- 1Univ Brest, Lab-STICC, CNRS, UMR, 6285, Brest, France
- 2Laboratoire de Cyberpsychologie, Département de psychoéducation et de psychologie, Université du Québec en Outaouais, Gatineau, QC, Canada
Introduction: This exploratory study aims to participate in the development of the VR framework by focusing on the issue of cybersickness. The main objective is to explore the possibilities of predicting cybersickness using i) field dependence-independence measures and ii) head rotations data through automatic analyses. The second objective is to assess the impact of cybersickness on visuomotor performance.
Methods: 40 participants completed a 13.5-min VR immersion in a first-person shooter game. Head rotations were analyzed in both their spatial (coefficients of variations) and temporal dimensions (detrended fluctuations analyses). Exploratory correlations, linear regressions and clusters comparison (unsupervised machine learning) analyses were performed to explain cybersickness and visuomotor performance. Traditional VR human factors (sense of presence, state of flow, video game experience, age) were also integrated.
Results: Results suggest that field dependence-independence measured before exposure to VR explain ¼ of the variance of cybersickness, while the Disorientation scale of the Simulator Sickness Questionnaire predicts 16.3% of the visuomotor performance. In addition, automatic analyses of head rotations during immersion revealed two different clusters of participants, one of them reporting more cybersickness than the other.
Discussion: These results are discussed in terms of sensory integration and a diminution of head rotations as an avoidance behavior of negative symptoms. This study suggests that measuring field dependence-independence using the (Virtual) Rod and Frame Test before immersion and tracking head rotations using internal sensors during immersion might serve as powerful tools for VR actors.
1 Introduction
At the beginning of the 21st century, virtual reality (VR) has spread to many fields, from industry, with applications in tourism (Beck et al., 2019), real estate (Brenner, 2017), sports and video games (Neumann et al., 2018), to society, with applications in journalism (Sirkkunen et al., 2016), education (Kavanagh et al., 2017), and history (Fleury and Madeleine, 2012), to sciences, with applications in philosophy of mind (Sanchez-Vives and Slater, 2005), social behaviors (Pan and Hamilton, 2018), and of course to health, with applications in psychological therapy (Riva, 2022), rehabilitation (Laver et al., 2017), or pain relief (Hitching et al., 2023). It is important to note that most of these applications revolve around the notion of the ecological dimension, the possibility that this technology presents of immersing an individual in a custom-made environment while keeping total experimental control over it (Parsons, 2015; Dawson and Marcotte, 2017). This is the case, for example, of the cognitive-behavioral therapy practitioner who wants to make their patient work on their fear of flying by immersing them in a plane, while being able to play on the scene variables that are the weather, the height, the noise, the disturbances … without having to actually accompany their patient on a plane (Scozzari and Gamberini, 2011; Miloff et al., 2019). Indeed, and as Bryson (2013) says, “VR” means “to have the effect of having concrete existence without actually having concrete existence”. It is because the virtual plane produces, in the phobic patient, the concrete effects of psycho-physiological anxiety that the therapist will be able to work on it without having to pay the costs of the concrete existence of such a situation. However, all the potential benefits promised by VR are still largely hindered by the appearance, during its use, of negative symptoms similar to those of motion sickness: cybersickness (Rebenitsch and Owen, 2016; Stanney et al., 2020b).
Cybersickness is the most famous, prevalent and problematic side effect of VR, often suggested as being a “visually induced motion sickness” (LaViola, 2000; Bos et al., 2008). This phenomenon is experienced by many if not most users at different levels, with some rare users too deeply affected to use the tool (Caserman et al., 2021). Cybersickness takes the form of symptoms such as headache, nausea, stomach awareness, disorientation, dizziness, vertigo, sweating, blurred vision, drowsiness and very rarely vomiting (Rebenitsch and Owen, 2016). Not only do cybersickness symptoms slow down the use and development of VR, but they also lead to potential biases inherent to the tool. For example, the symptoms of cybersickness seem to have a negative impact on cognitive performance, notably spatial cognition (Gresty et al., 2008; Gresty and Golding, 2009; Mittelstaedt et al., 2019; Maneuvrier et al., 2020). Then, if the impairment caused by cybersickness is not properly measured, it could lead to erroneous measurement of performance, for example, for VR diagnosis of neurodegenerative diseases, where spatial cognition is often impaired (Parnetti and Calabresi, 2006; 2006; Possin, 2010). As a consequence, cybersickness is still considered an urgent problem to be understood, and solved (Stanney et al., 2020b).
Numerous theories try to explain cybersickness. The most famous family of theories used to explain cybersickness are the “sensory conflict theories” initially presented by Reason and Brand (1975). In these views, it is the incongruence between the information of different sensory modalities (e.g., visual and vestibular systems, non-vestibular proprioceptors), also called the sensory or perceptive mismatch, that triggers the symptoms. This theory is somehow compatible with the poison theory of Treisman (1977). Treisman states that evolutionary processes identify the sensory mismatch as the result of the ingestion of toxins and tries to expel them from the body by provoking nausea and vomiting symptoms. Another common theory is the theory of the subjective vertical conflict (Bles et al., 1998; Bos et al., 2008; Chung and Barnett-Cowan, 2023). In these views, it is only the sensory conflicts that concern the perception of the vertical (gravity) that will trigger negative symptoms. Thus, this theory predicts that user movement along the pitch and roll axes should trigger more cybersickness than movements along the yaw axis. More recently, Palmisano et al. (2020, 2022) have suggested that cybersickness is caused by display lag induced by differences between the virtual and physical poses of the participant’s head, independently from the vertical. Another major theory is the postural instability (Riccio and Stoffregen, 1991; Stoffregen and Smart, 1998). In these views, postural instability precedes the emergence of negative symptoms: cybersickness emerges not with the sensory mismatch but rather with the difficulty of maintaining postural stability.
Cybersickness is mostly measured using subjective methods, and despite recent critics (Sevinc and Berkman, 2020; Bouchard et al., 2021) the most frequent questionnaire is the Simulator Sickness Questionnaire (SSQ), developed by Kennedy & al. (1993). Other tools have been developed, notably the Virtual Reality Sickness Questionnaire by Kim et al. (2018). An important problem is that post-hoc self-reported measures do not consider the dynamic nature of cybersickness, which might be of crucial importance to understand its psychophysiology. This is why the Fast Motion Sickness Scale is also used: it consists of multiple self-ratings (from 1 to 20) during the experiment. However, the multiple in virtuo measures of the FMSS lead to multiple breaks in presence (Slater et al., 2003), and might be considered as leading to non-ecological VR experience. In order to overcome the limitations of the subjective methods, physiological, postural and behavioral measures along with classifications and other machine learning methods have been used to measure and predict cybersickness in VR (Bailey et al., 2022; Hadadi et al., 2022; Yang et al., 2022). In these cases, subjective measures like the SSQ are used as regressors that physiological variables will try to predict, which is not without problems given the limitations of questionnaire bias: it is, for example, suggested that male participants report less cybersickness than female participants in order to appear strong (Rebenitsch and Owen, 2014; 2016). It is therefore important to remember that even the most complex models of physiological measurements can only predict participants’ own statements about cybersickness. Recently, many studies have explored the possibilities of predicting cybersickness scores, using either physiological data or oculomotor variables (Dennison et al., 2016; Garcia-Agundez et al., 2019; Islam et al., 2020; Wibirama et al., 2020), software and hardware factors (Rebenitsch and Owen, 2021), and perceived vection or changes in the subjective visual vertical (Nooij et al., 2017; Chung and Barnett-Cowan, 2023).
Many individual characteristics (or human factors) are suggested as modulating and/or moderating the appearance of cybersickness symptoms, for example, age (Jasper et al., 2023), the experience of video games and other virtual practices (Howarth and Hodder, 2008; Maneuvrier et al., 2020; 2022; Kourtesis et al., 2023), and obviously the sense of presence (Weech et al., 2019; Maneuvrier et al., 2020; 2022). Sense of presence is the qualia “of being there"(Heeter, 1992; Sheridan, 1992), essential to the ecological use of VR (Parsons, 2015; Cummings and Bailenson, 2016). However, we lack studies and theories to rule on a possible direction of causality between cybersickness and sense of presence (Weech et al., 2019). Similarly, there is little information on the link between negative symptoms and the state of flow, the state of optimal concentration on a task frequently measured in VR (Csikszentmihalyi, 1990; Bian et al., 2018; Yang and Zhang, 2022). This can be explained by the fact that all these psychological phenomena are measured subjectively post-immersion. On the contrary, a promising human factor measured before immersion using a behavioral tool is the Field Dependence-Independence continuum (FDI). FDI might be considered as a perceptive style revealing a more or less dominant use of visual cues among multisensory integration (Witkin et al., 1962). This cognitive and/or perceptive style has long been suggested as a defining trait in motion sickness susceptibility, and more recently of cybersickness susceptibility (Deich and Hodges, 1973; Kennedy, 1975; Maneuvrier et al., 2021). FDI is usually measured using the famous Rod and Frame Test where an individual with altered visual cues has to align a rod vertically. This is interesting since both the flexibility of the multisensory integration (re-weighting), and the perception of the subjective vertical are suggested as associated with cybersickness (Weech et al., 2020a; Maneuvrier et al., 2021; Chung and Barnett-Cowan, 2023). The idea is that individuals who dominantly use visual cues and/or are more sensitive to them and/or have more difficulty down-weighing them might be more susceptible to cybersickness (Fulvio et al., 2021).
Movement and/or the perceived illusion of movement, also called vection (Palmisano et al., 2015), seem to play a crucial role in the appearance of cybersickness in VR (Keshavarz et al., 2015). The main source of vection in VR comes from the optic flow: it is a common experience for a video game player to explore a virtual environment using a linear movement triggering an optic flow in order to simulate real-life walking. In VR, the strength of this vection is increased by the immersive first-person experience, which makes the static cues that a traditional gamer has access to disappear, such as the room behind the screen or even the edges of the screen. This explains why it is generally advisable in VR not to use linear movement as a means of locomotion (Clifton and Palmisano, 2019) even though an habituation is possible (Howarth and Hodder, 2008; Adhanom et al., 2022). Indeed, in many VR cases, the position of the head is tracked and used as a virtual 3D camera for rendering the virtual environment. As a result, an immobile VR user visually explores the environment by rotating the head along three axes of rotation: pitch, yaw, roll (Figure 1), in both their spatial and temporal dimensions. It is unsure how these head rotations directly and precisely relate to cybersickness (Rebenitsch and Owen, 2016). It is also unclear whether or not one axis is more provocative than other. What is known is that visual rotational oscillations (Bonato et al., 2009; Keshavarz and Hecht, 2011) and rotational movements are associated with the emergence of symptoms (Aykent et al., 2014; Palmisano et al., 2017; Arcioni et al., 2019; Islam et al., 2021; Porcino et al., 2022; Sumayli and Ye, 2023). In addition, “changing” vection seems to cause more negative effects than “steady” vection (Budhiraja et al., 2017). Mitigating vection also seems to reduce cybersickness, either by adding blur (Budhiraja et al., 2017), by adding a fixed visual frame (Kemeny et al., 2017), or by extrapolating head movements (Garcia-Agundez et al., 2017). The reason why head rotations in VR are associated with cybersickness depends on the theory: it is possible that because of head rotations a mismatch arises (due to tracking and latency) between the visual system and the other perceptive systems (Reason and Brand, 1975; Bos et al., 2008), or between the physical and virtual poses (Palmisano et al., 2020; 2022), just as it is possible for these rotations to impact the vertical subjective and the postural instability (Stoffregen and Smart, 1998; Chung and Barnett-Cowan, 2023).
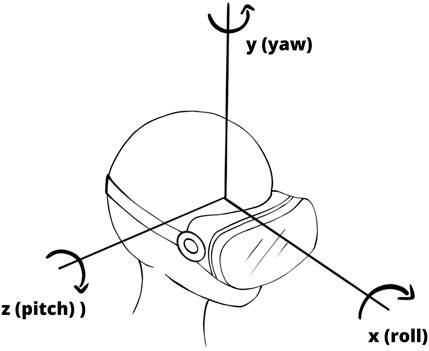
FIGURE 1. Graphical representation of the three axes of head rotations: x (roll), y (yaw), z (pitch).
Given that in most virtual spatial environments, head rotations are both necessary for visual exploration (tracked head rotations being the means of spatial exploration) and a potential source of cybersickness, it seems important to explore the question of their relationship, particularly with regard to visuomotor tasks. Indeed, hand-eye coordination is central to many processes in VR: beyond being the main game-play mechanism of many commercial successes, such as Beatsaber, Half-Life Alyx or The Lab, the use of VR for the evaluation and rehabilitation of visuomotor performance could well prove to be a new tool for cognitive and movement sciences (Carrieri et al., 2016; Choi et al., 2018; Pratviel et al., 2021; David et al., 2022; Grosprêtre et al., 2023), but also for health research and applications, for instance in the case of Parkinson’s disease (Eng et al., 2007; Seitz, 2014; Chen et al., 2020; Köster et al., 2021; Lahude et al., 2022). For example, the study of Pratviel & al. (2021) proposed the Dynavision task and showed its reliability and interest in order to assess participants’ visuomotor abilities using a light tracking paradigm. However, in the study of Pratviel et al. (2021), participants were facing a two-dimensional wall and were mostly immobile, which cannot be considered as an ecological experimentation. Thus, absence of vection and/or and head rotations may explain why cybersickness symptoms were not, in Pratviel et al. (2021), associated with visuomotor performance. On the contrary, during a task requiring large visual explorations of a spatial virtual environment (and thus triggering kinetogenic situations), we might expect a negative relationship between cybersickness and visuomotor performance, whether through visual fatigue (LaViola, 2000; Bos et al., 2008; Rebenitsch and Owen, 2016), cognitive fatigue and disorientation (Gresty et al., 2008; Gresty and Golding, 2009; Maneuvrier et al., 2020) detour of attentional resources to physiological symptoms, or allocation of these same attentional resources to the resolution/compensation of the psychophysiological state (Maneuvrier and Westermann, 2022).
We end up with an apparent paradox: in spatial environments, more head rotations (in both their spatial and temporal dimensions) should be associated with i) better visuomotor performance thanks to richer visual coordination and exploration, but also with ii) poorer visuomotor performance due to the deleterious effects of cybersickness. In order to explore this issue, we present an empirical study which aims to i) evaluate the impact of cybersickness on visuomotor performance and ii) predict cybersickness using head rotations and FDI. For the purposes of exploratory research and data generation, this study also proposes a correlational analysis of common VR human factors: namely, age, the sense of presence, the video game experience, and the state of flow. We expect visuomotor performance to be altered by the negative symptoms of cybersickness. In addition, we expect that head rotations, both in their spatial and temporal dimensions, will be predictors of cybersickness symptoms. Taken together, we aim to contribute to a better understanding of cybersickness and utilization of VR, with the ultimate goal of measuring, predicting and ultimately countering negative symptoms and their impact (Stanney et al., 2020a) during spatial environments. Indeed, the integrative and ecological benefits promised by VR, whether in the field of research, diagnosis or rehabilitation, require us to study the phenomena at play in virtual spatial environments and tasks. For this reason, this exploratory study focuses particularly on situations with visuomotor tasks and active head exploration by an almost-immobile user in a spatial environment, which is very common in VR applications and games (Maneuvrier et al., 2020; Barnett et al., 2022), but the results and discussions should be considered and/or applied to other situations.
2 Materials and methods
2.1 Participants
2.1.1 Recruitment
Participants were recruited through posters in the corridors of the first and second authors’ university. Exclusion criteria were: i) being under 18 or over 35 years of age, ii) having a known uncorrected psychological or physiological condition that could impair perception of a virtual environment and/or use of a visuomotor controller. Participants were not medically screened for these criteria, but were trusted by the experimenters. Those who could use corrective lenses or glasses in the head-mounted display were included.
2.1.2 Sample
40 participants (age 23.55 ± 4.03) were included in the definitive analysis sample. To the open-ended question “what sex were you assigned at birth?”, which was indicated as optional, 26 (age 23.03 ± 3.44) of them answered in the French lexical field “femme”, and 14 (age 24.5 ± 4.9) of them answered in the French lexical field “homme”. All participants lived in Western Europe and almost all of them were students at the University (average 2.85 ± 0.83 years of study after high school).
2.1.3 Ethics
All participants gave written consent and were considered by the experimenters in strict compliance with the Helsinki Convention (Declaration of Helsinki, 2001). The experimental protocol was validated by a local research ethics committee and the data management performed in conformity with the GDPR (Dove, 2018).
2.1.4 Experimenters
The lead experimenters were the first and second authors of the present study, along with other graduate students who did not want to pursue the process of written scientific production. Half of the experimenters identified themselves as men and half as women. Experiments were randomized and supervised preliminary data collection was carried out to ensure experimental consistency between experimenters, even though the protocol was highly computerized and automated.
2.2 VR visuomotor performance
The virtual shooting environment was custom created by the first author using Unity3D and the object-oriented programming language C#. The head-mounted display for immersion was the HTC-Vive Pro (1440 × 1600 resolution per eye, 98° horizontal field of view, 90 Hz refresh rate). The computer was running Windows 10–64-bit, its processor was an Intel Core i9 - 9900 K 3.6 GHz and it was equipped with 32 gigabytes of RAM. The graphics processing unit was a GeForce RTX 2080. This hardware ensured a consistent framerate above 60 frame per seconds.
Due to a mechanical technical problem with the head-mounted display hardware, the interpupillary distance could not be adjusted for each participant. However, the distance was set at 62.5 mm, which corresponds to the average interpupillary distance of men and women aged 16–40 in Northern Europe (Pointer, 1999), which is therefore equally suitable (or not) for both men and women, in contrast to the initial level of 67.5 mm of the head-mounted display favoring men (Stanney et al., 2020a).
After a brief tutorial explaining how to use the HTC Vive tracked controllers, participants were immersed in a Western-style cartoon world (Figure 2). They were placed on top of a moving train (Figure 2A) in order to achieve a linear, smooth optic flow that could trigger a very slight cybersickness (in order to prevent a basement effect) through a slight perceptual change (Clifton and Palmisano, 2019; Adhanom et al., 2022). The train’s path was straight to avoid the emergence of too much cybersickness, and the speed of the train was rather slow, 0.5 units of distance per second, where a unit is the size of the participant in the virtual environment (which corresponds to the visual optic flow of a slow walking pace).
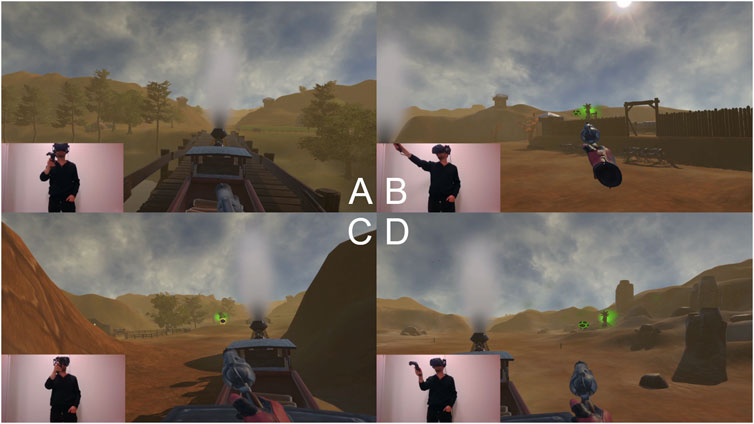
FIGURE 2. First person and third person views of the immersion in the virtual environment. (A) the moving train with no apparent target. (B) the hand and virtual pistol based on HTC-Vive controller tracking. (C) the participant firing a projectile (yellow sphere) at an enemy target. (D) an enemy firing a projectile (green sphere) at the moving train.
The tracked virtual controller was transformed into a gun that could fire one bullet per second using the trigger (Figure 2B). Instructions were given visually and orally by voice recording (male human voice) in the virtual environment. Participants were asked to defend the train which was being attacked en route (Figure 2C). The aliens appeared regularly (every 10 s) and were arranged pseudo-randomly (but identically for all participants) in a 180° arc in the direction of the train (Figures 2B, D). They started firing projectiles towards the train as soon as they appeared (and then every 1.5–2 s, randomly for each shot). The aliens lasted until they were neutralized or out of sight of the participants.
Participants could either shoot the projectiles to destroy them or shoot the aliens to neutralize them, with their only explicit objective being to protect the train. Total immersion lasted 13 min and 30 s, after which the virtual environment closed. Performance was measured by the number of enemy projectiles that actually hit the train.
The choice of a western shooter format game was made in order to use a classic popular culture scheme. This decision was made expecting an easy-to-induce sense of spatial presence and state of flow and an easy-to-implement ergonomics and affordances. In addition, this first-person shooter explores an attempt at the analysis of ecological integrated visuomotor performance in VR. Indeed, we assume that the future of ecological VR, whether for research purposes or applications (diagnostics, rehabilitation.) will involve highly integrative spatial environments, which requires us to study the phenomena at play during the latter. A video of the virtual task can be found in the Supplementary Materials.
2.3 Subjective measures
All the VR subjective variables and demographics data were measured post-immersion using computerized self-administered questionnaires.
Cybersickness was measured using the Simulator Sickness Questionnaire validated in French (Bouchard et al., 2007). However, traditional factoring from Kennedy et al. (1993) with three sub-scales was used. Nausea, oculomotor and disorientation scales were reported, as well as the overall score, in order to be compared with the validated international tools. In order to avoid suggesting an adverse effect, the pre-immersion baseline level was not measured, which was recently questioned (Brown et al., 2022).
Sense of presence was measured using the most common questionnaire validated in French (Witmer and Singer, 1998; Robillard et al., 2002), without haptic elements because the immersive system did not include the ability to explore the environment through touch. All other validated sub-scales were reported (Realism, Possibility to act, Quality of interface, Possibility to examine, Self-evaluation of performance, Sounds), as well as the overall score.
The state of flow was assessed using a translation of different items of the state of flow questionnaires used in VR, but translated into French as no validated version could be found. The overall score was used for reliability evaluation. As they are not validated in the literature, the items used for the state of flow questionnaire along with their English translation are given in the supplementary material.
Video game experience was measured using a single question: “How often do you play a video game?” on a 10-point scale, where 10 was “Every day” and 1 was “Never”. A 10-point scale was used rather than the standard 7-point Likert scale to avoid possible confusion with the number of days played per week.
2.4 Field dependence-independence
In order to assess its evolution during immersion (Maneuvrier et al., 2021), FDI was assessed before (FDIpre) and after immersion (FDIpost) using a custom made Virtual Rod and Frame Test built in Unity3D by the first author (Supplementary materials).
Placed in an upright sitting position with their feet not touching the ground, participants had to align, using the HTC Vive tracking controller, a rod initially inclined at either +27 or −27° from the gravitational vertical (0°) in a static frame inclined at either +18 or −18°. Sixteen trials were conducted, each combination being sequentially tested four times. For each trial, the absolute error from the gravitational vertical was measured in degrees.
The individual FDI level was measured using the mean absolute error. The higher the mean absolute error, the more the participant’s subjective visual vertical is influenced by the tilted frame and therefore the higher the level of FDI. The change in FDI (FDIev) was calculated using a ratio: FDIev = (FDIpost-FDIpre)/FDIpre. This last variable (FDIev) corresponded to the flexibility of the FDI. A video of the Virtual Rod and Frame Test is available in the supplementary materials, and the tool was made free to use by the authors.
2.5 Head rotations
Head rotations were measured by the HTC Vive headset integrated sensors associated to its base station. The Euler angles as reported by Unity3D with the SteamVR plugin were used, leading to 3 axes: x (roll), y (pitch), and z (yaw) (Figure 1). The origin (0, 0, 0) of the three axes was calibrated in the direction of train, aligned with the optic flow and the ground. Sampling rate of the recording was 15Hz, i.e. 45 measures (15 for each axis) by second. For each axis, 10 s of measures were systematically removed at the start and end of the recording when no enemies were spawning, in order to neutralize inter-individual variability in exploring the virtual environments and capture real “in action live” behavior. Thus, 11,850 frames in the 3 axes for each participant were considered for spatial and temporal analyses. Global angles of each frame of each axis, in degrees, were transformed into amount of rotation per frame (frame i1—frame i2). This was used (as absolute values) to build different measures allowing the calculation of the coefficients of variation (Standard deviation of rotation–average rotation per frame) in the three axes: x, y, z. These three coefficients of variation (x, y, z) were used as behavioral spatial quantification of head rotations during the virtual immersion (Lovie, 2005). Time series of head rotations in the three axes were also used for the temporal evaluation of head rotations using detrended fluctuation analysis (DFA, window sizes = 1000) in the three axes (Hardstone et al., 2012). DFA analyses are often used, for example, to detect distinct fractal perceptual-motor signatures.
2.6 Procedure
Participants were clearly informed of the experimental protocol and that they could stop the experiment at any time without explanation, but none chose to do so. Once written consent was obtained, participants were equipped with the VR headset and tracked controllers, and told that instructions would be given in the virtual environment. After performing the first part of the Virtual Rod and Frame test (FDIpre), the participants completed the 13.30 min long immersion in the first person shooter. Once the virtual visuomotor task completed, participants were un-equipped and asked to complete the VR self-reported computerized measures. Afterwards, the participants were briefly re-equipped and asked to perform the second part of the Virtual Rod and Frame Test (FDIpost). Finally, the participants were thanked, invited to ask questions and left the laboratory room.
2.7 Analyses
2.7.1 Outliers
JASP (0.17.1) and RStudio (2023.06) were used for the statistical analyses. First, we checked the data for outliers using the interquartile range (IQR) for each variable of interest (i.e., [25th percentile] - 1.5 x IQR and [75th percentile] + 1.5 x IQR), objectively and systematically removing each score out of the range. Due to the small sample size and the exploratory nature of the study, only outliers to the specific variable were removed, not the entire participant.
2.7.2 Descriptive analyses
Descriptive analyses were reported along with reliability analyses using McDonald’s Omega. Means and medians were reported along with their standard deviation: [mean; median ±standard deviation].
2.7.3 Correlation matrix
A global correlation matrix was calculated using Pearson’s r, mixing VR main variables (cybersickness subscales, visuomotor performance, FDIpre, FDIev), VR human factors (sense of presence, video game experience, sense of flow) and age.
2.7.4 Linear regressions
In order to assess the predictors of cybersickness and its impact on visuomotor performance, two exploratory linear regressions were performed. The first one with cybersickness (total SSQ) as the dependent variable, head rotations (Coef_X, Coef_Y, Coef_Z and DFA_X, DFA_Y, DFA_Z) and FDI variables (FDIpre and FDIev) as potential covariates, and the second one with visuomotor performance as the dependent variable and cybersickness variables (Nausea, Oculomotor and Disorientation scales) as potential covariates. Both were performed using the enter method based on the correlation matrix and previous findings: most potentially significant variables were added at each step and kept only if the global model’s p-value was below p = .05 and each predictor’s p-value below p = .05. Because of the sample size and the lack of theoretical basis, interactions were not considered.
2.7.5 Machine learning analyses
In order to apprehend the effect of head rotations on cybersickness in all three axes in both their spatial and temporal dimensions simultaneously, and to explore the possibilities of unsupervised machine learning analysis on this type of data to predict cybersickness, a cluster analysis was performed. The c-means clustering method was used. It is a soft clustering method that divides each data point into different groups, so that observations in the same group are similar and observations in different groups are more different (means center type, Hartigan-Wong algorithm, 25 max iterations and 25 random sets as recommended by JASP). Regressions or classifications algorithms were not used because of the small sample size: splitting the data into training, validation and testing groups would have considerably reduced the effectiveness of the algorithms. The clusters obtained were then compared on the cybersickness variables (Nausea, Oculomotor, Disorientation scales) using Mann-Whitney U test, in order to test the discriminant and predictive capacities of unsupervised algorithms.
2.7.6 Statistical method
Correlations coefficients were given by Pearson’s r, and effect size for Mann-Whitney U test by the rank biserial correlation. Effect size for linear regressions were given using the R2. Collinearity diagnostics were tested using the Variance Inflation Factor (VIF), and residuals autocorrelation using the Durbin-Watson test. Normality of residuals was screened using Q-Q plot standardized residuals and residuals histogram. 95% Confidence Intervals (95% CI) were systematically reported, along with p-values. As suggested by recent epistemological debates (Amrhein et al., 2019; Wasserstein et al., 2019), and because of the exploratory nature of the study, results were not discussed as significant or non-significant solely based on an arbitrary p-value threshold, but discussed with the combined help of different statistical estimators.
3 Results
3.1 Preliminary analyses
3.1.1 Visuomotor performance
After removing two outliers (x1 = 362, x2 = 246), the visuomotor performance variable revealed a distribution of [-59.65; −49.5 ± 36.96], which means that on average the participant let 59.65 enemy projectiles touch the train. Even though these variables were not considered in the analyses and are purely descriptive, on average the participants fired [832.7; 799 ± 167.59] shots, with an average accuracy (hitting either an enemy’s projectile or on the enemy rather than the background) of [53.1; 53.6 ± 7.9] %.
3.1.2 Subjective measures
One outlier on the disorientation scale score was removed (x1 = 88.24). Total self-reported scores of cybersickness (Total SSQ) revealed a distribution of [209.52; 164.59 ± 169.88], with [14.54; 19.08 ± 11.63] for the nausea scale, [19.89; 15.16 ± 18.22] for the oculomotor scale and [19.98; 13.92 ± 20.89] for the disorientation scale. Omega of McDonald’s for all items was: ω = 0.745, 95% CI[0.64, 0.82], and for all sub-scales: ω = 0.864, 95% CI[0.773, 0.924]. Considering this strong reliability score, the total SSQ scale was thus considered as a constructed variable called “cybersickness” in further analyses, when subscales were not considered.
Global self-reported scores of the sense of presence revealed a distribution of [108.9; 107 ± 12.06], with [35.17; 34.62 ± 5.84] for the realism scale, [19.92; 19 ± 2.64] for the possibility to act scale, [16.1; 16.5 ± 2.74] for quality of the interface scale, [11.15; 11.5 ± 2.3] for the possibility of examining scale, [10.27; 10 ± 1.26] for the self-evaluation of performance scale and [16.82; 17 ± 2.76] for the audio scale. Omega of McDonald’s for all items: ω = 0.83, 95% CI [0.75, 0.91], and for all subscales: ω = 0.75, 95% CI[0.61, 0.88]. Considering this strong reliability score, the total score of the sense of presence questionnaire was thus considered as a constructed variable called “sense of presence” in further analyses. Being a secondary variable, subscales of the sense of presence questionnaire were not considered in analyses.
The flow state questionnaire revealed a distribution of [67.87, 68 ± 8.5], and using the McDonald’s omega its items revealed a reliability of ω = 0.83, 95% CI [0.75, 0.91]. Considering this strong reliability score, the total score of the flow questionnaire was thus considered as a constructed variable called “state of flow” in further analyses.
The video game experience question revealed a distribution of [4.32; 4 ± 2.93]. Being a single-item question, this score was thus considered as a constructed variable called “video game experience” in further analyses.
3.1.3 Field (in)dependence
Four outliers were removed from the FDIpre scores (x1 = 10.42, x2 = 14.02, x3 = 15.08, x4 = 19.58). FDIpre scores revealed a distribution of [3.66; 3.08 ± 1.87] whereas FDIpost scores revealed a mean of [2.88; 2.56 ± 1.66]. FDI ratio evolution (FDIev) was [-0.36; −0.32 ± 0.32]. One outlier had to be removed from the FDIev score (x1 = 1.41). Wilcoxon signed-ranked outlined a global reduction of FDI during the immersion when comparing FDIpre and FDIpost: W = 572.5, z = 4.21, p < .001, Rank-Biserial Correlation = 0.81, 95% CI[0.64,0.91].
3.1.4 Head rotations
The distribution of head rotations per frame (15 Hz) measured during the immersion using the Euler Angles was [0.003; 0.003 ± 0.001) in the X-axis, [0.011; 0.011 ± 0.004] in the Y-axis and [0.002; 0.002 ± 0.0001] in the Z-axis. Concerning spatial analyses of head rotations, global sums of head rotations during immersion was [37.09; 35.41 ± 12.42] for X, [132.32; 133,14 ± 43.57] for Y and [26.95; 26.62 ± 9.6] for Z. Calculated coefficients of variation were [1.59; 1.56 ± 0.191] for the X-axis, [1.84; 1.85 ± 0.16] for the Y-axis, and [1.43; 1.45 ± 0.168] for the Z-axis. Concerning temporal analyses of head rotations, global DFA estimates were [0.76; 0.76 ± 0.036] for the X-axis, [0.75; 0.76 ± 0.33] for the Y-axis and [0.76; 0.76 ± 0.035] for the Z-axis.
3.2 Correlation matrix
For clarity reasons, three variables uncorrelated to other variables were removed from the correlation matrix: age, FDIev and video game experience. Correlation matrix can be found in Figure 3.
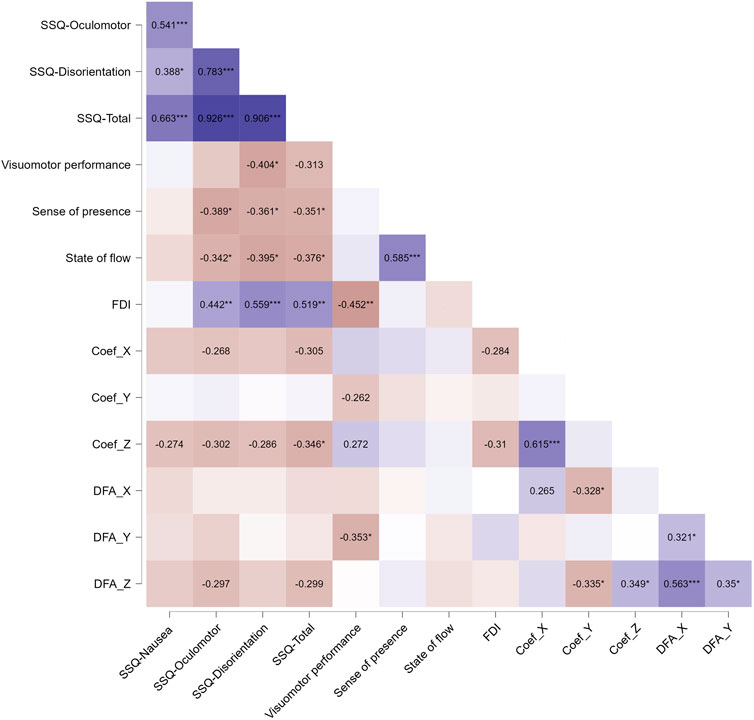
FIGURE 3. Graphical representation of the correlation matrix (Pearson’s r). An orangeish colour indicates a negative correlation and a bluish colour a positive correlation. The darker the colour, the stronger the correlation, and the lower the p-value. No indication means a p-value above 0.1. * = p < .05, ** = p < .01, *** = p < .001 Coef_i = coefficient of variation of head rotations in the i axis, DFA_i = Detrended fluctuation analysis estimate in the i axis.
3.3 Linear regressions
3.3.1 Cybersickness
The best model to explain cybersickness (total SSQ) that met the defined criterion explained 26.9% of variance in cybersickness (R2 = 0.269, F(1,34) = 12.53, p = .001). The only predictor was FDIpre (β = 0.519, t = 3.54, p < .001, 95% CI[20.47, 75.65]).
3.3.2 Visuomotor performance
The best model to explain visuomotor performance that met the defined criterion explained 16.3% of variance in visuomotor performance (R2 = 0.163, F(1,35) = 6.81, p = .013). The only predictor was the Disorientation scale (β = −0.404, t = −2.61, p = .013, 95% CI[-1.21, −0.15]).
3.4 Machine learning analyses
3.4.1 Unsupervised clustering
C-Means clustering analysis based on the head rotations variables (spatial and temporal) revealed two unequal clusters: C1 (N = 27) and C2 (N = 13). Global analysis (N = 40) metrics were: R2 = 0.308, AIC = 199, BIC = 219, Silhouette = 0.21. Descriptive statistics for the two clusters can be found in Table 1.
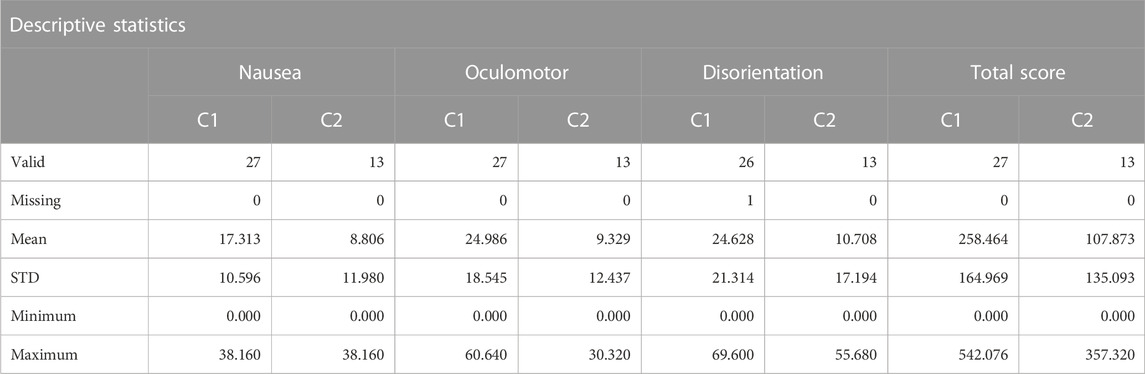
TABLE 1. Descriptive statistics of the two clusters split by the neighborhood-based algorithm. C1 = Cluster 1, C2 = Cluster 2. STD = Standard deviation.
3.4.2 Cluster comparisons
Mann-Whitney tests outline that the two clusters seem different on their total SSQ scale (W = 270, p = 0.006, Rank-Biserial Correlation = 0.541, 95% CI[0.21, 0.75]), on their Nausea scale (W = 253, p = 0.02, Rank-Biserial Correlation = 0.442, 95% CI[0.08, 0.69]), on their Oculomotor scale (W = 239, p = 0.007, Rank-Biserial Correlation = 0.53, 95% CI[0.2, 0.75]) and their Disorientation scale (W = 239, p = 0.032, Rank-Biserial Correlation = 0.41, 95% CI[0.05, 0.68]) (Figure 4).
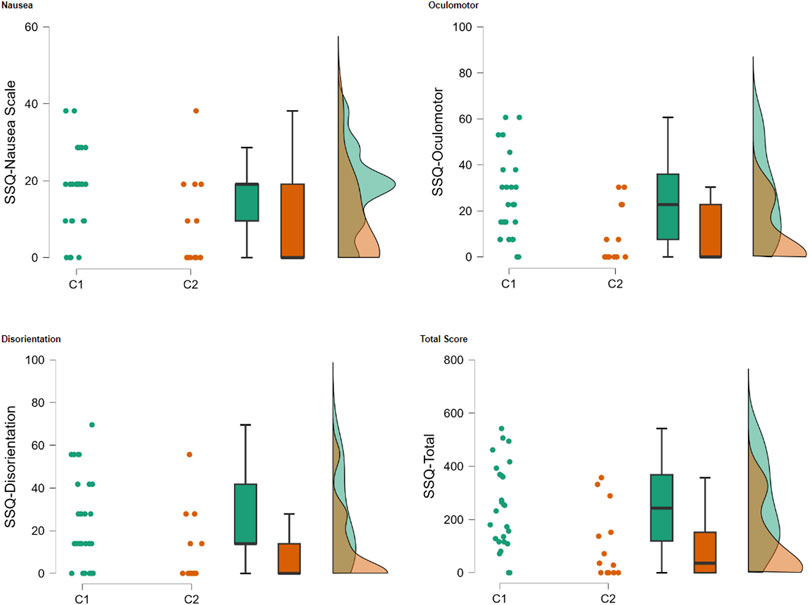
FIGURE 4. Graphical representations (Raincloud plots) of the distributions of the two clusters on cybersickness variables, C1 (N = 27) and C2 (n = 13) as split by a clustering unsupervised machine learning algorithm (c-means soft portioning method) on their spatial and temporal variables of head rotations in the three axes (pitch, yaw, roll).
4 Discussion
4.1 Impact of cybersickness
The empirical results of this study highlight the important impact of cybersickness in VR, even among young and (apparently) healthy adults during a relatively short immersion in a spatial virtual environment. Indeed, 16.3% of the variance of the visuomotor performance tasks was explained by the disorientation scale of the SSQ, which is understandable given the processes of the visuomotor virtual task involving a large amount of spatial cognition. It remains possible, however, to reverse the direction of the relationship and imagine that individuals with weaker visuomotor abilities are also, for one reason or another, more likely to present negative symptoms. However, results from previous studies seem to point in the direction of an impact of cybersickness on performance, for example, by inducing visual fatigue (Lambooij et al., 2009; Hirota et al., 2019), but most probably (regarding the disorientation scale) by deteriorating cognitive resources through spatial disorientation (Gresty et al., 2008; Gresty and Golding, 2009; Maneuvrier et al., 2020). Finally, even though 16.3% of the visuomotor performance variance may seem relatively small, we mustn't forget the high inter-variability of cybersickness and the threshold effect (Maneuvrier et al., 2020; Varmaghani et al., 2021): some individuals were probably much more affected than others. This effect should be controlled for rigorous use of VR, whether in research or for applications, as this part of variance explained by cybersickness very probably does not come from the phenomenon being tested. In addition, it is important to note that cybersickness as measured by the total SSQ was negatively associated with two important VR variables: the sense of presence and the state of flow. This appears to be in line with previous findings and theories (Weech et al., 2019; Maneuvrier et al., 2020), and seems rather logical. Indeed, when we consider the economy of attentional resources in VR (Draper and Blair, 1996; Draper et al., 1998), we can consider that individuals experiencing negative symptoms have to i) allocate attentional resources to symptom inhibition and/or regulation, ii) have their attentional resources directly altered by symptom salience and iii) have their attentional resources focus diverted from the virtual environment to the participant’s body (Maneuvrier and Westermann, 2022). All this reduces, both quantitatively and qualitatively, the amount of attentional resources available for the emergence and maintenance of a stable sense of presence and flow. However, the present study cannot infer directional causality about the sense of presence–cybersickness relationship, as both i) emerged at the same time during immersion and ii) were measured at the same time, sequentially, post immersion.
4.2 Field dependence-independence as predictor of cybersickness
On the contrary, FDI was measured before immersion, and explained more than ¼ of the variance of cybersickness. This suggests that the Virtual Rod and Frame Test assesses a cognitivo-perceptive profile more or less adapted to exposure to VR (Maneuvrier et al., 2021; Maneuvrier and Westermann, 2022). This result highlights the possibility of using FDI as a predictor of cybersickness in VR: it seems that the more field dependent an individual is, the more likely he or she is of experiencing negative symptoms in VR. This is in line with previous results or suggestions mostly based on correlations and/or motion sickness studies (Deich and Hodges, 1973; Kennedy, 1975; Mirabile et al., 1976; Maneuvrier et al., 2021; 2022). However, this is the first time (to our knowledge) that FDI scores measured before immersion by the Rod and Frame Test was able to predict such a high proportion of cybersickness. In comparison, cybersickness prediction models often require a large number of variables to explain more than 50% of the variance (Dennison et al., 2016; Islam et al., 2020; 2021; Chang et al., 2021; Rebenitsch and Owen, 2021; Yang et al., 2022; Jasper et al., 2023), whereas FDI, a single behavioral measure, explained 26.9% alone, which doesn't mean it cannot be combined with others. A possible explanation is that the Rod and Frame Test outlines some profiles and strategies more adapted to the VR sensory integration than other, probably because of i) better inhibition of non-congruent stimuli (Pithers, 2002; Evans et al., 2013; Jia et al., 2014) and ii) different use and flexibility in the using of visual cues (LaViola, 2000; Bos et al., 2008). These explanations are in line with recent papers which have suggested an association between sensory re-weighting and susceptibility to cybersickness (Weech et al., 2020a; Fulvio et al., 2021; Chung and Barnett-Cowan, 2023). It is interesting to note that the effect of the dynamic evolution of field (in)dependence during immersion, which seemed to be crucial in previous study as it was found to be associated with the subjective experience of VR (Maneuvrier et al., 2021), was not replicated, even though we measured a global reduction of FDI. Beyond a result of statistical artifacts, differences in the way of locomotion between the two studies and the resulting different optic flow could be leads of explanation (Clifton and Palmisano, 2019).
4.3 Head rotations and unsupervised machine learning analyses
While none of the head rotations variables taken independently seemed to explain cybersickness, when considered together they revealed an interesting result. Indeed, the clustering analysis based on the data of head rotations (in both their spatial and temporal aspects) split the participants in two groups, with one group reporting more cybersickness than the other. Since the individuals experiencing more cybersickness also registered less head rotations, this effect can be interpreted as an avoidance behavior: whether consciously or not, participants experiencing symptoms of cybersickness due to their rotational movements reduced these movements in order to alleviate the symptoms. Combined with other recent findings (Padmanaban et al., 2018; Li et al., 2019; Hadadi et al., 2022; Porcino et al., 2022; Yang et al., 2022; Asbee et al., 2023), this result amplifies the very promising aspect of automatic assessment of cybersickness. This method offers several advantages. Firstly, frame-by-frame analysis of head rotations allows us to consider a dynamic aspect inherent to cybersickness, which is impossible to assess using post-hoc questionnaire. In addition, automatic evaluation makes it possible to aggregate several variables (head rotations in their spatial and temporal components in the three axes) whose holistic aspect is difficult to detect otherwise. Finally, unsupervised learning enables the detection of patterns (for example, distinct fractal motor signatures) that are impossible to see with the naked eye and difficult to account for using more traditional statistics. The disadvantage is, of course, that these patterns are difficult to recognize and therefore to understand: for example, it becomes impossible to estimate how and when rotations in certain axes contribute more to cybersickness than others, which might be crucial to theoretical understanding (Palmisano et al., 2015; 2022).
4.4 Human factors of VR
Contrary to other recent studies, field (in)dependence levels were associated with neither the sense of presence nor the video game experience (Maneuvrier et al., 2021; 2022). It is unclear why, since the strong association between field (in)dependence and video game experience found in Maneuvrier et al. (2022), which should be independent from the virtual environment, was not replicated in the present study. This could be due to the sample sizes and effect sizes threshold, to statistical artefacts or to differences in the sensory processes of the virtual environments. Still, it is surprising to see that the video game experience was not associated with any other variable, not even the sense of presence nor the cybersickness, despite being regularly considered as an important human factor of VR (Lachlan & Krcmar, 2011; Weech et al., 2020b). Indeed, video game experience is often associated with a VR favorable experience because of i) a sensory mismatch habituation (Howarth and Hodder, 2008) and ii) common affordances and cognitive schemes (Gibson, 1966; Flach and Holden, 1998; Maneuvrier and Westermann, 2022). One could defend that the one-item question measuring video game experience was too little informative to differentiate the very different types of video games, for example, between casual puzzle games on smartphone and first person intensive shooters games (Bosser and Nakatsu, 2006; Baniqued et al., 2013; Kapalo et al., 2015). Concerning the state of flow, its positive relationship with the sense of presence replicated here is well documented (Draper and Blair, 1996; Draper et al., 1998; Bystrom et al., 1999; Faiola et al., 2013; Yang and Zhang, 2022). However, it is surprising to see that the state of flow was not associated with the visuomotor performance nor FDI, contrary to other studies (Bian et al., 2018; 2020). It can be speculated that the levels of difficulty were not adapted and that some individuals found the task too easy or too difficult to trigger an effective state of flow (Csikszentmihalyi, 1990). It is also possible that the flow questionnaire items taken in their entirety and its hand-crafted translation were not sufficiently relevant. Finally, a word must be said about the total absence of association between age and VR variables, which is easily explained by the very small age gaps in the sample.
4.5 Limits and criticisms
The main “limitation” of this study is the sample size, which is the reason for its exploratory nature. This study was initially part of a larger protocol which could not be fully completed because of the SARS-CoV-2 epidemic and the resources allocated (material and time). In accordance with the Open Science guidelines, we consider that this empirical study cannot truly test null hypothesis and infer causality: not only the experimentation was not pre-registered, but no a priori power calculations were performed for this specific hypothesis and sample size. Considering this, we call for confirmatory studies and we appeal to readers’ critical consideration of the empirical results. However, based on the results of previous studies and statistical estimators, we estimate an 80% chance of verisimilitude for these exploratory results (Goodman, 2018). In addition, we think this study and data should be publicly known in order to prevent publication biases (Rothstein, 2008; Fragkos et al., 2014). Other criticisms can be made on various subjects: the flow questionnaire, which was hand-crafted, and the question measuring video game frequencies, which might not be discriminant enough. In addition, the basal level of negative symptoms was not measured for cybersickness in order to avoid creating suggestion effects, which may have been a mistake, even though a suggestive effect could be argued (Brown et al., 2022). What’s more, the impossibility of precisely adapting the interpupillary distance may have been detrimental to some participants, both in terms of cybersickness and visuomotor performance (Stanney et al., 2020a). Last but not least, a big gap in this study that must be addressed in future studies, is the integration of the head-mounted display’s 6 degrees of freedom, not just rotations (3°). The combined study of all 6 degrees of freedom would enable us to go much further in our analyses, particularly with regard to unsupervised machine learning detection.
4.6 Future studies and applications
First, if these results are replicated, they could lead to the use of the Virtual Rod and Frame test as a predictive tool for VR experience. Indeed, it is a relatively inexpensive tool in time, money and energy is and rather easy to implement in VR. Sessions are short and easily automated as 16 trials seem to be enough. Secondly, assessing cybersickness using head rotations data offers several pragmatical advantages. Compared to physiological or demographics data, head rotations measures are inherent to VR: the sensors used are the same as those used for the visual display and can be found on every immersive system. They are also easy to implement, for example, as we did in this study using the SteamVR plugin in Unity3D. In the future, collaborative VR toolboxes implementing these measures could largely facilitate the study and/or controlling of negative symptoms. Indeed, systematic, easy and automatic assessments of cybersickness would greatly advance the field, not only by providing a better understanding of the phenomenon, but also by making it possible to neutralize it. This is fundamental when we consider their impact on performance and sense of presence. Indeed, and as we have previously called (Maneuvrier et al., 2020; 2022), neutralizing or at least assessing the psychophysiological effects of VR is a prerequisite for its methodologically rigorous use, in particular with the aim of carrying out more ecological research, diagnostics and rehabilitation in integrative and ecological spatial environments. At a more fundamental level, it would be particularly interesting to take a closer look at possible patterns associated with cybersickness, for example, in non-linear fractal analyses (Renaud et al., 2000; 2002; Bradley and Kantz, 2015; Tan et al., 2021). Indeed, the data procured by VR devices are particularly adapted to these types of analyses.
Data availability statement
The raw data supporting the conclusion of this article will be made available by the authors, without undue reservation.
Ethics statement
The studies involving humans were approved by the CICPPR—Comité institutionnel consultatif pour la protection des personnes dans la recherche. The studies were conducted in accordance with the local legislation and institutional requirements. The participants provided their written informed consent to participate in this study.
Author contributions
AM: Conceptualization, Data curation, Investigation, Methodology, Software, Supervision, Writing–original draft, Writing–review and editing. N-D-TN: Data curation, Investigation, Writing–original draft, Writing–review and editing. PR: Conceptualization, Methodology, Resources, Validation, Writing–review and editing.
Funding
The author(s) declare that no financial support was received for the research, authorship, and/or publication of this article.
Acknowledgments
The author would like to thank Nathalie LeBigot for her important help with logistics, materials and beta-testing, all the following graduate students for their participation as experimenters and beta-testers (Alan Grysole, Laurent Lansonneur, Lou-Anna Le Guen, Luca Mourgaud, Magali Hamard, Nawël Schappacher, Tristan Feutren, Christine Tupaj), and all the anonymous participants.
Conflict of interest
The authors declare that the research was conducted in the absence of any commercial or financial relationships that could be construed as a potential conflict of interest.
Publisher’s note
All claims expressed in this article are solely those of the authors and do not necessarily represent those of their affiliated organizations, or those of the publisher, the editors and the reviewers. Any product that may be evaluated in this article, or claim that may be made by its manufacturer, is not guaranteed or endorsed by the publisher.
References
Adhanom, I., Halow, S., Folmer, E., and MacNeilage, P. (2022). VR sickness adaptation with ramped optic flow transfers from abstract to realistic environments. Front. Virtual Real. 3, 848001. doi:10.3389/frvir.2022.848001
Amrhein, V., Greenland, S., and McShane, B. (2019). Scientists rise up against statistical significance. Nature 567 (7748), 305–307. doi:10.1038/d41586-019-00857-9
Arcioni, B., Palmisano, S., Apthorp, D., and Kim, J. (2019). Postural stability predicts the likelihood of cybersickness in active HMD-based virtual reality. Displays 58, 3–11. doi:10.1016/j.displa.2018.07.001
Asbee, J., Kelly, K., McMahan, T., and Parsons, T. D. (2023). Machine learning classification analysis for an adaptive virtual reality Stroop task. Virtual Real. 27, 1391–1407. doi:10.1007/s10055-022-00744-1
Aykent, B., Merienne, F., Guillet, C., Paillot, D., and Kemeny, A. (2014). Motion sickness evaluation and comparison for a static driving simulator and a dynamic driving simulator. Proc. Institution Mech. Eng. Part D J. Automob. Eng. 228 (7), 818–829. doi:10.1177/0954407013516101
Bailey, G. S., Arruda, D. G., and Stoffregen, T. A. (2022). Using quantitative data on postural activity to develop methods to predict and prevent cybersickness. Front. Virtual Real. 3. doi:10.3389/frvir.2022.1001080
Baniqued, P. L., Lee, H., Voss, M. W., Basak, C., Cosman, J. D., DeSouza, S., et al. (2013). Selling points: what cognitive abilities are tapped by casual video games? Acta Psychol. 142 (1), 74–86. doi:10.1016/j.actpsy.2012.11.009
Barnett, M. D., Chek, C. J. W., Shorter, S. S., and Parsons, T. D. (2022). Comparison of traditional and virtual reality-based episodic memory performance in clinical and non-clinical cohorts. Brain Sci. 12 (8), 1019. Article 8. doi:10.3390/brainsci12081019
Beck, J., Rainoldi, M., and Egger, R. (2019). Virtual reality in tourism: a state-of-the-art review. Tour. Rev. 74 (3), 586–612. doi:10.1108/TR-03-2017-0049
Bian, Y., Yang, C., Zhou, C., Liu, J., Gai, W., Meng, X., et al. (2018). Exploring the weak association between flow experience and performance in virtual environments. Proc. 2018 CHI Conf. Hum. Factors Comput. Syst. 1-12. doi:10.1145/3173574.3173975
Bian, Y., Zhou, C., Chen, Y., Zhao, Y., Liu, J., and Yang, C. (2020). The role of the field dependence-independence construct on the flow-performance link in virtual reality. Symposium Interact. 3D Graph. Games, 1–9. doi:10.1145/3384382.3384529
Bles, W., Bos, J. E., de Graaf, B., Groen, E., and Wertheim, A. H. (1998). Motion sickness: only one provocative conflict? Brain Res. Bull. 47 (5), 481–487. doi:10.1016/S0361-9230(98)00115-4
Bonato, F., Bubka, A., and Palmisano, S. (2009). Combined pitch and roll and cybersickness in a virtual environment. Aviat. Space, Environ. Med. 80 (11), 941–945. doi:10.3357/asem.2394.2009
Bos, J. E., Bles, W., and Groen, E. L. (2008). A theory on visually induced motion sickness. Displays 29, 47–57. doi:10.1016/j.displa.2007.09.002
Bosser, A.-G., and Nakatsu, R. (2006). Hardcore gamers and casual gamers playing online together. Proc. 5th Int. Conf. Entertain. Comput., 374–377. doi:10.1007/11872320_53
Bouchard, S., Berthiaume, M., Robillard, G., Forget, H., Daudelin-Peltier, C., Renaud, P., et al. (2021). Arguing in favor of revising the simulator sickness questionnaire factor structure when assessing side effects induced by immersions in virtual reality. Front. Psychiatry 12, 739742. doi:10.3389/fpsyt.2021.739742
Bouchard, S., Robillard, G., and Renaud, P. (2007). Revising the factor structure of the simulator sickness questionnaire. Acte de colloque du Annu. Rev. CyberTherapy Telemedicine 5.
Bradley, E., and Kantz, H. (2015). Nonlinear time-series analysis revisited. Chaos Interdiscip. J. Nonlinear Sci. 25 (9), 097610. doi:10.1063/1.4917289
Brenner, A. (2017). Virtual reality: the game changer for residential real estate staging through increased presence. CMC Senior Theses Available at: https://scholarship.claremont.edu/cmc_theses/1471.
Brown, P., Spronck, P., and Powell, W. (2022). The simulator sickness questionnaire, and the erroneous zero baseline assumption. Front. Virtual Real. 3. doi:10.3389/frvir.2022.945800
Bryson, S. (2013). Virtual reality: a definition history - a personal essay. arXiv:1312.4322 [cs] Available at: http://arxiv.org/abs/1312.4322.
Budhiraja, P., Miller, M. R., Modi, A. K., and Forsyth, D. (2017). Rotation blurring: use of artificial blurring to reduce cybersickness in virtual reality first person shooters. arXiv:1710.02599). arXiv. doi:10.48550/arXiv.1710.02599
Bystrom, K.-E., Barfield, W., and Hendrix, C. (1999). A conceptual model of the sense of presence in virtual environments. Presence Teleoperators Virtual Environ. 8 (2), 241–244. doi:10.1162/105474699566107
Carrieri, M., Petracca, A., Lancia, S., Basso Moro, S., Brigadoi, S., Spezialetti, M., et al. (2016). Prefrontal cortex activation upon a demanding virtual hand-controlled task: a new frontier for neuroergonomics. Front. Hum. Neurosci. 10, 53. doi:10.3389/fnhum.2016.00053
Caserman, P., Garcia-Agundez, A., Gámez Zerban, A., and Göbel, S. (2021). Cybersickness in current-generation virtual reality head-mounted displays: systematic review and outlook. Virtual Real. 25 (4), 1153–1170. doi:10.1007/s10055-021-00513-6
Chang, E., Kim, H. T., and Yoo, B. (2021). Predicting cybersickness based on user’s gaze behaviors in HMD-based virtual reality. J. Comput. Des. Eng. 8 (2), 728–739. doi:10.1093/jcde/qwab010
Chen, W., Bang, M., Krivonos, D., Schimek, H., and Naval, A. (2020). An immersive virtual reality exergame for people with Parkinson’s disease. In K. Miesenberger, R. Manduchi, M. Covarrubias Rodriguez, and P. Peňáz (Éds.), Computers helping people with special needs (p. 138–145). Springer International Publishing. doi:10.1007/978-3-030-58796-3_18
Choi, W., Lee, J., Yanagihara, N., Li, L., and Kim, J. (2018). Development of a quantitative evaluation system for visuo-motor control in three-dimensional virtual reality space. Sci. Rep. 8 (1), 13439. Article 1. doi:10.1038/s41598-018-31758-y
Chung, W., and Barnett-Cowan, M. (2023). Sensory reweighting: a common mechanism for subjective visual vertical and cybersickness susceptibility. Virtual Real. 27, 2029–2041. doi:10.1007/s10055-023-00786-z
Clifton, J., and Palmisano, S. (2019). Effects of steering locomotion and teleporting on cybersickness and presence in HMD-based virtual reality. Virtual Real. 24, 453–468. doi:10.1007/s10055-019-00407-8
Csikszentmihalyi, M. (1990). Flow: the psychology of optimal experience. Book Club Edition. Harper & Row.
Cummings, J. J., and Bailenson, J. (2016). How immersive is enough? A meta-analysis of the effect of immersive technology on user presence. Media Psychol. 19 (2), 272–309. doi:10.1080/15213269.2015.1015740
David, E. J., Lebranchu, P., Perreira Da Silva, M., and Le Callet, P. (2022). What are the visuo-motor tendencies of omnidirectional scene free-viewing in virtual reality? J. Vis. 22 (4), 12. doi:10.1167/jov.22.4.12
Dawson, D. R., and Marcotte, T. D. (2017). Special issue on ecological validity and cognitive assessment. Neuropsychol. Rehabil. 27 (5), 599–602. doi:10.1080/09602011.2017.1313379
Declaration of Helsinki (2001). World medical association declaration of Helsinki. Ethical principles for medical research involving human subjects. Bull. World Health Organ. 79 (4), 373–374.
Deich, R. F., and Hodges, P. M. (1973). Motion sickness, field dependence, and levels of development. Percept. Mot. Ski. 36 (3), 1115–1120. doi:10.2466/pms.1973.36.3c.1115
Dennison, M. S., Wisti, A. Z., and D’Zmura, M. (2016). Use of physiological signals to predict cybersickness. Displays 44, 42–52. doi:10.1016/j.displa.2016.07.002
Dove, E. S. (2018). The EU general data protection regulation: implications for international scientific research in the digital era. J. Law Med. Ethics 46 (4), 1013–1030. doi:10.1177/1073110518822003
Draper, J. V., and Blair, L. M. (1996). Workload, flow, and telepresence during teleoperation. Proc. IEEE Int. Conf. Robotics Automation. doi:10.1109/ROBOT.1996.506844
Draper, J. V., Kaber, D. B., and Usher, J. M. (1998). Telepresence. Hum. Factors 40, 354–375. doi:10.1518/001872098779591386
Eng, K., Siekierka, E., Pyk, P., Chevrier, E., Hauser, Y., Cameirao, M., et al. (2007). Interactive visuo-motor therapy system for stroke rehabilitation. Med. Biol. Eng. Comput. 45 (9), 901–907. doi:10.1007/s11517-007-0239-1
Evans, C., Richardson, J. T. E., and Waring, M. (2013). Field independence: reviewing the evidence. Br. J. Educ. Psychol. 83 (Pt 2), 210–224. doi:10.1111/bjep.12015
Faiola, A., Newlon, C., Pfaff, M., and Smyslova, O. (2013). Correlating the effects of flow and telepresence in virtual worlds: enhancing our understanding of user behavior in game-based learning. Available at: https://scholarworks.iupui.edu/handle/1805/6148.
Flach, J. M., and Holden, J. G. (1998). The reality of experience: gibson’s way. Presence Teleoperators Virtual Environ. 7 (1), 90–95. doi:10.1162/105474698565550
Fleury, P., and Madeleine, S. (2012). Reviving ancient Rome: virtual reality at the service of cultural heritage. In M. Ioannides, D. Fritsch, J. Leissner, R. Davies, F. Remondino, and R. Caffo (Éds.), Progress in cultural heritage preservation (p. 159–169). Springer. doi:10.1007/978-3-642-34234-9_16
Fragkos, K. C., Tsagris, M., and Frangos, C. C. (2014). Publication bias in meta-analysis: confidence Intervals for rosenthal’s fail-safe number. Int. Sch. Res. Notices 2014, 1–17. doi:10.1155/2014/825383
Fulvio, J. M., Ji, M., and Rokers, B. (2021). Variations in visual sensitivity predict motion sickness in virtual reality. Entertain. Comput. 38, 100423. doi:10.1016/j.entcom.2021.100423
Garcia-Agundez, A., Reuter, C., Becker, H., Konrad, R., Caserman, P., Miede, A., et al. (2019). Development of a classifier to determine factors causing cybersickness in virtual reality environments. Games Health J. 8 (6), 439–444. doi:10.1089/g4h.2019.0045
Garcia-Agundez, A., Westmeier, A., Caserman, P., Konrad, R., and Göbel, S. (2017). An evaluation of extrapolation and filtering techniques in head tracking for virtual environments to reduce cybersickness. In M. Alcañiz, S. Göbel, M. Ma, M. Fradinho Oliveira, J. Baalsrud Hauge, and T. Marsh (Éds.), Serious games (p. 203–211). Springer International Publishing. doi:10.1007/978-3-319-70111-0_19
Goodman, S. N. (2018). How sure are you of your result? Put a number on it. Nature 564 (7734), 7. doi:10.1038/d41586-018-07589-2
Gresty, M. A., and Golding, J. F. (2009). Impact of vertigo and spatial disorientation on concurrent cognitive tasks. Ann. N. Y. Acad. Sci. 1164, 263–267. doi:10.1111/j.1749-6632.2008.03744.x
Gresty, M. A., Golding, J. F., Le, H., and Nightingale, K. (2008). Cognitive impairment by spatial disorientation. Aviat. Space, Environ. Med. 79 (2), 105–111. doi:10.3357/asem.2143.2008
Grosprêtre, S., Marcel-Millet, P., Eon, P., and Wollesen, B. (2023). How exergaming with virtual reality enhances specific cognitive and visuo-motor abilities: an explorative study. Cognitive Sci. 47 (4), e13278. doi:10.1111/cogs.13278
Hadadi, A., Guillet, C., Chardonnet, J.-R., Langovoy, M., Wang, Y., and Ovtcharova, J. (2022). Prediction of cybersickness in virtual environments using topological data analysis and machine learning. Front. Virtual Real. 3. doi:10.3389/frvir.2022.973236
Hardstone, R., Poil, S.-S., Schiavone, G., Jansen, R., Nikulin, V., Mansvelder, H., et al. (2012). Detrended fluctuation analysis: a scale-free view on neuronal oscillations. Front. Physiology 3, 450. doi:10.3389/fphys.2012.00450
Heeter, C. (1992). Being there: the subjective experience of presence. Presence Teleoperators Virtual Environ. 1 (2), 262–271. doi:10.1162/pres.1992.1.2.262
Hirota, M., Kanda, H., Endo, T., Miyoshi, T., Miyagawa, S., Hirohara, Y., et al. (2019). Comparison of visual fatigue caused by head-mounted display for virtual reality and two-dimensional display using objective and subjective evaluation. Ergonomics 62 (6), 759–766. doi:10.1080/00140139.2019.1582805
Hitching, R., Hoffman, H. G., Garcia-Palacios, A., Adamson, M. M., Madrigal, E., Alhalabi, W., et al. (2023). The emerging role of virtual reality as an adjunct to procedural sedation and anesthesia: a narrative review. J. Clin. Med. 12 (3), 843. Article 3. doi:10.3390/jcm12030843
Howarth, P. A., and Hodder, S. G. (2008). Characteristics of habituation to motion in a virtual environment. Displays 29 (2), 117–123. doi:10.1016/j.displa.2007.09.009
Islam, R., Desai, K., and Quarles, J. (2021). Cybersickness prediction from integrated HMD’s sensors: a multimodal deep fusion approach using eye-tracking and head-tracking data. 2021 IEEE International Symposium on Mixed and Augmented Reality (ISMAR), 31–40. doi:10.1109/ISMAR52148.2021.00017
Islam, R., Lee, Y., Jaloli, M., Muhammad, I., Zhu, D., Rad, P., et al. (2020). Automatic detection and prediction of cybersickness severity using deep neural networks from user’s physiological signals. 2020 IEEE International Symposium on Mixed and Augmented Reality (ISMAR), 400–411. doi:10.1109/ISMAR50242.2020.00066
Jasper, A., Sepich, N. C., Gilbert, S. B., Kelly, J. W., and Dorneich, M. C. (2023). Predicting cybersickness using individual and task characteristics. Comput. Hum. Behav. 146, 107800. doi:10.1016/j.chb.2023.107800
Jia, S., Zhang, Q., and Li, S. (2014). Field dependence-independence modulates the efficiency of filtering out irrelevant information in a visual working memory task. Neuroscience 278, 136–143. doi:10.1016/j.neuroscience.2014.07.075
Kapalo, K. A., Dewar, A. R., Rupp, M. A., and Szalma, J. L. (2015). Individual differences in video gaming: defining hardcore video gamers. Proc. Hum. Factors Ergonomics Soc. Annu. Meet. 59 (1), 878–881. doi:10.1177/1541931215591261
Kavanagh, S., Luxton-Reilly, A., Wuensche, B., and Plimmer, B. (2017). A systematic review of virtual reality in education. Themes Sci. Technol. Educ. 10 (2), 85–119.
Kemeny, A., George, P., Mérienne, F., George, P., Mérienne, F., Colombet, F., et al. (2017). New VR navigation techniques to reduce cybersickness. Electron. Imaging 29, 48–53. doi:10.2352/ISSN.2470-1173.2017.3.ERVR-097
Kennedy, R. S. (1975). Motion sickness questionnaire and field independence scores as predictors of success in naval aviation training. Aviat. Space, Environ. Med. 46 (11), 1349–1352.
Kennedy, R. S., Lane, N. E., Berbaum, K. S., and Lilienthal, M. G. (1993). Simulator sickness questionnaire: an enhanced method for quantifying simulator sickness. Int. J. Aviat. Psychol. 3 (3), 203–220. doi:10.1207/s15327108ijap0303_3
Keshavarz, B., and Hecht, H. (2011). Axis rotation and visually induced motion sickness: the role of combined roll, pitch, and yaw motion. Aviat. Space, Environ. Med. 82 (11), 1023–1029. doi:10.3357/asem.3078.2011
Keshavarz, B., Riecke, B. E., Hettinger, L. J., and Campos, J. L. (2015). Vection and visually induced motion sickness: how are they related? Front. Psychol. 6, 472. doi:10.3389/fpsyg.2015.00472
Kim, H. K., Park, J., Choi, Y., and Choe, M. (2018). Virtual reality sickness questionnaire (VRSQ): motion sickness measurement index in a virtual reality environment. Appl. Ergon. 69, 66–73. doi:10.1016/j.apergo.2017.12.016
Köster, H., Müller-Schmitz, K., Kolman, A. G. J., and Seitz, R. J. (2021). Deficient visuomotor hand coordination in normal pressure hydrocephalus. J. Neurology 268 (8), 2843–2850. doi:10.1007/s00415-021-10445-5
Kourtesis, P., Linnell, J., Amir, R., Argelaguet, F., and MacPherson, S. E. (2023). Cybersickness, cognition, & motor skills: the effects of music, gender, and gaming experience. IEEE Trans. Vis. Comput. Graph. 29 (5), 2326–2336. doi:10.1109/TVCG.2023.3247062
Lachlan, K., and Krcmar, M. (2011). Experiencing presence in video games: the role of presence tendencies, game experience, gender, and time spent in play. Commun. Res. Rep. 28 (1), 27–31. doi:10.1080/08824096.2010.518924
Lahude, A. B., Souza Corrêa, P., P Cabeleira, M. E., and Cechetti, F. (2022). The impact of virtual reality on manual dexterity of Parkinson’s disease subjects: a systematic review. Disabil. Rehabilitation Assistive Technol. 1-8, 1237–1244. doi:10.1080/17483107.2021.2001060
Lambooij, M., Fortuin, M., Heynderickx, I., Fortuin, M., Heynderickx, I., Ijsselsteijn, W., et al. (2009). Visual discomfort and visual fatigue of stereoscopic displays: a review. J. Imaging Sci. Technol. 53, 30201-1–30201-14. doi:10.2352/J.ImagingSci.Technol.2009.53.3.030201
Laver, K. E., Lange, B., George, S., Deutsch, J. E., Saposnik, G., and Crotty, M. (2017). Virtual reality for stroke rehabilitation. Cochrane Database Syst. Rev. 11, CD008349. doi:10.1002/14651858.CD008349.pub4
LaViola, J. J. (2000). A discussion of cybersickness in virtual environments. ACM SIGCHI Bull. 32 (1), 47–56. doi:10.1145/333329.333344
Li, Y., Liu, A., and Ding, L. (2019). Machine learning assessment of visually induced motion sickness levels based on multiple biosignals. Biomed. Signal Process. Control 49, 202–211. doi:10.1016/j.bspc.2018.12.007
Lovie, P. (2005). “Coefficient of variation,” in Encyclopedia of statistics in behavioral science (John Wiley & Sons, Ltd). doi:10.1002/0470013192.bsa107
Maneuvrier, A., Ceyte, H., Renaud, P., Morello, R., Fleury, P., and Decker, L. M. (2022). Virtual reality and neuropsychological assessment: an analysis of human factors influencing performance and perceived mental effort. Virtual Real. 27, 849–861. doi:10.1007/s10055-022-00698-4
Maneuvrier, A., Decker, L. M., Ceyte, H., Fleury, P., and Renaud, P. (2020). Presence promotes performance on a virtual spatial cognition task: impact of human factors on virtual reality assessment. Front. Virtual Real. 1. doi:10.3389/frvir.2020.571713
Maneuvrier, A., Decker, L. M., Renaud, P., Ceyte, G., and Ceyte, H. (2021). Field (In)dependence flexibility following a virtual immersion is associated with cybersickness and sense of presence. Front. Virtual Real. 2, 110. doi:10.3389/frvir.2021.706712
Maneuvrier, A., and Westermann, H. (2022). The phi angle: a theoretical essay on sense of presence, human factors, and performance in virtual reality. PRESENCE Virtual Augmented Real. 29, 141–169. doi:10.1162/pres_a_00359
Miloff, A., Lindner, P., Dafgård, P., Deak, S., Garke, M., Hamilton, W., et al. (2019). Automated virtual reality exposure therapy for spider phobia vs. in-vivo one-session treatment: a randomized non-inferiority trial. Behav. Res. Ther. 118, 130–140. doi:10.1016/j.brat.2019.04.004
Mirabile, C. S., Glueck, B. C., and Stroebel, C. F. (1976). Susceptibility to motion sickness and field dependence-independence as measured with the rod and frame test. Neuropsychobiology 2 (1), 45–51. doi:10.1159/000117528
Mittelstaedt, J. M., Wacker, J., and Stelling, D. (2019). VR aftereffect and the relation of cybersickness and cognitive performance. Virtual Real. 23 (2), 143–154. doi:10.1007/s10055-018-0370-3
Neumann, D. L., Moffitt, R. L., Thomas, P. R., Loveday, K., Watling, D. P., Lombard, C. L., et al. (2018). A systematic review of the application of interactive virtual reality to sport. Virtual Real. 22 (3), 183–198. doi:10.1007/s10055-017-0320-5
Nooij, S. A. E., Pretto, P., Oberfeld, D., Hecht, H., and Bülthoff, H. H. (2017). Vection is the main contributor to motion sickness induced by visual yaw rotation: implications for conflict and eye movement theories. PLOS ONE 12 (4), e0175305. doi:10.1371/journal.pone.0175305
Padmanaban, N., Ruban, T., Sitzmann, V., Norcia, A. M., and Wetzstein, G. (2018). Towards a machine-learning approach for sickness prediction in 360° stereoscopic videos. IEEE Trans. Vis. Comput. Graph. 24 (4), 1594–1603. doi:10.1109/TVCG.2018.2793560
Palmisano, S., Allison, R. S., and Kim, J. (2020). Cybersickness in head-mounted displays is caused by differences in the user’s virtual and physical head pose. Front. Virtual Real. 1, 24. doi:10.3389/frvir.2020.587698
Palmisano, S., Allison, R. S., Schira, M. M., and Barry, R. J. (2015). Future challenges for vection research: definitions, functional significance, measures, and neural bases. Front. Psychol. 6, 193. doi:10.3389/fpsyg.2015.00193
Palmisano, S., Allison, R. S., Teixeira, J., and Kim, J. (2022). Differences in virtual and physical head orientation predict sickness during active head-mounted display-based virtual reality. Virtual Real. 27, 1293–1313. doi:10.1007/s10055-022-00732-5
Palmisano, S., Mursic, R., and Kim, J. (2017). Vection and cybersickness generated by head-and-display motion in the Oculus Rift. Displays 46, 1–8. doi:10.1016/j.displa.2016.11.001
Pan, X., and Hamilton, A. F. de C. (2018). Why and how to use virtual reality to study human social interaction: the challenges of exploring a new research landscape. Br. J. Psychol. 109 (3), 395–417. doi:10.1111/bjop.12290
Parnetti, L., and Calabresi, P. (2006). Spatial cognition in Parkinson’s disease and neurodegenerative dementias. Cogn. Process. 7 (1), 77–78. doi:10.1007/s10339-006-0075-5
Parsons, T. D. (2015). Virtual reality for enhanced ecological validity and experimental control in the clinical, affective and social neurosciences. Front. Hum. Neurosci. 9, 660. doi:10.3389/fnhum.2015.00660
Pithers, R. (2002). Cognitive learning style: a review of the field dependent-field independent approach. J. Vocat. Educ. Train. 54 (1), 117–132. doi:10.1080/13636820200200191
Pointer, J. S. (1999). The far interpupillary distance. A gender-specific variation with advancing age. Ophthalmic & Physiological Opt. J. Br. Coll. Ophthalmic Opt. (Optometrists) 19 (4), 317–326. doi:10.1046/j.1475-1313.1999.00441.x
Porcino, T., Rodrigues, E. O., Bernardini, F., Trevisan, D., and Clua, E. (2022). Identifying cybersickness causes in virtual reality games using symbolic machine learning algorithms. Entertain. Comput. 41, 100473. doi:10.1016/j.entcom.2021.100473
Possin, K. L. (2010). Visual spatial cognition in neurodegenerative disease. Neurocase 16 (6), 466–487. doi:10.1080/13554791003730600
Pratviel, Y., Deschodt-Arsac, V., Larrue, F., and Arsac, L. M. (2021). Reliability of the Dynavision task in virtual reality to explore visuomotor phenotypes. Sci. Rep. 11 (1), 587. Article 1. doi:10.1038/s41598-020-79885-9
Rebenitsch, L., and Owen, C. (2014). Individual variation in susceptibility to cybersickness. Proc. 27th Annu. ACM Symposium User Interface Softw. Technol., 309–317. doi:10.1145/2642918.2647394
Rebenitsch, L., and Owen, C. (2016). Review on cybersickness in applications and visual displays. Virtual Real 20 (2), 101–125. doi:10.1007/s10055-016-0285-9
Rebenitsch, L., and Owen, C. (2021). Estimating cybersickness from virtual reality applications. Virtual Real. 25 (1), 165–174. doi:10.1007/s10055-020-00446-6
Renaud, P., Bouchard, S., and Proulx, R. (2002). Behavioral avoidance dynamics in the presence of a virtual spider. IEEE Trans. Inf. Technol. Biomed. 6 (3), 235–243. doi:10.1109/TITB.2002.802381
Renaud, P., Singer, G.-F., and Proulx, R. (2000). Head-tracking fractal dynamics in visually pursuing a virtual target, Nonlinear Dynamics in the Life and social sciences (in W. Sulis and I, Trofimova, 320, 333–346.
Riccio, G. E., and Stoffregen, T. A. (1991). An ecological theory of motion sickness and postural instability. Ecol. Psychol. 3 (3), 195–240. doi:10.1207/s15326969eco0303_2
Riva, G. (2022). Virtual reality in clinical psychology. Compr. Clin. Psychol., 91–105. doi:10.1016/B978-0-12-818697-8.00006-6
Robillard, G., Bouchard, S., Renaud, P., and Cournoyer, L.-G. (2002). Validation canadienne-française de deux mesures importantes en réalité virtuelle: L’Immersive Tendencies Questionnaire et le Presence Questionnaire, Poster presented at the 25ième congrès de la Société Québécoise pour la Recherche en Psychologie (SQRP).
Rothstein, H. R. (2008). Publication bias as a threat to the validity of meta-analytic results. J. Exp. Criminol. 4 (1), 61–81. doi:10.1007/s11292-007-9046-9
Sanchez-Vives, M. V., and Slater, M. (2005). From presence to consciousness through virtual reality. Nat. Rev. Neurosci. 6 (4), 332–339. doi:10.1038/nrn1651
Scozzari, S., and Gamberini, L. (2011). Virtual reality as a tool for cognitive behavioral therapy: a review. In S. Brahnam, and L. C. Jain (Éds.), Advanced computational intelligence paradigms in healthcare 6. Virtual reality in psychotherapy, rehabilitation, and assessment (p. 63–108). Springer Berlin Heidelberg. doi:10.1007/978-3-642-17824-5_5
Seitz, R. J. (2014). Monitoring of visuomotor coordination in healthy subjects and patients with stroke and Parkinson’s disease: an application study using the PABLOR-device. Int. J. Neurorehabilitation 01 (02). doi:10.4172/2376-0281.1000113
Sevinc, V., and Berkman, M. I. (2020). Psychometric evaluation of Simulator Sickness Questionnaire and its variants as a measure of cybersickness in consumer virtual environments. Appl. Ergon. 82, 102958. doi:10.1016/j.apergo.2019.102958
Sheridan, T. B. (1992). Musings on telepresence and virtual presence. Presence Teleoperators Virtual Environ. 1 (1), 120–126. doi:10.1162/pres.1992.1.1.120
Sirkkunen, E., Väätäjä, H., Uskali, T., and Rezaei, P. P. (2016). Journalism in virtual reality: opportunities and future research challenges. Proc. 20th Int. Acad. Mindtrek Conf., 297–303. doi:10.1145/2994310.2994353
Slater, M., Brogni, A., and Steed, A. (2003). Physiological responses to breaks in presence: a pilot study, 157.
Stanney, K., Fidopiastis, C., and Foster, L. (2020a). Virtual reality is sexist: but it does not have to Be. Front. Robotics AI 7, 4. doi:10.3389/frobt.2020.00004
Stanney, K., Lawson, B. D., Rokers, B., Dennison, M., Fidopiastis, C., Stoffregen, T., et al. (2020b). Identifying causes of and solutions for cybersickness in immersive technology: reformulation of a research and development agenda. Int. J. Human–Computer Interact. 36 (19), 1783–1803. doi:10.1080/10447318.2020.1828535
Stoffregen, T. A., and Smart, L. J. (1998). Postural instability precedes motion sickness. Brain Res. Bull. 47 (5), 437–448. doi:10.1016/S0361-9230(98)00102-6
Sumayli, Y., and Ye, Y. (2023). Motion sickness during roll motion: VR HMD view versus monitor view. Vibration 6 (1), 45–56. Article 1. doi:10.3390/vibration6010004
Tan, C. W., Bergmeir, C., Petitjean, F., and Webb, G. I. (2021). Time series extrinsic regression. Data Min. Knowl. Discov. 35 (3), 1032–1060. doi:10.1007/s10618-021-00745-9
Treisman, M. (1977). Motion sickness: an evolutionary hypothesis. Sci. (New York, N.Y.) 197 (4302), 493–495. doi:10.1126/science.301659
Varmaghani, S., Abbasi, Z., Weech, S., and Rasti, J. (2021). Spatial and attentional aftereffects of virtual reality and relations to cybersickness. Virtual Real. 26, 659–668. doi:10.1007/s10055-021-00535-0
Wasserstein, R. L., Schirm, A. L., and Lazar, N. A. (2019). Moving to a World Beyond “p < 0.05”. Am. Stat. 73 (sup1), 1–19. doi:10.1080/00031305.2019.1583913
Weech, S., Calderon, C. M., and Barnett-Cowan, M. (2020a). Sensory down-weighting in visual-postural coupling is linked with lower cybersickness. Front. Virtual Real. 1. doi:10.3389/frvir.2020.00010
Weech, S., Kenny, S., and Barnett-Cowan, M. (2019). Presence and cybersickness in virtual reality are negatively related: a review. Front. Psychol. 10, 158. doi:10.3389/fpsyg.2019.00158
Weech, S., Kenny, S., Lenizky, M., and Barnett-Cowan, M. (2020b). Narrative and gaming experience interact to affect presence and cybersickness in virtual reality. Int. J. Human-Computer Stud. 138, 102398. doi:10.1016/j.ijhcs.2020.102398
Wibirama, S., Santosa, P. I., Widyarani, P., Brilianto, N., and Hafidh, W. (2020). Physical discomfort and eye movements during arbitrary and optical flow-like motions in stereo 3D contents. Virtual Real. 24 (1), 39–51. doi:10.1007/s10055-019-00386-w
Witkin, H.-A., Dyk, R. B., Fattuson, H. F., Goodenough, D. R., and Karp, S. A. (1962). Psychological differentiation: studies of development. Wiley, 418. xii.
Witmer, B. G., and Singer, M. J. (1998). Measuring presence in virtual environments: a presence questionnaire. Presence Teleoperators Virtual Environ. 7 (3), 225–240. doi:10.1162/105474698565686
Yang, A. H. X., Kasabov, N., and Cakmak, Y. O. (2022). Machine learning methods for the study of cybersickness: a systematic review. Brain Inf. 9 (1), 24. doi:10.1186/s40708-022-00172-6
Keywords: motion sickness, virtual reality, field-dependence, sense of presence, eyes-hand coordination, kinetosis, virtual environments, immersion
Citation: Maneuvrier A, Nguyen N-D-T and Renaud P (2023) Predicting VR cybersickness and its impact on visuomotor performance using head rotations and field (in)dependence. Front. Virtual Real. 4:1307925. doi: 10.3389/frvir.2023.1307925
Received: 05 October 2023; Accepted: 16 November 2023;
Published: 27 November 2023.
Edited by:
Stephen Palmisano, University of Wollongong, AustraliaReviewed by:
William Chung, University Health Network, CanadaDiego Vilela Monteiro, ESIEA University, France
Copyright © 2023 Maneuvrier, Nguyen and Renaud. This is an open-access article distributed under the terms of the Creative Commons Attribution License (CC BY). The use, distribution or reproduction in other forums is permitted, provided the original author(s) and the copyright owner(s) are credited and that the original publication in this journal is cited, in accordance with accepted academic practice. No use, distribution or reproduction is permitted which does not comply with these terms.
*Correspondence: Arthur Maneuvrier, YXJ0aHVyLm1hbmV1dnJpZXJAcHJvdG9ubWFpbC5jb20=