- Chair for Human-Centered Artificial Intelligence, Faculty of Applied Computer Science, University of Augsburg, Augsburg, Germany
In a world increasingly driven by AI systems, controversial use cases for AI that significantly affect people’s lives become more likely scenarios. Hence, increasing awareness of AI bias that might affect underprivileged groups becomes an increasing challenge. As Virtual Reality has previously been shown to increase empathy through immersive perspective-taking, we conducted a laboratory study in which participants were confronted with a biased Wizard of Oz AI while embodying personas that varied widely in their ability to achieve high financial credit scores due to their age and gender. We found that participants embodying personas in VR felt significantly more empathy toward the characters they embodied and rated the AI as significantly less fair compared to a baseline condition in which they imagined to be these characters. Furthermore, we investigate differences between embodied personas and discuss qualitative results to gain insight into the participant’s mental model creation.
1 Introduction
With applications such as ChatGPT, DALL-E 2, or Midjourney, AI technologies are currently receiving increased attention from the general public and find use in many sectors, such as arts or creative writing. However, while there are benefits for many people using these technologies, dangers arise, e.g., professionals in such creative sectors that fear losing customers and potentially their profession. While these dangers need to be taken seriously, there are AI use cases that can cause harm in an even greater scope. These include governments, social goods provision institutions, or banks that use AI algorithms to assess actual people to make decisions that may substantially affect their lives. Example use cases include AI-based prediction of the likelihood of criminal relapse (Angwin et al., 2016), AI-based assistance for unemployment help (e Estoniacom, 2021), and credit scoring (Dheeru, 2017).
A problem that can arise when using AI systems for the assessment of humans is that the employed systems are usually trained on data from actual institutions or societies that used to conduct these tasks over extended periods. By doing so, such AI systems reproduce biases and injustices toward specific groups, for instance, by rating a bank’s female customers’ creditworthiness (called credit score in this paper) worse than for male customers. There are tools such as the IBM Fairness 360 toolkit (Bellamy et al., 2019) that can help to identify and mitigate such biases. However, policies on whether biases should be reproduced, mitigated, or altered should not only be made by individuals but also be part of a public social debate (European Commission, 2020). One idea to include stakeholders that represent the broad society in decisions on how to handle AI design is to conduct co-creative workshops that educate people on problems with AI and let them participate in the design of decision policies or even solutions that tackle goals such as AI transparency or trust (consortium, 2021). However, means and strategies that aim to include broad society stakeholders in such collaborative and participatory processes still need to be researched and designed.
Two essential steps towards the vision of collaborative AI design are to explain the phenomenon of AI bias to non-experts in AI and to increase awareness of its severe potential effects on discriminated people and their lives. If one is privileged, however, it can be hard to really “feel” the severity of AI-based discrimination. It can be hard to put oneself into the perspective of, e.g., underprivileged people to increase empathy, which was coined by Alfred Adler in 1956 as “[…] seeing with the eyes of another, listening with the ears of another, and feeling with the heart of another.” (Ansbacher and Ansbacher, 1956), since AI-based decision-making might be too abstract for people to grasp.
In previous studies, VR technologies have been the focus of researchers aiming to promote empathy (Piumsomboon et al., 2017; Troeger and Tümler, 2020) or even reduce racial bias (Peck et al., 2013; Banakou et al., 2016) through perspective-taking and embodying virtual avatars of other persons. However, it remains unclear whether such means of VR-based perspective-taking can increase awareness of AI bias or empathy for victims of AI discrimination. As such, we conducted a user study that compared two means for perspective-taking in terms of effectiveness to achieve these goals: Classical “mental” perspective-taking or role-playing (condition: Mental Embodiment) and VR-based embodiment of virtual characters, including whole-body and motion tracking (condition: Virtual Embodiment). In our study, we confronted participants who embodied various personas with a highly biased Wizard of Oz AI that seemingly assessed their creditworthiness based on personality traits.
By analyzing quantitative data, we investigated the effectiveness of the aforementioned perspective-taking modalities regarding empathy for the embodied personas and perceived AI fairness. Furthermore, we analyzed open questions regarding the AI’s bias in order to understand how accurate participants identified personality features that were relevant to the AI’s decisions in order to assess the quality of their mental model of the AI. After diving into related work in various research fields, we describe our study methodology in Section 4. Then, we report our results in Section 5 and discuss our findings in Section 6.
2 Related work
AI and VR have changed our lives, including decision-making processes and how stakeholders interact with digital systems. In this section, we delve into three relevant aspects of these technological advancements: the impact of AI and AI biases on decision-making and using VR to support empathy-building and bias-awareness in stakeholders. Here, we explore the existing literature on the impact of AI on decision-making and the strategies proposed to address biases. We also examine the utilization of VR in empathy-building experiences and the benefits it offers compared to real-world settings. By understanding the interplay between AI, biases, and empathy, we aim to contribute to developing ethically responsible and socially beneficial technologies.
2.1 AI for social assessment
AI has emerged as a powerful tool that aids decision-making processes across multiple domains (e.g., healthcare (Rajpurkar et al., 2022) to manufacturing companies (Waltersmann et al., 2021)). With its ability to analyze vast amounts of data, AI algorithms can provide valuable insights and recommendations to support decision-makers. The potential of AI-based decision support is attracting increasing interest from the general public and policymakers, as it can help quantify risks and assist in human decision-making processes (Cresswell et al., 2020).
The specific situation and challenges of using AI for decision-support in social service provision lie in the intersection of technology and human welfare. As AI-based decision support gains attention in this domain, it raises important questions about ethics, fairness, and transparency. One challenge is ensuring that AI algorithms are free from biases that could perpetuate social inequalities. The data used to train these algorithms may reflect historical biases, leading to biased outcomes in social service decisions. Addressing this challenge is important for not intensifying already existing power asymmetries (Kuziemski and Misuraca, 2020) and instead minimizing discriminatory effects. The empirical investigation of the (possible) impact of AI on areas of the public sector is still neglected (Sun and Medaglia, 2019).
Studying and understanding methods to explain and visualize AI is crucial to avoid mindlessly relying on AI systems and to become aware of any biases they may have. In the next section, we will delve deeper into why creating this awareness is essential, especially when examining how AI affects privileged and unprivileged groups of people.
2.2 AI bias understanding
The increasing reliance on AI systems brings concerns about biases inherent within the algorithms or the data used to train them (Roselli et al., 2019). Bias in AI systems has gained substantial attention in recent years, as it poses significant ethical and societal challenges (Yapo and Weiss, 2018; Nelson, 2019; Ntoutsi et al., 2020). When trained on biased or unrepresentative data, AI algorithms can perpetuate and amplify societal biases. Such biases can lead to discriminatory outcomes, reinforcing existing inequalities and marginalizing certain groups of individuals. Therefore, understanding the impact of AI on decision-making and identifying strategies to mitigate biases is crucial for building fair, just, and inclusive systems (Ntoutsi et al., 2020). AI biases can be found in different AI systems (Buolamwini and Gebru, 2018). Here, authors differentiate between various sources of biases, for example, machine biases, human biases, or societal biases (Zajko, 2021). In our paper, we focus on societal biases. Therefore we will now dive further into this domain.
When delving into societal biases, it’s essential to understand their nature and impact on various aspects of society. Societal biases refer to the biases that exist within social structures, institutions, and cultural norms, which can influence people’s beliefs, behaviors, and opportunities based on their social characteristics, such as race, gender, ethnicity, religion, or socioeconomic status (Zajko, 2021). One example of societal bias in the area of employment is the detected AI bias in the Amazon recruiting tool: The tool showed bias against female candidates, as it was trained on historical data predominantly composed of male hires, resulting in a preference for male applicants (Dastin, 2022) (gender bias). Another example is AI bias in recidivism prediction with the COMPAS algorithm, a tool to calculate the probability of recidivism of criminals: The algorithm predicted a higher likelihood of future criminal behavior for individuals from minority communities (i.e., People of Color), contributing to unjust outcomes and perpetuating social inequalities (Angwin et al., 2016) (racial bias). These examples have in common unequal treatment based on the characteristics of a privileged group (i.e., Amazon: men; COMPAS: white people) and an unprivileged group (i.e., Amazon: women; COMPAS: People of Color). By conducting sociological analysis, researchers can gain insights into the origins of inequality within society Rosanvallon (Rosanvallon, 2014). We argue that VR offers a unique and necessary perspective to complement it. VR goes beyond theoretical observations and allows individuals to experience firsthand the realities that marginalized groups face. In the following subsection, we focus on the benefits of this technology for AI bias research.
2.3 VR as methodology for AI bias research
In parallel with the advancements in AI, VR has emerged as a compelling technology for creating immersive experiences. VR enables users to enter virtual environments and engage with digital content in a highly realistic and interactive manner. Immersion influences the experience of presence and empathy. Troeger and Tümler (Troeger and Tümler, 2020) show that interactions in VR lead to a more vital experience of presence and a more intensive experience of empathy than interactions on a computer screen.
The immersive nature of VR has paved the way for innovative applications, including perspective-taking and empathy-building experiences (Yee and Bailenson, 2007; Ahn et al., 2013; Bailenson, 2018; Stavroulia and Lanitis, 2023). Empathy is fundamental in understanding others’ emotions, experiences, and perspectives (Cohen and Strayer, 1996). Slater and Sanchez-Vives (Slater and Sanchez-Vives, 2014) illustrate the concept of body ownership in VR: Body ownership refers to feeling connected or embodied with a virtual avatar or body representation within the virtual environment. When users wear VR headsets and interact with virtual worlds, tracking of body features can allow them to see a virtual body or hands that mirror their movements. This visual and sometimes haptic feedback creates a sense of ownership and agency over the virtual body, tricking the brain into perceiving the virtual body as an extension of the user’s physical self. By enabling stakeholders to embody different identities or situations virtually, VR can foster empathy and promote a deeper understanding of diverse viewpoints (Ahn et al., 2013; Peck et al., 2013). This immersive medium allows stakeholders to gain firsthand experiences that simulate real-world settings, providing a unique opportunity to bridge gaps in understanding and foster empathy toward different social, cultural, and personal contexts.
Importantly, empathy can act as a powerful tool in mitigating biases. By enabling stakeholders to experience firsthand the challenges and biases others face, VR can facilitate a shift in perspective and promote empathy-driven decision-making. Through empathy-building experiences, stakeholders can develop a heightened awareness of their biases and become more open to alternative viewpoints. This, in turn, can support creation of AI systems that are more equitable, inclusive, and sensitive to the needs of diverse populations. Peck et al., 2013 use VR to induce illusions of ownership over a virtual body and its potential impact on implicit interpersonal attitudes. They found that when light-skinned participants embodied a dark-skinned virtual body significantly reduced their implicit racial bias against dark-skinned people. The work of Chen et al., 2021 found similar results. The results suggest that embodiment in a dark-skinned virtual body led to a greater decrease in implicit racial bias than other conditions, indicating that the VR technique could be a powerful tool for exploring societal phenomena related to interpersonal attitudes. In the work of Banakou et al., 2016, the authors found that this reduction of implicit biases lasts over a longer period (i.e., more than 1 week). This indicates that virtual experiences may have lasting impacts on the cognition and behavior of people. Similar positive impacts of VR on reducing societal biases were found for gender bias (Schulze et al., 2019; Beltran et al., 2021) and age bias (Banakou et al., 2018).
While previous research has primarily focused on addressing societal bias in VR within human-to-human interactions, our investigation delves into the significant impact of VR on user perception of age and gender biases generated by AI. To the best of our knowledge, our study is the first to investigate the potential of VR-based perspective-taking to promote AI bias awareness in a social assessment context.
3 Hypotheses
Previous work gave strong evidence VR can reduce social prejudices and biases towards other humans (Peck et al., 2013; Chen et al., 2021) and was shown to be a potent tool for perspective-taking and empathy-building experiences (Yee and Bailenson, 2007; Ahn et al., 2013; Bailenson, 2018; Stavroulia and Lanitis, 2023). Based on these findings, we hypothesize that similar effects can be achieved in a VR-based perspective-taking scenario in which one embodies a persona that AI unfairly assesses in a credit scoring context, which can be used to increase awareness of the AI’s bias. As a baseline, we chose traditional perspective-taking, which is done by solely imagining to be a person. More specifically, the hypotheses that we aim to prove statistically are as follows:
H1: Participants that embody personas in VR (virtual embodiment condition) experience increased (total) empathy towards these personas compared to embodying similar personas in a role-playing scenario (condition: mental embodiment).
H2: Participants that embody personas in VR rate the highly biased Wizard of Oz AI as less fair than in the mental embodiment condition.
The next chapter will explain the experiment setup and our methods, including both conditions, in greater detail.
4 Materials and methods
4.1 Experiment setup
4.1.1 Condition design
We conducted a laboratory study in autumn of 2022 in Germany. We measured and compared perceived AI fairness and empathy towards eight distinct embodied personas (four per condition, see Figures 4, 5) within the following conditions:
ME—Mental Embodiment: In this condition, participants were given profiles of four personas (See Figure 4) in printed form in advance. Subsequently, after memorizing the profile data, they were asked to role-play these personas while being assessed by a (Wizard of Oz) credit scoring AI (See Figure 6) in a laboratory environment (See Figure 1).
VE—Virtual Embodiment: In this condition, participants embodied the virtual avatars of four personas (See Figure 5) in VR though motion capturing devices. Similar to the ME condition, they would receive a digital profile of their persona in VR before entering a digital replica of the laboratory environment (See Figure 2) and being assessed by a digital replica of the Wizard of Oz AI (See Figure 7).
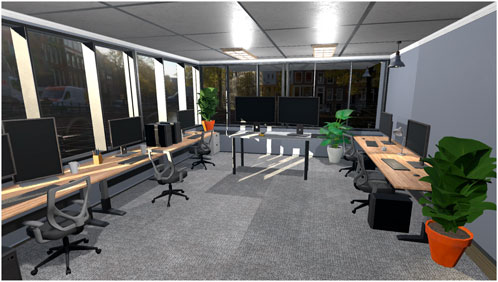
FIGURE 2. Virtual environment that was experienced in the VE condition. Created with Unity Editor®. Unity is a trademark or registered trademark of Unity Technologies.
As perceived AI fairness and empathy towards persona are expected to deviate substantially between participants, we chose a within-subjects experiment design, greatly increasing the sensitivity regarding conditional effects at the cost of each participant going through the experiment two times. Since a certain amount of AI interactions is required to get a “feel” for it and its bias, we considered the repetitions advantageous. To mitigate order effects, each participant saw both conditions, ME and VE, in an order that we selected randomly. We used an HTC Vive Pro Headset with standard controllers and a gaming PC, including a GeForce RTX 3070 Ti graphic card, for the VE condition. Additionally, each participant wore three HTC VIVE trackers1 for full body tracking (see left image in Figure 3).
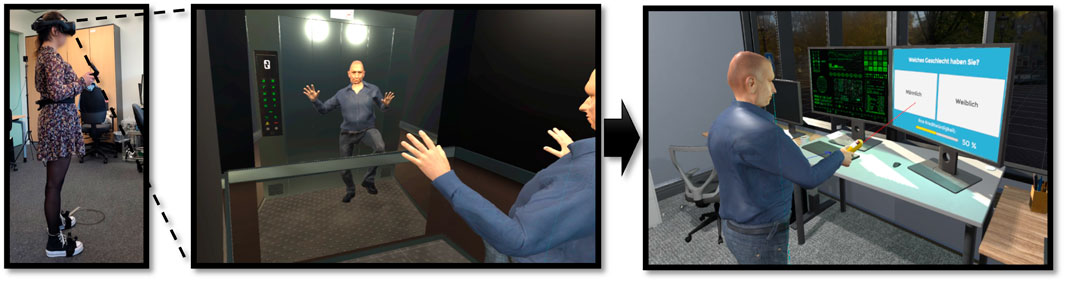
FIGURE 3. A participant embodies a persona representing a differently privileged demographic group through full-body tracking in VR. In our experiment, she would get comfortable with her avatar in a mirrored elevator (second image) before being exposed to a heavily biased AI system (right image). We investigated empathy and AI awareness for such scenarios. Created with Unity Editor®. Unity is a trademark or registered trademark of Unity Technologies.
4.1.2 Persona design
A crucial decision we needed to make during the experiment design process was to either use the same four personas per condition or to use distinct personas for each condition, resulting in a total of eight personas. While the first option would have minimized the impact of persona-related confounding variables, it would have made it harder for the participants to develop a mental model or “feel” for the AI’s decision. For instance, participants might have assumed that the AI memorized the individual personas between conditions. Furthermore, as the amount of individual AI persona assessments that participants would experience would be halved, distinguishing relevant from irrelevant features would be more challenging, which is crucial to understanding AI bias.
As such, we designed four distinct personas per condition (see Figures 4, 5; Supplementary Figure S5). As the AI was (hard-) coded to prefer male over female and older over younger personas heavily, we combined these traits in a 2 × 2 matrix so that each condition had a persona that was heavily under-privileged (female and young), two personas were medium privileged (female and old/young and male) and one persona was over-privileged (male and old). In order to make the identification of relevant features for the biased AI (age and gender) less trivial, we added the additional features name, origin, yearly net income, financial assets, profession to the persona profiles that were handed out to participants (see Supplementary Material for translated profiles that list these features).
We designed virtual characters for each of the eight personas using the MakeHuman open source tool for 3D character creation2. The four avatars we used for the virtual embodiment condition were integrated into a VR environment that we created with Unity engine3. Full-body tracking was implemented by using three HTC VIVE trackers, and the Final IK package by RootMotion.4 The remaining four avatars for the mental embodiment condition were used as profile pictures for the printed persona profiles that participants received.
4.1.3 Wizard of Oz AI design
We hard-coded a Wizard of Oz AI, which was seemingly trained to estimate a credit score based on the persona’s traits that needed to be put in by the participants, either using a mouse on a PC that ran a software application in the ME condition (see Figure 6) or a digital replica of that PC with the same application in VR for condition VE (See Figure 7). The ruleset of the credit scoring AI was assigning a given credit score to each persona. The credit score results were heavily biased in favor of male and older personas (see Table 1), which was inspired by German credit scoring data (Hofmann, 1994) that was used as an AI bias illustration for IBM’s AI Fairness 360 Toolkit5. In order to make the results more credible, we deviated the percentages between similarly privileged personas by 1–2 percent. We designed the AI assessment rules only to take age and gender into account while neglecting other additional persona traits such as net income, origin, profession, and others.
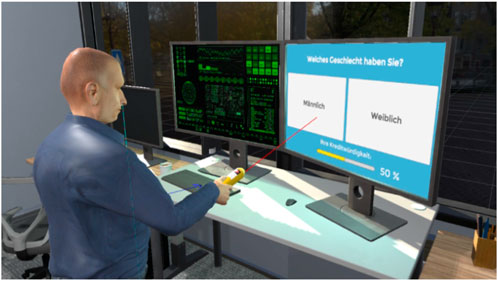
FIGURE 7. Wizard of Oz AI in the VE condition. Created with Unity Editor®. Unity is a trademark or registered trademark of Unity Technologies.
4.2 Measures
For hypothesis H1, we adopted the questionnaire items by Schutte et al. (Schutte and Stilinović, 2017) and translated them into German before including its items in questionnaire A. The questionnaire measures two subscales for empathy, Empathic Perspective Taking and Empathic Concern, with four items each. However, we did not consider these individual subscales as dependent variables. Instead, we focused on the Total Empathy score, which is the sum of these subscales. To the authors’ knowledge, there is no validated questionnaire for perceived AI fairness in H2. Hence, we included the original question How fair do you think was the AI that calculated the credit score? (translated from German) into questionnaires A and B, which participants answered on a 7-point Likert scale from not fair (1 point) to fair (7 points).
Additionally, in order to assess the quality of the reasoning or understanding that participants derived from interacting with the Wizard of Oz AI (the quality of their mental model creation), we analyzed qualitative data from an open question in questionnaire B. In this question, we asked participants to give possible reasons that could have led to the AI’s decision. Similar to previous studies on mental model elicitation about AI-Systems (Anderson et al., 2019; Huber et al., 2021; Weitz et al., 2021; Mertes et al., 2022), we subsequently conducted an inductive thematic analysis (Braun and Clarke, 2012). The results are discussed in Section 5.3.
4.3 Study procedure
The core study flow is a standard within-subjects experiment design, where each participant sees both conditions, ME and VE, in randomized order. Before the stimuli and measurements, we introduced participants to the overall experiment procedure, including the information that they will embody various personalities who try to get financial credit from a bank that uses an AI to assess them. An illustration of the overall study procedure can be found in Figure 8.
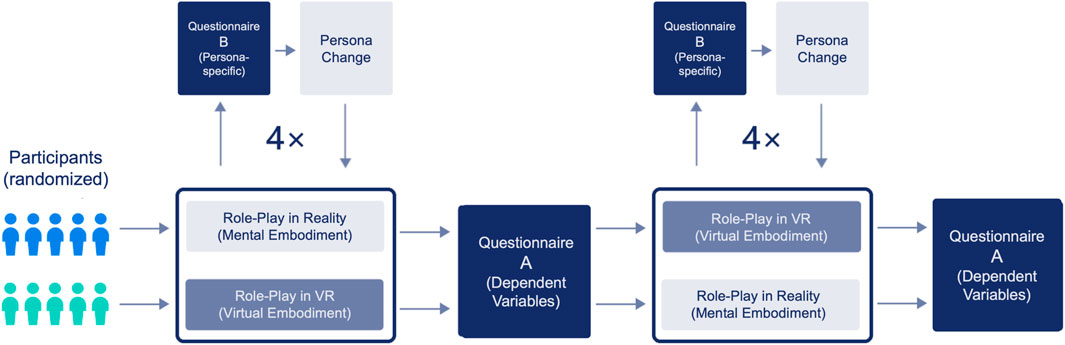
FIGURE 8. Overview of the study procedure. Questionnaire A measured dependent variables, and Questionnaire B persona-specific AI fairness as additional and exploratory data.
Dependent variables were measured by filling out Questionnaire A after both stimuli. In each condition, participants embodied four personas while going through the following steps four times per condition:
1. Handing out of persona information either in printed form (ME) or as a virtual sheet (VE).
2. Participants spent some time familiarizing themselves with the persona information and the character. In the VE condition, participants spent time in a virtual elevator with a mirror to get a feel for their digital avatar. In the ME condition, they were asked to imagine being the given persona for the same amount of time before proceeding to the credit scoring stage.
3. After familiarization and memorization of the persona traits, participants would enter the assessment room and answer questions that demanded the input of their personality traits on a physical computer screen (ME) or digital screens in VR (VE).
4. After answering the questions, participants pressed a calculate button and needed to wait for a short while which resembled the AI assessment stage, before seeing their overall credit score.
5. After receiving their credit score, participants filled out the persona-specific questionnaire B, which we included to gather additional exploratory data.
6. Finally, participants switched personas and avatars (in the VE condition) before starting again at step 1.
We measured perceived AI fairness using the question we formulated for H2 for all embodied characters in a persona-specific questionnaire (Questionnaire B in Figure 8). However, our experiment design did not consider the persona type as an independent variable. We included the short Questionnaire B to gain additional insights for future developers or researchers who want to design personas that can be used to increase awareness of biased AI. To mitigate order effects for the persona-specific AI fairness scores in questionnaire B, we randomized the order of the four personas within each condition.
4.4 Participants and sample size
A pre-test power analysis revealed a required sample size of 21 participants for a power of 0.8, an α-error probability of 0.05, and an estimated effect size of 0.5. With one participant headroom, we acquired 22 participants at the university campus for our study. Participants were primarily students from Germany aged between 18 and 26 years (M = 23,32, SD = 1,96). Nine of them identified as female, and 13 identified as male. All 22 participants had little to no prior experience with VR or full-body tracking.
5 Results
5.1 Perceived empathy (H1)
To test for H1 (see 3), we checked for normal distribution using the Shapiro-Wilk-Test (Shapiro and Wilk, 1965) and for equal variances using Levene’s test (Levene, 1961). The scores for both conditions were found to be normally distributed (Shapiro-Wilk test p = 0.13 for condition ME and p = 0.4 for condition VE). The null hypothesis for unequal variances was rejected (Levene’s test F (Shapiro and Wilk, 1965; Angwin et al., 2016) = 2.93, p = 0.09). Consequently, we tested parametrically using Student’s paired sample t-test (Student, 1908), which yielded a value of p = 0.001, indicating that there is a statistically significant difference between the two samples (Condition ME: M = 2.97, SD = 0.62; Condition VE: M = 4.01, SD = 0.42). This difference can also be seen in Figure 9. After p-value correction using the Holm-Bonferroni method (Holm, 1979), the p-value increased to 0.0167, but the result remains significant. A cohen’s d of 0.59 indicates a medium effect.
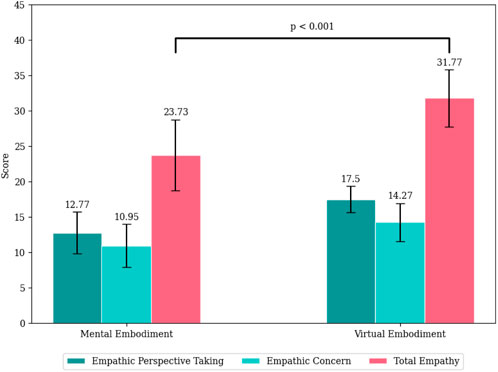
FIGURE 9. Empathy towards the embodied personas for both conditions. Error bars represent standard deviations. The maximum score for total empathy was 40 and 20 for both subscales.
5.2 Perceived AI fairness (H2)
The scores for the ME condition were found to be normally distributed (Shapiro-Wilk test p = 0.002 for condition ME and p = 0.057 for condition VE). The null hypothesis for unequal variances was rejected (Levene’s test F (Shapiro and Wilk, 1965; Angwin et al., 2016) = 0.07, p = 0.79). Consequently, the non-parametric Wilcoxon signed-rank test (Wilcoxon, 1992) was used to test H2, which yielded a value of p = 0.004, indicating that there is a statistically significant difference between the two samples (Condition ME: M = 2.95, SD = 0.95; Condition VE: M = 1.9, SD = 0.92). This difference can also be seen in Figure 10. After p-value correction using the Holm-Bonferroni method (Holm, 1979), the p-value increased to 0.025, but the result remains significant. A pearson’s r of 0.61 indicates a large effect. Besides this result, Figure 10 also reports on persona-specific credit scores that we calculated from Questionnaire B (see Figure 8). However, as we did not consider them dependent variables, we did not calculate statistical tests for them.
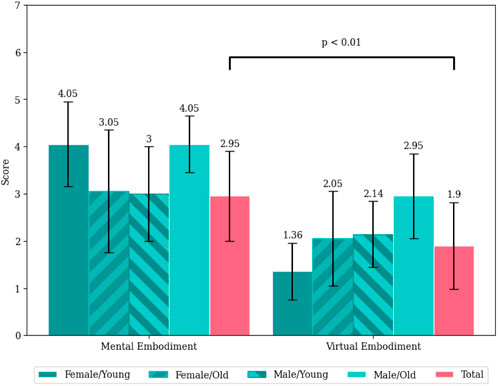
FIGURE 10. Perceived AI fairness for individual personas and in total. Darker color means less privilege for the persona-specific turquoise bars. Error bars represent standard deviations.
5.3 Mental model creation
We evaluated the participant’s ratios of correctly identifying features that reflect the AI’s bias to assess the mental model creation for both conditions. Thus, we first conducted an inductive thematic analysis (Braun and Clarke, 2012) using MaxQDA software6. During this analysis, we assigned codes to relevant phrases in participants’ answers to the open question in Questionnaire A, which asked them to name decision criteria they thought were relevant to the AI’s decision. Subsequently, we counted their frequencies for both conditions. Table 2 lists these frequencies. As can be seen, the features that were most accurate regarding the AI’s biases (age and gender) were the most prominent in both conditions. In the VE condition, correct decision criteria were mentioned more often (35 mentions) than in the ME condition (27 mentions). However, since more codes are present in the VE condition, the relative proportion of correct answers is similar (∼63%) for both conditions.
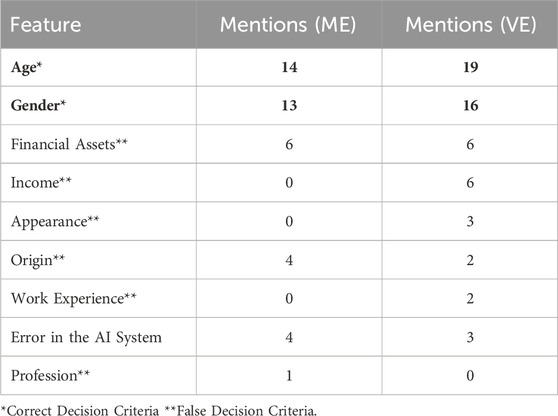
TABLE 2. Results of the Thematic Analysis for AI Decision Criteria. The table list the frequency of mentions of the features in the open questions in both conditions (ME and VE).
6 Discussion
In this section, we discuss our results and limitations. Each subsection’s heading is a key takeaway distilled by the authors.
6.1 Embodying persona in VR significantly increased empathy towards them
Participants that embodied personas through full-body tracking in VR (condition VE) scored significantly higher in regards to (total) empathy towards the embodied characters than in the mental role-playing situation (condition: ME). Hence, we accept hypothesis H1. This observation is consistent with findings from previous studies that VR can increase empathy (Ahn et al., 2013; Peck et al., 2013), e.g., through perspective-taking. We observed increased empathy across both subscales, Empathic Perspective Taking and Empathic Concern uniformly (see Figure 9). Hence, participants could take the embodied persona’s perspective more effectively and be more concerned regarding the embodied persona that the Wizard of Oz AI assessed. According to participant feedback, a critical stage for the success of the increased perspective-taking capabilities was the familiarization stage, during which participants in the VE condition needed to watch themselves in a mirror in an elevator before entering the virtual environment in which they interacted with the AI system.
6.2 Increased empathy goes hand in hand with a decrease in perceived AI fairness
Participants that were assessed by the Wizard of Oz AI while embodying virtual characters in the VE condition gave the AI significantly lower fairness scores than in the ME condition. As such, we accept hypothesis H2. As we hard-coded the Wizard of Oz AI to be heavily biased in favor of older and male demographics, such lower ratings reflect the AI behavior more accurately. As our qualitative data analysis regarding mental model creation did not unveil a difference between both conditions in terms of accurate bias or decision criteria estimation, this difference is most likely to stem from the emotional response that results from increased empathic concern through perspective-taking in VR. However, decreased AI fairness ratings could also be an effect of slight deviations between the persona we used in the conditions to stimulate mental model creation (refer to Section 4.1.2 for the detailed reasoning behind this experiment design choice). Further, it shall be noted that our questionnaire item that measured perceived AI fairness was not psychometrically validated.
6.3 Embodying personas with lower privilege reduced perceived AI fairness
In addition to measuring dependent variables in the post-stimulus questionnaires (Questionnaire A in Figure 8), we exploratively measured perceived AI fairness for individual personas within both conditions after each AI assessment iteration (Questionnaire B in Figure 8). The results show that for both conditions, embodying less privileged personas received lower AI fairness ratings (see Figure 10). An exception to this is the relatively high fairness score for the female and young persona in the ME condition. While the reason for this observation is unclear to the authors, a possible explanation might be a correlation with the demographic of our study participants, which happened to be mostly younger and female. It could be possible that participants in the ME condition were more willing to accept lower credit scores for the female and young persona as it came closest to them demographically, and they did not necessarily consider themselves creditworthy (they were primarily students with low income). Furthermore, there might have been a general tendency to perceive lower credit scores as less fair. Nevertheless, as we did not include persona demographics as an independent variable, our reported persona-specific fairness scores should be considered an exploratory measurement with limited empirical validity. Furthermore, the slight deviations in the hard-coded credit scores we implemented to make the scores more credible (see Section 4.1.3) might have been a small confounding factor.
6.4 Perceived AI Fairness is not necessarily feature-dependent
Even though the age and gender-based biases in our AI system had similar numerical impacts on the credit score ratings, they might not necessarily be perceived as equally unfair by participants. After all, unfairness is highly subjective and might depend on personal political stances or values. As such, one cannot assume that the bias regarding age (which might be associated with net worth or income) was assessed to be equally as severe as the bias concerning gender. However, we did not observe different fairness ratings between the medium privileged persona types Female/Old and Male/Young in both conditions (see Figure 10). This observation suggests that either both biases were perceived as equally severe or that perceived AI fairness directly reflects the assessment scores, independent of their causes. Either way, persona-specific fairness ratings did not hint at a feature dependency but rather at dependence on the overall AI assessment result.
6.5 Our sample demographic imposes limitations
Since our sample mainly consisted of participants of a younger demographic (18–26 years), our findings remain limited to this particular demographic, which tends to be more affine towards new technologies such as VR and more left-leaning regarding political stance and values. Furthermore, conducting such experiments with people from non-western cultures would be valuable to investigate the effects of varying social norms and values. Furthermore, as our sample mainly consisted of demographics that would be under or medium-privileged if the AI assessed themselves, participants might have been more likely to develop empathy and rate the AI lower in fairness for personas that are closer to their demographic (compare study by Fowler et al., 2021). However, we expect this effect to only alter the persona-specific (exploratory) data and not dependent variables that are related to hypotheses H1 and H2 (see Section 3). Since our young sample is not representative of broader society, we are hesitant to universally confirm our approach’s effectiveness. However, we still regard the results of this study as a great motivation to invest in empathy-oriented VR research when it comes to increasing awareness of AI bias and as a first proof of concept.
6.6 Cultural limitations and practical use cases remain to be addressed in future research
Our results suggest that VR can be a powerful tool for enhancing the awareness and understanding of AI biases. For instance, similar to the work of Salmanowitz (Salmanowitz, 2016), VR-based perspective-taking can be a helpful tool to foster empathy among decision-makers (e.g., in courtrooms) and to make AI biases more visible. In VR, decision-makers can freely explore AI biases without fear of real-world consequences, facilitating deeper introspection and self-awareness. This safe space encourages people to be more receptive to AI bias awareness efforts and more motivated to address biases actively. However, even though we did not hear of any negative study consequences from participants, we note that emotional impacts on recipients of VR-based perspective-taking may manifest themselves in unpredictable ways. As such, we note that emotional consequences should be considered and discussed in advance for the target demographic and the respective perspective-taking scenario (e.g., credit scoring in our study). In order to overcome some limitations of our study, we aim to conduct similar experiments with participants from other demographics or cultural backgrounds to reveal the cultural-specific limitations of our approach. Furthermore, we want to investigate the effect of increasing awareness of AI bias through VR-based perspective-taking for stakeholders that represent the broad society before they participate in design workshops for AI solutions that are considered fair in social assessment contexts. VR and mixed reality are becoming increasingly adopted in everyday life, e.g., through newly revealed products such as the Apple Vision Pro. Specialized use cases, such as awareness and empathy training through VR-based perspective-taking, will become more and more viable and available for a broader audience. Our results suggest that such experiences can be a new medium for intercultural and intersubjective understanding through the experience of feeling “as if” one is somebody else, which was historically achieved through means featuring storytelling from a different perspective, such as film, theater, or books.
7 Conclusion
In this paper, we reported on a study that investigates the means of embodiment of virtual characters in VR in order to increase awareness for AI bias and empathy and towards persona that represent demographics that differ widely in terms of their privilege in a credit scoring scenario. In this study, we compared perspective-taking using full-body tracking in VR to a baseline condition during which participants were asked to imagine being other persona before receiving a score for creditworthiness from a biased Wizard of Oz AI.
Our main findings that we derived from both qualitative and quantitative data analysis are:
1. Embodying virtual avatars in VR significantly increased empathy towards the embodied persona compared to the baseline condition.
2. Embodying virtual avatars in VR significantly decreased perceived AI fairness when compared to the baseline condition, which more accurately reflects the severity of the AI’s bias towards certain demographics.
3. Mental model creation was of similar quality in both conditions, which suggests a link between increased empathy and decreased perceived AI fairness in perspective-taking scenarios that incorporate social assessment AI.
4. Exploratory data revealed that participants rated the AI to be less fair when embodying less privileged personas, which indicates an increased effectiveness for AI bias sensitization when embodying underprivileged groups.
To our knowledge, the study at hand is the first to address the use case of promoting awareness of AI bias through perspective-taking in VR. Our results suggest that this approach can be a promising tool to promote AI bias awareness by building empathy for underprivileged groups. Hence, we encourage the application of VR-based empathy-building measures in domains such as education, public debates on AI fairness, or even participatory design workshops that aim to design AI systems for social assessment.
Data availability statement
The original contributions presented in the study are included in the article/Supplementary Material, further inquiries can be directed to the corresponding author.
Ethics statement
Ethical approval was not required for the studies involving humans because No potentially harmful procedures or vulnerable groups were involved. The studies were conducted in accordance with the local legislation and institutional requirements. The participants provided their written informed consent to participate in this study. Written informed consent was obtained from the individual(s) for the publication of any potentially identifiable images or data included in this article.
Author contributions
RS: Conceptualization, Methodology, Project administration, Writing–original draft, Writing–review and editing. MV: Formal Analysis, Investigation, Software, Writing–original draft. KW: Writing–original draft, Writing–review and editing. SM: Formal Analysis, Writing–review and editing. JK: Writing–review and editing. EA: Funding acquisition, Project administration, Resources, Writing–review and editing.
Funding
The author(s) declare financial support was received for the research, authorship, and/or publication of this article. The authors gratefully acknowledge funding of this work by the German VolkswagenStiftung under grant agreement number 98 560.
Conflict of interest
The authors declare that the research was conducted in the absence of any commercial or financial relationships that could be construed as a potential conflict of interest.
Publisher’s note
All claims expressed in this article are solely those of the authors and do not necessarily represent those of their affiliated organizations, or those of the publisher, the editors and the reviewers. Any product that may be evaluated in this article, or claim that may be made by its manufacturer, is not guaranteed or endorsed by the publisher.
Supplementary material
The Supplementary Material for this article can be found online at: https://www.frontiersin.org/articles/10.3389/frvir.2024.1340250/full#supplementary-material
Footnotes
1https://www.vive.com/accessory/tracker3/
2http://www.makehumancommunity.org
4https://assetstore.unity.com/packages/tools/animation/final-ik-14290
5https://github.com/Trusted-AI/AIF360/blob/master/examples/README.md
References
Ahn, S. J., Le, A. M. T., and Bailenson, J. (2013). The effect of embodied experiences on self-other merging, attitude, and helping behavior. Media Psychol. 16, 7–38. doi:10.1080/15213269.2012.755877
Anderson, A., Dodge, J., Sadarangani, A., Juozapaitis, Z., Newman, E., Irvine, J., et al. (2019). Explaining reinforcement learning to mere mortals: an empirical study. arXiv preprint arXiv:1903.09708.
Angwin, J., Larson, J., Mattu, S., and Kirchner, L. (2016). Machine bias: there’s software used across the country to predict future criminals. it’s biased against blacks. ProPublica 23, 77–91.
Bailenson, J. (2018). Experience on demand: what virtual reality is, how it works, and what it can do. WW Norton and Company.
Banakou, D., Hanumanthu, P. D., and Slater, M. (2016). Virtual embodiment of white people in a black virtual body leads to a sustained reduction in their implicit racial bias. Front. Hum. Neurosci. 10, 601. doi:10.3389/fnhum.2016.00601
Banakou, D., Kishore, S., and Slater, M. (2018). Virtually being einstein results in an improvement in cognitive task performance and a decrease in age bias. Front. Psychol. 9, 917. doi:10.3389/fpsyg.2018.00917
Bellamy, R. K., Dey, K., Hind, M., Hoffman, S. C., Houde, S., Kannan, K., et al. (2019). Ai fairness 360: an extensible toolkit for detecting and mitigating algorithmic bias. IBM J. Res. Dev. 63, 1–4. doi:10.1147/jrd.2019.2942287
Beltran, K., Rowland, C., Hashemi, N., Nguyen, A., Harrison, L., Engle, S., et al. (2021). “Reducing implicit gender bias using a virtual workplace environment,” in Extended abstracts of the 2021 CHI conference on human factors in computing systems, 1–7.
Buolamwini, J., and Gebru, T. (2018). “Gender shades: intersectional accuracy disparities in commercial gender classification,” in Conference on fairness, accountability and transparency (PMLR), 77–91.
Chen, V. H. H., Ibasco, G. C., Leow, V. J. X., and Jyy, L. (2021). The effect of vr avatar embodiment on improving attitudes and closeness toward immigrants. Front. Psychol. 12, 705574. doi:10.3389/fpsyg.2021.705574
Cohen, D., and Strayer, J. (1996). Empathy in conduct-disordered and comparison youth. Dev. Psychol. 32, 988–998. doi:10.1037//0012-1649.32.6.988
consortium, T. A. F. (2021). Ai fora: better-ai lab. Available at: https://www.ai-fora.de/better-ai-lab/(Accessed July 31, 2023).
Cresswell, K., Callaghan, M., Khan, S., Sheikh, Z., Mozaffar, H., and Sheikh, A. (2020). Investigating the use of data-driven artificial intelligence in computerised decision support systems for health and social care: a systematic review. Health Inf. J. 26, 2138–2147. doi:10.1177/1460458219900452
Dastin, J. (2022). Amazon scraps secret ai recruiting tool that showed bias against women. Ethics of data and analytics Auerbach Publications, 296–299.
e Estoniacom (2021). Ai to help serve the Estonian unemployed. Available at: https://e-estonia.com/ai-to-help-serve-the-estonian-unemployed/(Accessed July 31, 2023).
European Commission, (2020). White paper on artificial intelligence - a european approach to excellence and trust.
Fowler, Z., Law, K. F., and Gaesser, B. (2021). Against empathy bias: the moral value of equitable empathy. Psychol. Sci. 32, 766–779. doi:10.1177/0956797620979965
Hofmann, H. (1994). Statlog (German credit data). UCI Machine Learning Repository. doi:10.24432/C5NC77
Holm, S. (1979). A simple sequentially rejective multiple test procedure. Scand. J. statistics, 65–70.
Huber, T., Weitz, K., André, E., and Amir, O. (2021). Local and global explanations of agent behavior: integrating strategy summaries with saliency maps. Artif. Intell. 301, 103571. doi:10.1016/j.artint.2021.103571
Kuziemski, M., and Misuraca, G. (2020). Ai governance in the public sector: three tales from the frontiers of automated decision-making in democratic settings. Telecommun. policy 44, 101976. doi:10.1016/j.telpol.2020.101976
Levene, H. (1961). Robust tests for equality of variances. Contributions Probab. statistics. Essays honor Harold Hotelling, 279–292.
Mertes, S., Huber, T., Weitz, K., Heimerl, A., and André, E. (2022). Ganterfactual—counterfactual explanations for medical non-experts using generative adversarial learning. Front. Artif. Intell. 5, 825565. doi:10.3389/frai.2022.825565
Nelson, G. S. (2019). Bias in artificial intelligence. N. C. Med. J. 80, 220–222. doi:10.18043/ncm.80.4.220
Ntoutsi, E., Fafalios, P., Gadiraju, U., Iosifidis, V., Nejdl, W., Vidal, M. E., et al. (2020). Bias in data-driven artificial intelligence systems—an introductory survey. Wiley Interdiscip. Rev. Data Min. Knowl. Discov. 10, e1356. doi:10.1002/widm.1356
Peck, T. C., Seinfeld, S., Aglioti, S. M., and Slater, M. (2013). Putting yourself in the skin of a black avatar reduces implicit racial bias. Conscious. cognition 22, 779–787. doi:10.1016/j.concog.2013.04.016
Piumsomboon, T., Lee, Y., Lee, G. A., Dey, A., and Billinghurst, M. (2017). “Empathic mixed reality: sharing what you feel and interacting with what you see,” in 2017 international symposium on ubiquitous virtual reality (ISUVR) (IEEE), 38–41.
Rajpurkar, P., Chen, E., Banerjee, O., and Topol, E. J. (2022). Ai in health and medicine. Nat. Med. 28, 31–38. doi:10.1038/s41591-021-01614-0
Rosanvallon, P. (2014). The society of equals: restoring democratic equality in relations. Juncture 20, 249–257. doi:10.1111/j.2050-5876.2014.00762.x
Roselli, D., Matthews, J., and Talagala, N. (2019). “Managing bias in ai,” in Companion proceedings of the 2019 world wide web conference, 539–544.
Salmanowitz, N. (2016). Unconventional methods for a traditional setting: the use of virtual reality to reduce implicit racial bias in the courtroom. UNHL Rev. 15, 117.
Schulze, S., Pence, T., Irvine, N., and Guinn, C. (2019). “The effects of embodiment in virtual reality on implicit gender bias. Virtual, Augmented and Mixed Reality,” in Multimodal interaction: 11th international conference, VAMR 2019, held as part of the 21st HCI international conference, HCII 2019 (Orlando, FL, USA: Springer), 361–374. Proceedings, Part I 21.
Schutte, N. S., and Stilinović, E. J. (2017). Facilitating empathy through virtual reality. Motivation Emot. 41, 708–712. doi:10.1007/s11031-017-9641-7
Shapiro, S. S., and Wilk, M. B. (1965). An analysis of variance test for normality (complete samples). Biometrika 52, 591–611. doi:10.2307/2333709
Slater, M., and Sanchez-Vives, M. V. (2014). Transcending the self in immersive virtual reality. Computer 47, 24–30. doi:10.1109/mc.2014.198
Stavroulia, K. E., and Lanitis, A. (2023). The role of perspective-taking on empowering the empathetic behavior of educators in vr-based training sessions: an experimental evaluation. Comput. Educ. 197, 104739. doi:10.1016/j.compedu.2023.104739
Sun, T. Q., and Medaglia, R. (2019). Mapping the challenges of artificial intelligence in the public sector: evidence from public healthcare. Gov. Inf. Q. 36, 368–383. doi:10.1016/j.giq.2018.09.008
Troeger, J., and Tümler, J. (2020). Virtual reality zur steigerung empathischer anteilnahme. GI VR/AR Workshop.
Waltersmann, L., Kiemel, S., Stuhlsatz, J., Sauer, A., and Miehe, R. (2021). Artificial intelligence applications for increasing resource efficiency in manufacturing companies—a comprehensive review. Sustainability 13, 6689. doi:10.3390/su13126689
Weitz, K., Vanderlyn, L., Ngoc, T. V., and André, E. (2021). “It’s our fault!”: insights into users’ understanding and interaction with an explanatory collaborative dialog system,” in Proceedings of the 25th conference on computational natural language learning, CoNLL 2021. Editors A. Bisazza, and O. Abend (Association for Computational Linguistics), 1–16. Online, November 10-11, 2021. doi:10.18653/v1/2021.conll-1.1
Wilcoxon, F. (1992). Individual comparisons by ranking methods. in Breakthroughs in statistics. Springer, 196–202.
Yee, N., and Bailenson, J. N. (2007). The Proteus effect: the effect of transformed self-representation on behavior. Hum. Commun. Res. 33, 271–290. doi:10.1111/j.1468-2958.2007.00299.x
Keywords: virtual reality, virtual embodiment, AI bias, empathy, perspective-taking, empirical study
Citation: Schlagowski R, Volanti M, Weitz K, Mertes S, Kuch J and André E (2024) The feeling of being classified: raising empathy and awareness for AI bias through perspective-taking in VR. Front. Virtual Real. 5:1340250. doi: 10.3389/frvir.2024.1340250
Received: 17 November 2023; Accepted: 20 February 2024;
Published: 04 March 2024.
Edited by:
Thammathip Piumsomboon, University of Canterbury, New ZealandReviewed by:
Shuo Zhou, University of Colorado Anschutz Medical Campus, United StatesKangsoo Kim, University of Calgary, Canada
Copyright © 2024 Schlagowski, Volanti, Weitz, Mertes, Kuch and André. This is an open-access article distributed under the terms of the Creative Commons Attribution License (CC BY). The use, distribution or reproduction in other forums is permitted, provided the original author(s) and the copyright owner(s) are credited and that the original publication in this journal is cited, in accordance with accepted academic practice. No use, distribution or reproduction is permitted which does not comply with these terms.
*Correspondence: Ruben Schlagowski, cnViZW4uc2NobGFnb3dza2lAdW5pLWEuZGU=