- 1Department of Geography, Ludwig-Maximilians-Universität München (LMU), Munich, Germany
- 2Ouranos Consortium, Montreal, QC, Canada
Drought, caused by a prolonged deficit of precipitation, bears the risk of severe economic and ecological consequences for affected societies. The occurrence of this significant hydro-meteorological hazard is expected to strongly increase in many regions due to climate change, however, it is also subject to high internal climate variability. This calls for an assessment of climate trends and hot spots that considers the variations due to internal variability. In this study, the percent of normal index (PNI), an index that describes meteorological droughts by the deviation of a long-term reference mean, is analyzed in a single-model initial-condition large ensemble (SMILE) of the Canadian regional climate model version 5 (CRCM5) over Europe. A far future horizon under the Representative Concentration Pathway 8.5 is compared to the present-day climate and a pre-industrial reference, which is derived from pi-control runs of the CRCM5 representing a counterfactual world without anthropogenic climate change. Our analysis of the SMILE reveals a high internal variability of drought occurrence over Europe. Considering the high internal variability, our results show a clear overall increase in the duration, number and intensity of droughts toward the far future horizon. We furthermore find a strong seasonal divergence with a distinct increase in summer droughts and a decrease in winter droughts in most regions. Additionally, the percentage of summer droughts followed by wet winters is increasing in all regions except for the Iberian Peninsula. Because of particularly severe drying trends, the Alps, the Mediterranean, France and the Iberian Peninsula are suggested to be considered as drought hot spots. Due to the simplicity and intuitivity of the PNI, our results derived from this index are particularly appropriate for region-specific communication purposes and outreach.
1. Introduction
With progressing climate change droughts have become a critical high-impact hydro-meteorological hazard globally, and particularly in Europe, in recent years (Spinoni et al., 2018). In combination with high temperature anomalies, the deficit of precipitation has caused large economic, social and environmental costs in the years 2003, 2010 and 2018 (Bastos et al., 2020), e.g., through crop losses (Spinoni et al., 2018) or a drop in (renewable) power generation production (Naumann et al., 2021). Climate projections show that more damages are expected for the end of the twenty-first century (Spinoni et al., 2018). However, regional differences between single drought events are high (Beillouin et al., 2020) and thus call for the identification of geographical hot-spots of droughts under climate change.
Due to the complexity of drought impacts on diverse different sectors like water supply, agriculture, and ecosystems (Spinoni et al., 2018; Liu et al., 2019), a universal definition of droughts that satisfies all users is impractical (Lloyd-Hughes, 2014). Instead, droughts are classified as meteorological, hydrological, agricultural and socio-economic droughts (Mishra and Singh, 2010; Liu et al., 2019). Meteorological droughts focus on the deficit of precipitation over a region and period of time compared to normal conditions (Mishra and Singh, 2010; Sheffield and Wood, 2012) and do not consider factors like streamflow, soil moisture or water demand (Mishra and Singh, 2010; Lloyd-Hughes, 2014). Because meteorological droughts are a potential predecessor of other drought types, their investigation is key for diverse sectors of implications nonetheless. Within each category a variety of drought indices are in use, which enable the assessment of drought frequency, duration, severity and spatial extent (Mishra and Singh, 2010). A common index for meteorological droughts is the Palmer Drought Severity Index (PDSI), which includes the effects of temperature and evapotranspiration (Palmer, 1965). A popular drought index solely based on precipitation is the Standardized Precipitation Index (SPI; McKee et al., 1993), which compares the precipitation sum of a given period with a long-term reference by fitting it to a probability distribution and then transforming it to a normal distribution. The SPI expresses the deviation of a given period from the reference in measures of the standard deviation (Mishra and Singh, 2010). A less complex index for meteorological droughts is the Percent of Normal Index (PNI; Werick et al., 1994; Willeke and Hosking, 1994). It directly represents the percentage of precipitation of a specific period compared to the long-term mean. Due to its simple calculation and intuitive meaning it can serve for communication and outreach purposes (Smakhtin and Hughes, 2004; Nikbakht et al., 2013).
In the study of Spinoni et al. (2018), climate projections using simulations from the Coordinated Regional Downscaling Experiment (CORDEX) show an increase in the frequency and severity of droughts over the entire European continent under the Representative Concentration Pathway 8.5 (RCP 8.5): This increase occurs especially in spring and summer while during winters in northern Europe the occurrence of droughts is projected to decrease. The regions with the highest increase in drought frequency in the mentioned study are the Iberian Peninsula, southern Europe, France, the British Isles and north-eastern Scandinavia. Using another type of scenarios, Lehner et al. (2017) stress the potential to reduce mean dryness conditions and drought lengths in Europe in a scenario with 1.5°C increase with respect to pre-industrial conditions as opposed to a 2°C increase scenario by employing the PDSI in a 10-member ensemble. While there is a high agreement among different climate projections on a general drying trend over Europe in the course of the twenty-first century (Stagge et al., 2015; Dai and Zhao, 2017; Cook et al., 2020), results differ significantly between studies in regards to a potential drying trend in historical data (Blenkinsop and Fowler, 2007; Dai and Zhao, 2017; Stagge et al., 2017; Hänsel et al., 2019; Vicente-Serrano et al., 2021). The study by Stagge et al. (2017) reveals diverging drying trends over Europe from 1970 to 2014 depending on the choice of the drought index. Hänsel et al. (2019) state that the observed trend largely depends on the chosen study period due to a large temporal variability of drought conditions.
Several studies show that the occurrence of droughts is subject to a high natural variability (Bonaccorso et al., 2003; Santos et al., 2010; Hawkins and Sutton, 2011; Cook et al., 2016; Zhao and Dai, 2017; Mikšovskỳ et al., 2019; Vicente-Serrano et al., 2021). Vicente-Serrano et al. (2021) state that current long-term drought trends in Western Europe are dominated by internal variability. Zhao and Dai (2017) show the same effect for several regions worldwide, whereas they find a consistency of observed drying trends and simulated forced signal over Southern Europe. These studies underline that natural variability is an important source of uncertainty and the understanding of the temporal and spatial variability of drought occurrence is a crucial knowledge that may help to enhance the management practices of these complex extreme events e.g., with respect to the management of water resources (Santos et al., 2010). A recent study of Spinoni et al. (2020) used a very large multi-model ensemble of regional climate models (RCMs) on a grid of 0.44° spatial resolution. While such a model-setup allows robust climate projections, it cannot distinguish between model uncertainty and internal variability. A single-model initial-condition large-ensemble (SMILE), which consists of several model runs of the same model driven by the same boundary conditions but slightly differed initial conditions, offers the possibility of studying the internal variability of extreme events under climate change (Kay et al., 2015).
In this study, we present the analysis of meteorological droughts in a SMILE that consists of 50 simulations of a RCM over Europe. We take advantage of the SMILE dataset in order to assess climate change signals of drought occurrence over Europe under the consideration of their high internal variability. We analyze regional hot-spots and the frequency, severity and duration of droughts using the PNI. Climate trends for the far future under the RCP8.5 scenario are compared to present-day and pre-industrial climate. The comparison to a pre-industrial climate state is possible through the employment of novel pi-control simulations, which accompany the SMILE. These allow to relate present-day drought conditions and future trends to a counterfactual world without anthropogenic climate change. The choice of a simple drought index and the comparison with a pre-industrial reference directly aligns this study for communication and outreach purposes. To our knowledge this study is the first region-specific analysis of droughts over Europe that employs a SMILE of a high-resolution RCM in order to assess the uncertainty of internal climate variability.
2. Data and Methods
2.1. CRCM5-LE
Precipitation based indices are known to show strong internal variability (e.g., Vicente-Serrano et al., 2021). They are also highly sensitive to orographic processes (Basist et al., 1994) and so a sufficient spatial resolution of the data used is crucial for meaningful analyses inside a topographically heterogeneous domain like Europe. We therefore use data from a SMILE of a RCM in order to assess the variability of meteorological droughts over Europe at high spatial resolution. The Canadian Regional Climate Model version 5 (CRCM5; Martynov et al., 2013; Šeparovic et al., 2013) Large Ensemble (CRCM5-LE) provides 50 members at 0.11° spatial resolution, which were obtained by dynamically downscaling the Canadian Earth System Model Large Ensemble (CanESM2-LE; Fyfe et al., 2017) during the period of 1950–2099 (Leduc et al., 2019). The CRCM5-LE and the CanESM2-LE were produced as described in Fyfe et al. (2017) and Leduc et al. (2019): The CanESM2-LE was produced by applying small random atmospheric perturbations to a 1,000-year equilibrium run (CMIP5 pi-control; Arora et al., 2011) in order to obtain five historical simulations starting in 1850. In 1950 new atmospheric perturbations were applied to each of these five families resulting in 50 CanESM2-LE members, which are (after a 5-year spin-off phase) independent realizations of the modeled climate. From 2006 onward, the RCP8.5 scenario serves as external forcing. These 50 CanESM2 members were used as boundary conditions for the CRCM5-LE during the dynamical downscaling process. By construction, all members share the same climatology, but span a range which allows to estimate internal climate variability. von Trentini et al. (2019) showed that the CRCM5-LE variability of precipitation change between 2070–2099 and 1980–2009 reaches the spread of a multi-model ensemble of 22 different RCM setups.
Furthermore, a set of dynamically downscaled pi-control runs exists, which consists of 35 simulations of the CRCM5 driven by the CanESM2-pi-control run in pre-industrial mode (Arora et al., 2011). The atmospheric CO2 level of those pi-control runs corresponds to 285 ppm in the year 1850. The aerosol concentration of the pi-control runs also represents the pre-industrial mode (IPCC, 2013). The CanESM2-pi-control run covers 1,000 virtual years. During the downscaling with the CRCM5, 35 members with 22 years each are generated, whereby the first 2-years are considered as spin-up phase. The control runs thus provide a pre-industrial ensemble with a total of 700 years, which represents a climate without anthropogenic global warming.
2.2. PNI
The percent of normal index (PNI) is a precipitation-based index for the evaluation of meteorological droughts (Werick et al., 1994; Willeke and Hosking, 1994). The PNI reveals the percentage of precipitation in a given period compared to the normal precipitation in the reference period (Falzoi et al., 2019). Due to its simplicity it is particularly appropriate for region-specific communication purposes and outreach. The PNI is computed for monthly or seasonal precipitation sums and is calculated by dividing the actual precipitation sum of a given period (P) by the climatological mean of that period in the reference period (Pref) and multiplying it with 100 (Falzoi et al., 2019):
PNI values below 80% are considered as drought conditions and are further categorized into four classes of increasing severity based on Falzoi et al. (2019) (see Table 1). Note that the classes cover uneven percentages of PNI values. The PNI is calculated individually for each pixel in the European domain of the CRCM5. A disadvantage of the PNI is that it implies by construction that the precipitation sums would follow a normal distribution, for which mean and median are the same. As this is not the case, misleading cases can occur when the median of a certain period and region is ≥ 20% lower than the mean. In this case, the median has a PNI of ≤ 80% and thus 50% of the distribution would appear as dry compared to the mean (Hayes, 2002; Yihdego et al., 2019). The monthly and seasonal precipitation sums are derived from hourly data. The drizzle is removed with a threshold of 1 mm per day (Kjellström et al., 2010).
2.3. Pre-industrial Reference
We use the pre-industrial climate state derived from pi-control runs of the CRCM5 as reference for the PNI calculation. This allows the comparison of future scenarios and the present climate to a reference climate state without any anthropogenic global warming. The relevance of such a pre-industrial reference increases with progressing climate change. Since 2017 global warming has reached approximately 1.0°C above pre-industrial levels (IPCC, 2018). Using a pre-industrial reference period ensures that this substantial amount of warming is considered. To further allow a comparison of future scenarios with present-day climate we analyze two 20-year periods: 2001–2020 as present-day and 2080–2099 in the far future. This provides 50 members × 20 years per analysis period and therefore a large database to investigate extreme events. The difference between the climatological mean of the ensemble in a present-day climate vs. a pre-industrial climate state is illustrated in Figure 1. Figure 1A shows the averaged precipitation sums per season of 20-years of the 35 CRCM5 pi-control runs. This represents a pre-industrial climate state. High precipitation values are visible in mountain regions and on the west coasts of land masses (e.g., Norway, Great Britain, Galicia, the Balkans), especially in winter (DJF). The averaged precipitation sums of the present-day period 2001–2020 in the 50 members of the CRCM5-ensemble differ from that in a range of –45–74 mm (see Figure 1B). This difference reveals the climate change signal between present-day and pre-industrial and mainly shows a drying trend over large parts of Europe in summer and a trend toward wetter conditions in winter. The spatial patterns of this change go in line with near-future climate projections e.g., in the study of Kjellström et al. (2018), who compare a future 1.5°C warm world with the reference 1971–2000. Supplementary Figures 1, 2 show timeseries of the PNI values of 20-year slices in the ensemble in comparison to the pre-industrial PNI values. For summer this reveals in most regions a trend toward higher PNI values in the early period of 1955–1974 compared to pre-industrial, before a continuous decreasing trend applies until the end of the century. This intermediate increase in summer PNI values might be due to a lower radiative forcing because of higher aerosol concentrations in 1955–1974 as shown in IPCC (2013).
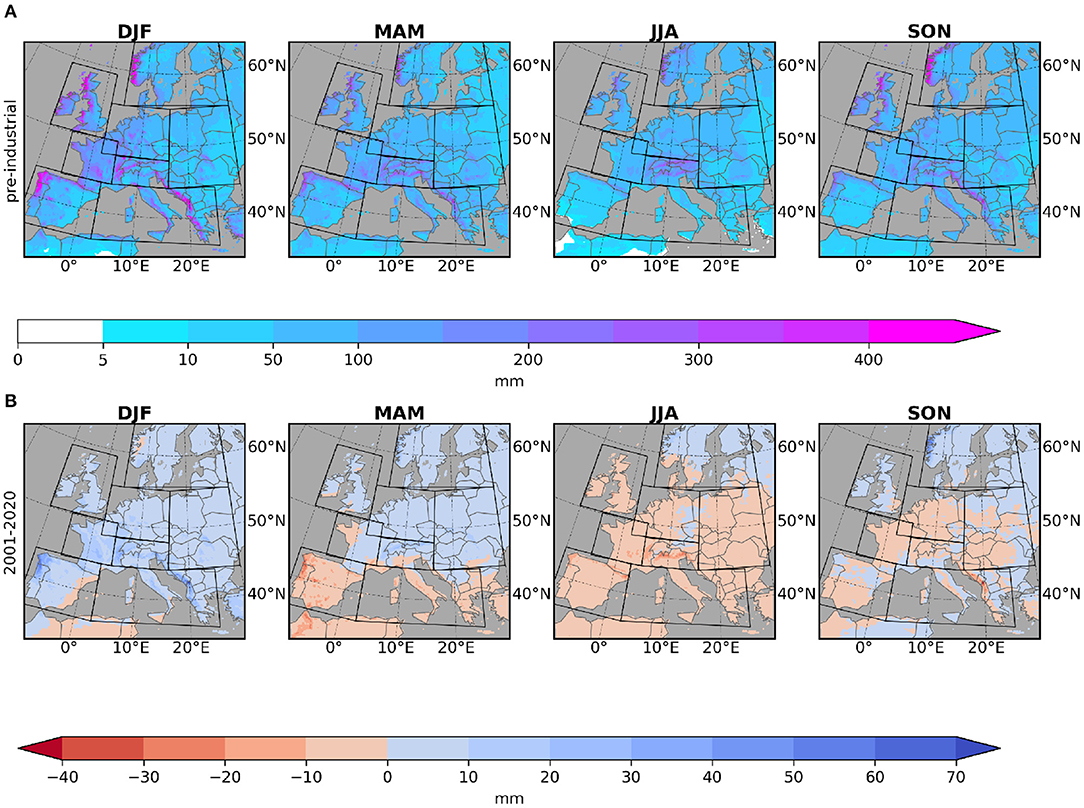
Figure 1. (A) Averaged absolute precipitation values [mm] of the pi-control runs, which are used as reference for the PNI calculation. Values <5 mm are plotted in white. (B) Absolute deviation [mm] of the 2001–2020 ensemble mean from the pre-industrial values in (A) with a general trend toward wetter winters and drier summers. The categorized colorbar shows values from the 1st to the 99th percentile.
2.4. Region-Specific Analysis
For region-specific analysis the European domain is divided into eight regions with different climate characteristics: the British Isles, Scandinavia, Mid-Europe, the Alps, Eastern Europe, France, the Mediterranean and the Iberian Peninsula (see Figure 2). The regions are based on the defined regions of the PRUDENCE project (Christensen and Christensen, 2007), but clipped to the smaller ClimEx-domain as previously done in von Trentini et al. (2019). If not stated otherwise, only land surface pixels within these regions are taken into account in order to avoid that drought conditions over landmasses are weighted out by precipitation over sea pixels for maritime regions. In order to summarize the state of drought over the regions, the pixel-based PNI values are summarized into the category, whose PNI threshold is fulfilled by at least 60% of the pixel. For example, if 60% of the pixel in the Iberian Peninsula have PNI values ≤ 55%, then the region is classified to the severe drought category. The threshold of 60% is derived from Stefanon et al. (2012).
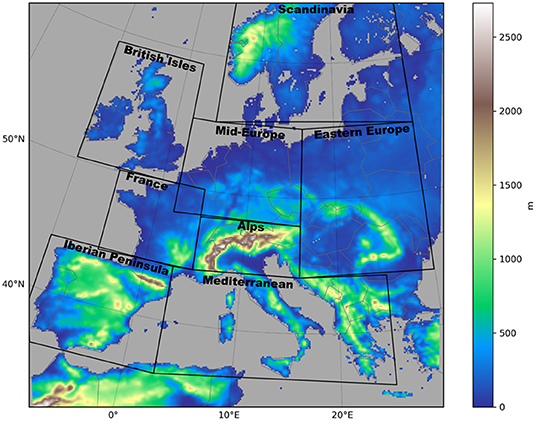
Figure 2. ClimEx-regions and the orography [m] over the European domain of the CRCM5-LE in 0.11° resolution.
2.5. Signal Maps
Areas of particularly robust and strong changes in a quantity may be efficiently visualized using the signal maps approach by Pfeifer et al. (2015): these maps compile the information of ensembles by using the local ensemble mean change signal with respect to a reference period under the condition that a pre-defined percentage of ensemble members agrees on change sign and shows a robust signal, which is obtained by statistically testing the significance of local changes. Since the PNI by construction is an index of change, we adjust this method in the following way: rather than analyzing the difference of our quantity between a future and the reference period, we use the average drought categories of the PNI in the future period, if more than 66% of members agree on the sign, i.e., PNI <100 or PNI > 100, and show a category equal to or stronger than the indicated one. Furthermore, we assume a particularly robust signal if more than 90% of members agree on a given category instead of using a statistical test. This map type allows to identify distinct regions of strong changes at a glance. The PNI used for this type of analysis is calculated seasonally (DJF, MAM, JJA, SON), i.e., seasonal precipitation sums with respect to seasonal climatological means, and for each pixel individually.
3. Results
3.1. PNI Evaluation
We first assess PNI internal variability in the CRCM5-LE using an approach that is described in Suarez-Gutierrez et al. (2021) and Wood et al. (2021): underestimation of ensemble PNI variability is identified if observed PNI values exceed the ensemble 12.5–87.5th percentile range (i.e., the central 75% of the distribution) in more than 80% of the analyzed years. Additionally, the amount of observed PNI values falling outside the ensemble range, thus being more extreme than covered by the ensemble, is calculated. To this end, seasonal PNI values are calculated for 1955–2019 with the E-OBS gridded dataset (daily precipitation sums of E-OBS variable RR, version 22.0e at 0.1° spatial resolution, regridding to CRCM5 grid followed by drizzle removal; Haylock et al., 2008; Cornes et al., 2018) and the CRCM5-LE with respect to 1955–1974. The comparison of CRCM5-LE and E-OBS climatological means reveals a wet bias in all seasons (strongest in winter) and regions (especially in mountainous regions, where winters are also subject to a warm bias) which is documented in Supplementary Figure 3 (for a single member and another time period see Leduc et al., 2019). In most of the regions and seasons, however, E-OBS PNI variability is fully covered by the CRCM5-LE as can be seen in Figure 3. The central 75% of the ensemble encompass between 60% (e.g., British Isles MAM and SON) and 90% (e.g., Eastern Europe JJA) of all E-OBS years. For this figure, the seasonal PNI value was aggregated over all land pixels of the regions to derive time series showing trends and variability of the PNI. Single E-OBS years outside the ensemble range in a given year are to be expected, so the percentage numbers Figures 3A-C,I refer to years exceeding the total minimum/maximum range of the ensemble during 1955–2019. Only the British Isles show a slight underestimation of the variability according to the total ensemble range of CRCM5-LE. These time series also give a good indication of the amount of inter-annual variability that is to be expected within the depicted regions: whereas for the Alps and British Isles PNI variability in all seasons is low (PNI values of 50–150% during most seasons), the Iberian Peninsula is affected by large PNI variability, ranging from almost 0 to >200%. This is mostly tied to the low absolute precipitation values in the latter case. The four regions in Figure 3 all show very weak trends (e.g., Iberian Peninsula and Alps in JJA), if any, which are masked by the large variability, during the period under consideration. Whilst PNI values of Figure 3 are not directly comparable to subsequent Figure 4 due to different reference periods and seasonal aggregation, a division into a period of little trends (before the 2001–2020 period) and a period with strong trends (after the 2001–2020 period) becomes apparent. A corresponding figure with the four missing regions can be found in the Supplementary Figure 4.
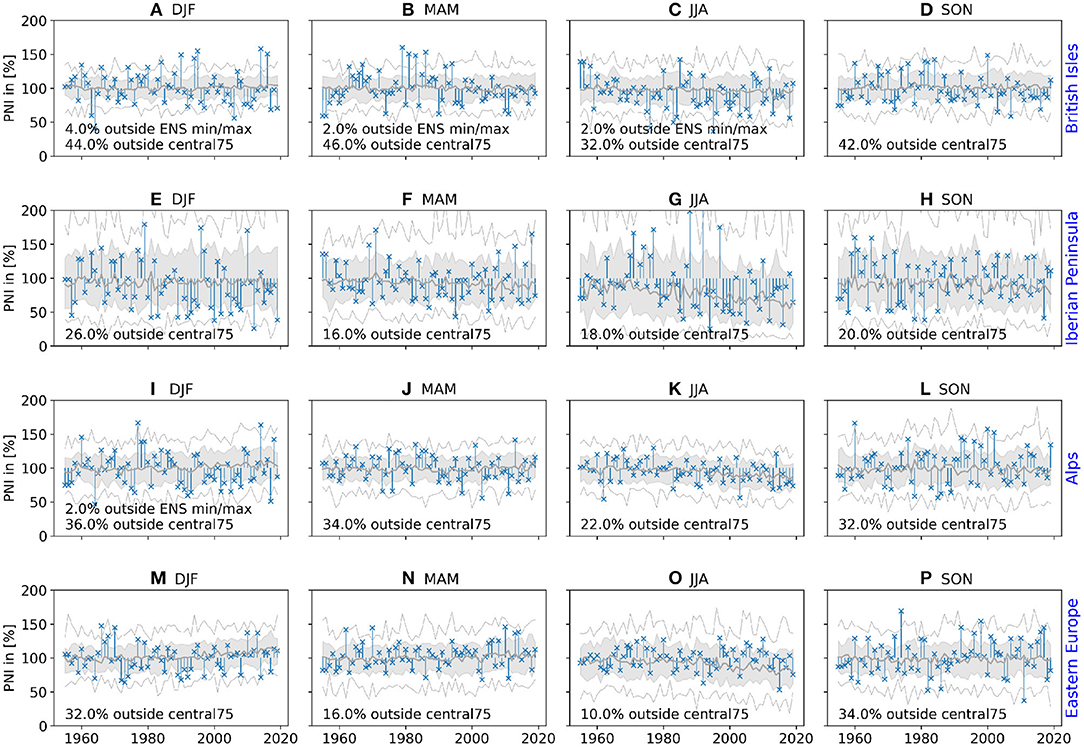
Figure 3. Evaluation of CRCM5-LE with E-OBS for seasonal PNI time series in four regions [(A–D) British Isles, (E–H) Iberian Peninsula, (I–L) Alps, (M–P) Eastern Europe] during the period 1955–2019 with PNI reference period 1955–1974. Blue bars and crosses represent E-OBS time series; gray thin lines: annual ensemble maximum and minimum range, gray shading: central 75% of ensemble; gray thick line: ensemble median. Text insertions: first row if applicable percentage of E-OBS years falling outside the total 1955–2019 minimum/maximum ensemble (ENS) range, second row percentage of E-OBS years falling outside the central 75% range of the CRCM5-LE.
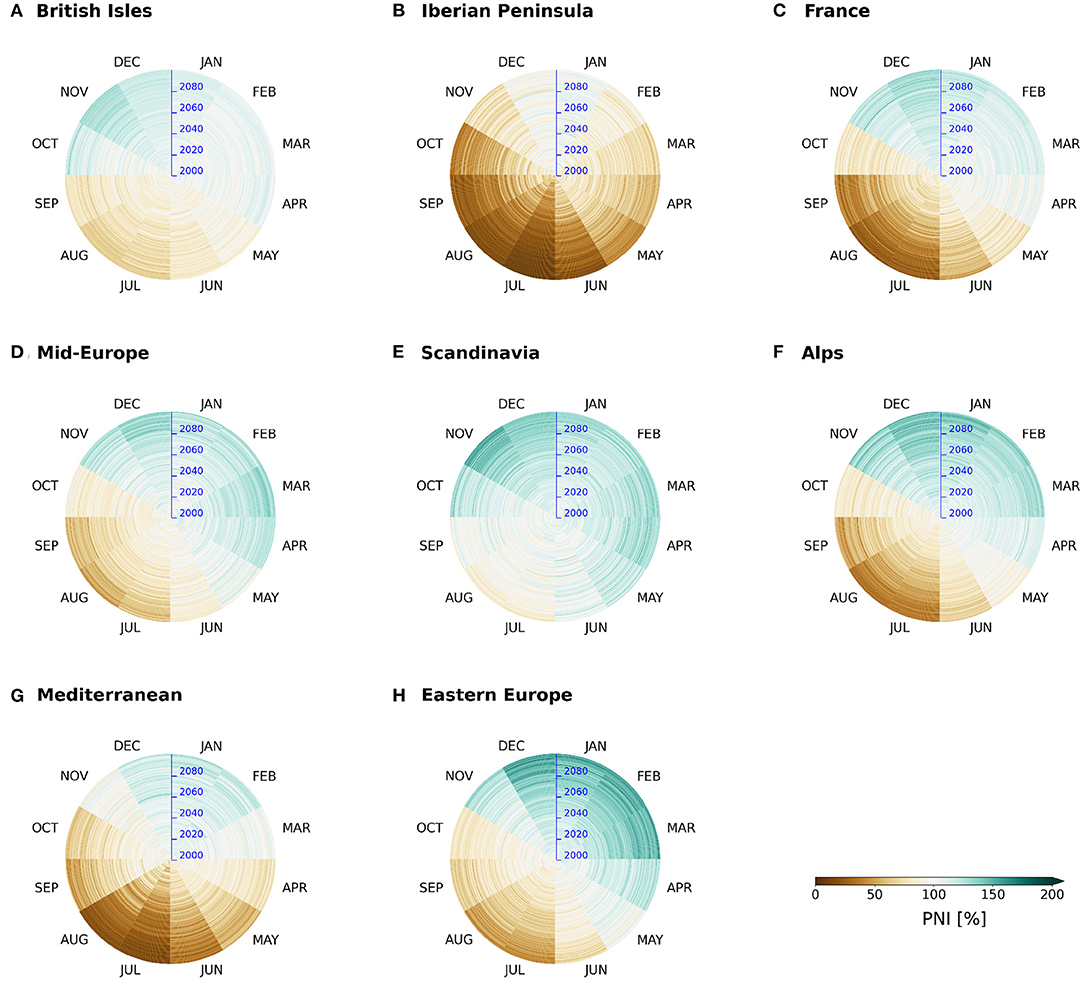
Figure 4. Ensemble medians of monthly PNI values from 2000 to 2099 averaged over the ClimEx regions [(A–H) drying stripes]. The blue axis corresponds to a time axis, starting at 2000 in the center and extending to 2099 at the outer limit of the discs. Monthly labels are indicated clockwise.
3.2. Seasonality: Seasonal Hot Spots and Drought Categories
Inspired by the warming stripes by Hawkins (2018), Figure 4 provides an overview of regionally averaged ensemble median monthly PNI values. Each ring of these drying stripes represents a single year, starting at the center of the circles in the year 2000. Green colors correspond to a PNI>100%, i.e., wetter conditions than normal, whereas brown colors symbolize a drying trend (PNI <100%). Using the ensemble median allows to picture the long-term trend; internal variability, e.g., wetter years during drier conditions, is nevertheless visible during the shown period of 2000–2099. This figure also highlights months with particularly strong PNI reductions (e.g., summer months in Figures 4B,C,F–G) and PNI increases (e.g., winter months in Figures 4F,H). Note that the PNI trends in the later years tend to be stronger than in the earlier years. This is especially visible in Figures 4B,C,F–G for summer months and Figure 4H for winter months. Most regions experience a precipitation decrease during summer months and an increase during the winter months of differing intensity (weak in the British Isles region, strong in Eastern Europe, with a general tendency for a stronger divergence between summer and winter toward the eastern regions, i.e., with increasing continentality). The largest exceptions can be found in the Iberian Peninsula (general decrease) and in Scandinavia (general increase).
In the following, analyses focus on distinct drought categories (see Table 1) to better assess drought severity. The Figures 5, 6 show spiderplots for four regions each, which illustrate the seasonality of each drought category (slight, moderate, severe, and extreme). Two spidernets are plotted for the two periods: present-day from 2001 to 2020 (blue) and the far future 2080–2099 (orange). The line of the spidernet illustrates the percentage of years in the ensemble and period that belongs to this drought category in the particular month. One hundred percent represent 1,000 years (50 members × 20 years). A blue value of 10% in August in the upper left spiderplot for example means that under the present-day climate in the British Isles 10% of all months August in the ensemble belong to the category of a slight drought. Keep in mind, that the drought categories are defined based on pre-industrial precipitation sums. For the British Isles and Scandinavia, the percentages of droughts are low (<20%) even in summer in the far future (see Figure 5). In the British Isles moderate and severe droughts are becoming more frequent by up to 10% between far future and present-day. In Mid-Europe the occurrence probability of an extreme drought strongly increases in the far future amounting to 25% in August. In the Alps (see Figure 5) and in Eastern Europe (see Figure 6) severe as well as extreme droughts have higher probabilities in the far future with values around 20% (severe), respectively 40% (extreme) in July and August. In France (see Figure 6), on the contrary, there is hardly a growth in the number of severe droughts in summer and even a decrease for the moderate drought category. This is due to the strong increase of extreme droughts, because summer droughts in the far future seem to fall in the category of highest severity most of the time. The percentages range up to >60% in July and August. In spring and fall, however, moderate and severe droughts are increasing, although only slightly. This effect is even stronger for the Mediterranean region and the Iberian Peninsula. In the Mediterranean, the percentages of extreme droughts in the far future reach around 80% in July and August. The percentage of severe droughts is around 15% in May, June, September and October. In the Iberian Peninsula the percentage of extreme droughts in the far future is the highest of all regions, reaching 96% in July. In August the value is 88 and 76% in June and September. However, it is important to keep in mind that the absolute precipitation in the Iberian Peninsula in summer is very low and contributes only to 2% of the yearly precipitation in July and August (see Figure 8B). Considering this, the high value in June is even more remarkable as it contributes about 6% to the annual precipitation in present-days. Overall, the results regarding the PNI values during drought events reveal an increase in the intensity of summer droughts in the far future in all regions. For the winter months, a considerable decrease in slight and moderate droughts is shown for Scandinavia and Mid-Europe and less intense for several further regions e.g., the Alps, Eastern Europe, France and the Mediterranean between the far future and present-day.
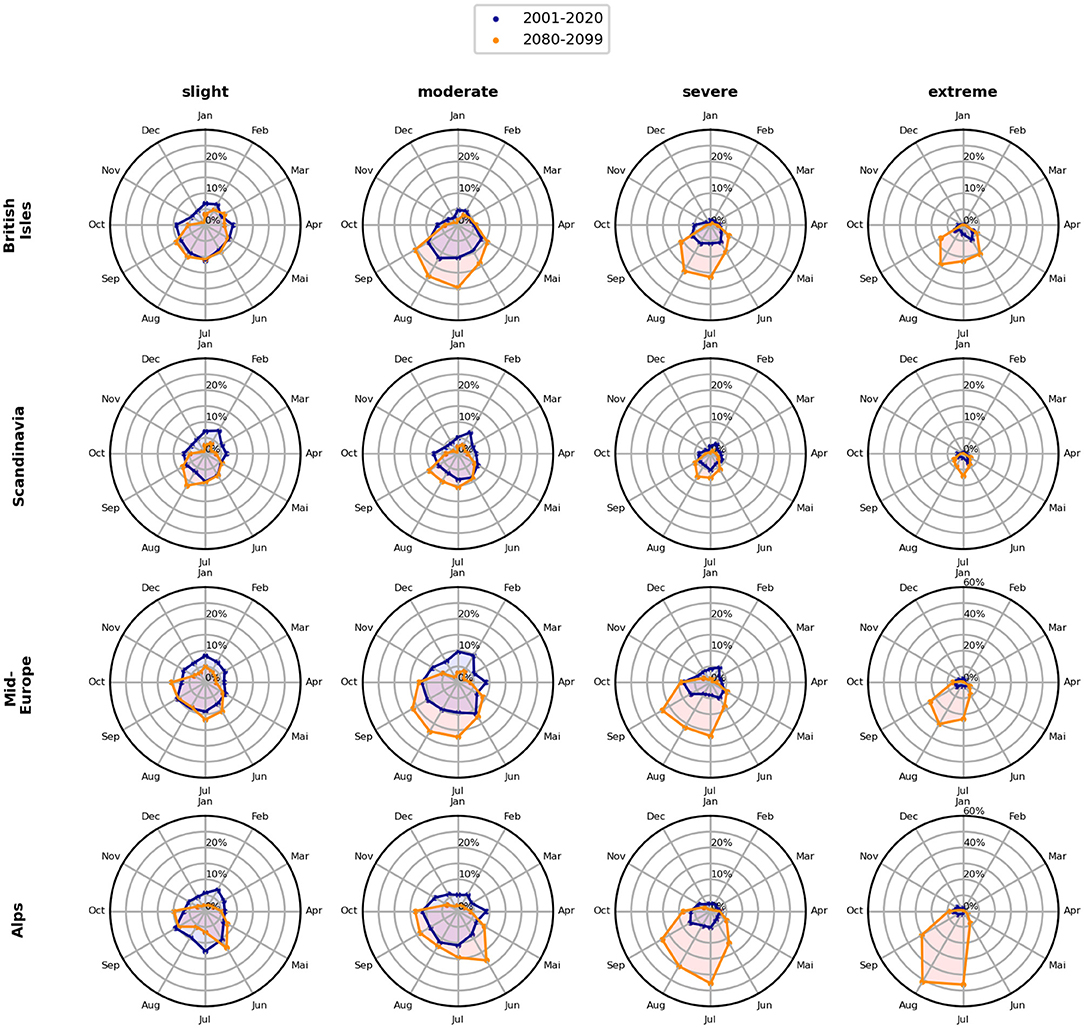
Figure 5. Spiderplots showing the percentage of months in the CRCM5-LE that belong to the specific drought category (columns: slight, moderate, severe, extreme) for the two periods: present-day (2001–2020; blue) and far future (2080–2099; orange) for each of the four regions: British Isles, Scandinavia, Mid-Europe and Alps. One hundred percent stands for 1,000 values (20 years × 50 members). The range of the y-axis in the fourth column differs between the regions (ascending order).
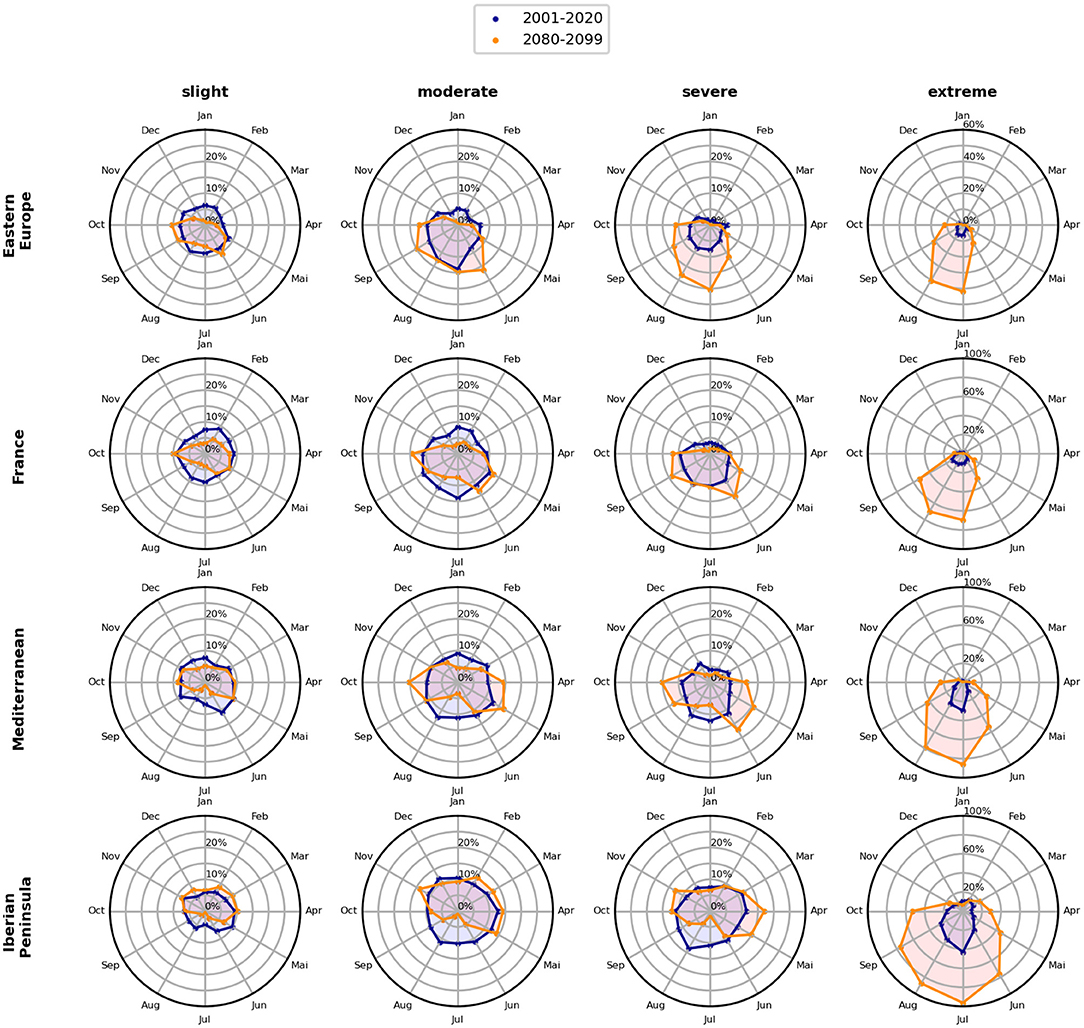
Figure 6. Same as in Figure 5 but for the four regions: Eastern Europe, France, Mediterranean and Iberian Peninsula. The range of the y-axis in the fourth column differs between the regions (ascending order).
3.3. Seasonality of the Spatial Distribution
The seasonally varying spatial distribution of drought categories is visualized in Figure 7. These maps are adjusted versions of signal maps described by Pfeifer et al. (2015). Compared to the pre-industrial reference, most parts of Europe show a reduced PNI, with the largest reduction occurring in JJA in Southern Europe. For example, the mean precipitation state during 2080–2099 over the Iberian Peninsula is robustly classified as an extreme drought compared to the pre-industrial reference. Central Europe also shows slight to moderate drought conditions with high agreement among all ensemble members, equaling 55–80% of the reference summer precipitation (see hatching in Figure 7). During the winter months, the largest part of Europe is classified as non drought, apart from single regions in Northern Africa, parts of the Mediterranean coast and a small region in Norway with a weak PNI reduction. Spring sees a wide extension of slight to severe droughts in the southwestern regions of the domain, whereas additionally in SON mountainous regions are affected by a weak PNI reduction. Since colored and hatched regions show generally a strong correspondence (apart from JJA eastern regions and SON), this seasonal derivation of local hot spots may be seen as highly robust. A similar map showing the very weak changes between the pre-industrial period and 2001–2020 is provided in the Supplementary Figure 5. We address the influence of low absolute precipitation sums, especially in the southern regions, in the subsequent sections.
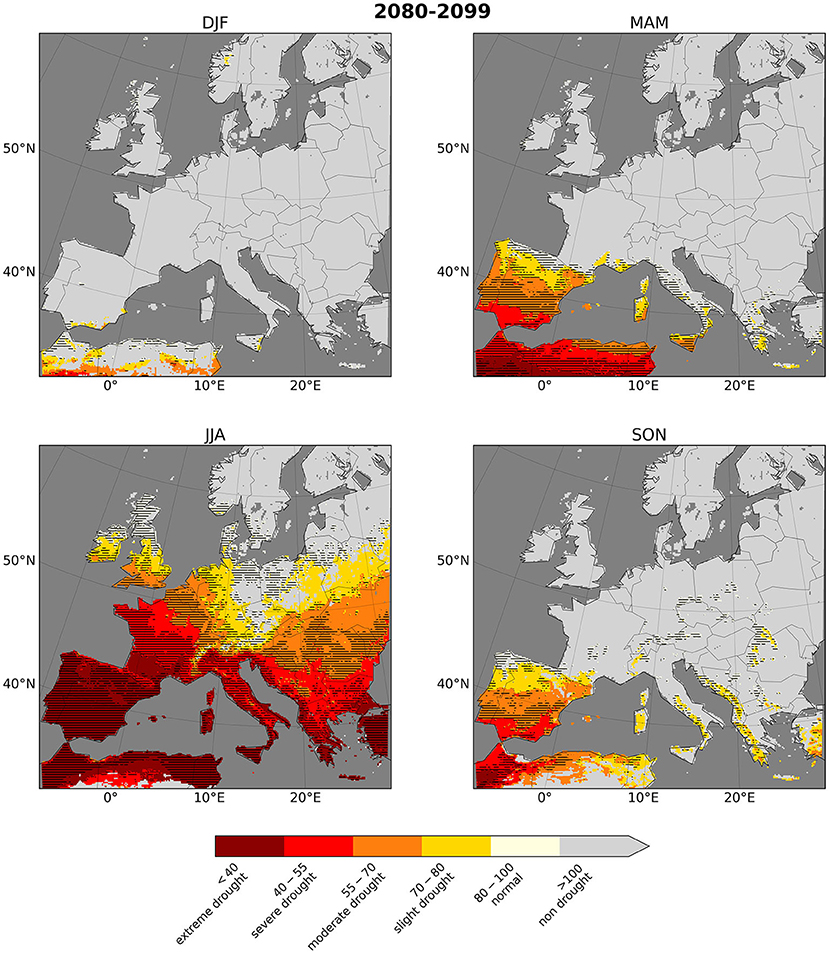
Figure 7. Seasonal signal maps for the period 2080–2099 with respect to the pre-industrial period. Colors show where at least 66% of all members agree on the indicated PNI category (or higher severity), hatching indicates an agreement of more than 90% of members.
3.4. Drought Intensities
Figure 8 shows four selected regions: the Alps with its high altitude climate, the Iberian Peninsula with the most pronounced trend toward extreme droughts, Eastern Europe with a continental climate and the British Isles with strong maritime influences. The remaining regions are shown in the Supplementary Figure 6. The analysis in Figure 8 looks in more detail at the drought months (at least 60% of the regions' pixel have PNI values <80%). For all drought months, the mean PNI value of the drought pixels (PNI value <80%) is calculated. This gives insights about the severity of the drought event, directly expressed as percentage of precipitation relative to the pre-industrial normal condition. The boxplots illustrate the distribution of the mean PNI values of all drought months in the specific period in the CRCM5-ensemble. The highest theoretically possible sample size for a boxplot is 1,000 (20 years × 50 members). The barplots below the boxplots delineate the percentage that each month contributes to the yearly precipitation sum in 2001–2020. This information is crucial for months that have low monthly precipitation sums and a small share of the yearly precipitation. A low PNI value, which represents a major relative change, is in such cases triggered by only a minor reduction in the monthly precipitation sum. The actual sample size closest to the theoretical maximum is 940 and occurs in the far future over the Iberian Peninsula in July (see Figure 8B). This means that in the far future over the Iberian Peninsula only 60 out of 1,000 years in the ensemble have a July that does not show a drought condition. In other words, 94% of all future months July have a total precipitation <80% of what used to be normal in pre-industrial times. The median PNI value of those drought events amounts to 7.6%, which means that 50% of the distribution have even lower PNI values. However, the total precipitation sums of the summer months in the Iberian Peninsula are low and contribute in the case of July only 2% to the yearly precipitation sum (see Figure 8B). All four regions show the tendency of lower PNI values during droughts in summer than in winter. This is the case in the present-day period and the far future and particularly strong in the Iberian Peninsula. In the far future this effect amplifies in all four regions. The internal variability of PNI values of droughts is large, which is shown in a large boxplot spread. For example in the far future in the British Isles (see Figure 8D) the mean PNI value of drought pixels of a May under drought condition ranges from almost 70% to about 10 %. In the Alps the decrease of the median of the far-future PNI values compared to present-day is almost 15% in July and August and thus particularly strong. The spread is large and covers PNI values from 59 to 2%. Such an extremely low PNI value denotes a tremendous precipitation deficit as July and August hold about 7.6 and 7.3% of the yearly share.
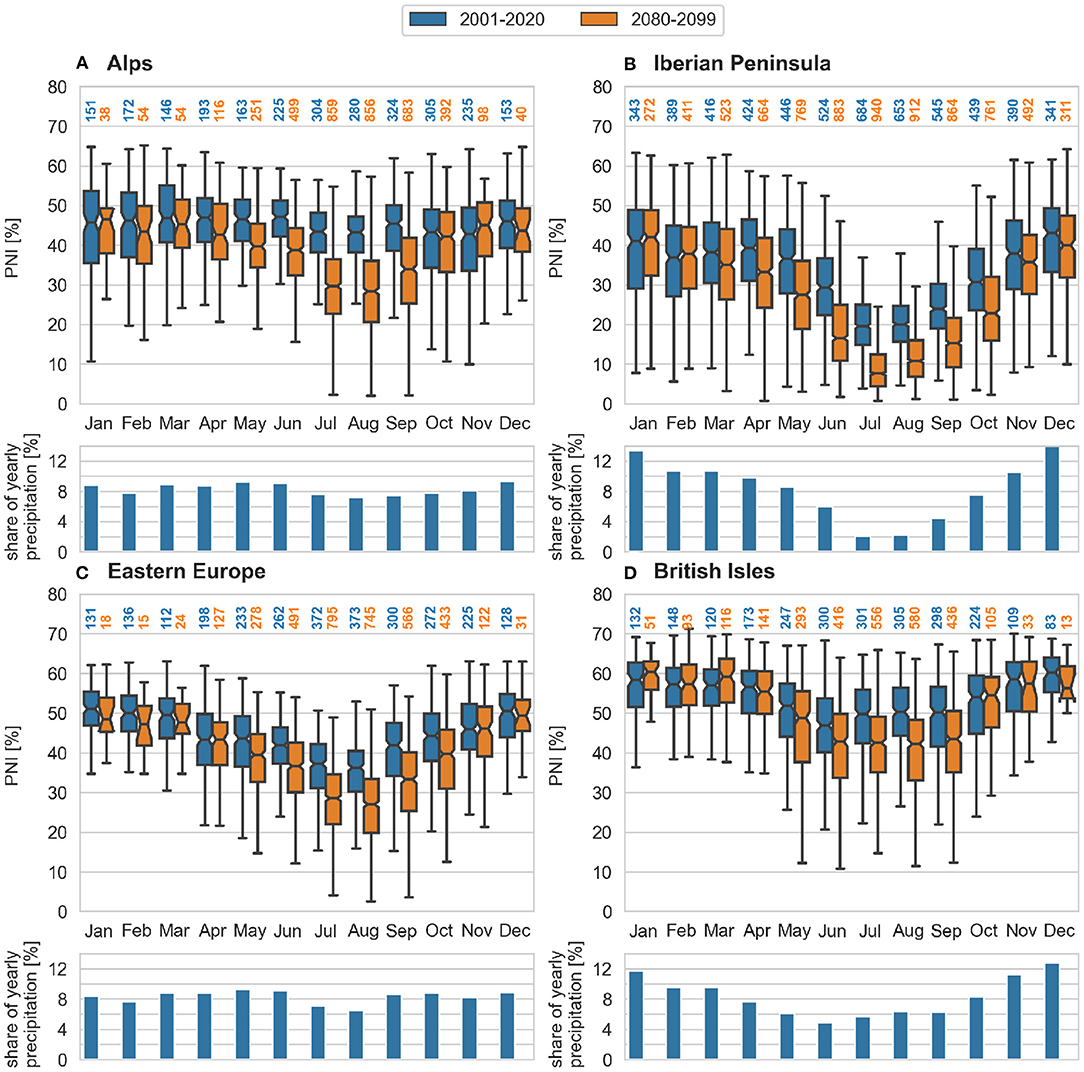
Figure 8. Boxplots (upper part) and barplots (lower part) for the four regions: Alps (A), Iberian Peninsula (B), Eastern Europe (C), and British Isles (D). The boxplots show the average PNI values of drought pixels (PNI <80%) of all drought months (≥60% of the regions' pixel have PNI values <80) in the 50 members of the CRCM5-ensemble and the 20-year periods present-day (2001–2020; blue) and far future (2080–2099; orange). Colored values above the boxplots state the sample size of the underlying distribution, which consists of the drought months of the specific period in the ensemble (maximum possible value: 1,000). The barplots (lower part) illustrate the share of yearly precipitation of each month in the present-day climate (2001–2020; blue).
3.5. Drought Length and Frequency
Since the harmful potential of droughts is connected to their duration, we next investigate the length of events due to climate change. Therefore, only drought events with a minimum length of three consecutive months are considered. We choose this minimum length since it equals the length of the previously analyzed seasons. Figure 9 presents the frequency of occurrences (Figures 9A–D) and the length (Figures 9E–H) of these continuous drought events for four regions. Insights on the remaining four regions are provided in the Supplementary Figure 7. The categories refer to the least severe category within a continuous drought event, e.g., a continuous severe drought event may contain drought months of the category extreme and severe, but not moderate. This is why frequency and length are generally higher for the low-severity categories. In general, all regions experience an increase in frequency and length of these continuous drought events across all categories. This increase is considerably stronger between 2080–2099 and 2001–2020 than between 2001 and 2020 and the pre-industrial period. This finding is supported by the fact that most frequency boxplots in the far future are significantly different from the pre-industrial boxplots, whereas the present-day boxplots are not (black triangles in Figure 9). The simultaneous increase of event frequency and length suggests, e.g., over the Iberian Peninsula (Figures 9B,F), that shorter events do not simply merge to longer events. The median shows 2–5 times more frequent slight/moderate drought events in the far future horizon, which results on average in 1 event in 2 years over the British Isles, yearly events in the Alps and Eastern Europe and more than 1 event per year over the Iberian Peninsula. Due to internal variability, however, the frequency may even be higher (or lower). The Iberian Peninsula also experiences yearly events of extreme droughts longer than 3 months which most likely occur during the summer months (see Figure 7). In Eastern Europe and the Alps, extreme droughts are projected to occur in 2–4 years out of 20 (Figures 9C,D), whereas during the pre-industrial and present period they only occurred occasionally. Drought length reaches more than 6 months in all regions except the British Isles for all drought categories with an absolute maximum over the Iberian Peninsula with up to 1 year of continuous extreme drought and around 2 years of continuous drought with at least slight severity. In this region, boxplots become very similar during the far future period, indicating that especially extreme droughts increase in frequency and length. Ensemble ranges show that on the one hand the projected frequencies are quite robust (short boxes and whiskers in the 2080–2099 horizon for most cases in Figures 9A–D). On the other hand, the probability of longer drought event duration increases with wider distributions (larger boxes during 2080–2099 compared to pre-industrial and 2001–2020 in Figures 9E–H). Changes in these properties from pre-industrial to 2080–2099 are reflected by the black triangles, indicating the rejection of a null-hypothesis on same distributions (p ≤ 0.05, using a Kolmogorov-Smirnov test).
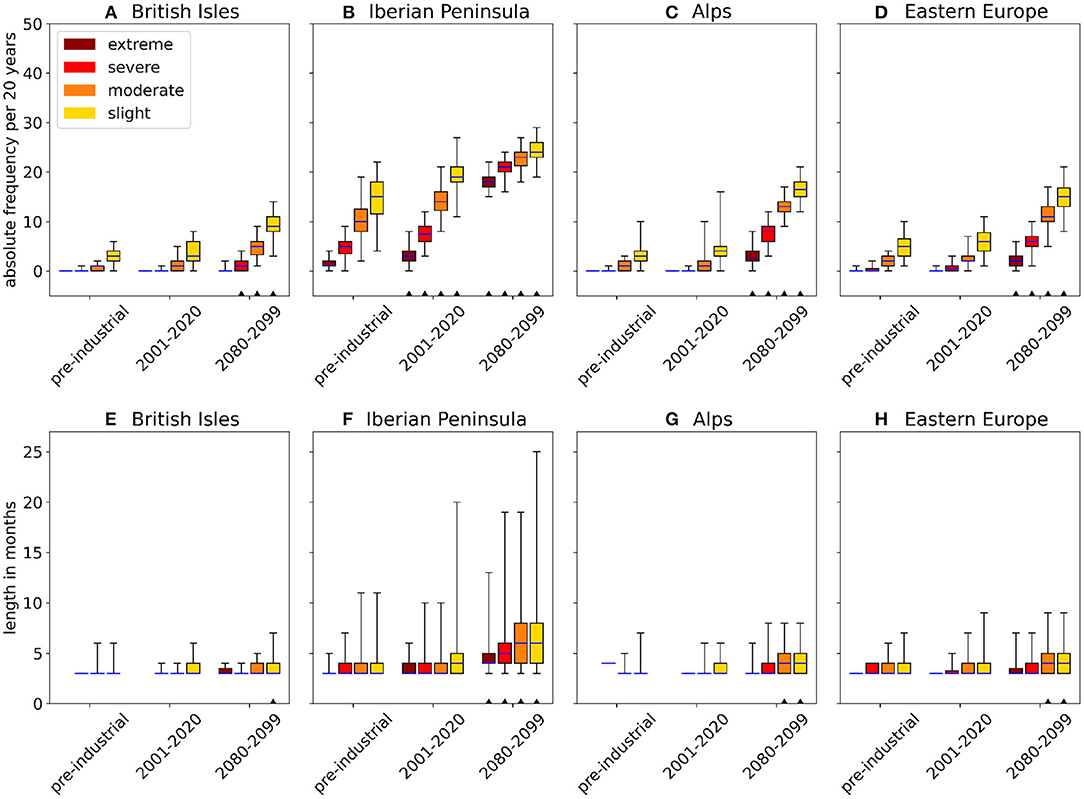
Figure 9. Frequency (A–D) and length (E–H) of consecutive (minimum of 3 months) drought events (definition see Figure 8) within three 20-year periods (pre-industrial period, 2001–2020 and 2080–2099) across all seasons and all 50 members of the CRCM5-LE (35 members for pre-industrial) for four regions (British Isles, Iberian Peninsula, Alps, Eastern Europe). Frequency boxplots consist of one value per member, whereas length boxplots consist of all drought events ≥3 months. Colors indicate the drought category, boxes span the interquartile range, whiskers extend to minimum/maximum of the ensemble, blue lines represent the median. Black triangles mark boxplots which are significantly different (p ≤ 0.05 using a ks-test) from the corresponding pre-industrial samples.
3.6. Compensation of Drought Seasons
As Figure 9 indicates, e.g., for the Iberian Peninsula region, some drought events may extend over 1–2 years, thereby affecting usually drier and wetter seasons. It is highly relevant to ask whether drought events of one season may be—in principle—compensated by subsequent wetter seasons. This is not meant to be a quantitative water budget analysis, but rather an estimate of the general tendency since e.g., evapotranspiration increases due to rising temperature may by larger than potential projected precipitation gains (see e.g., Spinoni et al., 2020). Specifically, what is the probability of having a wet winter (summer), given a precedent drought summer (winter)? Winters and summers were chosen since these seasons tend to show the highest share of annual precipitation and the strongest PNI reductions, respectively (Figure 8). Table 2 provides the corresponding values for the pre-industrial, present day and far future periods. A table containing a similar analysis with respect to fall and spring is provided in the Supplementary Table 1. A season is defined as drought if at least 60% of the land pixels within a region show PNI <80%. The PNI for the next but one season is spatially averaged over the same pixels. This subsequent season is considered as drought as well if PNI <80%. Since dry conditions of the PNI are defined as more than 20% less precipitation than normal conditions (Table 1), we also decide to use a threshold of more than 20% higher than normal conditions for wet seasons, i.e., PNI > 120%. We first consider the probability of having a drought in a given summer (or winter): What is the probability that the following winter (summer) will be wet (upper half of Table 2)? The first notion is a general increase in the case of wet winters following dry summers with a maximum value of 91% during 2080–2099 in Eastern Europe and a minimum of 27% over the Iberian Peninsula and the British Isles. The Iberian Peninsula is the only region, in which the percentage of summer droughts followed by wet winters is not increasing in the far future, but is slightly lower than in the present-day climate. In all other regions the trend points clearly toward an increasing percentage of summer droughts that are compensated by higher than normal precipitation in winter. This mirrors the general tendency for wetter winters and drier summers with a larger seasonal gradient in the more southeastern regions as mentioned previously (e.g., Figure 4). Wet summers following a winter drought, occurring at a similar probability to the inverse combination during pre-industrial times for most regions, disappear for all but one region in the far future horizon. So if a given winter is classified as a drought, the next summer will not show wet conditions. The lower half of Table 2 provides the absolute numbers of drought events within the ensemble per period to put the conditional probabilities in perspective. JJA droughts become more frequent in all regions whereas the projected increase between the 80 years between far future and present day is remarkably stronger than between present day and pre-industrial times. At the same time, DJF droughts show a general decrease between pre-industrial times and far future with a peak during the present time in some regions. With more summer droughts and less winter droughts, the decrease in wet summers following a winter drought seems reasonable. The increase in wet winters following a summer drought points toward an increasing number of wet winters at a comparable or even stronger magnitude as dry summers. So on the one hand, although in far future, a winter drought will most likely not be compensated by subsequent wet summers, the case of winter droughts becomes less frequent itself. On the other hand, inter-seasonal variability regarding precipitation tends to increase.
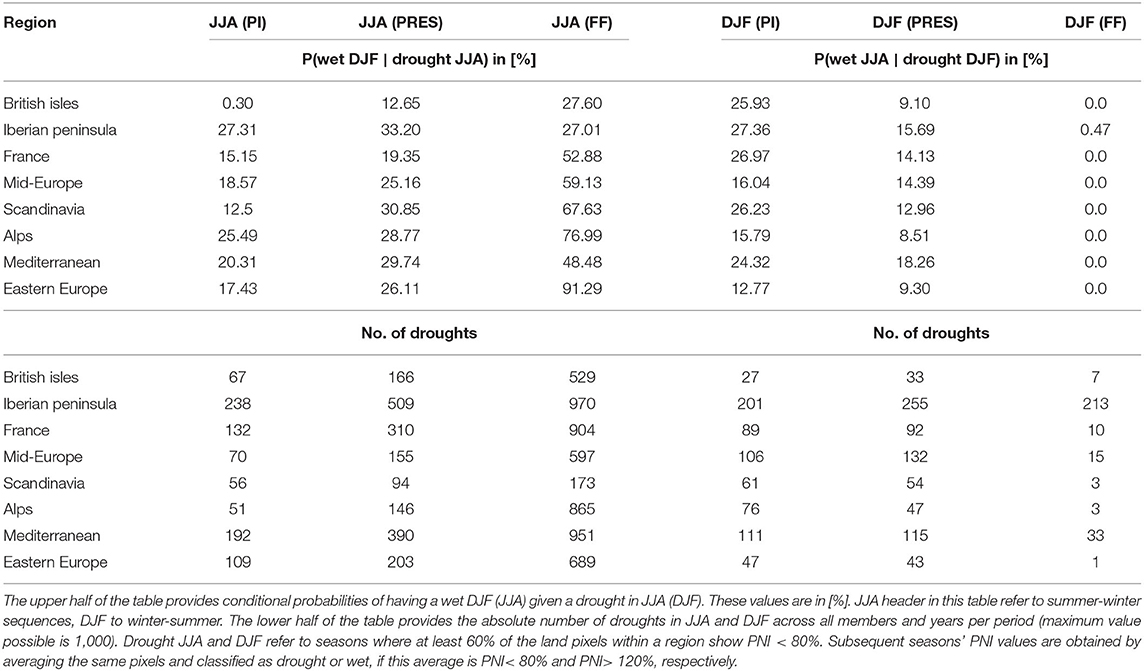
Table 2. Consecutive seasons of dry summers (winters) and wet winters (summers) in eight regions for pre-industrial (PI), present day (PRES, 2001–2020) and far future (FF, 2080–2099) horizons.
4. Discussion
4.1. Seasonal Trends
The most pronounced seasonal trends identified in this study are an increase in summer drought frequency and intensity and a decreasing number of winter droughts in several regions of various climates. This goes in line with a general tendency toward larger differences between winter and summer precipitation, with precipitation increases during winter and precipitation decreases during summer in the far future horizon. The identified decrease of winter droughts in several regions are in accordance with Spinoni et al. (2018) apart from the regions France and the Mediterranean, where these authors do not find a drought decrease in winter.
A deficit in precipitation is rated most critical for months that have a high or above-average share of the regions' annual precipitation. This is for example the case for the summer months in the Alps or spring and winter in the Iberian Peninsula. In this regard, the question if a drought season is compensated by a wetter than normal subsequent season is of high relevance, especially if the season of the drought shows a high share of annual precipitation under normal circumstances. For example in a region like the Iberian Peninsula, high absolute winter precipitation sums bear the potential to compensate precipitation deficits of dry summers by a subsequent wet winter. Wet summers, however, correspond to absolute precipitation amounts lower than the winters, such that they likely lack the potential to compensate winter droughts. In a region like the Alps, where precipitation is less concentrated in a single season, a compensation depends more strongly on the magnitude of precipitation deficit and surplus.
Wet seasons experiencing precipitation increases and dry seasons experiencing precipitation losses result in a higher inter-seasonal variability of precipitation in the far future. There is one caveat to this particular conclusion because of the wet bias in the CRCM5-LE, which is most pronounced during winter and in the mountainous regions whereas it is small during summer. However, as our evaluation shows, the ensemble reflects well observed PNI variability and minimum/maximum values such that the projected changes are considered robust and reliable.
4.2. Regional Trends
Most regions share the seasonally divergent PNI trends, with differences regarding the annual distribution. Exceptions are the Iberian Peninsula with a drying tendency throughout the year and Scandinavia with precipitation increase in all seasons but July and August at the very end of the twenty-first century. This is generally in line with the findings in Spinoni et al. (2018). Additionally, we find longer drought periods in most regions by the end of the twenty-first century. While these tend to show around 6 months in some regions, in line with the aforementioned seasonal trends of more wet winters following drought summers, hyperannual droughts are also projected to occur. This is partly tied to the overall increase of drought occurrences with respect to our reference. Long lasting meteorological droughts, however, hold the potential to turn into e.g., hydrological droughts, which have strong impacts on water supply (Van Lanen et al., 2016). Lorenzo-Lacruz et al. (2010) for example state an increasing correlation between drought indices and hydrological variables in central Spain for longer drought periods. Nevertheless, for impact assessment other indices, accounting e.g., for the effects of temperature or evapotranspiration, may be better suited, e.g., in conjunction with the use of impact models like hydrological models (Dobson et al., 2020). Lorenzo-Lacruz et al. (2010) find that the SPEI shows more severe droughts within their Spanish study area than the precipitation-only based SPI, suggesting that without temperature the (hydrological) impact of droughts may be underestimated. In line with this, Vicente-Serrano et al. (2014) show for the entire Iberian Peninsula that an increase of temperatures and therefore of evaporative demand in the atmosphere already resulted in higher (SPEI) drought intensities since 1961.
In addition to drought length, the spatial extent of droughts is of importance. Very localized droughts of high intensity may have weaker impacts than moderate droughts of larger spatial extent (Dobson et al., 2020). We address this point by using the spatial constraints (of having droughts in at least 60% of a given region) to ensure that we investigate events of large extent.
4.3. Hot Spots
The four regions selected for a detailed analysis in this study show diverging future drought trends across various climates in Europe: whereas the Alps and Eastern Europe, corresponding to high mountain and continental climate conditions, respectively, are marked by increasing differences between winter and summer precipitation, the Iberian Peninsula, located in a Mediterranean climate, sees an overall drying in all seasons and the British Isles, subject to maritime climate conditions, exhibit only slight trends. Taking all regions considered in this study into account, particularly strong changes and drought increases are found in the Alps, the Iberian Peninsula, but also France and the Mediterranean. These regions can therefore be considered as drought hot spots. All four regions show a strong decrease of summer PNI values (see Figure 4) and a severe increase in the number of extreme droughts in summer (see Figures 5, 6). The Iberian Peninsula, the Mediterranean and France are furthermore highlighted as hot spots in Figure 7 with a high agreement among the members of the CRCM5-LE. A characteristic of the Alps is the high contribution of the summer months to the yearly precipitation sum (see Figure 8), which makes summer precipitation deficits even more critical. The frequency and lengths of consecutive drought events (of at least 3 months) is increasing in all four regions (see Figure 9 and Supplementary Figure 7). The Iberian Peninsula, in contrast to the other regions, is additionally affected by a decreasing percentage of summer droughts compensated by wet winters (see Table 2) and can, inter alia, therefore be particularly postulated as a hot spot. In general, the drying trend we identified in the Iberian Peninsula goes in line with Spinoni et al. (2018), who find similarly frequent droughts using a different drought metric in the EURO-CORDEX multi-model ensemble.
4.4. Index Choice
The advantage of the PNI lies in its intuitive meaning as it directly represents the percentage of precipitation in a certain month or season compared to the long-term mean. This simplicity in turn also involves the main disadvantage of the PNI. Because no normalization is undertaken in the calculation of this index, the PNI implies that the precipitation sums follow a normal distribution (Hayes, 2002; Yihdego et al., 2019). Still, our findings on climate signals on droughts are in line with the study by Spinoni et al. (2018), who employ a normalized drought index.
In analyzing meteorological droughts based on precipitation deficit only, we investigate the framing conditions for further impact assessment, which may employ drought definitions that are specifically tailored toward a certain impact. Using a relative measure for estimating meteorological droughts reduces the impact of model bias because we also refer to model climatological references. Drought impact assessment on the other hand requires well-chosen model bias adjustment (Ruffault et al., 2014).
4.5. Perspectives Due to Pre-industrial Reference
The relative measure also allows to relate future changes with familiar conditions to which most human systems and behaviors are adapted. This we achieved by comparing a present day period with a far future period. However, we showed that for most drought characteristics this present day period is already affected by climate change. As previously discussed, this is a good reason for using a pre-industrial reference period. By doing this, we have two perspectives from the present day period: we show recent, comparably small climate changes since the pre-industrial period that are already inherent nowadays, and we can relate them with what is projected for a far future horizon. These changes regarding drought frequency and length are non-significant for the present day period, in line with findings as in Vicente-Serrano et al. (2021), where no clear trend during the historical period is found. Internal variability of drought characteristics during this period is thus too large to identify clear trends, even within a SMILE.
4.6. Internal Variability of Drought Trends
The ensemble provides two key messages: first, we see robust increases in overall drought numbers and severity over Europe using an extreme RCP8.5 emissions scenario. Second, we note a higher inter-seasonal variability of wet seasons becoming wetter, dry seasons becoming drier on the one hand, and more long lasting drought events on the other hand. The latter tend to be rare events, e.g., ≥ 12 months in Iberian Peninsula results in 1, 2, 7, and 32 events per thousand years for categories extreme, severe, moderate and slight, respectively. We even find one, albeit slight, event in thousand years of more than 2 years in length (Figure 9). The SMILE provides the means to detect these as well. The spread of drought intensities throughout the CRCM5-LE is also high and stresses the high internal variability of droughts. We also note that results concerning the most extreme drought classes tend to be more robust than less severe categories. This refers to the projected numbers of droughts as well as to the spatial location of most extreme PNI changes.
5. Conclusion
To summarize, we find an overall increase in drought numbers, and a high internal variability of drought intensities both in the present day period and the projected far future across various European climate regions. Drought lengths are projected to show higher values and variability in the future. The changes in length and frequency are significant in most regions only in the far future horizon. Additionally, summer droughts are projected to become more intense with more droughts reaching the category “extreme,” while winter droughts become less frequent in several regions. The percentage of summer droughts followed by wet winters increases in all regions, except for the Iberian Peninsula. Our results suggest the consideration of four pronounced hot spots due to especially strong drought trends: the Alps, France, the Mediterranean and the Iberian Peninsula, whereof the Iberian Peninsula is particularly affected by the drying trend toward the far future.
This study is to our knowledge the first region-specific analysis of droughts over Europe using the SMILE of a high-resolution RCM. It assesses hot spots and region-specific climate signals of droughts over Europe against the backdrop of a high internal variability in drought occurrence. Through the employment of the 50-member SMILE we assess the change in drought occurrence under the consideration of this major source of uncertainty. The choice of the simple PNI index furthermore aligns this study directly for communication and outreach purposes, especially with respect to the plot type of drying stripes.
Data Availability Statement
The datasets presented in this study can be found in online repositories. The names of the repository/repositories and accession number(s) can be found below: CRCM5-LE: https://www.climex-project.org/de/datenzugang E-OBS: https://surfobs.climate.copernicus.eu/dataaccess/access_eobs.php (07.04.2021).
Author Contributions
AB and MM contributed to the conception of the study, statistical analyses and manuscript preparation in equal parts. RL is founder and head of the ClimEx project. ML conducted the simulation of the CRCM5-LE and the pi-control runs. RL and ML monitored and supported the research process and revised the manuscript. All authors contributed to the article and approved the submitted version.
Funding
This work was conducted within the ClimEx project (Leduc et al., 2019). Funding from the Bavarian State Ministry for the Environment and Consumer Protection is gratefully acknowledged. The CRCM5 was developed by the ESCER center of UQAM (www.escer.uqam.ca) in collaboration with Environment and Climate Change Canada. We acknowledge CCCma for executing and making available the CanESM2-LE and CanSISE for proposing the simulations. Computations with the CRCM5 for the ClimEx project were made on the LRZ's SuperMUC of BAdW and funded via GCS by BMBF and StMWFK.
Conflict of Interest
The authors declare that the research was conducted in the absence of any commercial or financial relationships that could be construed as a potential conflict of interest.
Publisher's Note
All claims expressed in this article are solely those of the authors and do not necessarily represent those of their affiliated organizations, or those of the publisher, the editors and the reviewers. Any product that may be evaluated in this article, or claim that may be made by its manufacturer, is not guaranteed or endorsed by the publisher.
Acknowledgments
We would like to thank Raul Wood and Ben Poschlod for valuable input and inspiring discussions.
Supplementary Material
The Supplementary Material for this article can be found online at: https://www.frontiersin.org/articles/10.3389/frwa.2021.716621/full#supplementary-material
References
Arora, V. K., Scinocca, J., Boer, G. J., Christian, J. R., Denman, K. L., Flato, G. M., et al. (2011). Carbon emission limits required to satisfy future representative concentration pathways of greenhouse gases. Geophys. Res. Lett. 38:L05805. doi: 10.1029/2010GL046270
Basist, A., Bell, G. D., and Meentemeyer, V. (1994). Statistical relationships between topography and precipitation pattern. J. Clim. 7, 1305–1315. doi: 10.1175/1520-0442(1994)007andlt;1305:SRBTAPandgt;2.0.CO;2
Bastos, A., Fu, Z., Ciais, P., Friedlingstein, P., Sitch, S., Pongratz, J., et al. (2020). Impacts of extreme summers on European ecosystems: a comparative analysis of 2003, 2010 and 2018. Philos. Trans. R. Soc. Lond. B Biol. Sci. 375:20190507. doi: 10.1098/rstb.2019.0507
Beillouin, D., Schauberger, B., Bastos, A., Ciais, P., and Makowski, D. (2020). Impact of extreme weather conditions on European crop production in 2018. Philos. Trans. R. Soc. Lond. B Biol. Sci. 375:20190510. doi: 10.1098/rstb.2019.0510
Blenkinsop, S., and Fowler, H. (2007). Changes in European drought characteristics projected by the PRUDENCE regional climate models. Int. J. Climatol. 27, 1595–1610. doi: 10.1002/joc.1538
Bonaccorso, B., Bordi, I., Cancelliere, A., Rossi, G., and Sutera, A. (2003). Spatial variability of drought: an analysis of the SPI in Sicily. Water Resour. Manag. 17, 273–296. doi: 10.1023/A:1024716530289
Christensen, J., and Christensen, O. (2007). A summary of the PRUDENCE model projections of changes in European climate by the end of this century. Clim. Change 81, 7–30. doi: 10.1007/s10584-006-9210-7
Cook, B., Mankin, J., Marvel, K., Williams, A., Smerdon, J., and Anchukaitis, K. (2020). Twenty-first century drought projections in the CMIP6 forcing scenarios. Earths Future 8:e2019EF001461. doi: 10.1029/2019EF001461
Cook, B. I., Anchukaitis, K. J., Touchan, R., Meko, D. M., and Cook, E. R. (2016). Spatiotemporal drought variability in the Mediterranean over the last 900 years. J. Geophys. Res. Atmospheres 121, 2060–2074. doi: 10.1002/2015JD023929
Cornes, R. C., van der Schrier, G., van den Besselaar, E. J. M., and Jones, P. D. (2018). An ensemble version of the E-OBS temperature and precipitation data sets. J. Geophys. Res. Atmospheres 123, 9391–9409. doi: 10.1029/2017JD028200
Dai, A., and Zhao, T. (2017). Uncertainties in historical changes and future projections of drought. Part I: Estimates of historical drought changes. Clim. Change 144, 519–533. doi: 10.1007/s10584-016-1705-2
Dobson, B., Coxon, G., Freer, J., Gavin, H., Mortazavi-Naeini, M., and Hall, J. W. (2020). The spatial dynamics of droughts and water scarcity in england and wales. Water Resour. Res. 56:e2020WR027187. doi: 10.1029/2020WR027187
Falzoi, S., Gleeson, E., Lambkin, K., Zimmermann, J., Marwaha, R., O'Hara, R., et al. (2019). Analysis of the severe drought in Ireland in 2018. Weather 74, 368–373. doi: 10.1002/wea.3587
Fyfe, J. C., Derksen, C., Mudryk, L., Flato, G. M., Santer, B. D., Swart, N. C., et al. (2017). Large near-term projected snowpack loss over the western United States. Nat Commun. 8:14996. doi: 10.1038/ncomms14996
Hänsel, S., Ustrnul, Z., Łupikasza, E., and Skalak, P. (2019). Assessing seasonal drought variations and trends over Central Europe. Adv. Water Resour. 127, 53–75. doi: 10.1016/j.advwatres.2019.03.005
Hawkins, E. (2018). Warming Stripes. Available online at: http://www.climate-lab-book.ac.uk/2018/warming-stripes/ (accessed May 8, 2021).
Hawkins, E., and Sutton, R. (2011). The potential to narrow uncertainty in projections of regional precipitation change. Clim. Dyn. 37, 407–418. doi: 10.1007/s00382-010-0810-6
Haylock, M. R., Hofstra, N., Klein Tank, A. M. G., Klok, E. J., Jones, P. D., and New, M. (2008). A European daily high-resolution gridded data set of surface temperature and precipitation for 1950–2006. J. Geophys. Res. Atmospheres 113:D20. doi: 10.1029/2008JD010201
IPCC (2013). “Anthropogenic and natural radiative forcing,” in Climate Change 2013: The Physical Science Basis. Contribution of Working Group I to the Fifth Assessment Report of the Intergovernmental Panel on Climate Change, eds T. Stocker, D. Qin, G. -K. Plattner, M. Tignor, S. Allen, J. Boschung, A. Nauels, Y. V. B. Xia, and P. Midgley (Cambridge: Cambridge University Press), 659–740.
IPCC (2018). “Summary for policymakers,” in Global Warming of 1.5°C. An IPCC Special Report on the Impacts of Global Warming of 1.5°C Above Pre-Industrial Levels and Related Global Greenhouse Gas Emission Pathways, in the Context of Strengthening the Global Response to the Threat of Climate Change, Sustainable Development, and Efforts to Eradicate Poverty, eds V. Masson-Delmotte, P. Zhai, H. -O. Pörtner, D. Roberts, J. Skea, P. Shukla, A. Pirani, W. Moufouma-Okia, C. Péan, R. Pidcock, S. Connors, J. Matthews, Y. Chen, X. Zhou, M. Gomis, E. Lonnoy, T. Maycock, M. Tignor, and T. Waterfield Geneva: (World Meteorological Organization), 32.
Kay, J. E., Deser, C., Phillips, A., Mai, A., Hannay, C., Strand, G., et al. (2015). The community earth system model (CESM) large ensemble project: a community resource for studying climate change in the presence of internal climate variability. Bull. Am. Meteorol. Soc. 96, 1333–1349. doi: 10.1175/BAMS-D-13-00255.1
Kjellström, E., Boberg, F., Castro, M., Christensen, J. H., Nikulin, G., and Sánchez, E. (2010). Daily and monthly temperature and precipitation statistics as performance indicators for regional climate models. Clim. Res. 44, 135–150. doi: 10.3354/cr00932
Kjellström, E., Nikulin, G., Strandberg, G., Christensen, O. B., Jacob, D., Keuler, K., et al. (2018). European climate change at global mean temperature increases of 1.5 and 2 C above pre-industrial conditions as simulated by the EURO-CORDEX regional climate models. Earth Syst. Dyn. 9, 459–478. doi: 10.5194/esd-9-459-2018
Leduc, M., Mailhot, A., Frigon, A., Martel, J.-L., Ludwig, R., Brietzke, G. B., et al. (2019). The climex project: a 50-member ensemble of climate change projections at 12-km resolution over europe and northeastern north america with the canadian regional climate model (CRCM5). J. Appl. Meteorol. Climatol. 58, 663–693. doi: 10.1175/JAMC-D-18-0021.1
Lehner, F., Coats, S., Stocker, T. F., Pendergrass, A. G., Sanderson, B. M., Raible, C. C., et al. (2017). Projected drought risk in 1.5 c and 2 c warmer climates. Geophys. Res. Lett. 44, 7419–7428. doi: 10.1002/2017GL074117
Liu, Y., Zhu, Y., Ren, L., Singh, V. P., Yong, B., Jiang, S., et al. (2019). Understanding the spatiotemporal links between meteorological and hydrological droughts from a three-dimensional perspective. J. Geophys. Res. Atmospheres 124, 3090–3109. doi: 10.1029/2018JD028947
Lloyd-Hughes, B. (2014). The impracticality of a universal drought definition. Theor. Appl. Climatol. 117:3–4. doi: 10.1007/s00704-013-1025-7
Lorenzo-Lacruz, J., Vicente-Serrano, S., López-Moreno, J., Beguería, S., García-Ruiz, J., and Cuadrat, J. (2010). The impact of droughts and water management on various hydrological systems in the headwaters of the Tagus River (central Spain). J. Hydrol. 386, 13–26. doi: 10.1016/j.jhydrol.2010.01.001
Martynov, A., Laprise, R., Sushama, L., Winger, K., Šeparovi,c, L., and Dugas, B. (2013). Reanalysis-driven climate simulation over CORDEX North America domain using the Canadian Regional Climate Model, version 5: model performance evaluation. Clim. Dyn. 41, 2973–3005. doi: 10.1007/s00382-013-1778-9
McKee, T. B., Doesken, N. J., and Kleist, J. (1993). “The relationship of drought frequency and duration to time scales,” in Proceedings of the 8th Conference on Applied Climatology, Vol. 17 (Boston), 179–183.
Mikšovskỳ, J., Brázdil, R., Trnka, M., and Pišoft, P. (2019). Long-term variability of drought indices in the Czech Lands and effects of external forcings and large-scale climate variability modes. Clim. Past 15, 827–847. doi: 10.5194/cp-15-827-2019
Mishra, A. K., and Singh, V. P. (2010). A review of drought concepts. J. Hydrol. 391, 202–216. doi: 10.1016/j.jhydrol.2010.07.012
Naumann, G., Cammalleri, C., Mentaschi, L., and Feyen, L. (2021). Increased economic drought impacts in Europe with anthropogenic warming. Nat. Clim. Chang 11, 485–491. doi: 10.1038/s41558-021-01044-3
Nikbakht, J., Tabari, H., and Talaee, P. H. (2013). Streamflow drought severity analysis by percent of normal index (PNI) in northwest Iran. Theor. Appl. Climatol. 112, 565–573. doi: 10.1007/s00704-012-0750-7
Pfeifer, S., Bülow, K., Gobiet, A., Hänsler, A., Mudelsee, M., Otto, J., et al. (2015). Robustness of ensemble climate projections analyzed with climate signal maps: seasonal and extreme precipitation for Germany. Atmosphere 6, 677–698. doi: 10.3390/atmos6050677
Ruffault, J., Martin-StPaul, N. K., Duffet, C., Goge, F., and Mouillot, F. (2014). Projecting future drought in Mediterranean forests: bias correction of climate models matters! Theor. Appl. Climatol. 117, 113–122. doi: 10.1007/s00704-013-0992-z
Santos, J. F., Pulido-Calvo, I., and Portela, M. M. (2010). Spatial and temporal variability of droughts in Portugal. Water Resour. Res. 46:W03503. doi: 10.1029/2009WR008071
Šeparovi,c, L., Alexandru, A., Laprise, R., Martynov, A., Sushama, L., Winger, K., et al. (2013). Present climate and climate change over North America as simulated by the fifth-generation Canadian regional climate model. Clim. Dynamics 41, 3167–3201. doi: 10.1007/s00382-013-1737-5
Smakhtin, V. U., and Hughes, D. (2004). Review, Automated Estimation and Analyses of Drought Indices in South Asia. Working Paper 83. Colombo: International Water Management Institute.
Spinoni, J., Barbosa, P., Bucchignani, E., Cassano, J., Cavazos, T., Christensen, J. H., et al. (2020). Future global meteorological drought hot spots: a study based on CORDEX Data. J. Clim. 33, 3635–3661. doi: 10.1175/JCLI-D-19-0084.1
Spinoni, J., Vogt, J. V., Naumann, G., Barbosa, P., and Dosio, A. (2018). Will drought events become more frequent and severe in Europe? Int. J. Climatol. 38, 1718–1736. doi: 10.1002/joc.5291
Stagge, J., Tallaksen, L., and Rizzi, J. (2015). “Future meteorological drought: projections of regional climate models for Europe,” in EGU General Assembly Conference Abstracts (Vienna), 7749.
Stagge, J. H., Kingston, D. G., Tallaksen, L. M., and Hannah, D. M. (2017). Observed drought indices show increasing divergence across Europe. Sci. Rep. 7, 1–10. doi: 10.1038/s41598-017-14283-2
Stefanon, M., D'Andrea, F., and Drobinski, P. (2012). Heatwave classification over Europe and the Mediterranean region. Environ. Res. Lett. 7, 9. doi: 10.1088/1748-9326/7/1/014023
Suarez-Gutierrez, L., Milinski, S., and Maher, N. (2021). Exploiting large ensembles for a better yet simpler climate model evaluation. Clim. Dyn. doi: 10.1007/s00382-021-05821-w
Van Lanen, H. A., Laaha, G., Kingston, D. G., Gauster, T., Ionita, M., Vidal, J.-P., et al. (2016). Hydrology needed to manage droughts: the 2015 European case. Hydrol. Process 30, 3097–3104. doi: 10.1002/hyp.10838
Vicente-Serrano, S. M., Domínguez-Castro, F., Murphy, C., Hannaford, J., Reig, F., Peña-Angulo, D., et al. (2021). Long-term variability and trends in meteorological droughts in Western Europe (1851-2018). Int. J. Climatol. 41, E690–E717. doi: 10.1002/joc.6719
Vicente-Serrano, S. M., Lopez-Moreno, J.-I., Beguería, S., Lorenzo-Lacruz, J., Sanchez-Lorenzo, A., García-Ruiz, J. M., et al. (2014). Evidence of increasing drought severity caused by temperature rise in southern europe. Environ. Res. Lett. 9:044001. doi: 10.1088/1748-9326/9/4/044001
von Trentini, F., Leduc, M., and Ludwig, R. (2019). Assessing natural variability in RCM signals: comparison of a multi model EURO-CORDEX ensemble with a 50-member single model large ensemble. Clim. Dyn. 53, 1963–1979. doi: 10.1007/s00382-019-04755-8
Werick, W., Willeke, G., Guttman, N., Hosking, J., and Wallis, J. (1994). National drought atlas developed. Eos 75, 89–90. doi: 10.1029/94EO00706
Willeke, M., and Hosking, J. (1994). The National Drought Atlas Institute for Water Resources Rep. Fort Belvoir, VA: Army Corps of Engineers, 587.
Wood, R. R., Lehner, F., Pendergrass, A. G., and Schlunegger, S. (2021). Changes in precipitation variability across time scales in multiple global climate model large ensembles. Environ. Res. Lett. 16, 084022. doi: 10.1088/1748-9326/ac10dd
Yihdego, Y., Vaheddoost, B., and Al-Weshah, R. A. (2019). Drought indices and indicators revisited. Arabian J. Geosci. 12, 69. doi: 10.1007/s12517-019-4237-z
Keywords: drought, SMILE, pre-industrial, percent of normal, internal variability, large ensemble, Europe
Citation: Böhnisch A, Mittermeier M, Leduc M and Ludwig R (2021) Hot Spots and Climate Trends of Meteorological Droughts in Europe–Assessing the Percent of Normal Index in a Single-Model Initial-Condition Large Ensemble. Front. Water 3:716621. doi: 10.3389/frwa.2021.716621
Received: 28 May 2021; Accepted: 09 August 2021;
Published: 07 September 2021.
Edited by:
Maria Pregnolato, University of Bristol, United KingdomReviewed by:
Alfredo Rocha, University of Aveiro, PortugalNazrul Islam, King Abdulaziz University, Saudi Arabia
Copyright © 2021 Böhnisch, Mittermeier, Leduc and Ludwig. This is an open-access article distributed under the terms of the Creative Commons Attribution License (CC BY). The use, distribution or reproduction in other forums is permitted, provided the original author(s) and the copyright owner(s) are credited and that the original publication in this journal is cited, in accordance with accepted academic practice. No use, distribution or reproduction is permitted which does not comply with these terms.
*Correspondence: Magdalena Mittermeier, bS5taXR0ZXJtZWllckBsbXUuZGU=
†These authors have contributed equally to this work and share first authorship