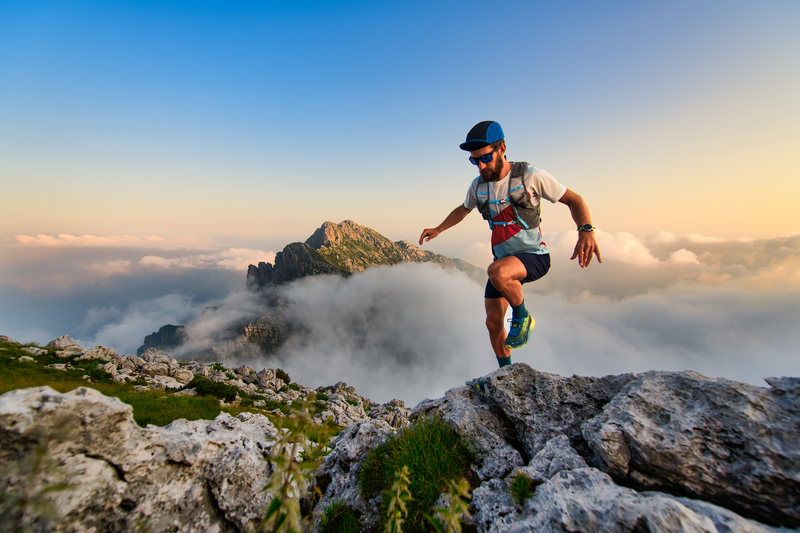
94% of researchers rate our articles as excellent or good
Learn more about the work of our research integrity team to safeguard the quality of each article we publish.
Find out more
ORIGINAL RESEARCH article
Front. Water , 28 January 2021
Sec. Water and Hydrocomplexity
Volume 2 - 2020 | https://doi.org/10.3389/frwa.2020.544847
This article is part of the Research Topic Innovative Methods for Non-invasive Monitoring of Hydrological Processes from Field to Catchment Scale View all 9 articles
Investigations of neutron transport through air and soil by Monte Carlo simulations led to major advancements toward a precise interpretation of measurements; they particularly improved the understanding of the cosmic-ray neutron footprint. Up to now, the conversion of soil moisture to a detectable neutron count rate has relied mainly on the equation presented by Desilets and Zreda in 2010. While in general a hyperbolic expression can be derived from theoretical considerations, their empiric parameterization needs to be revised for two reasons. Firstly, a rigorous mathematical treatment reveals that the values of the four parameters are ambiguous because their values are not independent. We found a three-parameter equation with unambiguous values of the parameters that is equivalent in any other respect to the four-parameter equation. Secondly, high-resolution Monte-Carlo simulations revealed a systematic deviation of the count rate to soil moisture relation especially for extremely dry conditions as well as very humid conditions. That is a hint that a smaller contribution to the intensity was forgotten or not adequately treated by the conventional approach. Investigating the above-ground neutron flux through a broadly based Monte-Carlo simulation campaign revealed a more detailed understanding of different contributions to this signal, especially targeting air humidity corrections. The packages MCNP and URANOS were used to derive a function able to describe the respective dependencies, including the effect of different hydrogen pools and the detector-specific response function. The new relationship has been tested at two exemplary measurement sites, and its remarkable performance allows for a promising prospect of more comprehensive data quality in the future.
Techniques for determining the environmental water content are mostly bound to local instrumentation or remote sensing products, neither of which meet the typical correlation lengths for soil moisture. This lack of spatial coverage makes the interpretation of available data difficult (Vereecken et al., 2008), and it is called the intermediate scale gap (Robinson et al., 2008). The method of Cosmic-Ray Neutron Sensing (CRNS) (Kodama et al., 1985; Zreda et al., 2008; Desilets, 2012) is a promising tool for hydrological and environmental applications, such as irrigation (Li et al., 2019), water resource management (Franz et al., 2016), and predictions of hydrological extremes like floods, droughts, and snow height measurements (Schattan et al., 2017, 2019). Its non-invasive nature in combination with a horizontal footprint radius in the order of 200 m (Köhli et al., 2015; Schrön et al., 2017) extending down to 75 cm (Desilets and Zreda, 2013) makes it attractive for hydrological modeling (Shuttleworth et al., 2013) and a large variety of hydrological applications. Moreover, the CRNS technique is increasingly applied in arid to semi-arid climates to support farming, irrigation, and hydro- and meteo services. CRNS is based on the principle that neutrons in the epithermal-to-fast energy range (1–105 eV) are highly sensitive to hydrogen, which turns neutron detectors into efficient proxies for changes of the environmental water content. It follows an inverse relationship between the above-ground epithermal-to-fast cosmic-ray neutron intensity N and the surrounding amount of hydrogen atoms, i.e., predominantly the volumetric water content θ (cm3/cm3). The originally proposed N0 method by Desilets et al. (2010)
included the fitting parameters ai. Bogena et al. (2013) further suggested to multiply it with the dry soil bulk density ϱbd in order to convert gravimetric to volumetric moisture. N has to be corrected for air pressure and incoming cosmic-ray variation, leading to the quantity Npi. A third correction factor Ch = 1 + 0.00054h is used to account for the water vapor in the air column above the sensor (Rosolem et al., 2013). One free calibration parameter N0 represents the intensity over dry soil at a reference location (Zreda et al., 2012). This transfer function from neutrons to soil moisture, however, has been developed for homogeneous soil and under idealized conditions, while its parameters were validated empirically from only a few measurements.
To date, many studies were carried out for finding a sensor calibration routine and to compare the performance to conventional instruments (Rivera Villarreyes et al., 2011; Franz et al., 2012a; Almeida et al., 2014; Coopersmith et al., 2014; Hawdon et al., 2014). The authors found a good agreement between measured neutron flux and soil moisture determinations. However, it was reported that unexplained features in the CRNS data could not be described by the Desilets equation (Desilets et al., 2010). Some authors explained the deviations by additional hydrogen pools or hydrological uncertainties (Franz et al., 2013a; Baatz et al., 2014; Baroni and Oswald, 2015). Others tried to fit the parameters of the hyperbola according to their data (Rivera Villarreyes et al., 2011; Lv et al., 2014; Heidbüchel et al., 2016; Sigouin and Si, 2016), which achieved a better correlation at the cost of site-specific calibrations. In their overview, Iwema et al. (2015) provided a comparison between existing N(θ) methods, especially at the Santa Rita site used in this work as well, finding that there is no conclusive solution for soil moisture retrieval. As shown in Rosolem et al. (2013), the Desilets equation remains not steep enough to consequently follow the change in intensity, particularly for dry conditions with soil moisture below 10%Vol.
The CRNS probe is usually mounted 1–2 m above the ground surface and equipped with two detection units—one bare counter for determining the thermal neutron flux and one counter enclosed by a moderator of 25 mm polyethylene. This makes the system most suited for rate changes in the epithermal-to-fast energy range. The energy sensitivity of the detector, the so-called response function, extends, however, into the thermal as well as the fast neutron regime (Köhli et al., 2018). Therefore, the moderated detector is partly sensitive to high energy neutrons, which partly accounts for the “road effect” (Schrön et al., 2018). It also suffers from the thermal neutron contamination that constitutes up to 20% of its signal. Both categories exhibit a different and much smaller dependence on the environmental hydrogen content than epithermal-to-fast neutrons. Desilets et al. (2010) and Andreasen et al. (2016), therefore, already suggested to disentangle the signals to provide a higher contrast. Although recent studies tried to make use of spectral information (Baatz et al., 2015; Tian et al., 2016) by comparing the two signals, the correlation between both signals and different environmental conditions is yet to be investigated in detail.
To assess the complex nature of neutron interactions, Monte-Carlo-based n-body simulations have proven to be the only efficient tool to support and interpret neutron observations in hydrology (Desilets et al., 2006; McKinney et al., 2006; Desilets and Zreda, 2013; Franz et al., 2013a; Shuttleworth et al., 2013). The first calculations for typical environmental conditions have been carried out by Zreda et al. (2008) in simplified domains using the MCNPX code (Waters et al., 2007). More precise calculations regarding the CRNS footprint for various scenarios with homogeneous domains have shown the complex neutron transport conditions (Köhli et al., 2015; Schrön et al., 2017).
Since the previously recognized approach is often based on site-specific parameters and shows weaknesses under dry conditions, we will fundamentally revisit the search for the relationship between water content and neutron count rate. The aim is to find a function that is as generally valid as possible, which combines all physically relevant processes, and which, generally formulated, gets by with as few free parameters as possible. In this context, we look at the relationship between neutrons and soil moisture as well as air humidity.
The scope of the paper is to develop an analytical intensity relation for various environmental conditions. The general shape of such a function is motivated theoretically and parameterized. With the help of neutron transport simulations, this model is fitted to the synthetic data sets and finally evaluated using timeseries from field sites.
The simulation toolkits used in this study are MCNP 6.2 (Werner et al., 2018) and URANOS (Köhli et al., 2015). MCNP (Monte Carlo N-Particle) is a general-purpose software that can simulate the propagation and interaction of neutrons, electrons, protons, pions, and others. Versions until MCNP4 (Briesmeister, 2000) were capable of simulating neutrons up to 20 MeV. Since the release of MCNPX (Waters et al., 2007), it is capable to simulate the propagation of particles in the Earth's atmosphere by extension of the energy range for many isotopes up to 150 MeV and some to GeV by using the continuously improved Cascade-Exciton Model (CEM) (Gudima et al., 1983) and the Los Alamos Quark-Gluon String Model (LAQGSM) (Gudima et al., 2001). MCNPX in particular was used in several studies to understand the CRNS signal (Desilets, 2012; Rosolem et al., 2013; Andreasen et al., 2016). With version 6 (Werner et al., 2018), the MCNPX branch was merged into the main development line featuring an optional cosmic-ray source (McKinney, 2013). The Monte Carlo code URANOS (Ultra Rapid Neutron-Only Simulation) was developed at the Physikalisches Institut, Heidelberg University, in collaboration with the UFZ Leipzig. This code has been specifically tailored to the needs of the CRNS method. It is based on a voxel engine and excludes any particles other than neutrons replacing them with effective models. Thereby, URANOS is a computationally efficient code that allows us to simulate the large environmental setups typically found in the context of CRNS on standard desktop computers. It uses the validated near-ground cosmic-ray neutron spectrum by Sato (2016). The code was employed for CRNS footprint revision by Köhli et al. (2015) and Schrön et al. (2017), in roving (Schrön et al., 2018) and irrigation studies (Li et al., 2019) as well as understanding the signal for snow height measurements (Schattan et al., 2019).
MCNP allows us to exchange the standard physics interaction cross-sections and also the use of different high-energy models. In this study, the standard databases ENDF/B-VII.1 (Chadwick et al., 2011) and ENDF/B-VIII.0 (Brown et al., 2018) were employed as well as JEFF 3.2 (Koning et al., 2011) and JENDL-4/HE (High Energy) (Shibata et al., 2011). URANOS couples to a combination of the ENDF/B-VII.1 and JENDL/HE 2007 (Watanabe et al., 2011) database. This JENDL/High Energy database includes cross-sections up to 3 GeV, but the data above 150 MeV were simply evaluated by JAM (Niita, 2002), an intra-nuclear cascade model. As this model leads to larger deviations for highest energies, in the JENDL-4.0/High Energy release the limit was set back to 200 MeV. This database was evaluated by CCONE (Iwamoto et al., 2016), which is a more sophisticated model compared to INCL (Boudard et al., 2013) and JAM but with many adjustable parameters based on experimental data.
The use of both toolkits enables the simulation of a wide range of typical environmental conditions, which have different effects at different stages of neutron transport. In MCNP we included the most relevant particles participating in the generation of hadrons, that is neutrons, protons, pions, and muons. We also implemented their typical energy spectra in order to achieve a representative spectrum. Protons are much less abundant at sea level, but they produce on average three neutrons. Muons are responsible for only a few percent of the neutron production; however, their attenuation length is twice as long.
The air medium consists of 78%Vol nitrogen, 21%Vol oxygen, and 1%Vol argon usually at a pressure of 1,020 mbar. The soil extends to a depth of 1.6 m and the air to 1,000 m. The vertical dimensions are chosen to cover the tracks for the relevant above-ground flux to at least 99.9%. Both soil and air are typically represented by planes of infinite extension, which can have subdomains, either to create an in-depth density profile or to add specific entities like water or a detector. The soil consists of 50%Vol solids and a scalable amount of H2O. The solid domain is comprised of 75%Vol SiO2 and 25%Vol Al2O3 at a compound density of 2.86 g/cm3. Thus, the total densities vary from 1.43 to 1.93 g/cm3 for 0%Vol and 50%Vol soil moisture, respectively. Chemical constituents regarding rock types are not relevant for the characteristics in the epithermal regime (Franz et al., 2012a; Zreda et al., 2012); however, the amount of chemically bound water in rocks lies in the order of a few percent. Cutoff rigidity and air pressure variations have not been studied and require an independent treatment, the latter being also analyzed in Köhli et al. (2015).
The input spectrum used in this work relies on the cosmic-ray propagation models by Sato and Niita (2006) and Sato et al. (2008), which are based on PHITS (Iwase et al., 2002) and PARMA (Sato et al., 2008). The latest version (Sato, 2015) provides an energy- and angle-dependent spectrum of cosmic-ray neutrons for a variety of altitudes, cutoff-rigidities, solar modulation potentials, and surface conditions. These simulations have been validated with various independent measurements, i.e., Goldhagen et al. (2004) and Gordon et al. (2004), at different altitudes and locations on Earth. Moreover, the analytical formulations of the spectra turned out to be effective in use for subsequent calculations. The presented energy-dependent flux ϕ(E) is described by a mean basic spectrum ϕB and a modifier fG for the geometry of the interface, which is defined by the ratio in comparison to a hypothetical spectrum of a semi-infinite atmosphere. In order to take into account air humidity effects, spectra were released at a height of usually 450 and 650 m for the simulations with atmospheric gradients.
In order to support the analytical relationship derived later in this work (15), we discuss and merge the basic theoretical concepts behind neutron transport and interaction.
It is important to realize that the diffusive spatial transport of epithermal neutrons in air follows an exponential law, which mainly determines the influence of air on neutrons. Considering a point source in an infinite medium, the integral version of the transport equation (Beckurts and Wirtz, 1964) reduces to a description of the radial flux Φ(r):
with Σt being the total cross section and Σs the scattering cross section for changes from E → E′ in the volume dV′. The first term describes the direct “geometric” transport without any collision from a source of strength Q to a surface proportional to r2. At larger distances the integration of the second term leads to the asymptotic solution of
with κ being a function of the ratio of the cross sections. The derivation can be found in Glasstone and Edlund (1952). In systems of weak absorption the absorption cross section Σa is much smaller than Σs. In other words the total cross section Σt is approximately the scattering cross section Σs and κ can be written as
In general such terms have to fulfill the diffusion equation, which can be described by a transport equation for the neutron balance in a specific volume:
Hence, in order to describe a plane or a volume source, Φ has to be described by terms for which the integration over the total volume in spherical coordinates dV = r2sin ϑdϑdϕdr converges. Therefore, terms in Φ involving exp(−r)/rn fulfill the norm for n ≤ 2. In the case of (2) with Φ ∝ exp(−r)/r2 and Φ ∝ exp(−r)/r this is satisfied. In general, solutions in the form of
can also be allowed within individual parameters describing a diffusion length and absorption-to-scattering ratios and overall source contributions Si, for example, for different energies. For a simple diffusion approach the resulting transport equation can characterize Φ by a sum of exponential functions. Such has been found in Köhli et al. (2015) and Schrön et al. (2017) with two terms, one describing a long-range transport from high energy neutrons mainly over the air and a second describing the transient near-field contribution. For a more complex configuration with a two-medium interface, a spectral range for the source emission energies and the detector acceptance energy and an exponentially described volume source there is no simple general solution using Fermi Age transport theory; nevertheless, the exponential range dependency of the footprint can be motivated by the approach presented here.
The mean logarithmic reduction of the neutron energy E per collision, ξ, is an important quantity in slowing-down theory that describes the rate of energy loss per interaction in the elastic scattering regime (Dobrzynski and Blinowski, 1994):
where A is the atomic mass number of the considered element. The logarithm represents the fact that, by elastic collisions, not an absolute quantity but always a fraction of the kinetic energy is lost. This formulation can be directly linked to the number of collisions, ncol, necessary to slow a neutron of energy E0 down to E1:
The variable u is called lethargy and ξ represents the average change in lethargy per collision. Following these relationships, it can be estimated that fast neutrons (≈ 106eV) need ≈ 18 collisions with hydrogen to get thermalized below 10−5eV, whereas collisions with large nuclei like iron take more than 500 collisions. This is the reason why the effect of metallic cases around the moderator of the detector is negligible. According to Equation (7), the lethargy is a property of a material and decreases with increasing nuclide mass. An overview of different atoms is provided in Table 1.
Table 1. Slowing down of neutrons by interaction with different isotopes from 2 MeV to thermal and to an exemplary energy relevant for CRNS.
For an inhomogeneous medium, the effective is an average of material-specific ξi weighted by their elastic cross sections σi:
In a macroscopic medium with the material density ϱ we can consider the macroscopic cross section, Σ = ϱ·σ. Hence, a typical ground medium can be described with the macroscopic cross sections of Σsoil plus a fraction w of added water Σwater:
Since the neutron flux Φel, epith in the relevant energy range of 0.5 eV to 0.5 MeV is proportional to the number of scatterings required for thermalization (ncol), we can conclude that the above-ground neutron intensity is inversely proportional to the water fraction w:
As a consequence, the relationship between above-ground neutron intensity and soil water content is hyperbolic and scales with the combined lethargy of soil and water. This concept is already expressed in the conventional N0 method (1) and will be also used for a revised approach in this work. The statements here are to be regarded as basic analytical approaches. They only apply to homogeneous transport problems. For the combination of different media interface effects have to be taken into account.
The cosmic-ray neutron spectrum (see Figure 1) exhibits a triple peak structure. The rightmost peak at ~100 MeV makes up neutrons generated in atmospheric cascades from mainly extrasolar particles or spallation reactions induced by protons in the upper atmosphere. The flux of these particles directed toward the ground is attenuated by several orders of magnitude. On their path, high-energy protons and neutrons also excite nuclei, which evaporate neutrons at energies around 1 MeV. Resonant nuclear excitations are responsible for the comb-like structure of this peak. Toward lower energies, elastic scattering becomes the dominant interaction as long as neutrons are epithermal. Due to the mass of hydrogen being nearly equal to that of the neutron, this following energy band is most sensitive to water and organic molecules and thus most relevant for the method of cosmic-ray neutron sensing. Below 1 eV the kinetic energy of the target, which is usually in thermal equilibrium (25 meV), significantly contributes to the neutron's energy during a collision. As a consequence, neutrons finally become thermalized and perform a random walk until they are absorbed.
Figure 1. Cosmic-ray neutron spectrum above dry ground. Three distinct peaks can be observed, which correspond to three physical processes. High-energy neutrons are generated in cascades in the upper atmosphere with energies up to several GeV. By interaction with atoms in the air or ground evaporation, neutrons are emitted with energies around 1 MeV. Finally, by elastic scattering, neutrons are slowed down until they are in thermal equilibrium with their environment. The total spectrum (black outline) can be separated into one part comprising albedo neutrons that have been in the soil (blue) and therefore carry soil moisture information and the incoming fraction (light blue), which can be considered a background. The spectrum which is emitted from the soil (“direct soil emission,” dark blue), mainly due to neutrons which have been generated in the ground, shows that the overall intensity is the result of several oscillations around the air-ground interface.
In the air, the mean free path for neutrons is ~1,000 times greater than in the soil. In an artificial scenario aiming to visualize the transport at the interface, a flux column is released onto the ground. By focusing such a “neutron beam” onto one spot, on the surface, the three-dimensional spatial distribution can be seen more directly, as the flux of the cosmic-ray neutron spectrum of Figure 1 is otherwise mostly omnidirectional. A rather dry condition is chosen in order to show a more spatially extended distribution. Figure 2 shows the tracks of all neutrons in the domain in three different energy regimes. Most high-energy neutrons entering the soil are scattered in a forward direction, and the possibility of leaving the ground is therefore considerably low; the exception is those originating from evaporation processes, which emit secondary particles nearly isotropically. However, only neutrons within the top few dozen centimeters below the interface border exhibit a significant probability of leaving. In general, this also leads to slant soil emission angles being suppressed. Epithermal neutrons below 1 MeV behave rather diffusively until they are moderated to thermal energies. As a first-order approach, one can expect neutrons to behave as a diffusive gas, as it was formulated by Glasstone and Edlund (1952), and applied to a footprint estimate by Desilets and Zreda (2013). But since every collision results in an energy loss for the neutrons, their mean free path between collisions changes and pure diffusion theory loses validity. The Fermi Age theory, e.g., applied in Barkov et al. (1957), accounts for these energy losses in a diffusive system, but analytical solutions exist only for mono-energetic particles and are not feasible for the cosmic-ray neutron spectrum exposed to a wide range of environmental conditions with different cross-sections. The cosmic-ray spectrum is partly also made up of neutrons slowed down in the air, which have a higher probability of being emitted back into the air. For thermalized neutrons, the soil can be regarded as a source. It can be explained by the fact that the moderation due to the presence of hydrogen is effective and no isotope with a large capture cross-section is present, unlike the case of air in which argon and especially nitrogen are comparably strong absorbers.
Figure 2. Flux calculation of an air-ground interface in which neutrons are artificially released centered straight down but with a CR spectrum according to Figure 1. The simulated neutron tracks from evaporation (MeV) to absorption (thermal) of 80·104 histories are displayed in a domain of 3 × 3 × 3 m with a track density scaling from dark blue to white (Köhli, 2019).
In order to analyze the above-ground neutron intensity relation to the environmental variables soil moisture θ and absolute air humidity h, simulations were carried out for a set of 11 × 11 values from θ= 1 to 50% and h = 1 to 35 g/m3 with 106–107 initial neutrons each. A detector layer was placed at a height of 1.3–1.5 m, and it records the tracks of neutrons passing through. The energy sensitivity of this layer can be adjusted. In this study the following two settings have been used: a fixed energy window (THL) scores neutrons from 1 eV to 10 keV and a more realistic approach, which employs the detector response function (drf) from Köhli et al. (2018). The discussion does not explicitly distinguish between incoming and albedo flux; however, in order to interpret the signal changes as a function of environmental variables, it is necessary to understand the transport paths of neutrons to the detector. Besides the fraction of incoming radiation, which acts as a background, there are three main types of transport processes: so-called geometric transport from the soil surface directly to the detector and typical mid-range transport of neutrons which cross the air-ground interface several times. The far-field transport can be understood as neutrons originating from a remote ground location and being transported mainly over the air with long path lengths. A set of such tracks is exemplarily visualized in the simulated detector of Figure 3.
Figure 3. Transport of cosmic-ray neutrons within a domain of 600 × 600 × 250 m. A detector is placed in the center at a height of 1.5 m. The tracks that are shown in blue correspond to 35 neutrons that probed the soil at some point on the plane and ended up in the detector volume. These exemplary tracks show typical paths neutrons travel until detection. Besides the importance of air as a transport medium it is noteworthy that most last scatterings occur in the direct vicinity of the sensor.
Neutron simulation toolkits provide very similar results for the well-understood physics below 20 MeV. Yet, there are differences with respect to the cross-section database and the high energy transport models. Most resources agree with each other and exhibit differences on the level of a few percent on the low energy cross-section. By far the best-known isotope is 1H with an uncertainty of 0.3%. In a first study, we compared different cross-section databases, which extend into the range above 20 MeV. However, as described in section 2, most of the necessary high-energy interactions are calculated by specific models. Therefore, the exchange of cross-section databases does not exclusively determine the result of a cosmic-ray neutron transport study. A broader overview of available toolkits can be found in Köhli (2019).
The ensemble of different cross-section databases with the same high-energy model (Table 2) leads to a relative variation of the predicted flux by 3% for a range of dry and wet conditions. The deviation of URANOS and MCNP6 is found to be ~2%, which amounts to a relative difference of 10%. There is a clear difference for both toolkits between the predicted flux for air humidity and soil moisture changes. URANOS produces an attenuation length in air, depending on the cutoff rigidity, of λair = 150–160 g/cm2, similar to MCNP6. Yet, the attenuation length in water is λwater ≈ 135 g/cm2. In MCNP6 for a neutron-only transport scenario one finds λwater ≈ 110 g/cm2, and if protons, pions, and muons are included, λwater ≈ 120 g/cm2. Cosmogenic nuclide studies, however, suggest values rather in the order of 130 g/cm2 (Nesterenok and Naidenov, 2012). As a result in MCNP6 high-energy neutrons are attenuated faster and the relative production of evaporation neutrons in the top soil layers is higher. This could lead to a larger difference in the flux between dry and moist conditions.
Taking into account the actual detector response function significantly reduces the predicted flux change, whereas the energy window method leads to a factor of ~4.5 for a soil moisture change from 1 to 50%, including the response function reduces this by 40% to a factor of about 3. This can be attributed to the fact, that the energy bands above and below the water-sensitive domain are less affected by environmental hydrogen changes (see Figure 1). The detector is susceptible to contamination by thermal neutrons, which scale differently with environmental water, and on the other hand, the evaporation peak includes more neutrons, which have never probed the soil (Köhli et al., 2018). A more comprehensive analysis of the detector response function can furthermore be found in Weimar et al. (2020).
As air humidity can be distributed vertically inhomogeneous in a second simulation set, a humidity profile with an e-fold length of 2.3 km was assumed according to Rosolem et al. (2013). The results are shown in Table 3. One finds, that using a vertically inhomogeneous atmosphere especially has an effect on the soil moisture scaling and less on air humidity variations. In that scenario, MCNP and URANOS agree well with each other for the predicted flux changes. However, it does not agree with the results of Rosolem et al. (2013), especially it is interesting to note that even with ten times larger statistics the MCNP6 runs still have larger uncertainties than shown in the referenced publication.
The relative reduction in neutron intensity at the surface for different soil moisture conditions when humid atmosphere layers are added is shown in Figure 4. The plots do not qualitatively change for simulations using the energy window settings or the detector response function and likewise not for URANOS or MCNP6. For humid compared to dry air the maximum achievable count rate is reduced by 20% in case of dry soil conditions. This quantitatively agrees with Rosolem et al. (2013) who studied the change from dry to 22 g/m3. However, a strictly linear relationship for water vapor cannot be verified. The presented reduction rate of 0.0054 per gram air humidity in Rosolem et al. (2013) seems to hold only for dry conditions. The scaling to moist soils is non-linear, as seen by the contour lines in Figure 4. Within the parameter space of this study, the relative intensity change for scaling water vapor lies in all cases in the order of 20% for 1 g/m3 → 35 g/m3 (see Table 2). This observation can be attributed to the fact that, for dry conditions, neutrons travel much longer paths and start with higher energies, both of which increase the transport through air, which, in case of a decreasing vertical humidity profile, is even amplified. The results presented in Figure 5 also show that using fixed upper and lower boundaries for scoring the neutron flux (energy window), the intensity scaling as a function of soil moisture is significantly higher. While the latter reduces the intensity by ~75% from dry to wet soils, using a detector response function reduces the measured flux by 65%. In Franz et al. (2013b) the authors already experimentally found a scaling factor of 2.5–3 between wet and dry conditions by comparing the data of ~ 40 COSMOS stations. This disparity could imply that further studies with fixed lower and upper energy boundaries would overstate intensity changes regarding soil moisture. Yet, applying the response function of a neutron detector using a shield, which absorbs more than 90% of the thermal neutron spectrum, yields a scaling between both cases. This study finds, based on Table 3, that the performance of CRNS detectors with regard to the measured intensity differences can be improved by at least 20% using such a thermal neutron shield. Although it reduces the overall measured flux, the improvement in the steepness of the I(θ) relation can be beneficial especially for moist conditions.
Figure 4. Above-ground neutron intensity as a function of air humidity and soil moisture simulated by URANOS applying a simulated detector response function. The contour lines show the extrapolated intensity change in steps of 5%. For dry soils air humidity has a stronger effect as neutrons travel over longer distances. The effect of water vapor is non-linear.
Figure 5. CRN intensity scaling for a simulation set with MCNP and URANOS in comparison with MCNP6, indicating a neutron-only simulation and MCNP6 full including all particle species. (Left) The intensity scaling with the shaded area indicating the variation from minimum to maximum air humidity shows the N0 method (1), the COSMIC forward operator and different simulations with flux recording set to fixed lower and upper bounds (energy window), or using a typical detector response function. (Right) The water vapor correction is compared to simulations of 1 and 50% soil moisture, which all use the detector response function. The UTS fit for URANOS is shown exemplarily.
The major outcome of this study is that the N0 method (1) is not steep enough to describe measurements, especially in dry regions. The hyperbolic characteristics reflect well local gradients, which is the reason why different adaptations of the parameters of this equation led to site-specific solutions. If the neutron intensity at a specific station does not change significantly, a locally adapted hyperbola like (1) can lead to an acceptable fit quality given the fact that there are most often unknown systematic errors. In different studies, including the literature cited here, typical calibration plots indicate a more steep I(θ) relation than can be achieved by (1). Especially when calibrated to rather moist conditions, the gradient from the Desilets equation is able to follow the simulations over a broad range of the variable space (see Figure 5 left). The reason is that the solution (15) for the above-ground neutron flux can require an additional exponential term (6), which leverages the intensity changes especially for dry conditions (see section 3). The possibility of such a description had already been indicated by Köhli (2019). COSMIC (Shuttleworth et al., 2013) relies on an exponential description for I(θ) and is able to better reproduce the intensity changes as can be seen for an exemplary evaluation in Figure 5. Yet, one can likewise ascertain a limited steepness for dry conditions. In conclusion, the findings here underline that for CRNS two important factors have to be taken into account. First, air humidity corrections are non-linear, yet the relative changes can be linearized, and second, the intensity scaling is much steeper than until now assumed based on the N0 method.
We have shown in section 3 that a hyperbolic formulation is reasonable to express the relationship between neutron intensity and soil moisture (see relation 11). The Desilets equation (1) satisfies this condition; however, it is mathematically overdefined. The four parameters (a0, a1, a2, and N0) are correlated, i.e., one of them is redundant. Any attempt to optimize or fit the parameters will lead to multiple, non-unique solutions as a hyperbola is defined by only three parameters. We believe that this is one of the reasons why different researchers found different parameter sets for their sites.
For a rigorous treatment, and to allow for unique fitting solutions, it is necessary to reduce the hyperbolic part to three parameters. We start from the Desilets Equation (1):
where the new parameters ã0, ã1, and Nmax can be expressed in terms of the classic parameters:
Here, Nmax is slightly larger than N0 and represents the absolute upper bound of the above-ground neutron flux. With these three, instead of four, parameters, this function is now uniquely defined and should be much better suited for calibration and optimization methods.
The inverse relation can be expressed as
As explained in section 3, there are reasons to assume a strong link between above-ground neutron flux and soil moisture as well as air humidity. For this reason, we propose to extend this function with physically reasonable terms that express this complex relationship. The intensity scaling in the water-sensitive domain can be very well-described by a hyperbolic expression like (14), which originates from the stopping power of the medium slowing down the neutrons as described in (11). An offset can be added that amounts for the 'incoming' part of the neutron spectrum. The diffusive spatial transport of neutrons in an absorbing medium however can be described by an exponential law like (6). These are the main effects that contribute to the above-ground neutron flux. As a rigorous analytical solution would be too complex, we use these findings as a mathematical structure and evaluate their different contributions by fitting this generalized approach to a simulation data set.
As Rosolem et al. (2013) have shown that the influence of water vapor, h, in the air column above the sensor can be expressed by a correction factor Ch, which is, in a first-order approximation, linear in h and especially accounts for the increased density. We found that the linear relationship is not enough to account for the changes in the very dry air regime, attributed to the long-range neutrons which probed the soil at distances of 100m and beyond. Those neutrons are exceptionally exposed to air humidity above the surface and also interact with the soil 2–3 times on their way to the detector (Köhli et al., 2015). Therefore, we propose a non-linear correction factor using at least a 2nd order Taylor expansion.
The equation in the final form becomes
This universal transport solution (UTS) is a general description of I(θ, h). The parameters pi are derived from a two-dimensional fit on simulated data sets (see also Table A1). The scaling constant ND accounts for the average specific detector count rate and can be determined with any combination of I, θ, and h. For soil moisture retrieval θ(I, h) has to be inverted numerically (e.g., using the Newton-Raphson method), which is beyond the scope of this work. UTS can be used with volumetric or gravimetric soil moisture by rescaling θ.
Every parameter set from different simulation settings for the presented function has its own justification depending on a specific site and detector conditions. It is beyond the scope of this work to conclusively clarify which function would be generally best suited for CRNS applications, as it would require a statistically sound study with a large set of data from various stations around the world.
We exemplarily pick two distinct sites to illustrate the general performance of the proposed UTS approach compared to the conventional relationship. The first site is the COSMOS station at the Santa Rita Experimental Range (SRER) in Arizona, US, which is exposed to a very dry climate with rapidly changing air humidity (Zreda et al., 2008; Franz et al., 2012b). For example, SRER provided the data basis of the works by Franz et al. (2013b) and Rosolem et al. (2013). SRER has also been used by Iwema et al. (2015) for a study concerning a new N(θ) approach since the N0 method was known to not perform well enough at this site. We would expect to see a stronger dependency of the neutron intensity on soil moisture and air humidity in very dry periods.
The second data set is from the Rollesbroich grassland test site in Central Europe, part of the TERENO Rur Observatory (Bogena et al., 2018) and with contrasting climatic conditions compared to SRES. Annual precipitation of 1,037 mm (2012) is distributed throughout the year, while evapotranspiration focusses on the months April to September and sums to 480 mm in 2012 (Gebler et al., 2015). Dry aboveground biomass is negligible with 0.2 kg/m2 (Baatz et al., 2015). The Rollesbroich test site features a network of 84 nodes with each three TDT (Time Domain Transmissometry) sensors installed in 5, 20, and 50 cm depth (Qu et al., 2016). The grassland is structured into several smaller fields which are in part and irregularly subject to management activities (mowing, manuring). Both soil moisture intensity conversions well represent soil moisture dynamics. The TDT data of both sites were weighted horizontally and vertically according to Schrön et al. (2017). In the rather wet and humid climate, we would expect no substantial difference to the performance of the conventional N0 approach, except for a slightly stronger influence of air humidity according to Figure 4.
The SRER data set, however, incorporates systematic uncertainties, which are significant at the level of the achieved precision. The supplement data provided by T. Franz compensates for the partial lack of environmental data by the sensor itself. However, there are small differences to the level 0 website data in relative air humidity and temperature as well as a noticeable pressure offset in 2011 by 2 mbar. With none of the in situ probes being closer to the sensor, the large contribution from the near-field remains unknown. As the uppermost TDT data is retrieved from a depth of 10 cm, the weighted soil moisture is dominated by this layer. Given that in deserts the largest dynamics can be observed at the surface, especially great signal deviations are therefore expected for hourly data. For the periods of extremely dry conditions the uncertainty on the lattice water, which can constitute half of the measured moisture, also becomes a relevant quantity. As there is no neutron monitor close to the experimental site or worldwide at the same cutoff rigidity we analyzed several stations statistically for their correction significance. We found that data from the NEWK station (Newark) slightly outperformed the data from the conventional JUNG station (Jungfraujoch), especially during Forbush decreases (Cane, 2000).
For each of the settings described in section 4.2, Equation (15) was fitted to the full set of simulation data with an atmospheric profile of water vapor in height h according to exp(−h/(2,300 m)). The resulting parameter sets are provided in the Table A1. UTS provides an excellent agreement with the data outperforming by far the hitherto used approach of the Desilets equation (1) with the water vapor correction (Rosolem et al., 2013) (see Figures 6, 7). It is especially interesting to notice that the short-term variation in the data seems to be entirely due to air humidity changes. This can also be concluded from the statistical analysis of hourly and daily accumulation intervals (see Table A1), in which the latter show much better agreement. In another parameter set (not shown) in which we doubled the air humidity scaling, we could achieve a better statistical significance. We therefore deduce that the water vapor changes at this site are much higher than actually measured by ground-based instruments. It could also mean that atmospheric profiles, at least under such extreme conditions, can play a role in precise soil moisture retrieval. An underestimation of the surface moisture dynamics might also be the reason why the energy window functions show a slightly better agreement to the measured neutron intensity. As the uppermost TDT probe depth is located 10 cm below the surface, the near-ground variations may enforce larger intensity fluctuations than is actually reflected by the data set. As URANOS and MCNP6 provide comparable results in order to analyze the best detector representation further sites will be necessary for testing.
Figure 6. The COSMOS site “Santa Rita” exhibits periods of extremely dry soil and air. The measured neutron flux Npi (black) was corrected for air pressure and incoming radiation. Daily aggregation is applied on all data. Soil moisture θ from the surrounding TDT network has been converted to neutron intensity using two approaches: (blue) the equation from Desilets et al. (2010) with the inverse water vapor correction from Rosolem et al. (2013), and (orange) the formula (15) presented in this work, using the parameter set “MCNP drf.” Kling-Gupta Efficiency (KGE), Nash-Sutcliffe Efficiency (NSE), and root mean square error (RMSE) are reported with respect to Npi. Note that the Desilets fit (blue) has been shifted by −200cph to better illustrate the poor performance toward dry conditions (i.e., high neutron counts).
Figure 7. Cutout section of the data sequence in Figure 6 with a temporal aggregation of 3 h for the summer period of 2013, where soil moisture is almost constant and the diurnal variations of air humidity are predominantly influencing the count rate. Note that the Desilets fit (blue) has been shifted by −200cph to better illustrate the poor performance toward dry conditions (i.e., high neutron counts).
The UTS approach proposed here shows significantly higher skill in soil moisture representation for all three measures, KGE, RMSE, NSE (Figure 8) compared to the traditional four-parameter approach. Several periods show varying performance but the “MCNP drf” approach is consistently closer to the observed reference neutron flux throughout most of the year. Short-term offsets, such as those in May and August, could be explained by unmonitored management. Considering high humidity and rather wet soils, the here proposed method based on “MCNP drf” is a promising advance to previous soil moisture neutron intensity conversions.
Figure 8. The TERENO site “Rollesbroich” features a humid climate with wet grassland soils. The measured neutron flux Npi (black) was corrected for air pressure and incoming radiation with data aggregated in daily intervals. Soil moisture θ from the surrounding TDT network has been converted to neutron intensity using two approaches: (blue) the equation from Desilets et al. (2010) with the inverse water vapor correction from Rosolem et al. (2013) and (orange) the formula (15) presented in this work using the parameter set “MCNP drf.”
In this study, we investigated the relationship between near-surface epithermal neutron intensity and water content in the soil and atmosphere. The analytical form has been derived from physical principles while the parameter sets were determined from neutron transport simulations for various types of model setups. We demonstrated the performance of our approach exemplarily at a dry and a wet instrument site using data from cosmic-ray neutron sensors and soil moisture monitoring networks.
A variety of modeling concepts have been evaluated using MCNP6 vs. URANOS, different cross-section databases, and different detector energy response functions (Tables 2, 3). MCNP simulations greatly benefited from the inclusion of protons and muons while they showed good agreement to URANOS on the level of 2–10%. The discrepancy might be attributed to the missing consensus about the effective attenuation length in water, which will be investigated in future research. The choice of the right detector response is crucial to the relationship between neutrons and soil moisture. For example, the dynamic range of N(θ) would be reduced by up to 40% using the detector-specific response function compared to the conventional energy-window approach. Additional shielding material would be able to exclude thermal neutrons and to partly restore this dynamic range.
The neutron response to air humidity has been investigated using homogeneous, exponential, and heterogeneous atmospheric profiles. Similar to Rosolem et al. (2013) we found that only the lowest 600 m are relevant for CRNS modeling. Our experimental results also suggest that complex atmospheric profiles could have previously undiscovered effects on CRNS measurements under dry conditions. Our simulations also suggest that the neutron response to water vapor depends on soil moisture itself. Hence, we recommend a non-linear correction approach as an alternative to the conventional method from Rosolem et al. (2013).
The hitherto accepted N(θ, N0) approach was found to be overdefined by one redundant parameter. This might be one of the reasons for the growing number of studies proposing site-specific parameter calibrations. Furthermore, our revised simulations with MCNP and URANOS showed a significantly steeper neutron response to soil moisture at the dry end. Based only on our simulations, we deduced a new universal transport solution (UTS, Equation (15)) that implicitly includes the correction for air humidity. The parameters only depend on the physical model used, except for the detector-specific scaling parameter ND. A reversed formulation, θ(I, h), could be performed numerically. We hope that this solution could contribute to a more general and unique sensor calibration.
Our new approach has been evaluated at dry and a wet site in Arizona (US) and Germany, respectively, which cover a wide range of soil moisture (1–50%Vol) and air humidity (1–25 g/m3). At both sites, the UTS led to significantly improved CRNS performance compared to the conventional Desilet's equation (e.g., KGE 0.60 → 0.97). Future studies are encouraged to investigate the performance of this approach on a larger number of locations. The UTS function can serve as a base description for further CRNS related studies, such as biomass effects or hydrological profiles, which look for rather small deviations from the overall signal.
The raw data supporting the conclusions of this article will be made available by the authors, without undue reservation.
MK and US wrote the manuscript. JW provided the MCNP simulations. MS and RB provided the experimental verification data. MS conducted the analysis on the field data. All authors contributed to the article and approved the submitted version.
URANOS was developed within the project Neutron Detectors for the MIEZE method and Forschung und Entwicklung hochauflösender Neutronendetektoren, funded by the German Federal Ministry for Research and Education (BMBF), grant identifier: 05K10VHA and 05K16PD1. The project Large-scale and high-resolution mapping of soil moisture on field and catchment scales boosted by cosmic-ray neutrons was funded within the DFG research group Cosmic Sense FOR 2694. The TERrestrial Environmental Observatory (TERENO) was funded by the Helmholtz Association and the Federal Ministry of Education and Research. We acknowledge financial support by the Baden-Württemberg Ministry of Science, Research and Arts and by Ruprecht-Karls-Universität Heidelberg.
The authors declare that the research was conducted in the absence of any commercial or financial relationships that could be construed as a potential conflict of interest.
The reviewer AG declared a past co-authorship with the authors MK and MS to the handling editor.
MK and MS acknowledge Trenton Franz for providing the extended Santa Rita data set and Lena Scheiffele for the weighting of the in situ soil moisture data.
Almeida, A., Dutta, R., Franz, T., Terhorst, A., Smethurst, P., Baillie, C., et al. (2014). Combining cosmic-ray neutron and capacitance sensors and fuzzy inference to spatially quantify soil moisture distribution. IEEE Sens. J. 14, 3465–3472. doi: 10.1109/JSEN.2014.2345376
Andreasen, M., Jensen, H. K., Zreda, M., Desilets, D., Bogena, H., and Looms, C. (2016). Modeling cosmic ray neutron field measurements. Water Resour. Res. 52, 6451–6471. doi: 10.1002/2015WR018236
Baatz, R., Bogena, H., Hendricks-Franssen, H.-J., Huisman, J., Montzka, C., and Vereecken, H. (2015). An empirical vegetation correction for soil water content quantification using cosmic ray probes. Water Resour. Res. 51, 2030–2046. doi: 10.1002/2014WR016443
Baatz, R., Bogena, H., Hendricks-Franssen, H.-J., Huisman, J., Qu, W., Montzka, C., et al. (2014). Calibration of a catchment scale cosmic-ray probe network: a comparison of three parameterization methods. J. Hydrol. 516, 231–244. doi: 10.1016/j.jhydrol.2014.02.026
Barkov, L., Makarin, V., and Mukhin, K. (1957). Measurement of the slowing down of neutrons in the energy range 1.46–0.025 eV in water. J. Nucl. Energy 4, 94–102. doi: 10.1016/0891-3919(57)90124-9
Baroni, G., and Oswald, S. (2015). A scaling approach for the assessment of biomass changes and rainfall interception using cosmic-ray neutron sensing. J. Hydrol. 525, 264–276. doi: 10.1016/j.jhydrol.2015.03.053
Beckurts, K., and Wirtz, K. (1964). Neutron Physics. Berlin; Heidelberg: Springer Berlin Heidelberg.
Bogena, H., Huisman, J., Baatz, R., Hendricks-Franssen, H.-J., and Vereecken, H. (2013). Accuracy of the cosmic-ray soil water content probe in humid forest ecosystems: the worst case scenario. Water Resour. Res. 49, 5778–5791. doi: 10.1002/wrcr.20463
Bogena, H., Montzka, C., Huisman, J., Graf, A., Schmidt, M., Stockinger, M., et al. (2018). The TERENO-Rur hydrological observatory: a multiscale multi-compartment research platform for the advancement of hydrological science. Vadose Zone J. 17:180055. doi: 10.2136/vzj2018.03.0055
Boudard, A., Cugnon, J., David, J.-C., Leray, S., and Mancusi, D. (2013). New potentialities of the Liège intranuclear cascade model for reactions induced by nucleons and light charged particles. Phys. Rev. C 87:014606. doi: 10.1103/PhysRevC.87.014606
Briesmeister, J. (2000). MCNP-A General Monte Carlo N-Particle Transport Code. Los Alamos, NM: Los Alamos National Laboratory.
Brown, D., Chadwick, M., Capote, R., Kahler, A., Trkov, A., Herman, M., et al. (2018). ENDF/B-VIII.0: The 8th major release of the nuclear reaction data library with CIELO-project cross sections, new standards and thermal scattering data. Nucl. Data Sheets 148, 1–142. doi: 10.1016/j.nds.2018.02.001
Cane, H. (2000). Coronal mass ejections and forbush decreases. Space Sci. Rev. 93, 55–77. doi: 10.1023/A:1026532125747
Chadwick, M., Herman, M., Obložinský, P., Dunn, M., Danon, Y., Kahler, A., et al. (2011). ENDF/B-VII.1 nuclear data for science and technology: cross sections, covariances, fission product yields and decay data. Nucl. Data Sheets 112, 2887–2996. doi: 10.1016/j.nds.2011.11.002
Coopersmith, E., Cosh, M., and Daughtry, C. (2014). Field-scale moisture estimates using COSMOS sensors: a validation study with temporary networks and leaf-area-indices. J. Hydrol. 519, 637–643. doi: 10.1016/j.jhydrol.2014.07.060
Desilets, D. (2012). “Probe background, technology, calibration, MCNP,” in Third COSMOS Workshop (Tucson, AZ).
Desilets, D., and Zreda, M. (2013). Footprint diameter for a cosmic-ray soil moisture probe: theory and Monte Carlo simulations. Water Resour. Res. 49, 3566–3575. doi: 10.1002/wrcr.20187
Desilets, D., Zreda, M., and Ferré, T. (2010). Nature's neutron probe: land surface hydrology at an elusive scale with cosmic rays. Water Resour. Res. 46:W11505. doi: 10.1029/2009WR008726
Desilets, D., Zreda, M., and Prabu, T. (2006). Extended scaling factors for in situ cosmogenic nuclides: new measurements at low latitude. Earth Planet. Sci. Lett. 246, 265–276. doi: 10.1016/j.epsl.2006.03.051
Dobrzynski, L., and Blinowski, K. (1994). Neutrons and Solid State Physics. New York, NY; London: Prentice Hall.
Franz, T., Wahbi, A., Vreugdenhil, M., Weltin, G., Heng, L., Oismueller, M., et al. (2016). Using cosmic-ray neutron probes to monitor landscape scale soil water content in mixed land use agricultural systems. Appl. Environ. Soil Sci. 2016:4323742. doi: 10.1155/2016/4323742
Franz, T., Zreda, M., Ferré, T., and Rosolem, R. (2013a). An assessment of the effect of horizontal soil moisture heterogeneity on the area-average measurement of cosmic-ray neutrons. Water Resour. Res. 49, 6450–6458. doi: 10.1002/wrcr.20530
Franz, T., Zreda, M., Ferré, T., Rosolem, R., Zweck, C., Stillman, S., et al. (2012a). Measurement depth of the cosmic ray soil moisture probe affected by hydrogen from various sources. Water Resour. Res. 48:8515. doi: 10.1029/2012WR011871
Franz, T., Zreda, M., Rosolem, R., and Ferré, T. (2012b). Field validation of a cosmic-ray neutron sensor using a distributed sensor network. Vadose Zone J. 11:vzj2012.0046. doi: 10.2136/vzj2012.0046
Franz, T., Zreda, M., Rosolem, R., and Ferré, T. (2013b). A universal calibration function for determination of soil moisture with cosmic-ray neutrons. Hydrol. Earth Syst. Sci. 17, 453–460. doi: 10.5194/hess-17-453-2013
Gebler, S., Hendricks-Franssen, H.-J., Pütz, T., Post, H., Schmidt, M., and Vereecken, H. (2015). Actual evapotranspiration and precipitation measured by lysimeters: a comparison with eddy covariance and tipping bucket. Hydrol. Earth Syst. Sci. 19, 2145–2161. doi: 10.5194/hess-19-2145-2015
Glasstone, S., and Edlund, M. (1952). The Elements of Nuclear Reactor Theory. Princeton, NJ; New York, NY: D. Van Nostrand Company.
Goldhagen, P., Clem, J., and Wilson, J. (2004). The energy spectrum of cosmic-ray induced neutrons measured on an airplane over a wide range of altitude and latitude. Radiat. Protec. Dosimetry 110, 387–392. doi: 10.1093/rpd/nch216
Gordon, M., Goldhagen, P., Rodbell, K., Zabel, T., Tang, H., Clem, J., et al. (2004). Measurement of the flux and energy spectrum of cosmic-ray induced neutrons on the ground. IEEE Trans. Nucl. Sci. 51, 3427–3434. doi: 10.1109/TNS.2004.839134
Gudima, K., Mashnik, S., and Sierk, A. (2001). User Manual for the Code LAQGSM. Los Alamos, NM: Los Alamos National Laboratory.
Gudima, K., Mashnik, S., and Toneev, V. (1983). Cascade-exciton model of nuclear reactions. Nucl. Phys. A 401, 329–361. doi: 10.1016/0375-9474(83)90532-8
Hawdon, A., McJannet, D., and Wallace, J. (2014). Calibration and correction procedures for cosmic-ray neutron soil moisture probes located across Australia. Water Resour. Res. 50, 5029–5043. doi: 10.1002/2013WR015138
Heidbüchel, I., Güntner, A., and Blume, T. (2016). Use of cosmic-ray neutron sensors for soil moisture monitoring in forests. Hydrol. Earth Syst. Sci. 20, 1269–1288. doi: 10.5194/hess-20-1269-2016
Iwamoto, O., Iwamoto, N., Kunieda, S., Minato, F., and Shibata, K. (2016). The CCONE code system and its application to nuclear data evaluation for fission and other reactions. Nucl. Data Sheets 131, 259–288. doi: 10.1016/j.nds.2015.12.004
Iwase, H., Niita, K., and Nakamura, T. (2002). Development of general-purpose particle and heavy ion transport Monte Carlo code. J. Nucl. Sci. Technol. 39, 1142–1151. doi: 10.1080/18811248.2002.9715305
Iwema, J., Rosolem, R., Baatz, R., Wagener, T., and Bogena, H. (2015). Investigating temporal field sampling strategies for site-specific calibration of three soil moisture–neutron intensity parameterisation methods. Hydrol. Earth Syst. Sci. 19, 3203–3216. doi: 10.5194/hess-19-3203-2015
Kodama, M., Kudo, S., and Kosuge, T. (1985). Application of atmospheric neutrons to soil moisture measurement. Soil Sci. 140, 237–242. doi: 10.1097/00010694-198510000-00001
Köhli, M. (2019). The CASCADE 10B thermal neutron detector and soil moisture sensing by cosmic-ray neutrons (Doctoral thesis), Physikalisches Institut, Heidelberg University, Heidelberg, Germany.
Köhli, M., Schrön, M., and Schmidt, U. (2018). Response functions for detectors in cosmic ray neutron sensing. Nucl. Instr. Methods Phys. Res. Sect. A Acceler. Spectrom. Detect. Assoc. Equip. 902, 184–189. doi: 10.1016/j.nima.2018.06.052
Köhli, M., Schrön, M., Zreda, M., Schmidt, U., Dietrich, P., and Zacharias, S. (2015). Footprint characteristics revised for field-scale soil moisture monitoring with cosmic-ray neutrons. Water Resour. Res. 51, 5772–5790. doi: 10.1002/2015WR017169
Koning, A., Bauge, E., Dean, C., Dupont, E., Nordborg, C., Rugama, Y., et al. (2011). Status of the JEFF nuclear data library. J. Korean Phys. Soc. 59, 1057–1062. doi: 10.3938/jkps.59.1057
Li, D., Schrön, M., Köhli, M., Bogena, H., Weimar, J., Jiménez Bello, M., et al. (2019). Can drip irrigation be scheduled with cosmic-ray neutron sensing? Vadose Zone J. 18:190053. doi: 10.2136/vzj2019.05.0053
Lv, L., Franz, T., Robinson, D., and Jones, S. (2014). Measured and modeled soil moisture compared with cosmic-ray neutron probe estimates in a mixed forest. Vadose Zone J. 13:vzj2014.06.0077. doi: 10.2136/vzj2014.06.0077
McKinney, G. (2013). MCNP6 Cosmic and Terrestrial Background Particle Fluxes. Los Alamos, NM: Los Alamos National Laboratory.
McKinney, G., Lawrence, D., Prettyman, T., Elphic, R., Feldman, W., and Hagerty, J. (2006). MCNPX benchmark for cosmic ray interactions with the Moon. J. Geophys. Res. Planets 111:E06004. doi: 10.1029/2005JE002551
Nesterenok, A., and Naidenov, V. (2012). In situ formation of cosmogenic 14c by cosmic ray nucleons in polar ice. Nucl. Instr. Methods Phys. Res. Sect. B Beam Interact. Mater. Atoms 270, 12–18. doi: 10.1016/j.nimb.2011.09.026
Niita, K. (2002). QMD and JAM calculations for high energy nucleon-nucleus collisions. J. Nucl. Sci. Technol. 39, 714–719. doi: 10.1080/00223131.2002.10875198
Qu, W., Bogena, H., Huisman, J., Schmidt, M., Kunkel, R., Weuthen, A., et al. (2016). The integrated water balance and soil data set of the rollesbroich hydrological observatory. Earth Syst. Sci. Data 8, 517–529. doi: 10.5194/essd-8-517-2016
Rivera Villarreyes, C., Baroni, G., and Oswald, S. (2011). Integral quantification of seasonal soil moisture changes in farmland by cosmic-ray neutrons. Hydrol. Earth Syst. Sci. 15, 3843–3859. doi: 10.5194/hess-15-3843-2011
Robinson, D., Campbell, C., Hopmans, J., Hornbuckle, B., Jones, S., Knight, R., et al. (2008). Soil moisture measurement for ecological and hydrological watershed-scale observatories: a review. Vadose Zone J. 7, 358–389. doi: 10.2136/vzj2007.0143
Rosolem, R., Shuttleworth, W., Zreda, M., Franz, T., Zeng, X., and Kurc, S. (2013). The effect of atmospheric water vapor on neutron count in the cosmic-ray soil moisture observing system. J. Hydrometeorol. 14, 1659–1671. doi: 10.1175/JHM-D-12-0120.1
Sato, T. (2015). Analytical model for estimating terrestrial cosmic ray fluxes nearly anytime and anywhere in the world: extension of PARMA/EXPACS. PLoS ONE 10:e0144679. doi: 10.1371/journal.pone.0144679
Sato, T. (2016). Analytical model for estimating the zenith angle dependence of terrestrial cosmic ray fluxes. PLoS ONE 11:e0160390. doi: 10.1371/journal.pone.0160390
Sato, T., and Niita, K. (2006). Analytical functions to predict cosmic-ray neutron spectra in the atmosphere. Radiat. Res. 166, 544–555. doi: 10.1667/RR0610.1
Sato, T., Yasuda, H., Niita, K., Endo, A., and Sihver, L. (2008). Development of PARMA: PHITS-based analytical radiation model in the atmosphere. Radiat. Res. 170, 244–259. doi: 10.1667/RR1094.1
Schattan, P., Baroni, G., Oswald, S., Schöber, J., Fey, C., Kormann, C., et al. (2017). Continuous monitoring of snowpack dynamics in alpine terrain by aboveground neutron sensing. Water Resour. Res. 53, 3615–3634. doi: 10.1002/2016WR020234
Schattan, P., Köhli, M., Schrön, M., Baroni, G., and Oswald, S. (2019). Sensing area-average snow water equivalent with cosmic-ray neutrons: the influence of fractional snow cover. Water Resour. Res. 55, 10796–10812. doi: 10.1029/2019WR025647
Schrön, M., Köhli, M., Scheiffele, L., Iwema, J., Bogena, H., Lv, L., et al. (2017). Improving calibration and validation of cosmic-ray neutron sensors in the light of spatial sensitivity. Hydrol. Earth Syst. Sci. 21, 5009–5030. doi: 10.5194/hess-21-5009-2017
Schrön, M., Rosolem, R., Köhli, M., Piussi, L., Schröter, I., Iwema, J., et al. (2018). Cosmic-ray neutron rover surveys of field soil moisture and the influence of roads. Water Resour. Res. 54, 6441–6459. doi: 10.1029/2017WR021719
Shibata, K., Iwamoto, O., Nakagawa, T., Iwamoto, N., Ichihara, A., Kunieda, S., et al. (2011). JENDL-4.0: a new library for nuclear science and engineering. J. Nucl. Sci. Technol. 48, 1–30. doi: 10.1080/18811248.2011.9711675
Shuttleworth, J., Rosolem, R., Zreda, M., and Franz, T. (2013). The COsmic-ray Soil Moisture Interaction Code (COSMIC) for use in data assimilation. Hydrol. Earth Syst. Sci. 17, 3205–3217. doi: 10.5194/hess-17-3205-2013
Sigouin, M., and Si, B. (2016). Calibration of a non-invasive cosmic-ray probe for wide area snow water equivalent measurement. Cryosphere 10, 1181–1190. doi: 10.5194/tc-10-1181-2016
Tian, Z., Li, Z., Liu, G., Li, B., and Ren, T. (2016). Soil water content determination with cosmic-ray neutron sensor: correcting aboveground hydrogen effects with thermal/fast neutron ratio. J. Hydrol. 540, 923–933. doi: 10.1016/j.jhydrol.2016.07.004
Vereecken, H., Huisman, J., Bogena, H., Vanderborght, J., Vrugt, J., and Hopmans, J. (2008). On the value of soil moisture measurements in vadose zone hydrology: a review. Water Resour. Res. 44:W00D06. doi: 10.1029/2008WR006829
Watanabe, Y., Kosako, K., Kunieda, S., Chiba, S., Fujimoto, R., Harada, H., et al. (2011). Status of JENDL high energy file. J. Korean Phys. Soc. 59, 1040–1045. doi: 10.3938/jkps.59.1040
Waters, L., McKinney, G., Durkee, J., Fensin, M., Hendricks, J., James, M., et al. (2007). The MCNPX Monte Carlo radiation transport code. AIP Conf. Proc. 896, 81–90. doi: 10.1063/1.2720459
Weimar, J., Köhli, M., Budach, C., and Schmidt, U. (2020). Large-scale boron-lined neutron detection systems as a 3He alternative for cosmic ray neutron sensing. Front. Water 2:16. doi: 10.3389/frwa.2020.00016
Werner, C., Bull, J., Solomon, C., Brown, F., McKinney, G., Rising, M., et al. (2018). MCNP Version 6.2 Release Notes. Technical report, Los Alamos National Lab. (LANL), Los Alamos, NM, United States.
Zreda, M., Desilets, D., Ferré, T., and Scott, R. (2008). Measuring soil moisture content non-invasively at intermediate spatial scale using cosmic-ray neutrons. Geophys. Res. Lett. 35:L21402. doi: 10.1029/2008GL035655
Zreda, M., Shuttleworth, W., Zeng, X., Zweck, C., Desilets, D., Franz, T., et al. (2012). COSMOS: The COsmic-ray Soil Moisture Observing System. Hydrol. Earth Syst. Sci. 16, 4079–4099. doi: 10.5194/hess-16-4079-2012
The results for the χ2 minimization of the simulated data sets using (15) are presented in the following Table A1. Simulations for the parameter sets relied on soil with a bulk density of g/cm3. In order to rescale UTS to different local bulk densities it is necessary to convert soil moisture to and use θloc instead.
Keywords: Monte Carlo, neutron, soil moisture, air humidity, cosmic-ray neutron sensing, MCNP, URANOS
Citation: Köhli M, Weimar J, Schrön M, Baatz R and Schmidt U (2021) Soil Moisture and Air Humidity Dependence of the Above-Ground Cosmic-Ray Neutron Intensity. Front. Water 2:544847. doi: 10.3389/frwa.2020.544847
Received: 22 March 2020; Accepted: 30 November 2020;
Published: 28 January 2021.
Edited by:
Jianzhi Dong, United States Department of Agriculture, United StatesReviewed by:
Andreas Güntner, Helmholtz Centre Potsdam, GermanyCopyright © 2021 Köhli, Weimar, Schrön, Baatz and Schmidt. This is an open-access article distributed under the terms of the Creative Commons Attribution License (CC BY). The use, distribution or reproduction in other forums is permitted, provided the original author(s) and the copyright owner(s) are credited and that the original publication in this journal is cited, in accordance with accepted academic practice. No use, distribution or reproduction is permitted which does not comply with these terms.
*Correspondence: Markus Köhli, a29laGxpQHBoeXNpLnVuaS1oZWlkZWxiZXJnLmRl
†ORCID: Roland Baatz orcid.org/0000-0001-5481-0904
Disclaimer: All claims expressed in this article are solely those of the authors and do not necessarily represent those of their affiliated organizations, or those of the publisher, the editors and the reviewers. Any product that may be evaluated in this article or claim that may be made by its manufacturer is not guaranteed or endorsed by the publisher.
Research integrity at Frontiers
Learn more about the work of our research integrity team to safeguard the quality of each article we publish.