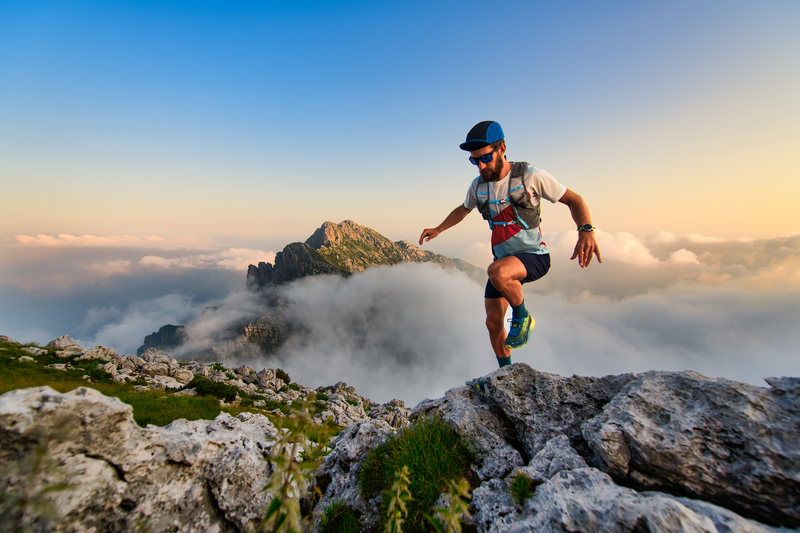
95% of researchers rate our articles as excellent or good
Learn more about the work of our research integrity team to safeguard the quality of each article we publish.
Find out more
ORIGINAL RESEARCH article
Front. Virtual Real. , 04 April 2024
Sec. Virtual Reality and Human Behaviour
Volume 5 - 2024 | https://doi.org/10.3389/frvir.2024.1358981
Practicing guided breathing at 0.1 Hz in virtual reality yields psychological and physiological benefits. Nonetheless, it remains uncertain whether these effects surpass those induced in a real-world setting. Indeed, the potential influence of the virtual environment on perceived stress and anxiety is not yet fully understood. In this experiment, we aimed to compare the effects of heart rate variability biofeedback combining both haptic and visual cues in real and virtual reality settings among the same group of participants. Additionally, to discern whether the psychological benefits arise from viewing an environment in virtual reality or from the act of performing guided breathing in this specific setting, a “control” immersion condition was introduced. 36 healthy sport students (9 females) participated in this study, performing both the real and virtual reality protocols in a randomized order. Anxiety and stress levels were assessed using the STAI-Y questionnaire and a visual analog scale, respectively. Physiological effects were assessed through measures of heart rate variability, and the performance of cardiac coherence was compared between the real and virtual implementations of guided breathing. As expected, both real and virtual reality heart rate variability biofeedback led to similar physiological modulations and cardiac coherence performances. A decrease in stress and anxiety was observed in both protocols, particularly among participants who initially reported higher stress or anxiety levels. However, no additional changes in psychological states were observed when performing guided breathing while immersed in the virtual environment.
Due to its ability to easily display worlds with particular visual characteristics, virtual reality (VR) is a promising tool for addressing stress and anxiety, which have been labeled as a major determinant of chronic diseases in our current society (Egger and Dixon, 2014). In particular, virtual nature scenery have demonstrated beneficial effects for relaxation and stress management (de Kort et al., 2006; Anderson et al., 2017; Liszio et al., 2018; White et al., 2018; Wang et al., 2019; Yin et al., 2020), across both psychological and physiological aspects (Spano et al., 2023), mirroring outcomes witnessed in real-world settings (Park et al., 2017; Corazon et al., 2019). These effects may be explained by the Attention Restoration Theory (Kaplan, 1995), which asserts that exposure to environments with “restorative” attributes, such as natural settings, facilitates the replenishment of attentional resources, thereby alleviating mental fatigue and stress. Therefore, as even a reproduction of nature environment yields psychological benefits, virtual reality has obvious advantages by directly bringing a calming environment to individuals, facilitating therapies for those with limited mobility or residing predominantly in urban areas. While passive observation of a relaxing environment can help cope with stress and anxiety, active techniques have recently emerged to achieve similar benefits. These include the practice of guided breathing exercises to achieve cardiac coherence.
Cardiac coherence is a physiological state where a subject breathes at the resonance frequency of the baroreflex (Lehrer et al., 2000). This frequency is close to 0.1 Hz, but may vary slightly from one individual to another (Vaschillo et al., 2006).
By adopting a breathing rate close to 6 breaths per minute (one every 10 s), the respiratory sinus arrhythmia shifts from 0.2–0.3 Hz, toward the frequency of 0.1 Hz. When these two oscillators (respiratory sinus arrhythmia and baroreflex) share the same frequency, a phenomenon of resonance occurs, considerably increasing the power of the autonomic nervous system. Essentially, during this state of cardiac coherence, heart rate fluctuations exhibit a nearly sinusoidal pattern, maintaining a rhythm with a period of 10 s.
To help participants achieve this physiological state, various cues can be given to help following the imposed breathing rhythm. It has recently been demonstrated that combining visual and haptic sensory inputs seems to be the best combination to potentiate cardiac coherence (Bouny et al., 2023). Additionally, feedback based on cardiac activity can be given to the participants in real time, allowing them to adapt their breathing pattern for best results. This method, so-called heart rate variability (HRV) biofeedback, has proved effective in helping participants achieve cardiac coherence (Vaschillo et al., 2006; Lehrer, 2013).
Cardiac coherence trained with HRV-biofeedback has been linked with physiological and psychological benefits. The technique was associated with an increased baroreflex activity (Vaschillo et al., 2002, 2006; Lehrer et al., 2003) and a greater vagal afferent input in the central autonomic network (Thayer and Lane, 2009; Lehrer and Gevirtz, 2014; Deschodt-Arsac et al., 2018; Mather and Thayer, 2018; Jester et al., 2019; Vanderhasselt and Ottaviani, 2022). These bottom-up heart-brain effects were associated in these studies with improved cognitive functioning and emotional management, congruent results regarding some previous works which demonstrate reduction of depressive symptoms after electrical stimulation of the vagus nerve (Sackeim et al., 2001a; Sackeim et al., 2001b; Nahas et al., 2005; Nahas et al., 2007; Daban et al., 2008; Cristancho et al., 2011). In particular, HRV-biofeedback helps reduce stress and anxiety (Prinsloo et al., 2013; Goessl et al., 2017; Deschodt-Arsac et al., 2018; Deschodt-Arsac et al., 2020), and could improve cognitive performance (Prinsloo et al., 2011; Sutarto et al., 2013; Blum et al., 2019).
Virtual reality (VR) devices are becoming more accessible and used in many industries. Based on this finding, head-mounted display (HMD) induction of cardiac coherence using HRV biofeedback has recently attracted increasing interest. In particular, the advantage of using a virtual environment lies in the possibility of modulating and reacting in real time according to the variations of the physiological state of the user over time, thus providing integrated feedback. At the same time, as virtual environments are able to induce a sense of presence in the user, they can be used to influence emotional states more than real environments (Riva et al., 2007). As biofeedback mechanisms are engaging and generate a sense of agency (Houzangbe et al., 2020), they can also enhance presence and therefore induce restorative effects.
The comparative implementation of HMD and desktop applications to induce cardiac coherence with HRV biofeedback in relaxing environments has already been studied in several experiments, but the conclusions are currently inconsistent. Implementation in HMD sometimes yields better results than 2D visualization (Blum et al., 2019), improving resilience to a stressful task following HRV biofeedback. However, most studies do not find significant differences in stress and anxiety reduction between virtual and 2D interventions (Rockstroh et al., 2020, 2019; Weibel et al., 2023). Likewise, even if a VR system may lead to a greater concentration of autonomous power below the resonant frequency (Weibel et al., 2023), no other differences in HRV indices were found. As reviewed by Lüddecke and Felnhofer (2022), VR HRV biofeedback is not more effective than traditional implementation. Nonetheless, virtual reality often leads to better motivation, user experience, attentional focus and engagement, which can help practitioners feel engaged in using the device and limit therapy dropouts. At last, it has been previously shown that guided breathing interventions performed with and without an additional relaxing underwater environment in VR are equally effective on the physiological and psychological levels (Soyka et al., 2016). However, we do not know yet if the same virtual environment, seen with and without performing HRV biofeedback, leads to similar results.
To the best of our knowledge, there is still a question about the rationale of using HRV biofeedback in virtual reality. Evidence on the effects of cardiac coherence, particularly when practiced with multisensory guidance (Bouny et al., 2023) suggests that it optimizes brain-heart coordination within the central autonomic network, leading to better stress and anxiety management. The restorative effects of a relaxing natural environment (Kaplan, 1995) presented in VR seem to achieve comparable psychological effects, through different neurophysiological pathways. Additionally, VR makes it possible to create environments that correspond to each individual’s needs, optimizing the motivational aspect and fostering the subjects’ commitment to practice, making it an ideal practice environment for cardiac coherence. Therefore, we wonder about the potential benefits of combining both practices: given that viewing relaxing environments can already reduce stress and anxiety, does an additional guided breathing exercise improve psychological states even further?
Previous studies that combined these practices suggest that they could bring additional benefits, but remain heterogeneous in their conclusions (Soyka et al., 2016; Rockstroh et al., 2020, 2019; Blum et al., 2019; Lüddecke and Felnhofer, 2022; Weibel et al., 2023). As far as we are aware, they have not used exactly the same tools and feedback during the breathing exercises performed in real and virtual settings. Here, using the same multisensory guidance should allow participants to reach similar levels of cardiac coherence in both settings. In this context, the present study aims to improve our understanding of the effects of both cardiac coherence and relaxing natural VR environment - either isolated or combined—on stress and anxiety. By measuring cardiac coherence performance with physiological data, we will be able to decipher whether the potential differences observed in stress and anxiety reduction are due to better cardiac coherence practice enabled by virtual reality, or to an additional effect of the relaxing environment. Finally, while the physiological benefits of cardiac coherence have already been well evidenced, there is still a question concerning the impact of viewing a relaxing environment on physiological variables.
To this end, we carried out an experiment composed of two distinct protocols in which all the participants took part: the Real protocol, during which they performed HRV biofeedback using a handheld device, and the HMD protocol in virtual reality, where subjects were immersed twice in a relatively relaxing virtual environment, first without any stimulation, and then performing guided breathing with the same visual and haptic cues as in the Real protocol.
We hypothesized that: H1A–Performing guided breathing, either in a real or in a virtual environment, should help reduce stress and anxiety values.
H1B – Viewing the “relaxing” environment in virtual reality while breathing at the resonance frequency should lead to an even greater reduction in perceived stress and anxiety levels than performing guided breathing alone.
H2 – Guided breathing in Real and HMD conditions should help achieve the same level of cardiac coherence assessed by physiological data.
H3 – Improvements in psychological states should come with improved physiological states.
Testing these hypotheses should enable us to determine whether the beneficial effects observed in the literature related to the practice of cardiac coherence in virtual reality are due to the practice of cardiac coherence itself, to immersion in a relaxing virtual environment, or a combination of both. These results could be used to adapt the type of stimulation to the needs and profiles of end-users.
36 healthy sport students (9 females, 19.2 ± 1.3 years old) gave their informed consent to participate in this experiment for which they received credits as part of their academic curriculum. The institutional review board “Faculté des STAPS” approved the procedure that respected all ethical recommendations and followed the declaration of Helsinki. All the participants had normal or corrected-to-normal vision. Participants were instructed not to consume caffeine or alcohol at least 24 h before the experiment. None of them had any experience with the VR task used here, but some had previously participated in protocols including HRV biofeedback.
The sample size was determined from pre-tests concerning anxiety measurements before and after a 5-minute immersion in a relaxing environment. An a priori power analysis with G*Power (Faul et al., 2007) for anxiety measurements with an effect size of 0.67, α = 0.05 and power = 0.95 indicated a theoretical sample size of n = 26. To add more statistical power, and as more dropouts were expected between both passages or due to cybersickness, 41 participants were recruited, 36 of whom completed the entire protocol and had fully exploitable data (1 did not show up for both protocols, and 4 electrocardiogram signals were of poor quality).
The experiments were carried out in a silent experiment room, at a constant temperature of 20°C, with windows whose curtains were lowered so that the lighting remained more or less the same at all times of day. The experimental setup consisted of two distinct protocols (Real and HMD protocols). Upon arrival, the experimenter explained the protocol to the participants, and equipped them with electrodes for electrocardiogram (ECG) recording. Both the Real and HMD protocols started with 5 min of questionnaires, acting as a baseline for heart rate variability (HRV) measurements (Figure 1). After that, during the Real protocol, participants performed 5 min of guided breathing at 0.1 Hz, using the device presented in Section 2.3, and then answered a second set of questionnaires. In the HMD protocol, participants were equipped with an HTC Vive Pro headset (HTC America, Inc., Seattle, WA, United Stated), and were immersed in the virtual environment. Then, they performed two conditions: Immersion and Cardiac Coherence. Participants were first instructed to look roughly in front of them, without making sudden movements, to avoid generating cybersickness. After 5 min of immersion, they completed a questionnaire outside of the virtual environment. They were then immersed again, performing 5 min of guided breathing. At the end of the protocol, they completed the last iteration of the questionnaire.
Figure 1. Experimental protocol. Every condition lasts 5 min. Completing questionnaires in both protocols took 5 min on average. During baselines, if the participants had completed the questionnaire before the end of the 5-minute recording, they were instructed to read an emotionally neutral text displayed on the screen.
The protocol order (Real or HMD protocol first) was randomly determined. The two protocols were separated by a minimum delay of 1 week. Inside the HMD protocol, the conditions Immersion and Cardiac Coherence could not be randomized, as performing HRV biofeedback produces long-lasting physiological effects. The Immersion condition is thus always performed first.
As the effects of cardiac coherence appear after only a few minutes (Lehrer and Gevirtz, 2014; Lehrer et al., 2020), we chose to perform 5-minute blocks. This specific duration of HRV biofeedback session has previously been associated with beneficial effects on stress and anxiety (Bouny et al., 2023).
To perform guided breathing, participants used a tool held in their dominant hand giving visual and haptic cues Figure 2, already used in a previous study (Bouny et al., 2023). The breathing pace was set to 0.1 Hz, with 5 s of inspiration and 5 s of expiration. During the inspiration phase, the vertical bar is progressively filled with a light, before emptying during expiration. The color of the light acted as a biofeedback, with three different levels of performance computed based on the smoothness of the recorded HRV. The colors chosen for the low, medium and high levels, expressed in RGB values (range 0–1) were orange (1, 0.59, 0), violet (0.71, 0.29, 0.85) and turquoise (0.44, 0.86, 0.91). The haptic cue was given in the form of a vibration with increasing and decreasing intensities during inspiration and expiration, respectively. During the Real protocol, the participants were asked to keep their eyes on the device and focus on the visual and haptic cues. In the HMD protocol, the device was still handheld, thus giving the same haptic feedback as in the Real protocol, but the visual feedback was presented in the virtual environment, as described in Section 2.5.
A coherence score is calculated in real time to give feedback to the user. For that, a photoplethysmography (PPG) sensor, located below the thumb, detects the user’s heartbeat and compares its variation to the 0.1 Hz sinus wave expected in a cardiac coherence state. This calculation takes place after each detected heartbeat, and updates a coherence score. Heart-rate-variability biofeedback is represented as a color change on the vertical bar of the device. The participants are aware of the meanings of the different colors, with blue, purple and orange representing good, medium and bad coherence, respectively.
For both protocols, the cardiac coherence score presented in the results is recalculated a posteriori with ECG data.
The virtual environment was developed with Unity (Unity Technologies, San Francisco, CA, United States), and represents an island floating in a sea of clouds (Figure 3).
Figure 2. Guided breathing device. The participant’s thumb is placed on a photoplethysmography captor, recording interbeat intervals in real time and showing coherence performance as the light’s color. The light and vibration go up and down in 5-s phases to guide breathing cycles at a 0.1 Hz resonance frequency.
Figure 3. Views of the virtual environment. Top: environment seen in the “Immersion” condition. Down: environment with the sphere used for guided breathing.
This scene has been designed according to the elements of the Restorative Attention Theory (Kaplan, 1995) to induce a feeling of “being away.” The environment includes nature elements (rocks, grass and trees), a large vista and dimmed lights. The main elements of the scene are all in front of the participants, to prevent them from moving their head when looking at details. This way, the head movements stay the same when the participants see the environment in the Immersion condition and the Cardiac Coherence condition, where the ball to focus on is present.
Some of the elements in the scene are moving, but none of them with a frequency that could be used as a respiratory guide by the user. During the guided breathing phase of the HMD protocol, a white sphere placed in the middle of the user’s view was added; the size of this element increased and decreased for 5 s in a sigmoidal pattern during the inspiration and expiration phases, respectively. Using the same palette as the real device (see Section 2.3), HRV biofeedback was provided as the color of the sphere.
There were no additional auditory stimuli in virtual reality, so that the only difference between the Real and HMD protocols lies in the visual stimulations.
Levels of stress and anxiety were measured twice during the Real protocol and three times in the HMD protocol (Figure 1). Participants were asked to provide their level of stress by moving a slider on a visual analog scale (VAS), labeled from “No Stress” (0) to “Extreme Stress” (100). The definition of stress given to participants was the following: Stress is a normal reaction of the body to an external event or to something perceived as threatening, which is an easy-to-understand version based on Hans Selye’s definition (Selye, 1956).
The level of anxiety was calculated using the Spielberger’s State-Trait Anxiety Inventory (STAI-Y, Spielberger, 1983) questionnaire, consisting of 20 questions and providing scores ranging from 20 to 80.
The sense of presence in the virtual environment, which has been linked with emotional states (Riva et al., 2007), workload and stress (Lackey et al., 2016), was measured using the Igroup Presence Questionnaire (Schubert et al., 2001).
Cybersickness was assessed with the Simulator Sickness Questionnaire (SSQ, French version, Bouchard et al., 2009), and was used to ensure that the evolution of the participants’ physiological and psychological states were effectively related to the different interventions, and not to a marked state of discomfort.
Both questionnaires were completed in the HMD protocol following the 5-min immersion and guided-breathing phases.
For the entire duration of the protocol, the participants’ electrocardiogram was recorded with three electrodes using a PowerLab 8/35 device (ADInstruments, Dunedin, New Zealand) and a dedicated bioamplifier (FE132, ADInstruments, Dunedin, New Zealand) at a 1 kHz sampling frequency.
ECG recordings were then cut into 5-minutes sequences corresponding to the different phases of both protocols: Baseline and Cardiac coherence in the Real protocol, and Baseline, Immersion and Cardiac coherence in the HMD protocol (Figure 1).
In the HMD protocol, cardiac interbeat intervals (R-to-R peaks interval duration) were computed in real time and transmitted to the virtual environment software to calculate the cardiac coherence score using the same algorithm as the one implemented in the real device (see Section 2.4).
The RR intervals time series were exported to Matlab (Matlab 2021b; Matworks, Natick, MA, USA), and several heart rate variability (HRV) indicators were computed using custom algorithms.
Artefacts were identified from differences between two successive intervals larger than 250 ms. Artefacted RR were replaced by the average value of the nearby values. Indices of HRV in time and frequency domains were then calculated. The root mean square of successive differences between heartbeats (RMSSD) for an RR time series of size N was calculated as follows:
RR times series were analyzed in the frequency domain to compute autonomous power. Each 5-minutes individual time series were first resampled at 4 Hz using a cubic spline interpolation, and a fast Fourier transform was used to compute the Power Spectral Density (PSD). From the PSD, we computed the total power in the signal within the range 0.04 Hz–0.4 Hz, the height of the resonance peak located around 0.1 Hz, and the P0.1 index, which corresponds to the ratio between the power of the highest peak (resonance) during cardiac coherence and the total power in Baseline. This ratio is used as a cardiac coherence performance metric, and measures to which extent the total autonomous power is concentrated under the resonant frequency (Bouny et al., 2023).
Normal distribution for datasets was assessed using a Shapiro-wilk test. Datasets from questionnaires were analyzed for the Real and HMD conditions independently.
As there are only two measuring points in the Real condition, statistical analysis concerning this part of the protocol are performed using either a Wilcoxon signed rank or a paired samples t-test. In the HMD protocol, one-way repeated measures ANOVAs or Friedman tests were first performed, followed by a post-hoc Tukey or Dunn (for parametric and non-parametric tests, respectively) if differences were found.
Bayesian equivalents to standard statistical tests were also used to deepen understanding and guide interpretation of significance, based on the probability of the alternative hypothesis relative to the null hypothesis. To circumvent the problem that non-significant p-values cannot be interpreted as support for the null hypothesis (Rouder et al., 2009; Keysers et al., 2020), we calculated the Bayes factor (BF) when it was interesting to measure the proximity of the effects provided by two methods. Specifically, we computed the log scale of BF (noted log(BF10), see (Bouny et al., 2021) for an interpretation scale of this parameter) that can be easily interpreted such that a negative value indicates support for the null hypothesis (H0), while a positive value indicates evidence for the alternative hypothesis.
All statistical analyses were performed using Matlab with the Statistical and Machine Learning Toolbox.
Considering answers to the presence questionnaire in virtual reality, no difference was observed for values of IPQ (paired samples t-test: p = 0.15, t = −1.46) measured after immersion (52.0 ± 7.4) and after guided breathing (54.0 ± 9.3). Considering cybersickness, a significant difference (Wilcoxon signed rank test: p = 0.004, z = −2.88) was described between values of SSQ measured after immersion (5.5 ± 3.8) and after guided breathing in virtual reality (7.1 ± 4.5) (Table 1).
Table 1. Scores recorded from questionnaires in both the Real and HMD protocols. Values are displayed in median (quartile 1 - quartile 3).
This increase in SSQ values is significant, but all scores remained low (five participants reported a score >10 at the beginning, nine at the end, and there were no scores >20). Moreover, there were no correlations between SSQ values - as well as their evolution - and other variables.
During the Real protocol, STAI-Y as well as VAS Stress values significantly decreased between Baseline and Cardiac coherence conditions (respectively p = 0.001, Z = 3.68 and p = 0.01, Z = 2.48).
In the HMD protocol, a decrease of STAI-Y values (Friedman: χ2(2) = 7.72, p = 0.02) and VAS Stress values (Friedman: χ2(2) = 25.62, p = 2.7 × 10−6) was found as well. Considering VAS Stress values, a post-hoc Tukey showed significant differences between conditions Baseline and Immersion (p = 1.6 × 10−4) and Baseline and Cardiac coherence (p = 9.1 × 10−6), but no differences between Immersion and Cardiac coherence (p = 0.80; log(BF10) = −0.69, moderate evidence for H0). For STAI-Y values, a significant decrease was found between Baseline and Immersion (p = 0.03), but no differences were found between Baseline and Cardiac coherence (p = 0.06) and between Immersion and Cardiac coherence (p = 0.98; log(BF10) = −1.55, very strong evidence for H0).
The absence of significant differences between the phases of the experiment could be explained by the fact that some participants already had low stress and anxiety scores at the beginning of the protocols. If a participant scores the minimal possible value at the start, improvements in psychological states cannot be detected. Moreover, as many participants reported no or little stress and anxiety, these values are not evenly distributed, with a big cluster of data located near the minimal scores. Then, to further explore the effects of the experimental conditions (Real and HMD) on psychological values, we divided the population into two groups (+ and -) according to the baseline values of the STAI-Y and the VAS Stress values in each protocol. Participants with STAI-Y scores above and below the median of the baseline condition for the Real and HMD protocols (both medians = 25) were placed in the Anxiety+ (n = 17) and Anxiety- (n = 19) groups, respectively.
For the less anxious participants (Anxiety-group), there were no evolutions of STAI-Y values measured during the two protocols, comparing Baseline and Cardiac Coherence for the Real one, and Baseline, Immersion and Cardiac coherence for the Virtual protocol (Real: Wilcoxon signed rank test, p = 0.16, Z = 1.40; HMD: Friedman, χ2(2) = 0.24, p = 0.89). However, for the most anxious participants, (Anxiety+ group), STAI-Y scores significantly lowered through the course of both protocols (Real: Wilcoxon signed rank test, p = 0.004, Z = 2.90; HMD: Friedman, χ2(2) = 17.37, p = 1.7 × 10−4).
In the HMD protocol, a post-hoc Dunn showed differences between the Baseline and Cardiac coherence conditions (p = 0.001) and between Baseline and Immersion (p = 0.001), but no differences between Immersion and Cardiac coherence (p = 1.00) (Figure 4).
Figure 4. Evolution of STAI-Y scores (left) and VAS Stress values (right), for the Anxiety-/Stress- groups (bottom) and the Anxiety+/Stress+ groups (top). Results are presented in median ± interquartile, with min and max values. Outliers are represented as individual points. Brown lines: Real protocol. Blue lines: HMD protocol. p-values comparing scores in the Real protocol are calculated from a Wilcoxon signed-rank test. p-values comparing scores in the HMD protocol are the results of the post-hoc Dunn following a Friedman test (STAI-Y scores) or a one-way ANOVA (VAS Stress values).
Participants with VAS Stress scores above and below the median of the baseline condition for the Real (median = 9.5) and HMD (median = 10) protocols were placed in the Stress+ (n = 18) and Stress- (n = 18) groups, respectively. For the Stress-group, there were no differences in VAS Stress values in both protocols (Real: Wilcoxon signed rank test, p = 0.86, Z = 0.18; HMD: Friedman, χ2(2) = 2.32, p = 0.31) (Figure 4). Conversely, VAS Stress scores significantly lowered for the Stress+ group in both protocols (Real: Wilcoxon signed rank test, p = 0.005, Z = 2.79; HMD: one-way ANOVA, F(2,34) = 19.88, p = 1.9 × 10−6). In the HMD protocol, a post-hoc Tukey showed significant decreases between the Baseline and Immersion conditions (p = 0.001) and between Baseline and Cardiac coherence (p = 2.0 × 10−4), but no differences between Immersion and Cardiac coherence (p = 0.22) (Figure 4).
In the Real protocol, RMSSD increased between Baseline and Cardiac coherence (Wilcoxon signed rank test: p = 1.2 × 10−4, Z = −3.85) Table 2.
Table 2. Physiological markers computed from the electrocardiogram recorded in both the Real and HMD protocols. Values are displayed in median (quartile 1 - quartile 3).
RMSSD increased during the HMD protocol (Friedman: χ2(2) = 41.06, p = 1.2 × 10−9), with significant differences between Baseline and Cardiac coherence (p = 1.2 × 10−9), between Cardiac coherence and Immersion (p = 3.9 × 10−5). No differences appeared between Baseline and Immersion conditions (p = 0.17; log(BF10) = −0.47, anecdotal evidence for H0).
The same results were shown for total power. A significant increase was found in both the Real protocol (paired samples t-test: p = 3.2 × 10−6, t = −5.5) and the HMD protocol (Friedman: χ2(2) = 36.72, p = 1.1 × 10−8). In the latter, total power increased between conditions Baseline and Cardiac Coherence (p = 2.3 × 10−5) and between Immersion and Cardiac coherence (p = 2.3 × 10−5), but no differences were found between Baseline and Immersion (p = 0.48; log(BF10) = −0.75, moderate evidence for H0) Figure 5.
Figure 5. Evolution of RMSSD (left) and Total Power (right). Results are presented in median ± interquartile, with min and max values. Brown lines: Real protocol. Blue lines: HMD protocol. p-values comparing scores in the Real protocol are calculated from a Wilcoxon signed-rank test. p-values comparing scores in the HMD protocol are the results of the post-hoc Dunn following a Friedman test.
All participants achieved cardiac coherence (for an example of signal, see Figure 6). Cardiac coherence scores during the guided breathing phase in Real and HMD protocols were not different (Wilcoxon signed-rank test: p = 0.80, Z = 0.25). This non-significant result was supported by the bayesian analysis which indicates strong evidence for H0 (log(BF10) = −1.33). A significant correlation was shown between the score obtained in Real condition (77.6% ± 10.4%) and the HMD condition (76.3% ± 10.7%) (Pearson correlation: r2 = 0.43, p = 1.4 × 10−5). The higher the participants’ score in one condition, the higher their score in the other.
Figure 6. Example of RR intervals recorded during the baseline (left) and guided breathing (right) phases, with their corresponding power spectral density. The PSD during cardiac coherence shows a clear peak at 0.1 Hz.
A two-way ANOVA performed on Protocol (Real or HMD) and Anxiety Level (- or +) as independent factors showed that the cardiac coherence score was neither affected by the display (F (1,71) = 0.27, p = 0.61) nor starting anxiety levels (F (1,71) = 0.58, p = 0.45). There was no interaction effect (F (1,71) = 0.01, p = 0.91).
There were no differences concerning maximum peak height in the PSD at the resonance frequency around 0.1 Hz in Real and HMD protocols during cardiac coherence (paired samples t-test: p = 0.89, t = −0.12; Real: 54.7 ± 22.3 ms2/Hz; HMD: 55.0 ± 20.5 ms2/Hz), and a significant correlation between peak heights in both protocols was observed (Pearson correlation: r2 = 0.64, p = 4.8 × 10−9). Likewise, there were no differences in total power computed within the range 0.04 Hz–0.4 Hz at baseline between both protocols (Wilcoxon signed rank test: p = 0.27, Z = 1.10; Real: 1.19 ± 0.48 ms2; HMD: 1.11 ± 0.51 ms2).
The analysis of the P0.1 index showed that cardiac coherence was identically achieved during the guided breathing phase in the Real and HMD protocols (log(BF10) = −1.62, very strong evidence for H0; Wilcoxon signed rank test: p = 0.19, Z = −1.32; Real: 51.3 ± 25.3 Hz-1; HMD: 53.3 ± 18.8 Hz-1), with a significant correlation between P0.1 values computed in the Real and HMD protocols (Pearson correlation: r2 = 0.17, p = 0.01).
A two-way ANOVA performed on Protocol (Real or HMD) and Anxiety Level (- or +) as independent factors showed that P0.1 was not affected by the display (F (1,71) = 0.10, p = 0.76) nor starting anxiety levels (F (1,71) = 1.80, p = 0.18). There was no interaction effect (F (1,71) = 1.13, p = 0.29).
There was no correlation between cardiac coherence scores and P0.1 values, neither in the Real (Pearson correlation: r2 < 0.01, p = 0.57) nor the HMD protocol (Pearson correlation: r2 = 0.02, p = 0.43).
The aim of the present study was to explore the relevance of incorporating HRV biofeedback within virtual reality. Under our experimental conditions, we confirmed that cardiac coherence significantly improved participants’ psychological state by reducing signs of stress and anxiety, thus confirming H1A. Notably, the participants who reported the highest levels of anxiety at baseline (Anxiety +) experienced the most significant improvements in their psychological state after the cardiac coherence session. This finding aligns with existing literature, which suggests the possible existence of different anxiety patterns (Dickerson and Kemeny, 2004; Dimitriev et al., 2016; Blons et al., 2019). However, the improvement in psychological states was no different in the Real and HMD protocols.
Initially, one might have assumed that the two tested methods (HMD and Real) would have different effects on the subjects. Some studies suggested that conducting HRV biofeedback in VR may help reduce mental wandering (Lüddecke and Felnhofer, 2022), enabling the subjects to maintain better focus during the HMD protocol, thus resulting in higher cardiac coherence scores and P0.1 values compared to real-life situations. Moreover, incorporating HRV biofeedback guidance with haptic stimuli in virtual reality has been demonstrated to enhance coherence performance (Bouny et al., 2023). As sensory inputs are not processed similarly in virtual reality, partly due to visuo-vestibular conflicts, some participants may have been more responsive to either the Real or HMD modalities. However, our findings show similar average results between HMD and Real protocols, with a significant correlation between P0.1 and cardiac coherence scores (see sections 3.4 and 3.5). In fact, it appears that both induction methods yield comparable effects, providing support for H2.
Experiencing a relaxing virtual reality environment with natural elements for 5 minutes proves sufficient in our study to induce significant psychological benefits, which were not further enhanced with the use of HRV biofeedback. As a result, our hypothesis H1B could not be confirmed. It is essential to note that these results were obtained from young, healthy participants with low levels of anxiety at the beginning of the protocol (Real: 25 (21–29); HMD: 25 (23–30.5), measured with a scale ranging from 20 to 80). This suggests that the potential for improving stress and anxiety management through methods like HRV biofeedback may be limited in this specific group. An intriguing direction for future research could involve applying this method to pathological and/or stressed populations.
There are two reasons for choosing a population of young athletes. Firstly, as we are measuring physiological data, testing young, active individuals avoids inducing too much variability linked to aging, sedentariness or other diseases. Then, even if this population is not highly stressed to begin with, young student athletes are subject to many stressful elements, such as competitions and exams. It has also been proven in the past that practicing cardiac coherence within this population helps reduce anxiety during exam periods (Deschodt-Arsac et al., 2018).
Despite the significant reduction in stress and anxiety achieved within 5 min of immersion in the pleasant VR environment without performing guided breathing, these changes are not accompanied by physiological alterations. In fact, the cardiac indices (RMSSD and total power, as shown in Figure 5) remained unchanged. Consequently, our findings suggest that immersion alone, without an optimization of autonomous regulation, might have only a transient effect, lacking a lasting impact over time, unlike the longer-term effects observed with cardiac coherence training (Deschodt-Arsac et al., 2018; Bögge et al., 2022; de Souza et al., 2022). Therefore, H3 is not supported in the control immersion condition.
There are several limitations to this study that should be addressed. Firstly, we employed a simple fixed breathing rate of 0.1 Hz for all participants. However, as demonstrated in previous works by Lehrer et al. (2003) and Vaschillo et al. (2006), the resonant frequency can vary between individuals, depending on their individual baroreflex frequency. It would be valuable to assess each individual’s resonance frequency and apply it to the breathing rate in both the Real and HMD protocols. Although this procedure introduces design complexities - and does not seem to induce significant physiological benefits in many cases (Tabor et al., 2022), it is worth considering due to the heterogeneity of some of our results, particularly among subjects with different levels of anxiety. If the HRV biofeedback method is optimized for everyone, its effects could potentially complement the benefits already observed in this study during virtual reality immersion in a natural environment.
Furthermore, a recent study on meditation in virtual reality, where participants performed exercises at a controlled respiratory rate (10 breaths per minute) higher than the resonant frequency, demonstrated significantly better anxiety reduction when virtual scene elements moved independently of the participant’s breathing (Tinga et al., 2019). This highlights the need for continued experimentation to better understand the relationship between virtual reality and physiological induction, especially when biofeedback is involved. It is important to note that the tested subjects were all young, healthy adults, and despite the results not being significant, individual data showed considerable heterogeneity, suggesting the possibility of individual response profiles to the effect of HRV biofeedback in VR. It could be interesting to test this protocol on a control group that did not receive any interventions, or in professionals who experienced a marked increase in workload or stress during their activities.
Lastly, our primary focus was to examine the effects of an immersion in a virtual reality environment, with or without cardiac coherence. However, it remains uncertain whether similar effects could be observed outside the virtual environment, such as when observing a pleasant nature sequence on a 2D screen or with a 360° view (Li et al., 2021).
The results of this experiment confirm the findings of previous studies describing HRV biofeedback practice with guided breathing at a fixed frequency of 0.1 Hz (resonance frequency) as a method that brings about such psychological and physiological advantages. These advantages have been found with both the device- and HMD-based implementations of HRV biofeedback, using visual and haptic cues.
As expected, seeing a visually pleasant virtual environment with nature elements while breathing normally already brings significant reductions in stress and anxiety levels, which are not further reduced when performing HRV biofeedback. However, if the effects are similar on a psychological level, they are different when considering physiological states. Based on the results of our study, the additional use of HRV biofeedback applied in this VR environment does not bring any additional effects regarding the psychological state of the subjects, which was already optimized by the initial immersion. Therefore, is there a rationale of using HRV biofeedback in VR, since it does not seem to provide any additional psychological benefits compared to simple immersion and the use of a real device?
First of all, it must be kept in mind that, even though it led to reduced states of stress and anxiety, the baseline immersion in virtual reality did not result in physiological modulations, which means that these effects may not last over time, and may not improve cognitive performances. Then, these results were obtained with a population of young athletes, many of whom were neither stressed or anxious at the time of the experiment, and may be dependent on the type of virtual environment presented. Finally, when comparing HRV biofeedback effects in real and virtual conditions, it is essential to keep in mind that VR offers various advantages, particularly concerning participant engagement and subjective appreciation. Therefore, since the adherence of subjects to perform cardiac coherence sessions (or similar practices) over time is a challenge that industry professionals are trying to address, better adherence could be achieved through the use of VR (due to its more enjoyable and engaging nature, and the possibility to simulate infinite environments). The type of stimulation and their induction methods should be tailored to the target audience.
The raw data supporting the conclusion of this article will be made available by the authors, without undue reservation.
The studies involving humans were approved by Institutional Review Board “Faculté des STAPS”. The studies were conducted in accordance with the local legislation and institutional requirements. The participants provided their written informed consent to participate in this study.
YP: Conceptualization, Data curation, Formal Analysis, Investigation, Methodology, Software, Writing–original draft, Writing–review and editing. PB: Conceptualization, Investigation, Methodology, Writing–original draft, Writing–review and editing. VD-A: Conceptualization, Methodology, Writing–original draft, Writing–review and editing.
The author(s) declare that financial support was received for the research, authorship, and/or publication of this article.
The authors declare that the research was conducted in the absence of any commercial or financial relationships that could be construed as a potential conflict of interest.
All claims expressed in this article are solely those of the authors and do not necessarily represent those of their affiliated organizations, or those of the publisher, the editors and the reviewers. Any product that may be evaluated in this article, or claim that may be made by its manufacturer, is not guaranteed or endorsed by the publisher.
Anderson, A. P., Mayer, M. D., Fellows, A. M., Cowan, D. R., Hegel, M. T., and Buckey, J. C. (2017). Relaxation with immersive natural scenes presented using virtual reality. Aerosp. Med. Hum. Perform. 88, 520–526. doi:10.3357/AMHP.4747.2017
Blons, E., Arsac, L. M., Gilfriche, P., McLeod, H., Lespinet-Najib, V., Grivel, E., et al. (2019). Alterations in heart-brain interactions under mild stress during a cognitive task are reflected in entropy of heart rate dynamics. Sci. Rep. 9, 18190. doi:10.1038/s41598-019-54547-7
Blum, J., Rockstroh, C., and Göritz, A. S. (2019). Heart rate variability biofeedback based on slow-paced breathing with immersive virtual reality nature scenery. Front. Psychol. 10, 2172. doi:10.3389/fpsyg.2019.02172
Bögge, L., Colás-Blanco, I., and Piolino, P. (2022). Respiratory sinus arrhythmia during biofeedback is linked to persistent improvements in attention, short-term memory, and positive self-referential episodic memory. Front. Neurosci. 16, 791498. doi:10.3389/fnins.2022.791498
Bouchard, S., St-Jacques, J., Renaud, P., and Wiederhold, B. K. (2009). Side effects of immersions in virtual reality for people suffering from anxiety disorders. J. CyberTherapy Rehabil. 2, 127–138.
Bouny, P., Arsac, L. M., Guérin, A., Nerincx, G., and Deschodt-Arsac, V. (2023). Guiding breathing at the resonance frequency with haptic sensors potentiates cardiac coherence. Sensors 23, 4494. doi:10.3390/s23094494
Bouny, P., Arsac, L. M., Touré Cuq, E., and Deschodt-Arsac, V. (2021). Entropy and multifractal-multiscale indices of heart rate time series to evaluate intricate cognitive-autonomic interactions. Entropy 23, 663. doi:10.3390/e23060663
Corazon, S. S., Sidenius, U., Poulsen, D. V., Gramkow, M. C., and Stigsdotter, U. K. (2019). Psycho-physiological stress recovery in outdoor nature-based interventions: a systematic review of the past eight years of research. Int. J. Environ. Res. Public. Health 16, 1711. doi:10.3390/ijerph16101711
Cristancho, P., Cristancho, M. A., Baltuch, G. H., Thase, M. E., and O†Reardon, J. P. (2011). Effectiveness and safety of vagus nerve stimulation for severe treatment-resistant major depression in clinical practice after FDA approval: outcomes at 1 year. J. Clin. Psychiatry 72, 1376–1382. doi:10.4088/JCP.09m05888blu
Daban, C., Martinez-Aran, A., Cruz, N., and Vieta, E. (2008). Safety and efficacy of Vagus Nerve Stimulation in treatment-resistant depression. A systematic review. J. Affect. Disord. 110, 1–15. doi:10.1016/j.jad.2008.02.012
de Kort, Y. A. W., Meijnders, A. L., Sponselee, A. A. G., and Ijsselsteijn, W. A. (2006). What’s wrong with virtual trees? Restoring from stress in a mediated environment. J. Environ. Psychol. 26, 309–320. doi:10.1016/j.jenvp.2006.09.001
Deschodt-Arsac, V., Blons, E., Gilfriche, P., Spiluttini, B., and Arsac, L. M. (2020). Entropy in heart rate dynamics reflects how HRV-biofeedback training improves neurovisceral complexity during stress-cognition interactions. Entropy 22, 317. doi:10.3390/e22030317
Deschodt-Arsac, V., Lalanne, R., Spiluttini, B., Bertin, C., and Arsac, L. M. (2018). Effects of heart rate variability biofeedback training in athletes exposed to stress of university examinations. PloS One 13, e0201388. doi:10.1371/journal.pone.0201388
de Souza, P. M., de Cássia Souza, M., Diniz, L. A., Araújo, C. R. V., Lopez, M., Volchan, E., et al. (2022). Long-term benefits of heart rate variability biofeedback training in older adults with different levels of social interaction: a pilot study. Sci. Rep. 12, 18795. doi:10.1038/s41598-022-22303-z
Dickerson, S. S., and Kemeny, M. E. (2004). Acute stressors and cortisol responses: a theoretical integration and synthesis of laboratory research. Psychol. Bull. 130, 355–391. doi:10.1037/0033-2909.130.3.355
Dimitriev, D. A., Saperova, E. V., and Dimitriev, A. D. (2016). State anxiety and nonlinear dynamics of heart rate variability in students. PLOS ONE 11, e0146131. doi:10.1371/journal.pone.0146131
Egger, G., and Dixon, J. (2014). Beyond obesity and lifestyle: a review of 21st century chronic disease determinants. Biomed. Res. Int. 2014, 1–12. doi:10.1155/2014/731685
Faul, F., Erdfelder, E., Lang, A.-G., and Buchner, A. (2007). G*Power 3: a flexible statistical power analysis program for the social, behavioral, and biomedical sciences. Behav. Res. Methods 39, 175–191. doi:10.3758/BF03193146
Goessl, V. C., Curtiss, J. E., and Hofmann, S. G. (2017). The effect of heart rate variability biofeedback training on stress and anxiety: a meta-analysis. Psychol. Med. 47, 2578–2586. doi:10.1017/S0033291717001003
Houzangbe, S., Christmann, O., Gorisse, G., and Richir, S. (2020). Effects of voluntary heart rate control on user engagement and agency in a virtual reality game. Virtual Real 24, 665–681. doi:10.1007/s10055-020-00429-7
Jester, D. J., Rozek, E. K., and McKelley, R. A. (2019). Heart rate variability biofeedback: implications for cognitive and psychiatric effects in older adults. Aging Ment. Health 23, 574–580. doi:10.1080/13607863.2018.1432031
Kaplan, S. (1995). The restorative benefits of nature: toward an integrative framework. J. Environ. Psychol. Green Psychol. 15, 169–182. doi:10.1016/0272-4944(95)90001-2
Keysers, C., Gazzola, V., and Wagenmakers, E.-J. (2020). Using Bayes factor hypothesis testing in neuroscience to establish evidence of absence. Nat. Neurosci. 23, 788–799. doi:10.1038/s41593-020-0660-4
Lackey, S. J., Salcedo, J. N., Szalma, J. L., and Hancock, P. A. (2016). The stress and workload of virtual reality training: the effects of presence, immersion and flow. Ergonomics 59, 1060–1072. doi:10.1080/00140139.2015.1122234
Lehrer, P. (2013). How does heart rate variability biofeedback work? Resonance, the baroreflex, and other mechanisms. Biofeedback 41, 26–31. doi:10.5298/1081-5937-41.1.02
Lehrer, P., Kaur, K., Sharma, A., Shah, K., Huseby, R., Bhavsar, J., et al. (2020). Heart rate variability biofeedback improves emotional and physical health and performance: a systematic review and meta analysis. Appl. Psychophysiol. Biofeedback 45, 109–129. doi:10.1007/s10484-020-09466-z
Lehrer, P. M., and Gevirtz, R. (2014). Heart rate variability biofeedback: how and why does it work? Front. Psychol. 5, 756. doi:10.3389/fpsyg.2014.00756
Lehrer, P. M., Vaschillo, E., and Vaschillo, B. (2000). Resonant frequency biofeedback training to increase cardiac variability: rationale and manual for training. Appl. Psychophysiol. Biofeedback 25, 177–191. doi:10.1023/a:1009554825745
Lehrer, P. M., Vaschillo, E., Vaschillo, B., Lu, S.-E., Eckberg, D. L., Edelberg, R., et al. (2003). Heart rate variability biofeedback increases baroreflex gain and peak expiratory flow. Psychosom. Med. 65, 796–805. doi:10.1097/01.psy.0000089200.81962.19
Li, H., Zhang, X., Wang, H., Yang, Z., Liu, H., Cao, Y., et al. (2021). Access to nature via virtual reality: a mini-review. Front. Psychol. 12, 725288. doi:10.3389/fpsyg.2021.725288
Liszio, S., Graf, L., and Masuch, M. (2018). The relaxing effect of virtual nature: immersive technology provides relief in acute stress situations. Annu. Rev. CyberTherapy Telemed. 16, 87–93.
Lüddecke, R., and Felnhofer, A. (2022). Virtual reality biofeedback in health: a scoping review. Appl. Psychophysiol. Biofeedback 47, 1–15. doi:10.1007/s10484-021-09529-9
Mather, M., and Thayer, J. F. (2018). How heart rate variability affects emotion regulation brain networks. Curr. Opin. Behav. Sci. Emotion-cognition Interact. 19, 98–104. doi:10.1016/j.cobeha.2017.12.017
Nahas, Z., Marangell, L. B., Husain, M. M., Rush, A. J., Sackeim, H. A., Lisanby, S. H., et al. (2005). Two-year outcome of vagus nerve stimulation (VNS) for treatment of major depressive episodes. J. Clin. Psychiatry 66, 1097–1104. doi:10.4088/jcp.v66n0902
Nahas, Z., Teneback, C., Chae, J.-H., Mu, Q., Molnar, C., Kozel, F. A., et al. (2007). Serial vagus nerve stimulation functional MRI in treatment-resistant depression. Neuropsychopharmacology 32, 1649–1660. doi:10.1038/sj.npp.1301288
Park, S.-A., Song, C., Oh, Y.-A., Miyazaki, Y., and Son, K.-C. (2017). Comparison of physiological and psychological relaxation using measurements of heart rate variability, prefrontal cortex activity, and subjective indexes after completing tasks with and without foliage plants. Int. J. Environ. Res. Public. Health 14, 1087. doi:10.3390/ijerph14091087
Prinsloo, G. E., Derman, W. E., Lambert, M. I., and Rauch, H. G. L. (2013). The effect of a single episode of short duration heart rate variability biofeedback on measures of anxiety and relaxation states. Int. J. Stress Manag. 20, 391–411. doi:10.1037/a0034777
Prinsloo, G. E., Rauch, H. G. L., Lambert, M. I., Muench, F., Noakes, T. D., and Derman, W. E. (2011). The effect of short duration heart rate variability (HRV) biofeedback on cognitive performance during laboratory induced cognitive stress. Appl. Cogn. Psychol. 25, 792–801. doi:10.1002/acp.1750
Riva, G., Mantovani, F., Capideville, C. S., Preziosa, A., Morganti, F., Villani, D., et al. (2007). Affective interactions using virtual reality: the link between presence and emotions. Cyberpsychol. Behav. 10, 45–56. doi:10.1089/cpb.2006.9993
Rockstroh, C., Blum, J., and Göritz, A. S. (2019). Virtual reality in the application of heart rate variability biofeedback. Int. J. Hum.-Comput. Stud. 130, 209–220. doi:10.1016/j.ijhcs.2019.06.011
Rockstroh, C., Blum, J., and Göritz, A. S. (2020). Combining VR and biofeedback. J. Media Psychol. 32, 176–186. doi:10.1027/1864-1105/a000270
Rouder, J. N., Speckman, P. L., Sun, D., Morey, R. D., and Iverson, G. (2009). Bayesian t tests for accepting and rejecting the null hypothesis. Psychon. Bull. Rev. 16, 225–237. doi:10.3758/PBR.16.2.225
Sackeim, H. A., Keilp, J. G., Rush, A. J., George, M. S., Marangell, L. B., Dormer, J. S., et al. (2001a). The effects of vagus nerve stimulation on cognitive performance in patients with treatment-resistant depression. Neuropsychiatry. Neuropsychol. Behav. Neurol. 14, 53–62.
Sackeim, H. A., Rush, A. J., George, M. S., Marangell, L. B., Husain, M. M., Nahas, Z., et al. (2001b). Vagus nerve stimulation (VNSTM) for treatment-resistant depression: efficacy, side effects, and predictors of outcome. Neuropsychopharmacology 25, 713–728. doi:10.1016/S0893-133X(01)00271-8
Schubert, T., Friedmann, F., and Regenbrecht, H. (2001). The experience of presence: factor analytic insights. Presence Teleoperators Virtual Environ. 10, 266–281. doi:10.1162/105474601300343603
Soyka, F., Leyrer, M., Smallwood, J., Ferguson, C., Riecke, B. E., and Mohler, B. J. (2016). “Enhancing stress management techniques using virtual reality,” in Proceedings of the ACM symposium on applied perception, SAP ’16 (New York, NY, USA: Association for Computing Machinery), 85–88. doi:10.1145/2931002.2931017
Spano, G., Theodorou, A., Reese, G., Carrus, G., Sanesi, G., and Panno, A. (2023). Virtual nature, psychological and psychophysiological outcomes: a systematic review. J. Environ. Psychol. 89, 102044. doi:10.1016/j.jenvp.2023.102044
Sutarto, A. P., Wahab, M. N. A., and Zin, N. M. (2013). Effect of biofeedback training on operator’s cognitive performance. Work 44, 231–243. doi:10.3233/WOR-121499
Tabor, A., Bateman, S., Scheme, E. J., and schraefel, m. c. (2022). Comparing heart rate variability biofeedback and simple paced breathing to inform the design of guided breathing technologies. Front. Comput. Sci. 4. doi:10.3389/fcomp.2022.926649
Thayer, J. F., and Lane, R. D. (2009). Claude Bernard and the heart–brain connection: further elaboration of a model of neurovisceral integration. Neurosci. Biobehav. Rev., Inevitable Link between Heart Behav. New Insights Biomed. Res. Implic. Clin. Pract. 33, 81–88. doi:10.1016/j.neubiorev.2008.08.004
Tinga, A. M., Nyklíček, I., Jansen, M. P., de Back, T. T., and Louwerse, M. M. (2019). Respiratory biofeedback does not facilitate lowering arousal in meditation through virtual reality. Appl. Psychophysiol. Biofeedback 44, 51–59. doi:10.1007/s10484-018-9421-5
Vanderhasselt, M.-A., and Ottaviani, C. (2022). Combining top-down and bottom-up interventions targeting the vagus nerve to increase resilience. Neurosci. Biobehav. Rev. 132, 725–729. doi:10.1016/j.neubiorev.2021.11.018
Vaschillo, E., Lehrer, P., Rishe, N., and Konstantinov, M. (2002). Heart rate variability biofeedback as a method for assessing baroreflex function: a preliminary study of resonance in the cardiovascular system. Appl. Psychophysiol. Biofeedback 27, 1–27. doi:10.1023/a:1014587304314
Vaschillo, E. G., Vaschillo, B., and Lehrer, P. M. (2006). Characteristics of resonance in heart rate variability stimulated by biofeedback. Appl. Psychophysiol. Biofeedback 31, 129–142. doi:10.1007/s10484-006-9009-3
Wang, X., Shi, Y., Zhang, B., and Chiang, Y. (2019). The influence of forest resting environments on stress using virtual reality. Int. J. Environ. Res. Public. Health 16, 3263. doi:10.3390/ijerph16183263
Weibel, R. P., Kerr, J. I., Naegelin, M., Ferrario, A., Schinazi, V. R., La Marca, R., et al. (2023). Virtual reality-supported biofeedback for stress management: beneficial effects on heart rate variability and user experience. Comput. Hum. Behav. 141, 107607. doi:10.1016/j.chb.2022.107607
White, M. P., Yeo, N. L., Vassiljev, P., Lundstedt, R., Wallergård, M., Albin, M., et al. (2018). A prescription for “nature&rdquo; – the potential of using virtual nature in therapeutics. Neuropsychiatr. Dis. Treat. 14, 3001–3013. doi:10.2147/NDT.S179038
Keywords: cardiac coherence, virtual reality, anxiety, stress, biofeedback HRV
Citation: Pratviel Y, Bouny P and Deschodt-Arsac V (2024) Immersion in a relaxing virtual reality environment is associated with similar effects on stress and anxiety as heart rate variability biofeedback. Front. Virtual Real. 5:1358981. doi: 10.3389/frvir.2024.1358981
Received: 20 December 2023; Accepted: 20 March 2024;
Published: 04 April 2024.
Edited by:
Lorenzo Picinali, Imperial College London, United KingdomReviewed by:
Justin Maximilian Mittelstädt, German Aerospace Center (DLR), GermanyCopyright © 2024 Pratviel, Bouny and Deschodt-Arsac. This is an open-access article distributed under the terms of the Creative Commons Attribution License (CC BY). The use, distribution or reproduction in other forums is permitted, provided the original author(s) and the copyright owner(s) are credited and that the original publication in this journal is cited, in accordance with accepted academic practice. No use, distribution or reproduction is permitted which does not comply with these terms.
*Correspondence: Pierre Bouny, cGllcnJlLmJvdW55QHUtYm9yZGVhdXguZnI=
Disclaimer: All claims expressed in this article are solely those of the authors and do not necessarily represent those of their affiliated organizations, or those of the publisher, the editors and the reviewers. Any product that may be evaluated in this article or claim that may be made by its manufacturer is not guaranteed or endorsed by the publisher.
Research integrity at Frontiers
Learn more about the work of our research integrity team to safeguard the quality of each article we publish.