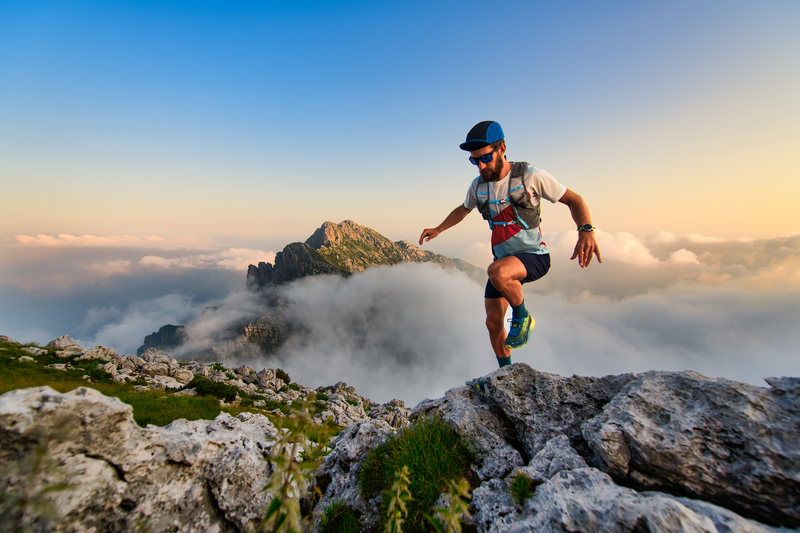
94% of researchers rate our articles as excellent or good
Learn more about the work of our research integrity team to safeguard the quality of each article we publish.
Find out more
ORIGINAL RESEARCH article
Front. Virtual Real. , 14 March 2024
Sec. Virtual Reality and Human Behaviour
Volume 5 - 2024 | https://doi.org/10.3389/frvir.2024.1343872
This study investigates the effects of immersion on spatial ability in virtual reality (VR) and the influence of sense of presence, simulation sickness, and cognitive load. Participants performed a spatial ability task using a head-mounted display in an immersive or non-immersive VR condition. Contrary to expectations, immersive VR did not enhance spatial performance compared to non-immersive VR. Interestingly, cognitive load was positively associated with spatial ability in immersive VR but negatively associated with it in non-immersive VR. A higher sense of presence was associated with increased spatial ability for both conditions. Predictably, simulation sickness negatively impacted spatial ability in the more immersive condition. Sense of presence and simulation sickness correlated in the immersive condition but not in the non-immersive condition, indicating the importance of immersion as a covariate. These findings highlight a complex interplay of factors in immersive environments, challenging existing assumptions and providing insights for designing spatial environments in immersive and non-immersive virtual environments.
The field of spatial computing, and specifically Virtual Reality (VR), has been extensively researched for its novel approach to learning and performance enhancement (Huang et al., 2020). Traditionally, VR has been identified as computer-based environments that can simulate real or imagined places presented on a screen (Freina and Ott, 2015). However, modern head-mounted displays (HMDs) are capable of delivering immersive virtual reality (IVR) that breaches the constraints of the two-dimensional perspective, giving individuals an immersive and embodied perceptual experience (Garcia-Betances et al., 2015). Researchers have suggested that the spatial ability of IVR technology, including visual, auditory, and haptic components, enables users to perceive the virtual world as if it were real, allowing for improved performance, training, and understanding of the virtual environment (VE, Bystrom, et al., 1999; Molina-Carmona et al., 2018).
Several studies support the idea that IVR offers advantages compared to traditional non-immersive VR (e.g., Kim et al., 2001; Kopciak et al., 2016; Webster, 2016). Researchers have argued that providing an immersive VE allows participants to better understand spatial relationships and distances, create clear and detailed mental maps, and develop spatial skills (Bower et al., 2014; Huang et al., 2019). IVR has become a popular tool for researchers aiming to simulate the real world to understand human spatial learning and processes, such as surgery, physics, or engineering (Aggarwal et al., 2006; Taube et al., 2013; Merchant et al., 2014).
However, recent studies by [Makransky et al. (2019), Makransky and Petersen, 2021] challenge the notion that IVR provides better learning experiences of spatial concepts. Comparing different levels of immersion on learning efficacy, they demonstrated reduced learning in the immersive HMD-mediated condition compared to the non-immersive condition (Makransky et al., 2019). The authors argue that the reduction in learning is attributed to immersive VR’s added extraneous Cognitive Load (CL) (see Meyer et al., 2019). CL is generally defined as the experienced task demand compared to the individual’s mental capacity (Chandler and Sweller, 1991; Mayer, 2014). This definition suggests that auditory and visual working memory aspects are limited, so only a few items can be stored at any time (Makransky and Petersen, 2021). Overloading user’s mental capacity has been found to detract from the learning experience, and allocating mental resources towards non-primary tasks has been linked to lesser performance (Navon and Gopher, 1979).
Another restriction of IVR is the prevalence of Simulation Sickness (SS), a subset of motion sickness experienced during or after IVR, that can lead to dizziness, vertigo, and nausea (Kennedy et al., 1993). Some scholars have used “cybersickness” to refer to similar symptoms, but the terms are generally used interchangeably (Grassini et al., 2021). SS is quite commonly experienced during IVR training, with some studies finding that up to 80% of users experience some discomfort (Knight and Arns, 2006). Previous research has reported SS symptoms to be significantly lower or even non-existent in non-immersive VR compared to using an HMD (see Agić and Mandić, 2021). Several studies have found symptoms of SS to decrease learning, training transferability, and general performance in a VE (Mittelstaedt et al., 2019; Thorp, 2021; Agić and Mandić, 2021; Thorp et al., 2023). Adverse SS symptoms can even negatively impact users’ ability to complete VR training, with Brooks (2010) reporting that 17% quit a driving simulation task before completion.
Another aspect of IVR is the experience of a location-based and multisensory experience, making immersive VR an embodied experience (Matamala-Gomez et al., 2019). In this manner, modern IVR provides a new paradigm compared to non-immersive VR, in which individuals are not external observers but actively a part of the VE (Riva et al., 2007). This unique quality of the technology can provide a subjective sense of feeling utterly involved in the VE, often described as the “sense of presence” (SoP; Cummings and Bailenson, 2016). SoP is not to be confused with the device’s objective technical attributes, as the technology’s quantifiable factors are called “immersion” (Slater, 2003). In contrast to SS and CL, a high SoP has generally been found to enhance performance in IVR (e.g., Stevens and Kincaid, 2015; Grassini et al., 2020; Thorp et al., 2023). However, some conflicting evidence has been found for the role of presence in promoting performance, i.e., the aforementioned study Makransky et al. (2019) found that increased immersion promotes presence but hinders trainees’ performance.
The promise of IVR as a uniquely effective and embodied experience for learning spatial concepts is contingent upon the idea that exploring, moving, and learning spatial aspects in a virtual setting will stand up to or surpass similar experiences in non-immersive VR. However, contradictory findings have created a notable gap in the literature concerning the efficacy of IVR over non-immersive VR for several reasons.
First, though the subject of immersive versus non-immersive VR performance is frequently studied, there is a noticeable absence of universally agreed-upon definitions regarding “spatial ability.” The term “spatial ability” encompasses a broad range of tasks, including but not limited to spatial memory, spatial orientation, spatial visualization, and spatial perception (Hegarty and Waller, 2005; Uttal et al., 2013). The generalization of “spatial” means that it is not well understood when or in which conditions IVR can enhance spatial learning outcomes.
Second, complication arises from the disparate immersive technologies researchers employ to present VEs, especially in research on human spatial processing (Taube et al., 2013). Often, findings are compared and presented without accounting for the mediating impact of immersion levels on spatial performance. This oversight is particularly concerning, given that highly immersive devices provided by modern HMDs offer a quality fundamentally distinct from less immersive VR (Grassini et al., 2021).
Third, the unique experiences induced by IVR in SS, CL, and SoP present opportunities and challenges. A substantial body of research has studied these factors and their relationship. However, the existing findings typically conclude either a positive or a negative association irresectable of the contents, design, or immersion of the VE (see, e.g., Weech et al., 2019). Little research has examined how differences in immersive modularity influence the impact of these factors on spatial ability, as well as how immersion affects the interaction of these variables.
To address the variability of defining “spatial ability,” the current study created a design that aims to measure spatial ability purely by its definition, i.e., “the ability to comprehend and conceptualize visual representations and spatial relationships” (Pellegrino et al., 1984; APA Dictionary of Psychology, 2018). The task encompassed participants exploring a VE in either non-immersive or immersive VR and were subsequently instructed to replicate the arrangement of objects from a top-down perspective. Spatial ability was calculated based on the correctness of participants’ recall. Additionally, the current study aims to explore how variables of SoP, SS, and CL impact individuals’ spatial ability in non-immersive and immersive VR and how these variables interplay in the different levels of immersion. Ultimately, we contend that this research holds significant implications for both the application of immersive VR in educational settings and the methodology employed by researchers utilizing varying levels of immersive VEs.
Specifically, the current study explores the following questions:
(1) How does immersion affect spatial ability performance?
(2) How does immersion influence the interplay between SS, SoP, and CL?
(3) How do CL, SoP, and SS interplay in the IVR and non-immersive conditions?
Given the unique aspect of IVR of facilitating a spatial, interactable, and immersive representation of the environment, scholars have posited that individuals may acquire spatial knowledge and skills more effectively when presented in IVR conditions compared to non-immersive conditions (Rose et al., 2000; Winn et al., 2002).
Several studies have found that immersive VR provides comparatively better learning opportunities when learning about spatial concepts such as surgery, physics, or engineering (Aggarwal et al., 2006; Molina-Carmona et al., 2018; Merchant et al., 2014). Studies have also found IVR to provide spatial orientation acquisition comparable to training in the real world (Kuliga et al., 2015; Pastel et al., 2022) and that neural activation during an immersive VE to more closely relate to real life compared to 2D screens (Garcia-Betances et al., 2015).
However, despite decades of research finding immersion effective for spatial ability learning (see Jerald, 2015), widespread adoption of IVR technology for training practices has not been achieved (Steffen et al., 2019; Ortega-Rodriguez, 2021). The limited adoption of IVR might be ascribed to substantial side effects. Makransky et al. (2019) argues that though VR has unique spatial properties that can facilitate training, VR can also cause extraneous cognitive load—detracting from the learning experience. Moreover, SS has significant negative implications for training in IVR, with findings suggesting SS to decrease performance (Mittelstaedt et al., 2019), cognitive abilities (Yörük Açıkel et al., 2018) and training effectiveness in IVR (Geyer and Biggs, 2018).
Studies show immersive factors such as higher display frame rates, less latency, and a higher field of view to increase SoP (Prothero, 1995; Meehan et al., 2002; 2003). Indeed, except for a few cases (e.g., Baños et al., 2008), research indicates that IVR technologies increase SoP compared to non-immersive VR (Rosa et al., 2016; MacQuarrie and Steed, 2017; Servotte et al., 2020; Lønne et al., 2023). However, the inducement of SoP does not need to comprise IVR technology. In particular, video games, movies, and even reading books have been found to elicit a high SoP (Nunez, 2004; Baños et al., 2005; Pallavicini et al., 2019). In fact, some researchers argue that there is not a direct relationship between immersion and SoP, as other factors, such as media contents and characteristics, influence the feeling of “being there” (Baños et al., 2005).
Simulation Sickness is most widely accepted to be caused by a mismatch between the perceived sensory stimulation and the vestibular system’s detection of motion and visual cues (Reason, 1978; Hancock et al., 2008). As such, in IVR, where users are provided with lifelike sensory inputs that suggest movement (e.g., visual and sometimes auditory cues), the absence of corresponding physical motion can cause the brain to receive conflicting signals, leading to the symptoms of SS. Studies investigating the impact of immersion generally find that higher levels of immersion lead to more SS sympathology. E.g., Martirosov et al. (2022) compared low-immersive (PC screen), semi-immersive (CAVE system), and high-immersive (Oculus Rift HMD), finding a significant increase in negative symptoms with increasing levels of immersion. Similar tendencies have been found in studies using virtual tours (Polcar and Horejsi, 2015) and navigation tasks (Kwok et al., 2018). Generally, SS symptoms have been reported to lesser degrees in low-immersive conditions such as watching movies or playing games on a computer screen (Dillon and Emurian, 1996; Guna et al., 2020; Chandra et al., 2022).
However, some evidence exists for a more nuanced relationship between immersion and SS. Following the logic of the sensory mismatch theory, immersive elements that decrease the sensory mismatch between the real and virtual worlds should decrease SS symptoms. I.e., an HMD with perfect simulation of all senses, including vision, motion, touch, and sound, would be perceivably indistinguishable from the real world, removing the possibility for sensory mismatch. Indeed, newer experiences and studies using the latest HMD technology, like the Apple Vision Pro (Apple, 2024), indicate that higher frame rates and lower motion-to-photon latency decrease the prevalence of negative symptoms (Wang et al., 2023).
The relationship between immersion and CL is not universally similar across studies. In the context of IVR, most studies find highly immersive environments to increase CL (Rose et al., 2000; Han et al., 2021). However, contradicting evidence exists, with a recent meta-review finding that 7 out of 46 studies found that CL did not increase in more immersive environments (Han et al., 2021). These studies suggest that high-immersive environments can, under certain conditions, facilitate learning by enhancing realism, interest, and motivation, thereby potentially reducing cognitive load (Johnson-Glenberg et al., 2014; Frederiksen et al., 2020).
Regarding the impact of CL on training outcomes, the study by Weech et al. (2019) found a direct correlation between increased levels of immersion, elevated CL, and worse training outcomes. The authors argue that the increased CL can be attributed to the necessity for learners to navigate and interpret sophisticated virtual spaces, which, while engaging, may saturate their cognitive capacity, leading to decreased efficiency in information processing and learning outcomes. However, immersion has been an advantage in several interventions, like healthcare (Rose et al., 2018), pain treatment (Theingi et al., 2022), and in several educational settings (See Freina and Ott, 2015). Seemingly, the directional impact between immersion, CL, and training outcomes depends on the contents of the simulation. E.g., Baceviciute et al., 2021 found that when the text was displayed in an IVR, it was difficult to read and led to high CL and worse training outcomes. Meanwhile, other studies finding the high CL provided by immersion to be beneficial argue that IVR technology is beneficial because the environment increases users sense of presence (Parong et al., 2020), was closer to real life (Lok et al., 2003), and increased engagement (Huang et al., 2021).
The interplay between CL, SoP, and SS is intricate, and immersive technologies have been shown to affect all three extraneous variables significantly (Cummings and Bailenson, 2016; Breves and Stein, 2023). On one side, you have SoP, which is generally found to enhance learning and performance in a VE (Stevens and Kincaid, 2015; Grassini et al., 2020). Conversely, SS can pull individuals out of the experience, hindering performance (Kennedy et al., 1993). Then, CL, the mental effort required to navigate the VE, impacts SS, SoP, and performance.
The relationship between SoP and SS has been a subject of considerable debate in academic literature. Numerous studies have attempted to quantify the relationship, yielding a spectrum of results from positive to negative associations. For instance, Liou et al. (2017), found a strong positive correlation (r = 0.67) in a virtual navigation experiment. Conversely, a meta-review by Weech et al. (2019) posits a negative correlation between the two constructs, arguing that positive associations are explainable by the covariate of immersion. Thorp et al. (2022) found that the development of SoP and SS follow a similar temporal development, positively correlating throughout the experiment. The authors propose that covariates such as immersion, excitement, engagement, or CL similarly impact SS and SoP, explaining the positive relationship.
The interplay between CL and SS in IVR has not been conclusively explained. Some findings have suggested a negative association, arguing that higher concentration and CL render resources away from negative symptoms, leading to less SS (Bos, 2015; Venkatakrishnan et al., 2020). Relatedly, studies have reported a negligible impact of CL on sickness (Milleville-Pennel and Charron, 2015). More recently, studies have generally argued for a positive association. Using a Structural Equation Modeling approach, Venkatakrishnan et al. (2020) found that increased control over self-motion stimuli in a driver simulation increased cognitive load, increasing SS. Similar tendencies of a positive association have been found by Ha (2020), Meusel (2014), Venkatakrishnan et al. (2019), and Venkatakrishnan et al. (2023). Evidence also exists for a more nuanced relationship between the relevant variables. Breves and Stein (2023) examined CL in an immersive media setting, focusing on the roles of spatial presence and SS. They found that the IVR conditions led to higher CL, spatial presence, and SS compared to the 2D conditions, arguing that the relationship between CL and SS differs based on levels of immersion. Further evidence for a non-linear relationship between SS and CL mediated by immersion was found by Breves and Stein (2023), finding CL to associate with higher SS (and SoP) in HMD-mediated VR but not in the 2D condition. Relatedly, Venkatakrishnan (2023) found CL from secondary distractions to be beneficial for reducing SS, underscoring a more non-direct relationship between CL and SS. Sepich et al. (2022) suggest that dependent on the VE and task, CL is a significant, but not sole, contributor to SS.
The relation between SoP and CL is generally found to be negative. In accordance with the Cognitive Load Theory (Chandler and Sweller, 1991) and Cognitive Theory of Multimedia Learning (Mayer, 2014), Parong et al. (2020) argue that feeling present alleviates extraneous CL caused by the HMD, allowing the learner to allocate attention towards learning and processing the spatial environment. The authors further argue that this effect is especially prevalent in spatial navigation tasks, where navigating VE on a monitor may require more cognitive resources to translate one’s position into the environment than in an environment where the user feels they are in the environment (feeling present). Makransky and Petersen (2021) found that participants engaged in HMDs reported high levels of SoP but demonstrated reduced learning efficacy. This limitation in learning was attributed to the added extraneous CL, which arised from the perceptual realism that was not directly relevant to the instructional objectives. The authors argue that immersive elements may serve as “seductive details” and that being engrossed in a VE diverts cognitive resources from learning.
Sixty-four participants took part in the study. Two participants from the IVR group were excluded because of missing data during the experiment. The remaining sample comprised 36 men and 26 women aged 20 to 29 (M = 23.17, SD = 1.94). The sample size was established based on similar studies (Ha, 2020; Evensmoen et al., 2021; Breves and Stein, 2023). Participants were mainly recruited from the student population at the Norwegian University of Science and Technology (Trondheim, Norway). Before the experiment, participants received a description of the study, gave written consent, and were screened for a history of epileptic seizures and having normal or corrected to normal vision.
The VE was created using the video game Minecraft (Mojang, 2011). The environment consisted of ten different 49 squares arranged in a 7 × 7 pattern. Each square consisted of 9 light brown blocks arranged in a three-by-three pattern and were separated by a 1-block line of darker brown blocks. In the south-western corner of each VE, there was a door to act as a reference point. Six objects were depicted: a car, a train, a storage unit, a cabinet, a cloud, and a motorbike, were placed in the center of randomly selected squares (see Figure 1). The objects’ positions and rotations (in 90° intervals) were unique to each of the ten environments. Each environment was completed in a fixed order by the participants.
FIGURE 1. Image of the virtual environment. Note. The image depicts the VE. Object placement was unique for each of the ten conditions.
Participants were evenly assigned to two groups. One group performed the tasks using an HMD (IVR condition), and the other using a computer monitor (low-immersive VR). Before the experiment started, participants in both the IVR condition and the low-immersive condition were given instructions for the task. They were familiarised with the VE and controllers by moving and looking around in a training room with the guidance of the experimenter.
The experimental task was similar for both conditions: a spatial ability processing task involving exploring the 7 × 7 checkerboard environment (see Figure 3). Participants were given 1 min of free exploration and the task of remembering the placement of the six objects. Once the exploration ended, participants fixated on a cross, followed by an odd-even judgment task. The fixation cross was used to equalize the process of memory consolidation across participants. In contrast, the odd-even judgment task was used to disrupt participants’ working memory, making it challenging to employ subvocal rehearsal methods of remembering objects (see Evensmoen et al., 2021). Lastly, participants’ recall was tested by replicating the position and rotation of the objects in a physical top-down representation of the VE. For this task, participants had to remove the HMD temporarily. This procedure was then repeated ten times with different object placements in the VE. Each round lasted approximately 4 min for a total experimental length of about 40 min. The experiment design is depicted in Figure 2.
FIGURE 3. Calculation of spatial ability. Note. The first figure represents the overlay of correct positions and participants’ positional remembrance. The following three figures represent the three-step calculation of participants’ spatial ability of translation, rotation, and scaling. The last figure shows the measurement of spatial ability for an experimental procedure, calculated as the average square mean error between objects relative to positional remembrance and correct positions. The final spatial ability score per participant was calculated as the average from the ten experimental procedures.
After the experiment ended, participants were asked to fill in the Presence Questionnaire (Witmer & Singer, 1998), the Simulation Sickness Questionnaire (Kennedy et al., 1993), and the NASA task load index (Hart and Staveland, 1988).
Participants in the IVR condition viewed the VE through a Meta Quest 2 headset with corresponding controllers. In the non-immersive VR condition, the screen was a 24-inch 1080P monitor with a 60 Hz refresh rate, and participants used an Xbox Elite Series v1 controller. In both conditions, the right stick controlled the player’s line of sight, while the left stick controlled their movement. Participants in the IVR conditions could also look around in the environment by moving their heads. The right stick’s sensitivity was set similarly in both conditions, and the player moved at the speed of 4.317 virtual m/s. Participants remained seated in both conditions throughout the experiment.
To assess spatial ability, a pattern of participants’ object placement was first compared to the correct positional pattern following the recommendations of Evensmoen et al. (2021). In the first step, a calculation was done by translating participants’ recalled patterns such that the geometric center matches the geometric center of the correct pattern. In the second step, the recalled pattern was rotated into alignment with the correct positional pattern by minimizing the root mean square deviation discrepancy between the recalled and correct pattern (Kabsch, 1988; Umeyama, 1993). This transformation aimed to separate the positional pattern from its connection to the environment’s boundaries, thereby emphasizing positional recall. In the third step, a scaling algorithm was applied to account for humans’ tendency to compress or expand spatial representations (Tversky, 1992). Participants’ final recall accuracy score was calculated by averaging the square mean error for each of the three steps mentioned above (translation, rotation, and scaling) for each of the ten VEs. Only the placement of objects, independent of object identity and rotation, was used for this calculation.
Before the experiment, participants were given a questionnaire including measures of prior experience with IVR and video games, as well as their vision, history of epileptic seizures, sex, and age.
After the experiment, the NASA task load index (NASA-TLX) was used to assess the degree of CL experienced by the participants (Hart and Staveland, 1988). The instrument consisted of six items: mental demands, physical demands, temporal demands, task complexity situational stress, and distractions, measured using 21-point scales ranging from “very low” to “very high.” The total score of the NASA-TLX was calculated using the average of all items.
To measure participants’ SoP, the Presence Questionnaire, developed by Witmer & Singer (1998) and revisited by the UQO Cyberpsychology lab (2013), was used. The questionnaire was chosen as it is the most used in IVR research (Hein et al., 2018). As sound was absent in the VE, questions about sound were removed. The Presence Questionnaire used a seven-point Likert scale and was scored by averaging the score of the individual items.
The Simulation Sickness Questionnaire was used to assess the degree of SS experienced by the participants after the experiment (Kennedy et al., 1993). The questionnaire is the most used measure of SS in research on spatial computing (Bimberg et al., 2020). The questionnaire consists of 16 symptoms, rated in the severity of each symptom on a four-point scale (no SS, slight SS, moderate SS, and severe SS). The final score is obtained using the calculation weights of Kennedy et al. (1993).
IBM SPSS (version 29) and the PROCESS macro (v.4.2; Hayes et al., 2017) were used for statistical analyses. Three items were missing and imputed using the mean function in SPSS. This function imputes an average score based on the remaining data points of the variable. Two-tailed significance levels were used for all analyses. Multiple linear regression with immersion as a dummy variable was performed to examine how performance differs between levels of immersion. Multiple regression analyses were conducted to examine the association of CL, SoP, and SS on performance. Lastly, Spearman’s rank-order correlations were performed to explore the association of the extraneous variables in the different conditions.
On average, participants in the non-immersive VR condition (N = 30, 18 males, 12 females) were 23.57 years old (SD = 2.30). The SoP score (M = 4.99) was notably higher for the non-immersive condition than in similar studies (Cummings and Bailenson, 2016; Makransky et al., 2019; Breves and Stein, 2023). Reported SS scores (M = 30.29) were also somewhat higher than other studies reporting SS using computer monitors, even being comparable to the lower ranges of studies using HMDs (e.g., Kim et al., 2018, which reported scores of 28.67). CL scores (M = 8.31) were similar for both the IVR and non-immersive VR conditions. Average scores were in line with similar studies (e.g., Breves and Stein, 2023).
The participants in the IVR condition (N = 32, 18 males, 14 females) were 22.91 years on average (SD = 1.57). The SoP score (5.23) for the IVR condition was generally in line with similar studies (Weech et al., 2019; Thorp et al., 2023), and the SS was generally in line with most VR studies (e.g., Somrak et al., 2019, which reports scores ranging from 45.95 to 56.41 for different HMD models). CL scores (M = 8.29) were similar to the 2D condition (M = 8.31), contradicting previous studies finding CL to be higher in IVR (Makransky et al., 2019; Breves and Stein, 2023). Descriptives of the study variables are presented in Table 1.
A multiple linear regression analysis was performed to examine whether individuals in the non-immersive condition (M = 2.63, SD = 1.74) performed better on the spatial ability task than those in the immersive condition (M = 2.06, SD = 1.33). Prior to conducting the analyses, assumptions were checked. Tolerance and VIF values did not indicate a violation of multicollinearity. A Durbin-Wattson statistic (1.72) was between the suggested threshold of 1–3, indicating that the values of the residuals are independent. The assumption of homoscedasticity was met by checking the variance of residuals in a scatterplot, and the assumption of normally distributed residuals was met by examining a P-P Plot. Cook’s Distance values were calculated to ensure no influential cases biasing the model. All values were below 1, suggesting no cases were biasing the model.
For the multiple regression, the results showed a non-significant equation F (1.60) = 2.06, p = .157, and accounted for a small proportion of the variance in spatial ability (R2 = .033). The results indicated that the performance of individuals in the non-immersive condition (β = .57, SE = .40) was, on average, 0.56 units higher compared to the immersive condition, but this effect was not significant. To explore the likelihood of a difference in spatial ability between the conditions, as recommended by Gönen et al. (2005), a Bayesian independent sample t-test was conducted. The analyses show some evidence favoring a difference (BF = 2.07), indicating that the difference between the conditions was 2.07 times more likely than the null hypothesis of no difference between the conditions.
To examine how CL, SoP, and SS impact spatial ability in different levels of immersion, we first performed separate multiple linear regressions for the two conditions.
The regression model for the non-immersive VR condition was statistically significant, F (3.26) = 7.35, p < .001, with R2 = .459, suggesting that the extraneous variables predict 45.9% of the variance in spatial ability. The coefficients reveal that SoP (β = .55, p < .001) had a large and significant impact on spatial ability. Contrastingly, CL (β = −.37, p = .025) significantly negatively impacted spatial ability. SS (β = .28, p = .084) showed a positive relationship to spatial ability but was insignificant.
The regression model for the IVR condition was also statistically significant, F (3.26) = 12.49, p = .002, with R2 = .40, suggesting that the extraneous variables predict 40% of the variance in spatial ability. The coefficients for the independent variables show that SoP, similarly to the non-immersive condition, positively impacts spatial ability (β = .35, p < .048). In contrast to the non-immersive condition, CL (β = .47, p = .013) positively impacted spatial ability. As predicted, SS (β = −.52, p = .011) negatively impacted spatial ability.
Moderation analyses were conducted to investigate the role of immersion (IVR vs. non-immersive VR) as a moderator on the relationship between the extraneous variables and spatial ability to examine further how the extraneous variables impact spatial ability differently between conditions. Moderation analysis was conducted using SPSS’s PROCESS macro (Hayes, 2012). The independent variables were centered and standardized to avoid potentially high multicollinearity with the interaction term. An interaction term between the independent variable and spatial ability was created (Aiken et al., 1991).
The results indicate a significant interaction term between CL and spatial ability [β = .36, SE = .17 t (58) = 2.32, p = .031] with an ΔR2 = .07, showing that higher CL significantly negatively affected spatial ability in the non-immersive condition, but positively in the IVR condition.
The interaction term between SoP and spatial ability showed a marginally non-significant relationship [β = −27, SE = .15, t (58) = −1.85, p = .068] with ΔR2 = .04. The interaction plot showed that individuals with a higher SoP perform better in the spatial ability task in both the 2D and VR condition.
The interaction term between SS and spatial ability was also marginally non-significant [β = −.40, SE = .20, t (58) = −1.95, p = .056] with an ΔR2 = .04. The interaction plot showed that individuals in the VR condition with lower SS scores perform better in the spatial ability task. Additionally, the marginally non-significant p-value and interaction plot might indicate that SS negatively impacted performance in IVR but not in non-immersive VR.
Before conducting the correlation analyses, assumptions were checked. Shapiro-Wilk test suggested that SoP, W (62) = .95, p = .01, and SS, W (62) = .92, p < .01, were not normally distributed. Consequently, Spearman’s rank-order correlation analysis was run to examine the associations between the extraneous variables for IVR and non-immersive VR conditions (see Table 2). For the non-immersive condition, no significant correlations were found. For the IVR condition, a significant negative association was found between CL and SS (rs = −.51, p < .01), and between SoP and SS (rs = −.43, p < .05).
TABLE 2. Correlation table for the extraneous variables in the non-immersive and immersive VR conditions.
The present investigation aimed to explore how immersion impacts individuals’ spatial ability, how immersion influences the interplay between SoP, SS, and CL, and spatial ability, and how these factors interplay in different levels of immersion. The current study design aimed to assess spatial ability in a standardized manner. The spatial task involved a memory task of object placements in a virtual environment consisting of a 7 × 7 grid. The current study’s findings pose critical questions for the experience and performance of spatial ability in HMD-moderated IVR.
We found no significant difference in immersion on spatial ability between the IVR condition (M = 2.06, SD = 1.33) and the non-immersive condition (M = 2.63, SD = 1.74). Given assumptions from prior research that IVR’s immersive spatial representations will lead to better spatial performance, it was somewhat surprising to find no significant difference. This finding somewhat contradicts prior research postulating that the increased immersive elements enable users to perceive the virtual world as if it were real, in turn increasing understanding of spatial concepts, spatial maps, navigation, and development of spatial skills (Taube et al., 2013; Bower et al., 2014; Molina-Carmona et al., 2018; Huang et al., 2019; Hill et al., 2023).
A few potential reasons exist why individuals did not perform better in the IVR condition. For one, it is possible that the individual characteristics of CL and SS had a negative impact, which may have canceled out any spatial advantages of the immersive IVR. Moreover, participants may not have been as familiar with using HMDs, which could have led to comparatively worse outcomes than with the more familiar gaming controller and screen configuration. Another explanation could be that the specific experimental task, which involved placing objects in the environment and in relation to each other, is easier to complete in a non-immersive environment, allowing for a better overview of the environment and object relations. Indeed, some studies have found that navigational remembrance tasks are performed better in non-immersive environments compared to IVRs (Santos et al., 2008; Srivastava et al., 2019).
A positive relationship was observed between SoP and spatial ability for both the low-immersive condition (β = .55, M = 4.99) and the IVR condition (β = .35, M = 5.23), indicating that better spatial ability is associated with higher reported SoP for both conditions. This finding challenges the belief that higher SoP positive association with performance is unique to immersive VEs (see Servotte et al., 2020).
Notably, the reported SoP score was only marginally higher in the IVR condition, contrasting previous studies arguing that IVR inherently and uniquely allows for a high SoP (Rosa et al., 2016; MacQuarrie and Steed, 2017). Perhaps the high concentration required for the experimental task explains the markedly high SoP scores in the non-immersive VR condition compared to other studies (Cummings and Bailenson, 2016; Makransky et al., 2019; Pallavicini et al., 2019; Paes et al., 2021; Breves and Stein, 2023).
The analyses showed SS to impact spatial ability significantly negatively in the IVR condition group (β = −.52, p = .011) but not a significant effect in the non-immersive setting (p = .084). This aligns with previous studies finding that SS hinders individuals’ ability to interact, perform, and learn in a VE (see Thorp et al., 2023). Additionally, the result is following several studies that found higher levels of immersion to be associated with higher SS (Polcar and Horejsi, 2015; Kwok et al., 2018; Martirosov et al., 2022).
Interestingly, the study found diverging behavior between CL and spatial ability in the non-immersive and immersive VR conditions. The moderation analyses showed a reverse relationship in that CL negatively predicted spatial ability in the non-immersive condition (β = −.37, p = .025) but positively in the IVR condition (β = .47, p = .013). The follow-up analyses [β = .36, SE = .17 t (58) = 2.32, p = .031] confirmed a strong negative relationship with immersion as moderator (r = −.42).
This result indicates that individuals who performed better at the spatial ability task reported lower CL scores in the non-immersive condition but reported higher scores in the IVR condition. Mean CL scores for the IVR group (M = 8.29) and the non-immersive group (M = 8.31) were similar. This finding is surprising, as the increased immersion and novelty from the IVR are generally found to increase reported CL. Perhaps individuals in the non-immersive condition mainly reported CL based on task difficulty, as the interface of a computer monitor was familiar and not viewed as cognitively challenging. In contrast, perhaps participants in the IVR group reported CL based on the sensory immersion provided by the IVR. If this interpretation is correct, this finding sheds light on the importance of immersion in perceived CL. Additionally, it would highlight the challenge of evaluating subjective measures across different modalities and VEs. However, more research is necessary to elucidate this phenomenon’s underlying mechanisms and reasons.
We explored the interplay of CL, SoP, and SS at the different levels of immersion. The results show significant correlations between all the constructs for the IVR condition but no significant relations in the non-immersive condition, suggesting that the immersive nature of IVR creates a unique dynamic among these factors and acts as a covariate. Interestingly, though not significant, all correlations of the IVR condition showed similar positive/negative tendencies.
The correlation between SS and SoP (rs = −.43) was negative in the IVR condition. This follows the meta-review of Weech et al. (2019), which found that most studies report a negative relation, indicating that individuals with higher presence report lower levels of SS. The correlation between SS and CL (rs = .51) was positively significant in the IVR condition, suggesting that the experience of SS increases as CL increases. This finding is generally in line with prior studies that argue that CL is a primary cause of SS and that levels of immersion moderate their relationship (Venkatakrishnan et al., 2020; Sepich et al., 2022; Breves and Stein, 2023). The finding could also imply that tasks requiring higher cognitive resources might exacerbate SS in IVR, thereby negatively impacting performance. However, the lack of significant association in the non-immersive VR setting might support research arguing a more nuanced relation dependent on immersive characteristics (e.g., Breves and Stein, 2023; Sepich et al., 2022; Venkatakrishnan, 2023). Understanding the relationship between SS and CL is a crucial consideration for the design of complex tasks or training simulations in immersive and non-immersive VR environments. No significant correlation was between CL and SoP in either of the conditions. This somewhat contradicts previous studies arguing that the relationship between CL and SoP is stronger in IVR compared to non-immersive VR (Parong et al., 2020; Makransky and Petersen, 2021).
There are some limitations to the current study that should be considered. First, while the current investigation employed contemporary head-mounted display (HMD) technology, research has indicated significant performance disparities when using different HMD equipment (Makransky et al., 2019). Additionally, the heterogeneity in simulation environments across different studies further complicates the direct comparison of outcomes. Thus, the current comparison of immersive conditions might not be generalizable to different simulations or equipment.
Secondly, we may have lacked sufficient statistical power to discover some effects. Several of the analyses were close to reaching significance, and a relatively small sample size of 30 participants per group could have caused this. Additionally, our sample mainly consisted of psychology students in a Norwegian university, reducing our results’ generalizability across samples.
The between-subject design could also have limited the study by not allowing participants to compare their experiences across modalities. Perhaps the participants did not have a sufficient point of reference when answering instruments such as the Presence Questionnaire, and this problem might have been resolved by utilizing a within-subject design. This could be an interesting option for future research. Another consequence of the study design might be that as participants were not as familiar with using HMDs, the unfamiliarity with the technology could lead to worse training outcomes, especially in the first rounds of the experiment.
Additionally, participants may not have been as familiar with using HMDs, which could have led to comparatively worse outcomes than with the more familiar gaming controller and screen configuration.
This study advances our understanding of how immersion impacts spatial ability. Contrary to common assumptions, we found that the immersive qualities of IVR do not inherently enhance spatial ability compared to non-immersive VR. Our findings on the varied influence of CL, SS, and SoP in different immersive settings suggest a complex relationship between these factors and spatial ability. Particularly intriguing is the negative association of CL with spatial ability in IVR, a positive trend in the non-immersive condition. Overall, our study questions assumptions about the influence of CL, SS, and SoP and adds valuable complexity to the discourse on the spatial efficacy of immersive virtual environments.
The raw data supporting the conclusion of this article will be made available by the authors, without undue reservation.
ST: Conceptualization, Formal Analysis, Methodology, Project administration, Supervision, Writing–original draft, Writing–review and editing, Investigation, Visualization, Data curation. LR: Funding acquisition, Resources, Supervision, Writing–review and editing. SL: Data curation, Writing–original draft. HE: Methodology, Writing–review and editing. SG: Methodology, Writing–review and editing.
The author(s) declare that no financial support was received for the research, authorship, and/or publication of this article.
The authors declare that the research was conducted in the absence of any commercial or financial relationships that could be construed as a potential conflict of interest.
The author(s) declared that they were an editorial board member of Frontiers, at the time of submission. This had no impact on the peer review process and the final decision.
All claims expressed in this article are solely those of the authors and do not necessarily represent those of their affiliated organizations, or those of the publisher, the editors and the reviewers. Any product that may be evaluated in this article, or claim that may be made by its manufacturer, is not guaranteed or endorsed by the publisher.
Aggarwal, R., Grantcharov, T. P., Eriksen, J. R., Blirup, D., Kristiansen, V. B., Funch-Jensen, P., et al. (2006). An evidence-based virtual reality training program for novice laparoscopic surgeons. Ann. Surg. 244 (2), 310–314. doi:10.1097/01.sla.0000218094.92650.44
Agić, A., and Mandić, L. (2021). Evaluation of cybersickness in virtual reality in driving simulator. Acta Graph. 30 (2), 11–16. doi:10.25027/agj2017.28.v30i2.210
Aiken, L. S., West, S. G., and Reno, R. R. (1991). Multiple regression: testing and interpreting interactions. Los Angeles, United States: Sage. doi:10.2307/2348581
APA Dictionary of Psychology (2018). APA dictionary of psychology. Available from: https://dictionary.apa.org/ (Accessed February 13, 2024).
Apple (2024). Apple vision Pro. https://www.apple.com/apple-vision-pro/.
Baceviciute, S., Terkildsen, T., and Makransky, G. (2021). Remediating learning from non-immersive to immersive media: using EEG to investigate the effects of environmental embeddedness on reading in Virtual Reality. Comput. Educ. 164, 104122. doi:10.1016/j.compedu.2020.104122
Baños, R., Botella, C., Alcañiz Raya, M., Liaño, V., Guerrero, B., and Rey, B. (2005). Immersion and emotion: their impact on the sense of presence. Cyberpsychology Behav. 7, 734–741. The Impact of the Internet, Multimedia and Virtual Reality on Behavior and Society. doi:10.1089/cpb.2004.7.734
Baños, R. M., Botella, C., Rubió, I., Quero, S., García-Palacios, A., and Alcañiz, M. (2008). Presence and emotions in virtual environments: the influence of stereoscopy. CyberPsychology Behav. 11 (1), 1–8. doi:10.1089/cpb.2007.9936
Bimberg, P., Weissker, T., and Kulik, A. (2020). On the usage of the simulator sickness questionnaire for virtual reality research. IEEE Conference on Virtual Reality and 3D User Interfaces Abstracts and Workshops (VRW), March 2020, Atlanta, GA, USA. IEEE, 464–467. doi:10.1109/VRW50115.2020.00098
Bos, J. E. (2015). Less sickness with more motion and/or mental distraction. J. Vestib. Res. 25 (1), 23–33. doi:10.3233/VES-150541
Bower, M., Howe, C., McCredie, N., Robinson, A., and Grover, D. (2014). Augmented reality in Education—cases, places, and potentials. Educ. Media Int. 51, 1–15. doi:10.1080/09523987.2014.889400
Breves, P., and Stein, J.-P. (2023). Cognitive load in immersive media settings: the role of spatial presence and cybersickness. Virtual Real. 27 (2), 1077–1089. doi:10.1007/s10055-022-00697-5
Brooks, A. (2010). Intelligent decision-support in virtual reality healthcare and rehabilitation. Stud. Comput. Intell. 326, 143–169. doi:10.1007/978-3-642-16095-0_8
Bystrom, K., Barfield, W., and Hendrix, C. (1999). A conceptual model of the sense of presence in virtual environments. Presence Teleoperators Virtual Environ. 8 (2), 241–244. doi:10.1162/105474699566107
Chandler, P., and Sweller, J. (1991). Cognitive load theory and the format of instruction. Cognition Instr. 8 (4), 293–332. doi:10.1207/s1532690xci0804_2
Cummings, J. J., and Bailenson, J. N. (2016). How immersive is enough? A meta-analysis of the effect of immersive technology on user presence. Media Psychol. 19 (2), 272–309. doi:10.1080/15213269.2015.1015740
Dillon, T. W., and Emurian, H. H. (1996). Some factors affecting reports of visual fatigue resulting from use of a VDU. Comput. Hum. Behav. 12 (1), 49–59. doi:10.1016/0747-5632(95)00018-6
Evensmoen, H. R., Rimol, L. M., Winkler, A. M., Betzel, R., Hansen, T. I., Nili, H., et al. (2021). Allocentric representation in the human amygdala and ventral visual stream. Cell Rep. 34 (3), 108658. doi:10.1016/j.celrep.2020.108658
Frederiksen, J. G., Sørensen, S. M. D., Konge, L., Svendsen, M. B. S., Nobel-Jørgensen, M., Bjerrum, F., et al. (2020). Cognitive load and performance in immersive virtual reality versus conventional virtual reality simulation training of laparoscopic surgery: a randomized trial. Surg. Endosc. 34, 1244–1252. doi:10.1007/s00464-019-06887-8
Freina, L., and Ott, M. (2015). A literature review on immersive virtual reality in education: State of the art and perspectives. eLearning Softw. Educ. (eLSE) 1 (133), 10–1007. doi:10.12753/2066-026X-15-020
Garcia-Betances, R. I., Jiménez-Mixco, V., Arredondo, M. T., and Cabrera-Umpiérrez, M. F. (2015). Using virtual reality for cognitive training of the elderly. Am. J. Alzheimer’s Dis. Other Dementias® 30 (1), 49–54. doi:10.1177/1533317514545866
Geyer, D. J., and Biggs, A. T. (2018). The persistent issue of simulator sickness in naval aviation training. Aerosp. Med. Hum. Perform. 89 (4), 396–405. doi:10.3357/AMHP.4906.2018
Gönen, M., Johnson, W. O., Lu, Y., and Westfall, P. H. (2005). The bayesian two-sample t test. Am. Statistician 59 (3), 252–257. doi:10.1198/000313005X55233
Grassini, S., Laumann, K., De Martin Topranin, V., and Thorp, S. (2021). Evaluating the effect of multi-sensory stimulations on simulator sickness and sense of presence during HMD-mediated VR experience. Ergonomics 64 (12), 1532–1542. doi:10.1080/00140139.2021.1941279
Grassini, S., Laumann, K., and Rasmussen Skogstad, M. (2020). The use of virtual reality alone does not promote training performance (but sense of presence does). Front. Psychol. 11, 1743. doi:10.3389/fpsyg.2020.01743
Guna, J., Geršak, G., Humar, I., Krebl, M., Orel, M., Lu, H., et al. (2020). Virtual reality sickness and challenges behind different technology and content settings. Mob. Netw. Appl. 25 (4), 1436–1445. doi:10.1007/s11036-019-01373-w
Ha, P. J. (2020). Correlation between cognitive load, vividness and cyber sickness for 360-degree education video. Int. J. Adv. Cult. Technol. (IJACT) 8 (4), 89–94. doi:10.17703/IJACT.2020.8.4.89
Han, J., Zheng, Q., and Ding, Y. (2021). Lost in virtual reality? Cognitive load in high immersive VR environments. J. Adv. Inf. Technol. 12 (4). doi:10.12720/jait.12.4.302-310
Hancock, P. A., Vincenzi, D. A., Wise, J. A., and Mouloua, M. (2008). Human factors in simulation and training. Cleveland, Ohio: CRC Press.
Hart, S. G., and Staveland, L. E. (1988). “Development of NASA-TLX (task load index): results of empirical and theoretical research,” in Advances in psychology. Editors P. A. Hancock, and N. Meshkati (North-Holland), 52, 139–183. doi:10.1016/S0166-4115(08)62386-9
Hayes, A. F. (2012). A versatile computational tool for observed variable mediation, moderation, and conditional process modeling [White paper]. Retrieved from http://www.afhayes.com/public/process2012.pdf.
Hayes, A. F., Montoya, A. K., and Rockwood, N. J. (2017). The analysis of mechanisms and their contingencies: PROCESS versus structural equation modeling. Australas. Mark. J. 25 (1), 76–81. doi:10.1016/j.ausmj.2017.02.001
Hegarty, M., and Waller, D. A. (2005). “Individual differences in spatial abilities,” in The cambridge handbook of visuospatial thinking (Cambridge University Press), 121–169. doi:10.1017/CBO9780511610448.005
Hein, D., Mai, C., and Hußmann, H. (2018). The usage of presence measurements in research: a review. Proc. Int. Soc. Presence Res., 21–22.
Hill, P. F., Bermudez, S., McAvan, A. S., Garren, J. D., Grilli, M. D., Barnes, C. A., et al. (2023). Age differences in spatial memory are mitigated during naturalistic navigation. bioRxiv: the preprint server for biology. doi:10.1101/2023.01.23.525279
Huang, C. L., Luo, Y. F., Yang, S. C., Lu, C. M., and Chen, A.-S. (2020). Influence of students’ learning style, sense of presence, and cognitive load on learning outcomes in an immersive virtual reality learning environment. J. Educ. Comput. Res. 58 (3), 596–615. doi:10.1177/0735633119867422
Huang, K., Ball, C., Francis, J., Ratan, R., Boumis, J., and Fordham, J. (2019). Augmented versus virtual reality in education: an exploratory study examining science knowledge retention when using augmented reality/virtual reality mobile applications. Cyberpsychology, Behav. Soc. Netw. 22 (2), 105–110. doi:10.1089/cyber.2018.0150
Huang, W., Roscoe, R. D., Johnson-Glenberg, M. C., and Craig, S. D. (2021). Motivation, engagement, and performance across multiple virtual reality sessions and levels of immersion. J. Comput. Assisted Learn. 37 (3), 745–758. doi:10.1111/jcal.12520
Jerald, J. (2015). The VR book: human-centered design for virtual reality. San Rafael, California: Morgan & Claypool.
Johnson-Glenberg, M. C., Birchfield, D. A., Tolentino, L., and Koziupa, T. (2014). Collaborative embodied learning in mixed reality motion-capture environments: two science studies. J. Educ. Psychol. 106 (1), 86–104. doi:10.1037/a0034008
Kabsch, W. (1988). Automatic indexing of rotation diffraction patterns. J. Appl. Crystallogr. 21 (1), 67–72. doi:10.1107/s0021889887009737
Kennedy, R. S., Lane, N. E., Berbaum, K. S., and Lilienthal, M. G. (1993). Simulator sickness questionnaire: an enhanced method for quantifying simulator sickness. Int. J. Aviat. Psychol. 3 (3), 203–220. doi:10.1207/s15327108ijap0303_3
Kim, H. K., Park, J., Choi, Y., and Choe, M. (2018). Virtual reality sickness questionnaire (VRSQ): motion sickness measurement index in a virtual reality environment. Appl. Ergon. 69, 66–73. doi:10.1016/j.apergo.2017.12.016
Kim, J.-H., Park, S.-T., Lee, H., Yuk, K.-C., and Lee, H. (2001). Virtual reality simulations in physics education. Interact. Multimedia Electron. J. Computer-Enhanced Learn. 3 (2), 1–7.
Knight, M. M., and Arns, L. L. (2006). The relationship among age and other factors on incidence of cybersickness in immersive environment users. Proc. 3rd Symposium Appl. Percept. Graph. Vis. 162. doi:10.1145/1140491.1140539
Kopciak, P., Kolar, P., Dollfuss, M., Hellmann, D., and El-Aeraky, M. (2016). “Virtual reality driving simulator prototype for teaching situational awareness in traffic,” in Recent advances in Multimedia processing, organization and visualization beyond domains and disciplines.
Kuliga, S. F., Thrash, T., Dalton, R. C., and Hölscher, C. (2015). Virtual reality as an empirical research tool—exploring user experience in a real building and a corresponding virtual model. Comput. Environ. urban Syst. 54, 363–375. doi:10.1016/j.compenvurbsys.2015.09.006
Kwok, K. K., Ng, A. K., and Lau, H. Y. (2018). “Effect of navigation speed and VR devices on cybersickness,” in 2018 IEEE International Symposium on Mixed and Augmented Reality Adjunct (ISMAR-Adjunct), October 2018 (IEEE), 91–92. doi:10.1109/ISMAR-Adjunct.2018.00041
Liou, H. H., Yang, S. J., Chen, S. Y., and Tarng, W. (2017). The influences of the 2D image-based augmented reality and virtual reality on student learning. J. Educ. Technol. Soc. 20 (3), 110–121.
Lok, B., Naik, S., Whitton, M., and Brooks, F. P. (2003). Effects of handling real objects and self-avatar fidelity on cognitive task performance and sense of presence in virtual environments. Presence 12 (6), 615–628. doi:10.1162/105474603322955914
Lønne, T. F., Karlsen, H. R., Langvik, E., and Saksvik-Lehouillier, I. (2023). The effect of immersion on sense of presence and affect when experiencing an educational scenario in virtual reality: a randomized controlled study. Heliyon 9 (6), e17196. doi:10.1016/j.heliyon.2023.e17196
MacQuarrie, A., and Steed, A. (2017). Cinematic virtual reality: evaluating the effect of display type on the viewing experience for panoramic video. 2017 IEEE Virtual Reality (VR), Los Angeles, CA, USA, March 2017, IEEE, 45–54. doi:10.1109/VR.2017.7892230
Makransky, G., and Petersen, G. B. (2021). The cognitive affective model of immersive learning (CAMIL): a theoretical research-based model of learning in immersive virtual reality. Educ. Psychol. Rev. 33, 937–958. doi:10.1007/s10648-020-09586-2
Makransky, G., Terkildsen, T. S., and Mayer, R. E. (2019). Adding immersive virtual reality to a science lab simulation causes more presence but less learning. Learn. Instr. 60, 225–236. doi:10.1016/j.learninstruc.2017.12.007
Martirosov, S., Bures, M., and Zítka, T. (2022). Cyber sickness in low-immersive, semi-immersive, and fully immersive virtual reality. Virtual Real. 26, 15–32. doi:10.1007/s10055-021-00507-4
Matamala-Gomez, M., Donegan, T., Bottiroli, S., Sandrini, G., Sanchez-Vives, M. V., and Tassorelli, C. (2019). Immersive virtual reality and virtual embodiment for pain relief. Front. Hum. Neurosci. 13, 279. doi:10.3389/fnhum.2019.00279
Mayer, R. E. (2014). Incorporating motivation into multimedia learning. Learn. Instr. 29, 171–173. doi:10.1016/j.learninstruc.2013.04.003
Meehan, M., Insko, B., Whitton, M., and Brooks, F. P. (2002). Physiological measures of presence in stressful virtual environments. Acm Trans. Graph. (Tog) 21 (3), 645–652. doi:10.1145/566654.566630
Meehan, M., Razzaque, S., Whitton, M. C., and Brooks, F. P. (2003). “Effect of latency on presence in stressful virtual environments,” in IEEE Virtual Reality, 2003. Proceedings, Los Angeles, CA, USA, March 2003 (IEEE), 141–148. doi:10.1109/VR.2003.1191132
Merchant, Z., Goetz, E. T., Cifuentes, L., Keeney-Kennicutt, W., and Davis, T. J. (2014). Effectiveness of virtual reality-based instruction on students’ learning outcomes in K-12 and higher education: a meta-analysis. Comput. Educ. 70, 29–40. doi:10.1016/j.compedu.2013.07.033
Meusel, C. R. (2014). Exploring mental effort and nausea via electrodermal activity within scenario-based tasks. Master Dissertation. Iowa State University. doi:10.31274/etd-180810-3757
Meyer, O. A., Omdahl, M. K., and Makransky, G. (2019). Investigating the effect of pre-training when learning through immersive virtual reality and video: a media and methods experiment. Comput. Educ. 140, 103603. doi:10.1016/j.compedu.2019.103603
Milleville-Pennel, I., and Charron, C. (2015). Do mental workload and presence experienced when driving a real car predispose drivers to simulator sickness? An exploratory study. Accid. Analysis Prev. 74, 192–202. doi:10.1016/j.aap.2014.10.021
Mittelstaedt, J. M., Wacker, J., and Stelling, D. (2019). VR aftereffect and the relation of cybersickness and cognitive performance. Virtual Real. 23 (2), 143–154. doi:10.1007/s10055-018-0370-3
Mojang (2011). Minecraft. Available from: https://minecraft.net.
Molina-Carmona, R., Pertegal-Felices, M. L., Jimeno-Morenilla, A., and Mora-Mora, H. (2018). Virtual reality learning activities for multimedia students to enhance spatial ability. Sustainability 10 (4), 1074. doi:10.3390/su10041074
Navon, D., and Gopher, D. (1979). On the economy of the human-processing system. Psychol. Rev. 86 (3), 214–255. doi:10.1037/0033-295X.86.3.214
Nunez, D. (2004). “How is presence in non-immersive, non-realistic virtual environments possible?,” in Proceedings of the 3rd International Conference on Computer Graphics, Virtual Reality, Visualisation and Interaction in Africa, November 2004, 83–86. doi:10.1145/1029949.1029964
Ortega-Rodriguez, P. J. (2021). From broad reality to the Metaverse: critical reflection on the contribution of education. Metaverse 2 (2), 12. Article 2. doi:10.54517/met.v2i2.1784
Paes, D., Irizarry, J., and Pujoni, D. (2021). An evidence of cognitive benefits from immersive design review: comparing three-dimensional perception and presence between immersive and non-immersive virtual environments. Automation Constr. 130, 103849. doi:10.1016/j.autcon.2021.103849
Pallavicini, F., Pepe, A., and Minissi, M. E. (2019). Gaming in virtual reality: what changes in terms of usability, emotional response and sense of presence compared to non-immersive video games? Simul. Gaming 50 (2), 136–159. doi:10.1177/1046878119831420
Parong, J., Pollard, K. A., Files, B. T., Oiknine, A. H., Sinatra, A. M., Moss, J. D., et al. (2020). The mediating role of presence differs across types of spatial learning in immersive technologies. Comput. Hum. Behav. 107, 106290. doi:10.1016/j.chb.2020.106290
Pastel, S., Petri, K., Chen, C.-H., Cáceres, A., Stirnatis, M., Nübel, C., et al. (2022). Training in virtual reality enables learning of a complex sports movement. Virtual Real. 27, 523–540. doi:10.1007/s10055-022-00679-7
Pellegrino, J. W., Alderton, D. L., and Shute, V. J. (1984). Understanding spatial ability. Educ. Psychol. 19 (4), 239–253. doi:10.1080/00461528409529300
Polcar, J., and Horejsi, P. (2015). Knowledge acquisition and cyber sickness: a comparison of VR devices in virtual tours. MM Sci. J. 2015, 613–616. doi:10.17973/MMSJ.2015_06_201516
Prothero, J. (1995). Widening the field of view increases the sense of presence within immersive virtual environments. Technical Report.
Ramaseri Chandra, A. N., El Jamiy, F., and Reza, H. (2022). A systematic survey on cybersickness in virtual environments. Computers 11 (4), 51. Article 4. doi:10.3390/computers11040051
Reason, J. T. (1978). Motion sickness adaptation: a neural mismatch model. J. R. Soc. Med. 71 (11), 819–829. doi:10.1177/014107687807101109
Riva, G., Mantovani, F., Capideville, C. S., Preziosa, A., Morganti, F., Villani, D., et al. (2007). Affective interactions using virtual reality: the link between presence and emotions. CyberPsychology Behav. 10 (1), 45–56. doi:10.1089/cpb.2006.9993
Rosa, P. J., Morais, D., Gamito, P., Oliveira, J., and Saraiva, T. (2016). The immersive virtual reality experience: a typology of users revealed through multiple correspondence analysis combined with cluster analysis technique. Cyberpsychology, Behav. Soc. Netw. 19 (3), 209–216. doi:10.1089/cyber.2015.0130
Rose, F. D., Attree, E. A., Brooks, B. M., Parslow, D. M., and Penn, P. R. (2000). Training in virtual environments: transfer to real world tasks and equivalence to real task training. Ergonomics 43 (4), 494–511. doi:10.1080/001401300184378
Rose, V., Stewart, I., Jenkins, K., Ang, C. S., and Matsangidou, M. (2018). A scoping review exploring the feasibility of virtual reality technology use with individuals living with dementia. Eurogr. Assoc. doi:10.2312/egve.20181325
Santos, B. S., Dias, P., Pimentel, A., Baggerman, J., Ferreira, C., Silva, S., et al. (2008). Head-mounted display versus desktop for 3d navigation in virtual reality: a user study. Multimedia Tools Appl. 41 (1), 161–181. doi:10.1007/s11042-008-0223-2
Sepich, N. C., Jasper, A., Fieffer, S., Gilbert, S. B., Dorneich, M. C., and Kelly, J. W. (2022). The impact of task workload on cybersickness. Front. Virtual Real. 3, 943409. doi:10.3389/frvir.2022.943409
Servotte, J.-C., Goosse, M., Campbell, S. H., Dardenne, N., Pilote, B., Simoneau, I. L., et al. (2020). Virtual reality experience: immersion, sense of presence, and cybersickness. Clin. Simul. Nurs. 38, 35–43. doi:10.1016/j.ecns.2019.09.006
Somrak, A., Humar, I., Hossain, M. S., Alhamid, M. F., Hossain, M. A., and Guna, J. (2019). Estimating VR Sickness and user experience using different HMD technologies: an evaluation study. Future Gener. Comput. Syst. 94, 302–316. doi:10.1016/j.future.2018.11.041
Srivastava, P., Rimzhim, A., Vijay, P., Singh, S., and Chandra, S. (2019). Desktop vr is better than non-ambulatory hmd vr for spatial learning. Front. Robotics AI 6, 50. doi:10.3389/frobt.2019.00050
Steffen, J. H., Gaskin, J. E., Meservy, T. O., Jenkins, J. L., and Wolman, I. (2019). Framework of affordances for virtual reality and augmented reality. J. Manag. Inf. Syst. 36 (3), 683–729. doi:10.1080/07421222.2019.1628877
Stevens, J. A., and Kincaid, J. P. (2015). The relationship between presence and performance in virtual simulation training. Open J. Model. Simul. 3 (02), 41–48. doi:10.4236/ojmsi.2015.32005
Taube, J. S., Valerio, S., and Yoder, R. M. (2013). Is navigation in virtual reality with fMRI really navigation? J. Cognitive Neurosci. 25 (7), 1008–1019. doi:10.1162/jocn_a_00386
Theingi, S., Leopold, I., Ola, T., Cohen, G. S., and Maresky, H. S. (2022). Virtual reality as a non-pharmacological adjunct to reduce the use of analgesics in hospitals. J. Cogn. Enhanc. 6, 108–113. doi:10.1007/s41465-021-00212-9
Thorp, S., Rimol, L. M., and Grassini, S. (2023). Association of the big five personality traits with training effectiveness, sense of presence, and cybersickness in virtual reality. Multimodal Technol. Interact. 7, 11. doi:10.3390/mti7020011
Thorp, S., Sævild Ree, A., and Grassini, S. (2022). Temporal development of sense of presence and cybersickness during an immersive VR experience. Multimodal Technol. Interact. 6 (5), 31. Article 5. doi:10.3390/mti6050031
Tversky, B. (1992). Distortions in cognitive maps. Geoforum 23 (2), 131–138. doi:10.1016/0016-7185(92)90011-R
Umeyama, S. (1993). Parameterized point pattern matching and its application to recognition of object families. IEEE Trans. Pattern Analysis Mach. Intell. 15 (2), 136–144. doi:10.1109/34.192485
Uttal, D. H., Meadow, N. G., Tipton, E., Hand, L. L., Alden, A. R., Warren, C., et al. (2013). The malleability of spatial skills: a meta-analysis of training studies. Psychol. Bull. 139 (2), 352–402. doi:10.1037/a0028446
Venkatakrishnan, R. (2023). The effects of primary and secondary task workloads on cybersickness in immersive virtual active exploration experiences. Doctoral dissertation. Clemson University.
Venkatakrishnan, R., Bhargava, A., Venkatakrishnan, R., Lucaites, K. M., Volonte, M., Solini, H., et al. (2019). “Towards an immersive driving simulator to study factors related to cybersickness,” in IEEE Conference on Virtual Reality and 3D User Interfaces (VR), Osaka, Japan, March 2019 (IEEE), 1201–1202. doi:10.1109/VR.2019.8797728
Venkatakrishnan, R., Venkatakrishnan, R., Anaraky, R. G., Volonte, M., Knijnenburg, B., and Babu, S. V. (2020). “A structural equation modeling approach to understand the relationship between control, cybersickness and presence in virtual reality,” in 2020 IEEE Conference on Virtual Reality and 3D User Interfaces (VR), Atlanta, GA, USA, March 2020 (IEEE), 682–691. doi:10.1109/VR46266.2020.00091
Venkatakrishnan, R., Venkatakrishnan, R., Raveendranath, B., Sarno, D. M., Robb, A. C., Lin, W. C., et al. (2023). The effects of auditory, visual, and cognitive distractions on cybersickness in virtual reality. IEEE Trans. Vis. Comput. Graph., 1–16. doi:10.1109/TVCG.2023.3293405
Wang, J., Shi, R., Zheng, W., Xie, W., Kao, D., and Liang, H.-N. (2023). Effect of frame rate on user experience, performance, and simulator sickness in virtual reality. IEEE Trans. Vis. Comput. Graph. 29, 2478–2488. doi:10.1109/TVCG.2023.3247057
Webster, R. (2016). Declarative knowledge acquisition in immersive virtual learning environments. Interact. Learn. Environ. 24 (6), 1319–1333. doi:10.1080/10494820.2014.994533
Weech, S., Kenny, S., and Barnett-Cowan, M. (2019). Presence and cybersickness in virtual reality are negatively related: a review. Front. Psychol. 10, 158. doi:10.3389/fpsyg.2019.00158
Winn, W., Windschitl, M., and Fruland, R. (2002). When does immersion in a virtual environment help students construct understanding.
Witmer, B. G., and Singer, M. J. (1998). Measuring presence in virtual environments: a presence questionnaire. Presence: Teleoperators Virtual Environ. 7 (2), 225–240. doi:10.1162/105474698565686
Keywords: immersion, sense of presence, performance, simulation sickness, cognitive load, cybersickness
Citation: Thorp SO, Rimol LM, Lervik S, Evensmoen HR and Grassini S (2024) Comparative analysis of spatial ability in immersive and non-immersive virtual reality: the role of sense of presence, simulation sickness and cognitive load. Front. Virtual Real. 5:1343872. doi: 10.3389/frvir.2024.1343872
Received: 24 November 2023; Accepted: 05 March 2024;
Published: 14 March 2024.
Edited by:
Panagiotis Kourtesis, American College of Greece, GreeceReviewed by:
Stephen B. Gilbert, Iowa State University, United StatesCopyright © 2024 Thorp, Rimol, Lervik, Evensmoen and Grassini. This is an open-access article distributed under the terms of the Creative Commons Attribution License (CC BY). The use, distribution or reproduction in other forums is permitted, provided the original author(s) and the copyright owner(s) are credited and that the original publication in this journal is cited, in accordance with accepted academic practice. No use, distribution or reproduction is permitted which does not comply with these terms.
*Correspondence: Sebastian Oltedal Thorp, c2ViYXN0aWFuLnRob3JwQG91dGxvb2suY29t
Disclaimer: All claims expressed in this article are solely those of the authors and do not necessarily represent those of their affiliated organizations, or those of the publisher, the editors and the reviewers. Any product that may be evaluated in this article or claim that may be made by its manufacturer is not guaranteed or endorsed by the publisher.
Research integrity at Frontiers
Learn more about the work of our research integrity team to safeguard the quality of each article we publish.