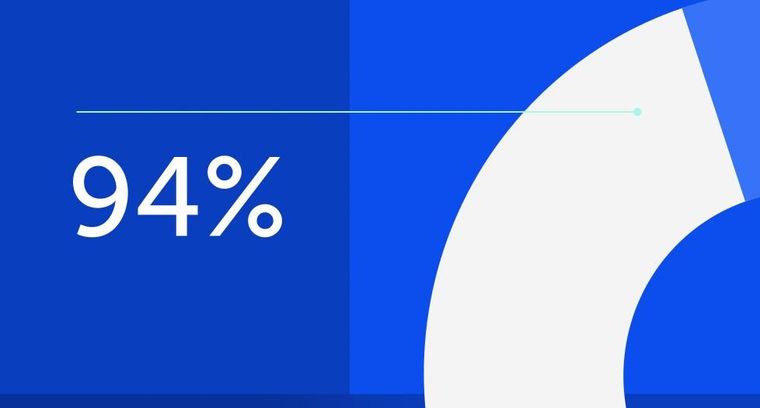
94% of researchers rate our articles as excellent or good
Learn more about the work of our research integrity team to safeguard the quality of each article we publish.
Find out more
BRIEF RESEARCH REPORT article
Front. Virtual Real., 21 May 2024
Sec. Virtual Reality and Human Behaviour
Volume 5 - 2024 | https://doi.org/10.3389/frvir.2024.1377210
This article is part of the Research TopicWorking mechanisms of design elements to facilitate learning in extended realityView all 5 articles
Virtual Reality (VR) technology allows the design and application of realistic but adaptive learning environments in medical education. In particular, virtual patient systems have logistical and methodological advantages compared to non-computerized interventions. However, evidence for their effectiveness is fragmented as any educational domain introduces its requirements regarding learning goals, measurements of learning outcomes, and application design. In this context, we present preliminary results of evaluating a VR training application for conducting a clinical interview to diagnose mental disorders in children and adolescents using virtual patients. The evaluation focuses on design elements related to the virtual patient’s appearance and natural language capabilities. Our results indicate that our virtual patient design is highly believable and that our dialog system is satisfying. However, conversational flow requires optimization. We discuss design directions and potential enhancements for learner-virtual patient interactions in VR and address future operations to evaluate the effectiveness of our approach.
Extended reality systems like VR have become increasingly relevant as a means for medical education Kononowicz et al., 2015, Kononowicz et al., 2019; Campillos-Llanos et al., 2020; Pantziaras et al., 2015; Mavrogiorgou et al., 2022; Graf et al., 2023b. Utilizing its high degree of sensory immersion and natural interaction affordances, VR enables the simulation of face-to-face interaction scenarios within an adaptive learning environment that is cost-effective, scalable, and applicable in a standardized way for different learners. Further, VR-simulated medical scenarios provide training opportunities in a less stressful learning environment using embodied digital technologies like virtual patients (VP) Barry Issenberg et al., 2005; Cook et al., 2010. A VP is “a specific type of computer program that simulates real-life clinical scenarios; learners emulate the roles of healthcare providers to obtain a history, conduct a physical exam, and make diagnostic and therapeutic decisions” Candler (2007). In a real-world educational context, access to patients for means of training is usually limited, and therefore, it is not feasible to provide a large group of students with individual patient contact. Thus, VPs are already used in medical education and show several advantages Plackett et al., 2022; Kocaballi et al., 2019. Compared to conventional interventions, like simulation patients (i.e., role plays with actors), VPs are independent of student schedules Cook et al. (2010), or the ability of the actors to portray the patients authentically Wuendrich et al. (2012). In particular, in pediatric contexts, the lack of children simulation patients introduces a fundamental challenge in training interaction with young patients. Besides those benefits, research is still ongoing to assess the learning effectiveness of using VPs. Several review articles report that VP systems show positive learning effects on clinical reasoning and knowledge acquisition in comparison with no practical intervention, but relatively small or no effects compared to conventional or non-computerized interventions Plackett et al., 2022; Cook et al., 2010; Milne-Ives et al., 2020. Thereby, design elements like specific feedback mechanisms McGaghie et al., 2010; Barry Issenberg et al., 2005 and the level of interactivity Cook et al. (2010) have been discussed as crucial factors for a positive learning outcome. However, the reviews conclude that it is hard to generalize the results, as on the one hand, the VP systems show a great variety in the design, their aim, as well as in the measurement of the learning outcome (e.g., clinical reasoning) Milne-Ives et al., 2020; Cook et al., 2010; Plackett et al., 2022. The evaluation of specific design elements of VP systems has received less attention in research so far. Our work addresses this gap and explores design elements expected to convey a sense of a “genuine” social interaction, which can enhance learning motivation when using systems with virtual tutor agents Baylor (2011). Specifically, we focus on design elements related to the appearance of a VP and its natural language capabilities and investigate whether these elements are decisive for the perceived believability of the interaction between learners and a VP in a specific educational context. In the following, we review related work on virtual patient systems and their design. Then, we describe our VR application and its interim evaluation. The paper concludes by discussing our preliminary findings regarding future research implications.
This brief research report presents preliminary results of the ongoing development of an educational VR application for learning how to conduct a clinical interview for diagnosis of mental disorders in children and adolescents using VPs. In an interim evaluation, we assessed a VP’s believability and conversational flow and its potential to promote future learning outcomes based on how users rate the design elements 1) VP’s appearance and 2) its conversational capabilities.
VP systems can provide explicit medical skills training recommended for health professionals’ education to reduce the impact of future diagnostic errors and potential patient harm Cleland et al., 2009; Balogh et al., 2015. Several research projects are investigating the use of VP systems in the education of medical students Campillos-Llanos et al., 2020; Pantziaras et al., 2015; Mavrogiorgou et al., 2022; Graf et al., 2023b. Thereby, the art of VPs can vary from chatbots Cameron et al. (2019) to embodied conversation virtual agents Campillos-Llanos et al., 2020; Pantziaras et al., 2015. They can be accessible via different devices like computers Pantziaras et al., 2015; Campillos-Llanos et al., 2020 or VR headsets Mavrogiorgou et al., 2022; Graf et al., 2023b. Several review articles have investigated the effectiveness of VP systems over the past years Cook et al., 2010; Milne-Ives et al., 2020; McGaghie et al., 2010; Plackett et al., 2022; Kocaballi et al., 2019; Isaza-Restrepo et al., 2018. A systematic review by Cook et al. (2010) evaluated computerized VPs, especially in educating health professionals on the learning outcome. They also focused on the design features of the respective virtual patients. Their review included 48 articles, including VPs for medicine students, nurses, and other health professionals. Their results show that VPs show positive learning effects on clinical reasoning and knowledge acquisition compared to no intervention but relatively small effects compared to non-computerized interventions. Regarding the design features, they could show that repetition, extended feedback from the VP system, and explicitly contrasting cases can improve learning outcomes. Furthermore, features essential for the students were natural case progression (including collecting data, offering more and less restricted options, and adapting to the actions of learners), case realism, realistic dialogue flow, and working together in a group of students. Another later review by Plackett et al. (2022) also investigated the effectiveness of VPs, especially regarding clinical reasoning skills. They included 19 research articles covering VP systems from a range of disciplines. Only 58% of the reviewed studies reported significant positive effects of the VP systems on clinical reasoning skills, while 21% indicated mixed effects and 21% no effects. However, compared to other teaching methods (i.e., tutorials), 75% of the students showed no effects. Thus, VP systems seem to outperform having no intervention but not other teaching interventions regarding improved clinical reasoning skills. Their review also identified two main intervention features in VP systems. Most of the VP systems (68%) use feedback on the learners’ performance and thus align with recommendations from studies about simulation-based learning Schubach et al., 2017; Isaza-Restrepo et al., 2018. 50% implement a high level of interactivity, requiring the learners to gather information from the VP. Another review by Milne-Ives et al. (2020), focused on evaluating conversational agents in healthcare that are supported by artificial intelligence. Again, the review indicates positive or mixed effectiveness (76.7%) of the VP systems. Additionally, the majority of the reviewed VP systems seems to have good usability (90%) and user satisfaction (83.9%). Further, qualitative user feedback revealed that the most common complaint with conversational agents was poor comprehension due to a lack of vocabulary, inaccurate voice recognition, or improper word input error management. Users disliked the repetitive conversations, and the conversational agents frequently had to ask questions more than once to process the response. Furthermore, negative aspects were the difficulty of empathizing with the VP and the lack of representation of the situation’s complexity by the agent. They liked that VPs provided a risk-free learning environment, as they were not actual patients.
There are just as many disciplines for VP systems as there are in the education of medical students, not only in practicing ambulatory medicine Buysse et al. (2002), medical ethics Fleetwood et al. (2000), but also for mental health assessment skills Washburn et al. (2016) or diagnostics skills Mavrogiorgou et al., 2022; Pantziaras et al., 2015 developed an interactive desktop application where medical assistants conducted a psychiatric interview. The VP responded with pre-recorded video sequences. They can also physically examine the patient and order laboratory and imaging examinations. The learners then draw up a differential diagnosis and a treatment plan. In addition, they receive feedback from the patient regarding the consultation and from a virtual consultant who refers to the clinical performance. Their results show that the acquisition of basic knowledge in the field of psychiatry was improved. Mavrogiorgou et al. (2022) also developed a VP system for adult psychiatry using VR and embodied agents that allows students to interview an embodied VP using natural language input and output. However, this system still needs to be evaluated.
To design embodied virtual agents in a learning context, Doering et al. (2008) developed a framework that implies an agent should be attentive and responsive during the interaction and ready to respond. It should be able to reply to queries and obtain feedback. The messages it communicates should be adapted to the user’s experience and needs and contain congruent verbal and non-verbal elements. Finally, the agent should awaken believability and trust. The believability of virtual characters describes the acceptance that someone or something in a virtual world is perceived as real Allbeck and Badler (2001). Aspects that play an essential role in increasing the believability of virtual characters can be their appearance, body language, and voice Lim and Aylett 2007; Demeure et al., 2011 or interactivity Knoppel 2009; De Rosis et al., 2003; Baylor and Kim 2009 showed in their study that a visible and physically present agent positively influenced users’ motivation compared to a voice or a text box. Thereby, the appearance of virtual characters affects a player’s perception. For example, while Baylor and Kim (2009) showed that realistically designed agents were more beneficial, as cartoon-style agents reduced motivation in users, Graf et al. (2023a) showed that a comic-like and even animal-like virtual character could influence the emotional experience, as well as the motivation and performance of the players. Again, Zibrek et al. (2018) showed that participants were more concerned with a realistically rendered character than with characters rendered in less realistic styles. Considering the uncanny valley effect is crucial when choosing a degree of realism. It describes the sudden change of a user’s evaluation of an artificial human from positive to negative if it approaches photorealism but still has subtle characteristics that limit its realism Mori et al. (2012). Besides the appearance, an appropriate display of emotions is crucial for the believability of virtual agents. A study by Lim and Aylett (2007) showed that virtual agents showing appropriate emotions are more believable than those showing no emotions. Studies showed that learners liked VPs showing empathy and when having a personality Cameron et al., 2019; Dimeff et al., 2020 or disliked it when it was missing it Ly et al., 2017; Borja-Hart et al., 2019; Cook et al., 2010 define interactivity as the “degree to which the course design encouraged learners to engage cognitively.” Former research results are inconclusive about the effect of interactivity on learning outcomes Homer and Plass (2014). According to studies, increased interactivity can encourage more engaged users and deeper learning, but it can also increase cognitive load, which can impede learning Kalet et al., 2012; Homer and Plass 2014. In the VP context, studies showed that learners liked the interactivity of the VPs Hudlicka 2013; Ly et al., 2017 or wished for more interactivity Cameron et al., 2019; Håvik et al., 2019.
We evaluated our application with medical students. Each student had a conversation with a 14-year-old female VP suffering from depression using the Meta Quest Pro. Before entering the virtual world, the catalog was shown and explained to the participants. They interacted with the VP in the virtual world for 25.13–54, 56 min (M = 33.7, SD = 11.7) while they had to ask all 58 questions from the catalog. Therefore, the time they spent in VR was at least the time they needed to ask all the given questions. The total number of questions for each participant could differ as participants could also try to ask questions not included in the catalog. It could take longer depending on how long it took the participants to ask the questions. After that, they filled out question items regarding the believability of the dialog between them and the VP and its appearance. In the end, we conducted a 10-min interview with each participant to identify the advantages and pitfalls of their conversations with the virtual patient and how believable they perceived the situation. Furthermore, we tracked the progress of the questions, the answers given by the VP, and whether and how many hints the participants had asked for while using the application. As shown in Figure 1, for example, in the category habits and consumption behavior, the first hint shows the keyword alcohol, which means that the user should ask a question regarding the VP’s alcohol consumption. The second hint then shows the specific question Do you drink alcohol?
Figure 1. Left: The tablet shows students the category of question and how many questions belong to the category by the filling circles. Furthermore, it shows the level one and level two hint button, that students can press for help. Right: The VP sitting on the virtual couch in front of a student.
We recruited five medical students (3 female, 2 male) aged 23–26 (M = 24.2, SD = 1.3) in their 7th to 12th clinical semester via advertisement on a digital bulletin board; previous experience in child and adolescent psychiatry was not mandatory. Two students had prior experience in psychiatry diagnostics and relatively little VR (M = 3.6, SD = 0.89, Mdn = 4.00), gaming (M = 3.8, SD = 2.17, Mdn = 3.00), or experiences with virtual agents (M = 2.0, SD = 1.23, Mdn = 2.00) measured on a scale from 1 (= no experience at all) to 7 (= a lot experience). The participants filled out the questionnaire and were interviewed in German.
We designed a VR application for the teaching of conducting clinical interviews and diagnosing mental disorders in child and adolescent psychiatry using embodied virtual agents as patients. The application is structured by following a catalog of questions we created based on the AMDP system together with a Child and adolescent psychiatrist (see the catalog in the Supplementary Material). The AMDP system1 is an international standard for the methodical documentation of psychiatric diagnoses, developed by a German association for methodology and documentation in psychiatry. The catalog covers 17 categories relating to different symptoms or characteristics (e.g., habits and consumption behavior or affective disorders). Each category then contains one to six subcategories (e.g., alcohol, drug, and media consumption, or aggressiveness and mood swing) the students need to address in their interview. A pre-defined sequence of the question catalog guides the students through the conversation. However, asking about any symptoms and repeating individual questions is possible. The application uses natural language understanding (NLU) as input using Wit.ai2 by Meta. Wit.ai converts the user’s question into text and then assigns it to an existing intention, which outputs a corresponding voice output from a selection of prerecorded audios (see Figure 2). Based on the virtual patient’s answers, the students eventually have to decide on a psychiatric diagnosis. The VP is an embodied virtual model of a teenager with stylized aesthetics. We chose a stylized yet realistic approach compared to photorealism due to the risk of the uncanny valley effect Mori et al. (2012). Furthermore, studies show that photorealism is unnecessary to achieve behavioral realism Blascovich et al. (2002) or believability Graf et al. (2023b). Further, we decided against an even more stylized design, as in previous discussions, medical students indicated the preference for a realistic VP Graf et al. (2023b). Accordingly, we made the facial features, skin, hair, and clothes childlike. We also mapped the prerecorded facial expressions of a real actress onto the agent’s face and created a corresponding body language in the form of animations. We used Elevenlabs3, a generative voice AI, to synthesize a natural voice for the VP’s versatile responses retaining the emotionality of a human voice. We also created a user interface integrated into a virtual tablet the users have in the virtual world (see Figure 1). The tablet gives the students an overview of the catalog of questions. It displays the current category and allows students to ask for two levels of hints. The first hint shows the keyword of the subcategory of questions, and the second hint shows a sample question if students do not know how to ask for the respective symptom. We chose this two-level hint system so that students can use the application regardless of their previous knowledge of child and adolescent psychiatry.
Figure 2. This is a visualization of the VR application and how teachers and students interact with it. Through a web interface, the teacher can choose a patient with whom the student will interact. The student can ask questions using natural language input. This input is transcribed by using Wit.ai, allocating the recognized intention to a certain response of the current VP. The allocated responses then trigger a respective audio and animation in the VP.
To measure the believability of the dialog and the VP and its appearance, we used self-formulated items measured on a scale from 1 (= do not agree at all) to 7 (= fully agree), see Table 1. Furthermore, we collected demographic data such as age and gender as well as previous experience with a single-item each “Please rate how familiar you are with the concept of [virtual reality/virtual agents/games]” on a scale from 1 (= not at all familiar) to 7 (= very familiar). Therefore, we gave participants the following definition of a virtual agent: virtual agents are the visual representation of a character (e.g., a person) whose behavior is controlled by a computer algorithm.
Table 1. Descriptive values of the quantitative data regarding believability of the dialog and the VP’s appearance.
To further evaluate the believability and investigate the pitfalls and advantages of our application, we conducted a semi-structured 10-min interview with each participant at the end of the evaluation. Five questions provided the basis for the interview:
1. How did you like the application?
2. How believable did you perceive the conversation between you and the virtual patient?
3. How did you generally like the way the conversation was conducted?
a. Were there any problems you noticed during the interview?
b. For example, were there any questions you asked that the patient did not recognize or answered inappropriately?
4. To what extent can this application be a useful addition to your studies?
5. Was there anything that you would have liked to have been added to the application?
Furthermore, we tracked the progress of the questions in a downloadable interaction log from the VR headset’s memory storage after the session. The log included the answers given by the VP and whether and how many hints the participants had asked for while using the application. Based on this interaction log, we have defined different types of errors: concept and system errors. A concepts error appears when the VP’s answer does not match the participant’s question because the question is not part of the catalog, so there is no implemented intention in Wit.ai. As a system error, we define errors when the VP’s answer does not match the participant’s question because wit.ai allocated the answer to a wrong intention. When the VP’s answer matched the participant’s question, we defined this turn as the correct allocation. We also counted how often participants used a hint when receiving a correct allocation or errors. Thereby, we differ between level one hints (H1), the keyword display, level two hints (H2), the display of the sample question, and the use of both hints.
Table 1 shows the descriptive values of the quantitative results regarding the believability of the dialog between participants and the VP and of the VP’s appearance. The believability of the dialog was rated on average on M = 4.2(SD = 1.92). But there was strong agreement that many hints were taken during the conversation. Satisfaction with the accuracy of the VP’s answers was rated particularly high (M = 5.2, SD = 1.92), and the lowest score was given to the question of how real the conversation with the VP felt (M = 3.6, SD = 1.52). Regarding the believability of the VP’s appearance, it received higher values. Here, the highest agreement was that the VP was appropriate for the situation (M = 6.8, SD = 0.45), and the lowest agreement was assigned to the VP’s facial expression (M = 4.4, SD = 1.52).
We analyzed the interaction logs (Table 2) and counted 19.8 (28.62%) errors in total by a total amount of 68.8 asked questions on average. All participants, besides participant (p01), asked all given questions and more. Participant (p01) had to stop early because the application crashed due to an internet connection error. The natural language understanding achieved 71.38% correct allocations on average. Overall, we found more errors defined as concept errors (20.3%) than system errors (8.32%). It can also be observed that a high number of hints are used for the correctly allocated turns (no errors). On average, both hints were used 13 times for the correct allocation, while both hints were used one time on average for concept errors and one time for system errors. With an average value of 30 times, participants used the level 2 hint (sample question) most frequently for the correct allocation. On average, up to three hints were used when errors appeared.
Table 2. Amount of errors for all participants and average, tracked by the interaction log. H1 = hint 1 (keyword), H2 = hint 2 (sample question), Both = both hints.
We transcribed all interviews and formed categories using the MAXQDA4. After one researcher categorized the answers into categories, another researcher independently checked whether they would assign the aspects to the same categories. We calculated no inter-rater agreement. In total, we derived five categories.
The participants identified problems in the flow of the conversation, such as the strict categories that one had to follow throughout the dialog (p01). Participant (p02) mentioned that they thought it was strange to ask every patient the same questions and, especially, that not all given questions fit the depressive young girl. They also said that they would ask an open-ended question to a patient first, not to direct the patient’s answer. Two reported repeated answers to differently formulated questions (p02, p04) and that the answers did not always fit the questions (p04). Two participants thought the system would not understand them when their formulation of a question differed too much from the given sample question (Hint 2) (p04) or when they formulated questions too long (p05). Participant (p04) also criticized that symptoms the VP mentioned had to be asked for explicitly, and additionally, that each student had their way of structuring such conversations with patients.
There were also positive statements about the dialog. Two mentioned that the system understood their questions well, and, therefore, the dialog proceeded well (p03, p05). One participant did not feel narrowed by the straight structure of the catalog of questions but praised that they could repeat questions and even ask questions that were not next in the sequence (p03).
All participants evaluated the believability of the virtual patient well. They highlight the voice (p01, p03, p04, p05), the posture (p02, p03, p04), as well as the mimic and facial expressions (p02, p04) of the patient. One participant said they “did not want to put their foot in their mouth, even though it was a computer” (p03), and another said they realized that the VP adjusted their facial expressions according to the respective questions (p05).
We asked the participants to rate the dialog between them and the VP to identify what already works well and what does not. A participant rated positively that, in general, the dialog felt believable, as they could communicate well with the VP (p01). The principle of asking questions and receiving answers felt natural and pleasant like in a real dialog (p03), and one could imagine that a dialog in real life would proceed similarly (p05). Nonetheless, participants also rated aspects negatively. They mainly mentioned that they had to ask particular questions and follow the predefined questions of the catalog, which felt unnatural to them (p02, p05). One added that they would usually go deeper into the symptoms and ask them further questions, and as this was impossible, it felt less natural to them (p04). They also said they were more focused on formulating the questions so the system could understand them than formulating the question for a young patient (p04).
Participants named different positive aspects of the application. Three of them mentioned that they were positively surprised by how well the VP appropriately answered the questions, even when participants formulated long questions (p01, p04, p05). Four participants said they liked the general idea of the application as a learning tool, especially for beginners. They find it helpful that the application guided them through the procedure of an anamnesis dialog by asking questions one after the other in a schematic sequence (p01, p02, p04, p05). Four participants highlighted the advantages compared to simulation patients (p01, p02, p03, p05); for example, the application provides a calmer surrounding and time, and it would be more believable compared to actors who do no do an excellent job or compared to classmates who you know are not patient. Furthermore, students would be more independent from the actors, and they could all practice independently or even in parallel. It would provide reasonable access for students with social phobia or inhibitions of patient contact (p01, p02). Two liked being together with the patient in the great virtual environment (p03, p05) and praised how well the application’s control system for the user was designed (p05).
Some participants mentioned limitations they accepted and why they think errors occur. One said even though it could be beneficial to ask more open-ended questions, the participant admitted that this would be more difficult to implement (p01) and that a strict sequence of questions could also be helpful for beginners (p05). Similarly, one participant also thought that the facial expression of the VP could be better, but that facial expressions are very complex, which is also difficult to simulate (p03). Furthermore, a few participants attributed recognition errors to their behavior. For example, when a “Hm” was recognized as a question, one participant thought that they should have stopped saying it (p03), or others saw the cause of the errors in their excessively long and convoluted sentences (p01, p02, p03). One also cited his lack of knowledge in psychiatry as the reason for the errors, which they could not compensate for even with the hints (p01).
Two participants explicitly mentioned expanding the possibility to ask questions more freely and individually (p02, p05). Another suggested that the diagnosis could be made by having to answer questions about the patient at the end (p04). Another person suggested that one should not always press the level 2 hint (sample question); otherwise, one could be tempted to use it all the time (p02). Instead, you could only see them every few minutes, so you must consider the question yourself. To create further motivation, you could receive points at the end the fewer times you have been given a tip. Participant (p05) would like more time and space in the interview for transitions so that they does not have to go from question to question. This would give the conversation more credibility because you would first have to gain the patient’s trust. They also suggested more direct feedback from the patient, e.g., if you have said something stupid, the VP tells you directly. The patient could also ask the students a question (p05).
We presented the evaluation results of a VR training application that simulates diagnostic interviews with embodied VPs. We let participants rate two design elements associated with the VP’s believability: its appearance and natural language-based dialog system.
The VP’s appearance was rated well in terms of believability. The quantitative results show moderate values, which are confirmed, in particular, by the qualitative results. Participants highlighted the patient’s voice, posture, and facial expression and rated it appropriate for the context. The second design element, the natural language-based dialog system, was also rated moderately regarding believability, but the qualitative results were unclear. For instance, asking questions and perceiving answers felt like in a real dialog; however, the fact that they had to ask particular questions and follow the predefined catalog felt unnatural. Also, the dialog’s believability was limited by the impossibility of going deeper and asking further questions. Although, the specification of the questions was considered helpful for beginners. The interaction logs indicate that system errors occur significantly less frequently and are more at a conceptual level. This was because participants felt disturbed or irritated by the given sequence. Subjectively, however, the participants attributed the errors more to the system, as they sought the cause, for example, in questions that were too long and confusing. Furthermore, fewer errors occur when participants use many hints, which again indicates that conceptual problems, rather than the speech recognition system, cause errors more often. Overall, participants were impressed by the VP’s current functionality and believability and consider this type of application a valuable learning tool for medical education.
Based on our results, our virtual patient has a highly believable appearance design and a satisfying dialog system. In the current state, the most significant limitation for increased believability is the catalog’s predefined questions, which prevent the natural flow of asking questions. However, the participants tended to accept certain restrictions if they are plausible in the given learning context, i.e., learning a set of standard questions. The participants see great potential here, especially for beginners. The findings from quantitative and qualitative results strengthen our belief in our approach.
To our knowledge, only a few research articles focused on believability of simulation patients (human actors) Baylor et al. (2017) or virtual patients Rizzo et al. (2011), though without investigating individual design aspects of the VR system. One significant finding was that the predefined questions of the catalog limited the participants in the flow of their conversation with the VP, which resulted in a student-VP interaction that felt less natural. Our design aimed to create a natural conversation using natural language input and to guide the students through the clinical interview by presenting the question catalog. The fact that more system errors occurred shows that we needed to consider more questions on a conceptual level in advance. It resulted in participants asking questions to which the VP had no answer. Even after revising the catalog of questions and implementing a more accessible design, such errors could still occur. It is difficult to predict and thus prepare prefabricated answers to all possible questions. One enhancement could be the generation of missing answers to unforeseen questions using AI. Studies show that dynamic response behaviors of virtual agents were rated more positively compared to predefined ones Toader et al., 2019; Hsu and Lin 2023. However, the accuracy of the statements generated by the AI needs to be better and apparent beforehand Wang et al. (2023). This limitation poses a particular problem in the education context and, especially in a psychiatric context, statements invented by the AI could be over- or misinterpreted. Generative AI, such as ChatGPT, also usually follows certain restrictions, such as not making statements about suicidal behavior to protect users. Therefore, future research should validate the adjustment of the prompts precisely to monitor response behavior in the best possible way regarding the teaching content.
We want to have a critical look at our evaluation. To measure the believability of the design elements, we did not use validated questionnaires but custom items applicable to our use case. Other researchers may consider using a scale like the one of Guo et al. (2023). This and the small number of medical students who tested our application must be considered when inferring conclusions from our results for other projects. Further, we have yet to assert the effectiveness of our system. In the future, we want to enhance, in particular, the dialog system and evaluate further elements like a feedback system (e.g., the VP giving verbal feedback during the dialog) or playful elements (e.g., receiving points for correct diagnoses) and their impact on believability and eventually students’ learning outcomes.
We presented the progress in our attempt to design a VR training application for conducting a clinical interview to diagnose mental disorders using embodied VPs. We have focused on the believability of the VP system as a decisive factor in the system’s eventual learning success. If VP systems are to provide advantages over simulation patients whose authenticity is questionable, the believability of the VPs must be considered. Users must perceive the VP as an actual patient suffering from the disease to behave genuinely towards them. Hence, believable VPs are the only way to ensure a real simulation of the situation. Due to the complex and varied use cases, it is difficult to generalize the evaluation results of individual VP systems. By focusing on individual design aspects of the application, which are then application-independent, we want to identify application-independent components that will help design future VP systems. With our preliminary findings, we want to show the technical basis for a believable component, such as a dialog system. Accordingly, our contribution lies in the methodological approach of examining individual design aspects for their believability in order to improve future VP systems. In the future, we will revise the design of the dialog system to allow more freedom and individuality when asking questions. Afterward, we will evaluate the updated version with medical students.
The raw data supporting the conclusion of this article will be made available by the authors, without undue reservation.
The requirement of ethical approval was waived. The studies wereconducted in accordance with the local legislation and institutionalrequirements. The participants provided their written informedconsent to participate in this study. Written informed consent was obtained from the individual(s) for the publication of anypotentially identifiable images or data included in this article.
LG: Conceptualization, Data curation, Formal Analysis, Funding acquisition, Investigation, Methodology, Project administration, Supervision, Validation, Visualization, Writing–original draft. PS: Visualization, Writing–review and editing. GG-D: Conceptualization, Funding acquisition, Supervision, Writing–review and editing. MM: Funding acquisition, Resources, Supervision, Writing–review and editing.
The author(s) declare that financial support was received for the research, authorship, and/or publication of this article. We acknowledge support by the Open Access Publication Fund of the University of Duisburg-Essen. As part of the project “DEVIA”, this work was supported by the Robert-Enke-Stiftung.
The authors declare that the research was conducted in the absence of any commercial or financial relationships that could be construed as a potential conflict of interest.
All claims expressed in this article are solely those of the authors and do not necessarily represent those of their affiliated organizations, or those of the publisher, the editors and the reviewers. Any product that may be evaluated in this article, or claim that may be made by its manufacturer, is not guaranteed or endorsed by the publisher.
The Supplementary Material for this article can be found online at: https://www.frontiersin.org/articles/10.3389/frvir.2024.1377210/full#supplementary-material
1AMDP = Arbeitsgemeinschaft für Methodik und Dokumentation in der Psychiatrie, https://www.amdp.de/, 03/10/2024.
2https://wit.ai/, 03/10/2024.
3https://elevenlabs.io/, 03/10/2024.
4https://www.maxqda.com/de/, 03/10/2024.
Allbeck, J., and Badler, N. I. (2001). Consistent communication with control. Cent. Hum. Model. Simul. 85.
Barry Issenberg, S., Mcgaghie, W. C., Petrusa, E. R., Lee Gordon, D., and Scalese, R. J. (2005). Features and uses of high-fidelity medical simulations that lead to effective learning: a beme systematic review. Med. Teach. 27, 10–28. doi:10.1080/01421590500046924
Baylor, A. L. (2011). The design of motivational agents and avatars. Educ. Technol. Res. Dev. 59, 291–300. doi:10.1007/s11423-011-9196-3
Baylor, A. L., and Kim, S. (2009). Designing nonverbal communication for pedagogical agents: when less is more. Comput. Hum. Behav. 25, 450–457. doi:10.1016/j.chb.2008.10.008
Baylor, C., Burns, M. I., Struijk, J., Herron, L., Mach, H., and Yorkston, K. (2017). Assessing the believability of standardized patients trained to portray communication disorders. Am. J. Speech-Language Pathology 26, 791–805. doi:10.1044/2017_ajslp-16-0068
Blascovich, J., Loomis, J., Beall, A. C., Swinth, K. R., Hoyt, C. L., and Bailenson, J. N. (2002). TARGET ARTICLE: immersive virtual environment technology as a methodological tool for social psychology. Psychol. Inq. 13, 103–124. doi:10.1207/s15327965pli1302_01
Borja-Hart, N. L., Spivey, C. A., and George, C. M. (2019). Use of virtual patient software to assess student confidence and ability in communication skills and virtual patient impression: a mixed-methods approach. Curr. Pharm. Teach. Learn. 11, 710–718. doi:10.1016/j.cptl.2019.03.009
Buysse, H., Van Maele, G., and De Moor, G. (2002). “The dynamic patient simulator: learning process, first results and students’ satisfaction,” in E-Health in Belgium and in The Netherlands (IOS Press), 19–24.
Cameron, G., Cameron, D., Megaw, G., Bond, R., Mulvenna, M., O’Neill, S., et al. (2019). “Assessing the usability of a chatbot for mental health care,” in Internet science: INSCI 2018 international workshops, st. Petersburg, Russia, october 24–26, 2018, revised selected papers 5 (Springer), 121–132.
Campillos-Llanos, L., Thomas, C., Bilinski, E., Zweigenbaum, P., and Rosset, S. (2020). Designing a virtual patient dialogue system based on terminology-rich resources: challenges and evaluation. Nat. Lang. Eng. 26, 183–220. doi:10.1017/s1351324919000329
Candler, C. (2007). “Effective use of educational technology in medical education,” in Colloquium on educational technology: recommendations and guidelines for medical educators (Washington, DC: AAMC Institute for Improving Medical Education).
Cleland, J. A., Abe, K., and Rethans, J.-J. (2009). The use of simulated patients in medical education: amee guide no 42. Med. Teach. 31, 477–486. doi:10.1080/01421590903002821
Cook, D. A., Erwin, P. J., and Triola, M. M. (2010). Computerized virtual patients in health professions education: a systematic review and meta-analysis. Acad. Med. 85, 1589–1602. doi:10.1097/acm.0b013e3181edfe13
Demeure, V., Niewiadomski, R., and Pelachaud, C. (2011). How is believability of a virtual agent related to warmth, competence, personification, and embodiment? Presence 20, 431–448. doi:10.1162/pres_a_00065
De Rosis, F., Pelachaud, C., Poggi, I., Carofiglio, V., and De Carolis, B. (2003). From greta’s mind to her face: modelling the dynamics of affective states in a conversational embodied agent. Int. J. human-computer Stud. 59, 81–118. doi:10.1016/s1071-5819(03)00020-x
Dimeff, L. A., Jobes, D. A., Chalker, S. A., Piehl, B. M., Duvivier, L. L., Lok, B. C., et al. (2020). A novel engagement of suicidality in the emergency department: virtual collaborative assessment and management of suicidality. General Hosp. psychiatry 63, 119–126. doi:10.1016/j.genhosppsych.2018.05.005
Doering, A., Veletsianos, G., and Yerasimou, T. (2008). Conversational agents and their longitudinal affordances on communication and interaction. J. Interact. Learn. Res. 19, 251–270.
Fleetwood, J., Vaught, W., Feldman, D., Gracely, E., Kassutto, Z., and Novack, D. (2000). Medethex online: a computer-based learning program in medical ethics and communication skills. Teach. Learn. Med. 12, 96–104. doi:10.1207/s15328015tlm1202_7
Graf, L., Abramowski, S., Born, F., and Masuch, M. (2023a). Emotional virtual characters for improving motivation and performance in vr exergames. Proc. ACM Human-Computer Interact. 7, 1115–1135. doi:10.1145/3611063
Graf, L., Gradl-Dietsch, G., and Masuch, M. (2023b). “Depressed virtual agents: development of a playful vr application for the training of child and adolescent psychiatry students,” in Proceedings of the 23rd ACM international conference on intelligent virtual agents, 1–3.
Guo, S., Adamo, N., and Mousas, C. (2023). Developing a scale for measuring the believability of virtual agents
Håvik, R., Wake, J. D., Flobak, E., Lundervold, A., and Guribye, F. (2019). “A conversational interface for self-screening for adhd in adults,” in Internet science: INSCI 2018 international workshops, st. Petersburg, Russia, october 24–26, 2018, revised selected papers 5 (Springer), 133–144.
Homer, B. D., and Plass, J. L. (2014). Level of interactivity and executive functions as predictors of learning in computer-based chemistry simulations. Comput. Hum. Behav. 36, 365–375. doi:10.1016/j.chb.2014.03.041
Hsu, C.-L., and Lin, J. C.-C. (2023). Understanding the user satisfaction and loyalty of customer service chatbots. J. Retail. Consumer Serv. 71, 103211. doi:10.1016/j.jretconser.2022.103211
Hudlicka, E. (2013). Virtual training and coaching of health behavior: example from mindfulness meditation training. Patient Educ. Couns. 92, 160–166. doi:10.1016/j.pec.2013.05.007
Isaza-Restrepo, A., Gómez, M. T., Cifuentes, G., and Argüello, A. (2018). The virtual patient as a learning tool: a mixed quantitative qualitative study. BMC Med. Educ. 18, 1–10. doi:10.1186/s12909-018-1395-8
Kalet, A., Song, H., Sarpel, U., Schwartz, R., Brenner, J., Ark, T., et al. (2012). Just enough, but not too much interactivity leads to better clinical skills performance after a computer assisted learning module. Med. Teach. 34, 833–839. doi:10.3109/0142159x.2012.706727
Kocaballi, A. B., Berkovsky, S., Quiroz, J. C., Laranjo, L., Tong, H. L., Rezazadegan, D., et al. (2019). The personalization of conversational agents in health care: systematic review. J. Med. Internet Res. 21, e15360. doi:10.2196/15360
Kononowicz, A. A., Woodham, L. A., Edelbring, S., Stathakarou, N., Davies, D., Saxena, N., et al. (2019). Virtual patient simulations in health professions education: systematic review and meta-analysis by the digital health education collaboration. J. Med. Internet Res. 21, e14676. doi:10.2196/14676
Kononowicz, A. A., Zary, N., Edelbring, S., Corral, J., and Hege, I. (2015). Virtual patients-what are we talking about? a framework to classify the meanings of the term in healthcare education. BMC Med. Educ. 15, 11–17. doi:10.1186/s12909-015-0296-3
Lim, M. Y., and Aylett, R. (2007). “Feel the difference: a guide with attitude,” in International workshop on intelligent virtual agents (Springer), 317–330.
Ly, K. H., Ly, A.-M., and Andersson, G. (2017). A fully automated conversational agent for promoting mental well-being: a pilot rct using mixed methods. Internet interv. 10, 39–46. doi:10.1016/j.invent.2017.10.002
Mavrogiorgou, P., Böhme, P., Hooge, V., Pfeiffer, T., and Juckel, G. (2022). Virtuelle realität in der lehre im fach psychiatrie und psychotherapie. Der Nervenarzt 93, 728–734. doi:10.1007/s00115-021-01227-5
McGaghie, W. C., Issenberg, S. B., Petrusa, E. R., and Scalese, R. J. (2010). A critical review of simulation-based medical education research: 2003–2009. Med. Educ. 44, 50–63. doi:10.1111/j.1365-2923.2009.03547.x
Milne-Ives, M., de Cock, C., Lim, E., Shehadeh, M. H., de Pennington, N., Mole, G., et al. (2020). The effectiveness of artificial intelligence conversational agents in health care: systematic review. J. Med. Internet Res. 22, e20346. doi:10.2196/20346
Mori, M., MacDorman, K. F., and Kageki, N. (2012). The uncanny valley [from the field]. IEEE Robotics automation Mag. 19, 98–100. doi:10.1109/mra.2012.2192811
Pantziaras, I., Fors, U., and Ekblad, S. (2015). Training with virtual patients in transcultural psychiatry: do the learners actually learn? J. Med. Internet Res. 17, e46. doi:10.2196/jmir.3497
Plackett, R., Kassianos, A. P., Mylan, S., Kambouri, M., Raine, R., and Sheringham, J. (2022). The effectiveness of using virtual patient educational tools to improve medical students’ clinical reasoning skills: a systematic review. BMC Med. Educ. 22, 365. doi:10.1186/s12909-022-03410-x
Rizzo, A., Kenny, P., and Parsons, T. D. (2011). Intelligent virtual patients for training clinical skills, 8. JVRB-Journal of Virtual Reality and Broadcasting.
Schubach, F., Goos, M., Fabry, G., Vach, W., and Boeker, M. (2017). Virtual patients in the acquisition of clinical reasoning skills: does presentation mode matter? a quasi-randomized controlled trial. BMC Med. Educ. 17, 1–13. doi:10.1186/s12909-017-1004-2
Toader, D.-C., Boca, G., Toader, R., Măcelaru, M., Toader, C., Ighian, D., et al. (2019). The effect of social presence and chatbot errors on trust. Sustainability 12, 256. doi:10.3390/su12010256
Wang, C., Liu, S., Yang, H., Guo, J., Wu, Y., and Liu, J. (2023). Ethical considerations of using chatgpt in health care. J. Med. Internet Res. 25, e48009. doi:10.2196/48009
Washburn, M., Bordnick, P., and Rizzo, A. (2016). A pilot feasibility study of virtual patient simulation to enhance social work students’ brief mental health assessment skills. Soc. work health care 55, 675–693. doi:10.1080/00981389.2016.1210715
Wuendrich, M. S., Nissen, C., Feige, B., Philipsen, A. S., and Voderholzer, U. (2012). Portrayal of psychiatric disorders: are simulated patients authentic? Acad. Psychiatry 36, 501. doi:10.1176/appi.ap.11090163
Keywords: virtual patients, emotional virtual agents, embodied digital technology, adaptive virtual environments, medical education, agent design, human-agent interaction, virtual reality
Citation: Graf L, Sykownik P, Gradl-Dietsch G and Masuch M (2024) Towards believable and educational conversations with virtual patients. Front. Virtual Real. 5:1377210. doi: 10.3389/frvir.2024.1377210
Received: 26 January 2024; Accepted: 08 April 2024;
Published: 21 May 2024.
Edited by:
Bastian Kordyaka, University of Bremen, GermanyReviewed by:
Silvia Dopler, University of Applied Sciences Upper Austria, AustriaCopyright © 2024 Graf, Sykownik, Gradl-Dietsch and Masuch. This is an open-access article distributed under the terms of the Creative Commons Attribution License (CC BY). The use, distribution or reproduction in other forums is permitted, provided the original author(s) and the copyright owner(s) are credited and that the original publication in this journal is cited, in accordance with accepted academic practice. No use, distribution or reproduction is permitted which does not comply with these terms.
*Correspondence: Linda Graf, bGluZGEuZ3JhZkB1bmktZHVlLmRl
Disclaimer: All claims expressed in this article are solely those of the authors and do not necessarily represent those of their affiliated organizations, or those of the publisher, the editors and the reviewers. Any product that may be evaluated in this article or claim that may be made by its manufacturer is not guaranteed or endorsed by the publisher.
Research integrity at Frontiers
Learn more about the work of our research integrity team to safeguard the quality of each article we publish.