- 1Normandie Univ, UNICAEN, ENSICAEN, CNRS, GREYC, Caen, France
- 2Univ Rouen Normandie, CETAPS, UR 3832, Rouen, France
- 3Open Mind Neurotechnologies, Paris, France
- 4Normandie Univ, UNICAEN, INSERM, COMETE, Caen, France
Objective: The aim of the present study was to evaluate the impact of different task constraints on the participants’ adaptation when performing a 3D visuomotor tracking task in a virtual environment.
Methods: Twenty-three voluntary participants were tested with the HTC Vive Pro Eye VR headset in a task that consisted of tracking a virtual target moving in a cube with an effector controlled with the preferred hand. Participants had to perform 120 trials according to three task constraints (i.e., gain, size, and speed), each performed according to four randomized conditions. The target-effector distance and elbow range of movement were measured.
Results: The results showed an increase in the distance to the target when the task constraints were the strongest. In addition, a change in movement kinematics was observed, involving an increase in elbow amplitude as task constraints increased. It also appeared that the depth dimension played a major role in task difficulty and elbow amplitude and coupling in the tracking task.
Conclusion: This research is an essential step towards characterizing interactions with a 3D virtual environment and showing how virtual constraints can facilitate arm’s involvement in the depth dimension.
1 Introduction
In recent years, many studies have used immersive virtual reality (VR) to analyse perceptual-motor coordination as closely as possible to the real environment (Bideau et al., 2010; Bideau et al., 2004; Ranganathan and Carlton, 2007; Vignais et al., 2009; Faure et al., 2020). This strong interest in VR creates the possibility for accurately controlling the information provided by the device, the interactions with the environment and the constraints of the task (e.g., Vignais et al., 2009; Choi et al., 2021). In this context, there is a need for research that characterises the motor actions that are possible in the virtual environment, specifically in the depth dimension (Armbrüster et al., 2008; Vienne et al., 2020). To address this end, our research team has developed a visuomotor tracking task to manipulate and evaluate the impact of different virtual constraints on action involvement and particularly in the depth dimension. The principle of a tracking task is to move an effector with the aim of keeping it as close as possible to a moving target, either by interacting with the display screen (with the hand, for example,) or by using an external device to move an effector in the virtual environment (see Adams, 1961; Poulton, 1957 for first use of this task; Yang and Ferris, 2020). Such a task requires continuous perceptual-motor regulation. This regulation involves prospective control of action based on the exploitation of control laws (defined as the mathematical equation for the circular relation that links information to a motion parameter), consisting in minimizing the gap between current and required behaviour (Gibson, 1979; Warren, 2006; Pacheco et al., 2019). In addition, Limanowski et al. (2017) have used a similar task (i.e., continuous visuo-manual pursuit task) and showed, by varying the feedback delay, that specific brain areas were involved in order to compensate and maintain tracking accuracy, thus implicating specific neural processes.
It therefore appears that visually and dynamically guided tracking movements can be of great interest in analysis of the perceptual-motor process as they involve continuous arm mobilization and induce visuomotor regulation (Pacheco et al., 2019). By varying the speed of the target, some early studies demonstrated that participants were able to accurately track the movement of the target under various speed conditions (Fine et al., 2014). Ao et al. (2015) also observed the effects of different target speeds in stroke patients and showed a shift in sensorimotor control from feedback to feedforward control as target speed increased. Furthermore, the same authors observed a reduction in target-tracking accuracy as speed increased, suggesting an increase in neuromotor noise in the sensorimotor system (Ao et al., 2015). Similarly, (Beppu et al., 1984; Beppu et al., 1987) analysed motor control in ataxic patients (this disease disrupts smooth continuous movement, inducing saccadic patterns) using a visuomotor tracking movement involving elbow flexion. They were able to extract some parameters for assessing the severity of cerebellar ataxia. Three parameters were found to be helpful for assessing this disease: the initial error (defined as target position at the onset of hand movement, for the first approximation of such a predictive error), the initial peak velocity (as these patients have difficulty adapting the amplitude of initial peak velocity to the target velocity), and the ratio of the movement arrest period, to estimate the degree of undulation quantitatively.
Furthermore, it should be noted that visuomotor tracking tasks have mainly been developed in 2D environments (e.g., Buekers et al., 2000; Mallek et al., 2017). The addition of the depth component in visuomotor tracking tasks is of a great interest for testing the effect of this dimension in real and virtual interactions. Interaction with a 3D space is a key component for examining reaching, grasping and interaction tasks (Bingham, 2005; Naceri et al., 2011; Zhu, 2020). To perceive depth, humans rely on two main sources of information: binocular and monocular, which combine directional and depth components. Of these two sources of information, there are many cues, such as occlusion, height in the visual field, relative size, relative density, aerial perspective, binocular disparities, accommodation, convergence, and motion perspective (Cutting, 1997; Cutting, 2021). In fact, the notion of dimension (i.e., degrees of freedom) is important and authors have analysed sensorimotor control as a function of trajectory dimension (1D, 2D, 3D) in arm tracking (Fan et al., 2019). They have shown a deterioration in sensorimotor control (e.g., accuracy, fluidity) as the dimension, and therefore the length of the trajectory increases, no doubt due to the addition of this depth dimension.
Previous studies have analysed depth perception in a three-dimensional virtual environment showing differences in reaching performance depending on the quality of the environment, as well as the available depth cues (Naceri et al., 2011; Gerig et al., 2018; Vienne et al., 2020). Gielen et al. (2009) studied the coordination of eye and hand movements in 3D by recording binocular eye movements while participants moved their finger along a curved trajectory. These authors showed shifts between gaze and finger movements with a greater lead time for gaze in the depth direction. They suggested that these timing differences in the depth dimension were consistent with the idea that the control of vergence and saccades is mediated by distinct neural structures responding to different dynamics (Gielen et al., 2009). More recently, Gerig et al. (2018) studied the effects of depth cues on three-dimensional reaching movements. They showed that the use of a head-mounted display with the addition of stereopsis and motion parallax resulting from head movements, as well as aerial and linear perspectives, occlusion, shadows, and texture gradients, enabled participants to perform better in a reaching task.
More recently, some authors have used 3D virtual environments to examine the control characteristics of dominant and non-dominant hands during a circular tracking task with varying target speeds in healthy adults (Choi et al., 2018; Choi et al., 2021; Jo et al., 2020; Park et al., 2020). Their results showed a decrease in initial peak velocity in both hands as target speed increased. Moreover, the peak of velocity was reached earlier for the dominant hand than the non-dominant hand, regardless of target speed. The results concerning velocity-control performances suggest that the tracking skills of both hands were broadly the same, indicating that the task required essential processes related to interactions with the environment.
Very few studies have focused on the effects of gain, which is defined as the transformation of real movement in virtual movement according to scales that characterize each environment. Some authors have manipulated gain transformation (translational, rotational or curvature), and examined its effect on locomotion in healthy participants (Xie et al., 2010; Zhang et al., 2014; Nguyen et al., 2017) or the accuracy of reaching and its impact on manipulation of an object (Wilson et al., 2018). In the latter study, the participant had to walk to a table to pick up a highlighted cube, and then to walk to another table to place the cube in a designated position. Five levels of translational gain were tested [i.e., 1.0x (1-to-1 movement), 1.5x, 1.75x, 2.0x and 3.0x]. The results showed a degradation in cube handling accuracy for the highest translational gain level. An increase in motion sickness was also reported in this condition. This study suggests that increasing the gain beyond a certain threshold can have negative effects on the accuracy and comfort of interactions within virtual environments.
In sum, even if the role of the constraints has been investigated in previous studies, there is lack of data on specific constraints of great importance such as the space of interaction, the gain in the transformation of real movement into virtual movement and the speed of movement. Therefore, the overall aim of this study was to provide knowledge about the effects of constraints in a well-defined 3D virtual reality interaction task. This knowledge would be valuable for the design of virtual reality devices used in several areas, such as neuromotor rehabilitation of patients suffering from visuomotor coordination disorders.
As a result, the main research question was the following: What is the impact of the various task constraints on target tracking in a 3D virtual environment?
In the current study, participants had to perform a visuomotor tracking task a large number of trials, involving a continuous interaction with the virtual environment in the pursuit of a 3D moving target. We chose this task to study participants’ adaptation to 3D environments to different constraints in terms of precision and movement coupling. Based on previous research and the potential need for future experimental application design, we decided to manipulate the speed of the target, the size of the interaction space, and the gain of movement.
Several hypotheses were then put forward:
• (H1) a limited amount of practice should enable participants to adapt their responses to track the target with accuracy (Mallek et al., 2017);
• (H2) manipulating each of these three constraints should increase the difficulty level of the task (Wilson et al., 2018; Jo et al., 2020; Choi et al., 2021);
• (H3) the depth dimension is expected to be the main source of difficulty in the tracking task (Naceri et al., 2011; Gerig et al., 2018);
• (H4) target displacement in the depth dimension should specifically involves a coupling of the elbow with corresponding flexion/extension (Gielen et al., 2009; Gerig et al., 2018).
2 Materials and methods
2.1 Participants
Twenty-three voluntary participants (12 males and 11 females) with ages ranging from 19 to 60 years (32.8 ± 11.7 years) were recruited for this study. All participants were novice VR players. None of the participants had a history of physical disability or balance disorders. After an explanation of the purpose of the study, all participants gave written informed consent to participate in the study which is in accordance with the Declaration of Helsinki.
2.2 Task and experimental conditions
2.2.1 Apparatus
Participants were seated on a chair, the upper body against the back of the chair to avoid any movement and were equipped with the HTC Vive Pro Eye VR headset (HTC Corporation Inc., Xindian, New Taipei, Taiwan, with technology by Valve; screen: Dual AMOLED 3.6″ diagonal; field of view: 110°; resolution: 1440 * 1600 pixels per eye—2880 * 1600 pixels combined; refresh rate: 90 Hz; eye relief: interpupillary distance and lens distance adjustment for each participant), with two HTC Vive trackers positioned on the elbow and shoulder and an HTC Vive controller placed inside the preferred hand (Figure 1). The virtual environment and the task were developed with the Unity game engine (Unity Technologies, San Francisco, United States). In this virtual environment, the front of the cube whatever its size was located in front of the participant, at a distance of 1.75 m, and the center of the cube at a height of 1.60 m (Figure 1). The coordinate system in real space was a world-fixed coordinate system, where x, y, and z-axes corresponded to the horizontal, vertical and depth axes (towards the front of the participant), respectively. This coordinate system was transposed into virtual space at the center of the cube.
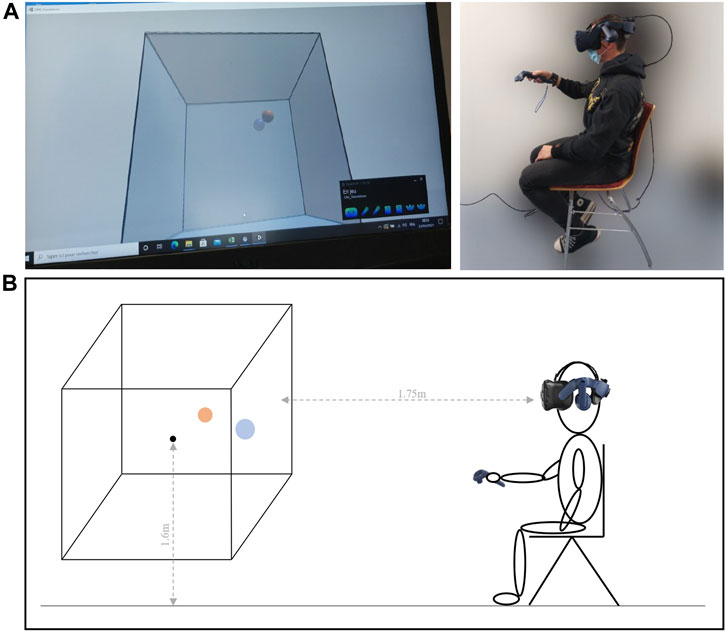
FIGURE 1. Pictures of experimentation set-up (A) and graphic depiction of this set-up in profile (B). Participants wore an HTC Vive Pro Eye VR headset and had to move the controller (right) to move an effector in the virtual environment to track the target (left).
2.2.2 Task
The instructions given to participants were to track a virtual target moving inside a virtual cube by using a controller with their preferred hand (22 participants used the right hand and 1 the left hand). Both target and effector were translucent spheres measuring 20 cm in diameter, which could interpenetrate. The starting position of the target was randomized within the cube. To start the trial, the participants had to reach the stationary target with the effector. At that moment, the target started, between 0 and 2 s, in a random direction with the corresponding velocity. The target could move in any direction with a linear displacement and at constant speed. The target bounced on the cube walls without any deformation or modification of the speed and with symmetry between the incident and reflected angles. Target trajectories were also randomized for each trial and each participant. Each trial lasted 10 s. Following the study by Gerig et al. (2018), the sources of information for the depth dimension were stereopsis (a different image for each eye), shadows on the cube surfaces, parallax, the similar size of the target and the effector, and when the objects overlapped.
2.2.3 Experimental design
Participants had to perform 120 trials according to three task constraints (i.e., gain, size, and speed), each of them including four randomized conditions, as presented below, and each condition being performed ten times (i.e., 3*4*10). The values for each condition were selected after pre-testing to avoid floor or ceiling effects. This experimental design was applied to counterbalance the order effect and therefore neutralize the training effect.
The Gain variable corresponded to the value that transformed the real movement produced by the participants into the movement of the effector in the virtual environment. Four transformation gains were used: 4.583, 6.111, 9.167 and 18.333. For each trial, the speed of the target and the size of the cube were 1.7 m/s and 20.8 m3 (side 2.75 m), respectively.
The Size variable corresponded to the volume of the cube delimiting the space of interaction with the target. Four sizes were used: 1.33 m3 (side 1.1 m), 10.65 m3 (side 2.2 m), 35.94 m3 (side 3.3 m) and 85.18 m3 (side 4.4 m). For each trial, the speed of the target and the gain were 1.7 m/s and 7.333, respectively.
The Speed variable corresponded to the speed of the target. Four speeds were used: 1.4 m/s, 1.6 m/s, 1.8 m/s and 2 m/s. For each trial, the size of the cube and the gain were 20.8 m3 (side 2.75 m) and 7.333, respectively.
After each trial, the mean distance between the effector and the target (centre-to-centre) and the percentage of time during which the effector was in contact with the target were displayed on the screen.
A check was made, for each condition, to ensure that no participant, due to their size, encountered any difficulty in covering the entire interaction space without moving their body. Moreover, each participant’s arm movement was calibrated for each condition so that the effector was located in the center of the cube.
2.2.4 Data processing
The positions of the markers (shoulder, elbow and remote) were recorded by the HTC Vive system and allowed us to calculate, through the 3D coordinates, the angle of the elbow for each participant. This angle was calculated using the following equation:
With x, y and z corresponding to the horizontal, vertical and depth axes, respectively; and a, b and c corresponding to the elbow tracker, hand controller and shoulder tracker, respectively.
The Vive’s tracking technology uses two laser emitters allowing to calculate the position and orientation of the headset and trackers [see Niehorster et al. (2017), for more details and validation of the system for experimental procedures].
To answer our hypotheses, we examined several variables:
• The percentage of target-effector contact (%), corresponded to the percentage of time during which the effector was in contact with the target (i.e., the distance between the centers of the target and the effector was less than the sum of their two radii) (H1).
• The absolute target-effector distance (in m) was calculated with the following formula (
• The target-effector distance (in m) was calculated separately on the x, y and z dimensions (horizontal, vertical, and depth) (H3).
• The maximal elbow range of movement (in °) corresponded to the difference between the maximal and minimal angles recorded during a trial (H4). These angles were calculated from the two markers placed on the shoulder and elbow, plus the controller.
• Regression analyses (R2) were performed between the position of the target in each dimension (x, y, z) and the angle of the elbow to determine with which dimension of the target motion the elbow movements were most coupled (H4).
2.3 Statistical analyses
Statistical analyses were performed using Statistica® 14.1.0. software (TIBCO Software Inc., Palo Alto, California, United States).
In a first stage, in order to evaluate participants’ adaptation to the task, ANOVAs were performed on the 12 blocks of 10 trials without considering the independent variables of gain, size and speed, which were presented in a randomized order. An ANOVA was conducted on the variables absolute target-effector distance and maximal elbow range of movement, with two-way repeated measures 12(Block) x 10 (Trial). Another ANOVA was performed on the variables target-effector distance for each dimension, x, y, z and the R2 from regression analyses between the position of the target in each dimension (x, y, z) and the maximal elbow range of movement, with three-way repeated measures: 3 (Dimension) x 12(Block) x 10 (Trial). This last analysis was performed to determine which dimension of the target’s movement mobilized the most the elbow. Moreover, a descriptive analysis was performed on the percentage of target-effector contact variable to evaluate the adaptation to the task.
In a second stage, an ANOVA was performed separately for each independent variable of gain, size and speed for the variables absolute target-effector distance and maximal elbow range of movement with one-way repeated measures corresponding to the 4 conditions for each variable. The trials were not considered as a variable after the first stage of analysis. An ANOVA was performed on the R2 calculated from the regression analyses between the position of the target in the three dimensions of space and the angle of the elbow with two-way repeated measures: 3 (Dimension) x 4(Condition).
For each analysis, the statistical threshold was established at p = 0.05. When the Mauchly test for sphericity was significant, the Greenhouse-Geisser correction was applied when the epsilon was lower than 0.75, otherwise the Hyun-Feld procedure was used. When a significant main effect or interaction was found, the Bonferroni method was used for post hoc comparisons.
3 Results
3.1 First stage: adaptation to the task
3.1.1 Percentage of target-effector contact
The percentage of target-effector contact (mean) showed that participants started with a target-effector contact score of about 30% and reached about 45% by the end of the 120 trials (Figure 2).
3.1.2 Absolute target-effector distance
The evolution pattern of the target-effector distance (absolute and in the x, y and z dimensions) is presented in Figure 3.
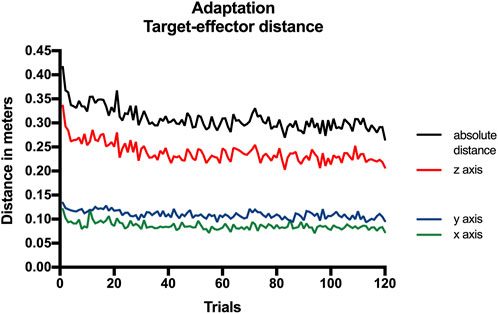
FIGURE 3. Depiction of adaptation to the task for the target-effector distance (absolute and in the x, y and z dimensions).
The 12(Block) x 10 (Trial) ANOVA performed on the absolute target-effector distance showed a significant main effect of Block, F (11,242) = 6.22, p < .001 (ƞ2 = 0.22), of Trial, F (9,198) = 7.44, p < .001 (ƞ2 = 0.25) and a significant Block * Trial interaction effect, F (99,2178) = 1.68, p < .001 (ƞ2 = 0,07; Figure 4).
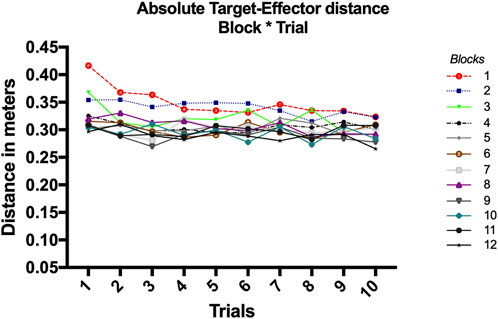
FIGURE 4. Depiction of interaction effects between Block * Trial for the absolute target-effector distance.
The post hoc method on the Block * Trial interaction effect indicated an absolute target-effector distance significantly higher for trials 1, 2 and 3 of block 1, trials 1 and 2 of block 2 and trial 1 of block 3, compared to the other trials of blocks (Figure 4).
3.1.3 Target-effector distance for each dimension (x, y, z)
The 3 (Dimension) x 12 (Block) x 10 (Trial) ANOVA performed on the target-effector distance revealed a significant main effect of the dimension (x, y, z), F (2,44) = 779.13, p < .001 (ƞ2 = 0.97) and two interaction effects: Dimension * Block, F (22,484) = 4.98, p < .001 (ƞ2 = 0.19) and Dimension * Block * Trial, F (198,4356) = 1.28, p < .05 (ƞ2 = 0,06; Figure 5).
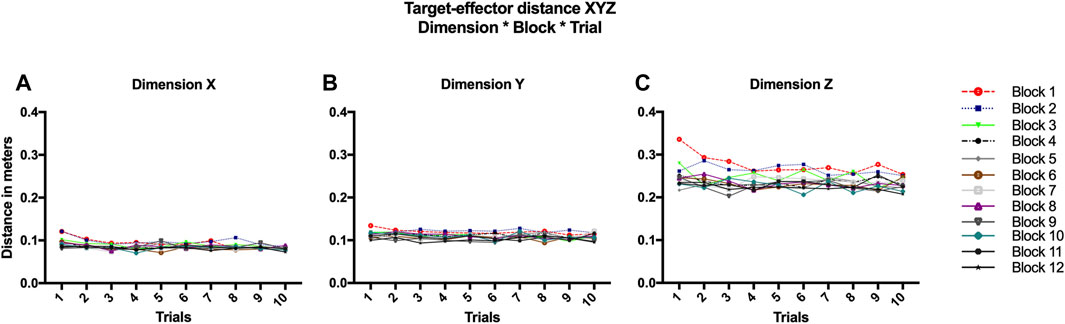
FIGURE 5. Depiction of interaction effect between Dimension * Block * Trial for the target-effector distance in x (A), y (B) and z (C) dimensions.
The post hoc tests performed on the Dimension * Block * Trial interaction effect showed significantly higher target-effector distance for all trials and all blocks in the z dimension (Figure 5).
3.1.4 Maximal elbow range of movement
The 12 (Block) x 10 (Trial) ANOVA performed on the maximal elbow range of movement revealed no significant effect and no interaction effect between blocks and trials.
3.1.5 Regression analyses between the position of the target in each dimension (x, y, z) and the maximal elbow range of movement
The 3 (Dimension) x 12(Block) x 10 (Trial) ANOVA on the R2 obtained from the regression analyses showed a significant main effect of Dimension F (2,44) = 706.51, p < .001 (ƞ2 = 0.97) and Block F (11,242) = 2.74, p < .05 (ƞ2 = 0.11). This analysis also showed four interaction effects: Dimension * Block, F (22,484) = 2.09, p < .05 (ƞ2 = 0.09); Dimension * Trial, F (18,396) = 2.15, p < .05 (ƞ2 = 0.09); Block * Trial, F (99,2178) = 2.13, p < .05 (ƞ2 = 0.09) and Dimension * Block * Trial, F (198,4356) = 2.38, p < .05 (ƞ2 = 0,10; Figure 6).
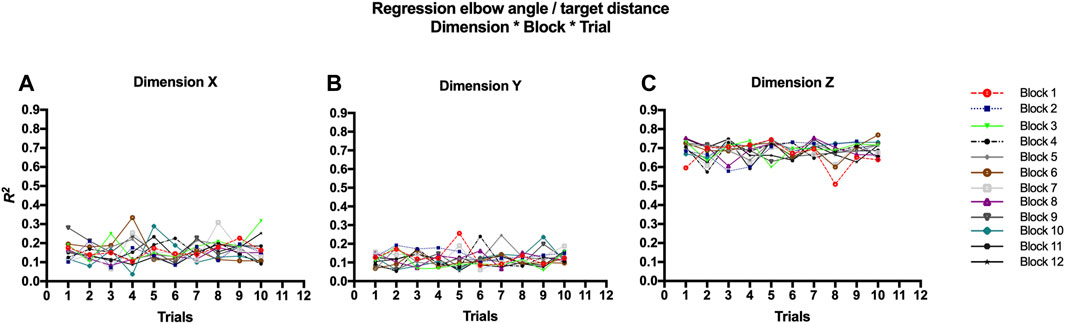
FIGURE 6. Depictions of interaction effect Dimension * Block * Trial for regression between elbow angle and target distance in the x (A), y (B) and z (C) dimensions.
The Bonferroni post hoc method performed on interaction effects indicated significant differences between blocks and between trials only in the z dimension. However, it is difficult to clearly distinguish the origin of these interactions (Figure 6). The main result remains the greater R2 correlation in the z dimension.
3.2 Second stage: effects of the variables gain, size of the cube, speed of the target
As exploratory analyses did not reveal significant effects of Trials at this stage, this factor was not involved in the current analyses. Moreover, the three dimensions x, y, z did not provide more information in relation to the variables Gain, Size of the cube, and Speed of the target than the absolute target-effector distance. In addition, the issue of the depth was addressed in previous analyses. Consequently, it was also decided to not include these three dimensions of target-effector distance in this second stage. Nevertheless, the regression analyses between the position of the target in each dimension (x, y, z) and the maximal elbow range of movement were retained, to provide information about the effect of the variables on the strength of the coupling between the elbow and the three dimensions in the displacement of the target as a function of the conditions of Gain, Size and Speed.
3.2.1 Absolute target-effector distance
3.2.1.1 The effect of gain
The 4 (Condition) ANOVA performed on the absolute target-effector distance revealed a significant main effect of the Gain Condition F (3,66) = 62.93, p < .001 (ƞ2 = 0.74) (Figure 7).
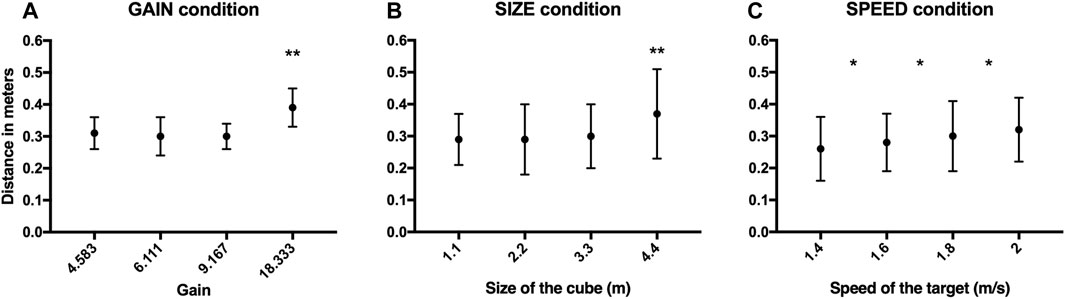
FIGURE 7. Depictions of target-effector distance (means ± standard deviation) for each variable and condition: gain (A), size (B) and speed (C). *significant differences between all variables, **significant differences of one variable on the others.
The results of the post hoc tests for the main effect gain condition indicated significantly higher absolute target-effector distance in the higher condition of gain compared to the other three (Figure 7).
3.2.1.2 The effect of the size of the cube
The 4(Condition) ANOVA revealed a significant main effect of the size of the cube, F (3,66) = 51.55, p < .001 (ƞ2 = 0.70) (Figure 7). The post hoc tests indicated a higher absolute target-effector distance for the larger cube as compared to the other three (Figure 7).
3.2.1.3 The effect of the speed of the target
The 4 (Condition) ANOVA indicated a significant main effect of the speed of the target, F (3,66) = 66.71, p < .001 (ƞ2 = 0.75) (Figure 7). The post hoc tests indicated significant differences in the absolute target-effector distance between each of the four conditions of speed (1.4 m/s ± 1.6 m/s ± 1.8 m/s ± 2 m/s), with an increase of the distance as a function of speed (Figure 7).
3.2.2 Maximal elbow range of movement
3.2.2.1 The effect of gain
The 4(Condition) ANOVA performed on the maximal elbow range of movement revealed a significant main effect of gain, F (3,66) = 488.04, p < .001 (ƞ2 = 0.96) (Figure 8). The post hoc tests indicated a significant decrease in maximal elbow range of movement with the increase in gain (gain 1 ± gain 2 ± gain 3 ± gain 4; Figure 8).
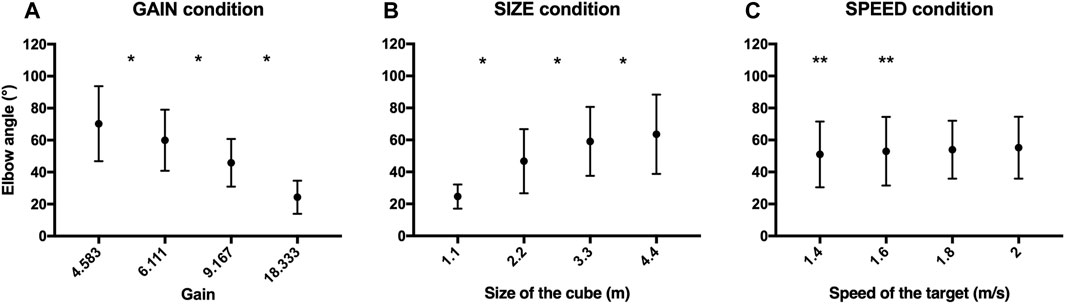
FIGURE 8. Depictions of maximal elbow range of movement (means ± standard deviation) for each variable: gain (A), size (B) and speed (C). *significant differences between all variables, **significant differences of one variable on the others.
3.2.2.2 The effect of the size of the cube
The 4 (Condition) ANOVA analysis indicated a significant main effect of the size of the cube, F (3,66) = 245.51, p < .001 (ƞ2 = 0.92) (Figure 8). The post hoc tests revealed a significant increase in maximal elbow range of movement with the increase in the size of the cube (size 1 ± size 2 ± size 3 ± size 4; Figure 8).
3.2.2.3 The effect of the speed of the target
The 4 (Condition) ANOVA revealed a significant main effect of the speed of the target, F (3,66) = 6.57, p < .001 (ƞ2 = 0.23) (Figure 8). The post hoc tests showed that the maximal elbow range of movement for the two fastest speeds was significantly larger than the amplitude for the slower speed (Figure 8).
3.2.3 Regression analyses between the position of the target in each dimension (x, y, z) and maximal elbow range of movement
3.2.3.1 The effect of gain
The 3 (Dimension) x 4 (Condition) ANOVA performed on the R2 from the regression analyses of elbow angle and target distance in 3 dimensions indicated a significant main effect of Dimension, F (2,44) = 771.24, p < .001 (ƞ2 = 0.97), of the Gain condition, F (3,66) = 10.93, p < .001 (ƞ2 = 0.33) and a significant Dimension * Condition interaction effect, F (6,132) = 3.29, p < .05 (ƞ2 = 0.13) (Figure 9). The post hoc tests performed on the interaction effect indicated significant higher R2 in the z dimension but a slight decrease in the regression score in the higher gain condition in this dimension (Figure 9).
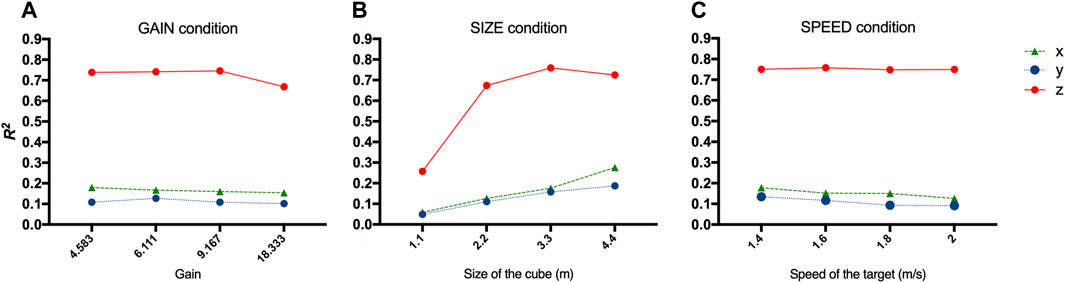
FIGURE 9. Depictions of R2 values (means) for each dimension and condition, in the gain (A), size (B) and speed (C) conditions.
3.2.3.2 The effect of the size of the cube
The 3 (Dimension) x 4 (Condition) ANOVA revealed a significant main effect of Dimension x, y and z, F (2,44) = 595.85, p < .001 (ƞ2 = 0.96), Size condition, F (3,66) = 492.59, p < .001 (ƞ2 = 0.96) and a significant Dimension * Condition interaction effect, F (6,132) = 66.89, p < .001 (ƞ2 = 0.75) (Figure 9). The post hoc tests performed on the interaction effect indicated significantly higher R2 in the z dimension but a decrease in the regression score for the smallest cube (condition 1.1) (Figure 9).
3.2.3.3 The effect of speed
The 3 (Dimension) x 4 (Condition) ANOVA indicated a significant main effect of Dimension F (2,44) = 875.24, p < .001 (ƞ2 = 0.98) and the Speed condition, F (3,66) = 7.33, p < .001 (ƞ2 = 0.25) (Figure 9). The post hoc tests showed significant differences in R2 values for each dimension. Moreover, the R2 values were significantly lower at conditions 1.8 and 2 compared to condition 1.4 m/s (Figure 9). Similarly, as with the gain and size conditions, the R2 values were more significant in the z dimension (Figure 9).
4 Discussion
The aim of the present study was to evaluate the impact of task constraints, specifically the speed of the target, the size of the interaction space, and the gain of movement, on participants’ adaptation to the task, tracking accuracy and the mobilization of the elbow in a 3D virtual target tracking task. We expected that a limited amount of practice would enable the participant to adapt to the task (H1). Moreover, we expected performance to decline (e.g., accuracy) when task constraints were greatest (H2). Similarly, we expected the depth dimension would significantly increase the level of difficulty of the task (H3). Finally, we expected an increase in both the amplitude and the coupling of the elbow in the depth dimension (H4).
4.1 Adaptation to the task (H1)
The adaptation to the task was evaluated through 120 trials, and the results showed that participants quickly adapted with a limited number of trials (Figures 2–4). These results suggest that the task was quickly understood by the participants and carried out with better efficiency, obtained with a limited number of trials (as indicated by the increase in percentage of contact and the decrease in the distance between target and effector with the plateau reached quickly). This rapid adjustment may be due to the clarity of the task goal, the quality of the virtual environment, but also to the feedback which facilitates rapid corrections (Gajda et al., 2016; van der Kooij et al., 2018). Other analyses showed a greater target-effector distance in depth, confirming the results by Gerig et al. (2018) and revealing that this dimension was the most challenging for the participants. To our knowledge, no prior study has attempted to examine tracking tasks in a real 3D environment, and as such, we have no direct comparison with tasks in a real environment, even if online regulation in such a task is probably similar to the regulations required in interceptive actions (e.g., Le Runigo et al., 2005). However, the selected depth cues were similar to those used in the study of Gerig et al. (2018) based on a virtual reaching task. Moreover, the improved accuracy for reaching a plateau after a small amount of practice, coupled with the level of errors which was situated around 0.20 and 0.25 m in a space of interaction of several meters both make us confident regarding the representativity and the feasibility of the task.
4.2 Effect of the speed of the target, the size of the interaction space and the transformation gain in movement on the difficulty of the task and elbow mobilization (H2, H4)
The results concerning the effects of task constraints showed a direct relationship between the task difficulty and the absolute distance between the target and the effector. For each condition of gain, size of the cube and speed of the target, an increase was observed in the absolute target-effector distance when task constraints were at their maximum (i.e., in Gain 4: 18.333, in Size 4: 4.4 m and in Speed 4: 2 m/s) (Figures 5, 6). These results are consistent with those obtained by Buekers et al. (2000), which showed better efficiency in the synchronization of movement (arm movement with a light) when constraints were minimal (movement in the same direction and continuous light). Likewise, in another study using a 2D tracking task, Mallek et al. (2017) showed decreased accuracy in the most constraining conditions of the tracking, when the target bounced on the side of the interaction space. Therefore, the present results confirm the first hypothesis that increasing task constraints induces a decrease in tracking accuracy.
Concerning the control of movement, it was observed that the increase in constraints influenced elbow range-of-motion (Figure 7). This was particularly clear for the Gain and the Size of the cube variables. The results showed that the smaller the gain, the greater the elbow range-of-motion. For the larger gain (18.333), very short movements were required and thus very low mobilization of the elbow. This decrease in movement amplitude did not mean that the task was easier. In this condition, the distance to the target was the highest as pointed out above. Similar results were observed with the size of the cube: there was a progressive increase in elbow mobilization when the size of the cube increased. It is interesting to note that the increase in the speed of the target resulted in a slight increase in elbow involvement. This was probably due to an increase in intensity in the movement produced with the increased speed. These results confirmed our second hypothesis that there is a direct influence of the selected task constraints on elbow amplitude (i.e., an increase in elbow flexions/extensions). These observations are consistent with the work by Van Emmerik and Newell (1990) which showed, in a drawing task, that joint arm kinematics (and the stylus) systematically varied under the effects of constraints. Therefore, in our study, the constraints had a discernible impact on visuomotor dynamics during the task inducing a modification in intra-segment kinematics and range-of-motion movement (Fan et al., 2019).
4.3 Effect of the depth dimension on the difficulty of the task and on elbow mobilization (H3, H4)
As presented above, the results clearly indicated that participants showed more difficulties in the depth dimension during the tracking task. It was also in this dimension that the greatest adaptations were observed across the trials and blocks (Figure 2). The difficulties were probably due to a less accurate perception of the distance between the target and the effector on the z-axis. However, as mentioned by Bingham (2005) and Naceri et al. (2011), depth perception is a key element in the evaluation of reaching, grasping and interaction tasks. In our design, the sources of information for the depth dimension were stereopsis, shadows, parallax, the similar size of the target and the effector, and the overlapping of these objects [see also Gerig et al. (2018), for similar cues]. Nevertheless, it was not possible to evaluate the quality of these sources in comparison to real situations or in comparison to other sources of information or other designs or apparatus (Raab et al., 2013; Gerig et al., 2018). In natural viewing, the changes in vergence and focal distances are the same (meaning that the vergence and accommodative demands are equal), and the slow and fast components work together to produce a relatively rapid and accurate accommodative response. However, a well-recognized limitation of virtual systems stems from the fact that the image is projected on to a single plane of focus. Therefore, the vergence and accommodative demands generally differ, so the slow and fast components attempt to drive accommodation toward different values. Thus, the vergence-accommodation conflict regardless of the targeted depth affects depth perception and can cause visual fatigue (Hoskinson et al., 2004; Hoffman et al., 2008). In this study, we used a typical system for virtual interaction in 3D with the HTC Vive system and the Unity game engine. This system has already been tested in the context of research on perception-motor interaction with conclusive results (e.g., Niehorster et al., 2017; Le Chénéchal and Chatel-Goldman, 2018). However, it does not mean that the system works perfectly, nor that other systems could not provide better information for interacting in the depth dimension. It is therefore possible that a small portion of the errors in the depth dimension may be attributed to the vergence/accommodation conflict inherent in virtual reality headsets. Therefore, as discussed by Brassel et al. (2021), further efforts are required in order to make virtual environments more realistic, thus improving the performances of participants.
The depth dimension played a role in increasing the difficulty of the task, as well as in increasing shoulder mobilization. Across all task constraints, the R2 values were seen to be more important in the z dimension. This shows that the task was clearly achievable in the depth dimension and that this dimension is the basis of the coupling between elbow amplitude and target movement.
5 Conclusion and perspective
Confirming our main hypotheses, the results clearly showed how the manipulation of task constraints (speed of the target, gain and size of the cube) could heighten the task’s level of difficulty. The visuomotor tracking task used in this study also confirmed that the dimension of depth is a key element in the interaction with a virtual environment wherein participants were able to quickly improve their accuracy. This resulted in a strong coupling of the elbow flexion/extension with the target displacement in the depth dimension.
Even if these hypotheses may have appeared straightforward, this research constitutes an essential step in characterizing our 3D tracking task. These results could be used for instance to implement this task in a rehabilitation program for patients with a wide range of disabilities of the upper limb. Importantly, they offer the means for customization through the precise control of task constraints, enabling progressive mobilization of the arm in various directions and amplitudes, according to the specific capabilities of each patient with perceptual-motor impairment. These mobilizations could make it possible to solicit degrees of freedom (Bernstein, 1967) that are generally not solicited in patients (or mobilized specifically in athletes) and specific anatomical positions of the upper limb, such as elbow flexion/extension. This approach could enhance the strength of perception-action coupling during this tracking task (Newell, 1996; Warren, 2006) and result in a possible transfer of these acquisitions in daily activities. These results are also relevant for designers and developers of virtual reality devices, as they identify constraints that can hamper the usability of virtual reality systems. On this basis, other interesting and relevant studies could be conducted, for instance, in the developing field of virtual reality rehabilitation.
Data availability statements
The original contributions presented in the study are included in the article/Supplementary Material, further inquiries can be directed to the corresponding author.
Ethics statement
The studies involving humans were approved by the local Ethics Committee of University of Caen Normandy. The studies were conducted in accordance with the local legislation and institutional requirements. The participants provided their written informed consent to participate in this study.
Author contributions
All authors listed have made a substantial, direct, and intellectual contribution to the work and approved it for publication.
Funding
This work was supported by the “RV rééduc” European project, co-funded by the Normandy County Council and the European Union in the framework of the ERDF-ESF operational programme 2014–2020.
Conflict of interest
Author ML was employed by Open Mind Neurotechnologies.
The remaining authors declare that the research was conducted in the absence of any commercial or financial relationships that could be construed as a potential conflict of interest.
Publisher’s note
All claims expressed in this article are solely those of the authors and do not necessarily represent those of their affiliated organizations, or those of the publisher, the editors and the reviewers. Any product that may be evaluated in this article, or claim that may be made by its manufacturer, is not guaranteed or endorsed by the publisher.
References
Ao, D., Song, R., and Tong, K.-Y. (2015). Sensorimotor control of tracking movements at various speeds for stroke patients as well as age-matched and young healthy subjects. PloS One 10, e0128328. doi:10.1371/journal.pone.0128328
Armbrüster, C., Wolter, M., Kuhlen, T., Spijkers, W., and Fimm, B. (2008). Depth perception in virtual reality: distance estimations in peri- and extrapersonal space. Cyberpsychol. Behav. 11, 9–15. doi:10.1089/cpb.2007.9935
Beppu, H., Nagaoka, M., and Tanaka, R. (1987). Analysis of cerebellar motor disorders by visually-guided elbow tracking movement. 2. Contribution of the visual cues on slow ramp pursuit. Brain J. Neurol. 110 (1), 1–18. doi:10.1093/brain/110.1.1
Beppu, H., Suda, M., and Tanaka, R. (1984). Analysis of cerebellar motor disorders by visually guided elbow tracking movement. Brain J. Neurol. 107 (3), 787–809. doi:10.1093/brain/107.3.787
Bernstein, N. (1967). The Co-ordination and regulation of movements. Oxford, England: Pergamon Press Ltd.
Bideau, B., Kulpa, R., Vignais, N., Brault, S., Multon, F., and Craig, C. (2010). Using virtual reality to analyze sports performance. IEEE Comput. Graph. Appl. 9. doi:10.1109/MCG.2009.134
Bideau, B., Multon, F., Kulpa, R., Fradet, L., Arnaldi, B., and Delamarche, P. (2004). Using virtual reality to analyze links between handball thrower kinematics and goalkeeper’s reactions. Neurosci. Lett. 372, 119–122. doi:10.1016/j.neulet.2004.09.023
Bingham, G. P. (2005). Calibration of distance and size does not calibrate shape information: comparison of dynamic monocular and static and dynamic binocular vision. Ecol. Psychol. 17, 55–74. doi:10.1207/s15326969eco1702_1
Brassel, S., Power, E., Campbell, A., Brunner, M., and Togher, L. (2021). Recommendations for the design and implementation of virtual reality for acquired brain injury rehabilitation: systematic review. J. Med. Internet Res. 23, e26344. doi:10.2196/26344
Buekers, M. J., Bogaerts, H. P., Swinnen, S. P., and Helsen, W. F. (2000). The synchronization of human arm movements to external events. Neurosci. Lett. 290, 181–184. doi:10.1016/S0304-3940(00)01350-1
Choi, W., Lee, J., Yanagihara, N., Li, L., and Kim, J. (2018). Development of a quantitative evaluation system for visuo-motor control in three-dimensional virtual reality space. Sci. Rep. 8, 13439. doi:10.1038/s41598-018-31758-y
Choi, W., Yanagihara, N., Li, L., Kim, J., and Lee, J. (2021). Visuomotor control of intermittent circular tracking movements with visually guided orbits in 3D VR environment. PLOS ONE 16, e0251371. doi:10.1371/journal.pone.0251371
Cutting, J. E. (1997). How the eye measures reality and virtual reality. Behav. Res. Methods Instrum. Amp Comput. 29, 27–36. doi:10.3758/BF03200563
Cutting, J. E. (2021). Movies on our minds: the evolution of cinematic engagement. Oxford: Oxford University Press.
Fan, M., Luo, J., Li, L., Huang, D. F., Zhan, Y., and Song, R. (2019). Kinematic analysis of trajectory dimension-dependent sensorimotor control in arm tracking. IEEE Access 7, 8890–8900. doi:10.1109/ACCESS.2019.2891132
Faure, C., Limballe, A., Bideau, B., and Kulpa, R. (2020). Virtual reality to assess and train team ball sports performance: a scoping review. J. Sports Sci. 38, 192–205. doi:10.1080/02640414.2019.1689807
Fine, J. M., Ward, K. L., and Amazeen, E. L. (2014). Manual coordination with intermittent targets: velocity information for prospective control. Acta Psychol. (Amst.) 149, 24–31. doi:10.1016/j.actpsy.2014.02.012
Gajda, K., Sülzenbrück, S., and Heuer, H. (2016). Financial incentives enhance adaptation to a sensorimotor transformation. Exp. Brain Res. 234, 2859–2868. doi:10.1007/s00221-016-4688-3
Gerig, N., Mayo, J., Baur, K., Wittmann, F., Riener, R., and Wolf, P. (2018). “Missing depth cues in virtual reality decrease performance of three-dimensional reaching movements,” in Proceedings of the 11th international symposium on computer science in sport (IACSS 2017), advances in intelligent systems and computing. Editors M. Lames, D. Saupe, and J. Wiemeyer (Cham: Springer International Publishing), 113–123. doi:10.1007/978-3-319-67846-7_12
Gibson, J. J. (1979). The ecological approach to visual perception, the ecological approach to visual perception. Boston, MA, US: Houghton, Mifflin and Company.
Gielen, C. C. A. M., Dijkstra, T. M. H., Roozen, I. J., and Welten, J. (2009). Coordination of gaze and hand movements for tracking and tracing in 3D. Cortex 45, 340–355. doi:10.1016/j.cortex.2008.02.009
Hoffman, D. M., Girshick, A. R., Akeley, K., and Banks, M. S. (2008). Vergence–accommodation conflicts hinder visual performance and cause visual fatigue. J. Vis. 8, 33–3330. doi:10.1167/8.3.33
Hoskinson, R., Akai, C., Fisher, B., Dill, J., and Po, B. (2004). “Causes of depth perception errors in stereo displays,” in Proceedings of the 1st symposium on applied perception in graphics and visualization - APGV ’04. Presented at the the 1st symposium (Los Angeles, California: ACM Press), 164. doi:10.1145/1012551.1012586
Jo, H., Choi, W., Lee, G., Park, W., and Kim, J. (2020). Analysis of visuo motor control between dominant hand and non-dominant hand for effective human-robot collaboration. Sensors 20, 6368. doi:10.3390/s20216368
Le Chénéchal, M., and Chatel-Goldman, J. (2018). “HTC Vive Pro time performance benchmark for scientific research,” in ICAT-EGVE 2018 (Cyprus: Limassol).
Le Runigo, C., Benguigui, N., and Bardy, B. G. (2005). Perception–action coupling and expertise in interceptive actions. Hum. Mov. Sci., Mot. control Learn. A contribution Eur. Sch. Sports Sci. 24, 429–445. doi:10.1016/j.humov.2005.06.008
Limanowski, J., Kirilina, E., and Blankenburg, F. (2017). Neuronal correlates of continuous manual tracking under varying visual movement feedback in a virtual reality environment. NeuroImage 146, 81–89. doi:10.1016/j.neuroimage.2016.11.009
Mallek, M., Benguigui, N., Dicks, M., and Thouvarecq, R. (2017). Sport expertise in perception-action coupling revealed in a visuomotor tracking task. Eur. J. Sport Sci. 17, 1270–1278. doi:10.1080/17461391.2017.1375014
Naceri, A., Chellali, R., and Hoinville, T. (2011). Depth perception within peripersonal space using head-mounted display. Presence Teleoperators Virtual Environ. 20, 254–272. doi:10.1162/pres_a_00048
Newell, K. M. (1996). “Change in movement and skill: learning, retention, and transfer,” in Dexterity and its development (Hove, England: Psychology Press), 393.
Nguyen, A., Cervellati, F., and Kunz, A. (2017). “Gain compensation in redirected walking,” in Proceedings of the 23rd ACM symposium on virtual reality software and technology. Presented at the VRST ’17: 23rd ACM symposium on virtual reality software and technology (Gothenburg Sweden: ACM), 1–4. doi:10.1145/31391313139167
Niehorster, D. C., Li, L., and Lappe, M. (2017). The accuracy and precision of position and orientation tracking in the HTC vive virtual reality system for scientific research. Percept 8, 204166951770820. doi:10.1177/2041669517708205
Pacheco, M. M., Lafe, C. W., and Newell, K. M. (2019). Search strategies in the perceptual-motor workspace and the acquisition of coordination, control, and skill. Front. Psychol. 10, 1874. doi:10.3389/fpsyg.2019.01874
Park, W., Choi, W., Jo, H., Lee, G., and Kim, J. (2020). Analysis of control characteristics between dominant and non-dominant hands by transient responses of circular tracking movements in 3D virtual reality space. Sensors 20, 3477. doi:10.3390/s20123477
Poulton, E. C. (1957). On the stimulus and response in pursuit tracking. J. Exp. Psychol. 53, 189–194. doi:10.1037/h0043798
Raab, M., de Oliveira, R. F., Schorer, J., and Hegele, M. (2013). Adaptation of motor control strategies to environmental cues in a pursuit-tracking task. Exp. Brain Res. 228, 155–160. doi:10.1007/s00221-013-3546-9
Ranganathan, R., and Carlton, L. G. (2007). Perception–action coupling and anticipatory performance in baseball batting.
van der Kooij, K., Wijdenes, L. O., Rigterink, T., Overvliet, K. E., and Smeets, J. B. J. (2018). Reward abundance interferes with error-based learning in a visuomotor adaptation task. PLOS ONE 13, e0193002. doi:10.1371/journal.pone.0193002
Van Emmerik, R. E. A., and Newell, K. M. (1990). The influence of task and organismic constraints on intralimb and pen-point kinematics in a drawing task. Acta Psychol. (Amst.) 73, 171–190. doi:10.1016/0001-6918(90)90078-T
Vienne, C., Masfrand, S., Bourdin, C., and Vercher, J.-L. (2020). Depth perception in virtual reality systems: effect of screen distance, environment richness and display factors. IEEE Access 8, 29099–29110. doi:10.1109/ACCESS.2020.2972122
Vignais, N., Bideau, B., Craig, C., Brault, S., Multon, F., and Kulpa, R. (2009). Virtual environments for sport analysis: perception-action coupling in handball goalkeeping. Int. J. Virtual Real. 8, 43–48. doi:10.20870/IJVR.2009.8.4.2748
Warren, W. H. (2006). The dynamics of perception and action. Psychol. Rev. 113, 358–389. doi:10.1037/0033-295X.113.2.358
Wilson, G., McGill, M., Jamieson, M., Williamson, J. R., and Brewster, S. A. (2018). “Object manipulation in virtual reality under increasing levels of translational gain,” in Proceedings of the 2018 CHI conference on human factors in computing systems. Presented at the CHI ’18: CHI conference on human factors in computing systems (Montreal QC Canada: ACM), 1–13. doi:10.1145/3173574.3173673
Xie, X., Lin, Q., Wu, H., Narasimham, G., McNamara, T. P., Rieser, J., et al. (2010). “A system for exploring large virtual environments that combines scaled translational gain and interventions,” in Proceedings of the 7th symposium on applied perception in graphics and visualization - APGV ’10. Presented at the the 7th symposium (Los Angeles, California: ACM Press), 65. doi:10.1145/1836248.1836260
Yang, S., and Ferris, T. K. (2020). Supporting multitracking performance with novel visual, auditory, and tactile displays. IEEE Trans. Hum.-Mach. Syst. 50, 79–88. doi:10.1109/THMS.2019.2947580
Zhang, R., Li, B., and Kuhl, S. A. (2014). “Human sensitivity to dynamic translational gains in head-mounted displays,” in Proceedings of the 2nd ACM symposium on spatial user interaction, SUI ’14. Association for computing machinery (New York, NY, USA: ACM), 62–65. doi:10.1145/2659766.2659783
Keywords: 3D virtual environment, target tracking task, constraints, motor control, depth dimension
Citation: Baillet H, Burin-Chu S, Lejeune L, Le Chénéchal M, Thouvarecq R, Benguigui N and Leconte P (2023) Impact of task constraints on a 3D visuomotor tracking task in virtual reality. Front. Virtual Real. 4:1119238. doi: 10.3389/frvir.2023.1119238
Received: 08 December 2022; Accepted: 02 October 2023;
Published: 17 October 2023.
Edited by:
Ronan Boulic, Swiss Federal Institute of Technology Lausanne, SwitzerlandReviewed by:
Sharif Razzaque, SentiAR, Inc., United StatesFrancesco De Pace, Polytechnic University of Turin, Italy
Copyright © 2023 Baillet, Burin-Chu, Lejeune, Le Chénéchal, Thouvarecq, Benguigui and Leconte. This is an open-access article distributed under the terms of the Creative Commons Attribution License (CC BY). The use, distribution or reproduction in other forums is permitted, provided the original author(s) and the copyright owner(s) are credited and that the original publication in this journal is cited, in accordance with accepted academic practice. No use, distribution or reproduction is permitted which does not comply with these terms.
*Correspondence: Héloïse Baillet, bailletheloise@gmail.com
†ORCID ID: Héloïse Baillet, orcid.org/0000-0002-8531-5655; Simone Burin-Chu, orcid.org/0000-0002-4439-1731; Laure Lejeune, orcid.org/0000-0003-0055-4671; Pascale Leconte, orcid.org/0000-0001-9241-7126