- 1Cognitive Leap Solutions Inc., Irvine, CA, United States
- 2Institute for Creative Technologies, University of Southern California, Los Angeles, CA, United States
Introduction: Virtual Reality (VR) is revolutionizing healthcare research and practice by offering innovative methodologies across various clinical conditions. Advances in VR technology enable the creation of controllable, multisensory 3D environments, making it an appealing tool for capturing and quantifying behavior in realistic scenarios. This paper details the application of VR as a tool for neurocognitive evaluation, specifically in attention process assessment, an area of relevance for informing the diagnosis of childhood health conditions such as Attention Deficit Hyperactivity Disorder (ADHD).
Methods: The data presented focuses on attention performance results from a large sample (n = 837) of neurotypical male and female children (ages 6–13) tested on a visual continuous performance task, administered within an immersive VR classroom environment. This data was collected to create a normative baseline database for use to inform comparisons with the performances of children with ADHD to support diagnostic decision-making in this area.
Results: Results indicate systematic improvements on most metrics across the age span, and sex differences are noted on key variables thought to reflect differential measures of hyperactivity and inattention in children with ADHD. Results support VR technology as a safe and viable option for testing attention processes in children, under stimulus conditions that closely mimic ecologically relevant challenges found in everyday life.
Discussion: In response to these stimulus conditions, VR can support advanced methods for capturing and quantifying users’ behavioral responses. VR offers a more systematic and objective approach for clinical assessment and intervention and provides conceptual support for its use in a wide variety of healthcare contexts.
1 Introduction
Over the past quarter-century, researchers and clinicians have harnessed Virtual Reality (VR) technology to push the boundaries of clinical assessment, intervention, and scientific research. With VR’s powerful confluence of modern computing, 3D graphics, body tracking, advanced user interfaces, gaming technology, big data analytics, and artificial intelligence, we are witnessing the dawn of a new era in mental health, rehabilitation, and general medical practices. VR-based testing, training, and treatment approaches that would be difficult, if not impossible, to deliver using traditional methods have now been developed that take advantage of these unique VR enabling technologies. VR allows for the creation of 3D computer-generated immersive simulations of real-world contexts, within which human performance can be tested under very precisely controlled stimulus conditions that can closely mimic ecologically relevant challenges found in everyday life. In response to these stimulus conditions, VR can support advanced methods for capturing and quantifying users’ behavioral responses. This capacity to create functionally relevant, systematically controllable, multisensory, interactive 3D stimulus environments, within which human behavior can be motivated and measured, offers a more systematic and objective approach for clinical assessment and intervention.
Thus, users can be immersed in VR simulations and interact with virtual content for human performance measurement and training, and by extension for the clinical purposes of assessment and intervention. This “Ultimate Skinner Box” perspective makes VR technology well-matched to the requirements of various experimental and clinical objectives and this provides conceptual support for its use in a wide variety of healthcare contexts (Maples-Keller et al., 2017; Rizzo and Koenig, 2017; Birckhead et al.,. 2019; Hoffman et al., 2019; Powers and Rothbaum, 2019; Riva et al., 2019; Slater et al., 2019; Schweitzer and Rizzo, 2022; Lacy et al., 2023; Rizzo et al., 2023). At the same time, a growing body of research evidence is emerging that supports the safety and added value for using VR across a diverse range of clinical applications. Thus, VR applications are being increasingly regarded as an innovative and evidence-based option for addressing the cognitive, psychological, motor, and functional impairments that are seen across a diverse range of clinical health conditions (Carl et al., 2019; Difede et al., 2022; Blanco et al., 2023; Demeco et al., 2023; Kwon et al., 2023; Perra et al., 2023; Pira et al., 2023; Satu et al., 2023).
1.1 VR and attention-deficit/hyperactivity disorder (ADHD) assessment
One clinical application where VR technology is especially well-matched is in the assessment of attention processes, specifically relevant in the evaluation of Attention-Deficit/Hyperactivity Disorder (ADHD) and other neurocognitive clinical conditions. ADHD is a chronic condition that has a significant impact on the wellbeing and academic success of children and is one of the more commonly diagnosed neurodevelopmental disorders with reported prevalence rates of 5%–8% in school children (Gnanavel et al., 2019). The definition of ADHD has evolved over time. In 1987, the Diagnostic and Statistical Manual of Mental Disorders (DSM)-III-R established a specific diagnostic checklist and identified three subtypes: primarily inattentive, primarily hyperactive, and combined. According to the DSM-V (APA, 2013) to receive an ADHD diagnosis, a child must exhibit symptoms (hyperactivity, impulsivity, inattentiveness) before the age of 12, for a minimum of 6 months, have an impact on at least two areas of life (e.g., home, school, with friends or relatives, etc.), and there is clear evidence that the symptoms interfere with (or reduce quality of) social, academic or occupational functioning. ADHD is considered a “symptom complex”—a condition with a combination of symptoms that result from various factors, including genetics, biology, and psychosocial influences. These factors can lead to different manifestations of ADHD. Because of this, there exists no single specific test that can be used to diagnose ADHD, and the most accepted method of assessment is the clinical judgment of the healthcare provider integrating multiple streams of assessment data.
The current standard for diagnosing ADHD is a multifaceted process that includes clinical interviews, behavior rating scales, and psychometric and computerized tests. While each method of assessment can provide data to inform diagnostic decisions regarding the presence or absence of ADHD, each method alone cannot be relied upon to confirm its presence. For example, information from clinical histories and behavior rating scales can provide some initial signal as to the possible presence of ADHD. However, the quality of behavioral ratings and patient history data can be biased by the subjective judgment of the person doing the ratings, as well as with their varied familiarity with the child’s history (e.g., teacher vs. parent). Paper and pencil psychometric and computerized versions of similar tests offer assessment methods that provide consistent stimulus delivery and are backed by a long history of research on their use to measure cognitive function. Yet these tests often lack the ecological relevance of real-world contexts, which can result in a disconnection between a child’s performance on traditional assessments and their everyday functioning. VR can address the limitations of these traditional assessment methodologies by leveraging assets available through the use of simulation technology, where the precise titration of stimulus presentation and quantification of naturalistic behavioral responses is possible. When combining these assets within the context of ecologically relevant virtual environments, a fundamental advancement emerges in how human functioning can be assessed.
The use of VR to test attention processes under conditions that mimic real-life performance challenges, like in a classroom context, is believed to provide compelling assessment data to inform diagnostic decisions regarding ADHD. When the VR world is delivered in a Head Mounted Display (HMD), the user’s experience is constrained to the content presented with the headset, as their view of distracting stimuli existing in the actual physical test setting is occluded. Additionally, VR leverages sophisticated tracking technology to capture and quantify the users’ physical head movement to drive the real time updating of audio-visual content in the HMD. This is required to create an immersive virtual experience where the user sees and hears what they would experience if they were looking around in the physical world. However, this capability also supports the measurement of head movement and serves added value as a measure of the hyperactivity component of the ADHD condition. This capability also supports quantification of the users’ response to the systematic delivery of distracting stimuli to assess performance under varied conditions of cognitive load. Distraction via naturally occurring classroom activities is a common experience in an actual physical classroom, and “virtual” distractions can add to the value of a VR approach as a method for quantifying distractibility. This is a capability that is not possible to objectively quantify with traditional paper and pencil and non-immersive computerized attention testing methods.
In the current study, we present results from a large United States normative sample (n = 837) of the performance of male and female neurotypical children (n = 409 females), aged 6–13 tested in what is referred to as the Virtual Classroom Assessment Tracker (vCAT™). The vCAT is a 13-min continuous performance test of visual attention, administered within a VR HMD. This initial study was the first step required to determine the system’s reliability and validity so as to establish its value as a psychometrically sound attention process assessment system. Specifically, this effort to create a standardization sample serves to determine the system’s feasibility for use by children at this age, and to create neurotypical performance norms that can later be used for performance comparisons against children being assessed for ADHD and other neurocognitive clinical conditions. Performance results from this effort were also collected to investigate sex differences and changes in status across this developmental period in neurotypical children. The construction of the test stimuli and VR classroom context was informed by the many years of research conducted using a previous research version of the system (Virtual Classroom; Rizzo et al., 2000; Schweitzer and Rizzo, 2022). Results reported from use of the previous system are well documented (see Rizzo et al., 2000; Rizzo et al., 2006; Parsons et al., 2007; Adams et al., 2009; Pollak et al., 2009; Bioulac et al., 2012; Parsons and Rizzo, 2019; Bioulac et al., 2020; Mangalmurti et al., 2020; Mühlberger et al., 2020); along with results from similarly developed systems by others (Iriarte et al., 2016; Rodríguez et al., 2018). The core assessment metrics presented from this normative study include errors of omission (missed targets—proxy for inattentiveness) and commission (responses in the absence of a hit target—proxy for impulsivity), accuracy, reaction time (speed of processing), reaction time variability (consistency over time), d-prime (signal to noise differentiation), and metrics of global head movement obtained during the assessment (measurements of hyperactivity and distractibility). An optimal result from this study would be to see clear performance improvements on these metrics across the age span. Male/female performance differences were expected, but predicted to vary significantly across different performance metrics depending upon whether CPT cognitive or motion variables are being measured.
2 Materials and methods
2.1 Participants
All participants were recruited from advertisements through multiple channels (including but not limited to local community social network, Facebook, student group (sports, after school clubs), community library, local summer camp collaboration, etc.). Before participation, all recruited participants completed the inclusion and exclusion criteria survey and were subsequently selected and rejected based on the study’s inclusion criteria and exclusion criteria (Supplementary Tables S1, S2). All participants’ parents or guardians gave informed consent along with participants’ assent. Data was collected from seven clinical centers across the United States (see Supplementary Table S3). All testing procedures were carried out by staff trained by Cognitive Leap Solutions Inc. A total of 16 participants dropped out due to adverse events or because they were unwilling to finish the study (see Supplementary Table S4).
The normative sample was composed of 837 participants (48.9% female) with an age range from 6 to 13 years old (M = 9.83 years, SD = 2.19 years), after accounting for attrition and age outliers (<6 years and >13 years). The normative dataset was grouped into eight groups according to age and gender across the 6–13 age span. The eight groups consisted of: Male 6–7 years old, Male 8–9 years old, Male 10–11 years old, Male 12–13 years old, Female 6–7 years old, Female 8–9 years old, Female 10–11 years old, and Female 12–13 years old. The number of participants within each group can be found in Table 1.
2.2 Testing instrument (vCAT™)
All participants were administered the Virtual Classroom Assessment Tracker (vCAT™), a 13-min continuous performance test which uses an HMD (HTC Vive) to immerse users in a VR classroom to assess attention processes under various conditions of distraction. Participants were seated at a desk and a technician fitted the HMD to each child’s head before activating the system, which presented a virtual classroom environment. Within the HMD, participants were immersed in a simulation of a standard rectangular classroom featuring three rows of desks, a teacher’s desk and whiteboard at the front, a female virtual teacher positioned to the right of the whiteboard, a large window on the left side displaying a roadway with buildings and vehicles, and on the opposite wall another window and doorway by which walking people and other activities occurred (Figure 1). The technician guided the participant in a brief exploratory session, encouraging them to look around the virtual room and identify various objects until they reported being familiarized with the vCAT environment. This was followed by a brief practice session prior to commencing the actual testing session.
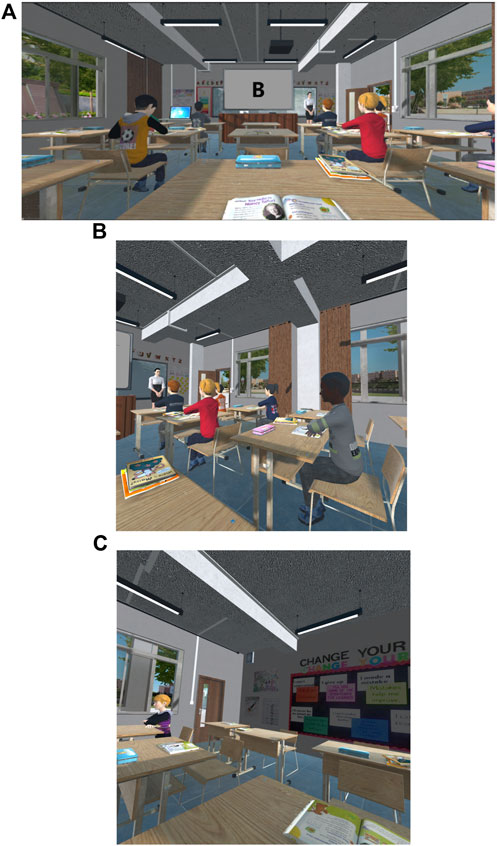
Figure 1. (A–C) The vCAT virtual classroom environment from the participant’s view of the front (A), right side (B) and back (C) of the classroom.
During the practice session, the virtual teacher then provided instructions to the participant directing them to watch a sequence of letters displayed on the virtual whiteboard and to press a response button as quickly and accurately as possible only when the letter “X” was preceded by the letter “A.” The practice session consisted of a sequence of 20 stimuli (letters A, B, C, D, E, F, G, H, J, L, and X), with 2 “A-X” targets and one “A-H-X” non-target sequence randomly assigned in the full practice sequence. Each letter appeared for 150 ms with an inter-stimulus interval of 1,350 ms. The participant was required to perform with 100% accuracy on the practice session before progressing to the actual testing session. If the participant failed to complete and pass the practice session three times, they were asked to remove the HMD and the test session was discontinued.
Following successful completion of the practice session, the participant was then guided into the real test session, which lasted for 13-min with no breaks. The stimuli presented included the letters A, B, C, D, E, F, G, H, J, L, and X. Stimulus letters appeared on the “virtual whiteboard” at the front of the classroom for a duration of 150 ms with an interstimulus interval of 1,350 ms. The entire testing session lasted 13 min and consisted of 520 stimuli. The target letter “X” (that follows a preceding “A”) occurred 52 times in total (10% of stimuli). The task stimuli were balanced across four blocks (13 targets per block).
During the CPT task, a variety of distractor stimuli possibly found in a real classroom were presented in the virtual classroom. These distractors were multi-sensory, including pure auditory (e.g., sounds of pencils dropping, footsteps), pure visual (e.g., a paper-plane flown across the classroom), and mixed (both auditory and visual), such as a bus or a group of students passing by the outside windows. Each distractor was visible for 5 s and occurred at randomly assigned intervals of 10, 15, or 25 s. In total, there were 36 distractors, with 12 different types presented three times each (see Supplementary Table S5).
Performance data was captured continuously across the 13-min virtual classroom task (detailed below) and distinctly quantified for each of the 4 defined blocks. By incorporating this form of attention processing assessment within an immersive virtual environment with dynamic stimulus delivery of distractions, the vCAT aims to assess attention and impulsivity under conditions that closely mimic real-life classroom settings. This is anticipated to provide more ecologically valid data regarding the attentional processes of children, particularly those with ADHD.
2.3 Key variables measured
Key variables measured include standard attention process performance metrics (e.g., speed, accuracy, consistency over time) obtained from the AX-CPT task, as well as head movement variables recorded via the HMD tracking system during test sessions. The following is a description of the different measures obtained with vCAT.
2.3.1 AX-CPT variables
2.3.1.1 Omission errors
Number of errors where the participant fails to respond to the target stimulus as required. Omission errors are indicative of challenges in selective and focused attention, and freedom from distractibility.
2.3.1.2 Commission errors
Number of errors where the participant makes a “hit” response in the absence of a target stimulus. These errors reflect deficiencies in motor control or response inhibition.
2.3.1.3 Accuracy (%)
Percentage of correct responses to both the targets and the non-targets, as a measure of general performance combining focused and sustained attention.
2.3.1.4 Latency (ms)
The average reaction time (ms) to correctly respond to a target, measured across all correct responses.
2.3.1.5 Variability of response time (ms)
This measure reflects the standard deviation of reaction times (ms) of correct hits, capturing the variability or inconsistency of responses, and may provide insight on vigilance across time as well.
2.3.1.6 D-prime
D-prime measures the participant’s ability to discriminate between signal and noise. It is calculated as a Z-score, providing a standardized metric for signal detection.
2.3.2 Motion signal variables
2.3.2.1 Immobility duration (ms)
The average time (ms) spent sitting still (not moving head more than 1 mm in virtual space) as a measure of physical stillness. The longer the Immobility Duration, the less movements the participant made during the 13-min test.
2.3.2.2 Movements
The number of head movements (more than 1 mm in virtual space) as a measure of the number of large magnitude movements. The number or count of movements indicates how frequently the participant is moving across the 13-min test.
2.3.2.3 Total displacement (cm)
The cumulative length (cm) that the head moved over the 13 min, as a measure of the total amount of movement that was made across the test period. A participant making large movements but infrequently will have a relatively smaller Total Displacement outcome. A participant making small to medium movements but frequently will end up with a relatively larger Total Displacement outcome.
2.3.2.4 Area (m2)
The total spatial area (expressed in m2 in 2D space) that is covered by the path of head movement, as a measure of the spatial range of movement. A larger number indicates the participant’s range of movement in space is bigger, and a smaller area indicates that the participant’s range of movement in space is smaller.
2.3.2.5 Temporal scaling
The proportion (0–1) of time spent moving (more than 1 mm in space) as a measure of frequency of movement. The higher the Temporal Scaling score, the more time was spent moving around during the 13-min test. The lower the Temporal scaling score, the less time was spent moving around.
2.3.2.6 Time at board (min)
Cumulative time (in minutes) spent facing towards the whiteboard and CPT task, as a measure of sustained attention and stillness. A higher score for Time At Board means the participant spent more time in minutes with their head facing directly towards the whiteboard. A lower score indicates the participant spent more time looking away from the board.
3 Results
All vCAT variables represent performance measured across the 13-min vCAT test.
3.1 Distribution and normality
Shapiro-Wilk tests were performed for each continuous variable to determine distribution normality. Results for all variables showed significant departure from normality (Supplementary Table S6). Non-parametric tests were used subsequently.
3.2 Age-group effects
To investigate the effect of age group in the normative dataset, Kruskal–Wallis tests were conducted for each of the vCAT measurement variables. Results indicate significant effect of age group for all variables [Omission Errors: H (3) = 230.34, p = 1.17e-49; Commission Errors: H (3) = 122.32, p = 2.44e-26; Accuracy: H (3) = 243.35, p = 1.79e-52; Latency: H (3) = 229.82, p = 1.51e-49; Variability: H (3) = 189.91, p = 6.40e-41; D-prime: H (3) = 225.04, p = 5.33e-55; Immobility Duration: H (3) = 76.84, p = 1.46e-16; Movements: H (3) = 76.15, p = 2.05e-16; Total Displacement: H (3) = 96.83, p = 7.47e-21; Area: H (3) = 42.36, p = 3.37e-09; Temporal Scaling: H (3) = 76.17, p = 2.04e-16; Time at Board: H (3) = 83.95, p = 4.36e-18].
Pairwise comparisons using Dunn’s post hoc test were performed to further delineate the group differences. Results show significant differences for all pairwise observations across all age groups for all vCAT measurement variables (see Supplementary Table S7), with the exception of non-significant group differences between the 10–11 and 12–13 age groups for the vCAT motion variables of Immobility Duration (p = 0.173), Movements (p = 0.127), Total Displacement (p = 0.071), Area (p = 0259), Temporal Scaling (p = 0.127) and Time at Board (p = 0.177) (see Figures 2A–F).
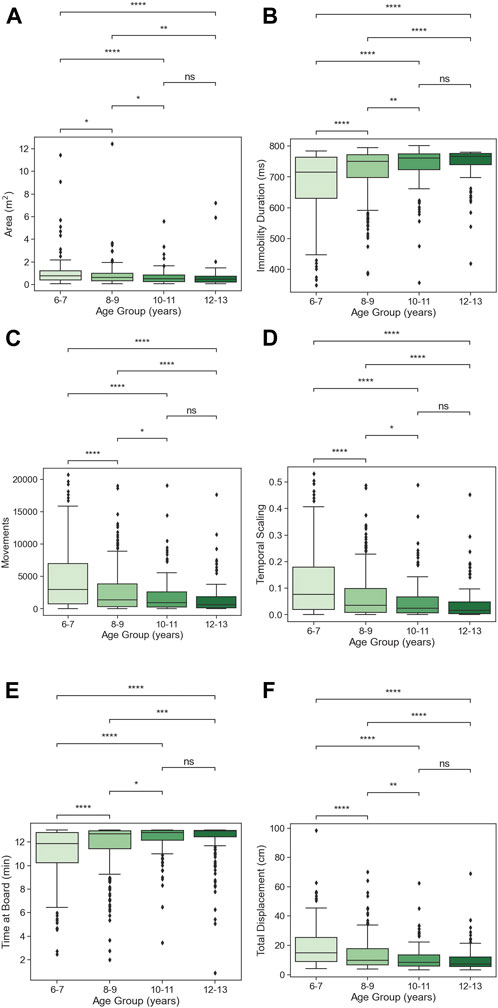
Figure 2. (A–F) Age-group effects, with Dunn’s post hoc pairwise comparisons for each vCAT motion variable. Pair-wise comparisons are non-significant between age groups 10–11 and 12–13, for all motion variables. (ns: p ≤ 1.00e+00; *: 1.00e-02 < p ≤ 5.00e-02; **: 1.00e-03 < p ≤ 1.00e-02; ***: 1.00e-04 < p ≤ 1.00e-03; ****: p ≤ 1.00e-04).
3.3 Sex differences
All vCAT measurement variables show trends of sex differences, where differences are observed in the same direction in each age group within the same vCAT measurement variable. To test the effect of sex on vCAT performance, two sided Mann-Whitney-Wilcoxon tests with Bonferroni corrections were performed for each age-group for each variable measured by vCAT.
Significant group differences in sex were found in the following variables: For Commission Errors, male participants made more errors than female participants in both the 6–7 age group (U = 6225, p = 0.027) and 8–9 age group (U = 1070, p = 0.008). For Latency, sex differences were observed for all but the 12–13 age groups, where male participants responded significantly faster than female participants (6–7 age group: U = 3842, p = 0.010; 8–9 age group: U = 6157, p = 0.0002; 10–11 age group: U = 4146, p = 0.004). For Immobility Duration, male participants maintained immobility for a significantly shorter duration compared to female participants in the 8-9 age group (U = 7216, p = 0.049) and 12–13 age group (U = 2363, p = 0.048). For Movements, male participants made significantly more movements than female participants in the 6–7 age group only (U = 6176, p = 0.038). For Total Displacement, male participants moved a significantly greater distance cumulatively compared to female participants in the 6–7 age group (U = 6281, p = 0.018) and the 8–9 age group (U = 1049, p = 0.025). For Area, male participants moved a significantly greater total area than female participants only in the 6–7 age group (U = 6415, p = 0.006). For Temporal Scaling, male participants moved significantly more frequently than females in the 6–7 age group only (U = 6176, p = 0.038).
3.4 Assessing performance over time
3.4.1 Distribution and normality per block
Shapiro-Wilk tests were performed for each variable per block to determine distribution normality across time. For all blocks, results for all variables showed significant departure from normality (see Supplementary Table S6). Non-parametric tests were used subsequently taking into consideration the non-normal distribution of all blocks of the motion and CPT variables.
3.4.2 Block effects
The effect of block as an indication of performance over time was calculated using a Friedman Test as a one-way repeated measures analysis of variance by ranks. Results indicate that there was no significant main effect of block for all CPT measures. However, there were significant differences in performance across time for all the motion variables. Results showed highly significant differences between blocks for the variables Area [Q (3) = 715.66, p = 8.44e-155], Immobility Duration [Q (3) = 729.37, p = 9.00e-158], Movements [Q (3) = 858.72, p = 7.95e-186], Temporal Scaling [Q (3) = 850.63, p = 4.52e-184], Time At Board [Q (3) = 498.45, p = 1.03e-107], and Total Displacement [Q (3) = 994.87, p = 2.33e-215].
Post-hoc analyses were performed using the Nemenyi post hoc test with Holm correction. Results indicate that for all motion variables, all pairwise contrasts were significantly different (see Supplementary Table S8) except for between the age groups 10–11 and 12–13 for the variable Time at Board (p = 0.30).
3.4.3 Block by age-group interaction
To examine the effect of age-group on performance over time, a two-way mixed ANOVA was performed to assess interaction between Block and Age-group. Results show significant Age Group by Block interactions for the variables Omission Errors [F (9, 2454) = 4.35, p = 1.21e-05], D Prime [F (9, 2454) = 3.06, p = 1.21e-03], Immobility Duration [F (9, 2493) = 8.48, p = 1.37e-12], Total Displacement [F (9, 2493) = 4.42, p = 9.33e-06], Movements [F (9, 2493) = 8.55, p = 1.03e-12] and Time At Board [F (9, 2493) = 3.79, p = 9.20e-05].
3.5 Construct validity
Construct validity was examined using principal component analysis (PCA) and exploratory factor analysis (EFA). Items with a loading of ≥.4 (16% of variance) were retained.
3.5.1 Correlation, sphericity, and sampling adequacy
Spearman rank correlations were computed to assess the relationship between all variables. When examining performance collapsed across four blocks, there were significant correlations between all variables except for the relationship between Latency and Commission Errors (see Figure 3), indicating that the data were likely to be factorable.
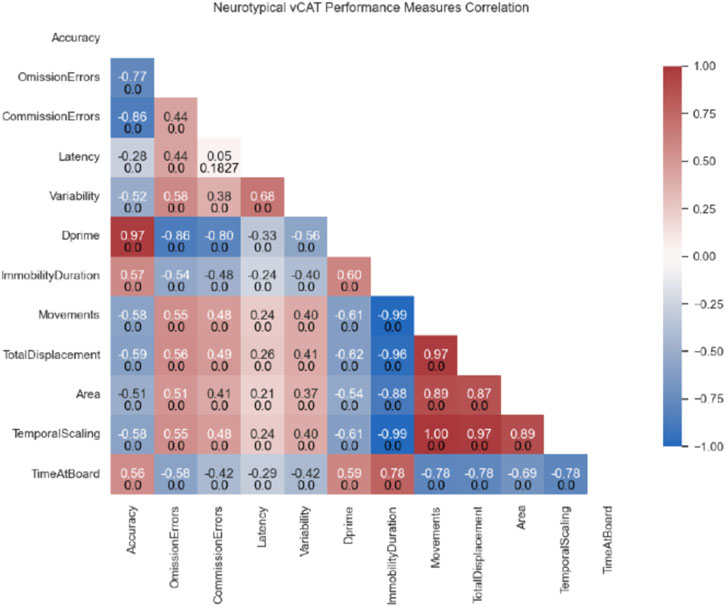
Figure 3. Spearman correlation tests were used to evaluate possible association between the different variables. Values indicate the correlation coefficient R (top) and corresponding p-value (bottom).
To further determine if the data were adequate for factor analysis, Bartlett’s test of sphericity was conducted to determine if the observed measurements intercorrelate, using the observed correlation matrix against the identity matrix. The Bartlett’s sphericity test yielded a statistically significant result [χ2 (11, N = 837) = 12,148.25, p = 2.91e-86], confirming the suitability of the correlation matrix for factor analysis and rejecting the null hypothesis. The Kaiser-Meyer-Olkin (KMO) measure of sampling adequacy was also calculated with a KMO index of 0.79, exceeding the recommended value of .6 (Kaiser, 1974), also supporting the factorability of the correlation matrix.
3.5.2 Principal component analysis and exploratory factor analysis
The number of components to be retained was determined using Kaiser’s criterion (eigenvalue ≥1) and using scree plot inspection (Cattell’s scree test) (Cattell, 1966). PCA identified three components with eigenvalues of ≥1.0 (Supplementary Table S9), suggesting that vCAT measurement variables may be divided into three components. In the subsequent factor analysis, orthogonal rotation (varimax) was used to further delineate the three components, with the assumption of independence between the variances explained by each factor. Factor rotation therefore increases the interpretability of the components.
Rotated component matrix sorted twelve variables into three groups, and items with a loading of ≥.4 (16% of variance) were retained. There were no blanks in the matrix where all weights were more than 0.5 (Supplementary Table S10). The factor column represents the rotated factors that were extracted out of the total factor.
Six items loaded onto component 1, containing all motion variables derived from the HMD motion signals (Immobility Duration, Total Displacement, Area, Temporal Scaling, Time At Board, Movements), and explaining 57.27% of the variability in the performance of vCAT. Component 1 was subsequently labeled Hyperactivity.
Three items loaded onto component 2, consisting of the CPT variables of Accuracy, Commission Errors and D-prime, and explained 14.79% of variance from PCA. Component 2 was subsequently labeled Impulsivity.
Four items loaded onto the component 3, consisting of the CPT variables Omission Errors, Latency, Variability and Dprime, which explained 10.92% of the variance, and component 3 was subsequently labeled Inattention (see Supplementary Table S9).
4 Discussion
The integration of VR with the neurocognitive assessment process represents a fusion of technology and human experience that holds significant potential for improving the quantity, quality, and relevance of data collected to inform diagnostic decision making. Within the realm of special education and cognitive research, VR presents an innovative platform for testing and observation. The virtual reality environment of the classroom offers a controlled yet immersive setting, which arguably mimics real-life scenarios more effectively than traditional 2-dimensional testing approaches. Such virtual settings might engage the participants’ attention and cognitive faculties more holistically, leading to a more accurate representation of their capabilities (Parsons, 2015). Additionally, VR’s capacity for dynamic multi-sensory stimulus presentations that can mimic naturalistic distractions found in a typical classroom setting, can afford the opportunity to assess their impact on attentional focus and distractibility as measured by movement tracking in VR. Moreover, the standard baseline data that this normative database provides will support comparative analyses with children suspected of having ADHD or other childhood neurocognitive conditions.
The current study utilized a VR classroom to administer a continuous performance cognitive test with 837 male and female neurotypical children, aged 6–13 years old. The results from this normative test clearly document the evolution of attention performance across this developmental age span and have also highlighted sex differences in different aspects of performance.
4.1 Improvement in inattention and hyperactivity-related measures with increasing age
Across the age span of 6–13, quantifiable improvements in attention process performance were observed on both the cognitive and motion-tracked components of the vCAT test. Results indicate significant effect of age group for all performance metrics (e.g., Omission Errors, Commission Errors, Accuracy, Latency, Variability, D-prime, Immobility Duration, Movements, Total Displacement, Area of movement, Temporal Scaling, and Time at Board). This aggregate collection of results corroborates the well-documented notion of neurotypical cognitive development advancing with age during this critical period (Lillas and Turnbull, 2009).
The improvement in attention and hyperactivity-inhibition in children with age is a complex process influenced by various factors, including cortical development, cognitive maturation, social experiences, and environmental influences. The prefrontal cortex, associated with executive function, working memory, cognitive flexibility, impulse control, attention, and decision-making, becomes more efficient during development, leading to improved impulse control and attentional abilities (Best and Miller, 2010; Shaw et al., 2012). With age, neural pathways related to attention and impulse control also become more efficient at allocating attention resources and suppressing irrelevant information, leading to improved focus and inhibition (Luna and Sweeney, 2004). External factors, like regular attendance at school, help hone executive function skills daily. Social interactions with peers and adults also reinforce appropriate behavior and impulse control, aiding the development of self-regulation and enhancing these skills over time (Brocki and Bohlin, 2004).
4.2 Age-related ceiling effects in hyperactivity measures
A striking result emerging from age-related improvements in inattention and hyperactivity-related performance is the ceiling effect observed in only hyperactivity measures, which occurs between ages 11 and 12. This developmental age coincides with the onset of puberty and the transition from elementary to middle school for some children. The ceiling effect observed in our normative dataset may represent the inability to further increase one’s physical impulse control or stability while engaged in an executive function task. This may be due to a wide range of reasons, from hormonal and neurophysiological changes to environmental and socioemotional factors.
During puberty, which can typically take place around 11–12 years old, significant changes in the brain’s catecholamine system occur. Catecholamines, neurotransmitters that include dopamine, norepinephrine, and epinephrine, play essential roles in regulating mood, attention, and other cognitive functions. Dopamine pathways in the brain, involved in reward and motivation, undergo significant increase during puberty (Laube et al., 2020), whilst norepinephrine receptor sensitivities also change and affect how adolescents respond to stress and emotional stimuli. These developmental changes in dopamine neurotransmission during puberty can have significant implications for ADHD symptoms, especially hyperactivity. As dopamine levels increase during puberty, there is a greater difficulty in regulating impulses and maintaining attention, which can translate into frequent and uncontrolled locomotor activity, and impulsive or novelty-seeking actions in pursuit of immediate gratification in children diagnosed with ADHD.
4.3 Sex differences in inattention and hyperactivity related measurements
Of interest were the sex differences in neurotypical children revealed in the current study. Male participants, particularly at younger ages, demonstrated a higher degree of physical activity within the virtual environment compared to their female counterparts, as captured by the sex difference in performance in hyperactivity-related measures. This was especially pronounced for the age 6–7 group. In this age group, males displayed more frequent movements measured by the Immobility Duration and Movement variables and produced greater total movement distance (i.e., Total Displacement and Area of Movement). This observation dovetails with past research highlighting the general propensity for males to be more kinesthetically active (Brand et al., 2002). In the current study, younger males were observed to produce faster reaction times compared to females (age groups 6–7 and 8–9) while also displaying more impulsive responding as reflected in the total number of commission errors.
Previous research suggests that there are developmental sex differences in terms of cortical development related to motor control, impulse control, and hyperactivity inhibition (Davies, 2014; Slobodin and Davidovitch, 2019), with preliminary research pointing towards genetic mechanisms contributing to the observed sex differences in hyperactivity (Tartaglia et al., 2012). For example, females often demonstrate slightly better fine motor skills at younger ages (Matarma et al., 2020). Fine motor skills involve the coordination of small muscles in movements, such as grasping small objects, and females may show earlier development in tasks requiring precision movement control, such as maintaining stillness of the head and neck positions in the vCAT test. Males of comparative age, on the other hand, may tend to excel in gross motor skills, which involve larger muscle groups and coordination for activities like running and jumping (Kokštejn et al., 2017). These differences are often attributed to both biological and social factors.
Past studies have also suggested that females might develop impulse control skills earlier than males, particularly in social contexts (Piek et al., 2006). This early development of impulse control might contribute to differences in behavior observed in various situations, including those observed in the current study. Females also often show slightly more advanced emotional regulation skills, as well as social skills such as empathy and communication, at younger ages than compared to their male counterparts (Zahn-Waxler et al., 2006; 2008), which can contribute to differences in social interactions and emotional processing. It is important to note that females’ observed ability to better stop themselves from acting impulsively at a young age may on the one hand be related to their ability to better regulate behavior and emotions, while on the other hand may be related to gender biases in behavioral expectations of obedience and compliance implicitly placed upon young females (Leaper and Brown, 2014; Leaper et al., 2002; Solbes-Canales et al., 2020). These factors may together contribute to female children exhibiting more advanced inhibitory control compared to male children in the current study (Ribeiro et al., 2021).
Although previous research examining sex differences in children with ADHD has explored potential differences in cortical functioning between males and females, individual studies have yielded varying results and existing biases may contribute to the research and interpretation narratives (Waddell and McCarthy, 2010). In children, males have historically been more frequently diagnosed with ADHD than females, having led to gender biases in ADHD research, with more studies involving males (Mowlem et al., 2019). For example, this concern has been cited many years ago in a U.S. National Institutes of Health ADHD Consensus report (NIH, 1998) that recommended more effort was needed in assessing the inattentive subtype, particularly since it may comprise a higher proportion of young females than the other subtypes. This underscores an area where social expectations for classroom behavior may result in biased behavioral observations that affect diagnostic accuracy and ultimately limit females’ access to appropriate clinical services. A VR approach in this area would be well suited to address this question via the differentiation between the attentional and movement variables. This lends support for the rationale underlying our ongoing efforts to conduct a vCAT study with children having well documented ADHD diagnoses for comparison research using the neurotypical normative data generated in the current study.
4.4 Differentiation between cognitive and motor measurements of inattention, impulsivity and hyperactivity
The above results from neurotypical behavior as measured by the vCAT demonstrates conspicuous disparities across multiple dimensions between the variables associated with motor functions and those related to cognitive processes when it comes to measures of attention. This differentiation between cognitive versus motor measurements of attention are further supported by two different aspects of results revealed in the current study. First, results showed significant decay in performance over time during vCAT testing, only for measures of hyperactivity, but not for inattention. Second, results from the exploratory factor analysis, where vCAT variables representing aspects of hyperactivity, inattention, and impulsivity, loaded distinctly into these identified factors. This pattern of results highlights clear differentiation in hyperactivity-related motion variables compared to the cognitive measurements derived from the CPT task. This is in line with the many levels of observations (genetic, neurophysiological, behavioral, and clinical) that point to the distinct demarcation in mechanisms underlying inattention and hyperactivity in both neurotypical and ADHD populations (Cai et al., 2021).
One of the most well-studied neural systems underlying inattention and hyperactivity is the fronto-striatal dopaminergic circuit, which is involved in executive functions such as attention, working memory, and inhibitory control (Durston, 2003; Castellanos and Proal, 2012; Mills et al., 2012; Vaidya, 2012). Research has shown that children with ADHD have decreased activity and connectivity in this circuit, particularly between the prefrontal cortex and basal ganglia (Leisman and Melillo, 2013; Riva et al., 2018). These regions are important for maintaining cognitive control over physical behavior; in particular, the basal ganglia is involved in reward processing, motivation and motor activity, and is critical for the regulation of executive functions. Dopaminergic pathways between the basal ganglia’s striatum and the thalamus are thought to be compromised in ADHD pathology (Leisman et al., 2014), leading to an imbalance between the direct and indirect thalamic pathways, and a reduced ability to control impulses and maintain attention, as well as impaired psychomotor control.
The differentiation between inattention and hyperactivity due to underlying mechanisms is further delineated in computational models of psychiatric neural dynamics (Hauser et al., 2016). ADHD and key symptoms of inattention and hyperactivity may be characterized by low neural gain (the ability of neural circuits to amplify or attenuate incoming signals), specifically in cortico-striatal loops influenced by catecholamine modulation. This causes an inability to differentiate among competing stimuli on a cortical signaling level, leading to behavioral instability and variability. Inattention is described as frequent shifting between different goals and an inability to focus on the most valuable option. Alternatively, neural gain impairments might occur only at a motor level, resulting in increased, undifferentiated motor actions and an inability to suppress inappropriate motor responses without marked inattentive symptoms, defining a hyperactive-impulsive subtype of ADHD.
5 Conclusion
The merger of Virtual Reality with neurocognitive assessment offers a promising future for enhancing diagnostic decision-making and treatment progress by collecting more abundant, high-quality, and relevant data. VR, especially within the domains of special education and cognitive research, emerges as a cutting-edge platform for testing and observation within precisely controlled, ecologically relevant simulated contexts. Testing administered in VR contexts can closely mimic the demands of real-world scenarios better than 2D methods and could possibly result in assessment outcomes that provide more accurate representations of participants’ abilities. The present study employed a VR classroom to conduct a continuous performance cognitive attention test on a large sample of neurotypical male and female children between the ages of 6–13. This vast dataset helped chart the progression of neurotypical attention performance through these developmental years and highlighted gender-specific performance variances. This effort also resulted in a normative database that will be used to support comparison analyses with children being assessed for ADHD or other neurocognitive conditions.
This research produced some key findings. Results indicated age-wise improvement in attention and hyperactivity. Both cognitive and motion-tracked elements of the vCAT test exhibited improvements in attention process performance from ages 6 to 13. This aligns with the well-known trajectory of neurotypical cognitive development. Factors like brain maturation, cognitive growth, social experiences, and environmental interactions influence attention and impulse control progression. With age, neural networks associated with these faculties become more adept at directing attention and filtering out irrelevant stimuli.
Hyperactivity ceiling effects were also observed. An interesting age-related trend is the ceiling effect seen in only hyperactivity measures, notably between the ages of 11 and 12. This period typically coincides with puberty and brings about significant brain changes, especially in dopamine levels, that can escalate ADHD symptoms in children.
The study also found distinct differences between male and female participants. Younger males showcased more physical activity in the VR setting and responded more impulsively than females. In contrast, females might develop impulse control abilities sooner than males, possibly due to biological and societal reasons. These findings prompt a re-evaluation of behavioral expectations and potential biases affecting diagnostic precision, especially in ADHD research where males have been historically overrepresented.
There was a clear differentiation between cognitive and motor measurements. The results underline the distinct separation between variables tied to motor functions versus those linked to cognitive processes concerning attention metrics. The evidence points towards distinct mechanisms underpinning inattention and hyperactivity, as cited in past research, and further substantiated by the results observed in the vCAT performances of these neurotypical children.
Future directions in fusing VR with neurocognitive testing would benefit from the inclusion of biological markers such as gaze fixation through eye-tracking, stress response through heart-rate and respiratory-rate derived metrics for comprehensive profiling. Combination with neurophysiological measures can also help to explain the underlying cortical mechanisms giving rise to the observed results in the current study. Calculation of a wider set of variables from the current task, such as distractor-related time-specific effects and how they are related to movement variables, can also increase understanding of group or individual differences in distractor response, which may lend precision to methods that ultimately can help reduce distraction. It will be important to compare the performance on the vCAT by age-matched children diagnosed with ADHD, as well as other neurocognitive disorders, as a means of pinpointing the potential pathological differences in cognition and psychomotor function, as well as how these differences vary according to age and sex. Finally, the demonstration of VR as a tool that sensitively detects and reflects differential underlying mechanisms of cognition and psychomotor function in neurotypical children serves as a prototypical doorway for VR to be incorporated more broadly into clinical diagnostic and therapeutic decision making processes.
In essence, VR’s integration with neurocognitive assessment opens new avenues for a deeper, more nuanced understanding of cognitive development and offers invaluable insights into conditions like ADHD. The technology holds the potential to reshape the landscape of cognitive testing, in a fashion that supports both the experimental precision and contextual relevance of neurocognitive assessment data. From a clinical perspective, these advances are anticipated to enhance diagnostic decision-making and provide a more objective method for documenting treatment response to interventions.
Data availability statement
The original contributions presented in the study are included in the article/Supplementary Material, further inquiries can be directed to the corresponding author.
Ethics statement
The studies involving humans were approved by the Advarra Global Institutional Review Board. The studies were conducted in accordance with the local legislation and institutional requirements. Written informed consent for participation in this study was provided by the participants’ legal guardians/next of kin.
Author contributions
CG: Conceptualization, Methodology, Writing–original draft, Writing–review and editing, Data curation, Formal Analysis, Project administration, Software. MY: Conceptualization, Data curation, Formal Analysis, Project administration, Software, Writing–original draft, Investigation. AR: Conceptualization, Investigation, Writing–original draft, Methodology, Supervision, Writing–review and editing.
Funding
The author(s) declare that no financial support was received for the research, authorship, and/or publication of this article.
Conflict of interest
Authors CG, MY, and AR were employed by Cognitive Leap Solutions Inc.
The author(s) declared that they were an editorial board member of Frontiers, at the time of submission. This had no impact on the peer review process and the final decision.
Publisher’s note
All claims expressed in this article are solely those of the authors and do not necessarily represent those of their affiliated organizations, or those of the publisher, the editors and the reviewers. Any product that may be evaluated in this article, or claim that may be made by its manufacturer, is not guaranteed or endorsed by the publisher.
Supplementary material
The Supplementary Material for this article can be found online at: https://www.frontiersin.org/articles/10.3389/frvir.2024.1309176/full#supplementary-material
References
Adams, R., Finn, P., Moes, E., Flannery, K., and Rizzo, A. S. (2009). Distractibility in attention/deficit/hyperactivity disorder (ADHD): the virtual reality classroom. Child neuropsychology a J. normal Abnorm. Dev. Child. Adolesc. 15 (2), 120–135. doi:10.1080/09297040802169077
American Psychiatric Association. (2013). Diagnostic and statistical manual of mental disorders (5th ed). doi:10.1176/appi.books.9780890425596
Best, J. R., and Miller, P. H. (2010). A developmental perspective on executive function. Child. Dev. 81 (6), 1641–1660. doi:10.1111/j.1467-8624.2010.01499.x
Bioulac, S., Lallemand, S., Rizzo, A., Philip, P., Fabrigoule, C., and Bouvard, M. P. (2012). Impact of time on task on ADHD patient’s performances in a virtual classroom. EJPN official J. Eur. Paediatr. Neurology Soc. 16 (5), 514–521. doi:10.1016/j.ejpn.2012.01.006
Bioulac, S., Micoulaud-Franchi, J. A., Maire, J., Bouvard, M. P., Rizzo, A. A., Sagaspe, P., et al. (2020). Virtual remediation versus methylphenidate to improve distractibility in children with ADHD: a controlled randomized clinical trial study. J. Atten. Disord. 24 (2), 326–335. doi:10.1177/1087054718759751
Birckhead, B., Khalil, C., Liu, X., Conovitz, S., Rizzo, A., Danovitch, I., et al. (2019). Recommendations for methodology of virtual reality clinical trials in health care by an international working group: iterative study. JMIR Ment. health 6 (1), e11973. doi:10.2196/11973
Blanco, D., Roberts, R. M., Gannoni, A., and Cook, S. (2023). Assessment and treatment of mental health conditions in children and adolescents: a systematic scoping review of how virtual reality environments have been used. Clin. child Psychol. psychiatry, 13591045231204082. doi:10.1177/13591045231204082
Brand, S., Dunn, R., and Greb, F. (2002). Learning styles of students with attention deficit hyperactivity disorder: who are they and how can we teach them? Clear. House 75 (5), 268–273. doi:10.1080/00098650209603953
Brocki, K. C., and Bohlin, G. (2004). Executive functions in children aged 6 to 13: a dimensional and developmental study. Dev. Neuropsychol. 26 (2), 571–593. doi:10.1207/s15326942dn2602_3
Cai, Y., Xing, L., Yang, T., Chai, R., Wang, J., Bao, J., et al. (2021). The neurodevelopmental role of dopaminergic signaling in neurological disorders. Neurosci. Lett. 741, 135540. doi:10.1016/j.neulet.2020.135540
Carl, E., Stein, A. T., Levihn-Coon, A., Pogue, J. R., Rothbaum, B., Emmelkamp, P., et al. (2019). Virtual reality exposure therapy for anxiety and related disorders: a meta-analysis of randomized controlled trials. J. anxiety Disord. 61, 27–36. doi:10.1016/j.janxdis.2018.08.003
Castellanos, F. X., and Proal, E. (2012). Large-scale brain systems in ADHD: beyond the prefrontal-striatal model. Trends cognitive Sci. 16 (1), 17–26. doi:10.1016/j.tics.2011.11.007
Cattell, R. B. (1966). The scree test for the number of factors. Multivar. Behav. Res. 1 (2), 245–276. doi:10.1207/s15327906mbr0102_10
Davies, W. (2014). Sex differences in attention deficit hyperactivity disorder: candidate genetic and endocrine mechanisms. Front. Neuroendocrinol. 35 (3), 331–346. doi:10.1016/j.yfrne.2014.03.003
Demeco, A., Zola, L., Frizziero, A., Martini, C., Palumbo, A., Foresti, R., et al. (2023). Immersive virtual reality in post-stroke rehabilitation: a systematic review. Sensors Basel, Switz. 23 (3), 1712. doi:10.3390/s23031712
Difede, J., Rothbaum, B. O., Rizzo, A. A., Wyka, K., Spielman, L., Reist, C., et al. (2022). Enhancing exposure therapy for posttraumatic stress disorder: virtual reality and imaginal exposure with a cognitive enhancer: a randomized clinical trial. Transl. Psychiatry 12 (1), 1–9. doi:10.1038/s41398-022-02066-x
Durston, S. (2003). A review of the biological bases of ADHD: what have we learned from imaging studies? Ment. Retard. Dev. Disabil. Res. Rev. 9 (3), 184–195. doi:10.1002/mrdd.10079
Gnanavel, S., Sharma, P., Kaushal, P., and Hussain, S. (2019). Attention deficit hyperactivity disorder and comorbidity: a review of literature. World J. Clin. cases 7 (17), 2420–2426. doi:10.12998/wjcc.v7.i17.2420
Hauser, T. U., Fiore, V. G., Moutoussis, M., and Dolan, R. J. (2016). Computational psychiatry of ADHD: neural gain impairments across marrian levels of analysis. Trends Neurosci. 39 (2), 63–73. doi:10.1016/j.tins.2015.12.009
Hoffman, H. G., Meyer, W. J., Drever, S. A., Soltani, M., Atzori, B., Herrero, R., et al. (2019). “Virtual reality distraction to help control acute pain during medical procedures,” in Virtual reality for psychological and neurocognitive interventions. Virtual reality Technologies for Health and clinical applications. Editors A. Rizzo, and S. Bouchard (New York, NY: Springer), 195–208.
Iriarte, Y., Diaz-Orueta, U., Cueto, E., Irazustabarrena, P., Banterla, F., and Climent, G. (2016). AULA-advanced virtual reality tool for the assessment of attention: normative study in Spain. J. Atten. Disord. 20 (6), 542–568. doi:10.1177/1087054712465335
Kaiser, H. F. (1974). An index of factorial simplicity. Psychometrika. 39, 31–36. doi:10.1007/BF02291575
Kokštejn, J., Musálek, M., and Tufano, J. J. (2017). Are sex differences in fundamental motor skills uniform throughout the entire preschool period? PloS one 12 (4), e0176556. doi:10.1371/journal.pone.0176556
Kwon, S. H., Park, J. K., and Koh, Y. H. (2023). A systematic review and meta-analysis on the effect of virtual reality-based rehabilitation for people with Parkinson's disease. J. neuroengineering rehabilitation 20 (1), 94. doi:10.1186/s12984-023-01219-3
Lacy, B. E., Cangemi, D. J., and Spiegel, B. R. (2023). Virtual reality: a new treatment paradigm for disorders of gut-brain interaction? Gastroenterology hepatology 19 (2), 86–94.
Laube, C., Bos, W. van den, and Fandakova, Y. (2020). The relationship between pubertal hormones and brain plasticity: implications for cognitive training in adolescence. Dev. Cogn. Neurosci. 42, 100753. doi:10.1016/j.dcn.2020.100753
Leaper, C., Breed, L., Hoffman, L., and Perlman, C. A. (2002). Variations in the gender-stereotyped content of children’s television cartoons across genres. J. Appl. Soc. Psychol. 32 (8), 1653–1662. doi:10.1111/j.1559-1816.2002.tb02767.x
Leaper, C., and Brown, C. S. (2014). Sexism in schools. Adv. Child Dev. Behav. 47, 189–223. doi:10.1016/bs.acdb.2014.04.001
Leisman, G., Braun-Benjamin, O., and Melillo, R. (2014). Cognitive-motor interactions of the basal ganglia in development. Front. Syst. Neurosci. 8, 16. doi:10.3389/fnsys.2014.00016
Leisman, G., and Melillo, R. (2013). The basal ganglia: motor and cognitive relationships in a clinical neurobehavioral context. Rev. Neurosci. 24 (1), 9–25. doi:10.1515/revneuro-2012-0067
Lillas, C., and Turnbull, J. (2009). Infant/child mental health, early intervention, and relationship-based therapies: a neurorelational framework for interdisciplinary practice. China: W. W. Norton and Company.
Luna, B., and Sweeney, J. A. (2004). The emergence of collaborative brain function: FMRI studies of the development of response inhibition. Ann. N. Y. Acad. Sci. 1021, 296–309. doi:10.1196/annals.1308.035
Mangalmurti, A., Kistler, W. D., Quarrie, B., Sharp, W., Persky, S., and Shaw, P. (2020). Using virtual reality to define the mechanisms linking symptoms with cognitive deficits in attention deficit hyperactivity disorder. Sci. Rep. 10 (1), 529. doi:10.1038/s41598-019-56936-4
Maples-Keller, J. L., Yasinski, C., Manjin, N., and Rothbaum, B. O. (2017). Virtual reality-enhanced extinction of phobias and post-traumatic stress. J. Am. Soc. Exp. Neurother. 14 (3), 554–563. doi:10.1007/s13311-017-0534-y
Matarma, T., Lagström, H., Löyttyniemi, E., and Koski, P. (2020). Motor skills of 5-year-old children: gender differences and activity and family correlates. Percept. Mot. Ski. 127 (2), 367–385. doi:10.1177/0031512519900732
Mills, K. L., Bathula, D., Dias, T. G., Iyer, S. P., Fenesy, M. C., Musser, E. D., et al. (2012). Altered cortico-striatal-thalamic connectivity in relation to spatial working memory capacity in children with ADHD. Front. psychiatry 3, 2. doi:10.3389/fpsyt.2012.00002
Mowlem, F., Agnew-Blais, J., Taylor, E., and Asherson, P. (2019). Do different factors influence whether girls versus boys meet ADHD diagnostic criteria? Sex differences among children with high ADHD symptoms. Psychiatry Res. 272, 765–773. doi:10.1016/j.psychres.2018.12.128
Mühlberger, A., Jekel, K., Probst, T., Schecklmann, M., Conzelmann, A., Andreatta, M., et al. (2020). The influence of methylphenidate on hyperactivity and attention deficits in children with ADHD: a virtual classroom test. J. Atten. Disord. 24 (2), 277–289. doi:10.1177/1087054716647480
National Institutes of Health (US), (1998). “NIH consensus development conference on diagnosis and treatment of attention deficit hyperactivity disorder,” in William H. Natcher Conference Center, National Institutes of Health. Bethesda, Maryland: National Institutes of Health, Continuing Medical Education, 16–18.
Parsons, T. D. (2015). Virtual reality for enhanced ecological validity and experimental control in the clinical, affective and social neurosciences. Front. Hum. Neurosci. 9, 660. doi:10.3389/fnhum.2015.00660
Parsons, T. D., Bowerly, T., Buckwalter, J. G., and Rizzo, A. A. (2007). A controlled clinical comparison of attention performance in children with ADHD in a virtual reality classroom compared to standard neuropsychological methods. Child neuropsychology a J. normal Abnorm. Dev. Child. Adolesc. 13 (4), 363–381. doi:10.1080/13825580600943473
Parsons, T. D., and Rizzo, A. S. (2019). “A review of virtual classroom environments for neuropsychological assessment,” in Virtual reality for psychological and neurocognitive interventions. Editors S. Rizzo, and S. Bouchard (Germany: Springer Nature Switzerland AG), 247–265. doi:10.1007/978-1-4939-9482-3_11
Perra, A., Riccardo, C. L., De Lorenzo, V., De Marco, E., Di Natale, L., Kurotschka, P. K., et al. (2023). Fully immersive virtual reality-based cognitive remediation for adults with psychosocial disabilities: a systematic scoping review of methods intervention gaps and meta-analysis of published effectiveness studies. Int. J. Environ. Res. public health 20 (2), 1527. doi:10.3390/ijerph20021527
Piek, J. P., Baynam, G. B., and Barrett, N. C. (2006). The relationship between fine and gross motor ability, self-perceptions and self-worth in children and adolescents. Hum. Mov. Sci. 25 (1), 65–75. doi:10.1016/j.humov.2005.10.011
Pira, G. L., Aquilini, B., Davoli, A., Grandi, S., and Ruini, C. (2023). The use of virtual reality interventions to promote positive mental health: systematic literature review. JMIR Ment. Health. 10 (1), e44998. doi:10.2196/44998
Pollak, Y., Weiss, P. L., Rizzo, A. A., Weizer, M., Shriki, L., Shalev, R. S., et al. (2009). The utility of a continuous performance test embedded in virtual reality in measuring ADHD-related deficits. J. Dev. Behav. Pediatr. JDBP 30 (1), 2–6. doi:10.1097/DBP.0b013e3181969b22
Powers, M. B., and Rothbaum, B. O. (2019). Recent advances in virtual reality therapy for anxiety and related disorders: introduction to the special issue. J. anxiety Disord. 61, 1–2. doi:10.1016/j.janxdis.2018.08.007
Ribeiro, F. F., Cavaglia, R., and Rato, J. R. (2021). Sex differences in response inhibition in young children. Cogn. Dev. 58, 101047. doi:10.1016/j.cogdev.2021.101047
Riva, D., Taddei, M., and Bulgheroni, S. (2018). The neuropsychology of basal ganglia. European Journal of Paediatric Neurology. Eur. J. Paediatr. Neurol. 22 (2), 321–326. doi:10.1016/j.ejpn.2018.01.009
Riva, G., Gutiérrez-Maldonado, J., Dakanalis, A., and Ferrer-García, M. (2019). “Virtual reality in the assessment and treatment of weight-related disorders,” in Virtual reality for psychological and neurocognitive interventions. Editors S. " Rizzo, and S. Bouchard (Germany: Springer Nature Switzerland AG), 163–193. doi:10.1007/978-1-4939-9482-3_7
Rizzo, A., Koenig, S., and Lange, B. (2023). “Clinical virtual reality: the state of the science,” in APA handbook of neuropsychology: neuroscience and neuromethods. Editors G. G. Brown, B. Crosson, K. Y. Haaland, and T. Z. King (American Psychological Association), 473–491. doi:10.1037/0000308-023
Rizzo, A. A., Bowerly, T., Buckwalter, J. G., Klimchuk, D., Mitura, R., and Parsons, T. D. (2006). A virtual reality scenario for all seasons: the virtual classroom. CNS spectrums 11 (1), 35–44. doi:10.1017/s1092852900024196
Rizzo, A. A., Buckwalter, J. G., Humphrey, L., van der Zaag, C., Bowerly, T., Chua, C., et al. (2000). The virtual classroom: a virtual reality environment for the assessment and rehabilitation of attention deficits. CyberPsychology Behav. 3 (3), 483–499. doi:10.1089/10949310050078940
Rizzo, A. A., and Koenig, S. (2017). Is clinical virtual reality ready for primetime? Neuropsychology 31 (8), 877–899. doi:10.1037/neu0000405
Rodríguez, C., Areces, D., García, T., Cueli, M., and González-Castro, P. (2018). Comparison between two continuous performance tests for identifying ADHD: traditional vs. virtual reality Int. J. Clin. health Psychol. IJCHP 18 (3), 254–263. doi:10.1016/j.ijchp.2018.06.003
Satu, P., Minna, L., and Satu, S. (2023). Immersive VR assessment and intervention research of individuals with neurodevelopmental disorders is dominated by ASD and ADHD: a scoping review. Rev. J. Autism Dev. Disord. doi:10.1007/s40489-023-00377-3
Schweitzer, J. B., and Rizzo, A. A. (2022). Virtual reality and ADHD: clinical assessment and treatment in the metaverse. ADHD Rep. 30 (30), 1–9. doi:10.1521/adhd.2022.30.3.1
Shaw, P., Malek, M., Watson, B., Sharp, W., Evans, A., and Greenstein, D. (2012). Development of cortical surface area and gyrification in attention-deficit/hyperactivity disorder. Biol. psychiatry 72 (3), 191–197. doi:10.1016/j.biopsych.2012.01.031
Slater, M., Neyret, S., Johnston, T., Iruretagoyena, G., Crespo, M. Á. C., Alabèrnia-Segura, M., et al. (2019). An experimental study of a virtual reality counselling paradigm using embodied self-dialogue. Sci. Rep. 9 (1), 10903. doi:10.1038/s41598-019-46877-3
Slobodin, O., and Davidovitch, M. (2019). Gender differences in objective and subjective measures of ADHD among clinic-referred children. Front. Hum. Neurosci. 13, 441. doi:10.3389/fnhum.2019.00441
Solbes-Canales, I., Valverde-Montesino, S., and Herranz-Hernández, P. (2020). Socialization of gender stereotypes related to attributes and professions among young Spanish school-aged children. Front. Psychol. 11, 609. doi:10.3389/fpsyg.2020.00609
Tartaglia, N. R., Ayari, N., Hutaff-Lee, C., and Boada, R. (2012). Attention-Deficit hyperactivity disorder symptoms in children and adolescents with sex chromosome aneuploidy. J. Dev. Behav. Pediatr. 33 (4), 309–318. doi:10.1097/dbp.0b013e31824501c8
Vaidya, C. J. (2012). Neurodevelopmental abnormalities in ADHD. Curr. Top. Behav. Neurosci. 9, 49–66. doi:10.1007/7854_2011_138
Waddell, J., and McCarthy, M. M. (2010). Behavioral neuroscience of attention deficit hyperactivity disorder and its treatment. Curr. Top. Behav. Neurosci. 9, 341–360. doi:10.1007/7854_2010_114
Zahn-Waxler, C., Crick, N. R., Shirtcliff, E. A., and Woods, K. E. (2006). “The origins and development of psychopathology in females and males,” in Developmental psychopathology: theory and method. Editors D. Cicchetti, and D. J. Cohen (China: John Wiley and Sons, Inc), 76–138.
Keywords: virtual reality, ADHD, attention processes, assessment, virtual classroom, neuropsychology
Citation: Goh C, Ma Y and Rizzo A (2024) Normative performance data on visual attention in neurotypical children: virtual reality assessment of cognitive and psychomotor development. Front. Virtual Real. 5:1309176. doi: 10.3389/frvir.2024.1309176
Received: 07 October 2023; Accepted: 12 March 2024;
Published: 04 April 2024.
Edited by:
Isabelle Esther Bauer, University of Texas Health Science Center at Houston, United StatesReviewed by:
Charles E. Hughes, University of Central Florida, United StatesMarta Reis, University of Lisbon, Portugal
Copyright © 2024 Goh, Ma and Rizzo. This is an open-access article distributed under the terms of the Creative Commons Attribution License (CC BY). The use, distribution or reproduction in other forums is permitted, provided the original author(s) and the copyright owner(s) are credited and that the original publication in this journal is cited, in accordance with accepted academic practice. No use, distribution or reproduction is permitted which does not comply with these terms.
*Correspondence: Albert Rizzo, rizzo@ict.usc.edu