products could potentially be incorporated into hydrologic models to increase the accuracy of the hydrologic flow as well as the ability to estimate river discharge in other basins with limited data. However, exact estimation of snow and glacier melt amount using the ASCAT data remains a challenge.
Groundwater is a component of the water balance in many glacier- and snow-dominated basins (Grah and Beaulieu, 2013) and can comprise a sizeable portion of the runoff budget in glaciated catchments (Gan et al., 2015). Contributions to river flow in several basins in Nepal were delayed by ~45 days as a result of flows through fractured aquifers (Andermann et al., 2012), and subsurface storage of glacier meltwater was found to be important to river flows in the Langtang basin (Wilson et al., 2016). In southwest China, Zeng et al. (2015) used a modeling approach to estimate glacier meltwater flows through karst terrain and Meng et al. (2015) found groundwater contained 35% of water derived from ice and snow in a region on the eastern edge of the Tibetan Plateau.
The many varied aquifer systems in the HMA are a major source of freshwater for agricultural activities, and it is likely that many such systems are overstressed under current rates of usage (Rodell et al., 2009, 2018; Aeschbach-Hertig and Gleeson, 2012; Wada et al., 2012; Richey et al., 2015). To date, existing groundwater assessments either rely on coarse resolution satellite gravimetry observations at a regional scale (Rodell et al., 2009, 2018) due to a lack of well-observations or focus on small regional scales that have access to observations at specific locations (Zaveri et al., 2016; Iqbal et al., 2017). Joshi et al. (2021) provided a recent and thorough comparison between groundwater level changes, abstraction rates, aquifer sedimentology and rainfall in the Northwestern Indian states of Punjab and Haryana, known for severe groundwater depletion (Rodell et al., 2009, 2018). They found high levels of heterogeneity in groundwater trends in both space and time that were mainly, but not always, explained by trends in abstraction, bore well-density and geologic structure. The heterogeneity was often at a scale too fine to be captured by coarser GRACE trends, but at a scale relevant to management decisions. These findings support the need for further development of groundwater modeling tools at high spatial resolution.
We assembled 2003 to 2016 depth-to-water measurements from thousands of wells for some regions of India and Pakistan and combined these with estimated storage coefficients to assess groundwater storage anomalies. As shown in Section Snow, we compared trends in total water storage (TWS) from GRACE to these groundwater observations (Loomis et al., 2019). We found that the modeled TWS trends could not fully explain the trends from GRACE and attribute this to the limited representation of groundwater dynamics in the models used, along with a lack of water management practices such as irrigation.
Lakes act as a buffer in the HMA hydrological cycle, providing a mechanism for water storage at a variety of time scales. When lakes are dammed either naturally or through human intervention, there can be numerous downstream impacts ranging from water shortages to outburst floods. We produced a global lake inventory (Shugar et al., 2020) and HMA specific inventory (Chen et al., 2021) using semi-automated techniques based on Google Earth Engine and Landsat imagery. Some variations exist between different inventories, primarily due to how these lakes are classified (connected vs. unconnected; proglacial vs. supraglacial vs. marginal; moraine-dammed vs. ice-dammed) and the sizes of lakes and the periods that were covered. Nonetheless, these databases reveal a complex pattern of lakes growing in some parts of the HMA while either shrinking or not developing rapidly in other regions. In addition, we provided glacier mass balance data (Shean et al., 2020) to a study that examined lakes on the Tibetan plateau, most of which have undergone an increase in area and volume from the 1990s to present (Qiao et al., 2019). These results support the findings of Zhang et al. (2019) showing an overall expansion of lake area over the Tibetan Plateau during 1970 to 2018. A comparison of lake volume change (Treichler et al., 2019) and these glacier mass balance data revealed that glacier mass loss had a relatively limited contribution to lake mass increase (Brun et al., 2020). The study suggests that the remaining lake mass loss could be attributed to evaporation and/or changes in seepage to deep groundwater.
Although severe degradation of permafrost is expected for HMA regions where it currently exists (Sun et al., 2019), the impacts of such degradation on downstream hydrology is poorly quantified (Sun et al., 2020). Potential impacts of permafrost degradation in response to climate warming include alterations to the surface energy budget due to changes in freeze-thaw cycles, alterations to soil moisture profiles, and a dropping of the groundwater table (Cheng and Wu, 2007). The growth of thermokarst lakes in HMA permafrost areas are also of concern given their impact on ecosystems, hydrology and emission of greenhouse gasses (Pandey et al., 2020, Gao et al., 2021). We did not incorporate permafrost into any of our analyses; below, we recommend the importance of considering permafrost in future assessments.
River flow at any location in a watershed ultimately depends on the total water budget calculated as the sum of individual components listed above. A complicating factor is that water generated from a source such as snow or glacier melt may then travel through various downstream systems where it may enter or leave storage, for example, in lakes or groundwater. Therefore, consideration of landscape characteristics, terrain, and human activity is necessary to predict the ultimate fate of water generated from high elevation watersheds. Total water budget calculations must also account for variations in evapotranspiration, which is a significant component of the budget in highly vegetated regions of HMA.
Land surface models are used to integrate individual components of the water budget and assess the total water budget in HMA as it varies in space and time. The spatial resolution of current land surface models has largely been dictated by the spatial resolutions of global climate (~100 km) and weather-forecast (~20 km) models from which they were derived. However, much higher resolutions are necessary for estimating the storage, movement, and quality of terrestrial carbon, energy, and water (Wood et al., 2011).
In this section, we describe downscaling approaches to generate spatially and temporally continuous forcing data to drive hydrology model simulations at resolutions needed to inform water resource planning. We highlight the utility of satellite gravimetric observations in measuring large scale temporal variations in total water storage. Finally, we summarize the latest generation of land surface models and their progress toward assimilating remote sensing and ground observations to estimate river discharge.
Land surface models typically require forcing variables of air temperature and humidity, wind speed and direction, incident longwave and shortwave radiation, and precipitation. Dynamical and statistical climate downscaling approaches can be used to provide high-resolution fields of these variables from coarser-resolution data. We used a random forest algorithm to statistically downscale MERRA-2 (Modern-Era Retrospective analysis for Research and Applications, version 2) precipitation products (form an original resolution of 0.5° 0.625° to 1 × 1 km) for HMA (Mei et al., 2020). This approach correlated atmospheric variables with landscape information such as topography, surface roughness, and vegetation, following Rouf et al. (2020). The downscaled precipitation had higher correlation coefficients and Nash-Sutcliffe indices with respect to the reference ground-based dataset than the original coarse resolution MERRA-2, as well as similar spatial patterns to those of several satellite precipitation products. We also demonstrated the superiority of high-resolution atmospheric variables when forcing a land surface model across complex terrain in HMA (Xue et al., 2021). Specifically, the downscaled forcing dataset yields the highest skill in the model output states (including runoff and snow depth), improving the model by 7% compared to the corresponding coarse-resolution simulations.
Dynamical downscaling is often computationally more intensive than statistical downscaling but is physically based, and thus provides additional information on the processes driving spatial and temporal patterns in climate variables. We used WRF to dynamically downscale climate over HMA for 2001–2015 in three nested domains: 36, 12, and 4 km (Dars et al., 2021). Similar to Mei et al. (2020) and Xue et al. (2021), we showed significant improvement in precipitation and temperature accuracy as model resolution increased. In addition, we used the 4 km WRF domain from Dars et al. (2021) to assess the characteristics of historical HMA precipitation (Riley et al., 2021). Importantly, our results show differences in amount, frequency, and seasonality of precipitation between valleys and the surrounding higher elevations. Thus, precipitation observations from lower elevations often do not capture the precipitation statistics of surrounding terrain, which can impact statistical downscaling that relies heavily on often biased distributions of in-situ data.
Dynamical downscaling can also directly inform statistical downscaling efforts by highlighting those processes that statistical approaches need to account for. In addition, dynamical downscaling can provide estimates for variables with limited or no observations. For example, the relative lack of information on LAP concentrations in HMA atmosphere, snow, and ice motivated our use of WRF-Chem to downscale the distribution of LAP concentrations in snow and their radiative effect across HMA (Sarangi et al., 2019). Because of a more realistic representation of terrain slopes over HMA, the higher-resolution WRF-Chem shows obviously better performance in simulating snow cover, snow albedo and snow grain size over HMA, as well as improvements in aerosol loading in the atmosphere and LAP concentration in snow. Himalayan snowpack has a high magnitude of LAP-induced snow albedo reduction (4 to 8%) during pre-monsoon seasons, which induces a snow-mediated radiative forcing of ~30–50 W m−2 (Sarangi et al., 2019). Coarse spatial resolution and absence of snow–aerosol interactions in modeling the Himalayan cryosphere will result in significant underestimation of LAP effects on snowmelt and regional hydroclimate.
Both dynamical and statistical downscaling have benefits and drawbacks. Leveraging both over the complex terrain of HMA will provide the high resolution variables with their associated uncertainties needed for driving land surface models and objectively interpreting the results.
GRACE results were discussed above in the context of evaluating glacier and precipitation changes. Because GRACE measures all variations in earth mass, it is also an ideal tool for estimating variations in the total water budget of HMA. Comparing GRACE observations with other water budget data can be challenging in HMA due to tectonic activity and the spatial heterogeneity of hydrological changes (Immerzeel and Bierkens, 2012). We used GRACE data to validate the accuracy of a series of land surface models and found large differences over watersheds with glaciers and significant anthropogenic groundwater abstraction, both water budget components not accounted for in the model (Yoon et al., 2019). In another study, we focused only on the glaciated watersheds of HMA and calculated the trends in mass from GRACE (Loomis et al., 2019). By forward modeling trends in glacier mass from Shean et al. (2020), we were able to close the water budget over high elevation glacier regions; however, existing groundwater data in the upper Indus basin did not account for all variations in mass due to groundwater depletion. This points toward the need for expanded ground observations of groundwater change to better understand these GRACE estimated mass variations.
Land surface models simulate energy and water exchanges at the earth's surface and provide a mechanism for exploring the sensitivity of the HMA water budget to climate variability. Model outputs include estimates of each component of the water budget, including runoff from precipitation, snow melt, and glacier melt, as well as groundwater storage and evapotranspiration fluxes. These integrated assessments also make extensive use of remote sensing products for validation, in particular data from the GRACE satellites. We summarize the results from three land surface models, including the Water Balance Model, NASA Land Information System Model, and Glacio-hydrological Degree-day Model.
The Water Balance Model (WBM), developed by University of New Hampshire, simulates both the vertical water exchange between the atmosphere and land surface and horizontal water transport, through both land surface runoff and via the river network (Grogan et al., 2015). Both natural and human processes, such as evapotranspiration, snowpack development and melt, glacier melt, river flow, and river impoundments from dams and diversions, are included in the model. Snowpack is based on a sub-grid approach using elevation bands (Lammers et al., 1997; Hartman et al., 1999) of 200 m to account for this region's strong vertical temperature gradients. Mishra et al. (2020) used the WBM at 0.8 km2 resolution for the Hunza and Trishuli basins in the Karakoram and central Himalaya.
The NASA Land Information System [LIS; Kumar et al. (2006)] is an interoperable land surface modeling and data assimilation framework that allows the integration of land and hydrology models, remote sensing data, and methods to combine their strengths. We employed the ensemble modeling and data assimilation software capabilities in LIS over the HMA domain, and showed that that large uncertainty exists in the simulated water budget, contributed by uncertainties in the meteorology, influence of anthropogenic processes, and limitations of the models (Yoon et al., 2019). The studies with LIS also show that assimilation of remote sensing data was useful in this data poor region. For example, assimilation of microwave brightness temperature measurements was able to provide improvements in snow simulation for snowpacks <200 mm. The use of snow cover data was only to provide marginal improvements in snow estimation in this area with large seasonal snowpacks. Assimilation datasets of vegetation changes from optical sensors and gravimetry-based terrestrial water storage changes were able to simulate the impacts of management impacts (agriculture, groundwater pumping). Though these approaches are promising, more reliable observations and multivariate data assimilation approaches that provide a simultaneous constraint on the water cycle are needed in this region.
The Glacio-hydrological Degree-day Model (GDM) simulates basin discharge as a combination of water from snowmelt, icemelt including melt under a debris layer, rainfall, and baseflow on a daily time scale (Kayastha and Kayastha, 2020; Kayastha et al., 2020). It is a distributed and gridded model which needs daily temperature and precipitation, degree-day factors for snow and icemelt, land use, land cover, and DEM data. We applied the GDM to three river basins in HMA and illustrated approaches for combining remote sensing products with hydrological flow models to improve the accuracy of river discharge predictions (Kayastha et al., 2020). We also combined GDM with a global glacier model to project the response of several HMA hydrological systems to future climate variability (Mishra et al., 2020). Our simulations showed that glacier runoff increased during the monsoon season and decreased during the post-monsoon season due to future changes in precipitation and melting.
General Circulation Models (GCMs) are used to generate ensemble estimates of future climate conditions that are used to force cryospheric and hydrological models. The resulting projections of future HMA water budgets are complicated by uncertainties in climate forcing and land use practices. For example, increases in agricultural activity and groundwater abstraction increase evapotranspiration with impacts on atmospheric moisture and precipitation rates (de Kok et al., 2020). Therefore, models should link atmospheric, land surface, and anthropogenic activities to fully account for future conditions. Models also need to account for the complex response of glaciers to climate change via changes in glacier surface area and debris cover.
Existing GCM simulations for 1861–2100 show long-term increases in annual mean temperature and total precipitation across the HMA during the Twenty-first century (Huang et al., 2014; Lutz et al., 2014), but with a decrease in the snow/rain fraction in the central and southeast Himalaya due to atmospheric warming (Kapnick et al., 2014). Downscaled GCM ensemble runs for RCP4.5 and RCP8.5 predict strong warming and likely precipitation increases for parts of the Upper Indus Basin through the end of the Twenty-first century, although both temperature and precipitation projections had high variability and uncertainty (Lutz et al., 2016). There is evidence that any warming in the HMA region will be amplified with elevation due to surface-based feedbacks (Pepin et al., 2015). Most models show an increase in summer precipitation over the Himalayas for this century, particularly for the central and eastern portions of HMA, while there is more disagreement between models on winter precipitation, with most simulating a small decrease (Palazzi et al., 2015). A lowering of aerosol concentrations due to reductions in biomass and coal burning in South Asia would have a positive impact on precipitation (Chuwah et al., 2015). Using ensemble GCM data to force a hydrological model, Lutz et al. (2014) predicted an increase in runoff through 2050 caused primarily by an increase in precipitation in the upper Ganges, Brahmaputra, and Salween and Mekong basins.
We used a 50 km horizontal resolution NOAA GFDL GCM (Vecchi et al., 2014) to explore changes in extreme precipitation in HMA (Kirschbaum et al., 2020). By comparing precipitation from historical sea surface temperature constrained simulations with observed precipitation from the Tropical Rainfall Measuring Mission (TRMM) Multisatellite Precipitation Analysis (TMPA) satellite mission, we were able to better validate the GCM's ability to reproduce historic precipitation extremes and test the ability of the model to reproduce landslide risk using a landslide hazard model (Kirschbaum et al., 2020). This allowed us to estimate the effect of future precipitation extreme events on landslide risk. Simulations showed increases in the likelihood of extreme precipitation events, and we illustrated the capacity for using GCM simulations to produce landslide hazard estimates to inform decision-making.
We made several advances in simulating the response of glaciers and the resulting changes to glacier runoff under future climate conditions. We developed the open source Python Glacier Evolution Model (PyGEM) that calculates glacier ablation using positive degree-days, accumulation with a temperature threshold, refreezing based on annual air temperature, and redistributes mass based on an empirical estimate of glacier dynamics. The primary advance relative to past efforts was the development of a new calibration technique using Bayesian inference to leverage the geodetic mass balance data from Shean et al. (2020) and Rounce et al. (2020b). This enabled us to quantify uncertainty associated with the model parameters and project glacier changes for an ensemble of RCPs (2.6, 4.5, 8.5) through 2100. Our simulations predict considerable spatial and temporal variability in glacier runoff projections. Specifically, most monsoon-fed river basins will hit peak water before 2050, while other major watersheds fed by the westerlies will hit peak water after 2050. Changes in glacier runoff will be the most significant at the end of the ablation season, which could have important impacts on water resources.
In an effort to link future climate changes to local conditions, we used an ensemble of GCM data to force hydrological model simulations of two high elevation drainage basins, the Hunza River basin at Naltar in the Karakorum, Pakistan and the Trishuli River basin at Trishuli in the Himalaya, Nepal (Mishra et al., 2020). We integrated the glacier runoff projections from PyGEM (Rounce et al., 2020a) with a hydrological model and coupled this with a hydropower model to assess the impacts of future climate variability on hydroelectricity generation. While inter-model annual and summer variability in river discharge was high, especially after 2050, we found the impact on hydroelectricity generation was higher at Karakorum site than at the Himalaya site. The model predicted a reduction in glacier meltwater by 40% and 60% for the Trishuli and Naltar sites, respectively. Earlier spring melt and later accumulation of the snowpack reduced the length of the winter low-flow period, and this had a more pronounced signal at Naltar and was accompanied by a reduced summer river flow at both sites.
Despite recent advances in statistical methods and computational power enabling high resolution modeling, HMA remains a very data-poor region, and gaps remain in our understanding of many fundamental physical processes. In this section, we provide an overview of challenges currently inhibiting further progress in quantifying hydrological variations in HMA and offer recommendations for ways to address these challenges.
Acquiring accurate, high resolution estimates of HMA precipitation is crucial because precipitation forces land surface models and is required to estimate streamflow contributions and snowfall rates. Our intercomparison efforts provided new information on uncertainty in precipitation fields, increasing our understanding of which products are best for specific applications and highlighting regions/conditions where precipitation uncertainty is large. Our downscaling efforts further improved our understanding of the complex interactions between terrain and precipitation, and emphasized the importance of high resolution products for estimating precipitation in complex terrain. However, direct observations are still needed as a way to eliminate systematic errors that may span multiple existing datasets and for validating models. The lack of high elevation precipitation observations remains a significant barrier to progress in improving reanalysis and model products. Data from satellite missions such as NASA's Global Precipitation Measurement platform can fill this data gap and will be a critical resource for assessing which gridded product and model parameters best represent reality. Any new satellite precipitation product, however, will need to be fully calibrated and validated, especially over complex topography. Thus, accurate satellite precipitation products still require in-situ precipitation observations. Given the challenges of funding, installing, and maintaining accurate networks of in-situ precipitation observations in the complex and high elevation terrain of HMA, one challenge moving forward will be to develop approaches, potentially including remotely sensed, that can leverage all direct (e.g., rain gauge data) and indirect (e.g., snow-covered area, stream gauge data) observations of precipitation to develop, improve and evaluate model, reanalysis, and satellite precipitation products.
We have developed new approaches for estimating SWE at relatively high spatial and temporal resolution via novel reanalysis and reconstruction methods. A core challenge of our existing methods is that they rely heavily on remote satellite observations, not all of which are directly related to SWE. For example, fSCA only provides information on the presence or absence of snow cover (or snow fraction) but provides no information on total snow volume. Recent work combining daily observations at 500 m scale with less frequent observations from higher resolution satellites like Landsat 8 (Rittger et al., 2021) allows improved estimates of snow surface properties, but still do not estimate snow volumes. One way to increase confidence in SWE derived from reanalysis is to compare it with concurrent climate model estimates (Bair et al., 2020). Discrepancies in these two approaches can then be explored using climate model sensitivity analysis to pinpoint sources of uncertainty, for example, due to cloud microphysics, model dynamics in complex terrain, and overall model resolution. Ground observations of SWE, especially at high elevations (Kirkham et al., 2019), are also needed to provide vital calibration data.
Direct observations of SWE from satellites remain a central challenge of global snow hydrology studies. Current SWE estimates from passive microwave sensors often fail due to their coarse resolution, signal saturation for deep snow, and the loss of information for wet snow. SWE estimates derived from height changes (e.g., LiDAR) can be improved through enhanced methods for estimating the temporal and spatial variability of snow density. A dedicated satellite mission for measuring SWE would greatly improve our capacity for quantifying snow variability in the HMA region and is a designated observable recommended by the 2018 Decadal Survey (National Academies of Sciences, 2018).
Another critical challenge to assessing the role of snow in HMA hydrology is quantifying the effects of LAPs on snow melting. While many advances have been made, many uncertainties and challenges still need to be addressed (Qian et al., 2015; Kang et al., 2020). For example, accurate estimates of radiative fluxes and climate require accurate representation of LAP deposition rates, snow/ice accumulation, snow aging processes, and the meltwater scavenging efficiency of LAP (Qian et al., 2014, 2015). All of these are difficult to constrain given the scarce observations and large variation in representing these processes in models. The LAP data paucity and model uncertainties also make it difficult to determine the relative contributions of LAP vs. climate change to the loss of snowpack (Qian et al., 2011). Limited in-situ and remote sensing LAP data has also led to a significant gap in model-observation comparisons. This is compounded by the complexities inherent in model-data comparisons, such as short-term measurement vs. long-term climatology in model and in-situ point measurement vs. model grid-cell mean. Finally, to accurately quantify the LAPs effect, it is critical for models to have a very high spatial resolution in order to resolve the topography-oriented snowpack and LAPs deposition over the complex terrain of HMA. However, very high resolution simulations are often hampered by computational resource constraints. Targeted in-situ and remote sensing observations of LAP in snowpack will be a critical step toward understanding snow hydrology and SWE variations, and our ability to accurately model snow variations.
While there have been significant advances in quantifying glacier mass balance in HMA, further refinements in observations and modeling of glaciers will help reduce uncertainty in assessing past, present, and future glacier mass balance and contributions to downstream hydrology. For example, glacier area estimates using optical sensors are often limited due to extensive cloud coverage and coarse resolution. Similarly, uncertainties in coarse resolution surface elevation data arise primarily from orientation errors, difficulty identifying surface properties, uneven climatic conditions, poor contrast in snow covered and shadow areas, and the inaccurate identification of corresponding features in the stereo models (Paul et al., 2016). We expect that the spectral, spatial and temporal data availability from satellite remote sensing will continue to improve in the coming years and decades, improving our ability to map and measure volume/mass change of all glaciers covering HMA, including debris-covered glaciers and small glaciers (<1 km2). These datasets will continue to provide unique opportunities to advance model calibration and how glacier evolution models account for important physical processes like glacier dynamics, surface feedback processes, and debris cover, which will greatly improve projections of glacier mass change and glacier runoff (Olson and Rupper, 2019; Johnson and Rupper, 2020; Rounce et al., 2021). Overall, the challenges include identifying and using remote sensing and reanalysis data, as well as models, to more accurately quantify glacier mass balance and radiative forcing changes over time. This includes estimating the evolution of glacier geometry and area in response to changes in climate, which requires models that couple glacier ice flow dynamics to glacier mass balance. Such models are under development, and will require validation of ice dynamic paramaterizations to provide accurate estimates of future glacier conditions (Maussion et al., 2019, Eis et al., 2021).
Our findings support the need for improved representation of groundwater, including explicit unconfined and confined groundwater layers, as well as water management in models. While in alpine drainage basins it is expected that snow and ice meltwater inputs to groundwater will be high (Vincent et al., 2019), the challenge is to understand how groundwater changes in the downstream regions of the larger basins, i.e., those that rely on irrigation for human food supply, are affected by cryospheric changes. Increased access to existing well data would provide much needed ground validation data to validate and calibrate existing models.
An important next step in improving the ability of models to accurately predict total basin runoff is to increase their capacity to resolve sub-grid scale processes. These processes include orographic precipitation and terrain impacts on melt that often dictate hydrological variability on scales of hundreds of meters or less. GCMs have historically not included sub-grid scale mountain hydroclimate processes, and the field is currently moving toward improved inclusion of smaller scale processes within coarser global models, but there is still significant progress needed (Chaney et al., 2016). With increased high performance computing, future global climate models may approach km-to-10 km scale resolutions in the coming decade, which will address the challenges posed by a lack of high resolution forcing data. Advancements in dynamical and statistical downscaling will further advance our ability to downscale GCMs to resolutions requisite for hydrological modeling.
Additional improvements to basin hydrology estimates will be achieved by accounting for all components of the hydrological budget. These should include permafrost and its impact on groundwater flow and the role of lakes in modulating runoff from high alpine regions. It will also be important to fully account for anthropogenic forcing on groundwater abstraction rates. These integrated assessments should include more complete uncertainty assessments, especially for those approaches that assimilate a wide variety of complex datasets.
Better precipitation data and scenarios allow for advance planning of potential increases in the rate at which water enters the region. An increase in total precipitation will be in the context of decreasing snowfall and increasing rainfall, particularly in central and eastern HMA (Kapnick et al., 2014). This will pose new challenges for water management, removing snowpack as a natural reservoir for precipitation (and glacier recharge) and altering the timing of liquid water entering the rivers. Future work should focus on providing estimates of changes in timing and type (snow vs. rain) of precipitation, evapotranspiration, as well as total water amount, for water resource planning.
Water, food, and energy security are inextricably linked to each other and warrant an integrated assessment approach to understand the effect of actions in one part of the nexus on the others. As such, integration of economic activities and infrastructure into coupled climate-hydrology models is necessary to generate information that supports building resilience to climate change (Mishra et al., 2018). Vulnerability of sub-basins to climate-led changes in water availability and quality vary depending upon their endowments of crops and cropland area, land use type, livestock population, infrastructure (e.g., hydropower facilities), and human populations. Crop characteristics and agricultural practices vary across sub-basins depending on the agroecological region, irrigation facilities, and status of agricultural commercialization that determine the water use intensity of crops and efficiency. Similarly, the types and number of existing and pipeline hydropower facilities vary across the sub-basins. Given that the magnitude of impacts depends upon the unmet water demand for food, electricity, and biodiversity, an improved understanding of the water-food-energy nexus at the sub-basin scale is necessary to assess potential impacts of climate change on each sector. Such sub-basin scale analyses and results can be integrated/aggregated to regional and national scale to generate information that supports policies to foster water-food-energy security.
We developed an integrated assessment framework that used economic models and biophysical models, including the positive degree-day model, hydrologic model, run-of-river power system model, and fishery suitability index (Mishra et al., 2018). The framework was used for gaining insights into the impacts of changed river flow on hydropower and fisheries of the Trishuli River in the HMA region. The Trishuli River is a transboundary river that originates in the Tibet Autonomous Region of China (at Kirong Tsangpo), flows into Nepal as Trishuli and Narayani Rivers, and into the Ganges River in India. In the Trishuli basin, the projected increase in river flow in the months of March and April due to increased snowmelt translated to a modest increase (by ~4 GWh) in electricity generation as compared to baseline. However, the increased flow during June and July when the glacier melt is coupled with monsoon precipitation could not be used to increase additional hydropower due to the plant's capacity constraint. The study also developed a framework for assessing intersectoral water allocation between hydropower and fisheries. Under both RCP 4.5 and RCP 8.5 scenarios, fishery suitability was projected to be >70%. The framework was applied for the run-of-the river type hydropower plant in Trishuli and does not represent the fishery suitability downstream of storage type power plant.
We used the same framework for comparing the impacts of climate change on two HMA river basins–Trishuli and Hunza Basins from Central Himalaya and Karakoram, respectively (Mishra et al., 2020). The study compared differences in estimated river flow over historic and future time using the WBM in sixteen scenarios (eight climate models and two emissions scenarios) for rivers upstream of hydropower plants in each sub-basin. The framework was used to assess the projected changes in hydropower generation at the Naltar IV hydropower plant, with an 18 MW capacity, located in Hunza, Karakoram, and the Trishuli hydropower plant, with a 19.6 MW capacity, in Trishuli, Central Himalaya. The study found that the estimated impact of climate change and temporal variability were higher for the Naltar plant than for the Trishuli plant.
A primary challenge is that of integrating vulnerable cropland and the vulnerable sub-basins at high resolutions necessary to generate information that supports interventions to maintain food and energy security in the HMA region under a changing climate. Similarly, linking and integrating a number of models necessary for the quantification of climate impacts on food and energy nexus is challenging. Another challenge is associated with lack of data and a poor understanding of the spatio-temporal variability in vulnerable areas. There is a lack of high resolution (sub-basin scale) analyses on (i) vulnerable crops (varieties) and area under the crops; (ii) the magnitude of impacts on crop productivity and production associated with water deficits; and (iii) the impacts of reduced production on food security and livelihoods, necessary for identifying the climate vulnerable sub-basins in the HMA region. More work is needed to identify the vulnerable sub-basins based on projected changes in water supply, impacts on food production, and population. Such an information gap poses challenges in guiding mitigation measures against the potential climate change impacts on food security in the already food insecure HMA region. A lack of reliable monitoring and validation data is a major challenge to launch crop insurance products for insurance industries. Without the current scheme where the government is bearing a high cost to support both farmers and insurance industries to improve the viability of the insurance products, farmers will be more vulnerable to risks posed by climate on food production. Climate change will have differential impacts at various sub-basins of the Ganga and Indus Basins (Mishra et al., 2020); however, more work is required to identify the most vulnerable sub-basins to focus on in order to maintain food security under climate change scenarios in the region.
More research is needed to understand future impacts on hydropower production at the sub-basin scale with a combination of hydro-climatological changes (e.g., increasing glacier retreat, decreasing snowpack, decreasing rainfall) and changes in water demand for hydropower (number and size) as well as agricultural and biodiversity in each sub-basin. Ali et al. (2018) showed that increased hydropower production in India is possible under future climates due to increases in rainfall, but the temporal distribution of rain may change within a year, which can result in a shift in how reservoirs are managed. Increasing temperatures are predicted to increase irrigation, energy demand, and evapotranspiration of stored water in dams, leading to challenging tradeoffs between water use for energy vs. downstream use for food production and biodiversity (Perrone and Hornberger, 2014; Zeng et al., 2017). Additional studies integrating cryosphere, hydrology and hydropower production modeling highlight the importance of coupling energy systems analyses with hydro-climatic projections (Beheshti et al., 2019; Bocchiola et al., 2020; Shirsat et al., 2021).
Even when the effects of climate change on the magnitude and variability of river flows can be predicted, it is extremely challenging to estimate the potential magnitude of climate induced changes in the production, abundance, and diversity of biological resources that could occur in Himalayan river basins. This difficulty arises from a paucity of historic data for examining relationships between flows and biological resources within the region and is compounded by the site-specific nature of the information needed to assess effects within specific river basins. In some cases, appropriate information can be obtained by examining data gathered or developed to prepare environmental impact assessments for planned developments such as hydropower facilities. For example, efforts to identify flow needs for maintaining fish populations or for protecting key fish habitats can be used to develop suitability requirements against which predicted changes in flow regimes can be evaluated.
HMA faces overarching challenges to understand how climate change will impact the food-energy-water nexus (FEWN) and to identify water resource management plans that can mitigate pending risks. Climate-mediated changes in precipitation patterns, radiative forcing agents, and snow and glacier melt are expected to substantially alter the river flow in HMA at various spatial and temporal scales. The changes in seasonal and long-term hydrological conditions have far-reaching impacts annually and over the next century. HMA nations have challenges to maintain the ecosystem stock and flow of ecosystem services generated from the altered river flow that supports the FEWN security. Sustainability of the highly interconnected FEWN will be a fundamental and increasing challenge in a changing regional climate regime.
The HMA moves from highly glacierized high mountainous terrain to agriculturally dominated floodplains and oceanic drainage. The region supports over 50 million people within the Himalaya area and over 600 million people in the larger combined drainage basin. Therefore, HMA is a unique domain in terms of the complexity of its natural systems and incredible support and dependencies of the global population and larger environment. Traditional in-situ networks are hard to establish in the high elevation source watersheds, but are crucially important to validate models of snow- and glacial-melt as well as downstream hydrology. Such complexity gives rise to innovation, both in terms of how resources may be managed as well as how scientific research can proceed. The first and now second rounds of the NASA HiMAT project demonstrate the type of research innovation that could push for collaborative, integrated methods and thinking across and within disciplines (Arendt et al., 2020) with over 50 publications coming from integrated projects thus far. These innovations included the integration of remote sensing data with novel reanalysis and downscaling approaches that provided best estimates of precipitation and SWE despite the overall lack of ground observations. We also advanced our capacity to leverage remote sensing observations in improving the accuracy of land surface models, and in generating high resolution downscaled meteorological grids. Our high resolution glacier observations provided a regionally comprehensive map of glacier change that greatly improved our capacity to calibrate glacier models and generate more accurate estimates of future glacier changes. Advances in our understanding of the impacts of LAPs on snow and glacier melt also provided new ways for us to quantify the impacts of atmospheric pollution on the surface energy budget of the HMA.
The challenges in understanding the projected impacts of climate-led changes on FEWN in predominantly agriculture based HMA nations were also discussed. In HMA, baseline information on ecosystem services stock and flow associated with HMA Rivers is extremely limited. These rivers support clean water and sanitation (SDG 6), food production (SDG 2), and in tackling poverty (SDG 1) in the HMA region in the changing climate regime. There is a pressing need for generating high resolution information on the interaction between water supply and water demand under projected climate change and the associated uncertainty. Mishra et al. (2018, 2020) attempted to develop and demonstrate an integrated assessment framework for HMA. The IAA integrates high resolution climate model outputs in hydrologic models and couples them with changes in downstream ecosystem services such as hydropower and fish biology. The analytical complexity and rigor increases quickly when multiple components need to be integrated into the modeling framework such as various types of hydropower facilities (run-of-the-river, storage type etc.), irrigation infrastructures, various crops (irrigated and rain-fed), and water supply to cities and towns as well as fisheries. Such multidisciplinary analyses for each of the several hundred HMA sub-basins require high computational facilities. Addressing the challenges is daunting; however, without a research effort commensurate with the scale of the problem, the livelihood of the 1/4th of the world's population residing in or downstream of the HMA region will be at increasing risk with changing climate.
SM conceived the manuscript. SM, AA, and SR coordinated the writing and preparation of the manuscript. All authors contributed to writing and editing the manuscript.
This work was enabled by the funds of the National Aeronautical and Space Administration, Earth Science Division's High Mountain Asia program Grant No. NNH15ZDA001NHMA under separate grants, including NASA Grant No. 15-HMA15-0016 to the NOAA/Geophysical Fluid Dynamics Laboratory, NASA Grant No. NNX16AQ61G to the University of Utah, NASA Grant No. NNH15ZDA001N to Argonne National Laboratory, NNX16AQ83G to City University of New York, NASA Grant No. NNX17AB27G to the University of Alaska, Fairbanks, NASA Grant No. NNX17AB28G and 80NSSC20K1595 to the University of New Hampshire, NASA Grant No. 80NSSC19K0653 to the University of Dayton, NASA Grant No. 80NSSC19K0653 to the Planetary Science Institute, NASA grant no. NNX16AQ62G to the University of Arizona, NASA Grant Number 80NSSC20K1595 to Carnegie Mellon University, NNX16AQ89G to George Mason University, NNX16AQ63G to the University of California, Los Angeles, and NASA Grant No. NNX16AQ88G to the University of Washington. Sandia National Laboratories is a multimission laboratory managed and operated by National Technology and Engineering Solutions of Sandia LLC, a wholly owned subsidiary of Honeywell a Inc. for the U.S. Department of Energy's National Nuclear Security Administration under contract DE-NA0003525.
The authors declare that the research was conducted in the absence of any commercial or financial relationships that could be construed as a potential conflict of interest.
All claims expressed in this article are solely those of the authors and do not necessarily represent those of their affiliated organizations, or those of the publisher, the editors and the reviewers. Any product that may be evaluated in this article, or claim that may be made by its manufacturer, is not guaranteed or endorsed by the publisher.
Aeschbach-Hertig, W., and Gleeson, T. (2012). Regional strategies for the accelerating global problem of groundwater depletion. Nat. Geosci. 5, 853–861. doi: 10.1038/ngeo1617
Ahmad, J. A., Forman, B. A., and Kwon, Y. (2019). Analyzing machine learning predictions of passive microwave brightness temperature spectral difference over snow-covered terrain in high mountain Asia. Front. Earth Sci. 7:212. doi: 10.3389/feart.2019.00212
Akhtar, M., Ahmad, N., and Booij, M. J. (2008). The impact of climate change on the water resources of Hindukush–Karakorum–Himalaya region under different glacier coverage scenarios. J. Hydrol. 355, 148–163. doi: 10.1016/j.jhydrol.2008.03.015
Ali, S. A., Aadhar, S., and Shah, H. L. (2018). Projected increase in hydropower production in India under climate change. Sci. Rep. 8:12450. doi: 10.1038/s41598-018-30489-4
Andermann, C., Longuevergne, L., Bonnet, S., Crave, A., Davy, P., and Gloaguen, R. (2012). Impact of transient groundwater storage on the discharge of Himalayan rivers. Nat. Geocsci. 5, 127–132. doi: 10.1038/ngeo1356
Arendt, A., Krakauer, N., Kumar, S. V., Rounce, D. R., and Rupper, S. (2020). Editorial: collaborative research to address changes in the climate, hydrology and cryosphere of high mountain Asia. Front. Earth Sci. 8:326. doi: 10.3389/feart.2020.605336
Armstrong, R. L., Rittger, K., Brodzik, M. J., Racoviteanu, A., Barrett, A. P., Khalsa, S.-J. S., et al. (2018). Runoff from glacier ice and seasonal snow in high Asia: separating melt water sources in river flow. Reg. Environ. Change 19, 1249–1261. doi: 10.1007/s10113-018-1429-0
Azam, M. F., Wagnon, P., Berthier, E., Vincent, C., Fujita, K., and Kargel, J. S. (2018). Review of the status and mass changes of himalayan-karakoram glaciers. J. Glaciol. 64, 61–74. doi: 10.1017/jog.2017.86
Bair, E., Stillinger, T., Rittger, K., and Skiles, M. (2021). COVID-19 lockdowns show reduced pollution on snow and ice in the Indus river basin. Proc. Nat. Acad. Sci. U.S.A. 118:e2101174118. doi: 10.1073/pnas.2101174118
Bair, E. H., Rittger, K., Ahmad, J. A., and Chabot, D. (2020). Comparison of modeled snow properties in Afghanistan, Pakistan, and Tajikistan. Cryosphere, 14, 331–347. doi: 10.5194/tc-14-331-2020
Bair, E. H., Rittger, K., Davis, R. E., Painter, T. H., and Dozier, J. (2016). Validating reconstruction of snow water equivalent in California's Sierra Nevada using measurements from the NASA airborne snow observatory. Water Resour. Res. 52, 8437–8460. doi: 10.1002/2016WR018704
Bair, E. H., Rittger, K., Skiles, S. M., and Dozier, J. (2019). An examination of snow albedo estimates From MODIS and their impact on snow water equivalent reconstruction. Water Resour. Res. 55, 7826–7842. doi: 10.1029/2019WR024810
Barnett, T. P., Adam, J. C., and Lettenmaier, D. P. (2005). Potential impacts of a warming climate on water availability in snow-dominated regions. Nature 438, 303–309. doi: 10.1038/nature04141
Beheshti, M., Heidari, A., and Saghafian, B. (2019). Susceptibility of hydropower generation to climate change: karun III dam case study. Water 11:1025. doi: 10.3390/w11051025
Beljaars, A., Balsamo, G., Bechtold, P., Bozzo, A., Forbes, R., Hogan, R. J., et al. (2018). The numerics of physical parametrization in the ECMWF model. Front. Earth Sci. 6:137. doi: 10.3389/feart.2018.00137
Bhushan, S., Syed, T. H., Arendt, A. A., Kulkarni, A. V., and Sinha, D. (2018). Assessing controls on mass budget and surface velocity variations of glaciers in Western himalaya. Sci. Rep. 8:8885. doi: 10.1038/s41598-018-27014-y
Biemans, H., Siderius, C., Lutz, A. F., Nepal, S., Ahmad, B., Hassan, T., et al. (2019). Importance of snow and glacier meltwater for agriculture on the indo-gangetic plain. Nat. Sust. 2, 594–601. doi: 10.1038/s41893-019-0305-3
Bocchiola, D., Manara, M., and Mereu, R. (2020). Hydropower potential of run of river schemes in the himalayas under climate change: a case study in the dudh koshi basin of Nepal. Water 12:2625. doi: 10.3390/w12092625
Bolch, T., Kulkarni, A., Kääb, A., Huggel, C., Paul, F., Cogley, J. G., et al. (2012). The state and fate of himalayan glaciers. Science 336, 310–314. doi: 10.1126/science.1215828
Bookhagen, B., and Burbank, D. W. (2010). Toward a complete Himalayan hydrological budget: spatiotemporal distribution of snowmelt and rainfall and their impact on river discharge. J. Geophys. Res. Earth Surf. 115:F03019. doi: 10.1029/2009JF001426
Bormann, K. J., Brown, R. D., Derksen, C., and Painter, T. H. (2018). Estimating snow-cover trends from space. Nat. Clim. Change 8, 924–928. doi: 10.1038/s41558-018-0318-3
Bothe, O., Fraedrich, K., and Zhu, X. (2011). Large-scale circulations and tibetan plateau summer drought and wetness in a high-resolution climate model. Int. J. Climatol. 31, 832–846. doi: 10.1002/joc.2124
Brauning, A. (2006). Tree-ring evidence of “little ice age” glacier advances in southern Tibet. Holocene 16, 369–380,. doi: 10.1191/0959683606hl922rp
Brun, F., Berthier, E., Wagnon, P., Kääb, A., and Treichler, D. (2017). A spatially resolved estimate of high mountain Asia glacier mass balances from 2000 to 2016. Nat. Geosci. 10, 668–673. doi: 10.1038/ngeo2999
Brun, F., Treichler, D. S., Shean, D., and Immerzeel, W. W. (2020). Limited contribution of glacier mass loss to the recent increase in tibetan plateau lake volume. Front. Earth Sci. 8:582060. doi: 10.3389/feart.2020.582060
Buri, P., Miles, E. S., Steiner, J. F., Ragettli, S., and Pellicciotti, F. (2021). Supraglacial ice cliffs can substantially increase the mass loss of debris-covered glaciers. Geophys. Res. Lett. 48:e2020GL092150. doi: 10.1029/2020GL092150
Casey, K. A., Kaspari, S. D., Skiles, S. M., Kreutz, K., and Handley, M. J. (2017). The spectral and chemical measurement of pollutants on snow near South pole, Antarctica. J. Geophys. Res. Atmosph. 122, 6592–6610. doi: 10.1002/2016JD026418
Chaney, N. W., Metcalfe, P., and Wood, E. F. (2016). HydroBlocks: a field-scale resolving land surface model for application over continental extents. Hydrol. Process. 30, 3543–3559. doi: 10.1002/hyp.10891
Chen, F., Zhang, M., Guo, H., Allen, S., Kargel, J. S., Haritashya, U. K., et al. (2021). Annual 30 m dataset for glacial lakes in High mountain Asia from 2008 to 2017. Earth Syst. Sci. Data 13, 741–766. doi: 10.5194/essd-13-741-2021
Cheng, G., and Wu, T. (2007). Responses of permafrost to climate change and their environmental significance, Qinghai-Tibet Plateau. J. Geophys. Res. Earth Surf. 112:F02S03. doi: 10.1029/2006JF000631
Christensen, M. F., Heaton, M. J., Rupper, S., Reese, C. S., and Christensen, W. F. (2019). Bayesian multi-scale spatio-temporal modeling of precipitation in the indus watershed. Front. Earth Sci. 7:210. doi: 10.3389/feart.2019.00210
Chuwah, C., Noije, T., Vuuren, D., Sager, P. L., and Hazeleger, W. (2015). Global and regional climate impacts of future aerosol mitigation in an RCP6.0-like scenario in EC-Earth. Climat. Change 134, 1–14. doi: 10.1007/s10584-015-1525-9
Ciracì, E., Velicogna, I., and Swenson, S. (2020). Continuity of the mass loss of the world's glaciers and ice caps from the GRACE and GRACE follow-on missions. Geophys. Res. Lett. 47:e2019GL086926. doi: 10.1029/2019GL086926
Cogley, J. G., Hock, R., Rasmussen, L. A., Arendt, A. A., Bauder, A., Braithwaite, R. J., et al. (2011). Glossary of glacier mass balance and related terms. IHP-VII Techn. Doc. Hydrol. 124.
Dars, G. H., Sattar, M., Tauseef, M., Strong, C., and Najafi, M. R. (2021). Study of multi-model ensemble high-resolution projections of major climatic variables over the indus river Basin and Pakistan. Mehran Univer. Res. J. Eng. Technol. 40, 104–115. doi: 10.22581/muet1982.2101.10
de Kok, R. J., Kraaijenbrink, P. D. A., Tuinenburg, O. A., Bonekamp, P. N. J., and Immerzeel, W. W. (2020). Towards understanding the pattern of glacier mass balances in high mountain Asia using regional climatic modelling. Cryosphere 14, 3215–3234. doi: 10.5194/tc-14-3215-2020
Dehecq, A., Gourmelen, N., Gardner, A. S., Brun, F., Goldberg, D., Nienow, P. W., et al. (2019). Twenty-first century glacier slowdown driven by mass loss in high mountain Asia. Nat. Geosci. 12, 22–27. doi: 10.1038/s41561-018-0271-9
D'Odorico, P., Davis, K. F., Rosa, L., Carr, J. A., Chiarelli, D., Dell'Angelo, J., et al. (2018). The global food-energy-water nexus. Rev. Geophys. 56, 456–531. doi: 10.1029/2017RG000591
Dozier, J., Painter, T. H., Rittger, K., and Frew, J. E. (2008). Time–space continuity of daily maps of fractional snow cover and albedo from MODIS. Adv. Water Resour. 31, 1515–1526. doi: 10.1016/j.advwatres.2008.08.011
Duan, K., Yao, T., Sun, W., and Li, X. (2002). Indian monsoon variability in the Himalaya since AD 1800. Ann. Glaciol. 35, 437–442. doi: 10.3189/172756402781816690
Duan, K., Yao, T., and Thompson, L. G. (2006). Response of monsoon precipitation in the Himalayas to global warming. J. Geophys. Res. 111:D19110. doi: 10.1029/2006JD007084
Eis, J., van der Laan, L., Maussion, F., and Marzeion, B. (2021). Reconstruction of past glacier changes with an ice-flow glacier model: proof of concept and validation. Front. Earth Sci. 30:595755. doi: 10.3389/feart.2021.595755
Gaire, N. P., Dhakal, Y. R., Shah, S. K., Fan, Z.-X., Bräuning, A., Thapa, U. K., et al. (2018). Drought (scPDSI) reconstruction of trans-Himalayan region of central Himalaya using Pinus wallichiana tree-rings. Palaeogeogr. Palaeoclimatol. Palaeoecol. 514, 251–264. doi: 10.1016/j.palaeo.2018.10.026
Gaire, N. P., Koirala, M., Bhuju, D. R., and Carrer, M. (2017). Site- and species-specific treeline responses to climatic variability in eastern Nepal Himalaya. Dendrochronologia 41, 44–56. doi: 10.1016/j.dendro.2016.03.001
Gan, R., Sun, L., and Luo, Y. (2015). Baseflow characteristics in alpine rivers—a multi-catchment analysis in Northwest China. J. Mount. Sci. 12, 614–625. doi: 10.1007/s11629-013-2959-z
Gao, Z., Niu, F., Wang, Y., Lin, Z., and Wang, W. (2021). Suprapermafrost groundwater flow and exchange around a thermokarst lake on the Qinghai–Tibet plateau, China. J. Hydrol. 593:125882. doi: 10.1016/j.jhydrol.2020.125882
Grah, O., and Beaulieu, J. (2013). The effect of climate change on glacier ablation and baseflow support in the Nooksack river basin and implications on Pacific salmonid species protection and recovery. Clim. Change 120, 657–670. doi: 10.1007/s10584-013-0747-y
Grogan, D. S., Zhang, F., Prusevich, A., Lammers, R. B., Wisser, D., Glidden, S., et al. (2015). Quantifying the link between crop production and mined groundwater irrigation in China. Sci. Total Environ. 511, 161–175. doi: 10.1016/j.scitotenv.2014.11.076
Guo, W., Liu, S., Xu, J., Wu, L., Shangguan, D., Yao, X., et al. (2015). The second Chinese glacier inventory: data, methods and results. J. Glaciol. 61, 357–372. doi: 10.3189/2015JoG14J209
Hartman, M. D., Baron, J. S., Lammers, R. B., Cline, D. W., Band, L. E., Liston, G. E., et al. (1999). Simulations of snow distribution and hydrology in a mountain basin. Water Resour. Res. 35, 1587–1603. doi: 10.1029/1998WR900096
Hawkins, E., Ortega, P., Suckling, E., Schurer, A., Hegerl, G., Jones, P., et al. (2017). Estimating changes in global temperature since the preindustrial period. Bull. Am. Meteorol. Soc. 98, 1841–1856. doi: 10.1175/BAMS-D-16-0007.1
He, C., Flanner, M. G., Chen, F., Barlage, M., Liou, K.-N., Kang, S., et al. (2018). Black carbon-induced snow albedo reduction over the Tibetan plateau: uncertainties from snow grain shape and aerosol–snow mixing state based on an updated SNICAR model. Atmos. Chem. Phys. 18, 11507–11527. doi: 10.5194/acp-18-11507-2018
He, Y., Zhang, Z., Theakstone, W. H., Chen, T., Yao, T., and Pang, H. (2003). Changing features of the climate and glaciers in China's monsoonal temperate glacier region. J. Geophys. Res. 108:4530. doi: 10.1029/2002JD003365
Huang, A., Zhou, Y., Zhang, Y., Huang, D., Zhao, Y., and Wu, H. (2014). Changes of the annual precipitation over central Asia in the twenty-first century projected by multimodels of CMIP5. J. Clim. 27, 6627–6646. doi: 10.1175/JCLI-D-14-00070.1
Immerzeel, W. W., and Bierkens, M. F. P. (2012). Asia's water balance. Nat. Geosci. 5, 841–842. doi: 10.1038/ngeo1643
Immerzeel, W. W., Lutz, A. F., Andrade, M., Bahl, A., Biemans, H., Bolch, T., et al. (2020). Importance and vulnerability of the world's water towers. Nature 577, 364–369. doi: 10.1038/s41586-019-1822-y
International Centre for Integrated Mountain Development (2005). Atlas of the Himalaya. Eds B. Bajracharya, B. Shrestha, D. Zurick, J. Pacheco. Kathmandu: ICIMOD Publication.
Iqbal, N., Hossain, F., Lee, H., and Akhter, G. (2017). Integrated groundwater resource management in Indus Basin using satellite gravimetry and physical modeling tools. Environ. Monit. Assess. 189, 733–749. doi: 10.1007/s10661-017-5846-1
Johnson, E., and Rupper, S. (2020). An Examination of physical processes that trigger the albedo-feedback on glacier surfaces and implications for regional glacier mass balance across high mountain Asia. Front. Earth Sci. 8:326. doi: 10.3389/feart.2020.00129
Joshi, S. K., Tiwari, A., Kumar, S., Saxena, R., Khobragade, S. D., and Tripathi, S. K. (2021). Groundwater recharge quantification using multiproxy approaches in the agrarian region of Bundelkhand, central India. Groundw. Sust. Dev. 13:100564. doi: 10.1016/j.gsd.2021.100564
Kääb, A., Berthier, E., Nuth, C., Gardelle, J., and Arnaud, Y. (2012). Contrasting patterns of early twenty-first-century glacier mass change in the Himalayas. Nature 488, 495–498. doi: 10.1038/nature11324
Kang, S., Zhang, Y., Qian, Y., and Wang, H. (2020). A review of black carbon in snow and ice and its impact on the cryosphere. Earth Sci. Rev. 210:103346. doi: 10.1016/j.earscirev.2020.103346
Kapnick, S. B., Delworth, T. L., Ashfaq, M., Malyshev, S., and Milly, P. C. D. (2014). Snowfall less sensitive to warming in Karakoram than in Himalayas due to a unique seasonal cycle. Nat. Geosci. 7, 834–840. doi: 10.1038/ngeo2269
Kayastha, R. B., and Kayastha, R. (2020). “Glacio-Hydrological degree-day model (GDM) useful for the himalayan river basins,” in Himalayan Weather and Climate and Their Impact on the Environment, eds A. P. Dimri, B. Bookhagen, M. Stoffel, and T. Yasunari (Cham: Springer International Publishing), 379–398. doi: 10.1007/978-3-030-29684-1_19
Kayastha, R. B., Steiner, N., Kayastha, R., Mishra, S. K., and McDonald, K. (2020). Comparative study of hydrology and icemelt in three Nepal river basins using the glacio-hydrological degree-day model (GDM) and Observations from the advanced scatterometer (ASCAT). Front. Earth Sci. 7:354. doi: 10.3389/feart.2019.00354
Kirkham, J. D., Koch, I., Saloranta, T. M., Litt, M., Stigter, E. E., Møen, K., et al. (2019). Near real-time measurement of snow water equivalent in the Nepal himalayas. Front. Earth Sci. 7:177. doi: 10.3389/feart.2019.00177
Kirschbaum, D., Kapnick, S. B., Stanley, T., and Pascale, S. (2020). Changes in extreme precipitation and landslides over high mountain Asia. Geophys. Res. Lett. 47:e2019GL085347. doi: 10.1029/2019GL085347
Kraaijenbrink, P. D. A., Bierkens, M. F. P., Lutz, A. F., and Immerzeel, W. W. (2017). Impact of a global temperature rise of 1.5 degrees celsius on Asia's glaciers. Nature 549, 257–260. doi: 10.1038/nature23878
Krakauer, N. Y., Lakhankar, T., and Dars, G. H. (2019). Precipitation trends over the Indus basin. Climate 7:116. doi: 10.3390/cli7100116
Krakauer, N. Y., Pradhanang, S. M., Lakhankar, T., and Jha, A. K. (2013). Evaluating satellite products for precipitation estimation in mountain regions: a case study for Nepal. Remote Sens. 5, 4107–4123. doi: 10.3390/rs5084107
Kramer, R. J., He, H., Soden, B. J., Oreopoulos, L., Myhre, G., Forster, P. M., et al. (2021). Observational evidence of increasing global radiative forcing. Geophys. Res. Lett. 48:e2020GL091585. doi: 10.1029/2020GL091585
Kripalani, R. H., Kulkarni, A., and Sabade, S. S. (2003). Western Himalayan snow cover and Indian monsoon rainfall: a re-examination with INSAT and NCEP/NCAR data. Theor. Appl. Climatol. 74, 1–18. doi: 10.1007/s00704-002-0699-z
Kumar, S. V., Peters-Lidard, C. D., Tian, Y., Houser, P. R., Geiger, J., Olden, S., et al. (2006). Land information system: an interoperable framework for high resolution land surface modeling. Environ. Model. Softw. 21, 1402–1415. doi: 10.1016/j.envsoft.2005.07.004
Kwon, Y., Forman, B. A., Ahmad, J. A., Kumar, S. V., and Yoon, Y. (2019). Exploring the utility of machine learning-based passive microwave brightness temperature data assimilation over terrestrial snow in high mountain Asia. Remote Sens. 11:2265. doi: 10.3390/rs11192265
Lammers, R., Band, L., and Tague, C. (1997). Scaling Behaviour of Watershed Processes. Scaling-up : From Cell to Landscape. Available online at: https://scholars.unh.edu/faculty_pubs/155
Lang, T. J., and Barros, A. P. (2004). Winter storms in the central Himalayas. J. Meteorol. Soc. Japan 82, 829–844. doi: 10.2151/jmsj.2004.829
Lehner, B., Verdin, K., and Jarvis, A. (2008). New global hydrography derived from spaceborne elevation data. Eos Trans. Am. Geophys. Union 89, 93–104. doi: 10.1029/2008EO100001
Liu, C., and Allan, R. P. (2012). Multi-satellite observed responses of precipitation and its extremes to interannual climate variability. J. Geophys. Res. Atmosp. 117:D03101. doi: 10.1029/2011JD016568
Liu, Y., Fang, Y., and Margulis, S. A. (2021). Spatiotemporal distribution of seasonal snow water equivalent in high-mountain Asia from an 18-year Landsat-MODIS era snow reanalysis dataset. Cryosph. Discuss. 1–25. doi: 10.5194/tc-2021-139. [Epub ahead of print].
Liu, Y., and Margulis, S. A. (2019). Deriving bias and uncertainty in MERRA-2 snowfall precipitation over high mountain Asia. Front. Earth Sci. Cryosph. Sci. 8:326. doi: 10.3389/feart.2019.00280
Loomis, B. D., Richey, A. S., Arendt, A. A., Appana, R., Deweese, Y.-J. C., Forman, B. A., et al. (2019). Water storage trends in high mountain Asia. Front. Earth Sci. 7:235. doi: 10.3389/feart.2019.00235
Lund, J., Forster, R. R., Rupper, S. B., Deeb, E. J., Marshall, H. P., Hashmi, M. Z., et al. (2020). Mapping snowmelt progression in the upper indus basin with synthetic aperture radar. Front. Earth Sci. 7:318. doi: 10.3389/feart.2019.00318
Lutz, A. F., Immerzeel, W. W., Kraaijenbrink, P. D. A., Shrestha, A. B., and Bierkens, M. F. P. (2016). Climate change impacts on the upper indus hydrology: sources, shifts and extremes. PLoS ONE 11:e0165630. doi: 10.1371/journal.pone.0165630
Lutz, A. F., Immerzeel, W. W., Shrestha, A. B., and Bierkens, M. F. P. (2014). Consistent increase in High Asia's runoff due to increasing glacier melt and precipitation. Nat. Clim. Change 4, 587–592. doi: 10.1038/nclimate2237
Maurer, J. M., Schaefer, J. M., Rupper, S., and Corley, A. (2019). Acceleration of ice loss across the Himalayas over the past 40 years. Sci. Adv. 5:eaav7266. doi: 10.1126/sciadv.aav7266
Maussion, F., Butenko, A., Champollion, N., Dusch, M., Eis, J., Fourteau, K., et al. (2019). The open global glacier model (OGGM) v1.1. Geosci. Model Dev. 12, 909–931. doi: 10.5194/gmd-12-909-2019
Mei, Y., Maggioni, V., Houser, P., Xue, Y., and Rouf, T. (2020). A nonparametric statistical technique for spatial downscaling of precipitation over high Mountain Asia. Water Resour. Res. 56:e2020WR027472. doi: 10.1002/essoar.10502607.1
Meng, Y., Liu, G., and Li, M. (2015). Tracing the sources and processes of groundwater in an alpine glacierized region in Southwest China. Water 7, 2673–2690. doi: 10.3390/w7062673
Mishra, S. K., Hayse, J., Veselka, T., Yan, E., Kayastha, R. B., LaGory, K., et al. (2018). An integrated assessment approach for estimating the economic impacts of climate change on river systems: an application to hydropower and fisheries in a Himalayan River, Trishuli. Environ. Sci. Policy 87, 102–111. doi: 10.1016/j.envsci.2018.05.006
Mishra, S. K., Veselka, T. D., Prusevich, A. A., Grogan, D. S., Lammers, R. B., Rounce, D. R., et al. (2020). Differential impact of climate change on the hydropower economics of two river basins in high mountain Asia. Front. Environ. Sci. 8:26. doi: 10.3389/fenvs.2020.00026
Moors, E. J., Groot, A., Biemans, H., van Scheltinga, C. T., Siderius, C., Stoffel, M., et al. (2011). Adaptation to changing water resources in the Ganges basin, northern India. Environ. Sci. Policy 14, 758–769. doi: 10.1016/j.envsci.2011.03.005
National Academies of Sciences E. (2018). Thriving on Our Changing Planet: A Decadal Strategy for Earth Observation from Space. Washington, DC: National Academies of Sciences.
Olson, M., and Rupper, S. (2019). Impacts of topographic shading on direct solar radiation for valley glaciers in complex topography. Cryosphere 13, 29–40. doi: 10.5194/tc-13-29-2019
O'Neel, S., Hood, E., Arendt, A., and Sass, L. (2014). Assessing streamflow sensitivity to variations in glacier mass balance. Clim. Change 123, 329–341. doi: 10.1007/s10584-013-1042-7
Painter, T. H., Bryant, A. C., and Skiles, S. M. (2012). Radiative forcing by light absorbing impurities in snow from MODIS surface reflectance data. Geophys. Res. Lett. 39:L17502. doi: 10.1029/2012GL052457
Painter, T. H., Deems, J. S., Belnap, J., Hamlet, A. F., Landry C. C., and Udall, B. (2010). Response of Colorado River runoff to dust radiative forcing in snow. Proc. Natl. Acad. Sci. U.S.A. 107, 17125–17130. doi: 10.1073/pnas.0913139107
Painter, T. H., Dozier, J., Roberts, D. A., Davis, R. E., and Green, R. O. (2003). Retrieval of subpixel snow-covered area and grain size from imaging spectrometer data. Remote Sens. Environ. 85, 64–77. doi: 10.1016/S0034-4257(02)00187-6
Painter, T. H., Rittger, K., McKenzie, C., Slaughter, P., Davis, R. E., and Dozier, J. (2009). Retrieval of subpixel snow covered area, grain size, and albedo from MODIS. Remote Sens. Environ. 113, 868–879. doi: 10.1016/j.rse.2009.01.001
Palazzi, E., von Hardenberg, J., Terzago, S., and Provenzale, A. (2015). Precipitation in the Karakoram-himalaya: a CMIP5 view. Clim. Dynam. 45, 21–45. doi: 10.1007/s00382-014-2341-z
Pandey, P., Ali, S. N., Sharma, V., and Ray, P. (2020). Focus on thermokarst lakes in indian himalaya: inception and implication under warming climate. J. Clim. Change 6, 59–69. doi: 10.3233/JCC200012
Paul, F., Winsvold, S. H., Kääb, A., Nagler, T., and Schwaizer, G. (2016). Glacier remote sensing using sentinel-2. Part II: mapping glacier extents and surface facies, and comparison to landsat 8. Remote Sens. 8:575. doi: 10.3390/rs8070575
Pepin, N., Bradley, R. S., Diaz, H. F., Baraer, M., Caceres, E. B., Forsythe, N., et al. (2015). Elevation-dependent warming in mountain regions of the world. Nat. Clim. Change 5, 424–430. doi: 10.1038/nclimate2563
Perrone, D., and Hornberger, G. M. (2014). Water, food, and energy security: Scrambling for resources or solutions? WIREs Water 1, 49–68. doi: 10.1002/wat2.1004
Pfeffer, W. T., Arendt, A. A., Bliss, A., Bolch, T., Cogley, J. G., Gardner, A. S., et al. (2014). The randolph glacier inventory: a globally complete inventory of glaciers. J. Glaciol. 60, 537–552. doi: 10.3189/2014JoG13J176
Pritchard, H. D. (2019). Asia's shrinking glaciers protect large populations from drought stress. Nature 569, 649–654. doi: 10.1038/s41586-019-1240-1
Qian, Y., Flanner, M. G., Leung, L. R., and Wang, W. (2011). Sensitivity studies on the impacts of Tibetan Plateau snowpack pollution on the Asian hydrological cycle and monsoon climate. Atmosph. Chem. Phys. 11, 1929–1948. doi: 10.5194/acp-11-1929-2011
Qian, Y., Wang, H., Zhang, R., Flanner, M. G., and Rasch, P. J. (2014). A sensitivity study on modeling black carbon in snow and its radiative forcing over the Arctic and Northern China. Environ. Res. Lett. 9:064001. doi: 10.1088/1748-9326/9/6/064001
Qian, Y., Yasunari, T. J., Doherty, S. J., Flanner, M. G., Lau, W. K. M., Ming, J., et al. (2015). Light-absorbing particles in snow and ice: measurement and modeling of climatic and hydrological impact. Adv. Atmosph. Sci. 32, 64–91. doi: 10.1007/s00376-014-0010-0
Qiao, B., Zhu, L., and Yang, R. (2019). Temporal-spatial differences in lake water storage changes and their links to climate change throughout the Tibetan plateau. Remote Sens. Environ. 222, 232–243. doi: 10.1016/j.rse.2018.12.037
Racoviteanu, A. E., Arnaud, Y., Baghuna, I. M., Bajracharya, S. R., Berthier, E., Bhambri, R., et al. (2014). “Himalayan glaciers (India, Bhutan, Nepal): satellite observations of thinning and retreat,” in Global Land Ice Measurements From Space, eds J. S. Kargel, G. J. Leonard, M. P. Bishop, A. Kääb, and B. H. Raup (Berlin: Springer), 549–582. doi: 10.1007/978-3-540-79818-7_24
Raleigh, M. S., Rittger, K., Moore, C. E., Henn, B., Lutz, J. A., and Lundquist, J. D. (2013). Ground-based testing of MODIS fractional snow cover in subalpine meadows and forests of the Sierra Nevada. Remote Sens. Environ. 128, 44–57. doi: 10.1016/j.rse.2012.09.016
RGI Consortium (2017). Randolph Glacier Inventory – A Dataset of Global Glacier Outlines: Version 6.0: Technical Report, Global Land Ice Measurements From Space, Colorado, USA. Colorado: Digital Media.
Richey, A. S., Thomas, B. F., Lo, M.-H., Reager, J. T., Famiglietti, J. S., Voss, K., et al. (2015). Quantifying renewable groundwater stress with GRACE. Water Resour. Res. 51, 5217–5238. doi: 10.1002/2015WR017349
Riley, C., Rupper, S., Steenburgh, J. W., Strong, C., Kochanski, A. K., and Wolvin, S. (2021). Characteristics of historical precipitation in high mountain Asia based on a 15-year high resolution dynamical downscaling. Atmosphere 12:355. doi: 10.3390/atmos12030355
Rittger, K., Bair, E. H., Kahl, A., and Dozier, J. (2016). Spatial estimates of snow water equivalent from reconstruction. Adv. Water Resour. 94, 345–363. doi: 10.1016/j.advwatres.2016.05.015
Rittger, K., Bormann, K. J., Bair, E. H., Dozier, J., and Painter, T. H. (2021). Evaluation of VIIRS and MODIS snow cover fraction in high-mountain Asia using landsat 8 OLI. Front. Remote Sens. 2:647154. doi: 10.3389/frsen.2021.647154
Rittger, K., Krock, M., Kleiber, M., Bair, E. H., Brodzik, M. J., Stephenson, T., Rajagopalan, B., Bormann, K. J., and Painter, T. H. (2021). Multi-Sensor Fusion Using Random Forests for Daily Fractional Snow Cover at 30 m. Remote Sens. Environ. 264:112608. doi: 10.1016/j.rse.2021.112608
Rittger, K., Raleigh, M. S., Dozier, J., Hill, A. F., Lutz, J. A., and Painter, T. H. (2020). Canopy adjustment and improved cloud detection for remotely sensed snow cover mapping. Water Resourc. Res. 56:e2019WR024914. doi: 10.1029/2019WR024914
Rodell, M., Famiglietti, J. S., Wiese, D. N., Reager, J. T., Beaudoing, H. K., Landerer, F. W., et al. (2018). Emerging trends in global freshwater availability. Nature 557, 651–659. doi: 10.1038/s41586-018-0123-1
Rodell, M., Velicogna, I., and Famiglietti, J. S. (2009). Satellite-based estimates of groundwater depletion in India. Nature 460, 999–1002. doi: 10.1038/nature08238
Rosenthal, W., and Dozier, J. (1996). Automated mapping of montane snow cover at subpixel resolution from the landsat thematic mapper. Water Resour. Res. 32, 115–130. doi: 10.1029/95WR02718
Rouf, T., Mei, Y., Maggioni, V., Houser, P., and Noonan, M. (2020). A physically-based atmospheric variables downscaling technique. J. Hydrometeorol. 21, 93–108. doi: 10.1175/JHM-D-19-0109.1
Rounce, D., Hock, R., and Shean, D. E. (2020a). Glacier mass change in high mountain asia through 2100 using the open-source python glacier evolution model (PyGEM). Front. Earth Sci. 7:331. doi: 10.3389/feart.2019.00331
Rounce, D., Khurana, T., Short, M., Hock, R., Shean, D., and Brinkherhoff, D. (2020b). Quantifying parameter uncertainty in a large-scale glacier evolution model using Bayesian inference – application to high mountain Asia. J. Glaciol. 256, 175–187. doi: 10.1017/jog.2019.91
Rounce, D. R., Hock, R., McNabb, R. W., Millan, R., Sommer, C., Braun, M. H., et al. (2021). Distributed global debris thickness estimates reveal debris significantly impacts glacier mass balance. Geophys. Res. Lett. 48:e2020GL091311. doi: 10.1029/2020GL091311
Sarangi, C., Qian, Y., Rittger, K., Bormann, K. J., Liu, Y., Wang, H., et al. (2019). Impact of light-absorbing particles on snow albedo darkening and associated radiative forcing over high-mountain Asia: high-resolution WRF-Chem modeling and new satellite observations. Atmosph. Chem. Phys. 19, 7105–7128. doi: 10.5194/acp-19-7105-2019
Sarangi, C., Qian, Y., Rittger, K., Ruby Leung, L., Chand, D., Bormann, K. J., et al. (2020). Dust dominates high-altitude snow darkening and melt over high-mountain Asia. Nat. Clim. Change 10, 1045–1051. doi: 10.1038/s41558-020-00909-3
Scherler, D., Wulf, H., and Gorelick, N. (2018). Global assessment of supraglacial debris-cover extents. Geophys. Res. Lett. 45, 798–805. doi: 10.1029/2018GL080158
Schwab, N., Schickhoff, U., Müller, M., Gerlitz, L., Bürzle, B., Böhner, J., et al. (2016). Treeline responsiveness to climate warming: Insights from a krummholz treeline in Rolwaling Himal, Nepal. Clim. Change 10, 307–345. doi: 10.1007/978-3-319-28977-9_16
Senan, R., Orsolini, Y. J., Weisheimer, A., Vitart, F., Balsamo, G., Stockdale, T. N., et al. (2016). Impact of springtime Himalayan–Tibetan plateau snowpack on the onset of the Indian summer monsoon in coupled seasonal forecasts. Clim. Dynam. 47, 2709–2725. doi: 10.1007/s00382-016-2993-y
Shean, D. E., Bhushan, S., Montesano, P., Rounce, D. R., Arendt, A., and Osmanoglu, B. (2020). A systematic, regional assessment of high mountain asia glacier mass balance. Front. Earth Sci. 7:363. doi: 10.3389/feart.2019.00363
Shirsat, T. S., Kulkarni, A. V., Momblanch, A., Randhawa, S. S., and Holman, I. P. (2021). Towards climate-adaptive development of small hydropower projects in Himalaya: a multi-model assessment in upper Beas basin. J. Hydrol. Reg. Stud. 34:100797. doi: 10.1016/j.ejrh.2021.100797
Shrestha, M., Koike, T., Hirabayashi, Y., Xue, Y., Wang, L., Rasul, G., et al. (2015). Integrated simulation of snow and glacier melt in water and energy balance-based, distributed hydrological modeling framework at Hunza river basin of Pakistan Karakoram region: integrated snow and glaciermelt model. J. Geophys. Res. Atmosph. 120, 4899–4919. doi: 10.1002/2014JD022666
Shugar, D. H., Burr, A., Haritashya, U. K., Kargel, J. S., Watson, C. S., Kennedy, M. C., et al. (2020). Rapid worldwide growth of glacial lakes since 1990. Nat. Clim. Change 10, 939–945. doi: 10.1038/s41558-020-0855-4
Skiles, S. M., Flanner, M., Cook, J. M., Dumont, M., and Painter, T. H. (2018). Radiative forcing by light-absorbing particles in snow. Nat. Clim. Change 8, 964–971. doi: 10.1038/s41558-018-0296-5
Smith, T., and Bookhagen, B. (2018). Changes in seasonal snow water equivalent distribution in high mountain Asia (1987 to 2009). Sci. Adv. 4:e1701550. doi: 10.1126/sciadv.1701550
Sun, A., Yu, Z., Zhou, J., Acharya, K., Ju, Q., Xing, R., et al. (2020). Quantified hydrological responses to permafrost degradation in the headwaters of the yellow river (HWYR) in high Asia. Sci. Total Environ. 712:135632. doi: 10.1016/j.scitotenv.2019.135632
Sun, A., Zhou, J., Yu, Z., Jin, H., Sheng, Y., and Yang, C. (2019). Three-dimensional distribution of permafrost and responses to increasing air temperatures in the head waters of the yellow river in High Asia. Sci. Total Environ. 666, 321–336. doi: 10.1016/j.scitotenv.2019.02.110
Tian, Y., and Peters-Lidard, C. D. (2010). A global map of uncertainties in satellite-based precipitation measurements. Geophys. Res. Lett. 37:L24407. doi: 10.1029/2010GL046008
Treichler, D., Kääb, A., Salzmann, N., and Xu, C.-Y. (2019). Recent glacier and lake changes in high mountain Asia and their relation to precipitation changes. Cryosphere 13, 2977–3005. doi: 10.5194/tc-13-2977-2019
Vecchi, G. A., Delworth, T., Gudgel, R., Kapnick, S., Rosati, A., Wittenberg, A. T., et al. (2014). On the seasonal forecasting of regional tropical cyclone activity. J. Clim. 27, 7994–8016. doi: 10.1175/JCLI-D-14-00158.1
Vincent, A., Sophie, V., and Guð*finna, A. (2019). Groundwater in catchments headed by temperate glaciers: a review. Earth Sci. Rev. 188, 1–540. doi: 10.1016/j.earscirev.2018.10.017
Viviroli, D., Archer, D. R., Buytaert, W., Fowler, H. J., Greenwood, G. B., Hamlet, A. F., et al. (2011). Climate change and mountain water resources: overview and recommendations for research, management and policy. Hydrol. Earth Syst. Sci. 15, 471–504. doi: 10.5194/hess-15-471-2011
Viviroli, D., Dürr, H. H., Messerli, B., Meybeck, M., and Weingartner, R. (2007). Mountains of the world, water towers for humanity: typology, mapping, and global significance. Water Resour. Res. 43:W07447. doi: 10.1029/2006WR005653
Wada, Y., Beek, L. P., and Bierkens, M. F. (2012). Nonsustainable groundwater sustaining irrigation: a global assessment. Water Resour. Res. 48:W00L06. doi: 10.1029/2011WR010562
Wagnon, P., Vincent, C., Arnaud, Y., Berthier, E., Vuillermoz, E., Gruber, S., et al. (2013). Seasonal and annual mass balances of Mera and Pokalde glaciers (Nepal himalaya) since 2007. Cryosphere 7, 1769–1786. doi: 10.5194/tc-7-1769-2013
Wilson, A., Williams, M. W., Kayastha, R. B., and Racoviteanu, A. (2016). Use of a hydrologic mixing model to examine the roles of meltwater, precipitation on groundwater in the Langtang River basin, Nepal. Ann. Glaciol. 57, 155–168. doi: 10.3189/2016AoG71A067
Wood, E., Roundy, J. K., Troy, T. J., Beek, R., Bierkens, M., Blyth, E., et al. (2011). Hyper-Resolution global land surface modeling: meeting a grand challenge for monitoring earth's terrestrial water. Water Resour. Res. 47:W05301. doi: 10.1029/2010WR010090
World Economic Forum. (2019). Global risks 2019: Insight Report, 14th Edn. Available online at: https://www3.weforum.org/docs/WEF_Global_Risks_Report_2019.pdf
Wu, G., Liu, Y., He, B., Bao, Q., Duan, A., and Jin, F.-F. (2012). Thermal controls on the Asian summer monsoon. Sci. Rep. 2:404. doi: 10.1038/srep00404
Xue, Y., Houser, P., Maggioni, V., Mei, Y., Kumar, S., and Yoon, Y. (2021). Evaluation of high mountain Asia—land data assimilation system (version 1) from 2003 to 2016, Part I: a hyper-resolution terrestrial modeling system. J. Geophys. Res. Atmosph. 126:e2020JD034131. doi: 10.1029/2020JD034131
Xue, Y., Houser, P. R., Maggioni, V., Mei, Y., Kumar, S. V., and Yoon, Y. (2019). Assimilation of satellite-based snow cover and freeze/thaw observations over high mountain Asia. Front. Earth Sci. 7:115. doi: 10.3389/feart.2019.00115
Yao, T., Thompson, L., Yang, W., Yu, W., Gao, Y., Guo, X., et al. (2012). Different glacier status with atmospheric circulations in Tibetan Plateau and surroundings. Nat. Clim. Change 2, 663–667. doi: 10.1038/nclimate1580
Yatagai, A., Kamiguchi, K., Arakawa, O., Hamada, A., Yasutomi, N., and Kitoh, A. (2012). APHRODITE: constructing a long-term daily gridded precipitation dataset for Asia based on a dense network of rain gauges. Bull. Am. Meteorol. Soc. 93, 1401–1415. doi: 10.1175/BAMS-D-11-00122.1
Yoon, Y., Kumar, S. V., Forman, B. A., Zaitchik, B. F., Kwon, Y., Qian, Y., et al. (2019). Evaluating the uncertainty of terrestrial water budget components over high mountain Asia. Front. Earth Sci. 7:120. doi: 10.3389/feart.2019.00120
Zaveri, E., Grogan, D. S., Fisher-Vanden, K., Frolking, S., Lammers, R. B., Wrenn, D. H., et al. (2016). Invisible water, visible impact: groundwater use and Indian agriculture under climate change. Environ. Res. Lett. 11:084005. doi: 10.1088/1748-9326/11/8/084005
Zeng, C., Liu, Z., Yan, J., and Yang, R. (2015). A groundwater conceptual model and karst-related carbon sink for a glacierized alpine karst aquifer, Southwestern China. J. Hydrol. 529, 12–133. doi: 10.1016/j.jhydrol.2015.07.027
Zeng, R., Cai, X., Ringler, C., and Zhu, T. (2017). Hydropower versus irrigation—an analysis of global patterns. Environ. Res. Lett. 12, 4–6. doi: 10.1088/1748-9326/aa5f3f
Zhang, G., Luo, W., Chen, W., and Zheng, G. (2019). A robust but variable lake expansion on the Tibetan plateau. Sci. Bull. 64, 1306–1309. doi: 10.1016/j.scib.2019.07.018
Keywords: hydrology, cryosphere, climate–impact of, high Mountain Asia (HMA), water resources
Citation: Mishra SK, Rupper S, Kapnick S, Casey K, Chan HG, Ciraci' E, Haritashya U, Hayse J, Kargel JS, Kayastha RB, Krakauer NY, Kumar SV, Lammers RB, Maggioni V, Margulis SA, Olson M, Osmanoglu B, Qian Y, McLarty S, Rittger K, Rounce DR, Shean D, Velicogna I, Veselka TD and Arendt A (2021) Grand Challenges of Hydrologic Modeling for Food-Energy-Water Nexus Security in High Mountain Asia. Front. Water 3:728156. doi: 10.3389/frwa.2021.728156
Received: 21 June 2021; Accepted: 31 August 2021;
Published: 05 October 2021.
Edited by:
Kuk-Hyun Ahn, Kongju National University, South KoreaReviewed by:
Jizu Chen, Chinese Academy of Sciences (CAS), ChinaCopyright © 2021 Mishra, Rupper, Kapnick, Casey, Chan, Ciraci', Haritashya, Hayse, Kargel, Kayastha, Krakauer, Kumar, Lammers, Maggioni, Margulis, Olson, Osmanoglu, Qian, McLarty, Rittger, Rounce, Shean, Velicogna, Veselka and Arendt. This is an open-access article distributed under the terms of the Creative Commons Attribution License (CC BY). The use, distribution or reproduction in other forums is permitted, provided the original author(s) and the copyright owner(s) are credited and that the original publication in this journal is cited, in accordance with accepted academic practice. No use, distribution or reproduction is permitted which does not comply with these terms.
*Correspondence: Shruti K. Mishra, c2Fpc2hydXRpQGdtYWlsLmNvbQ==
Disclaimer: All claims expressed in this article are solely those of the authors and do not necessarily represent those of their affiliated organizations, or those of the publisher, the editors and the reviewers. Any product that may be evaluated in this article or claim that may be made by its manufacturer is not guaranteed or endorsed by the publisher.
Research integrity at Frontiers
Learn more about the work of our research integrity team to safeguard the quality of each article we publish.