- 1School of Public Health and Sport Science, University of Exeter, Exeter, United Kingdom
- 2Newman and Spurr Consulting, Meadows Business Park, Camberley, United Kingdom
- 3Defence Science and Technology Laboratory, Porton Down, Salisbury, United Kingdom
- 4Cineon Training, Exeter Science Park Centre, Exeter, United Kingdom
Introduction: Simulation methods, including physical synthetic environments, already play a substantial role in human skills training in many industries. One example is their application to developing situational awareness and judgemental skills in defence and security personnel. The rapid development of virtual reality technologies has provided a new opportunity for performing this type of training, but before VR can be adopted as part of mandatory training it should be subjected to rigorous tests of its suitability and effectiveness.
Methods: In this work, we adopted established methods for testing the fidelity and validity of simulated environments to compare three different methods of training use-of-force decision making. Thirty-nine dismounted close combat troops from the UK’s Royal Air Force completed shoot/don’t-shoot judgemental tasks in: i) live fire; ii) virtual reality; and iii) 2D video simulation conditions. A range of shooting accuracy and decision-making metrics were recorded from all three environments.
Results: The results showed that 2D video simulation posed little decision-making challenge during training. Decision-making performance across live fire and virtual reality simulations was comparable but the two may offer slightly different, and perhaps complementary, methods of training judgemental skills.
Discussion: Different types of simulation should, therefore, be selected carefully to address the exact training need.
1 Introduction
Simulation training involves the use of synthetic or computerised environments to replicate real-world scenarios for developing skills. Simulation training is used by a wide variety of industries where real-world practice is challenging or impossible, for reasons including cost, practicality, safety, and availability of facilities. In certain situations, some form of simulation is unavoidable, even if it involves basic synthetic replications like practicing surgical suturing on fruit (e.g., Wong et al., 2018). In aviation, high-fidelity flight simulators form a fundamental part of pilot training because practicing in a real plane is both prohibitively expensive and poses a risk to life (Salas et al., 1998). Trainees in nuclear decommissioning often have to prepare to use equipment that does not yet exist or which cannot be taken out of service for training, in addition to the obvious safety concerns (Popov et al., 2021). In other scenarios, real-world practice is available, but simulation is chosen because it is more convenient or cost effective. For example, even though sporting tasks are rarely that difficult to recreate, there is growing interest in computerised simulations such as virtual reality (VR), augmented reality (AR), and mixed reality (MR) as a way to train sporting skills (Harris et al., 2020; Wood et al., 2020). In sport and related applications like rehabilitation (Alrashidi et al., 2022), computerised simulations enable individuals to perform additional practice on their own with almost endless variation, making them an attractive alternative (or addition to) physical training.
Recent technological advances mean that VR, AR, and MR technologies are now a highly attractive option for simulation training. These technologies enable high-fidelity simulations of a wide variety of environments, as well as being very cost effective and accessible to use. Concerns have, however, been raised about whether VR is appropriate for all types of training and whether VR environments are being appropriately tested before being adopted (Harris et al., 2020). Broadly speaking, a simulation (of whatever kind) is designed to replicate some aspects of a task (e.g., behavioural goals and task constraints) without reproducing others (e.g., danger and cost) (Stoffregen et al., 2003). Consequently, in order to conduct effective simulation training it is necessary to understand the degree of concordance between the simulated environment and the corresponding real-world task, and how any differences might impact learning (Harris et al., 2019; Valori et al., 2020). This assessment can be achieved through quantifying aspects of the fidelity and validity1 of the environment.
In previous work, researchers have identified several key aspects of fidelity and validity for performing simulation assessments. The physical fidelity of the environment—whether it looks and feels real—is often assessed by recording whether users feel they are fully immersed in the simulation, using self-reported presence ratings (Makransky et al., 2019; Harris et al., 2020). Researchers have previously compared participants’ performance on validated tests or measures with measures taken from the new simulator to establish concurrent validity, which refers to the amount of agreement between two different assessments (e.g., Xeroulis et al., 2009). As well as aligning with existing validated methods, an effective training simulation should also be sufficiently representative of the skill’s functionality that it can provide a good indicator of real-world expertise. This correspondence with the real-world is known as construct validity, which is often assessed by comparing the performance of experts and novices in the simulation (e.g., Bright et al., 2012; Wood et al., 2020). Lukosch et al. (2019) propose a framework for conceptualizing fidelity in human computer interfaces which focuses on the quality of the interaction between user and environment, not just realistic visual representation. Consequently, they also identify aspects of fidelity such as psychological and social fidelity. This emphasis on the realism of the interaction between user and environment is particularly pertinent when considering the application of VR to training complex perceptual-cognitive skills.
In the present work, we sought to examine three different methods of simulation that are used for training judgemental skills as part of military room clearance. In a military context, judgemental training refers to the process of developing the ability to identify threats and non-threats and deliver appropriate force with speed and accuracy. An example of this is shoot/don’t-shoot decision making. Making effective judgements requires situational awareness and the appropriate identification and use of information from the environment (Randel et al., 1996; Biggs et al., 2021). Realistic close quarter battle conditions are, however, very hard to recreate. Consequently, judgemental skills are normally trained using some form of synthetic or simulated training which allows these abilities to develop in a semi-realistic environment (Li and Harris, 2008; Armstrong et al., 2014; Nieuwenhuys et al., 2015; Staller and Zaiser, 2015).
The main options for judgemental training in the UK Ministry of Defence are screen-based firing-range simulations or live judgemental shoots. Screen-based firing-range simulations are delivered using a tool called the Dismounted Close Combat Trainer (DCCT), which consists of a large 2D video screen that trainees shoot at with decommissioned pistols, rifles, and support weapons. Alternatively, live judgemental shoots are undertaken in close quarters battle environments (physical simulations using mock-up rooms) using live ammunition or non-lethal training ammunition2. Live judgemental shoots provide a more realistic environment than the screen-based firing range but are restricted in both realism and flexibility by the static cardboard targets and homogenous physical room set-ups that must be manually reset. VR technologies may provide an attractive third option, that is both visually realistic and immersive while also allowing varied training possibilities. Given the need to test new training approaches to optimise human skills training across a range of industries (including Defence), we compared judgemental performance in these two existing options with head-mounted VR.
The approach we used to compare the simulation options for judgemental training was closely aligned with the previously discussed work that has assessed physical fidelity, concurrent validity, and construct validity (van Dongen et al., 2007; Bright et al., 2012; Perfect et al., 2014). To address these three aspects, we: i) collected user reports of presence to determine sufficient levels of fidelity; ii) conducted comparisons to (and correlations with) other approaches to test concurrent validity; iii) examined relationships with real-world expertise to test construct validity. As this work was a preliminary exploration of different methods of simulation for judgemental training, no specific hypotheses were made about the exact relationship between the different training environments.
2 Methods
2.1 Design
The study adopted a cross-sectional repeated-measures design, with all participants completing the three experimental conditions in a counterbalanced order to control for any learning effects. These conditions were a 2D video simulation, a live fire simulation, and a VR simulation of a room clearance task.
2.2 Participants
Participants were recruited from a population of dismounted close combat troops from the RAF Regiment’s Queen’s Colour Squadron (The RAF Force Protection Force). Participants were current and competent dismounted close combat personnel, ranging from Leading Aircraftman to Corporal in rank. All participants gave written informed consent prior to taking part in the study and were paid for their participation at a standard Ministry of Defence rate. The experimental procedures were reviewed by both the University of Exeter departmental ethics board and the Ministry of Defence Research Ethics Committee (reference number: 2102/MODREC/21).
An a priori power calculation was conducted before data collection to determine the sample size needed to support accurate conclusions. In a closely related study, Blacker et al. (2020) examined the relationship between a military grade simulator and a video game for shoot/don’t-shoot decision-making. Blacker et al. reported a relationship of r = 0.48 between the simulator and the video game for shot accuracy (i.e., hit rate). Consequently, given α = 0.05 and a power of 80%, 31 participants would be required to detect a similar sized effect in a bivariate correlation in the present study. Our sample consisted of 39 participants in total (see Table 1 for demographics) which was more than sufficient to detect similar sized effects. Thirty-six participants took part in all conditions, a further two participants also took part in the 2D video and VR conditions, and one took part in the VR condition only.
2.3 Conditions
For all three simulation conditions, participants completed 18 trials, 9 of which included a threatening target and 9 a non-threatening target. Trials were presented in a pseudorandomised order.
2.3.1 Virtual reality room clearance condition
For the VR room clearance condition, participants wore an HTC Vive Pro Eye head-mounted display (HTC Inc., Taoyuan City, Taiwan). This consumer-grade VR system uses two lighthouse base stations to track movements of the headset and hand controllers at 120 Hz. For this study, the VR system was specifically modified to record the use of a replica SA80 weapon (see Figure 1A, B). This replica device allowed participants to aim and shoot at targets using a trigger pull on the VR hand controller while they freely and naturally roamed the simulated training space. The virtual environment (see Figures 1C, D) consisted of a small rectangular room, which participants entered via an open door. On each trial, the inside of this room had slightly different visual design features (e.g., different furnishings and wall art), but its overall size and layout remained constant. Participants started outside and were required to enter and search each room, before deciding whether to shoot at the target or hold their fire. Participants were read a script instructing them to enter the room according to their training, which was standardised across all three conditions (see https://osf.io/vdk87/). To allow participants to familiarize themselves with the behaviour of the environment and the weapon, they were allowed to move around an empty room and fire the weapon, prior to any trials. They were not, however, shown any of the shoot/don’t-shoot stimuli before the trials began.
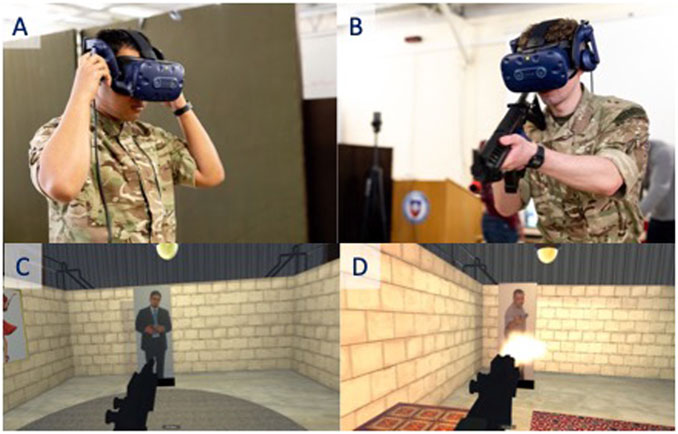
FIGURE 1. VR Hardware and software. The HTC Vive Pro VR head mounted display (A), the position tracked replica weapon (B), and in-play screenshots (C, D). Images are property of RAF Honington and protected by Crown Copyright©. Reproduced with permission.
A single target was generated within the virtual room on each training repetition. These simulated targets consisted of static images taken from the Sykes-McQueen 8,000 series threat assessment range (McQueen Targets, Scottish Borders, United Kingdom) (see Figure 3). The specific location of the targets was varied on a trial-by-trial basis. Crucially, the targets replicated those used in the live shooting conditions (see below) and were selected to provide closely matched variants of threatening and non-threatening cues. Each image displayed a single combatant, who was either holding a weapon (a gun) or a non-hostile object (e.g., a phone, torch, or camcorder). As such, correct decision-making responses would be demonstrated through shooting the threatening ‘enemy’ targets and withholding fire for ‘friendly’, non-threatening ones.
2.3.2 Live fire room clearance condition
The live shooting condition took place in a repurposed aircraft hangar that contained smaller rooms within it, designed for training room entry drills. The threatening/non-threatening stimuli for this condition consisted of cardboard targets displaying the same Sykes-McQueen images as in VR. Participants fired at the targets using SA80 weapons with the non-lethal training ammunition that discharged a small paint capsule that could then be identified on the target.
A single rectangular room was used for this condition. The trainee entered from the hallway, where the door to the room was already open, to match the automatic opening of the doors in the VR condition. As in the other conditions, each room contained one target, either a threat or a non-threat. After the participant had completed each room search (and exited the room), the experimenter entered from another door and changed the target in the room.
Participants’ decisions (shoot/don’t-shoot) were recorded in real-time by the experimenter and photographs were taken of the targets after each individual room search. Shooting accuracy was calculated later by measuring the distance of the shots from the centre of mass of the target, using the MATLAB programming environment (Mathworks, MA, United States). A GoPro Hero 4 camera was also attached to the helmet of the participant to record each trial from a first-person perspective. This video was later used to calculate reaction time using frame-by-frame video analysis (i.e., the time from appearance of the target in the participant’s field of view to the time a shot was made).
2.3.3 Video simulation room clearance condition
The 2D video simulation condition (using the Dismounted Close Combat Trainer) was undertaken on a virtual training range at RAF Honington, which comprised of a series of shooting lanes and a large projector screen (positioned at the front of the room; see Figure 2). Participants were equipped with a deactivated SA80 rifle that was connected to the controller’s base stations via Bluetooth signal. This cableless simulated training weapon was fitted with a specialised gas recycling system to replicate the action of the bolt.
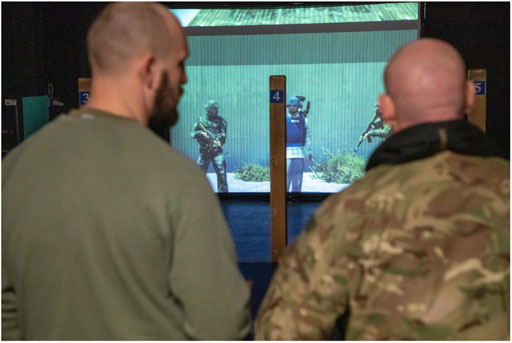
FIGURE 2. 2D video simulation condition. The Dismounted Close Combat Trainer. Image is property of RAF Honington and protected by Crown Copyright©. Reproduced with permission.
Participants were instructed by the experimenter to ready their weapon as they would when entering a room. Once ready, they were presented with a view of a target as though entry to the room had been made (i.e., as though they are standing in the doorway). They then made their shoot/don’t-shoot decision. As above, participants were presented with either a threatening or non-threatening target on each trial and were instructed to take lethal force actions when faced with an armed combatant. It was not possible to exactly replicate the same McQueen targets in this condition, so combatants were selected from those available in the control software (Virtual Battlespace 2, Bohemia Interactive Simulations, Farnborough, United Kingdom), but were closely matched and clearly hostile or non-hostile in nature. These targets made some small movements of the arms and head but remained in a single location until a shot had been fired.
To enable objective performance analyses in these conditions, a GoPro Hero 4 video camera (GoPro Inc, California, United States) was situated behind and slightly to the left of the participant for the duration of these trials. This recording device was positioned in a manner that would detect when the target objects appeared and if/when participants made a shot. The experimenter also viewed the computer screen and manually recorded when shots were taken.
2.4 Procedure
Participants were recruited at RAF Honington during a scheduled training exercise week. Eligible individuals were contacted in advance of this visit and received a detailed study information sheet via email. Those who were interested in taking part then attended an in-person briefing by the research team, which informed individuals of the study aims and procedures, and then provided written informed consent. They then completed self-report questionnaires relating to military experience and ranking. On each of the subsequent 3 days, participants completed one of each of the three experimental conditions, in a pseudo-randomised order based on a Latin squares design. Upon completing their final session, participants were debriefed about the research and thanked for taking part.
2.5 Measures
The following variables were obtained from all conditions:
2.5.1 Decision accuracy
A score of ‘1’ was given on occasions when the participant’s response (i.e., shoot/don’t-shoot action) matched the appropriate target cue, and a score of “0” was given when it did not match. Average scores for each participant were then converted into an accuracy percentage.
2.5.2 False alarms
The number of shots made to non-threatening targets in each condition was recorded, again converted to a percentage.
2.5.3 Shot accuracy
Calculated as a binary hit/miss variable converted to an accuracy percentage.
2.5.4 Response time
The time elapsed between the stimulus being presented (target appearing) and the trigger of the weapon being pulled.
2.5.5 D-prime and beta
Following Blacker et al. (2020), two additional variables derived from Signal Detection Theory (Green and Swets, 1966) were used to supplement the raw decision accuracy and false alarm measurements. Signal Detection Theory describes how the human perceptual system makes an inference about the presence or absence of a “signal,” which here is the nature of the target. Accordingly, we examined whether sensitivity to threat (d-prime) and response tendencies (beta) varied between the different simulated conditions (see Figure 3).
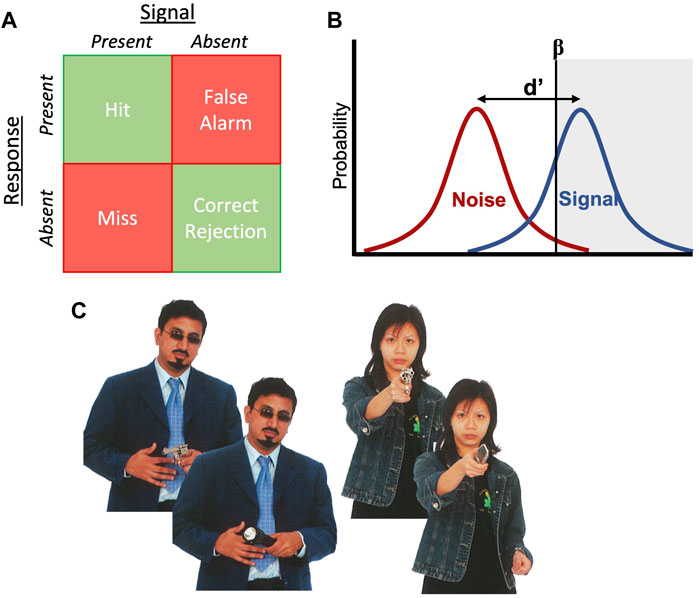
FIGURE 3. Illustration of Signal Detection Theory. The matrix in (A) shows four possible combinations of signals and responses. (B) illustrates the metrics d-prime and beta in relation to distributions of signal (weapon present or absent) and noise. As drawn here, d-prime would be large because the observer was able to perceive a clear difference between the signal and the noise. The beta value is to the right indicating a conservative response strategy. (C) shows examples of the Sykes-McQueen “8,000 range” threat assessment targets used in the synthetic and VR environments. Reproduced with permission.
D-prime provides an index of the person’s sensitivity to a stimulus and is calculated from the ratio of ‘hits’ (i.e., correctly firing when it is right to do so) to “false alarms” (i.e., firing when you shouldn’t). Specifically, d-prime represents the difference between the z-transformed proportions of hits and false alarms [d' = z(H)—z(F), where H=P (“yes” | YES) and F=P (“yes” | NO)]. Higher values indicate greater sensitivity (better ratio of hits to false alarms).
Beta (β) is a measure of response bias which captures how some individuals under-respond and will have only fired when certain (i.e., conservative bias, values below 0) whereas others will have had a bias towards over-responding (i.e., liberal bias, values above 0). Beta is calculated from the ratio of the normal density functions at the criterion of the z-values used in the computation of d-prime.
2.5.6 Presence
Recent research has discussed how a sense of “presence” (i.e., of really existing in a virtual environment) may influence learning and performance (Makransky et al., 2019a; Makransky et al.,2019b). To test whether the VR and 2D video systems were of sufficiently high fidelity to provide an immersive experience, participants were asked to provide a self-report measure of presence, adapted from Pan et al. (2016). The questionnaire (which can be viewed here: https://osf.io/vdk87/) consisted of 10 items which record the degree to which the participant felt as though they really existed in the simulated environment, compared to the physical environment. Questions such as “I had a sense of being there in the training environment” are responded to on a 1-7 Likert scale anchored between “at no time” and “almost all the time”. This questionnaire has previously been validated in relation to experimentally induced breaks in presence (Slater and Steed, 2000), and used in the context of medical assessment scenarios (Pan et al., 2016), aviation simulation (Harris et al., 2022), and social interaction (Pan et al., 2015). The presence questionnaire was used for the VR and 2D video conditions as the questions are not applicable to real-world activities.
2.5.7 Expertise
Demographic information was collected with a questionnaire administered immediately after the consent form. Participants were asked to report years of military experience and rank.
2.6 Data analysis
Data were firstly screened for outliers and extreme deviations from normality (Tabachnick and Fidell, 1996). A series of repeated measures ANOVAs were then used to compare the performance variables (decision-making, signal detection metrics, and shooting accuracy) between the three conditions. Non-parametric alternatives (Friedman’s test) were used when data substantially deviated from normality. Paired t-tests were also used to compare presence between VR and 2D video simulation to test fidelity. A series of bivariate correlations were then used to explore the relationships between the performance measures recorded from each condition as a test of concurrent validity. Finally, correlations between experience and each of the performance variables were calculated to establish whether real-world experience was related to performance in the simulations, i.e., construct validity. The decision-making variables for the 2D video condition were found to be at a ceiling–on only two occasions (out of >300) a threatening target was not shot at, and only one false alarm occurred. Consequently, there was almost no variance in these variables, making them inappropriate for standard statistical analysis, so they are included in figures but omitted from some of the statistical tests. The anonymised data for this study is available from the Open Science Framework (see https://osf.io/vdk87/).
3 Results
3.1 Correlations
To assess construct validity of the three simulations, bivariate correlations were run to examine the strength of the relationship between real-world expertise (years of military service) and the decision-making and shooting accuracy variables. Spearman’s rho was used as many of the data distributions deviated significantly from normality. Years of military experience showed no significant correlations with performance and decision-making variables from VR (ps > .51, rs < 0.11), video simulation (ps > .21, rs < 0.21)3 and live fire (ps > .19, rs < .23) simulations.
As a test of concurrent validity, we also explored the relationships between the three simulation types for decision-making and shooting accuracy variables. No significant correlations for decision accuracy (ps > .26, rs < .21) or “false alarms” (r = −0.02, p = .90)4 were observed. There were also no significant relationships between VR and live fire for d-prime (r = .21, p = .21) or beta (r = .03, p = .86)3. There were no significant correlations between VR and 2D video or VR and live fire for response time (ps > .31, rs < 0.18), but there was a significant relationship between 2D video and live fire (r = .35, p = .04) (see Figures 4A–C). There were also no significant correlations between conditions for shooting accuracy (ps > .30, rs < .17) (see Figures 4D–F).
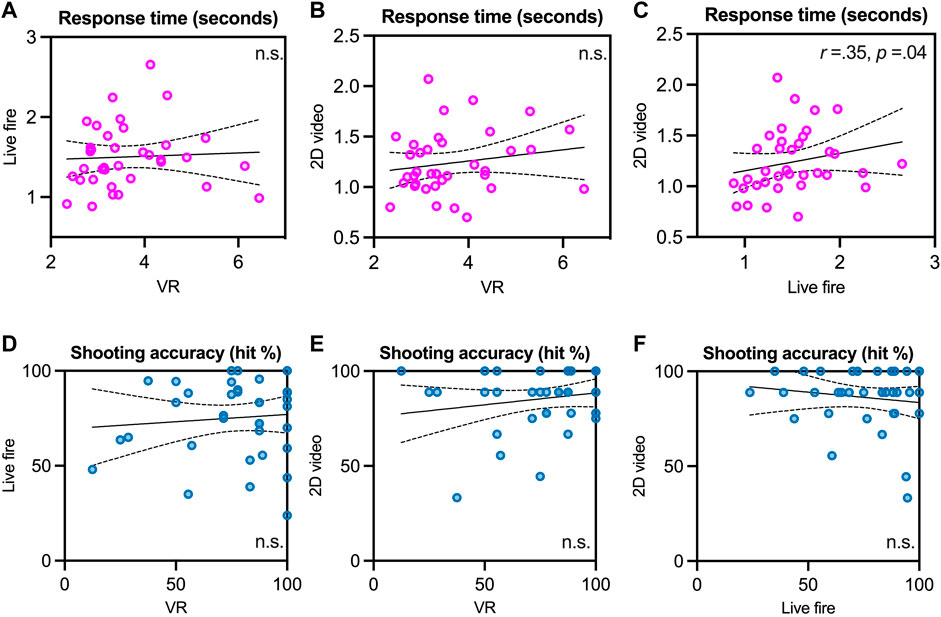
FIGURE 4. Scatter plots of concurrent validity correlations for response time (A–C) and shooting accuracy variables (D–F). The solid line represents the fitted regression line and dotted lines indicate 95% confidence interval of the line (n.s. = non-significant). Note in panels (A–C), different axis limits are used for VR, live fire, and 2D video due to the large differences in response times across the conditions.
3.2 Presence
As a comparison of fidelity, a paired student’s t-test was used to determine whether people reported higher presence in the VR (M = 49.61, SD = 8.72) or 2D video (M = 42.76, SD = 12.21) simulation conditions (see Figure 5). Results indicated that scores were significantly higher in the VR condition, with a large effect size [t (25) = 3.93, p < .001, d = 0.66].
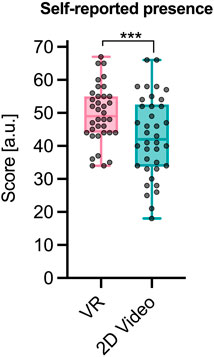
FIGURE 5. Box and whisker plot for presence scores (y-axis represents the full range of possible scores, from 0 to 70). ***p < .001, a.u. = arbitrary units.
3.3 Decision-making
To test whether there were differences in decision-making between the simulation conditions, a series of comparisons were run on decision accuracy and signal detection metrics. A Friedman test (one-way repeated measures analysis of variance by ranks) was used given the substantially non-normal distribution of the data from the 2D video condition. For decision accuracy (see Figure 6A), there were significant between-condition differences [χ2 (2) = 42.45, p < .001, W = 0.59]. Non-parametric post hoc tests (Conover’s Test) with a Bonferroni-Holm correction for multiple comparisons showed that decision accuracy (%) was significantly higher in the 2D video simulation condition (M = 99.54, SD = 1.56) than both the live fire (M = 91.84, SD = 10.63) [t (70) = 3.17, p = .003] and the VR condition (M = 83.04, SD = 9.66) [t (70) = 6.53, p < .001]. Decision accuracy in live fire was also significantly higher than in VR [t (70) = 3.31, p = .003].
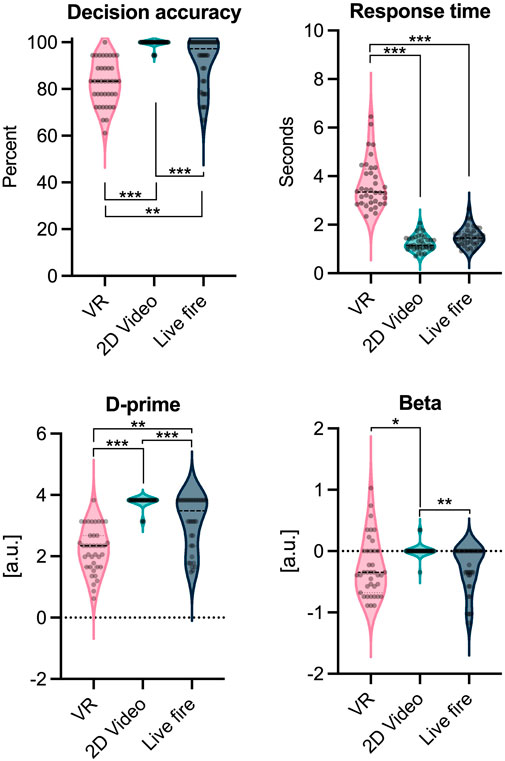
FIGURE 6. Violin plots comparing decision-making variables between the three simulation conditions. *p < .05, **p < .01, ***p < .001, a.u. = arbitrary units.
As there was only one false alarm (i.e., failures to inhibit fire) in the 2D condition (M = 0.03, SD = 0.17) it was omitted from statistical tests. Both the VR (M = 2.03, SD = 1.50) and live fire (M = 1.31, SD = 1.75) conditions therefore clearly had more false alarms. The difference between VR and live fire did not reach statistical significance [F (1,35) = 3.67, p = .06, η2 = 0.10].
For response time (see Figure 6B), repeated-measures ANOVA showed that there were significant between-condition differences [F (1.3,45.1) = 165.61, p < .001, η2 = 0.83]. Post-hoc tests with Bonferroni-Holm correction showed that response times in VR (M = 3.66, SD = 1.00) were significantly longer than both video simulation (M = 1.24, SD = 0.32) [t (35) = 16.59, p < .001, d = 2.44] and live fire (M = 1.50, SD = 0.40) [t (35) = 14.78, p < .001, d = 2.05] conditions. The difference between video simulation and live fire was not significant [t (35) = 1.80, p = .07, d = 0.59]. It therefore appears that in VR, shoot/don’t-shoot decisions took longer and accuracy was lower.
For d-prime (see Figure 6C), a Friedman test showed that there were again significant between-condition differences [χ2 (2) = 41.48, p < .001, W = 0.58]. Non-parametric post hoc tests with Bonferroni-Holm correction showed d-prime was significantly lower in VR (M = 2.22, SD = 0.77) than both 2D video (M = 3.77, SD = 0.19) [t (70) = 6.46, p < .001] and live fire (M = 3.10, SD = 0.88) [t (70) = 3.08, p = .003]. D-prime for 2D video was also significantly higher than in live fire [t (70) = 3.32, p = .002]. This suggests that participants showed lower response sensitivity (i.e., they made fewer ‘hits’ relative to ‘false alarms’) in VR, compared to the live fire and 2D simulations.
For beta (see Figure 6D), there were also significant between-condition differences [χ2 (2) = 12.21, p = .002, W = 0.17]. Non-parametric post hoc tests with Bonferroni-Holm correction showed significant differences between VR (M = −0.22, SD = 0.47) and 2D video (M = 0.01, SD = 0.10) [t (70) = 2.62, p = .02] and between live fire (M = −0.28, SD = 0.36) and 2D video [t (70) = 3.32, p = .004], with both VR and live fire eliciting a more conservative response tendency than 2D video. There was no significant difference between VR and live fire [t (70) = 0.77, p = .44].
3.4 Shooting accuracy
A one-way ANOVA comparing the percentage of targets hit when a shot was fired showed a significant difference between conditions [F (2.0,68.4) = 3.39, p = .04, η2 = 0.09] (see Figure 7). Post-hoc tests with Bonferroni-Holm correction revealed no significant differences between any groups (ps > .06), but the highest scores were observed in 2D video (M = 86.27, SD = 15.83), then VR (M = 76.49, SD = 23.77), then live fire (M = 75.22, SD = 20.23).
4 Discussion
In the present work we explored three types of simulation used for training judgemental skills in the military. Simulation forms an important part of human skills training in many industries and the increasing popularity of commercial head-mounted VR has created new possibilities for the field (Lele, 2013; Bhagat et al., 2016; Siu et al., 2016). Before new methods like VR are adopted, they should be systematically evaluated in comparison to other forms of simulation (e.g., existing and/or alternative training methodologies). We observed important differences in decision-making behaviour between three types of simulation training (VR, 2D video, and live fire), which suggests they did not present equivalent judgemental challenges. Pinpointing the exact reasons for these differences will require further work, but the current results suggest that different forms of simulation may provide fundamentally different learning conditions–posing differing levels of challenge, degrees of immersion, and variations in perceptual information–and should be selected carefully to address the exact training need.
Our first criteria for evaluating the simulation approaches was to test whether the VR and video methods provided a high-fidelity experience that elicited a sense of presence in the user. For both VR and video simulation approaches, scores on the presence questionnaire ranged from medium to high, relative to the limits of the scale and previous usage (Pan et al., 2016), highlighting that most participants felt as though they really existed in the room clearance scenario (see Figure 5). This indicates that there was a good level of fidelity in the simulation environments and that they were sufficiently realistic. The sense of presence in VR was significantly higher than in 2D video, confirming that VR was indeed more immersive. Whether or not presence provides direct benefits for learning is unclear (see Makransky et al., 2019), but it may contribute to more realistic behaviour and task engagement in some VR tasks (Slater and Sanchez-Vives, 2016).
Next, we sought to understand whether decision-making tendencies were similar across the different simulation conditions. While the physics of the weapons, and therefore shooting mechanics, were clearly different between conditions, we wanted to determine whether decision-making tendencies, and therefore participants’ judgemental training responses, were also different. One of the clearest findings was that 2D video provided little challenge for making shoot/don’t-shoot judgements, as participants made very few errors and were almost all at a performance ceiling. Consequently, there is very little room for improvement during training, so the 2D video condition is unlikely to develop users’ judgemental abilities. The reason for the ceiling effect may be the limited variation in the 2D video condition. For example, in live fire and VR the location of combatants varied, and the participant chose how to move around to locate them, whereas in 2D video they appeared consistently in the centre of the screen, so likely posed a reduced perceptual and decision-making challenge. The stationary nature of the 2D task, without any real need for using room clearing tactics, may also have reduced the decision-making challenge. Returning to Lukosch et al. (2019) fidelity framework, and the importance of quality user-environment interactions, the more interactive aspect of movement in VR environment may be where they are particularly superior to video-based approaches (Bermejo-Berros and Gil Martínez, 2021).
Decision-making was much more comparable between VR and live fire, with a similar range of scores across the two conditions. Decision accuracy was statistically better in live fire than VR, but this difference was relatively small. The d-prime and beta metrics derived from Signal Detection Theory (Green and Swets, 1966) also showed similar patterns between VR and live fire. D-prime values (detection sensitivity) were lower in VR than live fire, but beta values (indicating decision-making bias) were not significantly different, indicating that VR did not make people more liberal or conservative in their judgements. The reduced detection sensitivity and longer reaction times in VR, compared to live fire, could indicate that it poses an additional decision-making challenge. While some additional challenge could be beneficial for training, these differences could also be a function of additional cognitive load in VR (Han et al., 2021), greater task complexity, or simply difficulties with discerning the visual details of the environment. As we did not collect additional data on useability or workload we cannot determine the exact origin of these differences. Future work should, therefore, examine which exact features were responsible for these differences, perhaps by comparing different levels of visual detail, as well as different levels of complexity in the task, and closely measuring cognitive load. Despite these differences between VR and the live fire condition, the broad similarity between virtual and real-world conditions does provide some encouragement that VR may offer sufficient levels of psychological fidelity to be effective for training (see Harris et al., 2020).
Correlations between 2D video, VR, and live fire for the decision-making and signal detection metrics did not show strong relationships. This suggests that even though the spread of scores across VR and live fire was similar, individuals who performed well in live fire did not necessarily also do well in VR. While VR may have provided somewhat similar levels of decision-making challenge to live fire, it appears to be of a slightly different nature. Although VR-based training methods can often be limited in some aspects of training fidelity (e.g., users may rely on different movement strategies and perceptual cues: Liu et al., 2009; Harris et al., 2019; Wijeyaratnam et al., 2019), their ability to elicit “lifelike” affective states and cognitive responses could offer unique training advantages (for example, in military context, see: Pallavicini et al., 2016). In short, VR and other forms of simulation may not be equivalent, but they can be useful for training different aspects of skilled performance. For example, live fire is likely to be more effective for developing weapons handling, but complex and stressful VR environments could better prepare trainees for the stresses and distractions of the combat environment. Further studies comparing these different aspects of performance following periods of training on either VR or live fire simulations would be beneficial.
For the shooting performance measures, the differences between conditions were small, with pairwise comparisons of percentage of target hits not statistically different between any of the three groups. Again, however, the correlations between accuracy in the three conditions was weak, suggesting that skills in one simulation did not transfer well into the others. Nevertheless, it is important to note that the primary aim of the simulations was not to train weapons handling, which can be done in other ways. Moreover, our tests of construct validity (i.e., whether performance in the simulations was related to real-world experience) were largely inconclusive in this study, as all correlations between the performance variables and years of military experience were weak. This suggests that either the measure of expertise (years of experience) did not adequately capture real-world expertise sufficiently well, or none of these tests are sensitive to these differences.
Some speculative recommendations from conducting the trial are as follows. In the present context, live fire may be most effective for training the procedural elements of room clearance such as breaching the door and textbook methods for searching the room. This is because it allows the most realistic movements around the room, interaction with a physical door, and the use of a realistic weapon. However, because trainees are simply shooting at static cardboard targets, the judgemental element is quite simplified. In the current VR simulation, the targets were static to closely match the live fire condition, but the addition of dynamically moving and evolving targets (e.g., pulling out a weapon) would likely mean that VR poses the most realistic way to train the perceptual elements of shoot/don’t-shoot decision-making. Previous work has shown that visual search skills may be trainable in VR (Harris et al., 2021) so it could be a good option for this element of judgemental training. For example, during live fire training only one of two individuals were practicing at any one time due to the need for safety controls and trained instructors. Therefore, while a few trainees are doing live fire drills, accompanied by instructors and being given verbal feedback, others could be performing repetitions on the VR practicing the skills they have just learned. Given the potentially differing benefits offered by live fire and VR approaches, future research could look at the effectiveness of combining these training methods to elicit adaptive perceptuomotor effects. The only real benefit of the 2D video simulation over VR was that it did allow the use of a realistic weapon (a replica SA80 with gas cannisters to simulate kickback) so could be useful when other training options are not available.
5 Conclusion
In the present work we compared three methods of simulation in the context of judgemental training in the military. We used an evidence-based approach to conduct preliminary assessments of VR, 2D video, and live fire room clearance simulations. Our results suggest that there can be fundamental differences between different types of simulation of the same task, so those employing simulation methods in training should carefully consider the aims of training and whether the simulation method chosen can achieve the pedagogical goals.
Data availability statement
The datasets presented in this study can be found in online repositories. The names of the repository/repositories and accession number(s) can be found below: https://osf.io/vdk87/.
Ethics statement
The studies involving human participants were reviewed and approved by Ministry of Defence Research Ethics Committee (reference number: 2102/MODREC/21). The patients/participants provided their written informed consent to participate in this study.
Author contributions
All authors contributed to the design of the study and the preparation of the manuscript. DH, TA, and EH conducted data collection and DH performed the data analysis.
Funding
This work was supported by funding from the UK’s Defence Science and Technology Laboratory through SERAPIS MIITTE Lot 5.
Conflict of interest
Author JK is employed by Newman and Spurr Consulting. Author TB is employed by Cineon Training.
The remaining authors declare that the research was conducted in the absence of any commercial or financial relationships that could be construed as a potential conflict of interest.
Publisher’s note
All claims expressed in this article are solely those of the authors and do not necessarily represent those of their affiliated organizations, or those of the publisher, the editors and the reviewers. Any product that may be evaluated in this article, or claim that may be made by its manufacturer, is not guaranteed or endorsed by the publisher.
Footnotes
1We used fidelity to refer to whether a simulation recreates the real-world system, in terms of its appearance but also the affective states, cognitions or behaviours it elicits from its users. Validity refers more to whether the simulation provides an accurate representation of the target task, within the context of the learning objectives and the target population (Perfect et al., 2014; Gray, 2019; Harris et al., 2020).
2These training rounds resemble regular rounds and are fired through a service weapon but discharge a small paint charge at a reduced velocity.
3D-prime, beta, and decision accuracy excluded for 2D video.
4Correlations for 2D video were not possible due to the absence of variation in this measure.
References
Alrashidi, M., Wadey, C. A., Tomlinson, R. J., Buckingham, G., and Williams, C. A. (2022). The efficacy of virtual reality interventions compared with conventional physiotherapy in improving the upper limb motor function of children with cerebral palsy: A systematic review of randomised controlled trials. Disabil. Rehabilitation 0, 1–11. doi:10.1080/09638288.2022.2071484
Armstrong, J., Clare, J., and Plecas, D. (2014). Monitoring the impact of scenario-based use-of-force simulations on police heart rate: Evaluating the royal Canadian mounted police skills refresher program. Criminol. Crim. Just. L Soc’y 15, 51.
Bermejo-Berros, J., and Gil Martínez, M. A. (2021). The relationships between the exploration of virtual space, its presence and entertainment in virtual reality, 360o and 2D. Virtual Real. 25, 1043–1059. doi:10.1007/s10055-021-00510-9
Bhagat, K. K., Liou, W.-K., and Chang, C.-Y. (2016). A cost-effective interactive 3D virtual reality system applied to military live firing training. Virtual Real. 20, 127–140. doi:10.1007/s10055-016-0284-x
Biggs, A. T., Pettijohn, K. A., and Gardony, A. L. (2021). When the response does not match the threat: The relationship between threat assessment and behavioural response in ambiguous lethal force decision-making. Q. J. Exp. Psychol. 74, 812–825. doi:10.1177/1747021820985819
Blacker, K. J., Pettijohn, K. A., Roush, G., and Biggs, A. T. (2020). Measuring lethal force performance in the lab: The effects of simulator realism and participant experience. Hum. Factors 63, 1141–1155. doi:10.1177/0018720820916975
Bright, E., Vine, S., Wilson, M. R., Masters, R. S. W., and McGrath, J. S. (2012). Face validity, construct validity and training benefits of a virtual reality turp simulator. Int. J. Surg. 10, 163–166. doi:10.1016/j.ijsu.2012.02.012
Gray, R. (2019). “Virtual environments and their role in developing perceptual-cognitive skills in sports,” in Anticipation and decision making in sport. Editors A. M. Williams,, and R. C. Jackson (Routledge: Taylor & Francis). doi:10.4324/9781315146270-19
Han, J., Zheng, Q., and Ding, Y. (2021). Lost in virtual reality? Cognitive load in high immersive VR environments. J. Adv. Inf. Technol. 12. doi:10.12720/jait.12.4.302-310
Harris, D., Arthur, T., Burgh, T. de, Duxbury, M., Lockett-Kirk, R., McBarnett, W., et al. (2022). Assessing expertise using eye tracking in a Virtual Reality flight simulation. PsyArXiv. doi:10.31234/osf.io/ue58a
Harris, D. J., Bird, J. M., Smart, A. P., Wilson, M. R., and Vine, S. J. (2020a). A framework for the testing and validation of simulated environments in experimentation and training. Front. Psychol. 11, 605. doi:10.3389/fpsyg.2020.00605
Harris, D. J., Buckingham, G., Wilson, M. R., Brookes, J., Mushtaq, F., Mon-Williams, M., et al. (2020b). The effect of a virtual reality environment on gaze behaviour and motor skill learning. Psychol. Sport Exerc. 50, 101721. doi:10.1016/j.psychsport.2020.101721
Harris, D. J., Buckingham, G., Wilson, M. R., and Vine, S. J. (2019). Virtually the same? How impaired sensory information in virtual reality may disrupt vision for action. Exp. Brain Res. 237, 2761–2766. doi:10.1007/s00221-019-05642-8
Harris, D. J., Hardcastle, K. J., Wilson, M. R., and Vine, S. J. (2021). Assessing the learning and transfer of gaze behaviours in immersive virtual reality. Virtual Real. 25, 961–973. doi:10.1007/s10055-021-00501-w
Lele, A. (2013). Virtual reality and its military utility. J. Ambient. Intell. Hum. Comput. 4, 17–26. doi:10.1007/s12652-011-0052-4
Li, W.-C., and Harris, D. (2008). The evaluation of the effect of a short aeronautical decision-making training program for military pilots. Int. J. Aviat. Psychol. 18, 135–152. doi:10.1080/10508410801926715
Liu, L., van Liere, R., Nieuwenhuizen, C., and Martens, J.-B. (2009). “Comparing aimed movements in the real world and in virtual reality,” in 2009 IEEE Virtual Reality Conference, Lafayette, Louisiana, USA, 14-18 March, 219–222. doi:10.1109/VR.2009.4811026
Lukosch, H., Lukosch, S., Hoermann, S., and Lindeman, R. W. (2019). “Conceptualizing fidelity for HCI in applied gaming,” in HCI in games lecture notes in computer science. Editor X. Fang (Cham: Springer International Publishing), 165–179. doi:10.1007/978-3-030-22602-2_14
Makransky, G., Borre-Gude, S., and Mayer, R. E. (2019a). Motivational and cognitive benefits of training in immersive virtual reality based on multiple assessments. J. Comput. Assisted Learn. 35, 691–707. doi:10.1111/jcal.12375
Makransky, G., Terkildsen, T. S., and Mayer, R. E. (2019b). Adding immersive virtual reality to a science lab simulation causes more presence but less learning. Learn. Instr. 60, 225–236. doi:10.1016/j.learninstruc.2017.12.007
Nieuwenhuys, A., Savelsbergh, G. J. P., and Oudejans, R. R. D. (2015). Persistence of threat-induced errors in police officers’ shooting decisions. Appl. Ergon. 48, 263–272. doi:10.1016/j.apergo.2014.12.006
Pallavicini, F., Argenton, L., Toniazzi, N., Aceti, L., and Mantovani, F. (2016). Virtual reality applications for stress management training in the military. Aerosp. Med. Hum. Perform. 87, 1021–1030. doi:10.3357/AMHP.4596.2016
Pan, X., Gillies, M., and Slater, M. (2015). Virtual character personality influences participant attitudes and behavior – an interview with a virtual human character about her social anxiety. Front. Robotics AI 2, 1. doi:10.3389/frobt.2015.00001
Pan, X., Slater, M., Beacco, A., Navarro, X., Rivas, A. I. B., Swapp, D., et al. (2016). The responses of medical general practitioners to unreasonable patient demand for antibiotics - a study of medical ethics using immersive virtual reality. PLOS ONE 11, e0146837. doi:10.1371/journal.pone.0146837
Perfect, P., Timson, E., White, M. D., Padfield, G. D., Erdos, R., and Gubbels, A. W. (2014). A rating scale for the subjective assessment of simulation fidelity. Aeronaut. J. 118, 953–974. doi:10.1017/S0001924000009635
Popov, O., Iatsyshyn, A., Sokolov, D., Dement, M., Neklonskyi, I., and Yelizarov, A. (2021). “Application of virtual and augmented reality at nuclear power plants,” in Systems, Decision and Control in energy II studies in systems, decision and control. Editors A. Zaporozhets,, and V. Artemchuk (Cham: Springer International Publishing), 243–260. doi:10.1007/978-3-030-69189-9_14
Randel, J. M., Pugh, H. L., and Reed, S. K. (1996). Differences in expert and novice situation awareness in naturalistic decision making. Int. J. Human-Computer Stud. 45, 579–597. doi:10.1006/ijhc.1996.0068
Salas, E., Bowers, C. A., and Rhodenizer, L. (1998). It is not how much you have but how you use it: Toward a rational use of simulation to support aviation training. Int. J. Aviat. Psychol. 8, 197–208. doi:10.1207/s15327108ijap0803_2
Siu, K.-C., Best, B. J., Kim, J. W., Oleynikov, D., and Ritter, F. E. (2016). Adaptive virtual reality training to optimize military medical skills acquisition and retention. Mil. Med. 181, 214–220. doi:10.7205/MILMED-D-15-00164
Slater, M., and Sanchez-Vives, M. V. (2016). Enhancing our lives with immersive virtual reality. Front. Robot. AI 3. doi:10.3389/frobt.2016.00074
Slater, M., and Steed, A. (2000). A virtual presence counter. Presence 9, 413–434. doi:10.1162/105474600566925
Staller, M., and Zaiser, B. (2015). Developing problem solvers: New perspectives on pedagogical practices in police use of force training. J. Law Enforc. 4, 1.
Stoffregen, T. A., Bardy, B. G., Smart, L. J., and Pagulayan, R. (2003). “On the nature and evaluation of fidelity in virtual environments,” in Virtual and adaptive environments: Applications, implications and human performance issues. Editors L. J. Hettinger,, and M. W. Haas (Mahwah, NJ: Lawrence Erlbaum Associates), 111–128.
Tabachnick, B. G., and Fidell, L. S. (1996). Using multivariate statistics. Northridge. Cal.: Harper Collins.
Valori, I., McKenna-Plumley, P. E., Bayramova, R., Callegher, C. Z., Altoè, G., and Farroni, T. (2020). Proprioceptive accuracy in immersive virtual reality: A developmental perspective. PLOS ONE 15, e0222253. doi:10.1371/journal.pone.0222253
van Dongen, K. W., Tournoij, E., van der Zee, D. C., Schijven, M. P., and Broeders, I. A. M. J. (2007). Construct validity of the LapSim: Can the LapSim virtual reality simulator distinguish between novices and experts? Surg. Endosc. 21, 1413–1417. doi:10.1007/s00464-006-9188-2
Wijeyaratnam, D. O., Chua, R., and Cressman, E. K. (2019). Going offline: Differences in the contributions of movement control processes when reaching in a typical versus novel environment. Exp. Brain Res. 237, 1431–1444. doi:10.1007/s00221-019-05515-0
Wong, K., Bhama, P. K., d’Amour Mazimpaka, J., Dusabimana, R., Lee, L. N., and Shaye, D. A. (2018). Banana fruit: An “appealing” alternative for practicing suture techniques in resource-limited settings. Am. J. Otolaryngology 39, 582–584. doi:10.1016/j.amjoto.2018.06.021
Wood, G., Wright, D. J., Harris, D., Pal, A., Franklin, Z. C., and Vine, S. J. (2020). Testing the construct validity of a soccer-specific virtual reality simulator using novice, academy, and professional soccer players. Virtual Real. 25, 43–51. doi:10.1007/s10055-020-00441-x
Keywords: VR, shoot/don’t-shoot, decision making, skill acquisition, cognition
Citation: Harris DJ, Arthur T, Kearse J, Olonilua M, Hassan EK, De Burgh TC, Wilson MR and Vine SJ (2023) Exploring the role of virtual reality in military decision training. Front. Virtual Real. 4:1165030. doi: 10.3389/frvir.2023.1165030
Received: 13 February 2023; Accepted: 13 March 2023;
Published: 27 March 2023.
Edited by:
Doug A. Bowman, Virginia Tech, United StatesReviewed by:
Aaron L. Gardony, U. S. Army Combat Capabilities Development Command Soldier Center, United StatesKelly S. Hale, Draper Laboratory, United States
Copyright © 2023 Harris, Arthur, Kearse, Olonilua, Hassan, De Burgh, Wilson and Vine. This is an open-access article distributed under the terms of the Creative Commons Attribution License (CC BY). The use, distribution or reproduction in other forums is permitted, provided the original author(s) and the copyright owner(s) are credited and that the original publication in this journal is cited, in accordance with accepted academic practice. No use, distribution or reproduction is permitted which does not comply with these terms.
*Correspondence: D. J. Harris, ZC5qLmhhcnJpc0BleGV0ZXIuYWMudWs=