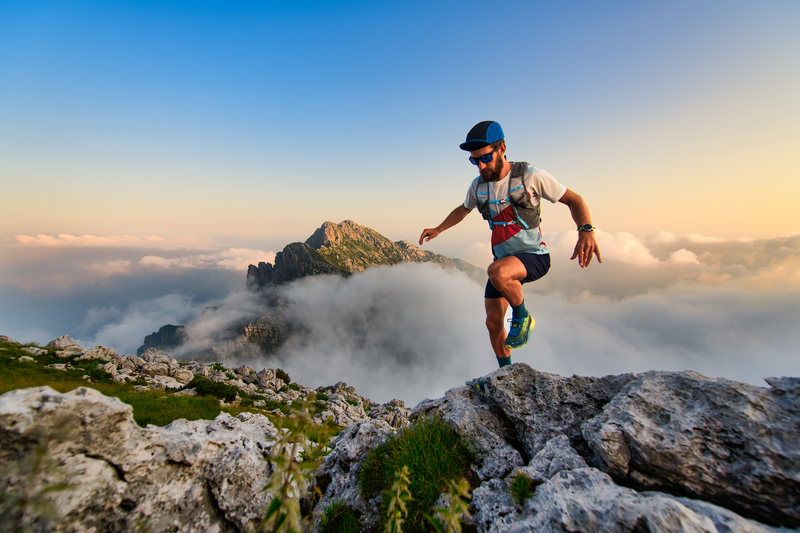
94% of researchers rate our articles as excellent or good
Learn more about the work of our research integrity team to safeguard the quality of each article we publish.
Find out more
ORIGINAL RESEARCH article
Front. Water , 10 March 2021
Sec. Water and Human Systems
Volume 3 - 2021 | https://doi.org/10.3389/frwa.2021.633046
This article is part of the Research Topic Hydrological Extremes and Society View all 5 articles
Studies on inequalities in exposure to flood risk have explored whether population of a lower socio-economic status are more exposed to flood hazard. While evidence exist for coastal flooding, little is known on inequalities for riverine floods. This paper addresses two issues: (1) is the weakest population, in socio-economic terms, more exposed to flood hazard, considering different levels of exposure to hazard? (2) Is the exposure to flood risk homogeneous across the territory, considering different scales of analysis? An analysis of the exposure of inhabitants of Liège province to flood risk was conducted at different scales (province, districts, and municipalities), considering three levels of exposure to flood hazard (level 1- low hazard, level 3- high hazard), and five socio-economic classes (class 1-poorest, class 5-wealthiest households). Our analysis confirms that weaker populations (classes 2 and 3) are usually more exposed to flood hazards than the wealthiest (classes 4 and 5). Still it should be stressed that the most precarious households (class 1) are less exposed than low to medium-range ones (classes 2 and 3). Further on the relation between socio-economic status and exposure to flood hazard varies along the spatial scale considered. At the district level, it appears that classes 4 and 5 are most exposed to flood risk in some peripheral areas. In municipalities located around the center of the city, differences of exposure to risk are not significant.
Worldwide, floods represent the most frequent natural disaster (UNISDR, 2015) and, over the period 1995–2015, they have affected more than 2 billion people (UNDRR, 2015). Moreover, the detrimental effects of floods are projected to further increase over the 21st century under the combined effect of climate and demographic evolutions, unprecedented urbanization as well as economic development (Blaikie et al., 1994; Ashley et al., 2005; Hirabayashi et al., 2013; Chen et al., 2015; Lehmann et al., 2015; Mallakpour and Villarini, 2015; Neumann et al., 2015; Ward et al., 2020).
Improving flood management is therefore of critical importance (e.g., Klijn et al., 2004; IPCC, 2012; Aerts et al., 2014). However, the design, optimal sizing, and prioritization of measures for reducing the flood impacts must be based on a reliable appraisal of the distribution of flood risk in space and in time under various scenarios. This requires accurate tools for modeling both flood hazard and flood vulnerability (Wright, 2014; Vorogushyn et al., 2018).
Birkmann (2006) distinguishes four complimentary dimensions of vulnerability, i.e., the economic, social, environmental, and institutional dimensions of vulnerability, acknowledging that the later one, the institutional dimension, is underpinning the first three.
In state-of-the-art quantitative risk modeling approaches, flood vulnerability is usually focusing on the economic dimension of vulnerability and evaluated as a combination of exposure and susceptibility (Greiving et al., 2007). The former is typically expressed in terms of land use in the floodplains, urban density, or economic value of the assets-at-risk; while the latter is estimated from damage models (Merz et al., 2010). In current approaches, the susceptibility in the housing sector is mostly related to the type of building and to construction characteristics (e.g., Dottori et al., 2016). Some multivariate flood damage models incorporate also the socio-economic status in the factors controlling susceptibility, by using parameters such as income, age, household size, house ownership, or aggregated indicators (Merz et al., 2013; Kreibich et al., 2017).
The social dimension of vulnerability “deals with aspects of justice, social differentiation and societal organization as well as individual strength” (Birkmann, 2006, p. 26). Social inequalities influence the susceptibility of various groups to harm and their ability to respond to hazards (Cutter et al., 2003). Social justice hence appears as a central challenge in flood risk assessment and mitigation (Vojinovic and Abbott, 2012). Addressing this issue in a comprehensive way is a precondition to promote an active stakeholder participation, where participants will progressively broaden the scope of their individual concerns through a regular interaction with other stakeholders as well as with simulation results (Abbot and Vojinovic, 2010a,b). Hydrological modeling can be viewed as an adequate means to promote a shared understanding of the issues at stake and to catalyze creative thinking about possible responses to water-related threats. Social justice here implies that “those who are impacted and who would benefit from water must have the opportunity to participate to its planning and management” (Abbot and Vojinovic, 2014, p. 524).
Social vulnerability studies are rooted in the assumption that interactions between social agents and exposure to floods are dynamic by nature (Turner et al., 2003). Thinking in systemic terms requires to move from a static to a process- and holistic-oriented understanding of vulnerability (Turner et al., 2003; Birkmann, 2006). Accordingly, differences between groups as regard with their exposure to floods have to be considered at all stages of the potential event, including the preparation, warning, recovery, and adaptation stages. Conversely floods may have an impact on people relationships and social networks, especially when they involve a temporary relocation of inhabitants (Tapsell et al., 2002). They can lead to a reappraisal of the adequacy of the warnings and support received from the community and the public authorities. Finally adaptation and coping strategies may reduce exposure or alleviate damages related to floods. Adaptation usually refers to long-term collective mechanisms, possibly involving changes in norms and structures that lead to crises, while coping would rather address short-term individual responses dedicated to the protection and conservation of the current system (Birkmann, 2006; Babcicky and Seebauer, 2017; Sorg et al., 2018).
There is no reason to believe that the socio-economic characteristics of the population living in floodplains is similar to those of the rest of the population. Wealthy households can typically trade-off the advantages of accessibility and available amenities, with the risk of hazard occurrence (Felsenstein and Lichter, 2014). This can lead to a self-sorting of weakest households into deprived, hazardous locations (Lall and Deichmann, 2009). In terms of response, wealthiest households usually have better resources to cope with hazard when it occurs, may it be in terms of economic assets, insurances, or social networks (Tapsell et al., 2002). Furthermore, they rather tend to be homeowners. They may hence be less likely to move when faced with flood hazards. Social capital “influences households' perception of and coping with flood risks” (Babcicky and Seebauer, 2017, p. 1017). Therefore, here, we consider the question of whether the socio-economic status acts as a determinant for living in a flood-prone area. This knowledge is of practical value as optimal risk reduction policies, such as risk communication strategies or support for preparedness and recovery, need to be tailored to the actual needs of the population, which in turn may depend on the socio-economic status. Ignoring this information may lead to suboptimal decision-making and resource allocation.
The appreciation of the socio-economic characteristics of population in flood-prone areas relates also closely to the concept of environmental inequalities. In general, environmental inequalities typically address the higher exposure to environmental disamenities (e.g., air pollution, noise) or risks faced by certain socio-economic groups (Cutter et al., 2003; Walker and Burningham, 2011; Lejeune and Teller, 2016; Lejeune et al., 2016). The definition of the socio-economic groups may differ depending on the socio-economic context and the type of pollution or risk considered. Exposure to floods is a potential source of environmental inequalities (Fielding and Burningham, 2005; Begg et al., 2015; Maldonado et al., 2016; La Rosa and Pappalardo, 2019).
Studies on inequalities in exposure to flood hazard in England and Wales found out that there is no significant difference of exposure to floods along riversides between more precarious and wealthier groups (Fielding and Burningham, 2005; Walker and Burningham, 2011; Fielding, 2012). In contrast, more precarious groups appeared to be more exposed to floods than the wealthier on the seaside. This finding was attributed to historical development: the working class used to settle preferentially near harbors on the seaside, but there was not such preferential distribution inland.
In the present research, we examine whether flood exposure differs between different socio-economic groups. It combines an engineering with a social science perspective on the concept of vulnerability (Karagiorgos et al., 2016). We consider as a case study the province of Liege in Belgium, where urbanization has taken place over the last two centuries nearby industrial sites, roads and railways situated to a great extent along the rivers and waterways (Beckers et al., 2013; Bruwier et al., 2018; Mustafa A. et al., 2018). Our findings are likely to differ from those conducted so far in different contexts (Fielding and Burningham, 2005; Walker and Burningham, 2011; Fielding, 2012). Our methodology aims at unveiling which socio-economic groups are more exposed to flood hazard, and how the links between flood exposure and socio-economic status vary across the territory, across scales (between the municipality and the province levels), and for different levels of flood hazard. Our approach takes the view that social vulnerability is inherently context-sensitive and should hence be analyzed at different scales so as to address variations in the drivers of exposure to flood hazard.
By addressing a research question at the interface between social and hydrological sciences, this study is an example of an interdisciplinary approach, as advocated by Carr et al. (2020) and others to advance sustainable flood risk management and enhance resilience of our societies.
In this section, we first introduce the Case Study. Next, we present the main input data, which consist in a flood hazard map (Flood Hazard Analysis) and a Socio-Economic Disparity map at the district level. Finally, we detail how data were processed (Data Processing Method).
Located in the eastern part of Belgium, Liege province (NUTS 2 level according to the European Union common classification of territorial units) has an area of 3,862 km2 and 1.1 million inhabitants. Besides the city of Liege, three major towns (Verviers, Huy, and Eupen) are located in the province (Figure 1). A portion of the eastern part of the province is hardly urbanized and is occupied by a national park (High Fens). The province is divided into four districts (NUTS 3 level) and 84 municipalities. The households are unevenly distributed in-between these four districts: 59% of the total population is located in Liège district, 25% in Verviers district, 9% in Huy district, and 7% in Waremme district.
Figure 1. Location of the Province of Liège (Belgium), with the main urban areas, the water bodies and the flood hazard zones.
The territory has long been structured along the main river, the Meuse, and its main tributaries, the Ourthe and the Vesdre (Figure 1). From a historical perspective, the industrial revolution in the 19th century lead to the development of urban centers nearby the rivers. This trend was reinforced by the construction of railways along the bottom of the valleys and remains visible nowadays, with a concentration of industrial brownfields and socio-economic disparities along the former industrial axes following the main water courses. Along these rivers, large floodplains are prone to riverine floods, especially at the end of the winter due to the combined effect of rainfall and snowmelt in the upper part of the catchments. This occurs mostly in the national park High Fens, which is also where large dams and reservoirs are located (Bruwier et al., 2015; Cuvelier et al., 2018).
The organization of the built environment, with dense settlements concentrated along rivers, is prone to environmental inequalities since more deprived socio-economic groups tend to be located in the inherited industrial axes along the water courses. The housing sector is predominantly dominated by owner-occupation in Belgium. This especially the case of periurban and rural areas. At the opposite, renting is dominant in city centers. This may be related to the structure of the housing stock and the resources of urban dwellers (Lejeune et al., 2016). Long-standing public policies in favor of home ownership indirectly promoted periurban development and the segregation of different income groups (Teller, 2009). This trend was further fueled by land use planning policies that were overtly oversized and allowed the development of very low-density settlements for those would could afford it (Mustafa A. M. E. S. et al., 2018). Nowadays, the socio-economic profile of the population of Liege province is medium to low (Grippa et al., 2015), which is mainly related to the impact of deindustrialization. Still, the territory is characterized by considerable spatial disparities between socio-economic groups, which makes it valuable case study for analyzing possible relations between socio-economic disparities and exposure to flood hazard.
The flood hazard map used here was generated based on two-dimensional hydraulic modeling for the river Meuse and its main tributaries. A simplified approach was used for the smaller streams. The resolution of the hydraulic model consisted of cells of 2 m by 2 m up to 5 m by 5 m and the calculation provided water depth and velocities in the main river bed and throughout the floodplains (Erpicum et al., 2010; Bruwier et al., 2015). As displayed in Figure 1, these results were then classified along three levels of hazard, considering flood frequency, water depths and velocities: low to high hazard (level 1), medium to high hazard (level 2), and high hazard zones (level 3). It should be stressed that these flood maps solely provide information on hazard and do not encompass the vulnerability component of risk. Data for assessing vulnerability at the building level is not readily available throughout the province and was considered beyond the scope of the present research.
A socio-economic disparity map was used to provide a classification of the population according to its socio-economic status. This information is available at the level of the statistical unit (census unit). The territory of the province of Liege is covered by 2,609 statistical units (Figure 2). The area of these statistical units varies between 2.3 and 5,834 ha with a median value of 43.3 ha. Statistical units typically correspond to neighborhoods in dense urban areas and encompass larger zones in rural areas (Dujardin et al., 2014).
The classification of the statistical units is based on a principal component analysis considering 23 indicators, available at the statistical unit level, grouped along the following four dimensions (Grippa et al., 2015):
(i) origin and nationality,
(ii) taxable income level,
(iii) unemployment and participation rate,
(iv) share of households with social security income.
The analysis allowed the classification of all statistical units based on a single index of social disparity (ISD) divided in five classes, with class 1 gathering the more deprived statistical units and class 5 the more affluent ones. As can be seen by comparing Figures 1, 2, social deprivation is mostly concentrated in the more densely urbanized parts of the province of Liege.
As sketched in Figure 3, the flood hazard map and the socio-economic disparity map were combined with cadastral data so as to analyze the exposure to flood hazard at the building level.
Figure 3. Combination of flood hazard and socio-economic data at the building level through the use of cadastral data. Squares represent building footprints. Cadastral data provides information about the number of housings per building. The combination of the data sets results in a database of housings with a given index of social disparity (ISD) class and a given hazard level covering the entire territory. This is used for estimating the number of households exposed to a certain level of hazard along the different socio-economic levels.
The socio-economic profile of the households in each individual building was inferred from the values available at the level of the statistical unit. Possible socio-economic disparities within a given statistical unit were assumed negligible. Despite this simplification, examining flood exposure at the building level remains nonetheless a major improvement compared to studies conducted at the level of predefined administrative units that do not coincide with the limits of the flood hazard zones (Maantay and Maroko, 2009). Considering disparities within statistical units would require disaggregated data at the building scale, which are not available throughout the case study area.
The number of households accounted for at the statistical unit level were attributed to each building in proportion with the number of housing units per building given by cadastral data. When a building was included in two or more flood hazard zones, its exposure was selected as the highest level of exposure.
We calculated the distribution of households, Hn,j, for the five socio-economic classes (n = 1–5) and the three level of exposure to flood hazard (j = 1–3) for the entire Province. These figures can then be compared with the distribution of households along the five socio-economic classes at the province level so as to get an estimate of the over- or under-exposition of some socio-economic groups to a given level of hazard.
The same calculations were applied at the district level for all four districts (Liege, Verviers, Waremme, and Huy) and compared with the distribution of households by ISD classes for each district.
The same calculations were also applied for all 84 municipalities of the province. It was then possible to identify those municipalities where:
• lower ISD households are the most exposed to a given level of flood hazard, meaning that the percentage of lower ISD households located in flood prone zones is more than 5% higher than the percentage of lower ISD households in the entire municipality,
• higher ISD households are the most exposed to a given level of flood hazard, meaning that the percentage of higher ISD households located in flood prone zones is more than 5% higher than the percentage of higher ISD households in the entire municipality,
• there is no significant difference between household distributions in the considered flood hazard zone and in the entire municipality.
For these three categories of municipalities, the exposure to flood at the municipal level was calculated as follows:
where Ei,k is the exposure of municipality i to the flood hazard level k (k = 1, 2, 3), θi,k is the number of households in the municipality located in the flood hazard zone of level k and ∑ θi is the total number of households located in the municipality i.
Thresholds Eup,k et Elow,k are then calculated for each flood level k on the basis of the mean exposure of the municipality (μFL,k) and its standard deviation:
The exposure to flood hazard of each municipality was compared to these thresholds in order to identify which municipality is characterized by a strong exposure (higher than upper threshold Eup,k), medium exposure [in between the two thresholds and low exposure (smaller than lower threshold Elow,k)] for each flood level.
The combination of the three categories of municipalities defined as regard with ISD with the three categories of exposure to flood hazard generated a 3 × 3 matrix of municipalities that could be easily mapped (Figure 4).
In this section, the results obtained at the Province Level, District Level, and Municipality (LAU, Formerly NUTS5) levels are presented.
The distribution of households across the five ISD classes was first compared between the whole province of Liege and in the flood hazard zones.
As can be seen in Figure 5, the distribution of households in the whole province peaks in class 1 (30% of households) and decreases down to class 5 (16% of households). This contrasts with the distribution of the households in flood hazard zones. Indeed, the distribution of households in flood hazard zones peaks in classes 2 and 3 (21 and 25%, respectively, compared to 20 and 18% for the whole province). The portion of households in class 4 is slightly higher in flood hazard zones compared to the whole province (18 vs. 17%), whereas it is considerably lower in class 1 (19 vs. 30%). No substantial difference can be found in class 5. This is also highlighted by the yellow bars in Figure 6B, which shows the difference between the distribution of households in the whole province and in flood-prone zones.
Figure 5. Distribution of households as a function of their ISD class, in the whole province of Liège as well as in flood hazard zones.
Figure 6. (A) Distribution of households as a function of their ISD class, in the whole province and in flood-prone areas defined by to different ranges of hazard (low to high, medium to high, or high only); and (B) difference with respect to the household distribution in the whole province.
Figure 6 aims at revealing whether the choice of a particular level of hazard (low, medium, or to high) for delineating the flood prone area has an influence on the results. It compares the distribution of households as a function of their ISD class between the whole province and in flood-prone areas characterized by different levels of hazard: either only “high hazard,” or “medium to high hazard” or “low to high hazard.” Figure 6A shows the obtained distributions, while Figure 6B displays the difference between the distribution in a particular flood prone area and the distribution in the whole province. Hence, Figure 6B highlights whether a given ISD class is over- or underrepresented in each flood prone area. Compared to Figures 5, 6 adds information on the distributions within the “medium to high” hazard and the “high” hazard zones.
Irrespective of the considered level of hazard, class 3 is largely overrepresented in flood hazard zones (+ 7 to + 11%) compared to the whole province. The same applies for class 2, particularly in the case of the “medium to high” hazard and the “high” hazard zones (+ 6 and + 7%, respectively). For all hazard zones, class 1 is strongly underrepresented (– 8 to – 11%) compared to the whole province. Under- or overrepresentation of class 4 depends on the considered level of hazard; but the magnitude of the changes remains small (between – 2 and + 2%). In class 5, a more considerable influence of the considered level of hazard could be detected: when all flood hazard zones are taken into account, there is virtually no difference in the share of class 5 compared to the whole province, whereas in “medium to high” and “high” hazard zones, class 5 is substantially underrepresented (– 4 and −7%, respectively). Overall, apart from the case of class 5, Figure 6B suggests that the main findings so far remain valid whatever the level of hazard considered to delineate the extent of the flood-prone areas.
The results can also be examined the other way round, i.e., by looking at the chance for households of a particular ISD class to be situated in a flood-prone area. To do so, the number of households of a particular ISD class situated in a flood hazard zone was divided by the total number of households of this ISD class in the province (Figure 7A).
Figure 7. (A) Chance for households of being situated in a particular flood hazard zone, as a function of their ISD class. (B) Comparative environmental risk index as a function of the level of hazard.
Additionally, Figure 7B displays the “comparative environmental risk index” proposed by Harner et al., 2002 and used by Fielding and Burningham, 2005. This index is evaluated as the ratio between the chance for a household of a particular ISD class to be situated in a flood hazard zone and the chance for households of all other classes to be situated in the same flood hazard zone. A ratio above 1 in Figure 7B means that the chance of this particular ISD class to be situated in a flood hazard zone is higher that the chance of being situated in this flood hazard zone for the rest of the population (i.e., all other ISD classes).
The results show that the chance of being situated in a flood hazard zone is higher for households in ISD classes 2 and 3 than for the rest of the population. Vice-versa, the chance of being situated in a flood hazard zone is lower for households in ISD classes 1 and 5 than for the rest of the population. For households in ISD class 4, the chance of being situated in a flood hazard zone is slightly higher when the level of hazard “low to high” is considered; but when the focus is set on higher levels of hazard (“medium to high” or high), households in ISD class 4, like those in ISD class 5, have a much lower chance of being situated in a flood hazard zone than the rest of the population.
Next, we explore whether the results found at the level of the whole province equally apply at the level of each individual district. For each of the four districts in the province, the distribution of the households as a function of their ISD class is shown in Figure 8, both for the whole district and in the flood hazard zones.
Figure 8. Distribution of households in the four districts of the Province regarding their ISD class and their location in flood hazard zones.
The distributions obtained for the district of Liege are very similar to those found at the province level. This was expected to a great extent since the population in Liege district represents almost 60% of the population in the whole province. In Verviers District, the results are also similar to those computed at the province level, except for ISD class 4, which is overrepresented in the three flood-prone zones. In contrast, the distributions obtained for the two other districts differ considerably from those at the province level. In Huy District, ISD class 1 is overrepresented in low to high hazard flood-prone zone and in medium to high hazard flood-prone zone, unlike ISD class 1 in the whole province. In Waremme district, results go in the opposite direction. ISD classes 4 and 5 are overrepresented in flood-prone zones whereas classes 2 and 3 are underrepresented in these zones. Class 1 is not significantly present in Waremme District.
The comparative environmental risk index evaluated for each district individually is shown in Figure 8. It highlights that, in the district of Huy, the chance for a household of ISD class 2 or 3 to be situated in a flood hazard zone is remarkably higher than in the other districts. It can also be observed that in the district of Waremme, the trends are opposite to those found in the other three districts: ISD classes 4 and 5 are much more exposed to flood hazard in Waremme district than in other districts.
Overall, Figures 8, 9 emphasize a substantial spatial variability in the influence of the ISD class on exposure to flood hazard. Indeed, the household distributions and the comparative environmental risk indices found, at least in some individual districts, differ noticeably from the corresponding distributions and comparative environmental risk indices obtained at the province level.
Figure 9. Comparative environmental risk index (CERI) in each district, as a function of the level of hazard.
Figure 4 displays in red municipalities where lower ISD classes are overrepresented in flood-prone area, in green municipalities where higher index of social disparity (ISD) classes are overrepresented in flood-prone area, and in gray municipalities where differences of exposure are not significant.
In municipalities located in Liège district along the Meuse River, differences of exposure between ISD classes are not significant (Figure 4). This area is the one that concentrates the greater number of statistical units with a low ISD class (Figure 6). It can be further observed that, in Waremme district, while the ISD level is generally higher, wealthy populations may be exposed to higher hazard. Consistently with the results found at the district level, Figure 4 stresses that the influence of the ISD class on exposure to flood hazard shows some great spatial variability at the municipal level, indicating that the results found do depend on scale of the analysis.
This study aimed at answering two questions in order to evaluate the existence of environmental inequalities as regard with exposure to flood hazard in Liège province. (1) Is the weakest population, in socio-economic terms, more exposed to flood hazard, considering different levels of exposure to hazard? (2) Is the exposure to flood risk homogeneous across the territory, considering various levels of analysis? The analysis addressed first the province level and then the district and municipal levels.
At the province level, results show that classes 2 and 3 are significantly overrepresented in all three flood-prone zones. The opposite observation was found for classes 1, 4, and 5. It confirms that the socio-economic conditions in hazard zones differ from those observed in the whole population of the province. Possible mechanisms to explain these differences are hypothesized in Figure 10. In the case study area like in many places elsewhere, the bottom of the valleys tends to be more densely urbanized. Besides, wealthier population is underrepresented in densely urbanized areas, and vice-versa in more rural areas. These aspects are consistent with the lower exposure of ISD classes 4 and 5, and the higher exposure of ISD classes 2 and 3. However, it does not explain why ISD class 1 is underrepresented in the flood hazard zones. Another mechanism probably at stake is the level of the standards of flood protection used for sizing flood defenses. These standards are higher in densely urbanized areas compared to more rural areas. This may be a reason why the ISD class 1 is less exposed to flood hazard. In particular, the methodology used for determining the flood hazard map considered here is based on flood scenarios with return periods up to 100 years, while the protection standard in the city of Liege is of the order of 300 years. As a consequence, none of the relatively poor neighborhoods close to the city center of Liege are situated in a flood hazard zone.
A comparison of the results at the province and district levels highlights that observations related to exposure to flood hazard somehow vary with the scale considered in the study. It is hence crucial to adopt a multi-scale analysis in the analysis of environmental inequalities. Otherwise dominant features of the issue may overshadow the situation faced by some communities. This further confirms the place-based character of social vulnerability (Cutter et al., 2003; Turner et al., 2003; Birkmann, 2006). The hydrosocial mechanisms at play in one district may basically differ from the ones observed in other districts. Place affects both the drivers governing the exposure to flood hazard and the adaptation capabilities of concerned households. One of the strengths of place-based analyses is their potential for a more efficient public involvement and a shared assessment of vulnerabilities (Turner et al., 2003; Abbot and Vojinovic, 2014). Collective and individual responses should be framed in this perspective, addressing the social and hydrological dimensions as a coupled system heavily reliant on local conditions.
Lower ISD classes tend to be more exposed to flood risk in rural areas and small-sized cities like Huy, whereas it is the case in only about half of the municipalities of post-industrial urban areas like Liege and Verviers. In more affluent and recently developed periurban areas located in the north of the province, higher ISD classes are more exposed to flood in most municipalities. These results confirm those computed at the district level. Here again they stress the need of a multi-scale analysis in order to reveal differences in exposure to flood hazard along places and groups that may be hidden by the aggregation of divergent situations.
Previous studies showed that the level of exposure to flood hazard varies with ISD class, especially on the seaside. Moreover, those results vary with the scale considered: differences of exposure between ISD classes are bigger on the seaside than in the countryside. However, in these studies, the level of exposure to flood hazard in the UK was found to decrease with precariousness, whereas in this paper, the level of exposure to flood hazard in Liège Province, Belgium, was found to peak in classes 2 and 3.
Several studies were carried out to evaluate environmental inequalities regarding flood hazard exposure in England and Wales (Fielding and Burningham, 2005; Walker and Burningham, 2011; Fielding, 2012). These three studies are based on different methods for computing the population at risk and different methods for calculating the level of exposure of each ISD class. Fielding and Burningham's study (2005) and Walker and Burningham study 2011 use disaggregation of data to compute the at-risk population, whereas Fielding's is based on a data base that provides postal addresses that are considered in at-risk areas. To calculate the level of exposure, Fielding and Burningham's study (2005) proposed the comparative environmental risk index that we used in this paper. It is important to consider both at risk and not-at-risk populations in the analysis of environmental inequities as it allows comparing the exposure of all groups with the one of the general population –a meaningful index in terms of decision making.
Home ownership and household size are not taken into consideration in the social disparity index we used. It is somehow debatable given their incidence on the coping capacity of individuals. Home ownership is reported to have an ambivalent influence on vulnerability to floods. When compared to renters, home owners were more often to leave temporarily their home due to floods (Fekete, 2009), which may be related to housing types (individual houses vs. apartments), but they are less likely to move (Thieken et al., 2007). Homeowners are usually more aware of the risks and prone to invest in mitigation and precautionary measures (Steinführer and Kuhlicke, 2007; Thieken et al., 2007). Household structure may also have an ambivalent effect. Lone parents and isolated elderly have less income and must cope singlehandedly with the impacts of the flood (Tapsell et al., 2002; Welle et al., 2014). In the case of isolated elderly, besides difficulties to follow emergency measures, they may be less aware of public warnings and informal communication channels (Steinführer and Kuhlicke, 2007). An increase of household size would then decrease vulnerability due to mutual help (Welle et al., 2014). On the opposite one may consider that “households with dependent persons are more vulnerable than households in which every person can rely on her-/himself” (Steinführer and Kuhlicke, 2007, p. 35).
Thieken et al., 2007 highlighted that home ownership and household size are better correlated with the adoption of emergency measures than household revenue itself. Ownership structure further influences the observed damage to goods, home owners being more susceptible of damage to household content and less susceptible to damage to residential buildings (Steinführer and Kuhlicke, 2007; Thieken et al., 2007). Still it should be stressed that these relations may vary from one place to another, according to the local urban structure, the effective experience with floods or local norms and values (the attitude toward insurance for instance).
Addressing social vulnerability, in a holistic and process-based approach, would require to consider more dimensions than the sole social disparity index that we adopted. Home ownership, household size, but also age, gender, occupation, or ethnicity, all these factors are usually combined in Social Vulnerability Indices (Tapsell et al., 2002, Cutter et al., 2003; Fekete, 2009; Sorg et al., 2018). The benefits of such an integrated approach is that it provides a wider and more complete understanding of vulnerability. Its limitations lie in the ambivalent character of some of these factors, and the spatial variability of their effects, which may somehow be blurred in composite indices. Furthermore, some of these factors may overlap, as for instance home ownership and economic revenues, which makes the interpretation even more complex.
This study shows that the socio-economic characteristics of people living in floodplains differs from the socio-economic characteristics of the rest of the population in the province of Liege, Belgium. On a scale from 1 to 5 (1 being the poorest and 5 being the wealthiest), populations that are the most exposed to flood risks are not ISD class 1, but ISD classes 2 and 3.
Moreover, the study reveals that the socio-economic characteristics vary across scale and from one location to the another. For the considered case study, the results in two districts (Liege and Verviers) are similar to those computed at level of the whole province, whereas in Huy District, lower ISD classes appear to be most exposed to flood hazard, and the opposite result was found in Waremme District. This highlights the importance of a multiscale analysis of environmental inequalities related to exposure to flood hazard.
Such a multi-scale perspective is important at the time of revealing divergent though significant results from one place to another. It may have deep implications in decision-making for flood risk management, particularly as regards the allocation of limited resources. Indeed, as analysis of flood vulnerability is a prerequisite for guiding risk mitigation policies, knowing the socio-economic characteristics of people-at-risk is important to inform sustainable and equitable flood risk management policies.
From a scientific perspective, the findings of our study constitute another challenge for the emerging science of socio-hydrological modeling (Elshafei et al., 2014; Di Baldassarre et al., 2018; Barendrecht et al., 2019; Carr et al., 2020), which aims at capturing the intertwined dynamics of hydrological and human systems. Populations in the latter may certainly not be considered as a single homogeneous group; but socio-economic disparities need to be reflected in the socio-hydrological models to ensure that they deliver realistic predictions. Moreover, in line with the concept of co-evolution (Sivapalan et al., 2012; Sivapalan and Blöschl, 2015), further research needs to disentangle to which extent do floods act as a driver for change in the socio-economic profile of people living in floodplains: do more frequent floods attract people of a lower socio-economic status?
A valuable follow-up of this research is to further investigate the links between socio-economic characteristics and flood risk. Indeed, in the present study, mostly the hazard factor of risk was taken into consideration, together with the exposure component of vulnerability. Social vulnerability is a complex, multifaceted system which requires dedicated modeling approaches (e.g., de Brito et al., 2018, Sorg et al., 2018). It should be stressed that the validation of such integrated social vulnerability models further requires to collect empirical evidence, at the household level, about the relation between hazard parameters, social attributes and disaster impact, considering both the preparation, preparedness, recovery and adaptation stages (Fekete, 2009). Such an information is not yet available in the region under study. Similarly, it would be of high relevance to extend the study to the case of pluvial flooding, which may require a more local analysis of flood hazard and vulnerability.
Overall, this interdisciplinary study is an example of collaboration across different research fields, particularly between social and natural sciences, which is recognized as vital to advance our understanding of the interplay between the human and water systems. Accounting explicitly for the multiple interactions between environmental and social processes, occurring at different timescales, will enable better informed projections into the future and, therefore more optimal decisions.
The raw data supporting the conclusions of this article will be made available by the authors, without undue reservation.
CP, BD, and JT contributed to conception and design of the study. CP organized the database and performed the statistical analysis. CP wrote the first draft of the manuscript. BD, PA, and JT wrote sections of the manuscript. All authors contributed to manuscript revision, read, and approved the submitted version.
The authors declare that the research was conducted in the absence of any commercial or financial relationships that could be construed as a potential conflict of interest.
We would wish to acknowledge the SPW Groupe Transversal Innondations (GTI) of the Walloon Region for the delivery of flood hazard maps and the CPDT (Conférence Permanente du Développement Territorial) for the Index of Social Disparity.
The Supplementary Material for this article can be found online at: https://www.frontiersin.org/articles/10.3389/frwa.2021.633046/full#supplementary-material
Supplementary Figure 1. Number of households as a function of their ISD class, for the whole territory as well as in flood hazard zones, in (A) the whole province of Liege, (B) the district of Liege, (C) the district of Huy, (D) the district of Verviers and (E) the district of Waremme.
Supplementary Figure 2. (A) Distribution of households as a function of their ISD class, in each district and in flood-prone areas defined by to different ranges of hazard (low to high, medium to high, or high only); and (B) difference with respect to the household distribution in the whole district.
Abbot, M. B., and Vojinovic, Z. (2010a). Realising social justice in the water sector: 1. J. Hydroinformatics 12, 97–117. doi: 10.2166/hydro.2010.065
Abbot, M. B., and Vojinovic, Z. (2010b). Realising social justice in the water sector: 2. J. Hydroinformatics 12, 225–239. doi: 10.2166/hydro.2009.065
Abbot, M. B., and Vojinovic, Z. (2014). Towards a hydroinformatic praxis in the service of social justice. J. Hydroinformatics 16, 516–530. doi: 10.2166/hydro.2013.198
Aerts, J. C., Botzen, W. W., Emanuel, K., Lin, N., De Moel, H., and Michel-Kerjan, E. O. (2014). Evaluating flood resilience strategies for coastal megacities. Science 344, 473–475. doi: 10.1126/science.1248222
Ashley, R. M., Balmforth, D. J., Saul, A. J., and Blanskby, J. D. (2005). Flooding in the future—predicting climate change, risks and responses in urban areas. Water Sci. Technol. 52, 265–273. doi: 10.2166/wst.2005.0142
Babcicky, P., and Seebauer, S. (2017). The two faces of social capital in private flood mitigation: opposing effects on risk perception, self-efficacy and coping capacity, J. Risk Res. 20, 1017–1037. doi: 10.1080/13669877.2016.1147489
Barendrecht, M. H., Viglione, A., Kreibich, H., Merz, B., Vorogushyn, S., and Blöschl, G. (2019). The value of empirical data for estimating the parameters of a sociohydrological flood risk model. Water Resour. Res. 55, 1312–1336. doi: 10.1029/2018WR024128
Beckers, A., Dewals, B., Erpicum, S., Dujardin, S., Detrembleur, S., Teller, J., et al. (2013). Contribution of land use changes to future flood damage along the river Meuse in the Walloon region. Nat. Hazard Earth Syst. Sci. 13, 2301–2318. doi: 10.5194/nhess-13-2301-2013
Begg, C., Walker, G., and Kuhlicke, C. (2015). Localism and flood risk management in England: the creation of new inequalities? Environ. Plann. 33, 685–702. doi: 10.1068/c12216
Birkmann, J. (2006). “Measuring vulnerability to promote disaster-resilient societies: conceptual frameworks and definitions,” in Measuring Vulnerability to Natural Hazards: Towards Disaster Resilient Societies, ed J. Birkmann (Tokyo: United Nations University Press), 9–54.
Blaikie, P., Cannon, T., Davis, I., and Wisner, B. (1994) At Risk: Natural Hazards, People's Vulnerability Disasters. London: Routledge.
Bruwier, M., Erpicum, S., Pirotton, M., Archambeau, P., and Dewals, B. J. (2015). Assessing the operation rules of a reservoir system based on a detailed modelling chain. Nat. Hazards Earth Syst. Sci. 15:365. doi: 10.5194/nhess-15-365-2015
Bruwier, M., Mustafa, A., Aliaga, D. G., Archambeau, P., Erpicum, S., Nishida, G., et al. (2018). Influence of urban pattern on inundation flow in floodplains of lowland rivers. Sci. Total Environ. 6229–623, 4469–458. doi: 10.1016/j.scitotenv.2017.11.325AQuncite
Carr, G., Barendrecht, M. H., Debevec, L., Kuil, L., and Blöschl, G. (2020). People and water: understanding integrated systems needs integrated approaches. J. Water Supply Res. Technol-Aqua 69, 819–832. doi: 10.2166/aqua.2020.055
Chen, Y., Zhou, H., Zhang, H., Du, G., and Zhou, J. (2015). Urban flood risk warning under rapid urbanization. Environ. Res. 139, 3–10. doi: 10.1016/j.envres.2015.02.028
Cutter, S., Boruff, B., and Lynn Shirley, W. (2003). Social vulnerability to environmental hazards. Soc. Sci. Q. 84, 242–261. doi: 10.1111/1540-6237.8402002
Cuvelier, T., Archambeau, P., Dewals, B., and Louveaux, Q. (2018). Comparison between robust and stochastic optimisation for long-term reservoir management under uncertainty. Water Resour. Manag. 32, 1599–1614. doi: 10.1007/s11269-017-1893-1
de Brito, M. M., Evers, M., and Almoradie, A. D. S. (2018). Participatory flood vulnerability assessment: a multi-criteria approach. Hydrol. Earth Syst. Sci. 22, 373–390. doi: 10.5194/hess-22-373-2018
Di Baldassarre, G., Kreibich, H., Vorogushyn, S., Aerts, J., Arnbjerg-Nielsen, K., Barendrecht, M., et al. (2018). Hess opinions: an interdisciplinary research agenda to explore the unintended consequences of structural flood protection. Hydrol. Earth Syst. Sci. 22, 5629–5637. doi: 10.5194/hess-22-5629-2018
Dottori, F., Figueiredo, R., Martina, M. L., Molinari, D., and Scorzini, A. R. (2016). INSYDE: a synthetic, probabilistic flood damage model based on explicit cost analysis. Nat. Hazards Earth Syst. Sci. 16, 2577–2591.doi: 10.5194/nhess-16-2577-2016
Dujardin, S., Marique, A.-F., and Teller, J. (2014). Spatial Planning as a driver of change in mobility and residential energy consumption. Energy Build. 68, 779–785. doi: 10.1016/j.enbuild.2012.10.059
Elshafei, Y., Sivapalan, M., Tonts, M., and Hipsey, M. R. (2014). A prototype framework for models of socio-hydrology: identification of key feedback loops with application to two Australian case-studies. Hydrol. Earth Syst. Sci. Discuss. 11, 629–689. doi: 10.5194/hessd-11-629-2014
Erpicum, S., Dewals, B., Archambeau, P., Detrembleur, S., and Pirotton, M. (2010). Detailed inundation modelling using high resolution DEMs. Eng. Appl. Comput. Fluid Mech. 4, 196–208. doi: 10.1080/19942060.2010.11015310
Fekete, A. (2009). Validation of a social vulnerability index in context to river-floods in Germany. Nat. Hazards Earth Syst. Sci. 9, 393–400. doi: 10.5194/nhess-9-393-2009
Felsenstein, D., and Lichter, M. (2014). Social and economic vulnerability of coastal communities to sea level rise and extreme flooding. Nat. Hazard 71, 463–491. doi: 10.1007/s11069-013-0929-y
Fielding, J. (2012). Inequalities in exposure and awareness of flood risk in England and Wales. Disasters 36, 477–494. doi: 10.1111/j.1467-7717.2011.01270.x
Fielding, J., and Burningham, K. (2005). Environmental inequality and flood hazard. Local Environ. 10, 1–17. doi: 10.1080/13549830500160875
Greiving, S., Fleischhauer, M., and Lückenkötter, J. (2007). A methodology for an integrated risk assessment of spatially relevant hazards. J. Environ. Plan. Manag. 49, 1–19. doi: 10.1080/09640560500372800
Grippa, T., Marissal, P., May, X., Wertz, I., and Loopmans, M. (2015). Dynamiques des quartiers en difficulté dans les régions urbaines belges. Brussels: SPP Intégration Sociale [in French].
Harner, J., Warner, K., Pierce, J., and Huber, T. (2002). Urban environmental justice indices. Prof. Geog. 54, 318–331. doi: 10.1111/0033-0124.00333
Hirabayashi, Y., Mahendran, R., Koirala, S., Konoshima, L., Yamazaki, D., Watanabe, S., et al. (2013). Global flood risk under climate change. Nat. Clim. Change 3, 816–821. doi: 10.1038/nclimate1911
IPCC (2012). Managing the Risks of Extreme Events and Disasters to Advance Climate Change Adaptation. A Special Report of Working Groups I and II of the IPCC. New York, NY: Cambridge University Press.
Karagiorgos, K., Thaler, T., Heiser, M., Hübl, J., and Fuchs, S. (2016). Integrated flash flood vulnerability assessment: insights from East Attica, Greece. J. Hydrol. 541, 553–562. doi: 10.1016/j.jhydrol.2016.02.052
Klijn, F., van Buuren, M., and van Rooij, S. A. M. (2004). Flood-risk Management Strategies for an Uncertain Future: Living with Rhine River Floods in The Netherlands?," AMBIO J. Hum. Environ. 33, 141–147. doi: 10.1579/0044-7447-33.3.141
Kreibich, H., Botto, A., Merz, B., and Schröter, K. (2017). Probabilistic, multivariable flood loss modeling on the mesoscale with BT-FLEMO. Risk Anal. 37, 774–787. doi: 10.1111/risa.12650
La Rosa, D., and Pappalardo, V. (2019). Planning for spatial equity—a performance based approach for sustainable urban drainage systems. Sustain. City Soc. 53:101885. doi: 10.1016/j.scs.2019.101885
Lall, S. V., and Deichmann, U. (2009). Density and Disasters: The Economics of Urban Hazard Risk. Policy Research Working Paper 5161, the World Bank Finance, Economics, And Urban Development Department and Development Research Group Environment and Energy Team, Washington, DC. doi: 10.1596/1813-9450-5161
Lehmann, J., Coumou, D., and Frieler, K. (2015). Increased record-breaking precipitation events under global warming. Clim. Change 132, 501–515. doi: 10.1007/s10584-015-1434-y
Lejeune, Z., and Teller, J. (2016). Incentives and barriers to environmental inequality mobilization: a case-study analysis in Wallonia, Belgium. Environ. Sci. Policy 66, 208–216. doi: 10.1016/j.envsci.2016.07.016
Lejeune, Z., Xhignesse, G., Kryvobokov, M., and Teller, J. (2016). Housing quality as environmental inequality: the case of Wallonia, Belgium. J. Hous. Built. Environ. 31, 495–512. doi: 10.1007/s10901-015-9470-5
Maantay, J., and Maroko, A. (2009). Mapping urban risk: flood hazards, race, and environmental justice in New York. Appl. Geogr. 29, 111–124. doi: 10.1016/j.apgeog.2008.08.002
Maldonado, A., Collins, T. W., Grineski, S. E., and Chakraborty, J. (2016). Exposure to flood hazards in miami and houston: are hispanic immigrants at greater risk than other social groups? Int. J. Environ. Res. Public Health 13:775. doi: 10.3390/ijerph13080775
Mallakpour, I., and Villarini, G. (2015). The changing nature of flooding across the central United States. Nat. Clim. Change 5, 250–254. doi: 10.1038/nclimate2516
Merz, B., Kreibich, H., and Lall, U. (2013). Multi-variate flood damage assessment: a tree-based data-mining approach. Nat. Hazards Earth Syst. Sci. 13, 53–64. doi: 10.5194/nhess-13-53-2013
Merz, B., Kreibich, H., Schwarze, R., and Thieken, A. (2010). Assessment of economic flood damage. Nat. Hazards Earth Syst. Sci. 10, 1697–1724. doi: 10.5194/nhess-10-1697-2010
Mustafa, A., Bruwier, M., Archambeau, P., Erpicum, S., Pirotton, M., Dewals, B., et al. (2018). Effects of spatial planning on future flood risks in urban environments. J. Environ. Manag. 225, 193–204. doi: 10.1016/j.jenvman.2018.07.090
Mustafa, A. M. E. S., Van Rompaey, A., Cools, M., Saadi, I., and Teller, J. (2018). Addressing the determinants of built-up expansion and densification processes at the regional scale. Urban Stud. 55, 3279–3298. doi: 10.1177/0042098017749176
Neumann, B., Vafeidis, A. T, Zimmermann, J., and Nicholls, R. J. (2015). Future coastal population growth and exposure to sea-level rise and coastal flooding—a global assessment. PLoS ONE 10:118571. doi: 10.1371/journal.pone.0118571
Sivapalan, M., and Blöschl, G. (2015). Time scale interactions and the coevolution of humans and water. Water Resour. Res. 51, 6988–7022. doi: 10.1002/2015WR017896
Sivapalan, M., Savenije, H. H., and Blöschl, G. (2012). Socio-hydrology: a new science of people and water. Hydrol. Process. 26, 1270–1276. doi: 10.1002/hyp.8426
Sorg, L., Medina, N., Feldmeyer, D., Sanchez, A., Vojinovic, Z., Birkmann, J., et al. (2018). Capturing the multifaceted phenomena of socioeconomic vulnerability. Nat. Hazards 92, 257–282. doi: 10.1007/s11069-018-3207-1
Steinführer, A., and Kuhlicke, C. (2007). Social Vulnerability and the 2002 Flood. FloodSite Research Project, Report Number T11-07-08, 164p.
Tapsell, S. M., Penning-Rwsell, E. C., Tunstall, S. M., and Wilson, T. L. (2002). Vulnerability to flooding: health and social dimensions. Phil. Trans. R. Soc. A 360, 1511–1525. doi: 10.1098/rsta.2002.1013
Teller, J. (2009). Développement de l'entre-soi communautaire dans les espaces résidentiels périurbains. Dévian. Soc. 33, 547–556. doi: 10.3917/ds.334.0547
Thieken, A. H., Kreibich, H., Müller, M., and Merz, B. (2007). Coping with floods: preparedness, response and recovery of flood-affected residents in Germany in 2002. Hydrol. Sci. J. 52, 1016–1037. doi: 10.1623/hysj.52.5.1016
Turner, B., Kasperson, R., Matson, P., McCarthy, J., Corell, R., Christensen, L., et al. (2003). A framework for vulnerability analysis in sustainability science. Proc. Natl. Acad. Sci. U.S.A. 100, 8074–8079. doi: 10.1073/pnas.1231335100
Vojinovic, Z., and Abbott, M. B., (eds.). (2012). Flood Risk and Social Justice: From Quantitative to Qualitative Flood Risk Assessment and Mitigation. London: IWA Publishing. doi: 10.2166/9781780400822
Vorogushyn, S., Bates, P. D., de Bruijn, K., Castellarin, A., Kreibich, H., Priest, S., et al. (2018). Evolutionary leap in large-scale flood risk assessment needed. WIREs Water 5:e1266. doi: 10.1002/wat2.1266
Walker, G., and Burningham, K. (2011). Flood risk, vulnerability and environmental justice: evidence and evaluation of inequality in a UK context. Crit. Soc. Policy 31, 216–240. doi: 10.1177/0261018310396149
Ward, P. J. V., Blauhut, N., Bloemendaal, J. E., Daniell, M. V., De Ruiter, M. J., Duncan, R., et al. (2020). Review article: natural hazard risk assessments at the global scale, Nat. Hazards Earth Syst. Sci. 20, 1069–1096. doi: 10.5194/nhess-20-1069-2020
Welle, T., Depietri, Y., Angignard, M., Birkmann, J., Renaud, F. G., and Greiving, S. (2014). “Vulnerability assessment to heat waves, floods, and earthquakes using the MOVE framework: test case Cologne, Germany,” in Assessment of Vulnerability to Natural Hazards, eds J. Birkmann, S. Kienberger and D. Alexander (Philadelphia, PA: Elsevier), 91–124. doi: 10.1016/B978-0-12-410528-7.00005-9
Keywords: flood hazard, environmental inequalities, GIS, scale, social vulnerability
Citation: Poussard C, Dewals B, Archambeau P and Teller J (2021) Environmental Inequalities in Flood Exposure: A Matter of Scale. Front. Water 3:633046. doi: 10.3389/frwa.2021.633046
Received: 24 November 2020; Accepted: 10 February 2021;
Published: 10 March 2021.
Edited by:
Heidi Kreibich, Helmholtz Centre Potsdam, GermanyReviewed by:
Mariele Evers, University of Bonn, GermanyCopyright © 2021 Poussard, Dewals, Archambeau and Teller. This is an open-access article distributed under the terms of the Creative Commons Attribution License (CC BY). The use, distribution or reproduction in other forums is permitted, provided the original author(s) and the copyright owner(s) are credited and that the original publication in this journal is cited, in accordance with accepted academic practice. No use, distribution or reproduction is permitted which does not comply with these terms.
*Correspondence: Jacques Teller, SmFjcXVlcy5UZWxsZXJAdWxpZWdlLmJl
Disclaimer: All claims expressed in this article are solely those of the authors and do not necessarily represent those of their affiliated organizations, or those of the publisher, the editors and the reviewers. Any product that may be evaluated in this article or claim that may be made by its manufacturer is not guaranteed or endorsed by the publisher.
Research integrity at Frontiers
Learn more about the work of our research integrity team to safeguard the quality of each article we publish.