- 1Institute of Arctic and Alpine Research, University of Colorado, Boulder, CO, United States
- 2Department of Civil, Environmental, and Architectural Engineering, University of Colorado, Boulder, CO, United States
- 3Pacific Northwest National Laboratory, Richland, WA, United States
Healthy river ecosystems require the interaction of many physical and biological processes to maintain their status. One physical process supporting biogeochemical cycling is hydrologic exchange (i.e., hydrologic exchange flows, HEFs) between relatively fast-flowing channel waters and slower-flowing surface and subsurface waters (lateral and vertical). Land uses adjacent to rivers have the potential to alter the water quality of off-channel surface and subsurface waters, and HEFs therefore have the potential to deliver solutes associated with river-adjacent land uses to rivers. HEFs can be nonpoint, diffuse sources of pollution, making the ultimate pollution source difficult to identify, especially in large rivers. Here, we seek to identify HEFs in the Columbia River near Richland, WA by looking for anomalies in temperature and electrical conductivity (EC) along the bed of the river in February, June, July, August, and November 2018. These are ultimately the “ends” of HEFs as they are locations of subsurface inflow to the river. We found these anomalies to be a combination of warmer or colder and higher (but not lower) EC than river water. We identified a majority of warm anomalies in February and July 2018, and majority cold anomalies in June, August, and November 2018. High-EC anomalies were found mostly in February, August, and November. Combined, we observe a shift from warm, high EC anomalies dominating in February to equivalent EC, warm anomalies in June, to equivalent EC, cool anomalies dominating July. In August, we also measured dissolved nitrate () in-situ to determine whether anomalies were associated with increased loading to the river, especially along the eastern shoreline, which is dominated by agricultural land use. Inflows along the eastern shoreline have greater concentrations of nitrate than river water (up to 10 mg N–/L). This research demonstrates that HEFs are temporally and spatially dynamic transferring heat and solutes to rivers.
Introduction
Healthy river ecosystems require the interaction of many processes to maintain a high level of water quality, including hydrologic exchange flows (HEFs)—exchanges between channel waters with subsurface and off-channel waters (Harvey and Gooseff, 2015). HEFs include exchanges between channels and water in riverbeds and riverbanks (i.e., hyporheic exchange, bank storage), slow-moving water alongside the main channel, overbank flow into and out of floodplains, gains of water into the river from surface flows, and gains of water from groundwater alongside the river. These complex exchanges may occur repeatedly with variations in source and destination. HEFs may originate at the river channel (i.e., hyporheic exchange) or on the landscape (e.g., irrigation water applied to fields adjacent to rivers) (see conceptual overview in Payn et al., 2009), but their direction and magnitude are determined by spatiotemporal dynamics in water tables and hydraulic conductivity fields of the subsurface (e.g., Woessner, 2000; Shuai et al., 2019). Rivers are the common termination point for HEFs because they typically occupy the lowest point of valley cross-sections. As such, rivers often receive water, solutes, and energy through HEFs. Rivers can also lose water, solutes and energy through HEFs, but these locations are often more difficult to identify than locations of HEF inputs.
Land uses in and adjacent to river corridors have a strong influence on river water quality (e.g., Lenat and Crawford, 1994). Floodplains are often attractive to development because of proximity to rivers and they are often fertile lands for agricultural development. Whereas urbanization often increases impermeable conditions and seeks to channelize overland flows in river corridors, agricultural activity often results in the addition of water and fertilizers to soils (Hodson and Donner, 2011). Excess fertilizer applied to soils can infiltrate to depth and be transported to rivers via groundwater flow paths, i.e., HEFs (Gilmore et al., 2016). Because HEFs are not obvious to the naked eye, the locations of fertilizer-derived solutes, such as nitrate () entry to the river are difficult to identify, especially in large rivers.
The motivations for this research are to improve the understanding of the dynamic nature of HEF inputs to rivers in space and time. Here we focus on an 82 km segment of the Columbia River in eastern Washington State, USA. The west side of the river corridor is dominated by the Department of Energy's Hanford Site, where land development is sparse, and the east side of the river corridor is dominated by agricultural land use (crops). The river discharge in this segment is controlled by a series of upstream dams for both flood control and power generation. This work aims to investigate the relationship between HEFs and land use by comparing spatially distributed measurements of the locations of subsurface inflow to the Columbia River to nitrate measurements in the river. Specifically, we seek to determine the degree of connectivity between irrigation, HEFs, and rivers by (1) characterizing the water quality of HEFs during irrigation and non-irrigation seasons, and (2) relating nitrate concentrations with associated HEFs to sources of inflows (i.e., by contrasting the east shore and west shore of the river). We identify subsurface inflows to the river by measuring anomalies in temperature and electrical conductivity (EC) compared to river values, and expect that with the exception of the known high-nitrate concentration groundwater plume at one location along the western shore of the river, that nitrate concentrations associated with subsurface inflows to the river are generally higher along the eastern shoreline.
Study Site and Methods
Study Site
We conducted this study along a reach of the Columbia River in eastern Washington. The Columbia River is a major river in the Pacific Northwest, originating in the Rocky Mountains of British Columbia, meandering through Washington State, and entering the Pacific Ocean along the border of Washington and Oregon. The Columbia River is highly developed, containing 19 hydroelectric dams and supporting 600,000 acres of farmland (USACE, 2018). Within the Columbia River, the study site for this research is located on the so-called Hanford Reach (e.g., Cardenas and Markowski, 2011) bounded upstream by Priest Rapids Dam and extending downstream to the city of Richland (Figure 1) [Northwest Power Conservation Council (NPCC), 2019]. These boundaries also define the only non-tidal, free-flowing section of the Columbia River in the United States (Clinton, 2000). Although the Hanford Reach is free-flowing, not backed up by dams, the river discharge is regulated by the upstream Priest Rapids Dam and others further upstream. River flows are typically high during the spring as snowmelt fills upriver reservoirs and during the summer due to high demands for power (Figure 2).
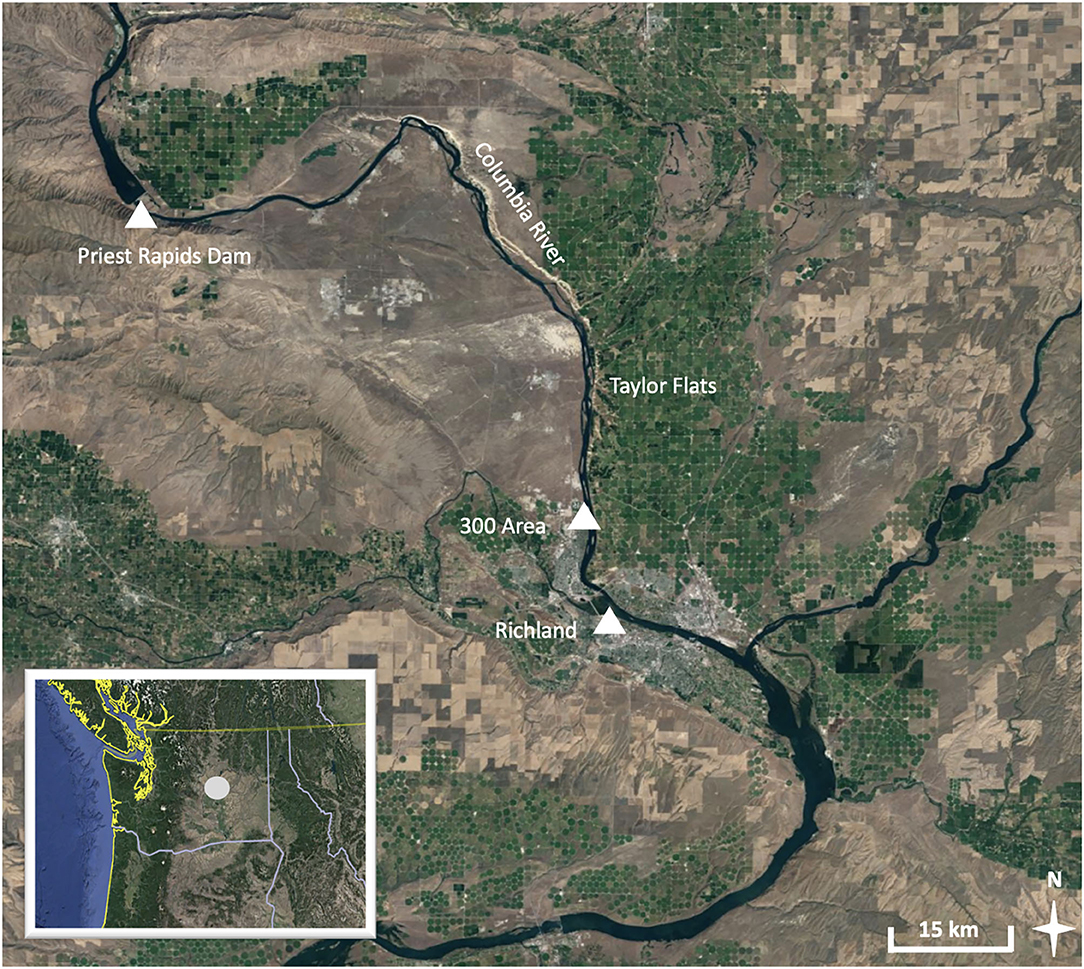
Figure 1. Map of Hanford reach. This map shows the location of the Hanford Reach in Washington State (inset) and a satellite view of Hanford Reach. The Reach begins below the Priest Rapids Dam (46.643578 N, 119.910357 W), and continues until the confluence with the Snake River. Note the green coloring, indicating locations of irrigation, in contrast to the native brown landscape. Map data from Google, Landsat.
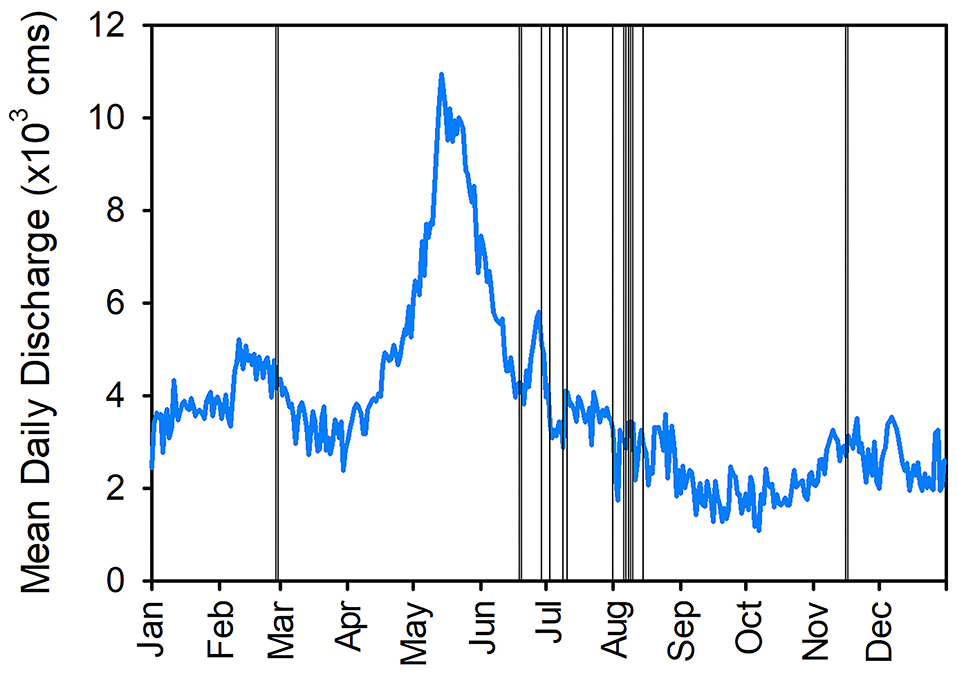
Figure 2. Mean daily discharge on the Columbia River for calendar year 2018, as observed at the Priest Rapids gage (USGS 12472800). Vertical lines indicate days of field data collection along the Hanford reach.
Irrigation east of the Hanford Reach is made possible by the Columbia Basin Project (USBR, 2014). The Columbia Basin Project diverts water from upstream of Grand Coulee Dam, located upstream of the Hanford Reach. The diverted water is routed through canals to local agricultural fields for irrigation. Excess irrigation water may reach constructed canals that divert return flows to specific points in the river or follow subsurface pathways that may end up in the river. Both of these fates contribute to HEFs for this reach.
In contrast to the eastern shoreline, the western shoreline of the Hanford Reach is the location of the Hanford Project, a plutonium development site from the Cold War. The Hanford Site is no longer used for plutonium development, and the current goal of the site is clean-up and remediation of the area. The western shoreline is largely undeveloped and has been generally inaccessible to the public for >80 years. Therefore, the topography and ecosystem of the land near the shoreline remains relatively undisturbed and is dominated by native shrub steppe compared to the western shoreline. The land use contrast between the two sides of the river allows for comparison of the occurrence of HEFs and associated nitrate concentrations on the irrigated eastern shoreline to the non-irrigated western shoreline.
Due to the adjacent location to the Hanford Site, and concern over resulting pollution from the site, the Hanford Reach of the river is well-studied. Previous field studies have identified sites of groundwater inflow into the river on the western shoreline (Lee et al., 1997). Hydrologic models of the reach predict HEFs along both shorelines but disagree on locations and timing of inflows (Zhou et al., 2018; Shuai et al., 2019), highlighting the need for field research, especially along the eastern shoreline.
Field Data Collection
Measuring HEFs in the field at the reach scale presents time and resource challenges, which, over the last several decades, has led to rapid development of numerical modeling approaches (Kasahara and Wondzell, 2003; Kiel and Cardenas, 2014; Gomez-Velez et al., 2017; Song et al., 2018; Shuai et al., 2019; Zachara et al., 2020). Reach-scale field data of HEFs are needed to validate the predicted exchanges by these models (Lee et al., 1997; Shuai et al., 2019).
The Hanford Reach is no exception to the challenges of measuring HEFs at the reach scale. Traditional tracer methods are impractical for this size of a river [low flows are on the order of 2,000 cm; though see Fernald et al. (2001) for an example], field studies of water exchanges in the reach focus on point-based measurements (Geist, 2000; Arntzen et al., 2006; Song et al., 2018) or transects (Cardenas and Markowski, 2011). However, these studies do not describe HEFs across the reach scale. To measure HEFs in smaller rivers and lakes, thermal probes have been towed along the beds and in the main water column simultaneously to identify inflows, which have a different temperature signal than river water (Harvey et al., 1997; Vaccaro and Maloy, 2006; Zhou et al., 2017). This approach allows for the collection of data over a large spatial scale. In order to characterize HEFs along the Hanford Reach, we chose to apply this method by towing sensors downstream.
We used both water temperature and electrical conductivity (EC) to identify subsurface inflows to the riverbed (Conner et al., 2020). There are known differences between EC and temperature values of river water and groundwater in the Columbia River (Dirkes et al., 1999; Zachara et al., 2020). Specifically, we used Campbell Scientific sensors: high-sensitivity 109-SS temperature probes (one in the shallow water column, one dragged along the bed), CS547 EC probes (one in the shallow water column, one dragged along the bed), and a GPS receiver. EC measurements are noted to be accurate to ±5% according to manufacturer guidance. All of these sensors were connected to and controlled by a CR1000 datalogger, collecting at a one-second interval. Electrical conductivity data is normalized to a specific conductivity. All data reported here are specific conductance. A Submersible Ultraviolet Nitrate Analyzer (SUNA) Seabird sensor was used to measure nitrate concentrations from August 8–16, 2018 (the only period it was available to our field team). The SUNA sensor collected 15 readings at 2 s intervals, every 2 min from the shallow water column (it was not dragged along the bed). To verify the SUNA measurements, 10 grab samples of river water were collected adjacent to the SUNA unit on August 15. These samples were analyzed for nitrate concentrations using a Dionex ICS-2000 anion chromatograph with AS40 auto sampler using an isocratic method. The nitrate concentration of grab samples ranged from 0 to 4.5 mg N–/L. Lab measurements confirmed SUNA measurements within 5% difference.
To compare the temperature and EC along the streambed with the water column, sensors were deployed on two ropes—one to measure along the riverbed, and the other ~1–2 m above the riverbed, depending on the depth of the water column (Figure 3). Several metal fishing weights were affixed to the end of each rope to keep them taught. Sensors were affixed ~0.3 m from the end of each rope to prevent collision with the weights. For the longer rope (to collect data along the bed), this design also allowed the sensors to skim over the rocks on the riverbed, avoiding sensor damage. The weights likely disturbed the bed in several places, but we expect that our velocity of travel was great enough that any material that was entrained into the flow was not directly reaching the sensors (30 cm above the bed).
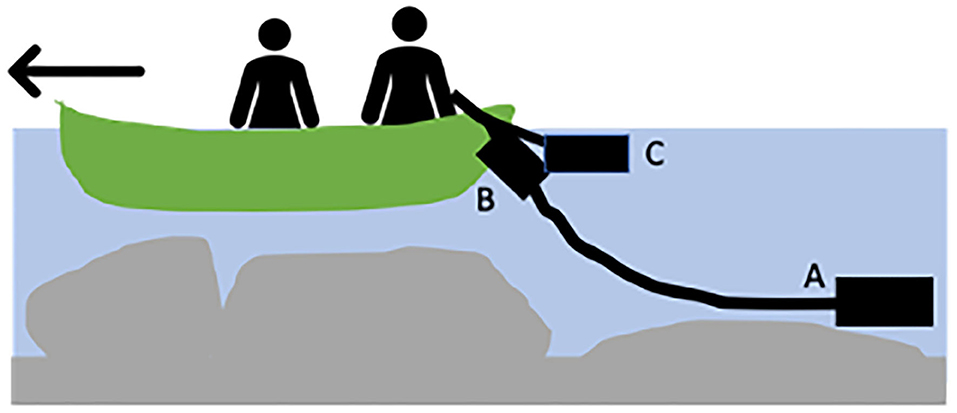
Figure 3. Data collection schematic; (A) temperature and EC sensors were deployed on a “long rope” to collect data near the riverbed, (B) temperature and EC sensors were also deployed in shallow water, and (C) during August 2018, the SUNA sensor was deployed near the shallow sensors.
Field campaigns were conducted during the summer (June, July, and August) with data collected from a canoe in order to provide navigability along the river shorelines. The canoe, a 4.2 m pointed Radisson model, provided enough stability to drag sensors while floating downstream. We used a small trolling electric motor (130 N of thrust) to power and steer the canoe. The motor allowed us to move upstream during low flows with currents around 2–3 kph. During high flows (~85,000 cm) and fast currents (max 10 kph), we could not travel upstream, but we managed to collect data at a higher spatial density. During the February and November 2018 field campaigns, we collected data from a jet boat in a similar method to the canoe. Like a canoe, a jet boat has the ability to navigate shallow waters, and because the boat can travel faster, it has the added ability to target specific locations.
Measurements were made along the entire length of the Hanford Reach, but we focused most of our data collection on the lower 28 km of the reach from Savage Island to Richland (Figure 4). We chose daily study traverses along the Hanford Reach that allowed comparison between irrigated and non-irrigated river shoreline (Figure 4). The specific sections of river floated each day depended on discharge, weather conditions, and time of year.
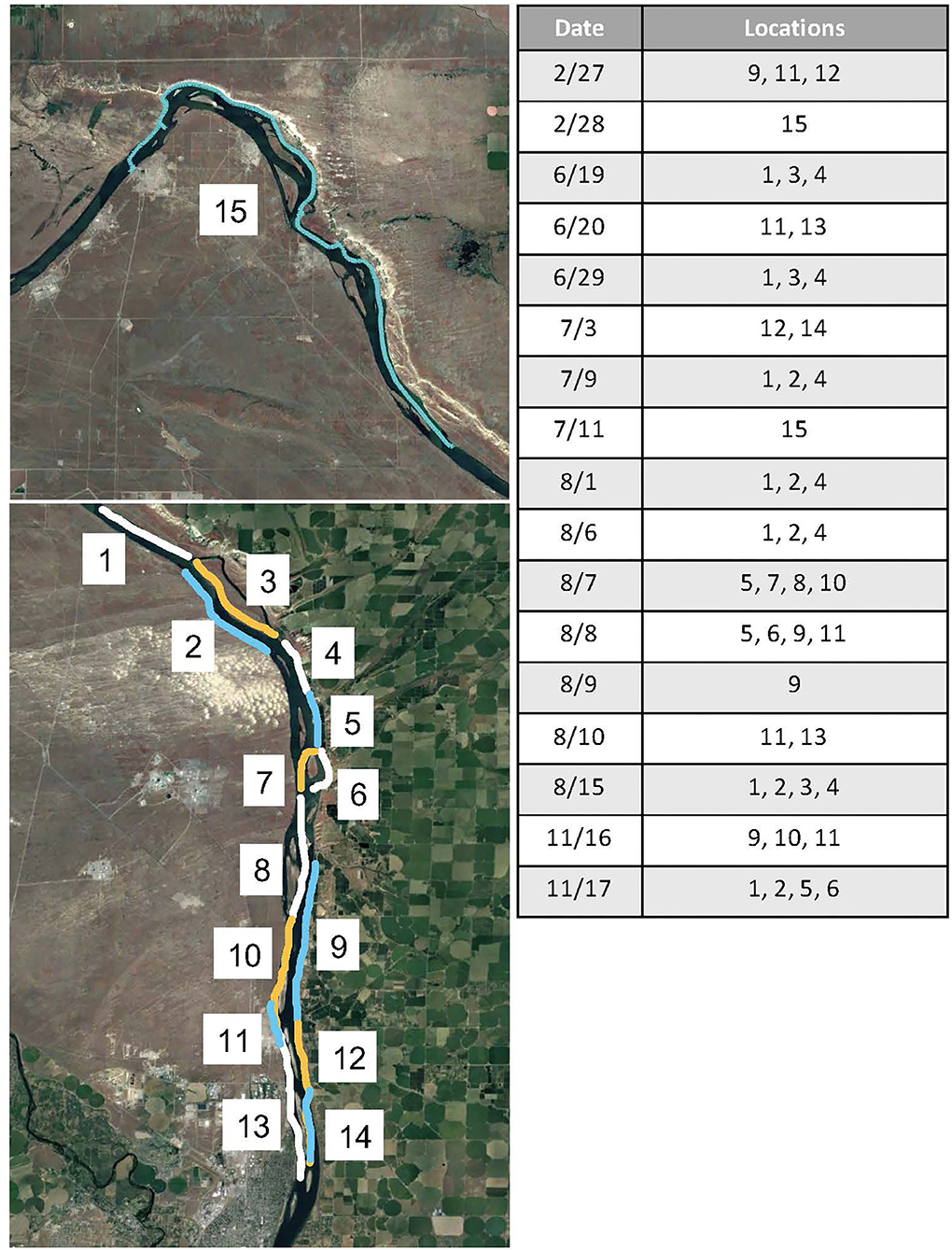
Figure 4. Sampling locations along the Hanford reach of the Columbia River as indicated by section and date (month/date) of 2018. The locations refer to the sections of river as labeled in the map. Each location is numbered, and colors are used to distinguish between sections. Map data from Google, Landsat.
Data Analysis
We developed a system for evaluating anomalies of water temperature and EC as we compared observations from the riverbed to those made in the water column (Table 1). We expected that inflow anomalies associated with broader (i.e., distal) groundwater inflows to the river would be warmer than the river water in February and November, and colder than river water June–August. However, we observed both warmer and colder anomalies in all times of the year. In all of our observations, EC was either equivalent between river water column and riverbed, or the bed EC was elevated compared to the river water column. Thus, we characterize the riverbed EC to be “elevated” or “not anomalous” in our characterization of measurements made.
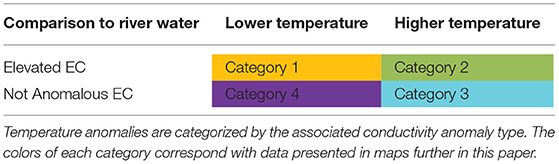
Table 1. Water quality anomaly categories (4) based on observed electrical conductivity (EC) and water temperature from the sensors on the riverbed, relative to river water column observations.
The categories of anomalies (Table 1) are interpreted differently in the summer vs. fall/winter months. For example, in summer months, distal groundwater would be expected to be lower in temperature than the river water and therefore its discharge leads to, for example Category 4 anomalies, and shallow HEFs (perhaps hyporheic exchange or surface water inputs) would be warmer than the river water and lead to Category 2 or 3 anomalies. However, these would be reversed in the late fall/winter field campaigns when the river water is relatively colder. During these campaigns, we expect the distal groundwater inflows to be warmer (Categories 2 or 3) and the shallow HEFs to be colder (Categories 1 or 4).
We also hypothesize that anomalies with elevated EC are associated with HEFs near irrigation and have higher concentrations of nitrate than the river. Furthermore, we expect anomalies without variation in EC from the river channel occur away from agriculture and that they would have low/background concentrations of nitrate (similar to those of the river). Nitrate concentrations were not measured in February, June, July, and November.
In order to identify anomalies within the temperature measurements, we developed a model of background temperature trends and calculated anomalies from measurements. Data analysis was completed in R 3.5.2, including R packages stats (loess model), and xts/zoo (time data manipulation). Background trend of river temperature was modeled using a local-smoothing model (loess) for summer data, and a linear model for fall and spring seasons (Figure 5). A linear model is better suited for capturing the trend during February and November temperatures than the loess model, due to the fewer days of data. Loess models capture the general trend by smoothing a specified span of the data (1/10). Since the Columbia River carries a large volume of well-mixed water, the spatial temperature fluctuations were minimal within the same day. The temperature values predicted by the chosen model for data, and those within an error range (June–August: ±0.25°C; February and November: ±0.1°C) are assumed to represent background river-water temperatures. A smaller error parameter was used for fall and winter data due to less fluctuation in temperature during these seasons. Measurements that fall outside of the error range are considered to be anomalies.
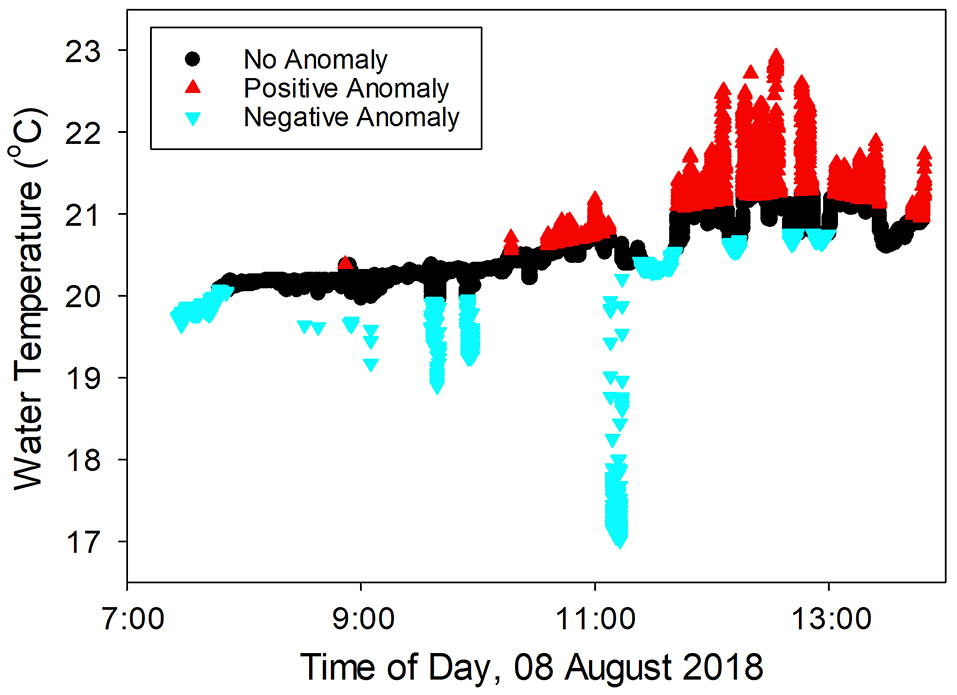
Figure 5. Example of background trends and anomalies from data collected on August 8, 2018. Temperature data is classified by relationship to the background trend identified by the loess model. Measured temperatures outside of this range are either marked as positive or negative anomalies.
To identify anomalies in EC, we identified a threshold to separate anomalies from background river-water values for both shallow and deep sensors. We determined the threshold by using the median EC value (123.46 μS/cm) of the main river channel from measurements taken in the center of the river. The position of each measurement on either shoreline or the center was approximated during data processing using GPS coordinates and research notes. Values of EC below this threshold are background values, while measurements above this value are considered anomalies (Figure 6).
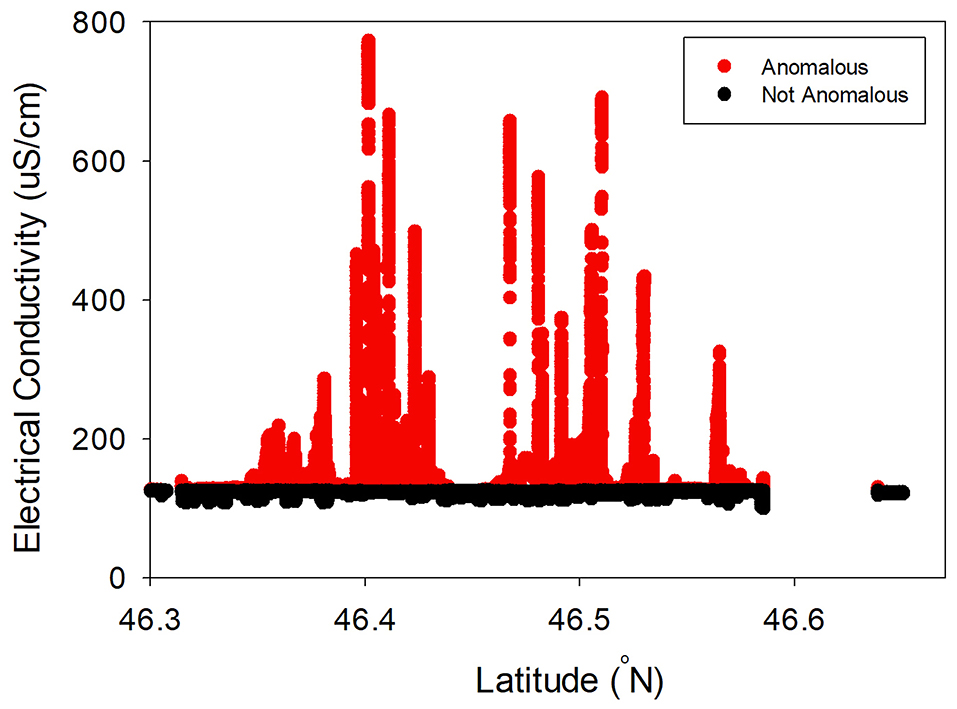
Figure 6. Example of EC anomalies determined from EC values measured during the summer, plotted in space (North to South along the river). Values below the median indicate values similar to river water (Categories 3 and 4), while those above the median indicate comparatively more saline inflows (Categories 1 and 2).
Results
Seasonal Variation of Anomalies
Water temperature anomalies of the riverbed demonstrated a surprising shift across months/seasons of 2018 (Figure 7). In February and July, the majority of anomalies had a higher temperature relative to the river (89 and 60%, respectively). In June, all identified anomalies had low temperatures relative to the river water column. In August, low temperature anomalies composed the majority (53%) of those observed, although only a 6% difference between the frequency of high temperature and low temperature anomalies was identified. Low temperature anomalies also were the majority of identified anomalies in November (60%).
Riverbed EC anomalies, relative to the river water also varied between months (Figure 7). In February and November, when irrigation does not occur, the majority of identified anomalies had high EC (68 and 57%, respectively). The majority of anomalies in August were also those with high EC (67%). However, in June and July, EC measurements that were not anomalous made up the majority of the measurements (55 and 60%, respectively).
After we determined anomalies in temperature and EC, locations were categorized based on the categories determined in Table 1. In 2018, the abundance of each category of anomaly changed across the seasons (Figure 8), and the category with the largest percentage of anomalies identified in each month varied. In February, Category 2 (high EC; high temperature) was the most commonly identified anomaly type (57%). In June, Category 3 (not anomalous EC; high temperature) was the most commonly identified anomaly (54%). In July, Category 4 (not anomalous EC; low temperature) was the most commonly identified (41%), and in August and November the majority returned to Category 2 (44 and 40%, respectively). At no time was Category 1 (high EC; low temperature) the majority of observed anomaly type. During February and June, Category 4 (not anomalous EC; low temperature) anomalies were identified as 0% of the anomalies identified, as was Category 1 (high EC; low temperature) in June. In February, no category 2 (high EC; low temperature) anomalies were observed, and in June, no Category 1 (high EC; low temperature) or 3 (not anomalous EC; high temperature) anomalies were observed.
Spatial Distribution of Anomalies
Locations of anomalies found along shorelines were more dispersed and smaller in July (Figure 9) than in August (Figure 10). In particular, Category 1 (high EC; low temperature) and Category 2 (high EC; high temperature) anomalies were clustered in a few locations in early July, whereas in early August, they were distributed along the much more of the length of river between Savage Island and Richland. Category 3 (not anomalous EC; high temperature) and Category 4 (not anomalous EC; low temperature) anomalies showed less of an increase in spatial distribution between early July and early August but did increase in distribution. The increased distribution of Category 3 (not anomalous EC; high temperature) was most evident near the 300-Area and the increase in distribution of Category 4 (not anomalous EC; low temperature) was particularly evident west of Savage Island. Anomalies were also more frequently identified on the eastern shoreline of the river (72% of total) than the western shoreline (28% of total) during July and August.
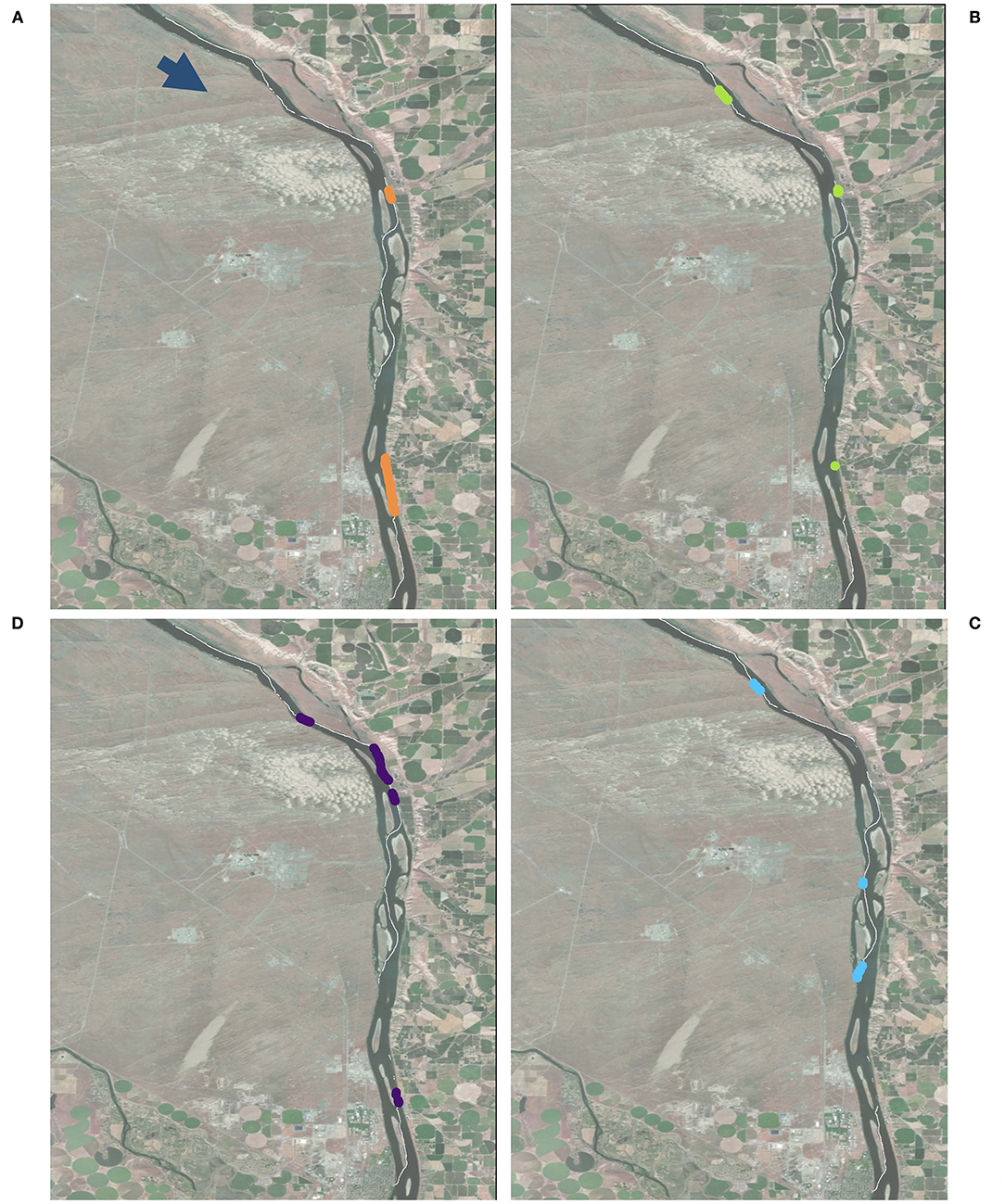
Figure 9. Anomalies found July 3–17 that were (A) Category 1 (high EC; low temperature), (B) Category 2 (high EC; high temperature), (C) Category 3 (not anomalous EC; high temperature), and (D) Category 4 (not anomalous EC; low temperature) are all present during this time range, but each category of anomaly is only present in a few locations. The white line shows all the paths traversed during this time period. Map data from Google, Landsat.
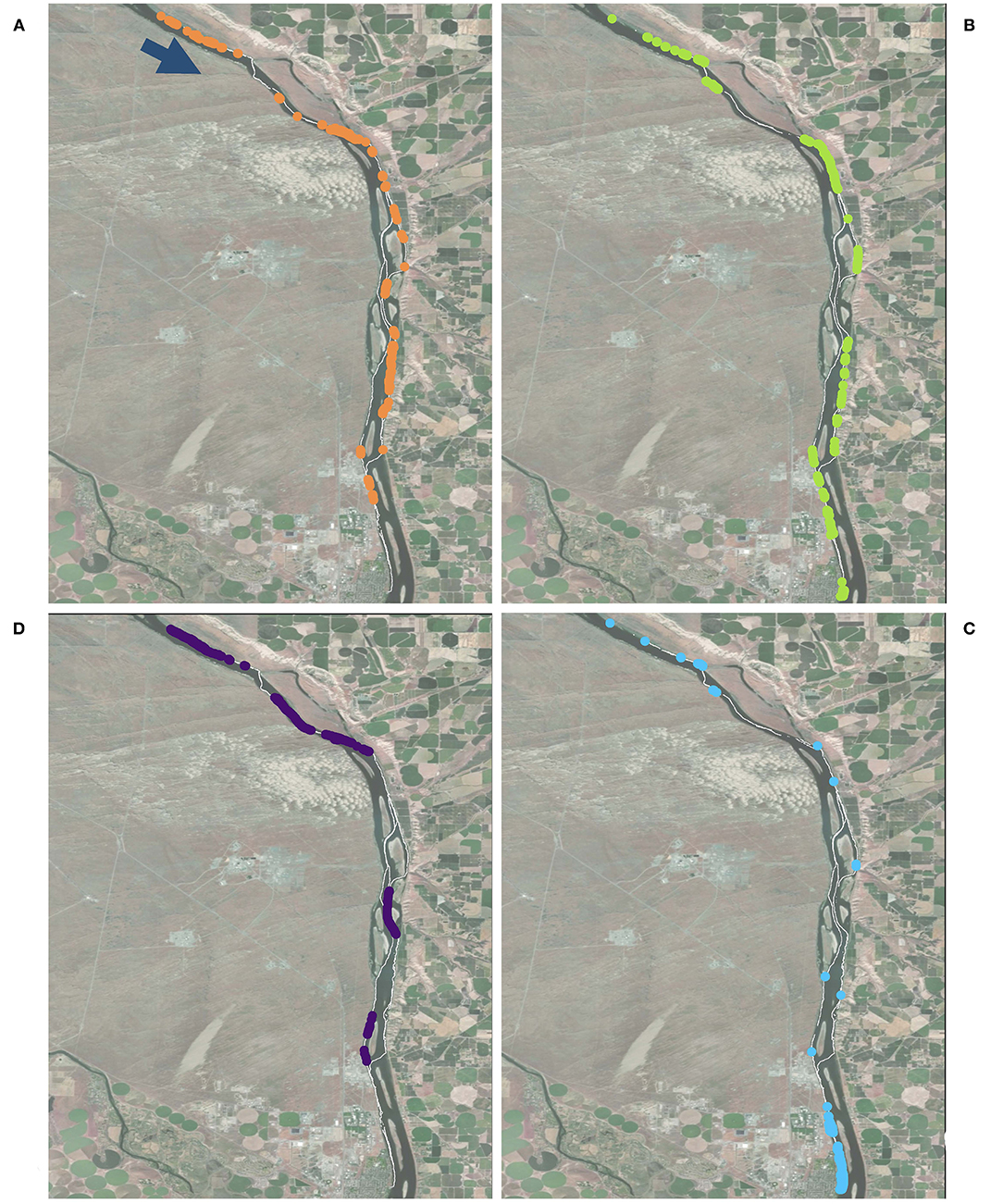
Figure 10. Anomalies observed from August 1–14 that were (A) Category 1 (high EC; low temperature), (B) Category 2 (high EC; high temperature), (C) Category 3 (not anomalous EC; high temperature), and (D) Category 4 (not anomalous EC; low temperature) are all present during this time range, but each category of anomaly is only present in a few locations. Category 3 anomalies are clustered near the southern end of the study site, and Category 4 anomalies are clustered near the northern end of the study site. The white line shows all the paths traversed during this time period. Map data from Google, Landsat.
The US Geological Survey has instrumented an agricultural water return channel (USGS 12473503) on the eastern bank of this segment of the Columbia River. Collected parameters include specific conductance and water temperature, among others. The station initiated data collection in April 2019. For the 2019 water year, specific conductance ranged from 369 to 582 μS/cm, temperature ranged from 9.3 to 26.5°C. The peak temperatures observed in this wasteway are higher than we observed in our data collection campaigns, though the conductance is lower than the peak values we observed (in excess of 600 μS/cm; Figure 6). It is worth noting that these data collection periods are different—our field campaigns completed in 2018, and the USGS started collecting data in 2019. However, the USGS data suggest that warm, high conductivity water is likely from irrigation sources.
Nitrate Concentration Correlation to Riverbed Anomalies
Dissolved nitrate concentrations observed in the river water column using the SUNA vary from 0 to 9.38 mg N–/L. Dissolved nitrate was observed along both shores of the river, though we measured the relatively high nitrate concentrations along the eastern shoreline of the reach. We identified three distributed clusters of nitrate measurements along the eastern shoreline. The most significant cluster of nitrate concentrations along the western shoreline is located near the 300-Area, where we observed the highest nitrate concentrations.
Here we highlight three locations of nitrate observation clusters. The first location is the western shoreline of the river adjacent to the 300-Area (Figure 11). Results show a cluster of Category 1 and Category 2 anomalies along this section and substantial nitrate concentrations along the 300 Area shoreline, despite the lack of irrigation on the adjacent shoreline. There is, however, a known plume of high-nitrate concentration groundwater adjacent to the river in this location (USDOE, 2010). While the western part of the river corridor here is not irrigated, it appears that the groundwater plume with high dissolved nitrate concentrations is indeed providing a strong identifiable signal of nitrate along the shoreline.
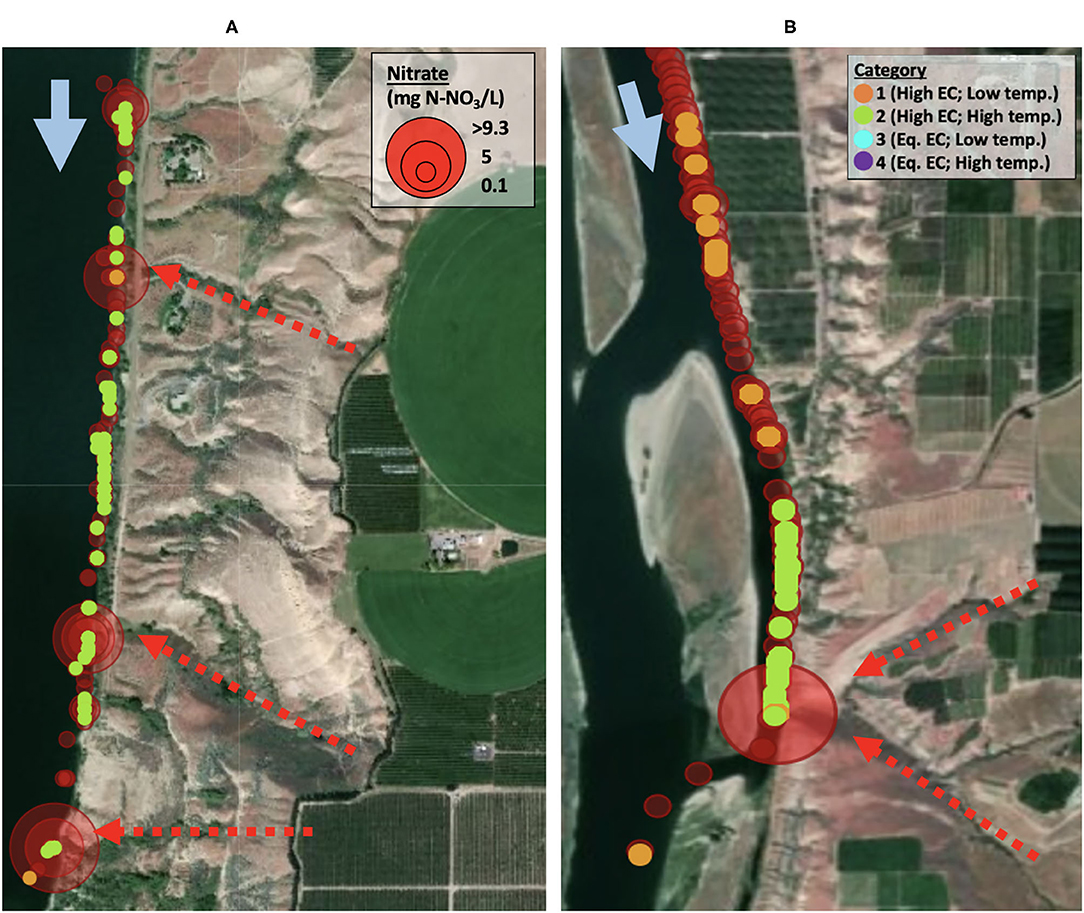
Figure 11. (A) Riverbed anomalies and (B) nitrate concentrations along the shoreline adjacent to the 300-Area of the Hanford Reserve. This shoreline is dominated by anomalies with high EC. The river water along the shoreline also has high nitrate concentrations. Map data from Google, Landsat.
A second area of interest, also along the western shoreline (where there is no irrigation of the lands) is across from Savage Island (Figure 12). Unlike the nitrate observations near the 300 Area, this location has a majority of Category 3 riverbed anomalies and generally low observed nitrate concentrations in August 2018.
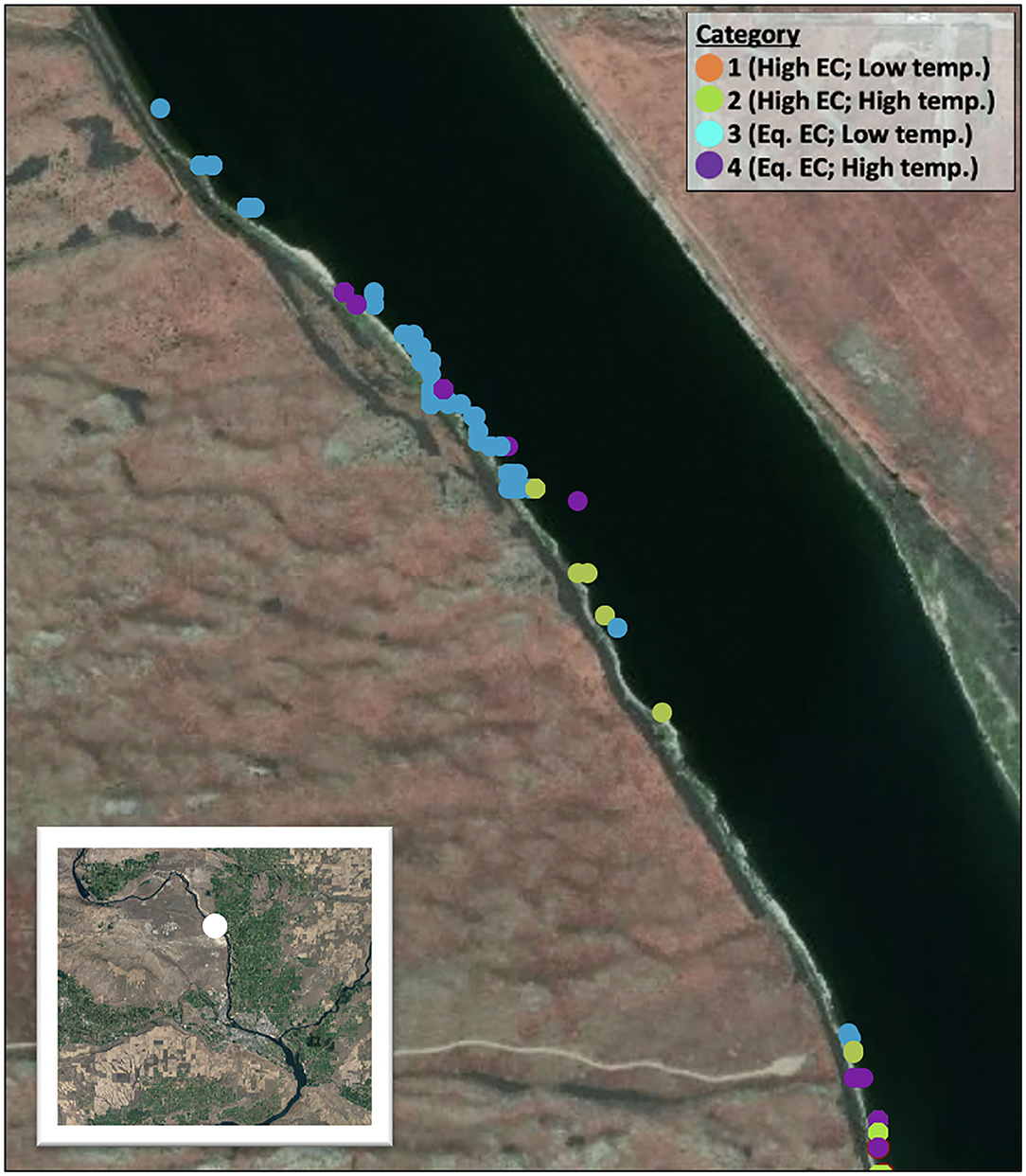
Figure 12. Anomalies along non-irrigated land. The anomalies shown along the western shoreline of the Columbia River next to Savage Island are dominated by Category 3 anomalies. There are also some instances of Category 4 anomalies. Map data from Google, Landsat.
Finally, the eastern shoreline adjacent to Taylor Flats is a heavily irrigated area and we observed the largest cluster of riverbed anomalies, suggesting extensive HEFs entering the river, and greatest extent of dissolved nitrate measurements (Figure 13). All riverbed anomalies along this shoreline are Categories 1 and 2—i.e., all associated with high EC. Several low-concentration nitrate observations occur in locations where no riverbed anomalies occur (i.e., no temperature or EC values abnormal from river water conditions). This may be the result of downstream transport from upstream inflows and inefficient mixing along the shoreline, or perhaps other HEFs return to the river here with EC and temperature signatures that are very similar to the river water (lateral hyporheic exchange perhaps). The largest nitrate concentrations along the eastern shore are coincident with riverbed anomalies.
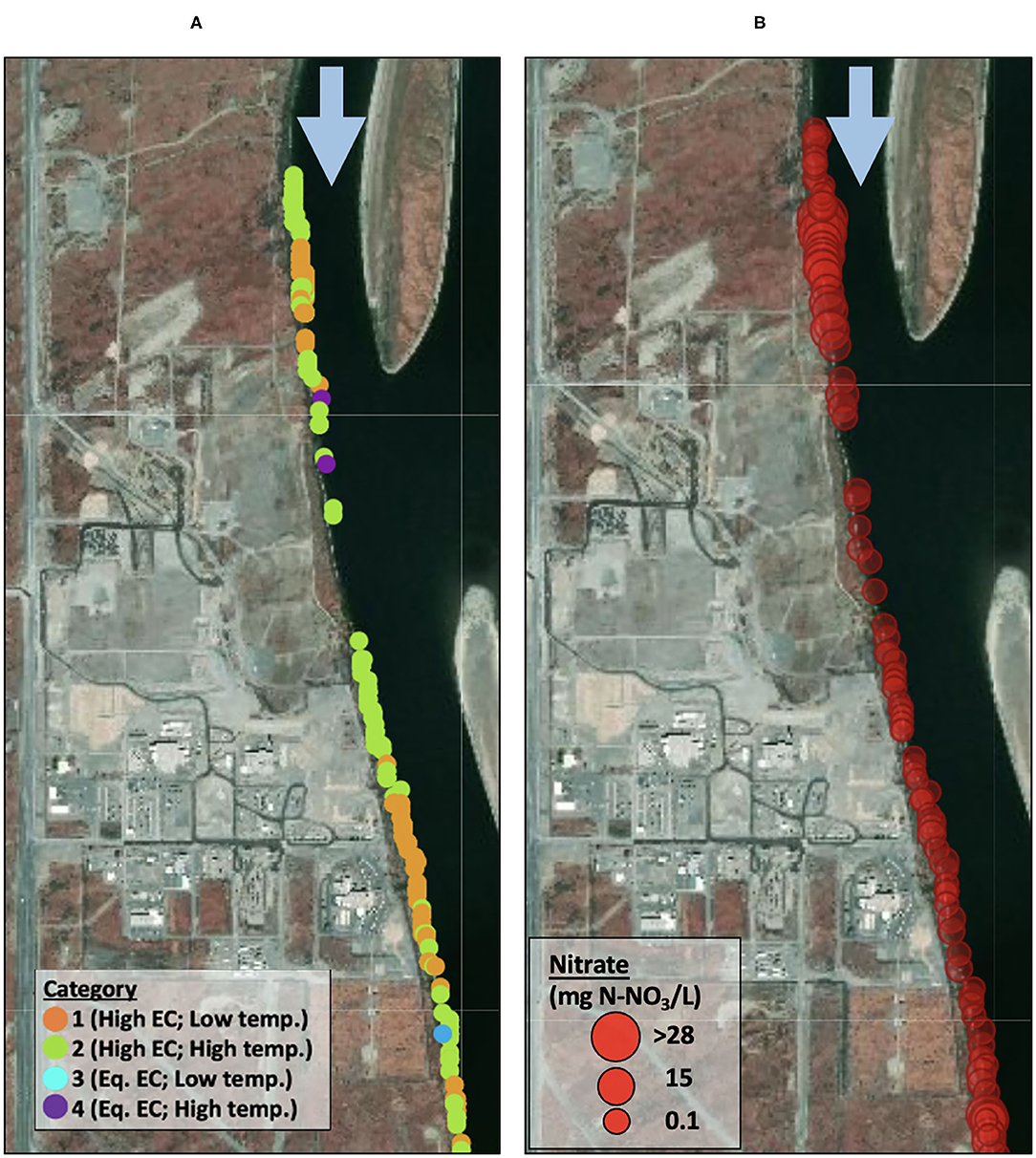
Figure 13. Nitrate concentrations and riverbed anomalies along irrigated eastern shoreline for (A) an area just upstream of Johnson Island, and (B) an area near Homestead Island. Potential surface flow paths from irrigated fields down to the river are indicated by red arrows. Map data from Google, Landsat.
Discussion
Seasonal Variation
The fluctuation in river flow impacts HEFs by changing the gradient between the river and adjacent groundwaters. River flow varies throughout the year, from its highest stage in the summer due to snowmelt, to its lowest stage in the fall at the end of the dry season (Figure 2). The annual fluctuation of the river flow creates a variation between conditions where the river is losing water to HEFs and conditions where the river is gaining water from HEFs in September due to hydraulic gradients (Shuai et al., 2019). Models suggest that the river is more frequently under losing conditions around the times of high annual flow, between approximately April and early June, and gaining conditions during the rest of the year (Zhou et al., 2018; Shuai et al., 2019). Diurnal variation in river stage also impacts HEFs, as water moves out of the river during high stages, and into the river during low stages. The identified anomalies agree with increases in the lower stage later in the summer, as many more inflows to the river were identified during early August compared to early July. During data collection in August and November, the stage of the river fluctuates the most dramatically of any time of the year. This fluctuation of up to 3 m at the stage logger below Priest Rapids Dam causes surface water intrusion into the groundwater (Johnson et al., 2012; Song et al., 2018; Shuai et al., 2019). During February data collection, the river is expected to be losing water, although less so than late summer.
Spatial Distribution of Anomalies, Nitrate, and Nearby Land Use
One cluster of anomalies on the west shoreline, adjacent to non-irrigated land, is identified west of Savage Island. Due to its accessibility, data were collected along this shoreline several times throughout the year, and serves as a representation of HEFs without irrigation influence. This shoreline is dominated by a cluster of Category 3 anomalies (not anomalous EC; higher temperature), with occasional Category 4 anomalies (not anomalous EC; lower temperature) and fewer Category 2 anomalies (higher EC; higher temperature). The majority of Category 3 and 4 anomalies in this location supports the prediction that these not anomalous EC categories of anomalies are associated with non-irrigated land use. The inflows along this shoreline create habitats with water warmer than river water (Category 2 and 3), and most likely surface-originating inflows. The occasional Category 4 anomalies along the shoreline are likely from groundwater seepage, as the hills lining the river in this location allow for natural pathways of groundwater seepage.
The irrigated eastern shoreline of the river showed a greater frequency of anomalies than the dry landscape of the western shoreline (Figure 13). The addition of irrigation water to the landscape, some of which is not used by crops, increases surface runoff and infiltration to groundwater. Of the ~3 billion cubic meters of water diverted from the Grand Coulee Dam within a year, much returns to the river, although there are no measurements of the total quantity of surface and groundwater return flow (USBR, 2014). In general, we observe high nitrate concentrations when we observe high EC (Supplementary Figure 1), however, there are several cases where high EC is not associated with high nitrate concentrations.
Not only is the frequency of identified anomalies greater on the eastern shoreline, the category of anomalies is different than on the western shoreline. On the eastern shoreline, Category 1 (high EC; low temperature) and Category 2 (high EC; high temperature) anomalies dominate the landscape. These anomaly categories are both associated with high EC relative to the river. High EC, indicative of high concentrations of dissolved solids in water, is a common occurrence in surface water and groundwater of irrigated landscapes, due to the influx of nutrients and pesticides from agricultural practices. However, high EC values do not indicate the source of the dissolved solids present in the water and may originate from soil types. Groundwater in the Hanford Reach is known to have naturally higher EC values than river water (Johnson et al., 2012). The corroboration of coincident nitrate concentrations in the water along the shoreline suggests that the high EC values can be attributed to irrigation runoff or associated seepage.
Although there are few nitrate concentration observations on the western shoreline, there are two clusters. One cluster of nitrate anomalies is located north of Richland, near the “300-Area.” The 300-Area was a site of plutonium development and is now the site of a known nitrate plume expanding south and east (USDOE, 2010). This groundwater plume is located in the same area as a cluster of nitrate anomalies along the shoreline, and so it is reasonable to assume this is the source of the nitrate observations clustered along the 300-Area shoreline. The other location of nitrate anomalies on the western shoreline is located west from Savage Island. This cluster is smaller than the one near the 300-Area and classified as Category 4 riverbed anomalies. There are no known contamination sites from Hanford in this area, nor irrigation adjacent to this shoreline. It is possible that contamination from upstream plumes travels through subsurface flow pathways and enters the river at this site.
The highest quantity of nitrate observations is located adjacent to irrigated lands along the eastern shoreline near Taylor Flats. The irrigated shoreline has high frequencies of Category 1 (high EC; low temp) and 2 anomalies, which are associated with high concentrations of nitrate. All nitrate observations are associated with riverbed anomalies categorized as Category 1 and 2.
The association of high-EC anomalies and nitrate near Taylor Flats suggests that part of the measured EC concentrations is a result of dissolved nitrate. The relationship between high-EC and high nitrate concentrations confirms our prediction that anomalies with high-EC originate from irrigation runoff. The presence of high-EC and high nitrate concentration measurements along shorelines that are adjacent to irrigated land suggests that there may be other agricultural-sourced solutes also entering the river through inflows. These may include dissolved phosphorus, pesticides, and insecticides. However, it is important to note that not all high-EC occurrences coincide with high nitrate concentrations (Supplementary Figure 1). Elevated nitrate concentrations could have been sourced from nitrification that may be associated with increased chemical weathering causing coincident high-EC conditions (Yue et al., 2015).
The data collected here suggest an interpretation of the riverbed categories identified using water temperature and EC (Table 2). In the summer season, when irrigation is occurring, we interpret Category 1 riverbed waters with low temperature and high EC to be associated with moderately deep groundwater that has a substantial irrigation water signature carrying increased solute loads including dissolved nitrate. We interpret Category 2 riverbed anomalies as those indicating very shallow or surface irrigation-sourced water that carry high concentrations of dissolved nitrate. We interpret Category 3 riverbed anomalies, having high temperature and not anomalous EC to the river water as those sourced from shallow or surface non-irrigation derived water, and therefore likely to have low nitrate concentrations. Finally, we interpret Category 4 riverbed anomalies with low temperatures and not anomalous EC to be indicative of relatively deep groundwater and therefore expected to have low dissolved nitrate concentrations.
High concentrations of nitrate measured in this reach are above toxic levels for salmon, but they are also very locally observed. Chinook salmon swim upriver to the Hanford Reach to return to their breeding grounds to spawn. Although adults have low nitrate sensitivity, likely due to the ameliorating effect of water salinity, eggs and young salmon (fry) are much more sensitive (Camargo et al., 2005). Chinook salmon fry experience a significant increase in mortality, known as nitrate toxicity, at 4.5 mg N–/L (Kincheloe et al., 1979). The maximum nitrate concentration measured in this study was 37.9 mg N–/L, more than nine times the reported lethal concentration. The localized high nitrate concentrations along the shoreline makes that particular habitat unsuitable for salmon fry.
In this study, we focused on the location and characteristics of the termini of HEFs and the expected correlation of the location of these to river-adjacent land use. These data and interpretations are useful for comparing to other groundwater flow modeling activities in the area and not anomalous related research. However, because we were not able to define the sources or entire paths of HEFs, which would have required extensive installation of wells and other subsurface interrogation, we rely on the correlation of nearby surface activities to subsurface conditions. Thus, additional study to better tie activities across the landscape to HEFs of the Columbia River (and other rivers) is warranted.
The goal of this study was to determine the impact of river corridor land use on river water quality. We used measurements of temperature and EC along the streambed to identify locations of water inflows. We demonstrate that along the Hanford reach, irrigation increases the spatial distribution of inflows into the river. The high concentrations of nitrate associated with irrigation-related HEFs indicate that agricultural land use on the east side of the river plays an important role in transferring nitrate to the river, at concentrations high enough to negatively interfere with the shoreline aquatic habitat. Future work could focus on the relative ages of groundwater in HEFs and the nitrate associated with them to evaluate how residence time and transport from source to river may be affecting the observed temporal and spatial patterns along the river shorelines.
Irrigation-influenced inflows were found to be most prevalent during late summer when river flows decrease. The large stage fluctuations from hydropower operations during late summer increase the gradients between groundwater/surface inflows and river water, increasing inflowing water. Since late summer is the driest time of the year, many of these exchanges involve irrigation runoff with high concentrations of nitrate.
Data Availability Statement
The datasets presented in this study can be found in online repositories. The names of the repository/repositories and accession number(s) can be found in the article/Supplementary Material.
Author Contributions
AC conducted all field research, conducted analyses, and wrote the first draft of the paper. MG and XC directed this research, performed some field data collection, guided field data collection and analyses, and helped to write the paper. EA provided field support and conducted some of the field data collection. VG-C analyzed samples and contributed to data analyses. All authors contributed to the article and approved the submitted version.
Funding
This material is based upon work supported by the Department of Energy, award grant numbers DE-SC0018165 and DE-SC0020339. Any opinions, findings, and conclusions or recommendations expressed in this material are those of the author(s) and do not necessarily reflect the views of the Department of Energy.
Conflict of Interest
The authors declare that the research was conducted in the absence of any commercial or financial relationships that could be construed as a potential conflict of interest.
The handling editor declared a past co-authorship with one of the authors MG.
Acknowledgments
This research was supported by the U.S. Department of Energy (DOE), Office of Biological and Environmental Research (BER), as part of BER's Subsurface Biogeochemical Research Program (SBR), grant nos. DE-SC0018165 and DE-SC0020339. Data were collected in collaboration with the SBR Scientific Focus Area (SFA) at the Pacific Northwest National Laboratory (PNNL). This paper describes objective technical results and analysis. Any subjective views or opinions that might be expressed in the paper do not necessarily represent the views of the U.S. Department of Energy or the United States Government. PNNL is operated for the DOE by Battelle Memorial Institute under contract DE-AC05-76RL01830.
Supplementary Material
The Supplementary Material for this article can be found online at: https://www.frontiersin.org/articles/10.3389/frwa.2021.574684/full#supplementary-material
Supplementary Figure 1. All nitrate observations compared to the coincident EC values measured in the shallow sensors (i.e., closest to the SUNA unit).
References
Arntzen, E. V., Geist, D. R., and Dresel, P. E. (2006). Effects of fluctuating river flow on groundwater-surface water mixing in the hyporheic zone of a regulated, large cobble bed river. River Res. Appl. 22, 937–946. doi: 10.1002/rra.947
Camargo, J. A., Alonso, A., and Salamanca, A. (2005). Nitrate toxicity to aquatic animals: a review with new data for freshwater invertebrates. Chemosphere 58, 1255–1267. doi: 10.1016/j.chemosphere.2004.10.044
Cardenas, M. B., and Markowski, M. S. (2011). Geoelectrical imaging of hyporheic exchange and mixing of river water and groundwater in a large regulated river. Env. Sci. Technol. 45, 1407–1411. doi: 10.1021/es103438a
Conner, A., Gooseff, M., and Chen, X. (2020). Boat-Dragged Riverbed and Mid-Column Water Quality along the Hanford Reach in the Columbia River Shorelines. Quantifying Distributed Exchanges of Groundwater with River Corridors. ESS Digital Repository.
Dirkes, R., Hanf, R., and Poston, T. (1999). Hanford Site Environmental Report for Calendar Year 1998. Pacific Northwest National Labs.
Fernald, A. G., Wigington, P. J. Jr., and Landers, D.H. (2001). Transient storage and hyporheic flow along the Willamette River, Oregon: Field measurements and model estimates. Water Resour. Res. 37, 1681–1694. doi: 10.1029/2000WR900338
Geist, D. R. (2000). Hyporheic discharge of river water into fall chinook salmon (Oncorhynchus tshawytscha) spawning areas in the Hanford Reach, Columbia River. Can. J. Fish. Aquat. Sci. 57, 1647–1656. doi: 10.1139/f00-102
Gilmore, T. E., Genereux, D. P., Solomon, D. K., Farrell, K. M., and Mitasova, H. (2016). Quantifying an aquifer nitrate budget and future nitrate discharge using field data from streambeds and well nests. Water Resour. Res. 52, 9046–9065. doi: 10.1002/2016WR018976
Gomez-Velez, J. D., Wilson, J. L., Cardenas, M. B., and Harvey, J. W. (2017). Flow and residence times of dynamic river bank storage and sinuosity-driven gyporheic exchange. Water Resour. Res. 53, 8572–8595. doi: 10.1002/2017WR021362
Harvey, F. E., Lee, D. R., Rudolph, D. L., and Frape, S. K. (1997). Locating groundwater discharge in large lakes using bottom sediment electrical conductivity mapping. Water Resour. Res. 33, 2609–2615. doi: 10.1029/97WR01702
Harvey, J., and Gooseff, M. (2015). River corridor science: hydrologic exchange and ecological consequences from bedforms to basins. Water Resour. Res. 51, 6893–6922. doi: 10.1002/2015WR017617
Hodson, M. E., and Donner, E. (2011). “Managing adverse soil chemical environments,” in Russell's Soil Conditions and Plant Growth, eds. P. J. Gregory and S. Nortcliff (Chichester: Wiley/Blackwell), 195–237.
Johnson, T. C., Slater, L. D., Ntarlagiannis, D., Day-Lewis, F. D., and Elwaseif, M. (2012). Monitoring groundwater-surface water interaction using time-series and time-frequency analysis of transient three-dimensional electrical resistivity changes. Water Resour. Res. 48, W07506. doi: 10.1029/2012WR011893
Kasahara, T., and Wondzell, S. M. (2003). Geomorphic controls on hyporheic exchange flow in mountain streams. Water Resour. Res. 39, 1005. doi: 10.1029/2002WR001386
Kiel, B. A., and Cardenas, M. B. (2014). Lateral hyporheic exchange throughout the Mississippi River network. Nat. Geosci. 7, 413–417. doi: 10.1038/ngeo2157
Kincheloe, J. W., Wedemeyer, G. A., and Koch, D. L. (1979). Tolerance of developing salmonid eggs and fry to nitrate exposure. Bull. Environ. Contam. Toxicol. 23, 575–578. doi: 10.1007/BF01770006
Lee, D. R., Geist, D. R., Saldi, K., Hartwig, D., and Cooper, T. (1997). Locating Ground-Water Discharge in the Hanford Reach of the Columbia River (PNNL-11516). United States Department of Energy.
Lenat, D. R., and Crawford, J. K. (1994). Effects of land use on water quality and aquatic biota of three North Carolina Piedmont streams. Hydrobiologia 294, 185–199. doi: 10.1007/BF00021291
Northwest Power Conservation Council (NPCC) (2019). Hanford Reach. Retrieved from: https://www.nwcouncil.org/reports/columbia-river-history/hanfordreach
Payn, R. A., Gooseff, M. N., McGlynn, B. L., Bencala, K. E., and Wondzell, S. M. (2009). Channel water balance and exchange with subsurface flow along a mountain headwater stream in Montana, United States. Water Resour. Res. 45, W11427. doi: 10.1029/2008WR007644
Shuai, P., Chen, X., Song, X., Hammond, G. E., Zachara, J., Royer, P., et al. (2019). Dam operations and subsurface hydrogeology control dynamics of hydrologic exchange flows in a Regulated River reach. Water Resour. Res. 55, 2593–2612. doi: 10.1029/2018WR024193
Song, X., Chen, X., Stegen, J., Hammond, G., Song, H.-S., Dai, H., et al. (2018). Drought conditions maximize the impact of high-frequency flow variations on thermal regimes and biogeochemical function in the hyporheic zone. Water Resour Res. 54, 7361–7382. doi: 10.1029/2018WR022586
USACE (2018) National Inventory of Dams US Army Corps of Engineers. Available online at: http://nid.usace.army.mil/cm_apex/f?p=838:4:0::NO (accessed June 19, 2020).
USBR (2014). The Story of the Columbia Basin Project. US Bureau of Reclamation. Available online at: https://www.usbr.gov/pn/project/brochures/columbiabasinproject.pdf
USDOE (2010). Hanford Site Groundwater Monitoring and Performance Report for 2009. US Department of Energy. Available online at: http://pdw.hanford.gov/arpir/index.cfm/viewDoc?accession=0084237 (accessed June 19, 2020).
Vaccaro, J. J., and Maloy, K. J. (2006). A Thermal Profile Method to Identify Ground-Water Discharge Areas and Preferred Salmonid Habitats for Long River Reaches. U.S. Geological Survey Scientific Investigations Report 2006–5136.
Woessner, W. (2000). Stream and fluvial plain ground water interactions: rescaling hydrogeologic thought. Ground Water 38, 423–429. doi: 10.1111/j.1745-6584.2000.tb00228.x
Yue, F., Li, S., Liu, C., Lang, Y., and Ding, H. (2015). Sources and transport of nitrate constrained by the isotopic technique in a karst catchment: an example from Southwest China. Hydrol. Process. 29, 1883–1893. doi: 10.1002/hyp.10302
Zachara, J. M., Chen, X., Song, X., Shuai, P., Murray, C., and Resch, C. T. (2020). Kilometer-scale hydrologic exchange flows in a gravel bed river corridor and their implications to solute migration. Water Resour. Res. 56, e2019WR025258. doi: 10.1029/2019WR025258
Zhou, T., Bao, J., Huang, M., Hou, Z., Arntzen, E., Song, X., et al. (2018). Riverbed hydrologic exchange dynamics in a large regulated river reach. Water Resour. Res. 54, 2715–2730. doi: 10.1002/2017WR020508
Keywords: stream-groundwater interaction, groundwater inflow, water quality, Columbia River, Hanford Reach, hydrologic exchange flows (HEFs)
Citation: Conner A, Gooseff MN, Chen X, Arntzen E and Garayburu-Caruso V (2021) Groundwater Inflows to the Columbia River Along the Hanford Reach and Associated Nitrate Concentrations. Front. Water 3:574684. doi: 10.3389/frwa.2021.574684
Received: 20 June 2020; Accepted: 22 February 2021;
Published: 08 April 2021.
Edited by:
Sarah E. Godsey, Idaho State University, United StatesReviewed by:
Si-Liang Li, Tianjin University, ChinaMegan J. Klaar, University of Leeds, United Kingdom
Copyright © 2021 Conner, Gooseff, Chen, Arntzen and Garayburu-Caruso. This is an open-access article distributed under the terms of the Creative Commons Attribution License (CC BY). The use, distribution or reproduction in other forums is permitted, provided the original author(s) and the copyright owner(s) are credited and that the original publication in this journal is cited, in accordance with accepted academic practice. No use, distribution or reproduction is permitted which does not comply with these terms.
*Correspondence: Michael N. Gooseff, michael.gooseff@colorado.edu