- 1Cognitive Assistants (COS), German Research Centre for Artificial Intelligence (DFKI), Saarbrücken, Germany
- 2Ubiquitous Media Technology Lab, Saarland University, Saarbrücken, Germany
During interaction with objects in Virtual Reality haptic feedback plays a crucial role for creating convincing immersive experiences. Recent work building upon passive haptic feedback has looked towards fabrication processes for designing and creating proxy objects able to communicate objects’ properties and characteristics. However, such approaches remain limited in terms of scalability as for each material a corresponding object needs to be fabricated. To create more flexible 3D-printed proxies, we explore the potential of metamaterials. To this aim, we designed metamaterial structures able to alter their tactile surface properties, e.g., their hardness and roughness, upon lateral compression. In this work, we designed five different metamaterial patterns based on features that are known to affect tactile properties. We evaluated whether our samples were able to successfully convey different levels of roughness and hardness sensations at varying levels of compression. While we found that roughness was significantly affected by compression state, hardness did not seem to follow the same pattern. In a second study, we focused on two metamaterial patterns showing promise for roughness perception and investigated their visuo-haptic perception in Virtual Reality. Here, eight different compression states of our two selected metamaterials were overlaid with six visual material textures. Our results suggest that, especially at low compression states, our metamaterials were the most promising ones to match the textures displayed to the participants. Additionally, when asked which material participants perceived, adjectives, such as “broken” and “damaged” were used. This indicates that metamaterial surface textures could be able to simulate different object states. Our results underline that metamaterial design is able to extend the gamut of tactile experiences of 3D-printed surfaces structures, as a single sample is able to reconfigure its haptic sensation through compression.
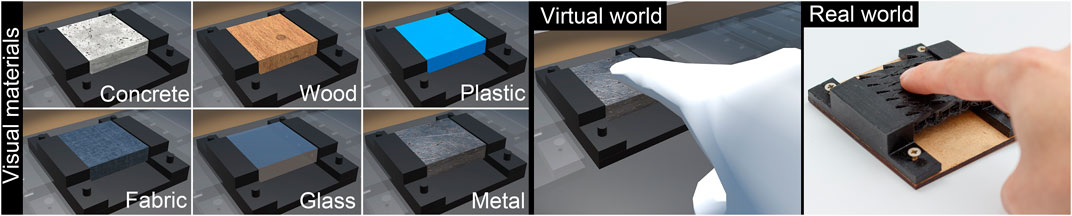
GRAPHICAL ABSTRACT | The six visual material textures: concrete, wood, plastic, fabric, glass and metal used in the main experiment. In addition, an example how participants interacted with the samples.
1 Introduction
Virtual Reality (VR) allows users to immerse themselves in artificially generated worlds. Today’s VR experiences are already compelling in terms of their visual and auditory aspects. However, technology for providing haptic feedback still remains in its infancy. As touch sensations are essential in understanding the physical world around us (Robles-De-La-Torre, 2006), haptic feedback remains crucial in creating realistic and plausible virtual experiences wherein users can feel present and act accordingly (Slater, 2009).
A variety of methods has been illustrated for simulating touch experiences in immersive virtual environments (IVEs). One common approach for generating detailed tactile sensations is called passive haptic feedback (PHF). Here, tangible objects serve as passive proxies for virtual representations, which has been shown to greatly benefit the user’s sense of presence (Insko, 2001). While this technique can provide highly realistic haptic details, it remains bound by several limitations (Nilsson et al., 2021). To successfully render haptic properties, proxies need to provide the user with physical sensations that are co-located with their virtual counterpart, and at the same time provide a sufficiently similar feeling in terms of its material, e.g., texture, and geometrical properties, e.g., shape. This results in scaling issues, as the number of proxies required to represent large amounts of virtual objects, each with varying haptic properties, rapidly increases.
Our work looks towards the field of fabrication to create more scalable and flexible proxy objects. As recent advancements of fabrication technologies support the manufacturing of highly detailed physical artifacts, they have been used to construct artifacts with varying haptic properties. Examples include methods for designing objects with desired mechanical behavior, such as elasticity or deformation through varying internal microstructures (Bickel et al., 2010; Schumacher et al., 2015), or for fabricating perceptually-varying surface texture qualities (Piovarči et al., 2016; Degraen et al., 2021b; Gedsun et al., 2022). Moreover, these approaches have been used in virtual settings to create tactile experiences through passive haptic proxies. For example, Degraen et al. (2019) utilized 3D-printed hair-like structures to serve highly detailed tactile sensations for virtual textures. Through variations of each structure’s hair length, their tactile perception in terms of hardness and roughness could reliably be influenced. While such abstract structures are able to support the visuo-haptic perception of different virtual materials, still a large enough set of objects needs to be produced.
In this work, we focus on fabricating proxy objects that are able to change their tactile properties. Firstly, we present an investigation into the perceptual gamut of 5 metamaterial patterns by changing their compliance and surface features through lateral compression (see Figure 1). We outline our designs and the implemented fabrication process. Our results show that compressing our metamaterials elicit different tactile perceptions in terms of hardness and roughness. However, the changes were not found to be consistent across all metamaterial patterns. Based on these insights, we narrowed our set of samples down to 2 patterns that provided a wide range of tactile impressions. We examine the use of these patterns as passive haptic proxies for simulating visuo-haptic material experiences in VR. From our results, we see that metamaterial patterns are able to support tactile material perception through visuo-haptic stimuli. However, we also note that high levels of metamaterial compression created discrepancies between the physical features and their visual overlays. This caused haptic dominance during exploration, as participants mostly relied on their tactile impressions for highly compressed scenarios. Specifically, we contribute the following.
1. A novel concept for simulating tactile experiences in VR based on 3D-printed metamaterial actuation;
2. The design of five metamaterials, including their fabrication process and open-sourced designs;
3. An initial investigation into the effectiveness of actuated metamaterials for simulating roughness and hardness, and their application for simulating texture and material experiences in VR.
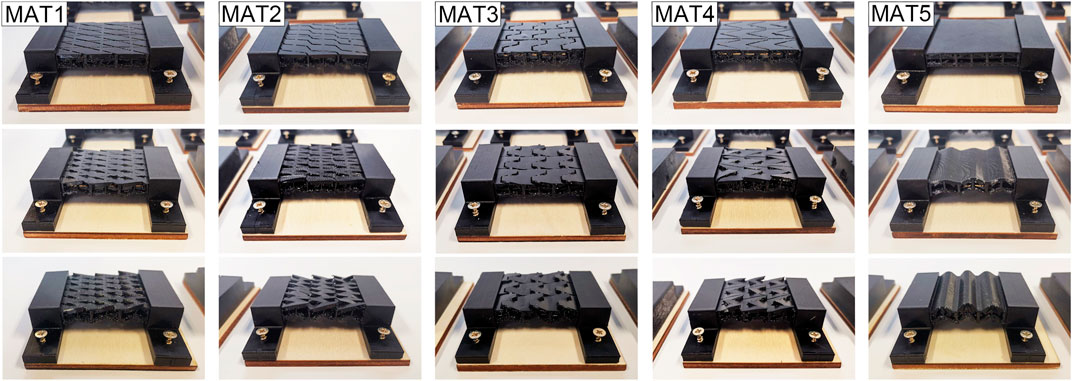
FIGURE 1. Metamaterial designs 1 to 5, each in three different actuation states 0 mm, 2 mm, and 6 mm.
2 Related work
In the following section, we provide an overview of the relevant literature.
2.1 Haptic feedback for Virtual Reality
Providing haptic feedback when touching or interacting with virtual objects remains a challenge in VR. To address this, researchers have divided approaches into three basic classes: active, passive and mixed haptic feedback. Active approaches use computer-generated actuation to provide force feedback for interactions with what otherwise would remain purely virtual content (Insko, 2001). For instance, the TextureTouch haptic controller (Benko et al., 2016), renders textures at the tip of a user’s index finger by utilizing an actuated pin-array. However, such devices are often challenging to design and develop due to their high computational and mechanical complexity.
On the other hand, there exist passive approaches, often called proxies (Insko, 2001; Nilsson et al., 2021)—physical “stand-ins” for virtual objects. Ideally, a single proxy object can be used for various virtual models, reassembling their relevant physical properties such as shape and size (Feick et al., 2020a) or weight (Zenner and Krüger, 2017). Especially interesting in the context of our work, are proxies for material texture perception. Here, most notably is the work by Degraen et al. (2019) on 3D-printed hairs. The authors were able to influence the feeling of roughness and hardness by changing the length of the printed hairs, and by overlaying visual textures, they effectively increased the resolution. Yet, the downside of purely passive approaches is their inflexibility, i.e., proxies cannot change themselves to extend their haptic rendering capabilities.
It is this gap that mixed approaches fit in. Here, a well-known concept is Dynamic Passive Haptic Feedback (DHPF) (Zenner and Krüger, 2017), describing passive proxies that have actuating parts in order to change their own properties, e.g., weight distribution. For example, the Haptic Revolver (Whitmire et al., 2018) which can dynamically change the haptically perceived material when touching virtual objects’ surfaces. HairTouch (Lee et al., 2021) dynamically renders stiffness, roughness and surface height differences realistically using reconfigurable brush hairs as a proxy. Finally, Snaker Charmer (Araujo et al., 2016) is an encounter-type haptic device consisting of a robotic arm with a box as an end-effector. The box is augmented with different textures on each square face and depending on which virtual surface a user touches, it quickly rotates the box to the face with the corresponding physical texture.
2.2 Mixed texture perception
Through multisensory integration, our brain combines signals from different sensory channels in order to create a coherent perception. It is commonly understood that different stimuli are weighted according to their reliability (Ernst, 2012). This results in scenarios where one sense can show dominance over another upon receiving mismatching information. In visuo-haptic environments, this effect has been explored for simulating different perceptions. For example, methods relying on visual dominance have been shown to be able to provide pseudo-haptic feedback for virtual UI elements (Speicher et al., 2019), while visual distortion approaches are able to influence the perception of an object’s weight (Samad et al., 2019), size (Bergström et al., 2019) or function (Feick et al., 2021).
In order to simulate tactile experiences, previous work has combined visual textures with influencing different tactile dimensions of texture perception (Okamoto et al., 2013). For example, Lécuyer (2009) utilized a pseudo-haptic approach to influence the perception of stiffness of a virtual spring. Other methods investigated the perception of textures in mixed reality (MR) environments using techniques that exploit visual dominance (Iesaki et al., 2008; Punpongsanon et al., 2015; Strohmeier et al., 2018; Sato et al., 2020). Here, Punpongsanon et al. (2015) influence the perception of softness by augmenting surfaces using different projection-based visual effects. More recently, Sato et al. (2020) proposed different visual hand augmentations to modify the user’s perception of surface textures in terms of unevenness, slipperiness, and softness.
Our work builds upon fabrication methods to influence tactile perception. During the exploration of textures and materials using one’s fingers, abstract fabricated structures have been used in combination with visually overlaid textures to simulate material experiences (Degraen et al., 2019). Here, hair-like structures were proposed to influence the perception of roughness and hardness. In combination with visual texture overlays, participants indicated a wide gamut of material impressions. Hair-like designs were further studied by Takahashi and Kim (2022) to create haptic displays using perforated plates to alter the length and behaviour of the available set of hairs.
2.3 Fabricated metamaterials
Metamaterials can be defined as: “…a novel class of complex composite materials [with the] ability to exhibit any desirable electromagnetic, acoustic, or mechanical property such as negative mass, stiffness, or Poisson’s ratio…” (Valipour et al., 2022). There exist many categories of metamaterials; however, we are most interested in the ones, allowing us to change the surface structure. Generally, metamaterials are composed of unique cells on a regular grid. Varying cell designs are used to achieve the desired behaviour (Gangwar and Gangwar, 2014; Valipour et al., 2022). An interesting class of metamaterials, are so-called auxetic materials. In contrast to regular materials, they can enlarge their surface area (negative Poisson ratio) when being stretched. An approach to reproduce surface structures using auxetic materials was presented by Steed et al. (2021). Their prototype consisted of a deformable auxetic material placed on mechanical pistons that can vary the curvature of the surface, resulting in different terrains, while remaining its local stiffness.
To design and manufacture metamaterial textures using FDM 3D-printing, Ion et al. (2018) developed a publicly available editor. The shape and structure of the metamaterial are inspired by Origami and surface wrinkling techniques. Using the different created surfaces, each metamaterial can be compressed, forcing certain cells to deform. For example, a bicycle handle with an adjustable grip, i.e., more or less friction caused by a compressed and uncompressed metamaterial, respectively. Their follow-up work presented an approach to enable reconfiguration of their designs without the need for reprinting (Yang et al., 2022). To enable compression of metamaterial designs, Neville et al. (2016) present actuated metamaterials inspired by Kirigami, i.e., the art of cutting and folding paper to get 3D shapes. They ran multiple strings through their metamaterial designs to achieve deformations that go beyond single axis compression. In fact, this opens-up an interesting design space for metamaterial actuation.
Our work aims to support the design and fabrication of novel proxy objects that can dynamically change their tactile properties upon actuation. We take inspiration from the most recent work in the fabrication space on 3D-printed metamaterials, studying their potential to enhance tactile experiences in VR.
3 Metamaterials for tactile texture perception
In this section, we outline the design, implementation and fabrication of our actuated 3D-printed metamaterial prototypes. We provide valuable insights and lessons learned to ensure that our approach is reproducible. Our central interest lies in changing roughness and hardness properties of a metamaterial by actuating (compressing) it, allowing it to act as a proxy for several virtual material textures.
3.1 Design
In total, we designed five different metamaterial patterns that can be actuated. To influence their tactile perception, we focus on the properties of hardness and roughness, which have been found to be the main contributors of texture and material perception (Okamoto et al., 2013). Below, we discuss the design choices for our two perceptional dimensions of interest, hardness and roughness.
3.1.1 Hardness
To change the hardness of the metamaterial, we utilize the concept of porous materials—solids that contain (penetrating) pores. A measurement for how porous a material is, is called porosity, which is defined by the fraction of pore volume to the total volume of a material. If a material has a porosity level of 0.2–0.95, it counts as porous according to the definition of Ishizaki et al. (2013). This criterion is fulfilled for our designed metamaterial patterns. Lu et al. (1999) investigated strength, the elastic modulus and the hardness of porous materials. They found that all of these material properties substantially decrease, when porosity increases. We build on their results by using porosity as our core design variable to change the hardness within the same metamaterial. To summarize, when we decrease the porosity within 3D-printed metamaterials, we increase their hardness. In Figure 2, we illustrate a compressed cell of our metamaterial 3D-prints, leading to a reduced fraction of the pores. Thus, the porosity decreases, and therefore we would expect an increase in the perceived hardness of the metamaterial.

FIGURE 2. Metamaterial deformation simulation. (A) Fixating metamaterial arches creates a uniform periodic deformation. (B) By not fixating uneven arches, the metamaterial deforms upwards.
Please note that compressing the metamaterial will ultimately lead to emerging features such as bumps (see Figure 1). In fact, this adds a second “haptic” layer, potentially affecting hardness sensations. To investigate this potential issue, we ran a preliminary experiment.
3.1.2 Roughness
To achieve changing roughness sensations within one metamaterial, we based our designs on two core principles. First, the emerging features from our metamaterials should be in the range of 2 mm–3.5 mm, because Klatzky et al. (2013) found that the perceived roughness increases consistently with inter-element spacing up to approximately 3.5 mm. Second, the features should still be perceived as one surface. Therefore, we incorporate findings from two-point discrimination experiments. Dellon (1978) describe this as the distance between two perceivable points can be distinguished from one another. Depending on age, this distance usually varies between 2 and 3 mm. Hence, we use a feature distance of maximum 3 mm, ensuring that our metamaterials are perceived as one surface.
3.1.3 Metamaterial patterns
Finally, we present our resulting metamaterial patterns based on the previously outlined design principles and many iterations. MAT1 and MAT2 are closest to the proposed feature spacing range of 2 mm–3.5 mm when they are compressed (see Figure 1). They contain the smallest bumps and spikes emerging that we were able to produce with our fabrication technique described below. Furthermore, their patterns aim to achieve the smallest feature size achievable with the way our metamaterials are structured—without the features being spaced too far apart. The main difference between MAT1 and MAT2 is the design of the spike shape emerging. MAT2 has more rounded edges. We include this slight variation, as it reassembles a worn off version of MAT1, because Myers (1962) found that worn off materials can result in different roughness sensations. Adding a worn off state of MAT1, enables us to collect insights, helping us to better understand longevity and potential perceptual drifts caused by material deterioration.
We included MAT3 design as a pattern that does not use “spiky” features. This comes with the sacrifice of the metamaterial features becoming larger—exceeding the 3.5 mm discrimination threshold. Hence, this design does not meet the criteria to achieve the feeling of one surface, as the features become clearly distinguishable. Yet, exploring a non-spiky pattern was an interesting design variation. MAT4 is the spiky counterpart to MAT3, allowing us to investigate how much the “spikiness” plays a role with larger surface features.
Finally, metamaterial MAT5 was specifically designed for simulating hardness. This material has the greatest change in porosity upon all metamaterials presented. With this, we aim to study whether the change in hardness found by Lu et al. (1999) also holds true for our metamaterial patterns. When looking at the structure of this material, the emerging features appear to be straight bumps and thus, will most likely not be associated with roughness.
3.2 Actuating 3D-printed metamaterials
In this section, we describe our actuation approach. Our ultimate goal was to implement a prototype that allows compressing metamaterials linearly, resulting in various different states. As a result, we can control surface changes as shown before.
3.2.1 Initial prototyping and design requirements
Ion et al. (2018) demonstrate how 3D-printed metamaterials behave when being compressed. An example for a non-actuated as well as an actuated metamaterial can be seen in Figure 3. In order to actuate our metamaterials, resulting in the desired equal deformation, every second pillar inside the metamaterials must freely move vertically upwards upon compression. For instance, in Figure 2 these are the uneven pillars 1, 3, 5 and 7; even pillars, i.e., 0, 2, 4, 6, and 8 are prevented from going upwards. The triangular shapes in the segments between the pillars encourage the behaviour of moving only every second pillar upwards.
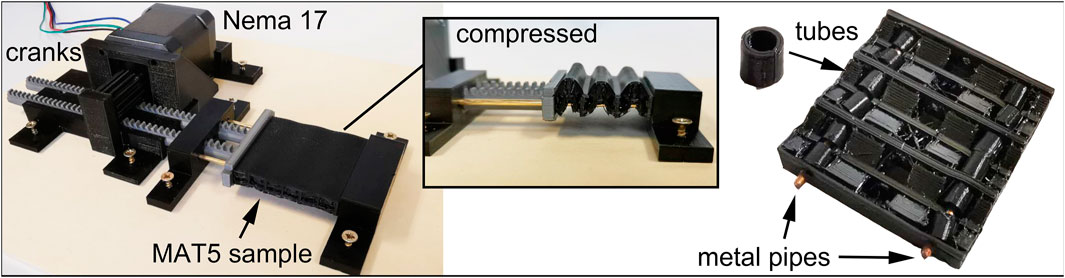
FIGURE 3. Actuation Setup. (left) A stepper motor and two cranks are used to compress the metamaterial. (center) Detailed view of a compressed metamaterial showing periodic deformation. (right) Embedded tubes guide metal support pipes to ensure equal distribution of applied force creating a uniform compression across the surface.
However, one key limitation is the flexibility of the metamaterials which causes the mechanism to not work properly, when metamaterial structures become larger. For instance, for two rows of cells, triangular shapes work properly, but once we added a third or more rows to the metamaterials, the deformation behaviour changes. Figure 2B illustrates the effect when compressing a larger metamaterial, only relying on the deformation mechanism 3D-printed directly into them. The metamaterial starts not deforming row by row, but instead deforms in total, and escapes as an arch unevenly upwards. Based on our initial experiments and several prototyping iterations, we formulated the following requirements for the actuation mechanism:
R1: Our actuation approach should force the metamaterials to move vertically upwards row by row, instead of the whole metamaterial arching as one unit.
R2: Our actuation approach should only allow every second pillar within the metamaterials to freely move vertically upwards and downwards.
R3: Our actuation approach should result in uniform distribution of emerging features throughout the entire actuation spectrum.
3.2.2 Actuation approach
Based on the requirements stated above, we developed an actuation approach inspired by Neville et al. (2016). In their work, authors fed strings through their metamaterials and used a pulling force on the strings to achieve the deformation. Applying this to our designs comes with the need to further modify the 3D-prints with holes running through the materials and cut-outs in the inner metamaterial pillars. We started testing with one hole through each metamaterial cell, which we directly 3D-printed into them.
Initially, we used standard fishing line fed through the metamaterials, allowing us to compress the material by pulling on the strings. However, the metamaterials unevenly deform, as illustrated in Figure 2B. This is caused by the strings, because they do not restrict the metamaterial to a single plane of motion. Therefore, we replaced the fishing line with small metal pipes that can be seen in Figure 3. The stiff metal pipes restrain the metamaterials to stay in one plane—solving the problem of the non-uniform deformation (R1). To achieve the desired deformation state, we switched to a pushing/pulling approach and added two fixation blocks, where either end of the metal pipes can be inserted. The cranks are moved by a Nema 17 stepper motor and controlled using an Arduino Uno and a DRV8825 driver. The setup can be seen in Figure 3.
As defined above, every second pillar within the metamaterial must be allowed to move vertically upwards and downwards (R2). With only the holes for the pipes inside the materials, pillars inside are now restricted to move because of the metal pipes. As a result, we changed the holes in every second pillar into full openings. For the material next to the holes to not rub at the stiff metal pipes, we also cut out the bottom layer of the metamaterial cells, which can be seen in Figure 3 (right).
In the last step, we specifically looked at (R3), because we observed differences in how the features emerged due to the way we achieved the material compression, i.e., the metamaterial cell row nearest to the crank will compress more than the second one and so on. This was unproblematic for achieving binary actuation states, compressed and uncompressed; however, it does not support consistent “infinite” states in-between. Hence, we attempted to create a more uniform force distribution while actuating. To do so, we first embedded small metal springs around the metal pipes. If metal springs are sufficiently stiff, they will transfer the applied pressure to the neighbouring cell, before they fully compress. Nevertheless, we were unable to find metal springs that had the correct stiffness given our form factor; most of them were not stiff enough or too large. Instead of springs, we then 3D-printed small tubes out of flexible filament that we fitted around the metal pipes shown in Figure 3. They offer suitable stiffness which can be adjust by changing their wall thickness. For our 3 × 3 metamaterial designs, we used tubes with a wall thickness of 2 mm and a length of cell space minus 1 mm. This allowed us to reach 4 visibility distinct actuation states, with 2 mm steps of compression added for each new state.
Currently, our metamaterial patterns have to be manually post-processed in CAD software after they have been procedurally generated. In addition, they require additional hardware embedding, e.g., metal pipes to serve as support structures. More advanced multimaterial 3D-printing may reduce the need for such additional support, as this would be able to embed internal structures directly into the design using rigid filaments such as PLA or carbon compounds. Additionally, the currently embedded metal pipes influence hardness. To address this, we experimented with different designs and found that up to a metamaterial size of 3 × 3 or 4 × 4 cells at a cell sizes of 15 mm, designers can rely on only two metal pipes in the outermost cell rows. This creates an area in the centre of the metamaterials that can be explored without the influence of the support structures.
3.3 Fabrication process
Our objective was to create a 40 mm × 40 mm intractable surface area that can be explored with a user’s index finger (Degraen et al., 2019). Similar to Ion et al. (2018), we aimed to fabricate our metamaterials with a low-cost off-the-shelf FDM printer. According to the authors, they used filament with a shore hardness rating of 85A. The lower the shore hardness rating (A-value), the softer the material. However, since our use case differs from their application, we 3D-printed metamaterial samples with multiple filaments: Prusa PLA (95A), Recreus FilaFlex (90A), Recreus FilaFlex (82A) and Recreus FilaFlex (60A). We used the most basic cell shape available in the editor and set the cell size to 15 mm.
All samples were 3D-printed on a Prusa i3 MK3S using the recommended settings from the corresponding filament providers. We investigated two properties: (1) how easy the metamaterial can be deformed, and (2) how well the prints return into their original shape after deformation. Both, the 82A and the 60A shore hardness filaments showed promising results, satisfying the two criteria. In next step, we 3D-printed varying cell sizes: 15 mm, 12 mm and 9 mm. The latter being the smallest cell size we could reliably print with the Prusa i3 MK3s. When testing the deformation of these prints, we found that the smaller the movable features of the metamaterial, the softer the filament needs to be. This appears to be an interesting trade-off between cell size and filament hardness, and an important aspect when fabricating metamaterial structures.
While experimenting with the printing settings, we observed that many variables such as slight variations in nozzle temperature or tightness of the printer screw significantly affect the hardness and quality of the resulting metamaterial prints. Therefore, we provide our final 3D-printing settings below. This should be a solid starting point for anyone who wants to fabricate and experiment with their own 3D-printed metamaterials.
• Basic settings: the basic profile and settings should be taken from the manufacture’s website.
• Print quality: 0.15 mm.
• Infill: 10%. May be increased for larger metamaterial prints.
• Printing speed settings: we set printing speeds settings to 20 mm/s.
• Nozzle temperature: 212°C.
• Printer screw position: unscrew 2–3 rotations.
• Extruder: set to zero in the slicer software.
Finally, our final prototypes use a 3 × 3 grid, with 15 mm cell sizes. This results in a 4.5 cm × 4.5 cm surface in the uncompressed state. The average minimal actuation of the metamaterials for the first features to emerge lies around 2 mm. Further actuation states are visibly distinguishable when actuating linearly in 2 mm steps. With each metamaterial design (MAT1–MAT5) we achieved four distinct actuation states, where the maximum compression will be reached at 6 mm. As a result, even maximal compression still results in a large enough surface of 4.5 cm × 3.9 cm to be explored.
4 Experiment 1: tactile metamaterial perception
To understand the tactile perception of our metamaterials, we conducted a preliminary experiment.
4.1 Design
The goal of this preliminary experiment was to gather first insights into how our actuated 3D-printed metamaterial patters are haptically perceived in terms of roughness and hardness. Additionally, we wanted to understand whether certain metamaterial patterns are more suitable for simulating roughness and hardness. To investigate this, we used a within-subjects design consisting of a baseline and similarity assessment phase.
4.1.1 Baseline assessment
In the baseline condition, we presented each of the 20 material states once to the participant. They were asked to explore them with the index finger of their dominant hand. Then, they rated each material in terms of roughness and hardness on a 9-point Likert scale. The order in which the metamaterials were presented to the participants was counterbalanced using Latin square. We asked the following questions:
Q1.1: How hard would you rate the inspected material? (1 = extremely soft; 9 = extremely hard).
Q1.2: How rough would you rate the inspected material? (1 = extremely smooth; 9 = extremely rough).
4.1.2 Similarity assessment
To directly compare the different 3D-printed metamaterial patterns and their actuation states to each other, we included a second condition. This resulted in
Q2.1: How similar does this material feel in terms of hardness? (1 = extremely different; 9 = extremely similar).
Q2.2: How similar does this material feel in terms of roughness? (1 = extremely different; 9 = extremely similar).
4.2 Hypothesis
We formulated the following two hypotheses:
H1.1: Increasing the compression of our 3D-printed metamaterial patterns leads to an increase in their perceived roughness.
H1.2: Increasing the compression of our 3D-printed metamaterial patterns leads to an increase in their perceived hardness.
4.3 Participants
We recruited six participants (two female and four male), aged between 23 and 59 (M = 25.5, SD = 15.2) with backgrounds in law, engineering, accounting, computer sciences and the medical domain. Regarding hand dominance, most participants indicated to be right-handed, while one participant noted they were ambidextrous with a preference for left-handed interaction. Participants stated they did not have any impairments that could influence both their visual or tactile perception. All participants indicated on a 5-point Likert scale they never worked with textiles (M = 2.0, SD = 1.0), and noted they did not often perform precise handwork (M = 1.1, SD = 0.3). Participants received candy for taking part in the experiment. The study was approved by the University’s Ethical Review board.
4.4 Apparatus
The experiment took place in a quiet room. Our set of metamaterials consisted of a total of 20 surfaces, i.e., 5 specific metamaterial patterns with each having 4 fixed compression states, see Figure 1. To avoid visual bias, participants were not allowed to see the surfaces during exploration. To this aim, they were seated in front of a table with a cardboard screen. The opening at the bottom of this screen ensured enough room for the hand of the participants to reach through. Behind the screen, the metamaterial patterns were manually placed by the experimenter in a fixed location in a laser-cut hold out of wood. A digital rendering of the setup is depicted in Figure 4.
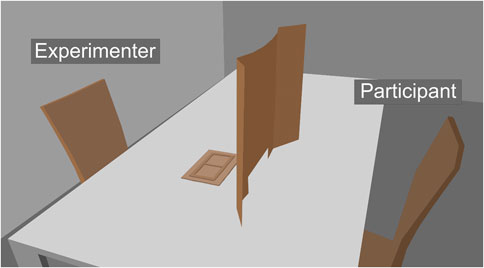
FIGURE 4. Digital rendering of the experimental setup. The experimenter prepared the samples for exploration, while the participant rated the tactile perception of the samples hidden behind the screen.
4.5 Procedure
Before starting the experiment, participants provided signed consent and were briefed regarding the upcoming course of events. We showed them the setup and explained the experiment’s purpose. Participants were asked to complete a questionnaire to collect their demographic information.
During the experiment, participants were instructed to explore the physical samples using only the index finger of their dominant hand. For each task, we demonstrated how to explore the materials to ensure consistency between samples. Specifically, participants were allowed to use circular lateral exploration to assess each structure’s surface information and could lightly press or tap each structure to assess hardness properties. Participants first completed the baseline task, before continuing with the comparison task. The observer noted the responses for each trial and activated the next sample.
Short breaks were scheduled between tasks and when the participant noted a feeling of numbness in their finger. Per participant, the total experiment took about 60 min.
4.6 Data collection and analysis
We collected data from four sources, i.e., demographic information for the pre-study questionnaire, and hardness, roughness and similarity ratings for each trial on a 9-point Likert scale. Statistical tests were chosen based on whether the data satisfied parametric test assumptions at α = .05 using Shapiro–Wilk test and QQ-plots. Reported p-values are corrected using Greenhouse-Geisser when the sphericity assumption was violated. For outlier removal, we initially applied the box plot method. Post-hoc pairwise comparisons were corrected using Bonferroni-Holm adjustments.
4.7 Results
In this section, we present the results of our preliminary experiment. The analysis is split into two parts corresponding to our study conditions. First, we report the roughness and hardness assessments of the metamaterials by our participants.
4.7.1 Baseline assessment
Here, we consider average ratings for roughness and hardness for each metamaterial and its compression states. We only ran our statistical analysis on each metamaterial, as we were primarily interested in the change of roughness and/or hardness within the same material.
4.7.1.1 Roughness
All metamaterials MAT1 (F(3,15) = 69.0, p < .0001, η2 = .828), MAT2 (F(3,15) = 97.8, p < .0001, η2 = .828), MAT3 (F(3,15) = 31.7, p < .0001, η2 = .725) and MAT4 (F(3,15) = 36.8, p < .0001, η2 = .763), except MAT5 (F(3,15) = 1.80, p < .0001, η2 = .121) showed a main effect when analysing their roughness scores. As stated above it is not surprising that MAT5’s actuation states did not show a main effect, because its features are well above the 3 mm threshold (Dellon, 1978). We ran post-hoc analysis on MAT1, MAT2, MAT3 and MAT4 using Bonferroni-Holm adjustments. The results are depicted in Figure 5 (top). All significant differences are in line with our H1.1, i.e., showing an increase in perceived roughness with increased compression of the metamaterial.
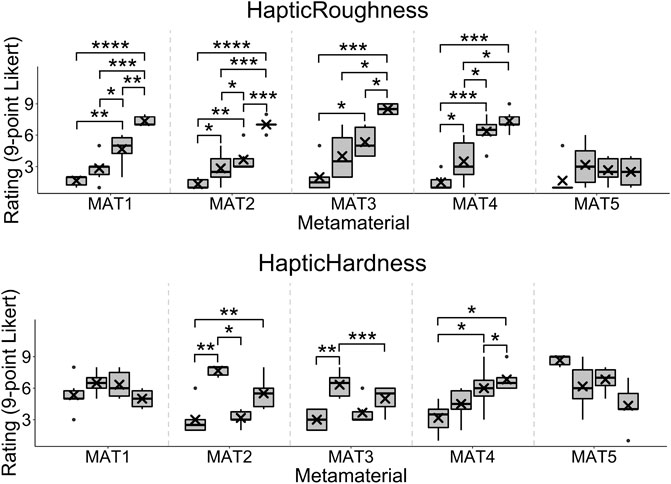
FIGURE 5. Participants separate assessments for haptic roughness and hardness for the different metamaterial actuation states 0, 2, 4 and 6 mm (left to right).
4.7.1.2 Hardness
MAT2 (F(3,15) = 20.1, p < .0001, η2 = .760), MAT3 (F(3,15) = 16.9, p < .0001, η2 = .578), MAT4 (F(3,15) = 6.82, p = .004, η2 = .491), and to our surprise even MAT5 (F(3,15) = 14.2, p = .002, η2 = .515) showed a main effect, when analysing their hardness scores. Only MAT1 (F(3,15) = 2.0, p = .157, η2 = .233) did not reveal a main effect. We ran post-hoc analysis on MAT4 using Bonferroni-Holm adjustments. The results are depicted in Figure 5 (bottom). For hardness, our results are mixed. Even though we found significant differences between the actuation states, they do not seem to follow a clear trend, an exception being MAT4 which follows our H1.2. For all other metamaterials our preliminary study fails to provide evidence.
4.7.2 Similarity assessment
Here, we consider the similarity ratings obtained through paired comparisons of our metamaterials.
4.7.2.1 Analysis of similarities
To analyse the ratings of similarity, we combined the similarity ratings of roughness and hardness for each metamaterial pair by averaging them. To determine consistency of the obtained data, we used Spearman’s rank correlation tests on the averaged similarity assessments. Here, we found the averaged similarity ratings for each participant to be correlated with those of every other participant (Mr = 0.52, p < .001). Given this result, we note that averaged similarity assessments remain consistent across participants.
For further analysis, the similarity assessments (1—9) were converted to normalized dissimilarity ratings (0—1). With these ratings, we created a symmetric dissimilarity matrix containing the perceptual distances between all metamaterials and compression states. Using an analysis of similarities, we compared different groupings within our distance matrix. We found a significant difference when comparing groups of different compression levels (R = 0.4762, p < .001). However, we did not find a significant difference when comparing groups of different metamaterials (R = 0.1569, p = .076).
4.7.2.2 Perceptual space
Analogous to literature (Vardar et al., 2019; Degraen et al., 2021b), we used the obtained symmetric dissimilarity matrix to perform a non-Metric Multi-Dimensional Scaling (NMDS) analysis. NMDS is an indirect gradient analysis approach which produces an ordination based on a distance or dissimilarity matrix. When dealing with human similarity data, such an approach is common for calculating and visualizing perceptual spaces of the distances (Cooke et al., 2006; Culbertson et al., 2013; Vardar et al., 2019; Degraen et al., 2021b). To understand how many axes are sufficient to visualize the perceptual space, we calculated the stress values for the first 6 dimensions. Here, the stress value of 0.09 for 3 dimensions approaches a faithful representation, similar to other methods dealing with tactile similarity metrics (Clarke, 1993).
Using Kruskal’s non-metric multidimensional scaling approach, we generated the perceptual space for our recorded assessments. Furthermore, we performed a k-means clustering analysis on the similarity data. The optimal amount of clusters was determined to be 5 (Charrad et al., 2014). Figure 6 illustrates the 3-dimensional perceptual space through visualizing the contrasting axis in different plots, with colouring representing the cluster membership.
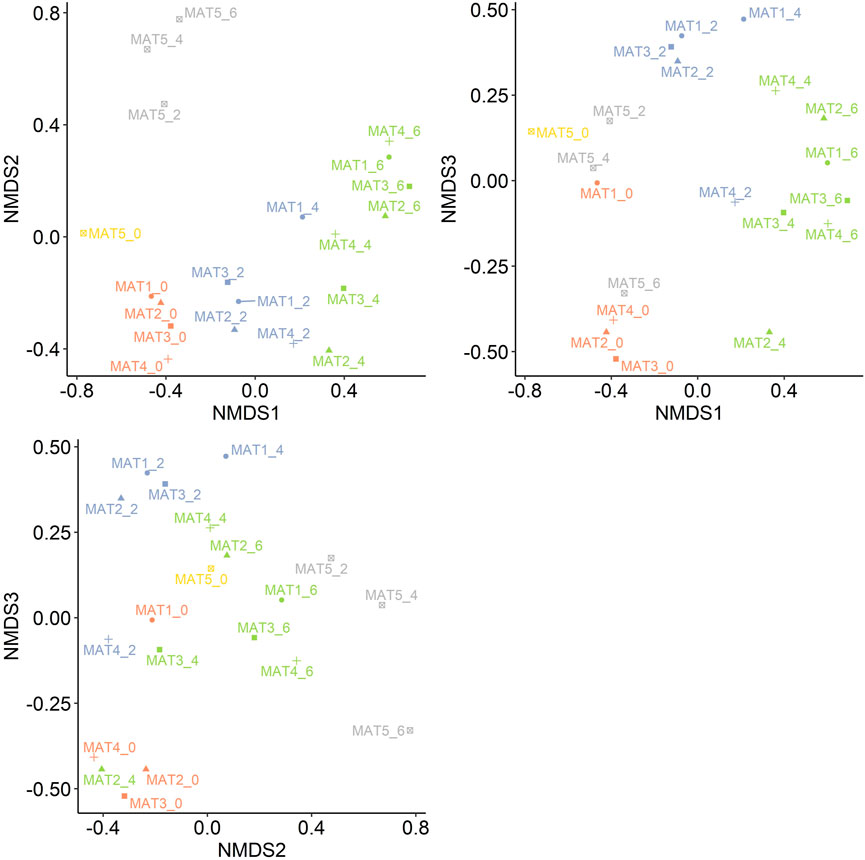
FIGURE 6. Perceptual space. Here, the distances between the named metamaterials (MAT1 through MAT5) in varying compression states (0, 2, 4, and 6 mm) are plotted in 3 views on the 3-dimensional space. Shapes are used to indicate the metamaterial type, while coloring indicates cluster membership (total k-means clusters = 5).
4.7.2.3 Insights
From the results of the analysis of similarities, we see that the driving force behind different assessments was the compression state of the materials, rather than the materials themselves. The perceptual space further shows that for all metamaterials excluding MAT5, the compression state drove the perception along the NMDS1 axis. Specifically for MAT5, the separate clustering of this metamaterial indicates its sensation to be distinct from other samples.
4.7.3 Summary and metamaterial selection
The main goal of the experiment was to collect early feedback on the prototypes and select the most promising metamaterials for our main study. Our preliminary findings suggest that MAT1, MAT2, MAT3 and MAT4 appear to be promising candidates for increasing the perceived roughness upon actuation. In contrast, for hardness only MAT4 followed our hypothesis, showing an upwards trend in hardness upon compression. Consequently, we decided to include MAT4. In addition, we also used MAT1 in the main experiment as it created the most distinct levels of roughness accordingly to participants assessments.
5 Experiment 2: visuo-haptic metamaterial perception
Using our selected metamaterials, we conducted a user study in VR to investigate how our designs were able to simulate visuo-haptic materials.
5.1 Design
The goal of experiment 2 was to study how adding visual material textures atop of our 3D-printed metamaterials affect their perception. Therefore, we combined our metamaterial patterns with virtual material textures in VR, and asked users to rate how well they match. Moreover, we were interested in the range of possible material textures that a single actuated metamaterial can simulate. We again used a within-subjects study design.
We focused on the two most promising metamaterial patterns from our preliminary experiment, MAT1 and MAT4 at the compression rates of 0, 2, 4 and 6 mm. This makes a total of eight metamaterial patterns participants ought to explore. Due to concerns regarding longevity of the metamaterials, we again fixated the different compression states by screwing our actuation mechanism with the metamaterials onto small wooden plates (see Figure 1).
The virtual material textures were chosen based on previous research by Degraen et al. (2019). We added two additional virtual materials, resulting in the following selection: metal, wood, glass, plastic, concrete and fabric, depicted in the Graphical Abstract. We believe that our selection covers a wide range of every day materials and therefore, provides a solid starting point.
Below, we describe the three conditions in the order participants completed them.
5.1.1 Haptic baseline
The participants were asked to explore all eight metamaterial patterns once, and rate them regrading their perceptual dimensions, roughness and hardness on a 9-point Likert scale. Additionally, we asked: “Which material do you think this is?” to better understand whether participants associate the 3D-printed metamaterials with known textures or objects from their every day environments. To ensure that the participants only relied on their haptic senses, the VR headset was turned off during this condition, only showing a dark screen. The order in which the participants explored the eight metamaterial patterns was counterbalanced using Latin square.
5.1.2 Visual baseline
Next, participants rated all visual material textures regarding their roughness and hardness, i.e., they rated how they would expect the materials that they saw to feel on a 9-point Likert scale. We also used the question “Which material do you think this is?”, to make sure all materials were properly recognizable by the participants. The order in which the virtual material textures were presented was again counterbalanced using a Latin square.
5.1.3 Visuo-haptic combination
Finally, we looked at the combination of visual material textures and haptic exploration of the metamaterial patterns. Each metamaterial pattern was paired with all six virtual material textures, resulting in 48 pairs. These pairs were presented to the participants in a randomized order. Repeatedly, one of the material spots in the VR environment was highlighted, indicating which material textures should be explored next. The participants then proceeded and explored the highlighted material both visually and haptically at the same time. Afterwards they gave a separate rating for roughness, hardness and similarity (between the visual and haptic material texture) on a 9-point Likert scale, and answered to the “Which material do you think this is?” question.
Q1.1: How hard would you rate the inspected material? (1 = extremely soft; 9 = extremely hard).
Q1.2: How rough would you rate the inspected material? (1 = extremely smooth; 9 = extremely rough).
Q1.3: How does the visual and the haptic perception match? (1 = not at all; 9 = extremely similar).
5.2 Hypothesis
Besides confirming our preliminary findings from experiment one, we formulated two additional hypotheses for our main experiment:
H2.1: Combining our 3D-printed metamaterials with visual material overlays leads to a shift in perception.
H2.2: Our 3D-printed metamaterial patterns provide matching haptic sensations for visual material textures.
5.3 Participants
We recruited 16 participants (six female and ten male), aged between 22 and 59 (M = 25.5, SD = 11.1) with various backgrounds including computer sciences, education, economics, biology, law, electrical and mechanical engineering. Twelve participants stated that they are right-handed and three left-handed. All participants confirmed that they have no impairment in their index finger which may affect their perception. Addtionally, we asked how often participants perform precise handwork, e.g., sewing or stitching on a 5-point Likert scale. Four participants rated themselves with a four or five, all others were three or below (M = 2, SD = 1.3). Participants answers about how often they work with textiles, e.g., clothing, design or tailoring on a 5-point Likert scale showed that only two answered with a higher value then four. All others being around one and two (M = 1, SD = 1.1). Out of the 16 participants, only three had prior experience with VR. Therefore, we highlighted very carefully to all participants that they can pause or stop the experiment at any time, e.g., in case they feel uncomfortable. Participants received unlimited candy as compensation for taking part in the experiment. The study was approved by the University’s Ethics and Hygiene Board.
5.4 Apparatus
Our apparatus consisted of an HTC VIVE Pro tracking system with SteamVR (v.1.22) and OpenVR SDK (v.1.16.8). The simple virtual scene was developed in Unity3D (v.2021.1.0). We used a Raubtier NBB01471 offering an Intel® Core i7 9700kf CPU, 32 GB RAM and an Nvidia® GeForce RTX 2080Ti for running the experiment. We included an androgynous hand representation without noticeable characteristics.
To enable hand tracking, a HTC Vive tracker was attached to the back of the participant’s hand. We used the calibration method from Zenner and Krüger (2017) asking participants to touch the touch-sensitive trackpad of a HTC Vive controller with the tip of their index finger. This was used to properly align the virtual hand model with the real hand. We rendered either a right or a left virtual hand, corresponding to a participant’s handedness.
To present the eight different metamaterial patterns to the user, we laser-cut a mount where the prepared metamaterial plates fit in. The mount was placed on a table in front of the user, allowing participants to explore the metamaterials while, at the same time, enabling the experimenter to quickly rearrange the metamaterial patterns throughout the study.
5.5 Procedure
First, participants received a general introduction to the study. Next, we gathered their consent and asked them to fill in a demographics questionnaire. Afterwards, we explained the task, including a demonstration of how to explore the metamaterial patterns with the index finger of their dominant hand. Then, we attached the Vive tracker to the participant’s hand; they entered the virtual environment, and we performed the hand calibration. Participants were guided through a practice round, showing them how to respond to the questions displayed in VR. Finally, they went through our three study conditions described above. Participants were prevented from seeing the metamaterial patterns before and during the study to avoid biases. Overall, the experiment took about 70–80 min.
5.6 Data collection and analysis
We collected data from four sources: a pre-study questionnaire for demographic information; hardness, roughness and similarity ratings on a 9-point Likert scale, participants material assessments, and a semi-structured interview to better understand participants’ experiences with our prototypes. Questionnaire responses were collected inside the virtual environment using the Virtual Reality Questionnaire Toolkit by Feick et al. (2020b). Interview responses and participants’ comments were coded for analysis. Analog to experiment one, we chose statistical tests based on parametric test assumptions at α = .05, and we used outlier removal with the box plot method. Post-hoc pairwise comparisons were corrected using Bonferroni-Holm adjustments.
5.7 Results
In this section, we present the results from our main experiment. The analysis is split into two parts corresponding to our study conditions. We start by reporting our results from the haptic and visual baseline conditions. Finally, we present the results from the visuo-haptic combination.
5.7.1 Baseline results
5.7.1.1 Haptic baseline
Participants rated the different actuation states of MAT1 and MAT4 significantly different from each other, in terms of roughness MAT1 (F(3,15) = 2.025, p = .023, η2 = .578) MAT4 (F(3,15) = 2.025, p = .023, η2 = .164) and hardness MAT1 (F(1.92,28.7) = 72.1, p < .0001, η2 = .774) MAT4 (F(1.9,28.6) = 182.0, p < .0001, η2 = .853), across various metamaterial actuation states (see Figure 7). This confirms the results from our preliminary experiment, and thus provides evidence for H1.1 and H1.2. One exception being that MAT1 in its maximum actuation state (6 mm), was rated significantly softer that its predecessor 4 mm, which contradicts with our hypothesis that increasing actuation leads to a perceived increase in hardness. This might be caused by the fully emerging features, adding up to 2 mm in height. This feature layer has fewer support structure; thus, causing the material to deform until the “base” layer by acting like a cushion. Considering that all other actuation states worked as expected, we believe that there is an interesting trade-off between feature design and compression rate that requires further exploration.
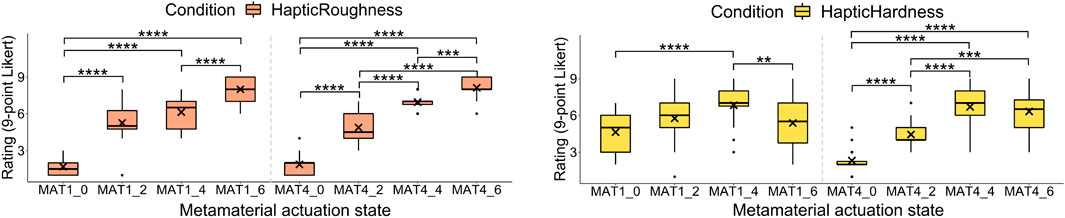
FIGURE 7. Haptic baseline. Participants separate assessments for roughness (left) and hardness (right) for the different metamaterial actuation states.
Participants’ responses when being asked to identify the material without visual information. 64% of the collected answers were rubber or plastic variations for MAT1. Interestingly, we mostly observed other answers such as fish scales, tar, grass, tree branching or fine stone, in the actuation states and not in the non-actuated state. This suggests that there exist materials that are closely reassembled by the actuated metamaterials. For MAT4 however, substantially fewer alternatives to rubber and plastic (only 15%) were given. For example, tree bark, sandstone or metal. While the non-actuated metamaterials, MAT1 and MAT4, were mostly associated with rubber or plastic, the higher compression rates are potentially capable of providing more complex material sensations. Moreover, participants often used adjectives such as “rough” and “damaged” to describe metamaterials with greater actuated states.
5.7.1.2 Visual baseline
The results confirm our selection of visual material textures vary in expected roughness (F(6,30) = 4.025, p = .003, η2 = .653) and hardness (F(6,30) = 2.025, p = .023, η2 = .853) (see Figure 8). For example, glass and concrete have significantly (p < .0001) different roughness ratings, comparable to fabric and metal for hardness (p < .0001). Participants were able to identify all textures without prior information, purely upon visual inspection. We only observed three mismatches, metal and concrete were perceived as marble twice, and glass was perceived as ice once. Please note, the fabric material was described as “jeans” in 81% of answers, which was not concerning to us. This is a confirmation that our visual materials were accurately represented in VR.
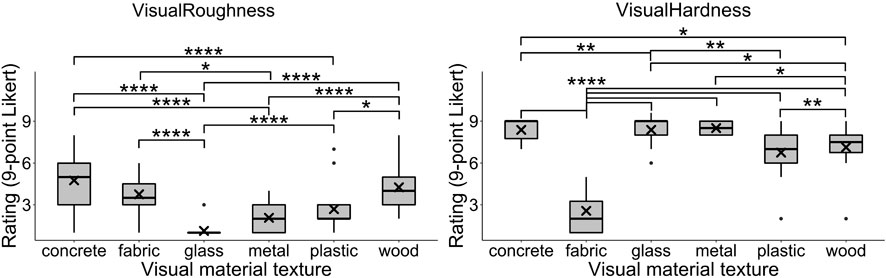
FIGURE 8. Visual baseline. Participants separate assessments for roughness (left) and hardness (right) for the different visual material textures.
5.7.2 Mixed perception results
5.7.2.1 Visuo-haptic perception
First, we analyse how adding visual material overlays affected the perceived roughness and hardness of our metamaterials. To do so, we compared participants’ roughness and hardness assessments from the haptic baseline to the visuo-haptic condition using multiple pairwise t-tests (Bonferroni-Holm adjusted) at each actuation state. The results are depicted in Figures 9, 10, suggesting that displaying a visual material texture on top of our metamaterials significantly affects the perception.
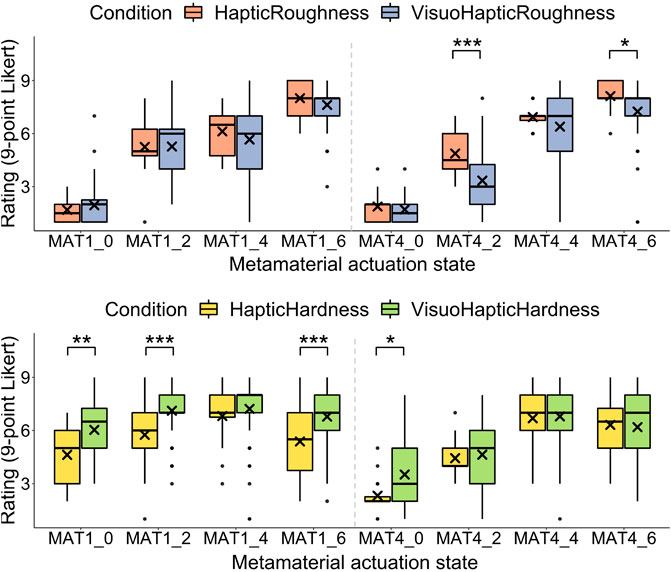
FIGURE 9. Haptic baseline vs. visuo-haptic comparison. Combined assessments for roughness (top) and hardness (bottom).
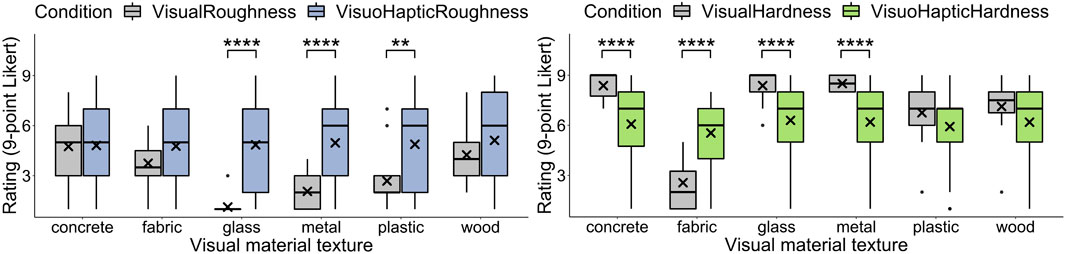
FIGURE 10. Similarity ratings in the visuo-haptic combination for MAT1 and MAT4 at each actuation state.
For hardness, MAT1, three out of four actuation states are perceived as significantly harder when a visual material was added. For MAT4, only zero actuation shows a significant increase in reported hardness (p < .05). When comparing the visual baseline to the visuo-haptic condition, we found similar effects. Expected hardness based on vision was significantly different to the visuo-haptic hardness score for four out of six visual material textures: concrete (p < .0001), fabric (p < .0001), glass (p < .0001) and metal (p < .0001). Fabric was predicted to be softer, but felt harder in contrast to concrete, glass and metal which were softer than anticipated.
For roughness, only MAT4 at the two actuation states 2 and 6 mm were perceived significantly less rough when a visual material was added compared to the haptic baseline. However, visual baseline and visuo-haptic condition, significantly differed for three out of six visual material textures: glass (p < .0001), metal (p < .0001), plastic (p < .01). Here, adding a metamaterial significantly increased roughness for the three materials.
Together with the findings above, we conclude that participants mostly relied on their haptic sense to assess roughness and hardness of a metamaterial, and that adding visual information can lead to a perceptual shift.
5.7.2.2 Perception of similarity
For each visuo-haptic combination, we recorded the similarity rating of participants’ visual and haptic sensations. The average matching rate for each combination is shown in Table 1.
For both metamaterials, we compared the ratings between the 4 compression levels using Friedman tests (α = .05) with post-hoc analysis using Wilcoxon signed ranks tests and Bonferroni-Holm correction. Overall, the similarity ratings were found to significantly differ depending on the level of compression the material was in (MAT1: χ2(3) = 55.590, p < .001, W = .191; MAT4: χ2(3) = 48.791, p < .001, W = .169). Pairwise comparisons found significant differences between all compression states excluding 2 mm and 4 mm for MAT1, and 0 mm and 2 mm, and 4 mm and 6 mm for MAT4 (see Figure 11). Furthermore, Kendall’s tau-b correlation tests indicated a medium, negative correlation between the rating of visuo-haptic similarity and the compression state of a metamaterial (τ = −0.273, p < .001, N = 768). These results indicate that as the metamaterial was compressed, the visual and haptic impressions deviated from each other, showing an increase in mismatching perception.
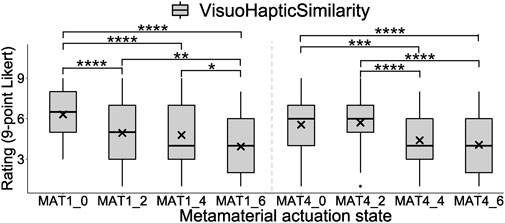
FIGURE 11. Similarity ratings in the visuo-haptic combination for MAT1 and MAT4 at each actuation state.
For each metamaterial, we additionally compared the similarity ratings between the 6 virtual textures using Friedman tests (α = .05) with post-hoc analysis using Wilcoxon signed ranks tests and Bonferroni-Holm correction. Overall, the similarity ratings were found to significantly differ depending on the visual material overlaid (MAT1: χ2(5) = 28.614, p < .001, W = .089; MAT4: χ2(5) = 25.844, p < .001, W = .081). Pairwise comparisons indicated significant differences between the pairs of glass-concrete, glass-metal, glass-wood, and wood-fabric for MAT1, and between the pairs of glass-concrete, glass-fabric, glass-plastic, and glass-wood for MAT4 (see Figure 12). From these results, we see that the similarity ratings were significantly lower for virtual glass, indicating that visuo-haptic mismatches were easily perceived for this texture.
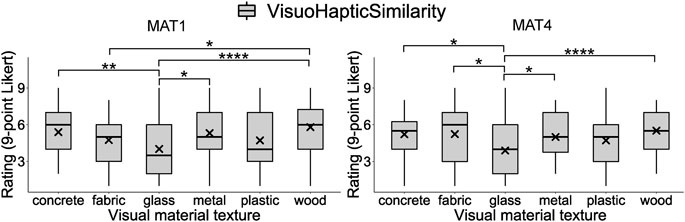
FIGURE 12. Similarity ratings in the visuo-haptic combination for MAT1 (left) and MAT4 (right) for each visual material overlay.
5.7.2.3 Subjective material perception
The anecdotal data of the perceived materials was further analysed by manually extracting the materials and objects participants identified. We characterized both abstract and concrete depictions into a set of 28 distinct perceptions. Here, we found 8 recurring categories, namely, stone-like (stone, tile, concrete, rock, marble, pebbles), wood-like (wood, bark), glass-like (glass), fabric-like (fabric, cotton, silk, wool, jeans, pad, car seat fabric), plastic-like (plastic, laminate, epoxy, plastic doormat, toy), metal-like (metal, sheet, splinter, tank armour), rubber-like (rubber, tire), and other (water). From this, we note that our set of metamaterials combined with visual textures was able to elicit a wider range of material perceptions.
To explain their perception, participants used adjectives to detail on the materials and objects in 28.52% of all indications. We further analysed adjectives by grouping them into 4 categories, i.e., tactile, visual, material, and geometric. In most cases where adjectives were used (66.52% of all adjectives), tactile properties indicated sensations such as roughness, smoothness, or the presence of a texture. Material properties (32.16%) were used to indicate the state of a perceived object, e.g., damaged, broken, or worn. Furthermore, a small amount of adjectives referred to either visual properties (0.88%), e.g., shiny, or geometric properties (0.44%), e.g., small. From this, we see that adjectives were mostly referring to users’ tactile perception.
We further investigated the relationship between the rating of visuo-haptic similarity, the compression state of the metamaterials, and the subjectively perceived materials. Kendall’s tau-b correlation tests indicated a small but significant positive correlation between the use of an adjective and the compression state of a metamaterial (τ = .110, p < .001, N = 768). However, there was no correlation found between the visuo-haptic similarity rating and the compression state of a metamaterial (τ = .038, p = .226, N = 768). From this, we see that adjectives were likely not used to explain mismatches between users’ visual and haptic perception, but more likely to elaborate on the increased amount of surface features upon compression of the metamaterials.
5.7.3 Summary
Our main study supports the findings and hypothesis H1.1 and H1.2 from our first preliminary experiment. Hence, we conclude that metamaterial patterns MAT1 and MAT4 can create distinct levels of roughness, and especially MAT4, also for hardness. When combining our metamaterials with visual material textures, we often observed a drift in perception H2.1. For example, glass was rated with the lowest roughness score; however, when combined with our metamaterials received significantly higher scores. Thus, participants relied more on their haptic sense in case of increasingly conflicting sensory (haptic and visual) information. Our analysis on the similarity ratings suggest that as the metamaterial was compressed, the visual and haptic impressions deviated from each other, showing an increase in mismatching perception—most likely caused by the lack of adaption of the virtual materials. This was supported by the high similarity ratings for the 0 and 2 mm actuation states, contrary to the significant decline at 4 and 6 mm H2.2.
6 Discussion
Motivated by recent advancements in the field of fabrication, we investigated the use of metamaterial textures for influencing tactile perception.
6.1 Metamaterials for touch experiences
Our work started out with the design and fabrication of 5 different metamaterials. Through lateral compression, the surfaces were able to dynamically change their physical configuration, which influenced their compliance and surface texture. From the results of our preliminary perceptual user study, we show these different physical configurations of our metamaterials translate to variations in their perceived tactile hardness and roughness. While we found a clear relationship between the compression state of a metamaterial and its tactile perception, not all designs consistently influenced perception in the same manner.
Our results contribute to the field of fabrication and underline that metamaterials are able to extend existing methods for fabricating feel aesthetics (Torres et al., 2015). The careful construction of metamaterials allows for the design of different tactile states that are able to communicate different sensations. Our psychophysical investigation bridges the gap between fabrication of metamaterial textures (Ion et al., 2018) and their perception. In a further step, a perceptual modelling approach would enable procedural design of different tactile states in single fabricated samples (Piovarči et al., 2016).
6.2 Metamaterials for passive haptic feedback
In a second study, we evaluated the use of a subset of our metamaterials in a virtual setting. The baseline analysis shows that the visual properties of our set of virtual textures clearly communicated different impressions. For our physical metamaterials, the haptic baseline indicated significant differences of roughness perception between compression states, with limited differences for hardness perception. Through combining visual and haptic stimuli, we see that our metamaterials were able to serve as passive haptic proxies for virtual textures.
Depending on the virtual texture and the metamaterial used, visuo-haptic integration was able to serve a consistent tactile experience. This is underlined by the similarity ratings of the visual and haptic stimuli. However, discrepancies between visual and tactile features drove perception to align with the haptic stimuli, causing haptic dominance. A similar effect shown by Iesaki et al. (2008), stated that although tactile impressions can be intentionally changed by providing appropriate visual stimulation, the coarseness of the visual and tactile textures has to be close to each other. This is further illustrated by the indicated material perceptions. As highly matching sensations were able to elicit clear material perceptions, discrepancies were explained through the use of adjectives.
Our work builds upon previous work in combining visual and haptic impressions to influence material perception (Kitahara et al., 2010; Degraen et al., 2019), and fabrication technologies for designing varying psychophysical impressions (Piovarči et al., 2016; Groeger et al., 2019; Degraen et al., 2021b). From our results, we see that haptically-varying metamaterial surfaces enable material perception in combination with visual textures. As users actively try to make sense of sensory input, further work investigating the thresholds of visuo-haptic discrepancies is needed to understand how consistent material perceptions can be simulated.
As underlined by the field of haptic design, there is a pressing need for intuitive and comprehensive approaches to enable designers to create and share convincing and immersive experiences (Schneider et al., 2017; 2022; Degraen et al., 2020). Rather than taking an active feedback approach (Degraen et al., 2021a; Wittchen et al., 2022), we envision the use of fabrication technologies for designing haptic experiences. Specifically in virtual environments, the visuo-haptic perception of fabricated artefacts is able to drive on-demand creation of haptic experiences.
7 Limitations and future work
Our work presents an initial investigation into the use of metamaterials for tactile experiences through passive haptic feedback in VR. Our results are bound by several limitations that should be improved.
7.1 Study limitations
In this first investigation, we asked participants to explore our metamaterials through circular lateral movement using their index finger, which was monitored by the experimenter. Since the perception of textures is highly motion depended (Sato et al., 2020), the lack of rigid control might have influenced our results. This needs to be addressed in future psychological experiments by, e.g., only allowing passive exploration through a robotic device, controlling for various interaction parameters such as velocity, pressure and trajectory. Future work also needs to improve the understanding of how the compression rate of metamaterials affect the magnitude of, e.g., perceived roughness. For example, by determining the just-noticeable-difference (JND) between actuation states to more deeply investigate their relationship.
7.2 Visuo-haptic matching
A main limitation illustrated by our results is the lack of appropriate adaptation of the used virtual models. In our naive approach, we did not alter the virtual model overlaid onto the fabricated samples. As the lateral compression of our samples influenced their physical configuration, participants clearly noted discrepancies between their tactile and visual perception. For high levels of compression, this visuo-haptic mismatch led to haptic dominance, i.e., the scenario where participants relied on their haptic sense to guide their entire perception. Future iterations need to ensure that the physical metamaterials are correctly aligned with the virtual object they are paired with (Nilsson et al., 2021).
To adapt virtual models appropriately, an accurate approach is needed to record the state of the metamaterial in question while tracking the user’s interaction with the sample. Recent advancements in multi-material fabrication have allowed for the integration of conductive materials that can be used for capacitive sensing. This would allow tracking a user’s finger during interaction. Recent work has shown that sensing mechanisms can be directly embedded into the fabrication process to track the state of the metamaterial (Gong et al., 2021). Through such sensing approaches, the virtual representation can be adapted to emphasize the presence of more features.
7.3 Actuating metamaterials
Our metamaterials changed their physical configuration through lateral compression. For this, we designed and create a setup that included an electromotor for actuating linear gears, controller by a microcontroller. Internal support for the surfaces was provided through embedded metal pipes that controlled unwanted deformations by stabilizing the samples. During our perceptual studies, we used pre-compressed samples to ensure consistency across trials and to reduce the study duration. As our current setup remains bulky and lacks precision, there is certainly room for improvement. For example, by embedding the actuating elements directly in the metamaterial design (Xiao et al., 2020).
7.4 Fabrication of perceptual features
One of the key limitations that we encountered while prototyping was the metamaterial’s feature size that we could reliably print with an off-the-shelf FDM 3D-printer. We believe that there is still potential to improve upon our results and create other metamaterials with different surface texture information. Perhaps other fabrication methods such as stereolithography (SLA) 3D-printing, can be used to fabricate metamaterials, including smaller features, which may benefit the haptic resolution. In addition, approaches such as Taclets by Groeger et al. (2019) which enable rich electro-tactile feedback on various 3D-printed geometries, may help to overcome such limitations. To further increase the resolution, our approach can be combined with methods that capture real world surface information (Degraen et al., 2021b). Finally, this can be used in a perceptual modeling to ensure the user’s perception aligns with the designer’s intention (Piovarči et al., 2016).
At this early stage, we did not conduct a technical evaluation investigating longevity and potential issues caused by material deterioration. We observed that our actuation approach suffers from inaccuracies that need be overcome in future iterations, and therefore decided to use fixed samples in our experiments.
7.5 Visuo-haptic integration
Our method builds upon passive haptic feedback approaches that pair physical objects with virtual representations to create visuo-haptic experiences in VR. As pointed out by related work, such an approach has the potential to improve the user’s sense of presence, positively impacting their experience (Insko, 2001). The idea of metamaterials builds upon the concept of fabricating abstract objects that influence tactile dimensions in order to build texture or material impressions in combination with visual information (Degraen et al., 2019). By capturing the baseline assessments, we were able to receive an initial impression of what users might expect for both modalities. For example, when visually assessing a texture, some users might interpret the texture completely different, leading to different tactile expectations. This might have cause a bias that influenced their further assessments.
Through the visuo-haptic combinations, we aimed to understand how different combinations matched and how they were able to build consistent material impressions. Future work would need to explore how the presence of roughness and hardness guides material perception in a more general manner, and how immersive environments benefit from these combinations in terms of realism and presence.
7.6 Beyond touch
Expanding our approach to real-world use cases may create the demand for local and global controllability, rather than uniform compression as designers will need to integrate local textures variations. We believe that more advanced metamaterials can fulfill these requirements, because they can actuate themselves in a cell-by-cell fashion (Xiao et al., 2020). We are also interested in taking this approach beyond one finger touch interactions. For example, Ion et al. (2018) presented a bike handle, differing in friction, depending on the compression of the metamaterial. Similarly, we could envision metamaterials that augment controller-based interfaces (Zenner and Krüger, 2017; Whitmire et al., 2018). Here, auxetics metamaterial designs might be worth investigating in this context, because their from factor remain unaffected upon actuation (Steed et al., 2021); instead, our current metamaterial designs become smaller, reducing the interaction area.
8 Conclusion
In this work, we presented a novel concept for simulating tactile experiences in VR using 3D-printed metamaterial actuation. We described the fabrication process of five metamaterials which we evaluated in two experiments. As a first proof of concept, we investigated how participants perceive the metamaterials and their actuation states across the perceptual dimensions roughness and hardness. In summary, MAT1–MAT4 showed promising results for roughness, but only MAT4 for hardness. Based on these insights, we selected two metamaterial patterns that provide the widest range of tactile impressions (MAT1 and MAT4). Next, we conducted our main experiment, exploring the use of these metamaterial patterns as passive haptic proxies for simulating visuo-haptic material experiences in VR. Our results suggest, that metamaterials are able to support tactile material perception through visuo-haptic stimuli. Considering the room for improvement beyond this first investigation, we believe that proxy design can truly benefit from applying metamaterial structures, and that our work only marks a first step in this direction.
Data availability statement
The original contributions presented in the study are included in the article/Supplementary Material, further inquiries can be directed to the corresponding authors.
Ethics statement
The studies involving human participants were reviewed and approved by Ethical review board of the faculty of Mathematics and Computer Science at Saarland University. The patients/participants provided their written informed consent to participate in this study.
Author contributions
MF and DD wrote the first draft of the manuscript. All authors contributed to the article and approved the submitted version.
Funding
This research was funded in part by the German Federal Ministry of Education and Research (BMBF) under grant number 01IS17043 (project VRGiO) and by the Deutsche Forschungsgemeinschaft (DFG, German Research Foundation), projects 450247716 and 425868555, the latter being part of Priority Program SPP2199 Scalable Interaction Paradigms for Pervasive Computing Environments.
Acknowledgments
We would like to thank our participants for their time and valuable feedback.
Conflict of interest
The authors declare that the research was conducted in the absence of any commercial or financial relationships that could be construed as a potential conflict of interest.
Publisher’s note
All claims expressed in this article are solely those of the authors and do not necessarily represent those of their affiliated organizations, or those of the publisher, the editors and the reviewers. Any product that may be evaluated in this article, or claim that may be made by its manufacturer, is not guaranteed or endorsed by the publisher.
Supplementary Material
The Supplementary Material for this article can be found online at: https://www.frontiersin.org/articles/10.3389/frvir.2023.1172381/full#supplementary-material
Supplementary Data Sheet 1 | 3D models of metamaterials.
References
Araujo, B., Jota, R., Perumal, V., Yao, J. X., Singh, K., and Wigdor, D. (2016). “Snake charmer: Physically enabling virtual objects,” in Proceedings of the TEI ’16: Tenth international conference on tangible, embedded, and embodied interaction (New York, NY, USA: Association for Computing Machinery), 16, 218–226. doi:10.1145/2839462.2839484
Benko, H., Holz, C., Sinclair, M., and Ofek, E. (2016). “Normaltouch and texturetouch: High-fidelity 3d haptic shape rendering on handheld virtual reality controllers,” in Proceedings of the 29th annual symposium on user interface software and technology (New York, NY, USA: Association for Computing Machinery), 16, 717–728. doi:10.1145/2984511.2984526
Bergström, J., Mottelson, A., and Knibbe, J. (2019). “Resized grasping in vr: Estimating thresholds for object discrimination,” in Proceedings of the 32nd Annual ACM Symposium on User Interface Software and Technology, New Orleans LA USA, October 20 - 23, 2019, 1175–1183. doi:10.1145/3332165.334793919
Bickel, B., Bächer, M., Otaduy, M. A., Lee, H. R., Pfister, H., Gross, M., et al. (2010). Design and fabrication of materials with desired deformation behavior. ACM Trans. Graph. 29, 1–10. doi:10.1145/1778765.1778800
Charrad, M., Ghazzali, N., Boiteau, V., and Niknafs, A. (2014). Nbclust: An r package for determining the relevant number of clusters in a data set. J. Stat. Softw. 61, 1–36. doi:10.18637/jss.v061.i06
Clarke, K. R. (1993). Non-parametric multivariate analyses of changes in community structure. Aust. J. Ecol. 18, 117–143. doi:10.1111/j.1442-9993.1993.tb00438.x
Cooke, T., Kannengiesser, S., Wallraven, C., and Bülthoff, H. H. (2006). Object feature validation using visual and haptic similarity ratings. ACM Trans. Appl. Percept. 3, 239–261. doi:10.1145/1166087.1166093
Culbertson, H., Unwin, J., Goodman, B. E., and Kuchenbecker, K. J. (2013). Generating haptic texture models from unconstrained tool-surface interactions. World Haptics Conf. (WHC) 2013, 295–300. doi:10.1109/WHC.2013.6548424
Degraen, D., Fruchard, B., Smolders, F., Potetsianakis, E., Güngör, S., Krüger, A., et al. (2021a). “Weirding haptics: In-situ prototyping of vibrotactile feedback in virtual reality through vocalization,” in The 34th Annual ACM Symposium on User Interface Software and Technology, United States, October 10 - 14, 2021, 936–953. doi:10.1145/3472749.3474797
Degraen, D., Piovarči, M., Bickel, B., and Krüger, A. (2021b). Capturing tactile properties of real surfaces for haptic reproduction. In The 34th annual ACM symposium on user interface software and technology (New York, NY, USA: Association for Computing Machinery), UIST ’21, 954–971. doi:10.1145/3472749.3474798
Degraen, D., Reindl, A., Makhsadov, A., Zenner, A., and Krüger, A. (2020). “Envisioning haptic design for immersive virtual environments,” in Companion publication of the 2020 ACM designing interactive systems conference (New York, NY, USA: Association for Computing Machinery), DIS’ 20 Companion), 287–291. doi:10.1145/3393914.3395870
Degraen, D., Zenner, A., and Krüger, A. (2019). “Enhancing texture perception in virtual reality using 3d-printed hair structures,” in Proceedings of the 2019 CHI conference on human factors in computing systems (New York, NY, USA: Association for Computing Machinery), CHI ’19, 1–12. doi:10.1145/3290605.3300479
Dellon, A. L. (1978). The moving two-point discrimination test: Clinical evaluation of the quickly adapting fiber/receptor system. J. Hand Surg. 3, 474–481. doi:10.1016/S0363-5023(78)80143-9
Ernst, M. O. (2012). “Optimal multisensory integration: Assumptions and limits,” in The new handbook of multisensory processing (Massachusetts, United States: The MIT Press). doi:10.7551/mitpress/8466.003.0048
Feick, M., Bateman, S., Tang, A., Miede, A., and Marquardt, N. (2020a). “Tangi: Tangible proxies for embodied object exploration and manipulation in virtual reality,” in Proccedings of the 2020 IEEE International Symposium on Mixed and Augmented Reality, 9-13 Nov. 2020, 195–206. doi:10.1109/ISMAR50242.2020.00042
Feick, M., Kleer, N., Tang, A., and Krüger, A. (2020b). “The virtual reality questionnaire toolkit,” in Adjunct proceedings of the 33rd annual ACM symposium on user interface software and technology (New York, NY, USA: Association for Computing Machinery), 68–69. UIST ’20 Adjunct. doi:10.1145/3379350.3416188
Feick, M., Kleer, N., Zenner, A., Tang, A., and Krüger, A. (2021). “Visuo-haptic illusions for linear translation and stretching using physical proxies in virtual reality,” in Proceedings of the 2021 CHI conference on human factors in computing systems (New York, NY, USA: Association for Computing Machinery). CHI ’21. doi:10.1145/3411764.3445456
Gangwar, K., and Gangwar, R. (2014). Metamaterials: Characteristics, process and applications. Adv. Electron. Electr. Eng. 4, 97–106.
Gedsun, A., Sahli, R., Meng, X., Hensel, R., and Bennewitz, R. (2022). Bending as key mechanism in the tactile perception of fibrillar surfaces. Adv. Mater. Interfaces 9, 2101380. doi:10.1002/admi.202101380
Gong, J., Seow, O., Honnet, C., Forman, J., and Mueller, S. (2021). “Metasense: Integrating sensing capabilities into mechanical metamaterial,” in The 34th annual ACM symposium on user interface software and technology (New York, NY, USA: Association for Computing Machinery), 1063–1073. UIST ’21. doi:10.1145/3472749.3474806
Groeger, D., Feick, M., Withana, A., and Steimle, J. (2019). Tactlets: Adding tactile feedback to 3d objects using custom printed controls. In Proceedings of the 32nd annual ACM symposium on user interface software and technology (New York, NY, USA: Association for Computing Machinery), UIST ’19, 923–936. doi:10.1145/3332165.3347937
Iesaki, A., Somada, A., Kimura, A., Shibata, F., and Tamura, H. (2008). “Psychophysical influence on tactual impression by mixed-reality visual stimulation,” in Proceedings of the 2008 IEEE Virtual Reality Conference, Reno, NV, USA, 08-12 March 2008 (IEEE), 265–266. doi:10.1109/VR.2008.4480793
Insko, B. E. (2001). Passive haptics significantly enhances virtual environments. Ph.D. thesis. Chapel Hill, NC: The University of North Carolina at Chapel Hill.
Ion, A., Kovacs, R., Schneider, O. S., Lopes, P., and Baudisch, P. (2018). “Metamaterial textures,” in Proceedings of the 2018 CHI conference on human factors in computing systems (New York, NY, USA: Association for Computing Machinery), 18, 1–12. doi:10.1145/3173574.3173910
Ishizaki, K., Komarneni, S., and Nanko, M. (2013). Porous materials: Process technology and applications, 4. Berlin, Germany: Springer science and business media.
Kitahara, I., Nakahara, M., and Ohta, Y. (2010). Sensory properties in fusion of visual/haptic stimuli using mixed reality. Adv. Haptics. doi:10.5772/8712
Klatzky, R. L., Pawluk, D., and Peer, A. (2013). Haptic perception of material properties and implications for applications. Proc. IEEE 101, 2081–2092. doi:10.1109/JPROC.2013.2248691
Lécuyer, A. (2009). Simulating haptic feedback using vision: A survey of research and applications of pseudo-haptic feedback. Presence Teleoperators Virtual Environ. 18, 39–53. doi:10.1162/pres.18.1.39
Lee, C.-J., Tsai, H.-R., and Chen, B.-Y. (2021). “Hairtouch: Providing stiffness, roughness and surface height differences using reconfigurable brush hairs on a vr controller,” in Proceedings of the 2021 CHI Conference on Human Factors in Computing Systems, Yokohama Japan, May 8 - 13, 2021. CHI ’21. doi:10.1145/3411764.3445285
Lu, G., Lu, G., and Xiao, Z. (1999). Mechanical properties of porous materials. J. Porous Mater. 6, 359–362. doi:10.1023/a:1009669730778
Myers, N. (1962). Characterization of surface roughness. Wear 5, 182–189. doi:10.1016/0043-1648(62)90002-9
Neville, R. M., Scarpa, F., and Pirrera, A. (2016). Shape morphing kirigami mechanical metamaterials. Sci. Rep. 6, 31067. doi:10.1038/srep31067
Nilsson, N. C., Zenner, A., and Simeone, A. L. (2021). Propping up virtual reality with haptic proxies. IEEE Comput. Graph. Appl. 41, 104–112. doi:10.1109/MCG.2021.3097671
Okamoto, S., Nagano, H., and Yamada, Y. (2013). Psychophysical dimensions of tactile perception of textures. IEEE Trans. Haptics 6, 81–93. doi:10.1109/toh.2012.32
Piovarči, M., Levin, D. I. W., Rebello, J., Chen, D., Ďurikovič, R., Pfister, H., et al. (2016). An interaction-aware, perceptual model for non-linear elastic objects. ACM Trans. Graph. 35, 1–13. doi:10.1145/2897824.2925885
Punpongsanon, P., Iwai, D., and Sato, K. (2015). Softar: Visually manipulating haptic softness perception in spatial augmented reality. IEEE Trans. Vis. Comput. Graph. 21, 1279–1288. doi:10.1109/TVCG.2015.2459792
Robles-De-La-Torre, G. (2006). The importance of the sense of touch in virtual and real environments. IEEE Multimed. 13, 24–30. doi:10.1109/MMUL.2006.69
Sato, Y., Hiraki, T., Tanabe, N., Matsukura, H., Iwai, D., and Sato, K. (2020). Modifying texture perception with pseudo-haptic feedback for a projected virtual hand interface. IEEE Access 8, 120473–120488. doi:10.1109/ACCESS.2020.3006440
Schneider, O., Fruchard, B., Wittchen, D., Joshi, B. R., Freitag, G., Degraen, D., et al. (2022). “Sustainable haptic design: Improving collaboration, sharing, and reuse in haptic design research,” in Proceedings of the CHI Conference on Human Factors in Computing Systems Extended Abstracts, New Orleans LA USA, 29 April 2022- 5 May 2022. CHI EA ’22. doi:10.1145/3491101.3503734
Schneider, O., MacLean, K., Swindells, C., and Booth, K. (2017). Haptic experience design: What hapticians do and where they need help. Int. J. Human-Computer Stud. 107, 5–21. doi:10.1016/j.ijhcs.2017.04.004
Schumacher, C., Bickel, B., Rys, J., Marschner, S., Daraio, C., and Gross, M. (2015). Microstructures to control elasticity in 3d printing. ACM Trans. Graph. 34, 1–13. doi:10.1145/2766926
Slater, M. (2009). Place illusion and plausibility can lead to realistic behaviour in immersive virtual environments. Philosophical Trans. R. Soc. B Biol. Sci. 364, 3549–3557. doi:10.1098/rstb.2009.0138
Speicher, M., Ehrlich, J., Gentile, V., Degraen, D., Sorce, S., and Krüger, A. (2019). “Pseudo-haptic controls for mid-air finger-based menu interaction,” in Proceedinngs of the Extended Abstracts of the 2019 CHI Conference on Human Factors in Computing Systems, Glasgow Scotland Uk, May 4 - 9, 2019, 1–6. CHI EA ’19. doi:10.1145/3290607.3312927
Steed, A., Ofek, E., Sinclair, M., and Gonzalez-Franco, M. (2021). A mechatronic shape display based on auxetic materials. Nat. Commun. 12, 4758. doi:10.1038/s41467-021-24974-0
Strohmeier, P., Boring, S., and Hornbæk, K. (2018). From pulse trains to ”coloring with vibrations”: Motion mappings for mid-air haptic textures. In Proceedings of the 2018 CHI Conference on Human Factors in Computing Systems, April 21 - 26, 2018, QC Canada, doi:10.1145/3173574.3173639
Takahashi, H., and Kim, J. (2022). “Designing a hairy haptic display using 3d printed hairs and perforated plates,” in Adjunct proceedings of the 35th annual ACM symposium on user interface software and technology (New York, NY, USA: Association for Computing Machinery). UIST ’22 Adjunct. doi:10.1145/3526114.3558655
Torres, C., Campbell, T., Kumar, N., and Paulos, E. (2015). “Hapticprint: Designing feel aesthetics for digital fabrication,” in Proceedings of the 28th annual ACM symposium on user interface software and technology (New York, NY, USA: ACM), 583–591. UIST ’15. doi:10.1145/2807442.2807492
Valipour, A., Kargozarfard, M. H., Rakhshi, M., Yaghootian, A., and Sedighi, H. M. (2022). Metamaterials and their applications: An overview. Proc. Institution Mech. Eng. Part L J. Mater. Des. Appl. 236, 2171–2210. doi:10.1177/1464420721995858
Vardar, Y., Wallraven, C., and Kuchenbecker, K. J. (2019). “Fingertip interaction metrics correlate with visual and haptic perception of real surfaces,” in Proceedings of the 2019 IEEE World Haptics Conference, Tokyo, Japan, 09-12 July 2019. doi:10.1109/whc.2019.8816095
Whitmire, E., Benko, H., Holz, C., Ofek, E., and Sinclair, M. (2018). “Haptic revolver: Touch, shear, texture, and shape rendering on a reconfigurable virtual reality controller,” in Proceedings of the 2018 CHI Conference on Human Factors in Computing Systems, Montreal QC Canada, April 21 - 26, 2018, 1–12. CHI ’18. doi:10.1145/3173574.3173660
Wittchen, D., Spiel, K., Fruchard, B., Degraen, D., Schneider, O., Freitag, G., et al. (2022). “TactJam: An end-to-end prototyping suite for collaborative design of on-body vibrotactile feedback,” in Proceedings of the Sixteenth International Conference on Tangible, Embedded, and Embodied Interaction, Korea, February 13 - 16, 2022. doi:10.1145/3490149.3501307
Xiao, S., Wang, T., Liu, T., Zhou, C., Jiang, X., and Zhang, J. (2020). Active metamaterials and metadevices: A review. J. Phys. D Appl. Phys. 53, 503002. doi:10.1088/1361-6463/abaced
Yang, W. Y., Zhuang, Y., Darcy, L. A., Liu, G., and Ion, A. (2022). “Reconfigurable elastic metamaterials,” in Proceedings of the 35th Annual ACM Symposium on User Interface Software and Technology, Bend OR USA, 29 October 2022- 2 November 2022. UIST ’22. doi:10.1145/3526113.3545649
Zenner, A., and Krüger, A. (2017). Shifty: A weight-shifting dynamic passive haptic proxy to enhance object perception in virtual reality. IEEE Trans. Vis. Comput. Graph. 23, 1285–1294. doi:10.1109/TVCG.2017.2656978
Samad, M., Gatti, E., Hermes, A., Benko, H., and Parise, C. (2019). Pseudo-haptic weight: Changing the perceived weight of virtual objects by manipulating control-display ratio. In Proceedings of the 2019 CHI Conference on Human Factors in Computing Systems, May 4 - 9, 2019, Glasgow Scotland Uk, CHI ’19, 1–13. doi:10.1145/3290605.3300550
Keywords: Virtual Reality, haptic (tactile) illusion, fabrication, 3D-print, metamaterial, haptic (tactile) perception, active haptics, passive haptics
Citation: Feick M, Degraen D, Hupperich F and Krüger A (2023) MetaReality: enhancing tactile experiences using actuated 3D-printed metamaterials in Virtual Reality. Front. Virtual Real. 4:1172381. doi: 10.3389/frvir.2023.1172381
Received: 23 February 2023; Accepted: 21 April 2023;
Published: 28 June 2023.
Edited by:
Anıl Ufuk Batmaz, Concordia University, CanadaCopyright © 2023 Feick, Degraen, Hupperich and Krüger. This is an open-access article distributed under the terms of the Creative Commons Attribution License (CC BY). The use, distribution or reproduction in other forums is permitted, provided the original author(s) and the copyright owner(s) are credited and that the original publication in this journal is cited, in accordance with accepted academic practice. No use, distribution or reproduction is permitted which does not comply with these terms.
*Correspondence: Martin Feick, bWFydGluLmZlaWNrQGRma2kuZGU=; Donald Degraen, ZG9uYWxkLmRlZ3JhZW5AZGZraS5kZQ==
†These authors have contributed equally to this work and share first authorship