- 1Swartz Center for Computational Neuroscience, Institute for Neural Computation, University of California San Diego, La Jolla, CA, United States
- 2Department of Computer Science, University of Colorado Denver, Aurora, CO, United States
- 3Department of Clinical Engineering, Komatsu University, Komatsu, Japan
- 4Department of Mechanical and Control Engineering, Handong Global University, Pohang, Republic of Korea
Although the stereoscopic effect in 3D virtual reality (VR) space has been studied, its influence on motor performance, specifically how stereoscopic objects affect behavioral outcomes like reaching, remains unclear. Previous research has primarily focused on perceptual aspects rather than on how stereoscopic visual input impacts motor control at the behavioral level. Thus, we investigated the effects of stereoscopic objects in a VR environment on reaching performance, examining whether the stereoscopic effect of objects is a significant aspect enough to affect performance at the behavioral level. While doing so, we investigated young and older adults separately, as age is a critical factor influencing motor performance. Fourteen young and 23 older participants performed a reaching task in the VR space. The target objects were 2D and 3D, deviating from the initial position by 10 and 20 cm. The movement attributed to feedforward control was analyzed using end-point error and smoothness of movement. Our results revealed that older participants had significantly worse predictive control than young participants in the 3D long task, suggesting that the positions of 3D objects were more difficult to predict than those of 2D objects. Young participants showed a significant difference in smoothness between 2D and 3D objects, which was not observed in older participants. This may reflect the floor effect in older participants. Under the short-distance condition, neither group showed a significant difference, suggesting the ceiling effect by distance. We confirmed that the effect of stereoscopic objects was not drastic but it did hamper the reaching performance.
1 Introduction
The integration of perception and control is crucial for enhancing the performance of robotic systems in complex and dynamic environments. Immersive virtual reality (VR) enables users to experience a sense of presence (Rose et al., 2018; Kuhne et al., 2023; Alazmi and Alemtairy, 2024; Pallavicini et al., 2019), making it an ideal tool for studying motor function and control, which are critical for designing effective actuators in robotics. VR environments offer practical advantages in a three-dimensional (3D) settings (Knight and Titov, 2009; Anoffo et al., 2018; Setti and Csapo, 2021) while few studies have been conducted in a non-immersive two-dimensional (2D) environment (Tam et al., 2005). In general, the quality of VR can change user behavior (Lee et al., 2020; Hoffman et al., 2006; Rosa et al., 2016). Creating accurate VR environments helps us understand human motor control and perception.
Reaching has been widely used to evaluate motor function (Roby-Brami et al., 2003; Cirstea et al., 2003; Igarashi et al., 2011; Kim et al., 2020a) because it is a fundamental task in daily life and can include other complex movements. Deployed in VR environments, reaching performance has been evaluated to investigate the effect of different viewing medium (Subramanian and Levin, 2011; Lin et al., 2019) or different displays (Camporesi and Kallmann, 2016). These previous studies successfully indicated that reaching performance could vary depending on visual representation. However, a task for 2D movement does not necessarily need to adopt complex 3D environments. Although planar reaching movements can be easily represented on a monitor using simple cursors (Kim et al., 2019; Brière and Proteau, 2011; Onagawa et al., 2022; de Brouwer and Spering, 2022; Ikegami et al., 2022), the experience provided by VR involving 3D objects is fundamentally different.
The 3D space enables users to perceive more information than the 2D space, leading to different perceptions of visual complexity because of depth information (Li et al., 2020), greater emotional arousal caused by binocular disparity (Tian et al., 2021) and different grip performances that depend on the view angle (Keefe and Watt, 2017), than in 2D environments. Several factors improve the quality of the 3D space and are associated with the mode of 3D information processing by the brain. Although some of these factors contribute to a better appearance, they are not indispensable in enabling people to recognize a VR environment as a 3D space. Thus, as an indispensable factor, we focused on the stereoscopic effects of objects in this study, breaking the 3D environment down into several aspects that compose it. Some previous studies have reported that stereoscopic effects in VR environments are negligible in public speaking (Ling et al., 2012) and in acquiring knowledge about heart disease by watching 2D or stereoscopic heart models (Patel et al., 2021). Nonetheless, the evaluation indicators used were too abstract to investigate the sophisticated role of stereoscopic objects in general immersive VR environments, calling for an investigation based on behaviors in more general and basic tasks to determine whether stereoscopic properties should be considered for creating an immersive VR environment for motor function assessment.
Therefore, in this study, we investigated the effects of stereoscopic objects during a reaching task in a systematical way by observing behavioral performance, examining whether the stereoscopic effect of objects is a significant aspect enough to affect behavioral level. We hypothesized that the stereoscopic effect of reaching objects would influence the reaching performance. As the stereoscopic property is processed for depth perception in the brain (Backus et al., 2001; Guo et al., 2022), cognitive function should be related to the performance, linked to age as a critical factor for the function (Deary et al., 2009). Thus, young and older individuals were recruited separately for the experiment. We adopted 2D and 3D objects as the cursor and target in a reaching task requiring the same reaching trajectory. Moreover, we assessed distance to the target, which is associated with difficulty of a task (Fitts, 1954), as two conditions (long and short distances) for the experiment. We expect the results to determine rough effects of the factors.
2 Methods
2.1 Participants
Fourteen young (11 male and 3 female; age: 24.14 ± 1.41 (mean ± standard deviation) years, range, 22–26 years) and 23 older (11 male and 12 female; age: 76.83 ± 5.38, range, 69–88 years) participants were included in the study. None of the participants had any neurological disorder. All participants were healthy enough to perform the experiment. All participants provided informed consent before participating in the experiment. This study was approved by the Institutional Review Board of Handong Global University and followed the principles of the Declaration of Helsinki.
2.2 Experimental environment
Figure 1 shows the experimental environment. During the experiment, the participants sat on a chair and wore a VIVE head-mounted display (HTC Corporation, Taipei, Taiwan) to experience an immersive VR environment. The head-mounted display also featured headphones for enabling the participants to hear sounds in the VR space used during the experiment. The VR environment was developed using Unity, where we optimized quality settings to enhance participants’ perception and interaction with the objects. We set the texture quality to full resolution to maximize detail, and applied 4x multi-sampling anti-aliasing to smooth object edges, minimizing visual artifacts like jagged lines. Shadow resolution was set to high, with two shadow cascades and a shadow distance of 40 units to ensure accurate and detailed shadows. For stereoscopic depth, Unity’s default settings were applied, with an inter-pupillary distance (IPD) set to 64 mm. The HTC VIVE, however, automatically adjusted IPD for each participant based on individual calibration, and we did not manually measure or adjust this for the experiment. No participants reported discomfort or visual distortion, confirming the suitability of the setup. We used Single Pass rendering to optimize performance, with near and far clipping planes set to 0.3 and 1,000 units, respectively.
The participants gripped the HTC VIVE controller, which was attached to a plastic apparatus as physical restraint for planar movement, to generate purely planar movements on the table. On the VR screen, participants could see a yellow initial position indicator with a radius of 0.5 cm, a yellow target with a radius of 2.5 cm, and a white cursor with a radius of 0.5 cm. View of 2D and 3D objects was induced not by control of the head-mounted display but by the characteristic of the objects. Participants controlled the cursor in the VR space by moving the controller, and the cursor positions were recorded at a sampling rate of 90 Hz.
2.3 Experimental procedure
Before data collection, participants were given approximately 5 min to familiarize themselves with the VR environment. During this time, they were seated and instructed to move the controller freely in a 2D space on the table to become accustomed to the setup.
At the beginning of the trial, the location of the cursor was matched with that of the initial position indicator. The participants were instructed to move the cursor to reach the target by moving the VIVE controller after hearing a beep. In the final phase of the reaching task, they moved the cursor to decrease the distance between the target and the cursor. Once they stopped moving the cursor, they were instructed to maintain the final position of the cursor until another beep. The objects were slightly transparent; therefore, the participants could recognize the cursor that overlapped with the target. After the second beep (4 s after the first), the participants moved the cursor back to the initial position. This trial was repeated.
The experiment consisted of two conditions: the distance between the target and the cursor was 20 cm (long) or 10 cm (short), and the objects were 2D or 3D. The 2D objects moved as if objects in 3D space would be projected on a plane. In total, the participants performed four types of tasks (2D long, 2D short, 3D long, and 3D short) involving five trials for each task. 2D and 3D conditions have the same physical constraints imposed by the joystick. The two conditions only differed in visual representation.
2.4 Data analysis
For pre-processing, the positional data were filtered using a 5th-order lowpass Butterworth filter with a cut-off frequency of 10 Hz. We then defined the first sub-movement (reaching) as the interval of interest to be analyzed. In this experiment, the participants could reach the target because the cursor and target were visible, and no obstacles existed during the experiment. Therefore, we segmented the reaching trajectory of each trial based on the velocity profile. Based on the idea that the velocity profile forms bell shapes during arm reaching (Suzuki et al., 1997; Karniel and Inbar, 1997; Matsui et al., 2006), we regarded the moment at which the velocity was zero as an indicator of sub-movement. Thus, the time when the velocity was zero for the first time determined the first sub-movement that reflected reaching by feedforward control. Intervals that could be associated with visual feedback were excluded from the analysis. Trials were manually inspected and rejected only if they met one of two specific conditions: (1) the participant did not reach the target by the second beep, or (2) the participant moved at a nearly constant speed without deceleration, with speed remaining non-zero at the second beep. Trials with either of these conditions lacked evidence of the first sub-movement phase. Approximately 0.9% of the trials were discarded.
We used the following two parameters to assess reaching performance: end-point error and smoothness of movement. Because we considered a trajectory by feedforward control only, end-point error was directly used to evaluate the performance of the prediction. End-point error was defined as follows:
where
where
Subsequently, we compared participants in the young and older age groups for each task. Additionally, for each distance, we compared the performances of both age groups with the 2D and 3D objects separately. A two-sample t-test was performed using MATLAB R2022b (MathWorks Inc., Natick, MA, United States) for smoothness, as all groups passed the normality test (Lilliefors test, significance level = 0.01). The error parameter was analyzed using the Wilcoxon rank-sum test.
3 Results
First, we compared end-point errors using feedforward control between the young and older age groups. Figure 2 shows the mean end-point error while comparing the young and older age groups for each condition. For all tasks, the mean end-point error of the young group was less than that of the older group. However, the difference in error was significant (p < 0.001, effect size |r| = 0.59) only in the 3D long task, where the mean errors for the two groups were 0.009 and 0.032 [m], respectively. The errors in the 2D long task were 0.008 and 0.018 [m], respectively. Tasks that required a long distance were associated with a large difference between the young and older groups.
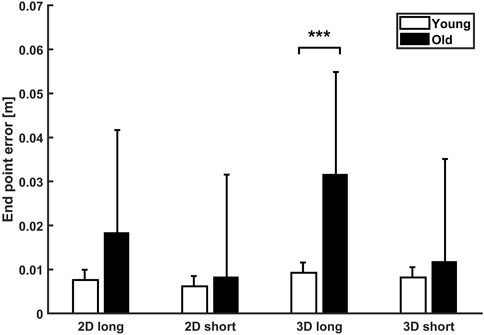
Figure 2. Comparison between the young and older groups in end-point error for each condition. Even though the young group showed a better performance than the older group in all tasks, the difference between the age groups was significant only in the 3D long task (p < 0.001, effect size |r| = 0.59). The differences in other tasks were not significant (p > 0.05). Error bar denotes standard deviation. ***p < 0.001, **p < 0.01, and *p < 0.05.
Figure 3 shows the smoothness of movement. In all tasks, the young group was significantly better in smoothness than the older group (p < 0.001). The Cohen’s d values for each task were 1.42, 1.57, 2.21, and 1.32, respectively. Consistent with end-point error, long tasks showed a significant difference. The values for smoothness in the young and older groups for the 2D objects were −1.507 and −1.743, respectively, and those for the 3D objects were −1.563 and −1.799, respectively. These data suggest that smoothness can be used for more accurate assessment of performance than end-point error.
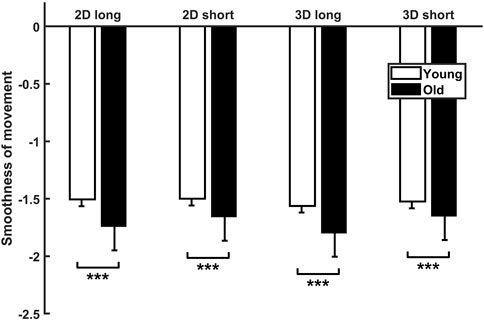
Figure 3. Mean smoothness of movement. In all tasks, the young group was significantly better in smoothness than the older group (p < 0.001, Cohen’s d = 1.42, 1.57, 2.21, and 1.32, respectively). Error bar denotes standard deviation. ***p < 0.001, **p < 0.01, and *p < 0.05.
Because we found that the smoothness parameter was appropriate for evaluating movement performance, we investigated whether stereoscopic objects would make a difference. Figure 4 shows the smoothness of movement in the young and older groups. Although the differences between 2D and 3D objects for the long tasks were larger than those for the short tasks, the difference was significant only for the long task by the young group (p < 0.05, Cohen’s d = 0.85). The mean smoothness in the long task was −1.506 (2D) and −1.563 (3D) for the young group and −1.743 (2D) and −1.799 (3D) for the older group. For both young and older groups, the difference in smoothness between 2D and 3D objects for the short task was almost negligible. No group showed a significant difference in the short task, suggesting the ceiling effect by distance.
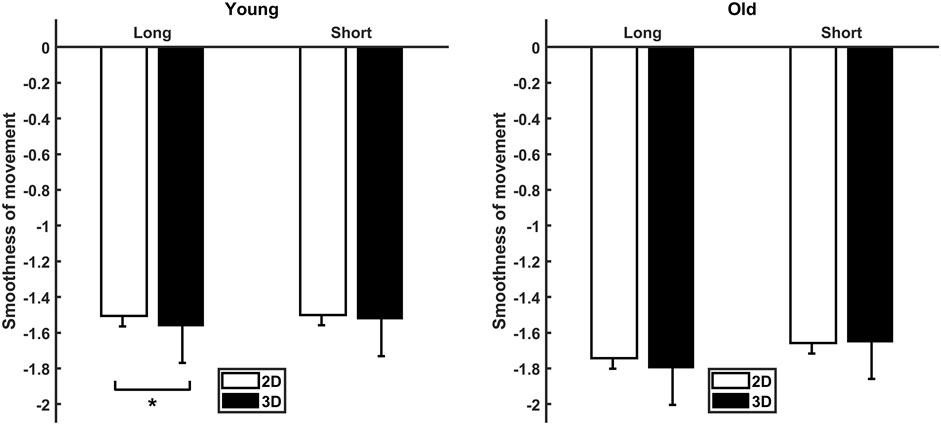
Figure 4. Comparison between 2D and 3D objects in smoothness. The left plot shows smoothness in the young group and the right plot shows smoothness in the older group. The short tasks showed a limited difference, while the long tasks contributed to larger differences. However, the difference was significant only for the young group (p < 0.05, Cohen’s d = 0.85). Error bar denotes standard deviation. ***p < 0.001, **p < 0.01, and *p < 0.05.
4 Discussion
In this study, we investigated whether stereoscopic objects affect reaching performance. Our results revealed that the older group had significantly worse predictive control than the young group in the 3D long task, suggesting that the positions of 3D objects were more difficult to predict than those of 2D objects. The young group showed a significant difference in smoothness between 2D and 3D objects, which was not observed in the older group. Given that older adults are reported to experience a decline in binocular vision and stereopsis, which are crucial for depth perception (Norman et al., 2009), the results for the older group may reflect a floor effect. Under the short-distance condition, neither group showed a significant difference, suggesting the ceiling effect by distance. We confirmed that the effect of stereoscopic objects was not drastic but it did hamper the reaching performance.
In a different situation, it was reported that an immersive stereoscopic picture impeded people from learning vocabulary and that learning with 2D images was more effective (Kaplan-Rakowski et al., 2022). The authors conjectured that the immersive nature of stereoscopic pictures was a distractor or drained some of the cognitive resources required for learning. Similarly, the perception of stereoscopic objects may necessitate additional information processing in the brain, which is not necessary when a 2D object is perceived. Considering this, prior experience with 2D or 3D devices could potentially influence participants’ performance. Although we did not collect information on participants’ prior exposure to these devices, it would be worthwhile for future studies to investigate whether such exposure affects performance. Several factors contribute to the perception of an object as stereoscopic. For example, shading is important for perceiving the shape of 3D objects (Ramachandran, 1988; Todd et al., 1997; Todd et al., 2023; Liu and Todd, 2004; Todd, 2004; Todd et al., 2014; Norman et al., 2004). The brain also uses binary disparity (Gonzalez and Perez, 1998; Murphy et al., 2016; Mitchison and Westheimer, 1984), motion parallax (Kim et al., 2016; Yoonessi and Baker, 2011), and textures (Ichihara et al., 2007; Thomas G. et al., 2002; O’Brien and Johnston, 2000) for depth perception, which are most likely involved in the perception of stereoscopic objects. In addition, the interaction effect among disparity, motion parallax, and shading was observed in a previous study (Schiller et al., 2011), indicating that the behavior of these factors is complicated, which makes it difficult to investigate their effects. Reaching performance can also be affected by irrelevant factors due to the added cognitive load of using virtual reality. It has been reported that simply adopting tasks where real movements are represented on a monitor, translated into a cursor on the screen, can induce a perceived delay that does not actually exist, even in non-immersive VR settings (Kim et al., 2020b, Kim et al., 2021). Considering this, the factors contributing to the floor effect in the older group observed in the present study remain elusive.
We considered movement over the interval using feedforward control in the analysis. Thus, the significant differences between the 2D and 3D objects indicate that stereoscopic information is involved in movement planning. When a reaching task performed in a 3D VR environment requires depth perception for successful performance, an extended Fitts’ law model that adds terms related to depth information to the traditional Fitts’ law model better predicts movements (Clark et al., 2020), which is associated with the relation between difficulty and target depth. The stereoscopic effect in this study may have served as an additional requirement in depth movement. Because a better performance was shown with 2D objects, visuomotor transformation during movement planning should not include the stereoscopic effect for better performance. Moreover, the difference in the stereoscopic effect may be attributed to visuomotor adaptation. The participants in the present study controlled the cursor on the screen using the controller. For visuomotor transformation, the brain must find an appropriate calibration model that matches the actual arm position to the cursor position in the VR space. In a previous study, a differently mapped cursor induced by visuomotor adaptation influenced depth perception (Volcic et al., 2013). Alterations in internal calibration caused by this classical visuomotor adaptation task may be intrinsically similar to the differences triggered by adding the stereoscopic effect. In addition, brain activities related to learning for visuomotor adaptation have been observed (Paz et al., 2003), supporting a neural basis. Another previous study reported that the brain may perform calibration suitable for binocular vision when monocular vision is input, leading to an underestimation of the distance in a reaching task (Servos, 2000). These incorrect internal calibration models may have been influenced by the stereoscopic effect.
What factors contributed to the age-related differences? Let’s revisit our aim and task. We aimed to observe behavior changes influenced by stereoscopic effects through a ballistic reaching task, which involves multiple cognitive processes. First, depth perception itself requires additional cortical processing, particularly in the visual cortex (Backus et al., 2001; Thomas O. M. et al., 2002; Smith and Parker, 2021). This processing becomes more demanding for older adults due to general declines in neural efficiency and adaptability. Furthermore, changes in binocular vision, such as near exophoria and reduced convergence ability, are common with aging (Leat et al., 2013), and studies have shown that binocular vision processing is altered in older adults (Yan et al., 2021). Such disturbances can significantly impact tracking performance, as seen in manual tracking tasks where performance is poorer under monocular vision compared to binocular (Kim et al., 2023). Aging is also associated with a decline in depth perception from binocular disparity (Norman et al., 2000; 2006). Collectively, these changes in visual processing, particularly in stereoscopic depth perception, contribute to the observed age-related differences in reaching performance. Beyond depth processing, aging also affects motor behavior through changes in sensory integration and control strategies. A critical factor is the increased reliance on visual feedback for motor tasks. Older adults tend to rely more on visual feedback (Seidler et al., 2010), which compensates for a decline in proprioceptive control (Boisgontier et al., 2012; Olszewska et al., 2016). Proprioceptive control is essential for effective feedforward control (Wolpert et al., 1995), enabling quick online corrections during reaching (Kasuga et al., 2022). Delayed responses in adjusting movements when the target position shifts suddenly (Sarlegna, 2006) suggest reduced use of proprioceptive input, which forces older adults to rely more on visual feedback for making corrections in reaching tasks. Given that our task emphasized feedforward control, similar to typical reaching actions in everyday life, these likely contributed to the observed differences in performance. Our findings on age-related differences in reaching performance align with previous research that observed a floor effect in tracking tasks, where older adults’ performance showed reduced sensitivity to depth-related visual information, likely due to age-induced constraints (Kim et al., 2024). Taken together, these findings indicate that changes in stereoscopic depth perception, combined with shifts in sensory integration and motor control strategies, could impact reaching performance in older adults. Our results suggest that the use of stereoscopic effects in VR-based tasks and interventions should be approached with caution, especially considering the age-related changes in visual and motor processing.
In this study, we confirmed the stereoscopic effect of objects in VR environments. However, we did not set sophisticated parameters to adjust the stereoscopic effect, as we initially aimed to determine a rough effect. We discovered the floor effect by age and the ceiling effect by distance. Additionally, movements through feedback control during ongoing reaching should be investigated in future studies. In this case, the effect of motion parallax could have affected the internal calibration. Naturally, users may subconsciously strive to estimate the distance between the objects and the virtual camera used for the first-person view implemented in the VR space. Thus, this distance should also be investigated in the future, including whether the perceived distance is identical to the actual distance between the target and the camera. Moreover, while we focused on two specific distances (10 cm and 20 cm) as proxies for task difficulty, future studies should investigate whether the stereoscopic effect generalizes across a broader range of distances. Furthermore, while we focused on young and older participants to capture distinct age-related differences, future studies could include middle-aged participants to explore potential regressive changes in movement planning or identify an inflection point in motor control across the lifespan. Additionally, future research should account for participants’ prior VR experience and systematically control IPD, as these factors may influence how participants interact with virtual environments. Including a group of experienced VR users could further provide valuable insights into performance differences across age groups. In conclusion, we confirmed that the effect of stereoscopic objects is not negligible in VR reaching tasks.
Data availability statement
The raw data supporting the conclusions of this article will be made available by the authors, without undue reservation.
Ethics statement
The studies involving humans were approved by the Institutional Review Board of Handong Global University. The studies were conducted in accordance with the local legislation and institutional requirements. The participants provided their written informed consent to participate in this study.
Author contributions
HK: Conceptualization, Formal Analysis, Methodology, Software, Validation, Visualization, Writing–original draft, Writing–review and editing. YK: Conceptualization, Formal Analysis, Methodology, Validation, Writing–review and editing. JL: Conceptualization, Methodology, Resources, Visualization, Writing–review and editing. JK: Conceptualization, Data curation, Formal Analysis, Funding acquisition, Investigation, Project administration, Resources, Supervision, Validation, Visualization, Writing–review and editing.
Funding
The author(s) declare that financial support was received for the research, authorship, and/or publication of this article. This work was supported by the National Research Foundation (NRF), Korea, under project BK21 FOUR (No. 5199990314060).
Conflict of interest
The authors declare that the research was conducted in the absence of any commercial or financial relationships that could be construed as a potential conflict of interest.
Publisher’s note
All claims expressed in this article are solely those of the authors and do not necessarily represent those of their affiliated organizations, or those of the publisher, the editors and the reviewers. Any product that may be evaluated in this article, or claim that may be made by its manufacturer, is not guaranteed or endorsed by the publisher.
References
Alazmi, H. S., and Alemtairy, G. M. (2024). The effects of immersive virtual reality field trips upon student academic achievement, cognitive load, and multimodal presence in a social studies educational context. Educ. Inf. Technol doi:10.1007/s10639-024-12682-3
Anoffo, Y. M., Aymerich, E., and Medda, D. (2018). “Virtual reality experience for interior design engineering applications,” in 2018 26th telecommunications forum (TELFOR), Belgrade, Serbia, 20-21 November 2018 (IEEE), 1–4. doi:10.1109/TELFOR.2018.8612026
Backus, B. T., Fleet, D. J., Parker, A. J., and Heeger, D. J. (2001). Human cortical activity correlates with stereoscopic depth perception. J. Neurophysiol. 86, 2054–2068. doi:10.1152/jn.2001.86.4.2054
Balasubramanian, S., Melendez-Calderon, A., and Burdet, E. (2012). A robust and sensitive metric for quantifying movement smoothness. IEEE Trans. Biomed. Eng. 59, 2126–2136. doi:10.1109/TBME.2011.2179545
Boisgontier, M. P., Olivier, I., Chenu, O., and Nougier, V. (2012). Presbypropria: the effects of physiological ageing on proprioceptive control. Age (Dordr) 34, 1179–1194. doi:10.1007/s11357-011-9300-y
Brière, J., and Proteau, L. (2011). Automatic movement error detection and correction processes in reaching movements. Exp. Brain Res. 208, 39–50. doi:10.1007/s00221-010-2458-1
Camporesi, C., and Kallmann, M. (2016). The effects of avatars, stereo vision and display size on reaching and motion reproduction. IEEE Trans. Vis. Comput. Graph. 22, 1592–1604. doi:10.1109/TVCG.2015.2440231
Cirstea, M. C., Ptito, A., and Levin, M. F. (2003). Arm reaching improvements with short-term practice depend on the severity of the motor deficit in stroke. Exp. Brain Res. 152, 476–488. doi:10.1007/s00221-003-1568-4
Clark, L. D., Bhagat, A. B., and Riggs, S. L. (2020). Extending Fitts’ law in three-dimensional virtual environments with current low-cost virtual reality technology. Int. J. Hum. Comput. Stud. 139, 102413. doi:10.1016/j.ijhcs.2020.102413
Deary, I. J., Corley, J., Gow, A. J., Harris, S. E., Houlihan, L. M., Marioni, R. E., et al. (2009). Age-associated cognitive decline. Br. Med. Bull. 92, 135–152. doi:10.1093/bmb/ldp033
de Brouwer, A. J., and Spering, M. (2022). Eye-hand coordination during online reach corrections is task dependent. J. Neurophysiol. 127, 885–895. doi:10.1152/jn.00270.2021
Fitts, P. M. (1954). The information capacity of the human motor system in controlling the amplitude of movement. J. Exp. Psychol. 47, 381–391. doi:10.1037/h0055392
Gonzalez, F., and Perez, R. (1998). Neural mechanisms underlying stereoscopic vision. Prog. Neurobiol. 55, 191–224. doi:10.1016/s0301-0082(98)00012-4
Guo, M., Yue, K., Hu, H., Lu, K., Han, Y., Chen, S., et al. (2022). Neural research on depth perception and stereoscopic visual fatigue in virtual reality. Brain Sci. 12, 1231. doi:10.3390/brainsci12091231
Hoffman, H. G., Seibel, E. J., Richards, T. L., Furness, T. A., Patterson, D. R., and Sharar, S. R. (2006). Virtual reality helmet display quality influences the magnitude of virtual reality analgesia. J. Pain 7, 843–850. doi:10.1016/j.jpain.2006.04.006
Ichihara, S., Kitagawa, N., and Akutsu, H. (2007). Contrast and depth perception: effects of texture contrast and area contrast. Perception 36, 686–695. doi:10.1068/p5696
Igarashi, K., Shibuya, S., Sano, H., Takahashi, M., Satomi, K., and Ohki, Y. (2011). Functional assessment of proximal arm muscles by target-reaching movements in patients with cervical myelopathy. Spine J. 11, 270–280. doi:10.1016/j.spinee.2011.02.003
Ikegami, T., Flanagan, J. R., and Wolpert, D. M. (2022). Reach adaption to a visuomotor gain with terminal error feedback involves reinforcement learning. PLoS ONE 17, e0269297. doi:10.1371/journal.pone.0269297
Kaplan-Rakowski, R., Lin, L., and Wojdynski, T. (2022). Learning vocabulary using 2D pictures is more effective than using immersive 3D stereoscopic pictures. Int. J. Human–Computer Interact. 38, 299–308. doi:10.1080/10447318.2021.1938394
Karniel, A., and Inbar, G. F. (1997). A model for learning human reaching movements. Biol. Cybern. 77, 173–183. doi:10.1007/s004220050378
Kasuga, S., Crevecoeur, F., Cross, K. P., Balalaie, P., and Scott, S. H. (2022). Integration of proprioceptive and visual feedback during online control of reaching. J. Neurophysiol. 127, 354–372. doi:10.1152/jn.00639.2020
Keefe, B. D., and Watt, S. J. (2017). Viewing geometry determines the contribution of binocular vision to the online control of grasping. Exp. Brain Res. 235, 3631–3643. doi:10.1007/s00221-017-5087-0
Kim, H., Kim, Y., Miyakoshi, M., Stapornchaisit, S., Yoshimura, N., and Koike, Y. (2021). Brain activity reflects subjective response to delayed input when using an electromyography-controlled robot. Front. Syst. Neurosci. 15, 767477. doi:10.3389/fnsys.2021.767477
Kim, H., Koike, Y., Choi, W., and Lee, J. (2023). The effect of different depth planes during a manual tracking task in three-dimensional virtual reality space. Sci. Rep. 13, 21499. doi:10.1038/s41598-023-48869-w
Kim, H., Lee, J., and Kim, J. (2020a). Muscle synergy analysis for stroke during two degrees of freedom reaching task on horizontal plane. Int. J. Precis. Eng. Manuf. 21, 319–328. doi:10.1007/s12541-019-00251-5
Kim, H., Tobisawa, S., Park, H., Kim, J., Lee, J., and Shin, D. (2024). Aging-induced degradation in tracking performance in three-dimensional movement. SICE JCMSI 17, 239–246. doi:10.1080/18824889.2024.2359183
Kim, H., Yoshimura, N., and Koike, Y. (2019). Characteristics of kinematic parameters in decoding intended reaching movements using electroencephalography (EEG). Front. Neurosci. 13, 1148. doi:10.3389/fnins.2019.01148
Kim, H., Yoshimura, N., and Koike, Y. (2020b). Investigation of delayed response during real-time cursor control using electroencephalography. J. Healthc. Eng. 2020, 1–9. doi:10.1155/2020/1418437
Kim, H. R., Angelaki, D. E., and DeAngelis, G. C. (2016). The neural basis of depth perception from motion parallax. Philos. Trans. R. Soc. Lond. B Biol. Sci. 371, 20150256. doi:10.1098/rstb.2015.0256
Knight, R. G., and Titov, N. (2009). Use of virtual reality tasks to assess prospective memory: applicability and evidence. Brain Impair. 10, 3–13. doi:10.1375/brim.10.1.3
Kuhne, C., Kecelioglu, E. D., Maltby, S., Hood, R. J., Knott, B., Ditton, E., et al. (2023). Direct comparison of virtual reality and 2D delivery on sense of presence, emotional and physiological outcome measures. Front. virtual real 4. doi:10.3389/frvir.2023.1211001
Leat, S. J., Chan, L., Maharaj, P.-D., Hrynchak, P. K., Mittelstaedt, A., Machan, C. M., et al. (2013). Binocular vision and eye movement disorders in older adults. Invest. Ophthalmol. Vis. Sci. 54, 3798–3805. doi:10.1167/iovs.12-11582
Lee, M., Lee, S. A., Jeong, M., and Oh, H. (2020). Quality of virtual reality and its impacts on behavioral intention. Int. J. Hosp. Manag. 90, 102595. doi:10.1016/j.ijhm.2020.102595
Li, L., Yamada, T., and Choi, W. (2020). The effect of depth information on visual complexity perception in three-dimensional textures. Appl. Sci. 10, 5347. doi:10.3390/app10155347
Lin, C. J., Abreham, B. T., and Woldegiorgis, B. H. (2019). Effects of displays on a direct reaching task: a comparative study of head mounted display and stereoscopic widescreen display. Int. J. Ind. Ergon. 72, 372–379. doi:10.1016/j.ergon.2019.06.013
Ling, Y., Brinkman, W.-P., Nefs, H. T., Qu, C., and Heynderickx, I. (2012). Effects of stereoscopic viewing on presence, anxiety, and cybersickness in a virtual reality environment for public speaking. Presence Teleoperators Virtual Environ. 21, 254–267. doi:10.1162/PRES_a_00111
Liu, B., and Todd, J. T. (2004). Perceptual biases in the interpretation of 3D shape from shading. Vis. Res. 44, 2135–2145. doi:10.1016/j.visres.2004.03.024
Matsui, T., Honda, M., and Nakazawa, N. (2006). “A new optimal control model for reproducing human arm’s two-point reaching movements: a modified minimum torque change model,” in 2006 IEEE international conference on robotics and biomimetics, Kunming, China, 17-20 December 2006 (IEEE), 1541–1546. doi:10.1109/ROBIO.2006.340158
Mitchison, G. J., and Westheimer, G. (1984). The perception of depth in simple figures. Vis. Res. 24, 1063–1073. doi:10.1016/0042-6989(84)90084-1
Murphy, A. P., Leopold, D. A., Humphreys, G. W., and Welchman, A. E. (2016). Lesions to right posterior parietal cortex impair visual depth perception from disparity but not motion cues. Philos. Trans. R. Soc. Lond. B Biol. Sci. 371, 20150263. doi:10.1098/rstb.2015.0263
Norman, J. F., Crabtree, C. E., Bartholomew, A. N., and Ferrell, E. L. (2009). Aging and the perception of slant from optical texture, motion parallax, and binocular disparity. Atten. Percept. Psychophys. 71, 116–130. doi:10.3758/APP.71.1.116
Norman, J. F., Crabtree, C. E., Herrmann, M., Thompson, S. R., Shular, C. F., and Clayton, A. M. (2006). Aging and the perception of 3-D shape from dynamic patterns of binocular disparity. Percept. Psychophys. 68, 94–101. doi:10.3758/bf03193659
Norman, J. F., Dawson, T. E., and Butler, A. K. (2000). The effects of age upon the perception of depth and 3-D shape from differential motion and binocular disparity. Perception 29, 1335–1359. doi:10.1068/p3111
Norman, J. F., Todd, J. T., and Orban, G. A. (2004). Perception of three-dimensional shape from specular highlights, deformations of shading, and other types of visual information. Psychol. Sci. 15, 565–570. doi:10.1111/j.0956-7976.2004.00720.x
O’Brien, J., and Johnston, A. (2000). When texture takes precedence over motion in depth perception. Perception 29, 437–452. doi:10.1068/p2955
Olszewska, D. A., Fearon, C., and Lynch, T. (2016). Loss of visual feedback revealing motor impairment - an early symptom of Parkinson’s disease in two Irish farmers. J. Clin. Mov. Disord. 3, 12. doi:10.1186/s40734-016-0040-0
Onagawa, R., Mukai, K., and Kudo, K. (2022). Different planning policies for the initial movement velocity depending on whether the known uncertainty is in the cursor or in the target: motor planning in situations where two potential movement distances exist. PLoS ONE 17, e0265943. doi:10.1371/journal.pone.0265943
Pallavicini, F., Pepe, A., and Minissi, M. E. (2019). Gaming in virtual reality: what changes in terms of usability, emotional response and sense of presence compared to non-immersive video games?. Simul. Gaming 50, 136–159. doi:10.1177/1046878119831420
Patel, N., Costa, A., Sanders, S. P., and Ezon, D. (2021). Stereoscopic virtual reality does not improve knowledge acquisition of congenital heart disease. Int. J. Cardiovasc. Imaging 37, 2283–2290. doi:10.1007/s10554-021-02191-6
Paz, R., Boraud, T., Natan, C., Bergman, H., and Vaadia, E. (2003). Preparatory activity in motor cortex reflects learning of local visuomotor skills. Nat. Neurosci. 6, 882–890. doi:10.1038/nn1097
Ramachandran, V. S. (1988). Perception of shape from shading. Nature 331, 163–166. doi:10.1038/331163a0
Roby-Brami, A., Feydy, A., Combeaud, M., Biryukova, E. V., Bussel, B., and Levin, M. F. (2003). Motor compensation and recovery for reaching in stroke patients. Acta Neurol. Scand. 107, 369–381. doi:10.1034/j.1600-0404.2003.00021.x
Rosa, P. J., Morais, D., Gamito, P., Oliveira, J., and Saraiva, T. (2016). The immersive virtual reality experience: a typology of users revealed through multiple correspondence analysis combined with cluster analysis technique. Cyberpsychol. Behav. Soc. Netw. 19, 209–216. doi:10.1089/cyber.2015.0130
Rose, T., Nam, C. S., and Chen, K. B. (2018). Immersion of virtual reality for rehabilitation - review. Appl. Ergon. 69, 153–161. doi:10.1016/j.apergo.2018.01.009
Sarlegna, F. R. (2006). Impairment of online control of reaching movements with aging: a double-step study. Neurosci. Lett. 403, 309–314. doi:10.1016/j.neulet.2006.05.003
Schiller, P. H., Slocum, W. M., Jao, B., and Weiner, V. S. (2011). The integration of disparity, shading and motion parallax cues for depth perception in humans and monkeys. Brain Res. 1377, 67–77. doi:10.1016/j.brainres.2011.01.003
Seidler, R. D., Bernard, J. A., Burutolu, T. B., Fling, B. W., Gordon, M. T., Gwin, J. T., et al. (2010). Motor control and aging: links to age-related brain structural, functional, and biochemical effects. Neurosci. Biobehav. Rev. 34, 721–733. doi:10.1016/j.neubiorev.2009.10.005
Servos, P. (2000). Distance estimation in the visual and visuomotor systems. Exp. Brain Res. 130, 35–47. doi:10.1007/s002210050004
Setti, T., and Csapo, A. B. (2021). A canonical set of operations for editing dashboard layouts in virtual reality. Front. Comput. Sci. 3. doi:10.3389/fcomp.2021.659600
Smith, J. E. T., and Parker, A. J. (2021). Correlated structure of neuronal firing in macaque visual cortex limits information for binocular depth discrimination. J. Neurophysiol. 126, 275–303. doi:10.1152/jn.00667.2020
Subramanian, S. K., and Levin, M. F. (2011). Viewing medium affects arm motor performance in 3D virtual environments. J. Neuroeng. Rehabil. 8, 36. doi:10.1186/1743-0003-8-36
Suzuki, M., Yamazaki, Y., Mizuno, N., and Matsunami, K. (1997). Trajectory formation of the center-of-mass of the arm during reaching movements. Neuroscience 76, 597–610. doi:10.1016/s0306-4522(96)00364-8
Tam, S.-F., Man, D., Chan, Y.-P., Sze, P.-C., and Wong, C.-M. (2005). Evaluation of a computer-assisted, 2-D virtual reality system for training people with intellectual disabilities on how to shop. Rehabil. Psychol. 50, 285–291. doi:10.1037/0090-5550.50.3.285
Thomas, G., Goldberg, J. H., Cannon, D. J., and Hillis, S. L. (2002a). Surface textures improve the robustness of stereoscopic depth cues. Hum. Factors 44, 157–170. doi:10.1518/0018720024494766
Thomas, O. M., Cumming, B. G., and Parker, A. J. (2002b). A specialization for relative disparity in V2. Nat. Neurosci. 5, 472–478. doi:10.1038/nn837
Tian, F., Hua, M., Zhang, W., Li, Y., and Yang, X. (2021). Emotional arousal in 2D versus 3D virtual reality environments. PLoS ONE 16, e0256211. doi:10.1371/journal.pone.0256211
Todd, J. T. (2004). The visual perception of 3D shape. Trends Cogn. Sci. Regul. Ed. 8, 115–121. doi:10.1016/j.tics.2004.01.006
Todd, J. T., Egan, E. J. L., and Phillips, F. (2014). Is the perception of 3D shape from shading based on assumed reflectance and illumination?. Iperception 5, 497–514. doi:10.1068/i0645
Todd, J. T., Norman, J. F., Koenderink, J. J., and Kappers, A. M. (1997). Effects of texture, illumination, and surface reflectance on stereoscopic shape perception. Perception 26, 807–822. doi:10.1068/p260807
Todd, J. T., Yu, Y., and Phillips, F. (2023). Qualitative perception of 3D shape from patterns of luminance curvature. J. Vis. 23, 10. doi:10.1167/jov.23.5.10
Volcic, R., Fantoni, C., Caudek, C., Assad, J. A., and Domini, F. (2013). Visuomotor adaptation changes stereoscopic depth perception and tactile discrimination. J. Neurosci. 33, 17081–17088. doi:10.1523/JNEUROSCI.2936-13.2013
Wolpert, D. M., Ghahramani, Z., and Jordan, M. I. (1995). An internal model for sensorimotor integration. Science 269, 1880–1882. doi:10.1126/science.7569931
Yan, F.-F., Lv, H., Fan, S., Chen, L., Wu, Y., and Huang, C.-B. (2021). Effect of physiological aging on binocular vision. Psych. J. 10, 340–351. doi:10.1002/pchj.437
Keywords: virtual reality (VR), stereoscopic effect, reaching, 3D objects, motor control
Citation: Kim H, Kim Y, Lee J and Kim J (2024) Stereoscopic objects affect reaching performance in virtual reality environments: influence of age on motor control. Front. Virtual Real. 5:1475482. doi: 10.3389/frvir.2024.1475482
Received: 04 August 2024; Accepted: 29 October 2024;
Published: 06 November 2024.
Edited by:
David C. Jeong, Santa Clara University, United StatesReviewed by:
Julián Nevárez Montes, University of Monterrey, MexicoCristobal Rodolfo Guerra-Tamez, University of Monterrey, Mexico
Copyright © 2024 Kim, Kim, Lee and Kim. This is an open-access article distributed under the terms of the Creative Commons Attribution License (CC BY). The use, distribution or reproduction in other forums is permitted, provided the original author(s) and the copyright owner(s) are credited and that the original publication in this journal is cited, in accordance with accepted academic practice. No use, distribution or reproduction is permitted which does not comply with these terms.
*Correspondence: Jaehyo Kim, amhraW1AaGFuZG9uZy5lZHU=; Jongho Lee, Sm9uZ2hvLmxlZUBrb21hdHN1LXUuYWMuanA=
†These authors have contributed equally to this work