- 1Tufts Applied Cognition Laboratory, Department of Psychology, Tufts University, Medford, MA, United States
- 2Musculoskeletal Health and Ergonomics Laboratory, Department of Occupational Therapy, Tufts University, Medford, MA, United States
Immersion is a crucial component to characterizing virtual reality (VR) and discovering its many implications for our lives. Studies have defined immersion as the extent that virtual environments submerge users’ perceptual systems, shut out the physical environments’ inputs, and induce engrossment in users. The present study sought to further understand the applications of immersion in virtual environments by leveraging traditional self-report measures, as well as investigating the use of distraction to quantify immersion objectively. We hypothesized that participants in immersive 3-dimensional VR would self-report higher levels of immersion and engagement compared to a non-VR condition. We also predicted that participants in the VR condition would have impaired responses to a secondary task compared to the non-VR condition. Using a within-subjects design, participants engaged in a VR meditation both on a computer screen (non-VR) and through a VR headset (VR). During the meditations, they completed a secondary detection-response task (DRT) by pressing a button in response to a tactile stimulus. We used DRT reaction times to objectively measure immersion. Following the meditations, participants completed questionnaires regarding their experiences. As predicted, participants reported that the VR condition increased their engagement and presence compared to the non-VR version of the same meditation scenario. With regard to the secondary detection task, we did not find a significant effect of VR on DRT performance, although DRT performance was numerically worse in the VR condition compared to the non-VR condition. These mixed results suggest that the use of a secondary task depends upon several factors, which we discuss.
1 Introduction
1.1 What is immersion?
Technology developers are constantly seeking to enhance users’ experiences in devices whose implications broadly range from gaming, medicine, education, manufacturing, and many other domains. One way to improve experiences is via immersion, which is sometimes referred to as presence, though see Lombard and Ditton (1997) and Mestre and Vercher (2011) for further distinctions. Immersion is a phenomenon characterized by a shift in users’ attention, often involving dissociation from the attentiveness of the real world, that occurs because of their cognitive processes in states of increased mental involvement (Agrawal et al., 2020). This definition’s critical part is the shift in users’ attentional states. Attention is central to the concept of immersion, for disassociation from the awareness of the real, physical world is critical in immersive experiences (Agrawal et al., 2020). An immersive experience entirely overrides sensations and perceptions from the real, physical world, allowing users to devote their attentional resources to the mediated world (Zhang, 2020).
When discussing immersion, previous studies have often also utilized the term “presence.” Defining presence as the subjective feeling of “being there” in a mediated virtual environment, they have used presence to capture users’ subjective experiences in mediated environments, or how users perceive virtual worlds (Lombard and Ditton, 1997; Mestre and Vercher, 2011). In contrast, these studies more utilized “immersion” as an objective evaluation of a technology, relating the term more to a VR experience’s perceptual and interactive aspects (Lombard and Ditton, 1997; Mestre and Vercher, 2011; George et al., 2018).
For the purposes of our research, the present study will consider the above distinction between presence and immersion and will utilize presence as immersion, as described by Lombard and Ditton (1997) and again by Nordahl and Korsgaard (2010). According to these studies, presence as immersion is the extent that virtual environments submerge users’ perceptual systems; shut out the physical environment’s inputs; and induce engagement, involvement, engrossment, and absorption in users (Lombard and Ditton, 1997; Nordahl and Korsgaard, 2010). This definition emphasizes using presence in understanding immersion and includes the context of virtual environments, which will be necessary for the present study. Therefore, we will utilize the term “immersion” to capture this concept.
1.2 Immersion in virtual environments
Previous researchers have proposed defining immersion as the perceptual and sensory experience of being physically situated in a simulated, mediated, or non-physical environment. Specifically, in a virtual environment, immersion is the degree to which stimuli in the virtual environment engross users’ perceptual systems (Zhang, 2020). These virtual environments may be displayed using VR. VR is a mediated reality format that allows users an unlimited field of view and a constantly updated viewpoint to facilitate natural interaction with and perception of an artificial environment (Pausch et al., 1997; Nilsson et al., 2016). Environments in VR enhance users’ immersive experiences compared to other media forms. For example, Frewen et al. (2020) reported greater satisfaction among participants from VR exercises compared with non-VR exercises. VR’s effects on brain activity may explain the augmented immersion and satisfaction levels. Immersive VR environments can induce enhanced neural activity and attention in users compared to non-interactive media (Klimmt et al., 2005). Greater neural activity may increase one’s sense of immersion in such environments.
1.3 Quantifying immersion
1.3.1 Why try to quantify immersion?
Understanding immersion is crucial to understanding users’ experiences in technology-mediated environments and tasks. There is a need to measure and quantify immersion because evaluating the quality of users’ experiences in VR is heavily dependent on our understanding of their immersion. Previous research has often used subjective methods to measure immersion (Gershon et al., 2004; Frewen et al., 2011; O’Brien et al., 2018; Armougum et al., 2019).
1.3.2 Subjective measures of immersion
One of the most common methods to measure immersion is subjective, post-test questionnaires. These measures are administered to participants following the immersive experience and have two different uses: to understand the impact of virtual environments’ technological factors on different individuals, for instance, by using a reported sense of immersion (Slater and Usoh, 1993); and to measure the subjective judgment and perception of the immersive environment, for they view immersion as a multidimensional construct (Zhang, 2020).
Subjective questionnaires have several advantages for measuring immersion in VR. They are relatively easy to interpret and administer because they do not require complex setups or equipment (van Baren and Ijsselsteijn, 2004). Post-test immersion questionnaires do not intrude upon the immersive experience during the experiment, reducing interruptions and allowing the results to reflect immersion’s nature more candidly. They can also examine many dimensions of the immersive experience simultaneously and consequently provide a robust measurement (Zhang, 2020). Questionnaires can measure overall immersive experience without being subject to individuals’ emotional, personal, and physiological conditions irrelevant to the tested stimuli (Zhang, 2020). Measuring several dimensions of immersion at once enables researchers to obtain an overall idea of the immersive experience. The questionnaires’ divisions into different questions allow participants to focus on one aspect of immersion at a time.
1.3.3 Can objective measures add to this characterization?
Objective measures are another way to measure immersion in virtual environments. Adding objective measures with existing subjective measures and looking for converging evidence may be beneficial. Indeed, prior research has leveraged physiological differences (e.g., skin conductance and heart rate) between VR and non-VR conditions as another way to quantify immersion in addition to self-report (Knaust et al., 2022; Lemmens et al., 2022).
Previous research has also begun to explore using secondary task performance as an objective metric to gauge participants’ immersion levels. The secondary task method involves having participants respond to a secondary task that requires some degree of attention during the primary task in which the participant is initially immersed in performing. Secondary tasks can be administered either once or at random or regular intervals, depending on the variable of interest.
One theory proposes that immersive experiences are multidimensional constructs in which one can measure continuous increments of cognitive load to indicate the degree to which the user’s attentional resources and mental capacity are absorbed in the task (Zhang, 2020). Cognitive load is a continuous increase in mental workload, characterized by a demand for higher-level cognitive operations like decision-making, error detection, planning, sustaining information in working memory, and overcoming habitual actions (Stojmenova and Sodnik, 2018; Robinson et al., 2021). Both primary and secondary tasks require attentional resource allocation. Secondary tasks and primary tasks are both resource-limited tasks: tasks in which increasing the number of available processing resources can lead to improved performance. Therefore, interference on either task implies that both tasks draw from and utilize processing resources from the same pool (Norman and Bobrow, 1975).
When primary tasks induce more cognitive load, fewer attentional resources can be allocated to secondary tasks (Norman and Bowbrow, 1975; Wickens, 2008). Thus, secondary tasks’ indirect measure of cognitive load can reveal how much one’s attentional resources and mental capacity are absorbed in a primary task. Applying this information, the more a participant’s attention is devoted to the virtual environment, the more immersive the experience. In addition, external stimuli coming from the real, physical environment are ignored, and it is more difficult for the participant to revert their attention to the physical environment where experimenters solicit rapid responses to a secondary task (Zhang, 2020).
The secondary task performance method has several advantages that support its use in immersion research. For example, researchers can easily combine this method with other attentional measures, like eye-tracking, to collect data in various modalities (Norman and Bobrow, 1975; Wickens, 2008). Because secondary task administration occurs during the primary task, researchers can easily conduct non-invasive observations like eye-tracking without interfering with the VR tasks. Also, task performance is a continuous feature during the immersive experience, so measuring immersion levels is not reliant on participant report after the experience has concluded. Research has demonstrated that using secondary task performance is reliable and ensures both convergent and discriminant validities because the involved primary and secondary tasks may be of a different nature, like switching to a motor task from one that is entirely cognitive (Armougum et al., 2019; Castro et al., 2019) Thus, the secondary task performance method is a sensitive, valid, and continuous measure that holds promise for future exploration. Despite this, there is a lack of research on secondary task performance for measuring immersion in VR.
1.3.3.1 Detection response tasks
One example of a secondary task is a detection-response task (DRT). This task, which researchers often use in a secondary task reaction time (STRT) paradigm, involves randomly providing a sensory stimulus every 3–5 s and having a participant respond by pressing a response button on their index finger (Stojmenova and Sodnik, 2018). Performance is measured by response times and hit rates (Stojmenova and Sodnik, 2018). Previous research in driving and VR contexts has used the DRT to measure cognitive load. For example, in their study using a VR driving simulator and cognitively loading tasks, Nilsson et al. (2018) found that increased cognitive load on a primary task increased DRT response times. However, responding to DRT stimuli does not appear to impose an additional cognitive load on the user, making it a potentially suitable secondary task measure of cognitive load in VR (Wickens, 2008; Stojmenova and Sodnik, 2018).
In another study, George et al. (2018) concluded that a DRT embedded within a virtual experience was appropriate for measuring attention in immersive VR. Their DRT task allowed experimenters to maintain constant presence levels without the secondary task disrupting performance (George et al., 2018). This prior study provided insight into incorporating a detection-response task into VR primary tasks. However, George et al., 2018 did not include a real-time measures of immersion in a non-VR control condition, leaving a gap for subsequent research to address.
1.4 Present study
In this study, we used self-report and secondary task performance to measure immersion in a VR scenario compared to a non-VR scenario. We hypothesized that participants in immersive VR would self-report higher levels of workload, engagement, and sense of presence compared to a non-VR condition. For secondary task performance, we used secondary task reaction time, or STRT, in the form of a DRT to add to the immersion characterization provided by self-reports. Immersion increases cognitive load, and increased cognitive load may result in increased DRT response times and reduced response accuracy (Nilsson et al., 2018; Zhang, 2020). Therefore, we hypothesized that participants in immersive VR would have impaired responses to a secondary task, specifically a detection-response task, compared to when in a non-VR version of the same scenario. Few studies have attempted to use both self-report and secondary tasks to quantify immersion (Darken et al., 1999; Klimmt et al., 2005; Bracken et al., 2014), and to our knowledge, no studies have used a detection-response task to measure this phenomenon. We aim to fill this knowledge gap.
2 Materials and methods
2.1 Design
We used a within-subjects design for this study. The independent variable was the level of VR immersion participants experienced (VR Level), and it involved the presentation of a VR and non-VR meditation scenario. We counterbalanced run orders across two experimental sessions and then randomized these orders across participants. The dependent variables included participants’ responses to post-experience questionnaires, as well as levels of distraction from real-world physical stimuli, measured using a DRT paradigm.
2.2 Participants
Participants were recruited via the psychology department at Tufts University. Given the novelty of our approach, we did not have sufficient information on anticipated effect sizes to conduct a meaningful power calculation to determine our sample size. Thus, prior to data collection, we determined that we would aim to collect data from roughly 50 participants since this would be at least double the sample size from the most similar prior study we could find (George et al., 2018). We recruited 41 participants for this study and paid them $10 per session. Eligibility requirements for the study included fluency in English, no prior experience with meditation, having normal or corrected-to-normal vision, and having no prior history of neurological impairment. Participants were on average 21.1 (SD = 3.72) years old, with one participant declining to provide their age. Of the 41 participants, 14 (34.1%) identified as male, 25 (61.0%) were female, and 2 (4.88%) declined to report their gender.
2.3 Materials
We administered the two types of environments (VR vs. non-VR) to participants as a computer-based meditation program, experienced either through the Oculus VR headset (VR) or by watching a computer screen (non-VR). We measured immersion using a secondary task as well as questionnaires that participants completed on an iPad following each meditation.
2.3.1 Oculus virtual reality
We used an Oculus Rift S VR headset to provide the immersive experience. Participants wore the headset during the VR condition. For the non-VR condition, we placed the headset on a manikin head positioned behind the participants, and the participants viewed the scenario on a computer screen. Refer to Figure 1 for a depiction of this setup. We used the Guided Meditation VR™ program as the experience for both conditions. We chose this type of VR experience in part because we were interested in leveraging immersive VR meditation for participants suffering from chronic pain in a subsequent study and also because the scenarios were very similar across the VR and non-VR sessions. Importantly, in both the VR and non-VR conditions, participants watched a peaceful visual scene while listening to guided audio meditation instructions. More details about this meditation program can be found at https://guidedmeditationvr.com/.
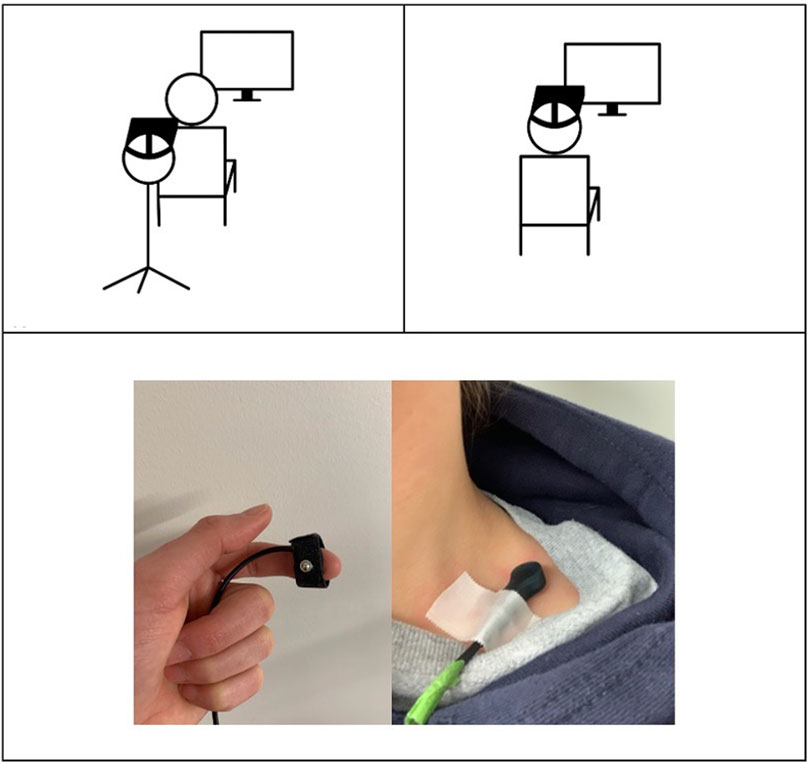
Figure 1. Experimental Set-Up for VR and DRT Devices. Note: Top left panel depicts the non-VR set-up with the VR headset positioned on the manikin head and the participant watching the meditation on the computer screen. Top right panel depicts the VR condition in which participants wore and experienced the meditation through the VR headset. Bottom panel depicts DRT placement. Participants placed the response button (left) on the tip of the left index finger and taped the vibrating tactor (right) to the skin just above the left collarbone.
2.3.2 Detection-response task during meditation
We used the detection-response task as a secondary task because of its widespread use in other virtual environments that usually involve simulated driving (Stojmenova and Sodnik, 2018). The DRT device was a small vibrating tactor attached to the participants’ skin just above their left collarbone and a response button attached to the left index finger (see Figure 1). The tactor produced a tactile stimulus of 1-s duration irregularly over intervals of 3–5 s, which is the gold standard in related DRT and VR studies (ISO 17488:2016, 2022). We instructed participants to press the button on their index finger as quickly as possible when they felt the vibration. The DRT device measured reaction times for each button press and hit rates. Importantly, we chose a haptic version of the DRT rather than a visual or auditory version since those modalities would already be engaged by the meditation scenarios (Wickens, 2008).
2.3.3 Post-meditation questionnaires
2.3.3.1 NASA task load index (NASA-TLX)
The NASA-TLX was a 6-item questionnaire assessing workload (Hart and Staveland, 1988). “Mental Demand” assessed the extent of cognitive resources’ requirement for the task, and “Physical Demand” assessed the level of physical exertion required. “Temporal Demand” examined whether the task’s pace was hurried or rushed; “Successful Performance” evaluated participants’ success in accomplishing their tasks. “Effort” measured how hard participants had to work to achieve their performance level. “Frustration” assessed how annoyed, insecure, irritated, stressed, or discouraged participants felt during the task. Each item was evaluated on a 21-point sliding scale, with each point representing an interval of 5 from 0 to 100. The scale ranged from “Very Low” to “Very High,” with scores closer to 0 representing a lower workload and scores closer to 100 representing a higher workload. We then averaged the scores of the six items to obtain a total NASA-TLX score, which represented a summary score of all the items. We used the total NASA-TLX score for analysis in this paper.
2.3.3.2 User engagement scale (UES)
We used the 12-item User Engagement Scale to evaluate participants’ engagement in the VR experience (O’Brien et al., 2018). Each item used a five-point Likert Scale (1 = “Strongly Disagree; ” 3 = “Neither Agree nor Disagree; ” 5 = “Strongly Agree.”). We calculated the average scores of the 12 items to obtain an overall engagement score.
2.3.3.3 IGroup presence questionnaire (IGPQ)
The IGroup Presence Questionnaire, a 14-item questionnaire, evaluated participants’ sense of presence, or “feeling of being there,” in the virtual environment (Schubert, 2003). The questions were subdivided into four subscales, “General Presence,” “Spatial Presence,” “Involvement,” and “Experienced Realism.” Each question was answered using a 7-point sliding scale with values of 0–6. We averaged the scores for items within each of the four subscales to obtain summary scores.
2.3.3.4 Meditative experiences questionnaire (MEQ)
The Meditative Experiences Questionnaire was a 13-item questionnaire evaluating participants’ experiences during a meditative state (Frewen et al., 2011). Each question was answered using a 5-point Likert Scale (1 = “never; ” 3 = “sometimes; ” 5 = “almost constantly” going through the experiences).
2.4 Procedure
Participants read and signed a consent form approved by the university ethics committee. Before beginning the experiment, participants completed a series of pre-meditation questionnaires, including questions about the experiment’s date, time, and session as well as the PANAS and the STAI-Short questionnaires. These questionnaires, as well as the Simulator Sickness Questionnaire administered at the end of the meditations, are included in our Supplementary Material but were not part of the analysis for the current study.
Next, participants donned the DRT device, placing the button on the tip of their left index finger and the vibrating tactor just above their left collarbone (Figure 1). Participants underwent a 1-min DRT baseline to acclimate to the device. Following the baseline, we divided the experimental session into two parts. During each part, participants underwent a round of a 10-min meditation, one with the DRT and one without it; however, we only focused on the part that included the DRT, given our guiding research questions. Participants chose a visual meditation scenario from a list of five scenarios (see Supplementary Material). The same scenario was used for both the VR and non-VR sessions and included both auditory and visual stimuli. More details about this type of meditation program can be found at https://guidedmeditationvr.com/focus-meditation-1/. Participants then completed either the VR or non-VR meditation depending upon their run order. During the meditation, participants slowly advanced through a rich visual scene as if on a glider while focusing on their breathing. We allowed participants to select the visual environment through which they glided (see Supplementary Material), but the audio experience was prescribed and remained consistent across participants and visual environments. The guided meditation audio remained the same across VR and non-VR conditions. First, the audio guide informed participants they could either close their eyes or leave them open, though participants mostly kept their eyes open. Next, the meditation led participants through a series of inhales and exhales and then gave participants intervals of 15–40 s to focus on their breathing without instruction. In between these periods, participants received reminders that mind wandering and fidgeting are normal but that they should continue to focus on their breathing. After about eight and a half minutes, the audio guide started to bring participants out of the meditation, instructing them to wiggle their fingers and toes, notice different body and mind sensations compared to before the meditation, and bring their attention back to the present moment. After completing the first round of the meditation, participants completed post-meditation questionnaires. Participants then returned for a second session at least 48 h following the first session and completed the VR or non-VR session, depending on their run order. We debriefed participants about the study at the end of the second session.
2.5 Data processing and analysis
Based on the ISO standard for the DRT task, we excluded DRT response times faster than 100 ms and slower than 2,500 ms after stimulus onset, (ISO 17488:2016, 2022). We calculated total scores for the post-meditation subjective measures as outlined in the respective sections above. Using Jamovi version 2.3.0 software, we analyzed each measure by means of a paired samples t-test to evaluate the effect of VR level on our dependent variables. Secondary task performance (i.e., DRT performance) included reaction times and response accuracy (hit rates). Self-report dependent measures included workload, engagement in the task, feelings of presence, and experiences with meditation. Alpha for all measures was set at p < .05.
3 Results
3.1 Secondary task performance (DRT)
The mean reaction time for the detection-response task for the non-VR condition was 356 ms (SD = 111). For the VR condition, the mean reaction time was 382 ms (SD = 168) (See Figure 2 and Table 1). Despite this numerical difference, the RT difference between VR levels did not reach statistical significance (See Figure 2 and Table 1). The mean experimental hit rate for the detection-response task when participants experienced the non-VR condition was 0.986 (SD = 0.040). In the VR session, the average hit rate was 0.967 (SD = 0.120). Counter to our predictions, this difference was not statistically significant (See Table 1).
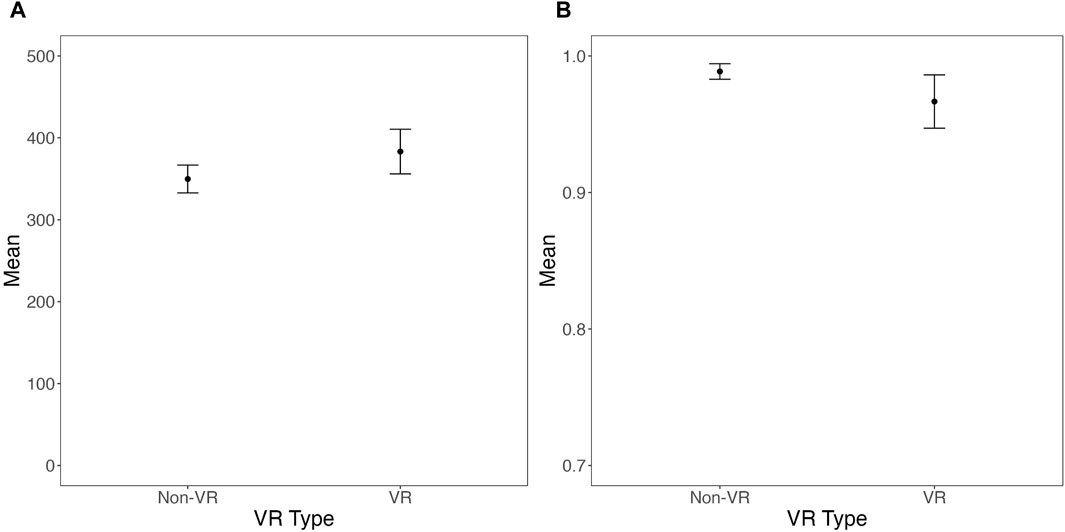
Figure 2. DRT Performance Estimated Marginal Means. Note. (A) depicts the average DRT response times for each VR level. (B) depicts the average accuracy (hit rate) of DRT responses in each VR level. The error bars represent a 95% confidence interval.
3.2 Self-report measures
3.2.1 Workload
We used the total NASA-TLX to measure participants’ workload. We did not observe a main effect of VR level on any individual workload score or for the total score (Table 2; Figure 3).
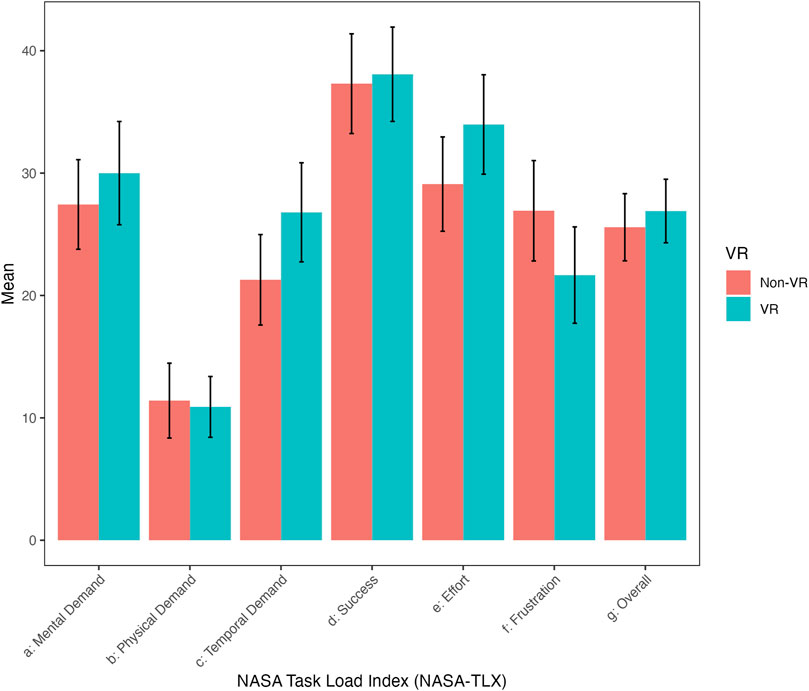
Figure 3. NASA-TLX Estimated Marginal Means. Note. From left to right: (A). Mental Demand = average mental demand required for each VR level; (B). Physical Demand = mean physical demand involved for each VR level; (C). Temporal Demand = average temporal demand each VR level elicited; (D). Success = average amount of success in task completion participants perceived for each VR level; (E). Effort = average required effort amounts during each VR level; (F). Frustration = average frustration levels during each VR level; (G). Overall = average total NASA-TLX scores across VR level. The error bars represent a 95% confidence interval.
3.2.2 Engagement in the task
The average total UES score for the non-VR condition was 2.74 (SD = 0.24) and was 2.89 (SD = 0.24) for the VR condition. We observed a main effect of VR level, t (38) = −3.15, p = 0.003, d = −0.51 (Table 3). Planned comparisons revealed that participants experienced more engagement in the VR condition compared to the non-VR condition (See Figure 4).
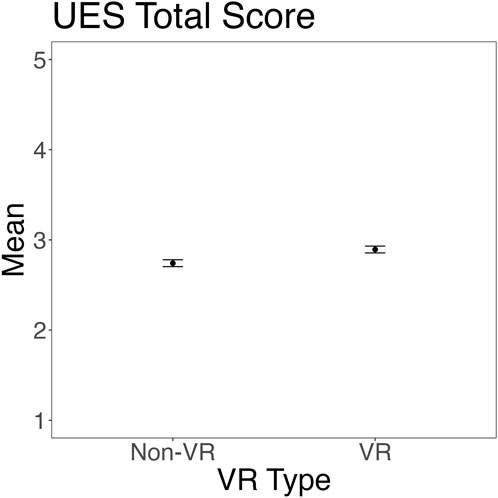
Figure 4. UES Estimated Marginal Means. Note. Average engagement reports for each VR level. The error bars represent a 95% confidence interval.
3.2.3 Presence
For the general presence item on the IGPQ, results demonstrated a main effect of VR level, t (38) = −9.59, p < .001, d = −1.54. Planned comparisons revealed that the VR condition induced higher general presence ratings compared to the non-VR condition (See Table 4 and Figure 5). For the spatial presence item, we observed a main effect of VR level, t (38) = −7.42, p < .001, d = −1.19. Planned comparisons indicated that participants experienced greater feelings of spatial presence in the VR condition than in the non-VR condition (p < .05). For involvement, we observed a main effect of VR level, t (38) = −5.32, p < .001, d = −0.85. Planned comparisons revealed that the VR condition induced greater feelings of involvement for participants than the non-VR condition (p < .05). For experienced realism, we found a main effect of VR level, t (38) = −9.18, p < .001, d = −1.47. Planned comparisons revealed greater experienced realism in the VR condition compared to the non-VR condition (p < .05).
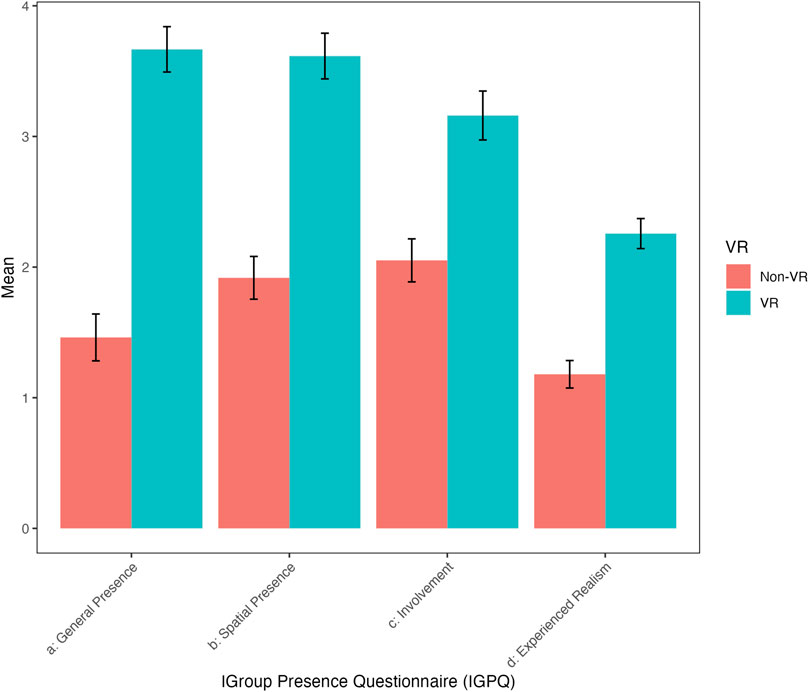
Figure 5. IGPQ Estimated Marginal Means. Note. From left to right: (A). General Presence = average reports of general presence in each VR level; (B). Spatial Presence = average spatial presence reports across VR levels; (C). Involvement = average involvement in the meditation task across VR level; (D). Experienced Realism = average experienced realism of the virtual environment for each VR level. The error bars represent a 95% confidence interval.
3.2.4 Experiences with meditation
For the MEQ item about reviewing a mental “to-do list,” we observed a main effect of VR, t (38) = 3.37, p = 0.011, d = 0.54. Planned comparisons revealed that participants reported more frequent list reviews in the non-VR condition than in the VR condition, which suggests less immersion during that condition. However, we did not observe a main effect of VR level on participants’ experiences of unpleasant thoughts, pleasant thoughts, calm feelings, or distraction awareness (See Table 5 and Figure 6). For the awareness of others item, we observed a main effect of VR level, t (38) = 2.68, p < .001, d = 0.43. Planned comparisons indicated that participants were more aware of others’ presence in the non-VR condition compared to the VR condition (See Table 5 and Figure 6). However, there was no main effect of VR level on participants’ awareness of the physical environment. For participants’ awareness of their own bodies during the meditation scenarios, we found a significant main effect of VR level, t (38) = 2.81, p = 0.008, d = 0.45. Planned comparisons indicated increased body awareness in the non-VR condition compared to the VR condition (See Table 5 and Figure 6). Similarly, we detected a main effect of VR participants’ reported discomfort, t (38) = 2.07, p = 0.045, d = 0.33. Planned comparisons revealed that discomfort scores were higher during the non-VR condition than during the VR condition (See Table 5 and Figure 6). Additionally, there was a main effect of VR on thoughts about leisure activities during the meditation, t (38) = 3.45, p = 0.001, d = 0.55. Planned comparisons revealed that these thoughts became more frequent during the non-VR condition than the VR condition (See Table 5 and Figure 6). We did not observe any main effects of VR level on the MEQ items concerning fatigue, participants’ attention to their breathing, and mantra use.
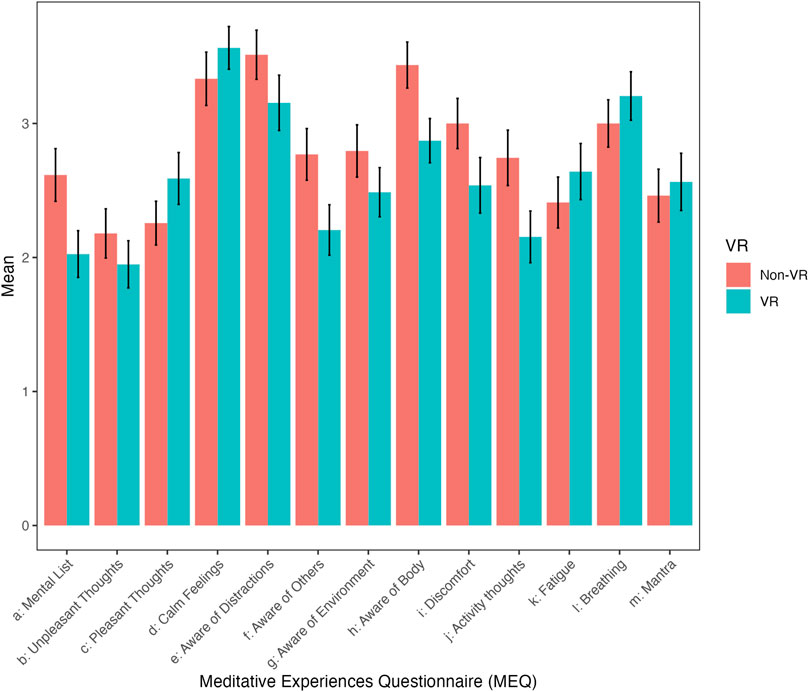
Figure 6. MEQ Estimated Marginal Means. Note. From left to right: (A). Mental List = average reports of reviewing mental lists during the meditation in each VR level; (B). Unpleasant Thoughts = average unpleasant thought occurrence in each VR level; (C). Pleasant Thoughts = average pleasant thought occurrence in each VR level; (D). Calm Feelings = average experience of calm feelings across VR levels; (E). Aware of Distractions = average distraction awareness during each VR level; (F). Aware of Others = reported awareness of others in each VR level; (G). Aware of Environment = average environmental awareness across VR levels; (H). Aware of Body = average body awareness reports during each VR level; (I). Discomfort = average discomfort levels during each VR level; (J). Activity thoughts = average occurrence of activity thoughts in each VR level; (K). Fatigue = each VR level’s induced fatigue; (L) Breathing = breathing awareness during each VR level; (M) Mantra = average use of a mantra to focus attention during the meditation in each VR level. The error bars represent a 95% confidence interval.
4 Discussion
4.1 Summary of results
The purpose of the present study was to measure immersion in a VR-guided meditation using self-report, as well as to investigate the potential use of secondary task distraction to further quantify immersion in VR. Participants experienced both a non-VR and VR meditation while simultaneously performing a secondary DRT to quantify their immersion during the scenarios, and then they completed several self-report surveys related to immersion. We hypothesized that DRT performance would be significantly slower and more error-prone in the VR condition compared to the non-VR condition and that self-reported immersion would be significantly greater in the VR condition than the non-VR condition.
We used multiple self-reported measures of immersion, and several of these self-report questionnaires yielded significant results. For example, the UES results showed a main effect of VR level on participants’ overall engagement, with more engagement in the VR condition compared to the non-VR condition, which aligns with prior research (Tieri et al., 2018; Flavian et al., 2021). We also observed that the VR condition yielded a significantly greater sense of general presence, spatial presence, involvement, and experienced realism than the non-VR condition, which also aligns with prior research (Regenbrecht and Schubert, 2002).
The VR level also had a pronounced effect on participants’ meditative experiences, with participants reporting more distraction and fewer effects of the meditative experience during the non-VR condition than during the VR condition. For example, during the non-VR task, participants experienced significantly more mental list reviewing, awareness of others, bodily awareness, bodily discomfort, and thoughts about leisure activities than during the VR task, which aligns with prior research (Sarkar et al., 2022).
In conjunction with the self-report measures, we used a secondary DRT task in an attempt to leverage distraction to further quantify immersion. Contrary to our prediction, we did not find any significant effect of the secondary DRT task in terms of the VR vs. non-VR conditions. This was unexpected given that three prior studies had also utilized secondary task reaction time (STRT) paradigms with measures of attention and immersion in virtual environments and found significant results, yet there are also several differences between those prior studies and ours, which we now detail.
For example, Bracken et al. (2014) used STRT to compare attention and telepresence measures and demonstrated that attention and telepresence-immersion were significantly related. Reaction times were slower when participants reported being mentally immersed, engaged, or involved with the video content, thus concluding that STRT may be used to measure telepresence, specifically its immersion factor, although they used a web survey to present video clips rather than a head-mounted VR system like we used, nor did they use any form of meditation.
Similarly, Klimmt et al. (2005) came to a similar conclusion when using three types of probes (visual, auditory, and audiovisual) in an STRT procedure to determine the specific qualities of the cognitive-perceptual resources not consumed by a primary task and to measure special presence in virtual environments. They found that STRT may be useful in assessing visual attention and involvement, although they did not use a head-mounted VR like we did but rather a 2D computer-based virtual environment that participants navigated via mouse. In addition, they did not include any type of meditation.
Finally, Darken et al. (1999)’s study used a dual-task paradigm involving a VR experience and a simultaneous video tape and reported that attention could be used, at least partially, as a measure of immersion and was a measurable artifact of virtual world experiences. Their comparison of VR experiences delivered via a head-mounted display (HMD), a mini-CAVE (Cave Automatic Virtual Environment), and a 2D flatscreen further revealed that the HMD allowed for a greater attentional focus on virtual experiences because it occluded a larger portion of the real world than the other two displays, although they did not include haptic stimuli like the current study nor did they use any type of meditation.
Taken together, these prior studies suggest that it is possible to measure immersion via secondary tasks, even though we did not observe this in the current study, at least in terms of our secondary task measures. Several of our self-report measures’ results aligned with those of the above studies. For example, our finding that reported engagement was significantly greater in the VR condition than the non-VR condition mirrored that of Darken et al. (1999), who concluded that the head mounted display to induced significant engagement in users. Also, our observation that VR meditation induced significantly greater general presence, spatial presence, involvement, and experienced realism than non-VR meditation mirrored Bracken et al. (2014)’s findings.
Our study differed from prior research in several other ways, which could further account for the differences in findings. For example, we are the first to use a tactile secondary task rather than an auditory or visual secondary task. We reasoned that using a tactile DRT would be beneficial because the tactile stimuli relied on haptics, an underutilized sensory modality. In contrast, auditory or visual secondary tasks like those used previously may not have yielded informative results as to whether performance on those secondary tasks reflect immersion or if they reflect simple resource competition. This is because immersive VR uses auditory and visual resources more than haptic or tactile resources.
4.2 Limitations and opportunities for future research
Several factors may have contributed to the lack of statistical significance for our secondary task, including the small sample size, aspects of the VR system, the nature of the meditation task, and aspects of our data collection. With only 41 participants, we had a relatively small sample upon which we could draw conclusions for the objective DRT measures. Since our objective findings trended both with our significant subjective immersion reports and previous reports from the literature (Darken et al., 1999; Klimmt et al., 2005; Bracken et al., 2014), it is possible that a larger sample would have yielded predicted significant differences in primary task immersion.
We also saw increased variability in the VR task compared to the non-VR condition. This increased variability may have arisen from participants’ different levels of experience with VR headsets, varying degrees of comfort and required adjustment time to the VR task, and novelty effects of VR (Wu et al., 2013; Miguel-Alonso et al., 2023). Each of these factors may have led to wider ranges of reaction time data and scores for subjective immersion and engagement reports in the VR condition than in the non-VR condition. Future studies should take into account participants’ familiarity with VR to better understand its potential role in impacting levels of immersion (Ochadleus et al., 2023).
In addition to the small sample size potentially reducing statistical significance, the lack of diversity in our sample may have yielded low generalizability. Our participants were from the healthy undergraduate student population, so future research should investigate the questions asked in the present study using populations with different ages, ethnicities, and health conditions to see if the conclusions drawn in the present study are generalizable to those populations and if perhaps research with more diverse samples might find more alignment between subjective and objective measures of immersion.
While using a secondary task as a measure of immersion has substantial benefits, it also comes with potential drawbacks. For example, secondary tasks can be intrusive to the immersive experience created by the primary task, so it may reduce the face validity of the measurement (Zhang, 2020). Also, factors unrelated to the system’s immersiveness may influence cognitive load and serve as confounds (Zhang, 2020). Therefore, impaired secondary task performance may not necessarily represent increased cognitive load due to the primary task’s immersiveness. Instead, it may reflect, at least in part, an outside source of cognitive load, such as participants simultaneously worrying about daily tasks. Such outside cognitive load sources could confound the results and reduce the certainty with which we can make our associated claims. In addition, it is possible that another source of extraneous cognitive load could have been introduced by differences in the novelty of the VR headset (Wu et al., 2013; Miguel-Alonso et al., 2023), which would have only impacted the VR condition. The novelty of the headset could have led participants to be concerned in the VR condition about possibly breaking the headset. If this happened, they might have been less immersed in the VR condition, which could explain the lack of statistically significant differences in our secondary task data, though it would not account for the statistically significant differences in self-reported levels of immersion. Still, future studies should consider ways to account for participants’ familiarity with VR headsets and to further minimize the possibility of extraneous cognitive load in VR conditions that involve wearing a headset (Han et al., 2021).
Also, for our secondary task, we used a probe frequency of every 3–5 s, which is a frequency that previous research has used in tactile DRT tasks and has been considered a standard metric (ISO 17488:2016, 2022). However, such frequency may have interfered with the participants’ immersion in the VR task. Specifically, immersion may be a state that takes time to enter, so such a short time in between the onset of each stimulus may have prevented participants from returning to their state of immersion before the next stimulus’s onset.
Since the task started immediately upon the starting the VR task, participants may not have even had a chance to initially enter an immersed state before being distracted by the tactile stimulus. Additionally, because the DRT task was a passive task, the relatively high stimulation frequency may have primed participants’ attention toward the next stimulus onset rather than to the intended VR task. Future studies should address this potential confound by varying stimulus frequency across multiple trials or decreasing the frequency to every 10 s or more, as demonstrated by traditional psychomotor vigilance tasks (Drummond et al., 2005).
Importantly, the nature of the meditation scenarios, combined with certain aspects of the VR system, may have contributed to the secondary task measures not aligning with the significance observed from the subjective immersion measures. Specifically, the meditation allowed for participants to briefly close their eyes as they meditated if they so desired since we could not force them to keep their eyes open. Doing so may have reduced some of the differences that we had expected to see between the non-VR and VR meditations, since having their eyes closed would have prevented them from becoming fully engaged with the graphics and seemingly real visual experiences that uniquely characterize VR. That said, participants did not appear to close their eyes given that there was a rich visual scene displayed in both conditions. Still, future research should avoid meditation scenarios that involve the possibility of closing the eyes to minimize this risk. By avoiding meditation scenarios in future studies, researchers could also minimize the risk of participants focusing on their bodily sensations. This can sometimes be a part of mindfulness meditation practices, and it could have minimized the impact of immersion in the VR condition since participants could have more easily noticed the secondary task tactile stimuli.
Finally, to better understand attention as a measure of immersion, it may be beneficial to use non-meditation tasks in which the participants’ attention can be more directed to the primary VR task rather than to their own bodies, such as simulations of riding a rollercoaster, going on a nature walk, or exploring the ocean, as seen with Oculus’s theBlu program. Ensuring that aspects of the VR system itself, including deprivation of or isolation from sensations in the physical environment, users’ perceptions of self-movement, and the content of the experience’s narrative, are sufficient to facilitate engrossment in the experience may further contribute to this goal, for previous research has demonstrated that they may influence immersion in virtual environments (Witmer and Singer, 1998; Zhang et al., 2018; Agrawal et al., 2020). If participants did not feel engrossed by the narrative of the meditation or if the movement sensations within the experience were not strong enough to feel real, DRT performance may have been faster and more accurate than expected in the VR condition. This may have been the case considering the nonsignificant MEQ results pertaining to distraction awareness and environmental awareness. Likewise, our meditation scenarios did not require any manual interactions with the controllers or handsets. This was in part to minimize any possible conflict with the secondary task response button affixed to participants’ index finger (Figure 1). Future studies should explore VR scenarios with more interactions, even if with just one controller, to better understand the potential impact that interactivity might have on immersion in VR.
4.3 Conclusion
As technology becomes an ever-present component of society, an increased knowledge of the phenomena surrounding users’ engagement with and immersion in these devices is necessary to more fully understand the full extent of their applications and better improve their features. Despite the mixed results we obtained, the present research contributes to knowledge of measuring VR-induced immersion and characteristics of tasks that may better optimize this phenomenon. Few studies have used tactile DRT immersion measurements, so our study also helps fill knowledge gaps concerning this method and the parameters for its use in such a context. Future studies should build on our shortcomings by identifying tasks that participants may find more immersive as well as using less frequent DRT stimuli and thus may yield more conclusive findings about real-time measures of immersion that add to the already rich tradition of using subjective measures to quantify immersion.
Data availability statement
The raw data supporting the conclusion of this article will be made available by the authors, without undue reservation.
Ethics statement
The studies involving humans were approved by Tufts University Institutional Review Board. The studies were conducted in accordance with the local legislation and institutional requirements. The participants provided their written informed consent to participate in this study.
Author contributions
EP, KB, NB, and NW: conceptualization. EP and KB: data curation and analysis. NW, EP, and NB: methodology. NW and NB: resources and supervision. EP: writing–original draft. EP, NW, and NB: writing–review and editing. All authors have read and agreed to the published version of the manuscript.
Conflict of interest
The authors declare that the research was conducted in the absence of any commercial or financial relationships that could be construed as a potential conflict of interest.
Publisher’s note
All claims expressed in this article are solely those of the authors and do not necessarily represent those of their affiliated organizations, or those of the publisher, the editors and the reviewers. Any product that may be evaluated in this article, or claim that may be made by its manufacturer, is not guaranteed or endorsed by the publisher.
Supplementary material
The Supplementary Material for this article can be found online at: https://www.frontiersin.org/articles/10.3389/frvir.2024.1212216/full#supplementary-material
References
Agrawal, S., Simon, A., Bech, S., Bærentsen, K., and Forchhammer, S. (2020). Defining immersion: literature review and implications for research on audiovisual experiences. J. Audio Eng. Soc. 68 (6), 404–417. doi:10.17743/jaes.2020.0039
Armougum, A., Orriols, E., Gaston-Bellegarde, A., Marle, C. J.-L., and Piolino, P. (2019). Virtual reality: a new method to investigate cognitive load during navigation. J. Environ. Psychol. 65, 101338. doi:10.1016/j.jenvp.2019.101338
Bracken, C. C., Pettey, G., and Wu, M. (2014). Revisiting the use of secondary task reaction time measures in telepresence research: exploring the role of immersion and attention. AI Soc. 29 (4), 533–538. doi:10.1007/s00146-013-0494-7
Castro, S. C., Strayer, D. L., Matzke, D., and Heathcote, A. (2019). Cognitive workload measurement and modeling under divided attention. J. Exp. Psychol. Hum. Percept. Perform. 45, 826–839. doi:10.1037/xhp0000638
Darken, R. P., Bernatovich, D., Lawson, J. P., and Peterson, B. (1999). Quantitative measures of presence in virtual environments: the roles of attention and spatial comprehension. Cyberpsychology Behav. Impact Internet, Multimedia Virtual Real. Behav. Soc. 2 (4), 337–347. doi:10.1089/cpb.1999.2.337
Drummond, S. P., Bischoff-Grethe, A., Dinges, D. F., Ayalon, L., Mednick, S. C., and Meloy, M. J. (2005). The neural basis of the psychomotor vigilance task. Sleep 28(9), 1059–1068. doi:10.1089/109493101300210222
Flavián, C., Ibáñez-Sánchez, S., and Orús, C. (2021). Impacts of technological embodiment through virtual reality on potential guests’ emotions and engagement through virtual reality on potential guests’ emotions and engagement. J. Hosp. Mark. Manag. 30 (1), 1–20. doi:10.1080/19368623.2020.1770146
Frewen, P., Lundberg, E., MacKinley, J., and Wrath, A. (2011). Assessment of response to mindfulness meditation: meditation breath attention scores in association with subjective measures of state and trait mindfulness and difficulty letting go of depressive cognition. Mindfulness 2, 254–269. doi:10.1007/s12671-011-0069-y
Frewen, P., Mistry, D., Zhu, J., Kielt, T., Wekerle, C., Lanius, R. A., et al. (2020). Proof of concept of an eclectic, integrative therapeutic approach to mental health and well-being through virtual reality technology. Front. Psychol. 11, 858. doi:10.3389/fpsyg.2020.00858
George, C., Demmler, M., and Hussmann, H. (2018). “Intelligent interruptions for IVR:investigating the interplay between presence, workload and attention,” in Extended Abstracts of the 2018 CHI Conference on Human Factors in Computing Systems, Montreal, QC, Canada, April 21-26, 2018, 1–6. Association for Computing Machinery.
Gershon, J., Zimand, E., Pickering, M., Rothbaum, B. O., and Hodges, L. (2004). A pilot and feasibility study of virtual reality as a distraction for children with cancer. J. Am. Acad. Child Adolesc. Psychiatry 43 (10), 1243–1249. doi:10.1097/01.chi.0000135621.23145.05
Han, J., Zheng, Q., and Ding, Y. (2021). Lost in virtual reality? Cognitive load in high immersive VR environments. J. Adv. Inf. Technol. 12 (4). doi:10.12720/jait.12.4.302-310
Hart, S. G., and Staveland, L. E. (1988). “Development of NASA-TLX (task load index): results of empirical and theoretical research,” in Advances in psychology (vol. 52) (Amsterdam, Netherlands: Elsevier), 139–183. doi:10.1016/S0166-4115(08)62386-9
ISO 17488:2016 (2022) ISO. Available at: https://www.iso.org/cms/render/live/en/sites/isoorg/contents/data/standard/05/98/59887.
Klimmt, C., Hartmann, T., Gysbers, A., and Vorderer, P. (2005). “The value of reaction-time measures in presence research: empirical findings and future perspectives,” in 8th International Workshop on Presence, London, UK, September 21-23, 2005, 293–298.
Knaust, T., Felnhofer, A., Kothgassner, O. D., Höllmer, H., Gorzka, R. J., and Schulz, H. (2022). Exposure to virtual nature: the impact of different immersion levels on skin conductance level, heart rate, and perceived relaxation. Virtual Real. 26 (3), 925–938. doi:10.1007/s10055-021-00595-2
Lemmens, J. S., Simon, M., and Sumter, S. R. (2022). Fear and loathing in VR: the emotional and physiological effects of immersive games physiological effects of immersive games. Virtual Real. 26 (1), 223–234. doi:10.1007/s10055-021-00555-w
Lombard, M., and Ditton, T. (1997). At the heart of it all: the concept of presence. J. Computer-Mediated Commun. 3 (2), 0. doi:10.1111/j.1083-6101.1997.tb00072.x
Mestre, D., and Vercher, J. (2011). “Chapter 5: immersion and presence,” in Virtual reality: concepts and technologies, 93–102.
Miguel-Alonso, I., Rodriguez-Garcia, B., Checa, D., and Bustillo, A. (2023). Countering the novelty effect: a tutorial for immersive virtual reality learning environments. Appl. Sci. 13 (1), 593. doi:10.3390/app13010593
Nilsson, E. J., Aust, M. L., Engström, J., Svanberg, B., and Lindén, P. (2018). Effects of cognitive load on response time in an unexpected lead vehicle braking scenario and the detection response task (DRT). Transp. Res. Part F Traffic Psychol. Behav. 59, 463–474. doi:10.1016/j.trf.2018.09.026
Nilsson, N., Nordahl, R., and Serafin, S. (2016). Immersion revisited: a review of existing definitions of immersion and their relation to different theories of presence. Hum. Technol. 12, 108–134. doi:10.17011/ht/urn.201611174652
Nordahl, R., and Korsgaard, D. (2010). Distraction as a measure of presence: using visual and tactile adjustable distraction as a measure to determine immersive presence of content in mediated environments. Virtual Real. 14 (1), 27–42. doi:10.1007/s10055-009-0140-3
Norman, D. A., and Bobrow, D. G. (1975). On data-limited and resource-limited processes. Cogn. Psychol. 7 (1), 44–64. doi:10.1016/0010-0285(75)90004-3
O’Brien, H. L., Cairns, P., and Hall, M. (2018). A practical approach to measuring user engagement with the refined user engagement scale (UES) and new UES short form. Int. J. Human-Computer Stud. 112, 28–39. doi:10.1016/j.ijhcs.2018.01.004
Ochadleus, C., Kirby, C., and Scollon, C. N. (2023). It’s awe-fully unfamiliar: the effect of familiarity on awe within a virtual reality setting. Front. Psychol. 14, 1096283. doi:10.3389/fpsyg.2023.1096283
Pausch, R., Proffitt, D., and Williams, G. (1997). Quantifying immersion in virtual reality. Proc. 24th Annu. Conf. Comput. Graph. Interact. Tech. - SIGGRAPH 97, 13–18. doi:10.1145/258734.258744
Regenbrecht, H., and Schubert, T. (2002). Real and illusory interactions enhance presence in virtual environments virtual environments. Presence Teleoperators Virtual Environ. 11 (4), 425–434. doi:10.1162/105474602760204318
Robinson, M. K., Ellis, D. M., Pitaes, M. M., Karoly, P., and Brewer, G. A. (2021). Acute pain impairs sustained attention. J. Exp. Psychol. Appl. 27 (3), 563–577. doi:10.1037/xap0000356
Sarkar, T. D., Edwards, R. R., and Baker, N. (2022). The feasibility and effectiveness of virtual reality meditation on reducing chronic pain for older adults with knee osteoarthritis reality meditation on reducing chronic pain for older adults with knee osteoarthritis. Pain Pract. 22 (7), 631–641. doi:10.1111/papr.13144
Schubert, T. W. (2003). The sense of presence in virtual environments. Z. Für Medien. 15 (2), 69–71. doi:10.1026//1617-6383.15.2.69
Schwarz, N. (1999). Self-reports: how the questions shape the answers. Am. Psychol. 54, 93–105. doi:10.1037//0003-066x.54.2.93
Slater, M., and Usoh, M. (1993). Representations systems, perceptual position, and presence in immersive virtual environments. Presence Teleoperators Virtual Environ. 2 (3), 221–233. doi:10.1162/pres.1993.2.3.221
Stojmenova, K., and Sodnik, J. (2018). Detection-response task—uses and limitations. Sensors 18 (2), 594. doi:10.3390/s18020594
Tieri, G., Morone, G., Paolucci, S., and Iosa, M. (2018). Virtual reality in cognitive and motor rehabilitation: facts, fiction and fallacies rehabilitation: facts, fiction and fallacies. Expert Rev. Med. devices 15 (2), 107–117. doi:10.1080/17434440.2018.1425613
van Baren, J., and Ijsselsteijn, W. (2004). “Measuring presence: a guide to current measurement approaches,” in Deliverable 5 of the OmniPres project IST-2001-39237 (Brussels, Belgium: Eur. Commission).
Wickens, C. D. (2008). Multiple resources and mental workload. Hum. Factors 50 (3), 449–455. doi:10.1518/001872008X288394
Witmer, B. G., and Singer, M. J. (1998). Measuring presence in virtual environments: a presence questionnaire. Presence Teleoperators Virtual Environ. 7 (3), 225–240. doi:10.1162/105474698565686
Wu, H. K., Lee, S. W. Y., Chang, H. Y., and Liang, J. C. (2013). Current status, opportunities and challenges of augmented reality in education. Comput. Educ. 62, 41–49. doi:10.1016/j.compedu.2012.10.024
Zhang, C. (2020). The why, what, and how of immersive experience. IEEE Access 8, 90878–90888. doi:10.1109/access.2020.2993646
Zhang, C., Zadtootaghaj, S., Hoel, A., and Perkis, A. (2018). “How long is long enough to induce immersion? Comparing the immersiveness of three variations of spatial immersion,” in Proceedings of the 10th International Conference on Quality of Multimedia Experience (QoMEX 2018). 10th International Conference on Quality of Multimedia Experience (QoMEX 2018), Sardinia, Sardinia, Italy, June 2018.
Keywords: virtual reality, immersion, presence, secondary task, detection-response task
Citation: Payne E, Battel K, Baker N and Ward N (2024) Considerations when trying to quantify immersion with secondary tasks. Front. Virtual Real. 5:1212216. doi: 10.3389/frvir.2024.1212216
Received: 25 April 2023; Accepted: 26 April 2024;
Published: 13 May 2024.
Edited by:
Thuong Hoang, Deakin University, AustraliaReviewed by:
William L. Raffe, Deakin University, AustraliaMurielle Gabriela Kluge, The University of Newcastle, Australia
Copyright © 2024 Payne, Battel, Baker and Ward. This is an open-access article distributed under the terms of the Creative Commons Attribution License (CC BY). The use, distribution or reproduction in other forums is permitted, provided the original author(s) and the copyright owner(s) are credited and that the original publication in this journal is cited, in accordance with accepted academic practice. No use, distribution or reproduction is permitted which does not comply with these terms.
*Correspondence: Emily Payne, ZXBheW5lMTIxNkBnbWFpbC5jb20=; Nathan Ward, bmF0aGFuLndhcmRAdHVmdHMuZWR1