- Department of Mechanical Engineering, Graduate School of Engineering, Kobe University, Kobe, Japan
Haptic feedback enables material perception via remote robotics. Both force and vibration information are essential for haptic feedback, and it is important to understand their applicability in different situations. In this study, the relationship between the effective type of haptic feedback and target properties in robot-mediated material discrimination was investigated. A remote-control system including a force presentation device and a wearable vibrotactile display was constructed. In the first experiment, the discrimination performance of material hardness was compared between two types of feedback, force and hybrid (vibrotactile and force) conditions. The results show that both feedback systems allow statistically-significant discrimination of the stimuli, and a significant difference in correct-answer rates between the two feedback conditions was not observed. This indicates that the force system was effective for hardness discrimination, and that there was no superimposed effect of the hybrid system. In the second experiment, the discrimination performance of material roughness was compared between three types of feedback (force, vibrotactile, and hybrid). The results indicate that the rate of correct responses for hybrid feedback condition are significantly higher than those for the force condition. This suggests that hybrid feedback is effective for roughness discrimination. Therefore, the effective type of feedback depends on the properties of target materials, and the superimposed effect of hybrid feedback was only observed in roughness discrimination. These findings play an important role in selecting the best feedback method for a given situation or constructing multiple feedback methods that achieve high discrimination performance.
1 Introduction
With the development of information and virtual reality technology, there are growing demands for remote work and experiences using robots. One such example of remote work is the remote operation of construction machinery. Since an operator can work from a safe location, the machinery can be used in restoration work in cases of disasters (Yoshinada et al., 2019). Remote work has also been applied to other fields of robotics such as humanoid robots (Stückler et al., 2016; Fernando et al., 2012), medical robots (Bodner et al., 2004), and industrial robots (Wang et al., 2014; Klamt et al., 2020). For remote experience, a system to remotely visit museums and art galleries has been reported (Trahanias et al., 2005). Such a remote experience system allows people to enjoy the experience of traveling to faraway places and experience art and culture irrespective of their illnesses or injuries. Another study reported that the experience of traveling to remote locations with the operator’s own legs can be achieved via a bipedal robot (Elobaid et al., 2019). Moreover, home care robots for watching over people at home and communication robots for interacting with others are also expected applications for remote experience robots (Michaud et al., 2007; Kristoffersson et al., 2013).
Haptic feedback technology is an important technology to extend remote work and remote experience (Yokokohji and Yoshikawa, 1994; McMahan et al., 2011; Fernando et al., 2012; Bolopion and Régnier, 2013; Pacchierotti et al., 2016). It has been reported that only using visual information decreases work efficiency and manipulating accuracy in remote work by construction robots (Crundall and Underwood, 1998). One of the possible reasons can be that it is difficult to estimate the force exerted on the environment by the robot using only visual information. To compensate for this, haptic feedback is expected to inform the operator of the presence or absence of contact with the environment and the state of the contact object. In addition, in remote experience and communication via robots, multimodal information can be conveyed to improve the quality of experience, such as a sense of presence (Onishi et al., 2016).
One of the various benefits of haptic feedback technology is robot-mediated material discrimination. The operator can perceive the difference between the materials touched through the robot. For teleoperation of construction robots, haptic feedback enabled the operator to perceive changes in the environment, such as differences in the hardness of the soil to be excavated and enabled sensitive remote work (Nagano et al., 2020). If haptic information can be transmitted in remote travel experiences, a highly realistic experience, which includes contact with various animals and nature, can be provided. In addition, for telepresence systems for the visually impaired (Park et al., 2015), the robot-mediated materials perception will be an added value.
This study focuses on two factors related to material discrimination performance by haptic feedback. A first factor is the type of haptic feedback. Since humans have two primary haptic channels, kinesthetic and cutaneous sensation, different types of feedback methods exist (Hannaford and Okamura, 2016; Choi and Kuchenbecker, 2012). Kinesthetic sensation is perceived via mechanoreceptors in muscles, tendons, and joints, and is the perception of motion and force information. Therefore, force information is commonly used as feedback information for kinesthetic. Cutaneous sensation is the sensation perceived by the mechanoreceptors existing inside the skin. Since the two primary haptic channels can be expected to each play an important role in haptic feedback, this study focuses on both vibration and force as information contributing to cutaneous and kinesthetic sensation, respectively.
A second factor relevant to robot-mediated material discrimination is target properties such as roughness, hardness, and warmness. It is generally agreed that perception of material properties is composed of three to five dimensions, and each dimension is related to different physical properties of materials (Okamoto et al., 2012; Tiest, 2010; Klatzky et al., 2013). Target properties may influence the feedback conditions suitable for material discrimination. For example, the effective type of feedback for roughness discrimination and hardness discrimination may differ. However, the relationship between target property and haptic feedback type has not been investigated. It is not clear whether vibrotactile and force feedbacks should be used differently depending on the situation (target property in this study) and whether there is a superimposed effect of multiple feedbacks.
Therefore, in this paper, we aim to investigate whether the effective feedback changes depending on target property. A remote-control system of a robot arm including a force feedback device and a wearable vibrotactile feedback device is constructed. Subsequently, using the developed system, two experiments are conducted: (1) discriminating materials based on hardness, and (2) discriminating materials based on roughness.
Although multiple haptic feedbacks have been proposed (Khurshid et al., 2016; Yamauchi et al., 2010), their effects when used together and their differences from when used independently are not known. Therefore, this study will contribute to providing methodologies and findings to effectively combine multiple feedbacks or successfully switch between them based on target material properties. Although it could be expected that hybrid feedback to always be more effective than force or vibration feedback alone, experimental results are not simple. The contribution of this study is to experimentally clarify under what conditions and how the effects of hybrid feedback emerge.
The remainder of the paper is organized as follows. Section 2 describes the constructed remote control system. Section 3 and Section 4 describe the methods and results of the material hardness and roughness discrimination experiments, respectively. Section 5 discusses the experimental results and future scope, and Section 6 concludes the paper.
2 Remote control system with force and vibrotactile feedback
2.1 System overview
This study constructed a remote control system capable of transmitting force and vibration information to an operator from a teleoperated robot arm. An overview of the system configuration is shown in Figure 1. The main components were a multi degree-of-freedom (DOF) remote robot arm, a local interface for user positional input and force feedback, two sensors installed on the robot to measure force and vibration, and a vibrotactile display used to provide vibrotactile feedback to an operator.
The remote robot arm was a 7-DOF robot arm (Panda, Franka Emika). For a touch interaction with a material, a 3D printed contactor was attached to the tip of robot. The local interface was a haptic interface (Touch, 3D Systems) that could acquire 6-DOF information of position and posture and present 3-DOF translational force. The frequency response of the haptic interface is shown in (Choi and Tan, 2004). A 6-DOF force sensor (PFS080YA501R6, Leptrino) to measure force information was attached between the contactor and the robot. To measure vibration information, a piezoelectric accelerometer (2302B, Showa Sokki) was attached to the contactor. The accelerometer could measure 1-DOF vibratory signal, and the direction of measurement was perpendicular to the direction of the robot tip, corresponding to the tracing direction. The vibrotactile display consisted of a Velcro belt and voice coil transducer (Vp2, Acoube Laboratory), and could control frequency and amplitude of vibrotactile feedback. The Velcro band was used to adhere the vibrator to the palm of the operator. An audio interface (Rubix 22, Roland Corp.) was used to measure the accelerometer signal at the robot side and display the vibrotactile signal at the operator side. The vibrotactile signal was transmitted to the voice coil transducer via an amplifier (SA-36A Pro, SMSL Technology Co.). The entire network was built using Robot Operating System (ROS).
2.2 Control method
There are three general control methods for robot teleoperation: joint mode, rate control mode, and position control mode (Kim et al., 1987; Morosi et al., 2019). In this study, we adopted the position control mode where the tip position of the local interface corresponds to that of the robot arm. When humans touch a material and recognize its properties, motion information such as tracing speed is used for recognition. It is necessary for the robot to perform the same movements as humans. Situations where the local and remote sides have different positional relationships, such as in joint mode or rate control mode, are considered to be unsuitable. The local interface can acquire 6-DOF information (position and posture), but only the position was commanded while the posture was fixed to simplify the experimental situation. A gain was set during for the process of position transfer since the range of movement differs between the local and remote sides. The gain was empirically determined by the authors based on the stability of the manipulation and the burden on the operator. A first-order Butterworth low-pass filter with a cutoff frequency of 10 Hz was applied to the joint angle commands converted from the position information using inverse kinematics calculations. The frequency of control command was 1.0 kHz.
2.3 Force feedback
A force feedback system was constructed to transmit force sensor information measured at the remote side to the local side via the haptic interface. The sampling frequency of the force sensor was 2.4 kHz. The update frequency of force presentation using the local interface was 1 kHz. The maximum force that could be constantly applied by the local interface was not high (0.88 N), and hence, an empirically determined gain was provided between the measured and commanded forces. To ensure stability (Lawrence, 1993) by taking into account the effect of disturbances on the force feedback system, the feedback gain is set 0.05. A third-order Butterworth low-pass filter with a cutoff frequency of 10 Hz was applied to the measured force information because noise caused by the motion of the remote robot was mixing into the force sensor information. The filter was also used to avoid measuring force when the tip of the robot not in contact with anything which may occur due to inertial forces generated when the robot starts or stops moving.
2.4 Vibrotactile feedback
A vibrotactile feedback system was constructed to reproduce the information from the accelerometer measured on the remote side using by the wearable vibrotactile display on the local side. The sampling frequency of the accelerometer and the update frequency of the reproduced vibration signal were both 24 kHz. The acquisition process between the PC and audio interface was performed at an update frequency of 200 Hz. The vibration information captured to the PC was converted to frequency information using a short-time Fourier transform (STFT) with update frequency 50 Hz, overlap 50%, and a Hamming window. The components below 50 Hz and above 2000 Hz were removed for noise reduction, and were then converted back to time information using an inverse STFT and subsequently reproduced. Figure 2 shows the amplitude-frequency response as a characteristic of the constructed vibrotactile feedback system.
3 Experiment 1: Material hardness discrimination
The first experiment investigated the type of feedback effective for discriminating material hardness. For this experiment, the push touch mode is used. The relationship between target material properties and feedback types is expected to be affected by touch mode such as pushing or stroking. Therefore, to simplify the relationship and ease the investigation, the target property of materials and the touch mode were mapped one-to- one. This idea is supported by the strong linkage between material property and touch mode (Jansen et al., 2013; Nagano et al., 2014; Yokosaka et al., 2016; Cavdan et al., 2021).
3.1 Participants
Ten volunteers (aged 22–24 years, nine right-handed and one left-handed, with no history of deficits in tactile processing) participated in the experiments and operated with their dominant hand. All the participants provided written informed consent. All experimental procedures were approved (number 2013) by the ethical review committee of Kobe University’s Graduate School of Engineering.
3.2 Stimuli
The four materials used in Experiment 1 were aluminum block, urethane rubber, ethylene sponge, and urethane sponge, as shown in Figure 3. All the materials were 100 × 100 × 30 mm in size. These materials were used as the authors felt they were different in hardness when touched.
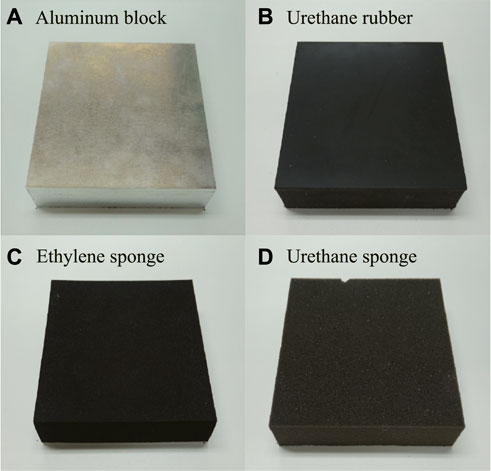
FIGURE 3. Stimuli used in Experiment 1. Four different types of materials are used, both hard and soft. The materials, in order of hardness, are: aluminum block, urethane rubber, ethylene sponge, and urethane sponge.
An example of the force and vibration generated when push motion is generated through the robot is shown in Figure 4. Figures 4A, B correspond to the results for aluminum block and urethane sponge, respectively. For the aluminum block, the push displacement was relatively small, the normal peak force was large, and transient vibrations associated with the collision occurred. On the other hand, for the urethane sponge, the push displacement was larger while the normal peak force was smaller, and significant vibrations were not observed. Therefore, it can be concluded that different materials behave differently in terms of force and vibration, and these are well suited to the two types of haptic feedback.
3.3 Procedure
Two feedback conditions were compared: force feedback and hybrid (force and vibrotactile) feedback. A vibrotactile feedback condition was not included in the experiment. This is because in preliminary trials conducted by the authors with the vibrotactile feedback condition, excessive force was applied to the robot as the material was pushed, causing the motor current value to exceed the maximum allowable current value that resulted in frequent robot stoppages. After explaining the manipulating method, the three phases described below were performed consecutively for each feedback condition. The order of the feedback conditions was randomized for each participant.
The three phases were: training phase using the real robot arm, material confirmation phase, and experimental phase. In the training phase using the actual robot arm, participants practiced pushing into the material using the robot arm. Feedback was also provided to familiarize the participants with the feedback. The participants were instructed to watch a demonstration video that was previously captured using a visualization tool (RViz), and were instructed to perform the pushing motion in accordance with the video. The instructional motion consisted of a repetition of pushing in for 1 s, returning to the original height for 1 s, and standing still for 1 s. The materials used in this phase were different from the four materials used in the experiment. During the material confirmation phase, participants held the robot probe with their hands and touched the four materials through it. This phase was conducted to experience the differences in hardness of the four materials in advance, and there were no time constraints. During the experimental phase, the participants imitated the demonstration video and performed the pushing motion five times, and subsequently they applied the pushing motion to the material, out the four materials, that they were presented with. The trials were performed six times for each material in random order.
During the training and experimental phases, the experimental participants listened to pink noise through headphones to block the influence of auditory information. During the discrimination task, participants were not presented with images of the actual robot contacting the material to avoid hardness discrimination based on visual information. If the robot stopped in the middle of the five pushing motions, the participants were instructed to perform another five pushing motions after the system resumed. Figure 5 shows the experimental phase. However, participants were not presented with the RViz screen in Experiment 1. The duration of the experiment for each participant was approximately 40 min.
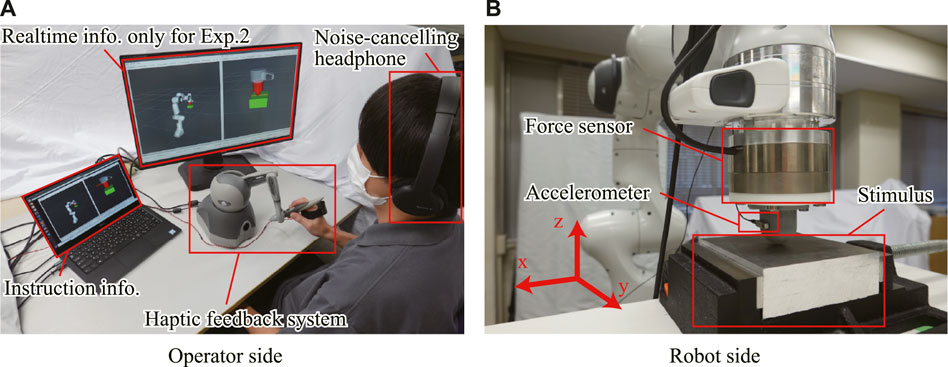
FIGURE 5. Experimental environment. (A) operator side and (B) robot side. The operator controls based on the visual information on the screens and discriminates the stimuli based on the haptic feedback information and the right screen for real-time robot condition feedback is used only in Experiment 2.
3.4 Results
To evaluate the performance of material hardness discrimination, a confusion matrix was calculated using the number of materials that were correctly identified. Figures 6A, B present the mean confusion matrix with force feedback and hybrid (force and vibrotactile) feedback, respectively. The average percentage of correct responses to the four types of stimuli was relatively high in both conditions; where, those for aluminum block and urethane sponge were greater than 90% and those for the urethane rubber and ethylene sponge were greater than 70%. Furthermore, all incorrect answers were in one neighboring material.
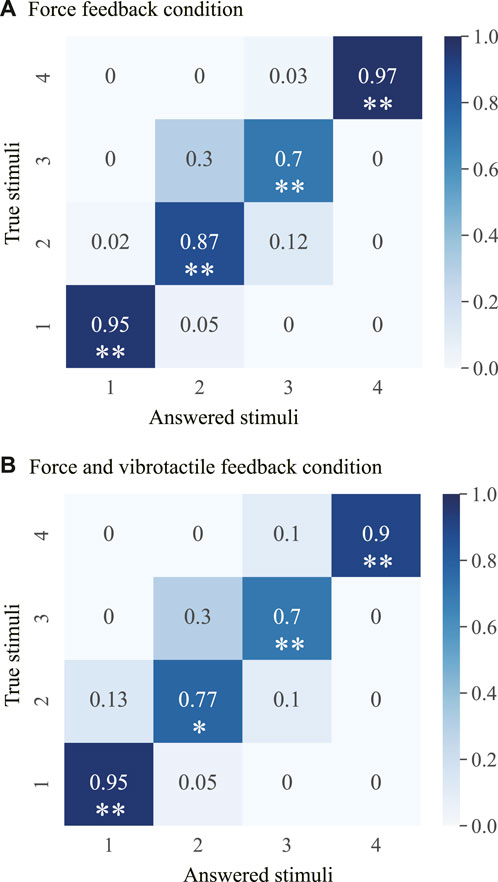
FIGURE 6. Confusion matrix for the material hardness discrimination using push touch mode. 1: hardest, 4: softest. (A) Force feedback condition. (B) Force and vibrotactile feedback condition. *: Bonferroni-adjusted p < 0.0125 and **: Bonferroni-adjusted p < 0.0025.
After the experiment, a brief questionnaire was conducted to determine which feedback condition was easier to understand in the hardness discrimination. The results indicated that two participants found force feedback easier to understand, four participants found both force and vibrotactile easy to understand, and four participants thought that there was no significant difference between the two conditions. The participants that found force and vibrotactile easier to understand felt that they could perceive the vibration when hitting a hard object such as aluminum.
3.5 Analysis
To compare the correct rates with the chance level (1/4) in Figure 6, one-sample Wilcoxon signed-rank test with Bonferroni correction (n = 4) was conducted. The results indicated that all correct rates for both feedback conditions were significantly higher than the chance level (p < 0.0125 (= 0.05/4)). These results imply that participants were able to discriminate the hardness of all four materials with high accuracy under both conditions.
To compare the force and hybrid feedback methods in terms of the performance in material hardness discrimination, Wilcoxon signed-rank test was conducted using the correct rates as shown in Figure 7. The analysis presents that there exists no significant difference between the two feedback conditions for all four material (p > 0.05). This implies that the addition of vibrotactile feedback does not affect the correct response rate, and that force feedback is effective for hardness discrimination of the four stimuli used in the experiment.
4 Experiment 2: Material roughness discrimination
The second experiment investigated the effect of feedback type on material roughness discrimination.
4.1 Participants
The 10 participants who participated in Experiment 1 participated in Experiment 2 after the completion of Experiment 1. As in Experiment 1, all participants in Experiment 2 operated with their dominant hand.
4.2 Stimuli
The four materials used in Experiment 2 were: #30, #240, #800, and #3000 sandpapers, as shown in Figure 8. All materials were attached to the same sponge base (100 × 100 × 30 mm). These materials were selected because the authors felt that the materials possess different roughness when touched.
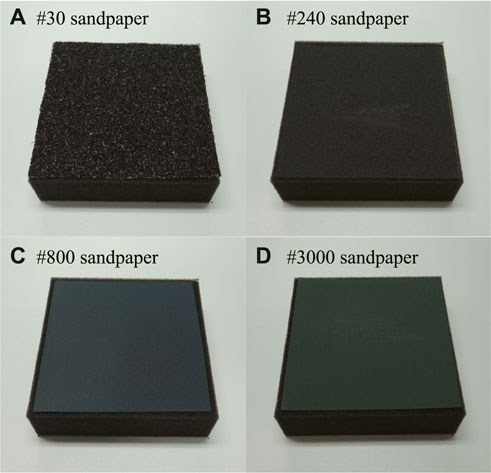
FIGURE 8. Stimuli used in Experiment 2. Four different types of rough and smooth materials. The order of roughness is #30, #240, #800, and #3000 sandpapers.
Figure 9 shows an example of the force and vibration generated by stroke touch mode through the robot. Figures 9A, B correspond to the results for #30 and #3000 sandpapers, respectively. Although there was not a significant difference in the contact force between the two materials when stroking, the vibrations generated seem to be different. The vibration amplitude when stroking on #30 sandpaper seems to be larger than that generated on #3000 sandpaper.
4.3 Procedure
The three feedback conditions to be compared were: force feedback, vibrotactile feedback, and hybrid feedback. As in Experiment 1, the three phases were performed consecutively for each feedback condition. The order of the feedback conditions was randomized for each participant.
The information provided to the participants during the experimental phase of Experiment 2 was also different from that in Experiment 1. In Experiment 2, in addition to the demonstration video, the current posture of the robot was presented to the participants using RViz. This was done because when the stroking motion was performed without the current posture, the direction of the stroking was skewed, and the positions of the beginning and the end of the stroking motion could not be determined. The experiment took an average of 100 min to complete.
4.4 Results
To evaluate the performance of material roughness discrimination, a confusion matrix was calculated using the number of materials that were correctly identified. Figures 10A–C show the results when using force, vibrotactile, and hybrid feedbacks, respectively. Under the force feedback, the answers were quite scattered, indicating a difficulty in discriminating the roughness of the materials. On the other hand, in the two conditions transmitting vibrotactile information, the answer rates were higher than the force condition.
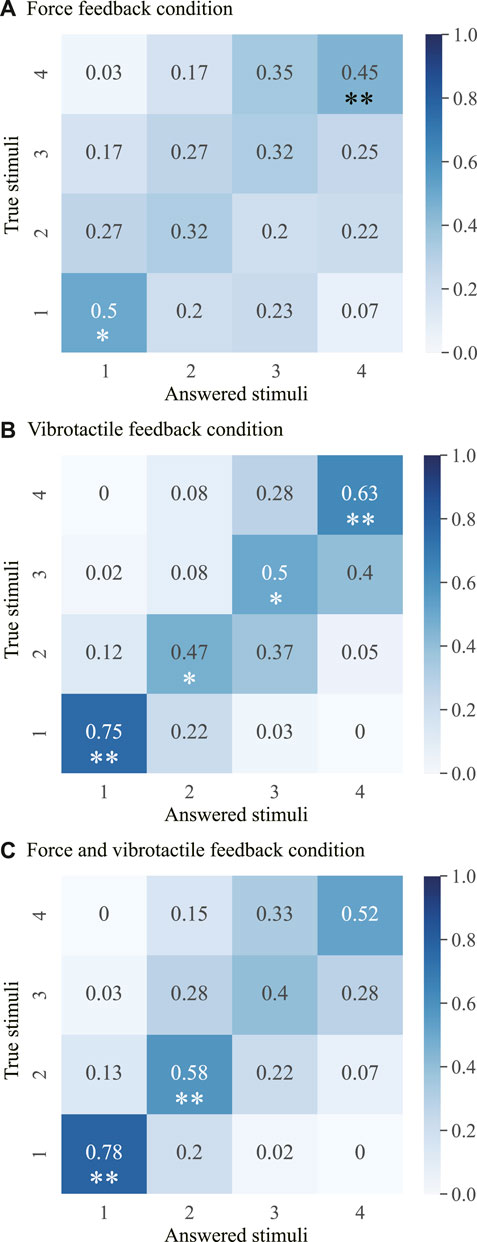
FIGURE 10. Confusion matrix for the material roughness discrimination using stroke touch mode. 1: roughest, 4: smoothest. (A) Force feedback condition. (B) Vibrotactile feedback condition. (C) Force and vibrotactile feedback condition. *: Bonferroni-adjusted p < 0.0125, **: Bonferroni-adjusted p < 0.0025.
The participants were presented with a brief questionnaire after the experiment. The results of the questionnaire indicated that one participant found force feedback condition easy to understand, five participants found vibrotactile feedback condition easy, and four participants found hybrid feedback condition easy.
4.5 Analysis
To compare the correct rates with the chance level (1/4) in Figure 10, one-sample Wilcoxon signed-rank test with Bonferroni correction (n = 4) was conducted. The results indicated that the force feedback condition includes two significant differences, vibrotactile includes four, and hybrid includes two as shown in Figure 10. This test indicated that there was a difference in performance among the three conditions.
The correct answer rates are summarized in Figure 11. The results of the Friedman test showed that there were differences between the three feedback conditions for #30 (p < 0.01), #240 (p < 0.01), and #3000 (p < 0.05) sandpapers. Subsequently, Wilcoxon signed-rank test with Bonferroni correction (n = 3) was applied, and the results showed significant differences between the force and hybrid feedback conditions in both #30 and #240 (p < 0.0167 (= 0.05/3)). For #3000 sandpaper, no significant difference was found among the three feedback conditions in the post hoc test (p > 0.0167 (= 0.05/3)). In the material roughness discrimination using the stroke touch mode, the hybrid feedback showed higher performance than the force feedback in relatively coarse materials (#30 and #240).

FIGURE 11. Correct answer rates for the material roughness discrimination using stroke touch mode. *: Bonferroni-adjusted p < 0.0167.
5 Discussion
5.1 Effective feedback for hardness discrimination
This section discusses the results of Experiment 1, in which no significant difference was found between the force and hybrid feedback conditions in the material hardness discrimination test. For the analytical discussion, the maximum values of contact force and vibration amplitude were used. Data from the last one from six trials in each feedback condition and each stimulus were recorded. The participant averages of the features are shown in Table 1. Although there were 10 participants, the data for one participant could not saved and, hence, an average of nine participants was considered.
Since the z-axis is in the push direction, the z-axis values are relatively large compared to the maximum contact forces in the x- and y-axis. The maximum contact force in the z-axis and the maximum acceleration amplitude were larger for aluminum block, urethane rubber, ethylene sponge, and urethane sponge, in that order, for both feedback conditions. Since both force and vibration seem to contain information about hardness, the hybrid feedback condition was expected to yield a higher percentage of correct responses than the single-feedback condition; however, the results seem to disagree. This can be attributed to the fact that the difference in maximum force is perceptually larger than the difference in vibration amplitude.
Adding vibrotactile feedback to force feedback did not improve the ability to discriminate the hardness of materials. However, force and vibration are not the only haptic channels related to hardness. Contact area (Ambrosi et al., 1999; Bicchi et al., 2000) and pressure distribution (Nagano et al., 2019) information are possible channels. Future directions to improve the discrimination performance of hardness would be to mix these feedbacks with the force feedback.
5.2 Effective feedback for roughness discrimination
In roughness discrimination, although no significant differences were found between the hybrid and vibration feedbacks, the hybrid one was significantly more effective than the force one. This section discusses the factors that contributed to the superior results of the hybrid method.
Similar to the discussion in Experiment 1, the participant averages of the maximum contact force in the three axes and the maximum acceleration amplitude in Experiment 2 are shown in Table 2. The maximum acceleration amplitude was larger for #30, #240, #800, #3000 in that order, for all feedback conditions. The hybrid condition and vibrotactile only condition could have conveyed information about this difference.
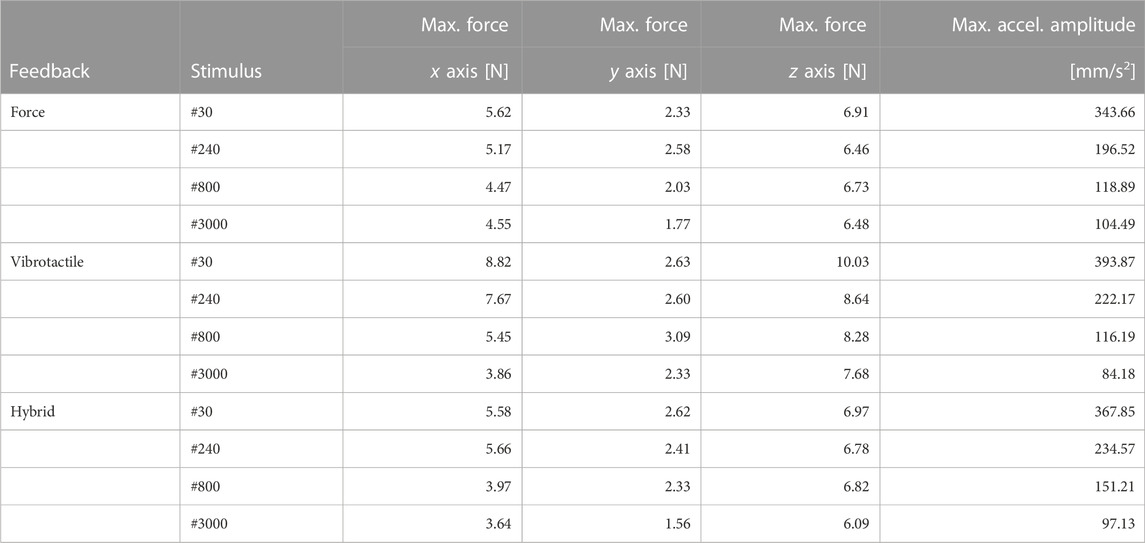
TABLE 2. Mean force and acceleration values during roughness discrimination using stroke touch mode.
In terms of the maximum contact force, compared to vibrotactile feedback, the values in hybrid feedback tend to be more stable across stimuli conditions. This means that the contact force was kept constant under the hybrid condition. This may have made it possible to eliminate differences in force information and concentrate on differences in vibration information, which may have led to the slight difference in performance.
Multiple feedback may be used to stabilize other information in order to focus on the information used for discrimination. This approach improves robustness to changes in information that is not used for discrimination. In this roughness discrimination experiment, the stimuli were of similar hardness, and it is thought that the change in the information to be avoided was a small condition. When stimuli of different hardness are used, the differences between feedback conditions are expected to be more pronounced if the robustness of the hybrid feedback is effective. A study to verify this point is expected as a future prospect.
5.3 Effects of vibrotactile feedback when using tap touch mode
Force feedback conditions were important in discriminating material hardness. However, vibrotactile feedback is also expected to be effective distinguishing between materials in the hard range when using tap touch mode.
Humans can perceive the difference between hardness of materials by vibration and repulsive force generated by tapping (Okamura et al., 2001; Kuchenbecker et al., 2006; Higashi et al., 2017; Higashi et al., 2018). The hardness of the material perceived using tap touch mode is in a harder range than the hardness perceived using push touch mode. Therefore, if vibrotactile feedback can be applied to a remotely piloted robot that can also perform tapping motions, it can be expected to be able to discriminate between a harder range of materials such as metal, glass and plastic.
In this study, it was difficult to perform tapping motion remotely via the robot due to the speed limitation of the robot. For investigations involving tap touch mode under advanced conditions, a robot equipped with robot fingers that can perform dynamic motions would be required. In addition, since it is important to have vibration properties similar to those of humans, robot fingers with viscoelasticity elements and soft skin (Kappassov et al., 2013; Hughes et al., 2018; Chang et al., 2019) may be suitable for this purpose.
In addition, many types of information contribute to material perception, not only collisional vibration caused by tapping. Therefore, ideally, the experiment should be developed to combine various feedback conditions other than just force and vibration. If the feedback stimuli used change, the contribution of each feedback condition will change. The results of this study are limited to the combination of feedbacks used in this study. However, this study contributes as a first step in making an integrated judgment with the findings of future studies using different feedbacks.
5.4 Limitation related to the number of participants
The present study has the limitation about the number of participants. The participants of this study was 10 students in their 20s, making it difficult to investigate the effects of gender and age of the participants on the results. In studies of related tactile perceptions, gender differences in perceived thresholds for pressure stimuli Weinstein, 1968 and age differences in perceived thresholds for vibration stimuli Verrillo, 1980; Stuart et al., 2003 have been reported. In order to investigate the effects of such subject characteristics in the future, it is necessary to design experiments with a large number of participants.
5.5 VR simulation environment as an alternative to real robots
The present study used a remote environment with a real robot to obtain information obtained from real materials. However, there are other approaches that use a VR simulation environment instead of a real robot. In fact, for interaction with VR environments, various force displays El Rassi and El Rassi, 2020; D’Antonio et al., 2021 and vibration displays Iizuka et al., 2016; Lin and Smith, 2018 have been reported. With force simulation in a VR environment, a single physical property such as the spring constant of a virtual plane can be freely adjusted and properties such as JNDs can be individually investigated. Although it is different from realistic materials, it is useful to be able to conduct preliminary studies. However, vibrotactile stimulation is not as easy to simulate based on physical properties as force stimulation, and the use of data-driven approaches Culbertson and Kuchenbecker, 2016 is one promising approach.
6 Conclusion
This study experimentally investigated whether the type of effective feedback depends on the target properties used for robot-mediated material discrimination. The teleoperation system with a force feedback device and a wearable vibrotactile feedback display was constructed; thus, the two experiments were conducted. The first experiment compared two feedback conditions (force and hybrid) for the discrimination of material hardness. The results indicate that both conditions produced high correct answer rates and that force feedback was more effective, while no effect of additional vibrotactile feedback was observed. The second experiment compared three conditions (force, vibrotactile, hybrid) for the discrimination of material roughness. The results indicate that the hybrid feedback condition had significantly higher discrimination performance than the force condition. It can be concluded from the two experiments that the effective type of feedback depends on target properties, and, in roughness discrimination, that the combination of the two types of feedback possibly improve performance. We firmly expect that our work contributes to the importance of using multiple haptic channels as feedback and of selecting the appropriate feedback for the situation. Furthermore, although roughness using stroke and hardness using push were targeted in this study, it is expected that the scope of application will be expanded to other target properties in the future. Future directions could include hardness using tapping, frictional sensation and shape perception.
Data availability statement
The raw data supporting the conclusions of this article will be made available by the authors, without undue reservation.
Ethics statement
The studies involving human participants were reviewed and approved by Human Ethics Committee of Graduate School of Engineering, Kobe University. The patients/participants provided their written informed consent to participate in this study. Written informed consent was obtained from the individual(s) for the publication of any potentially identifiable images or data included in this article.
Author contributions
MT and HN contributed to conception and design of the study and developed the experimental system. MT conducted experiments and performed the statistical analysis. MT and HN drafted the manuscript with feedback and revisions provided by YT and YY. All authors contributed to manuscript revision, read, and approved the submitted version.
Funding
This work was supported by JSPS KAKENHI Grant Number JP20K04398.
Conflict of interest
The authors declare that the research was conducted in the absence of any commercial or financial relationships that could be construed as a potential conflict of interest.
Publisher’s note
All claims expressed in this article are solely those of the authors and do not necessarily represent those of their affiliated organizations, or those of the publisher, the editors and the reviewers. Any product that may be evaluated in this article, or claim that may be made by its manufacturer, is not guaranteed or endorsed by the publisher.
References
Ambrosi, G., Bicchi, A., De Rossi, D., and Scilingo, E. P. (1999). “The role of contact area spread rate in haptic discrimination of softness,” in Proceedings 1999 IEEE International Conference on Robotics and Automation (Cat. No. 99CH36288C), Detroit, MI, USA, 10-15 May 1999 (IEEE), 305–310.
Bicchi, A., Scilingo, E. P., and De Rossi, D. (2000). Haptic discrimination of softness in teleoperation: The role of the contact area spread rate. IEEE Trans. Robotics Automation 16, 496–504. doi:10.1109/70.880800
Bodner, J., Wykypiel, H., Wetscher, G., and Schmid, T. (2004). First experiences with the da vinciTM operating robot in thoracic surgery. Eur. J. Cardio-thoracic Surg. 25, 844–851. doi:10.1016/j.ejcts.2004.02.001
Bolopion, A., and Régnier, S. (2013). A review of haptic feedback teleoperation systems for micromanipulation and microassembly. IEEE Trans. automation Sci. Eng. 10, 496–502. doi:10.1109/tase.2013.2245122
Cavdan, M., Doerschner, K., and Drewing, K. (2021). Task and material properties interactively affect softness explorations along different dimensions. IEEE Trans. Haptics 14, 603–614. doi:10.1109/toh.2021.3069626
Chang, C.-M., Gerez, L., Elangovan, N., Zisimatos, A., and Liarokapis, M. (2019). On alternative uses of structural compliance for the development of adaptive robot grippers and hands. Front. neurorobotics 13, 91. doi:10.3389/fnbot.2019.00091
Choi, S., and Kuchenbecker, K. J. (2012). Vibrotactile display: Perception, technology, and applications. Proc. IEEE 101, 2093–2104. doi:10.1109/jproc.2012.2221071
Choi, S., and Tan, H. Z. (2004). “Effect of update rate on perceived instability of virtual haptic texture,” in 2004 IEEE/RSJ International Conference on Intelligent Robots and Systems (IROS)(IEEE Cat. No. 04CH37566), Sendai, Japan, 28 September 2004 - 02 October 2004 (IEEE), 3577–3582.
Crundall, D. E., and Underwood, G. (1998). Effects of experience and processing demands on visual information acquisition in drivers. Ergonomics 41, 448–458. doi:10.1080/001401398186937
Culbertson, H., and Kuchenbecker, K. J. (2016). Importance of matching physical friction, hardness, and texture in creating realistic haptic virtual surfaces. IEEE Trans. haptics 10, 63–74. doi:10.1109/toh.2016.2598751
D’Antonio, E., Galofaro, E., Patané, F., Casadio, M., and Masia, L. (2021). “A dual arm haptic exoskeleton for dynamical coupled manipulation,” in 2021 IEEE/ASME International Conference on Advanced Intelligent Mechatronics (AIM), Delft, Netherlands, 12-16 July 2021 (IEEE), 1237–1242.
El Rassi, I., and El Rassi, J.-M. (2020). A review of haptic feedback in tele-operated robotic surgery. J. Med. Eng. Technol. 44, 247–254. doi:10.1080/03091902.2020.1772391
Elobaid, M., Hu, Y., Romualdi, G., Dafarra, S., Babic, J., and Pucci, D. (2019). “Telexistence and teleoperation for walking humanoid robots,” in Proceedings of SAI Intelligent Systems Conference (Springer), 1106–1121.
Fernando, C. L., Furukawa, M., Kurogi, T., Kamuro, S., Minamizawa, K., Tachi, S., et al. (2012). “Design of telesar v for transferring bodily consciousness in telexistence,” in 2012 IEEE/RSJ International Conference on Intelligent Robots and Systems, Vilamoura-Algarve, Portugal, 07-12 October 2012 (IEEE), 5112–5118.
Hannaford, B., and Okamura, A. M. (2016). “Haptics,” in Springer handbook of robotics (Springer), 1063–1084.
Higashi, K., Okamoto, S., Yamada, Y., Nagano, H., and Konyo, M. (2017). “Hardness perception by tapping: Effect of dynamic stiffness of objects,” in 2017 IEEE World Haptics Conference (WHC), Munich, Germany, 06-09 June 2017 (IEEE), 37–41.
Higashi, K., Okamoto, S., and Yamada, Y. (2018). Perceived hardness through actual and virtual damped natural vibrations. IEEE Trans. haptics 11, 646–651. doi:10.1109/toh.2018.2841820
Hughes, J., Maiolino, P., and Iida, F. (2018). An anthropomorphic soft skeleton hand exploiting conditional models for piano playing. Sci. Robotics 3, eaau3098. doi:10.1126/scirobotics.aau3098
Iizuka, S., Nagano, H., Konyo, M., and Tadokoro, S. (2016). “Whole hand interaction with multi-finger movement-based vibrotactile stimulation,” in International AsiaHaptics conference (Springer), 157–161.
Jansen, S. E., Tiest, W. M. B., and Kappers, A. M. (2013). Identifying haptic exploratory procedures by analyzing hand dynamics and contact force. IEEE Trans. Haptics 6, 464–472. doi:10.1109/toh.2013.22
Kappassov, Z., Khassanov, Y., Saudabayev, A., Shintemirov, A., and Varol, H. A. (2013). “Semi-anthropomorphic 3d printed multigrasp hand for industrial and service robots,” in 2013 IEEE International Conference on Mechatronics and Automation, Takamatsu, Japan, 04-07 August 2013 (IEEE), 1697–1702.
Khurshid, R. P., Fitter, N. T., Fedalei, E. A., and Kuchenbecker, K. J. (2016). Effects of grip-force, contact, and acceleration feedback on a teleoperated pick-and-place task. IEEE Trans. haptics 10, 40–53. doi:10.1109/toh.2016.2573301
Kim, W., Tendick, F., Ellis, S., and Stark, L. (1987). A comparison of position and rate control for telemanipulations with consideration of manipulator system dynamics. IEEE J. Robotics Automation 3, 426–436. doi:10.1109/jra.1987.1087117
Klamt, T., Schwarz, M., Lenz, C., Baccelliere, L., Buongiorno, D., Cichon, T., et al. (2020). Remote mobile manipulation with the centauro robot: Full-body telepresence and autonomous operator assistance. J. Field Robotics 37, 889–919. doi:10.1002/rob.21895
Klatzky, R. L., Pawluk, D., and Peer, A. (2013). Haptic perception of material properties and implications for applications. Proc. IEEE 101, 2081–2092.
Kristoffersson, A., Coradeschi, S., and Loutfi, A. (2013). “A review of mobile robotic telepresence,” in Advances in human-computer interaction 2013.
Kuchenbecker, K. J., Fiene, J., and Niemeyer, G. (2006). Improving contact realism through event-based haptic feedback. IEEE Trans. Vis. Comput. Graph. 12, 219–230. doi:10.1109/tvcg.2006.32
Lawrence, D. A. (1993). Stability and transparency in bilateral teleoperation. IEEE Trans. robotics automation 9, 624–637. doi:10.1109/70.258054
Lin, P.-H., and Smith, S. (2018). “A tactile feedback glove for reproducing realistic surface roughness and continual lateral stroking perception,” in International Conference on Human Haptic Sensing and Touch Enabled Computer Applications (Springer), 169–179.
McMahan, W., Gewirtz, J., Standish, D., Martin, P., Kunkel, J. A., Lilavois, M., et al. (2011). Tool contact acceleration feedback for telerobotic surgery. IEEE Trans. Haptics 4, 210–220. doi:10.1109/toh.2011.31
Michaud, F., Boissy, P., Labonte, D., Corriveau, H., Grant, A., Lauria, M., et al. (2007). “Telepresence robot for home care assistance,” in AAAI spring symposium: Multidisciplinary collaboration for socially assistive robotics (USA: California), 50–55.
Morosi, F., Rossoni, M., and Caruso, G. (2019). Coordinated control paradigm for hydraulic excavator with haptic device. Automation Constr. 105, 102848. doi:10.1016/j.autcon.2019.102848
Nagano, H., Okamoto, S., and Yamada, Y. (2014). Haptic invitation of textures: Perceptually prominent properties of materials determine human touch motions. IEEE Trans. haptics 7, 345–355. doi:10.1109/toh.2014.2321575
Nagano, H., Sase, K., Konyo, M., and Tadokoro, S. (2019). “Wearable suction haptic display with spatiotemporal stimulus distribution on a finger pad,” in 2019 IEEE World Haptics Conference (WHC), Tokyo, Japan, 09-12 July 2019 (IEEE), 389–394.
Nagano, H., Takenouchi, H., Cao, N., Konyo, M., and Tadokoro, S. (2020). Tactile feedback system of high-frequency vibration signals for supporting delicate teleoperation of construction robots. Adv. Robot. 34, 730–743. doi:10.1080/01691864.2020.1769725
Okamoto, S., Nagano, H., and Yamada, Y. (2012). Psychophysical dimensions of tactile perception of textures. IEEE Trans. Haptics 6, 81–93. doi:10.1109/toh.2012.32
Okamura, A. M., Cutkosky, M. R., and Dennerlein, J. T. (2001). Reality-based models for vibration feedback in virtual environments. IEEE/ASME Trans. mechatronics 6, 245–252. doi:10.1109/3516.951362
Onishi, Y., Tanaka, K., and Nakanishi, H. (2016). “Embodiment of video-mediated communication enhances social telepresence,” in Proceedings of the fourth international conference on human agent interaction, 171–178.
Pacchierotti, C., Scheggi, S., Prattichizzo, D., and Misra, S. (2016). Haptic feedback for microrobotics applications: A review. Front. Robotics AI 3, 53. doi:10.3389/frobt.2016.00053
Park, C. H., Ryu, E.-S., and Howard, A. M. (2015). Telerobotic haptic exploration in art galleries and museums for individuals with visual impairments. IEEE Trans. Haptics 8, 327–338. doi:10.1109/toh.2015.2460253
Stuart, M., Turman, A. B., Shaw, J., Walsh, N., and Nguyen, V. (2003). Effects of aging on vibration detection thresholds at various body regions. BMC Geriatr. 3, 1–10. doi:10.1186/1471-2318-3-1
Stückler, J., Schwarz, M., and Behnke, S. (2016). Mobile manipulation, tool use, and intuitive interaction for cognitive service robot cosero. Front. Robotics AI 3, 58. doi:10.3389/frobt.2016.00058
Tiest, W. M. B. (2010). Tactual perception of material properties. Vis. Res. 50, 2775–2782. doi:10.1016/j.visres.2010.10.005
Trahanias, P., Burgard, W., Argyros, A., Hahnel, D., Baltzakis, H., Pfaff, P., et al. (2005). Tourbot and webfair: Web-operated mobile robots for tele-presence in populated exhibitions. IEEE Robotics Automation Mag. 12, 77–89. doi:10.1109/mra.2005.1458329
Verrillo, R. T. (1980). Age related changes in the sensitivity to vibration. J. gerontology 35, 185–193. doi:10.1093/geronj/35.2.185
Wang, L., Mohammed, A., and Onori, M. (2014). Remote robotic assembly guided by 3d models linking to a real robot. CIRP Ann. 63, 1–4. doi:10.1016/j.cirp.2014.03.013
Weinstein, S. (1968). “Intensive and extensive aspects of tactile sensitivity as a function of body part, sex and laterality,” in The skin senses.
Yamauchi, T., Okamoto, S., Konyo, M., Hidaka, Y., Maeno, T., and Tadokoro, S. (2010). “Real-time remote transmission of multiple tactile properties through master-slave robot system,” in 2010 IEEE International Conference on Robotics and Automation, Anchorage, AK, USA, 03-07 May 2010 (IEEE), 1753–1760.
Yokokohji, Y., and Yoshikawa, T. (1994). Bilateral control of master-slave manipulators for ideal kinesthetic coupling-formulation and experiment. IEEE Trans. robotics automation 10, 605–620. doi:10.1109/70.326566
Yokosaka, T., Kuroki, S., Watanabe, J., and Nishida, S. (2016). Linkage between free exploratory movements and subjective tactile ratings. IEEE Trans. Haptics 10, 217–225. doi:10.1109/toh.2016.2613055
Keywords: vibrotactile display, force display, material perception, haptic feedback, robot teleoperation
Citation: Takahashi M, Nagano H, Tazaki Y and Yokokohji Y (2023) Effective haptic feedback type for robot-mediated material discrimination depending on target properties. Front. Virtual Real. 4:1070739. doi: 10.3389/frvir.2023.1070739
Received: 15 October 2022; Accepted: 20 January 2023;
Published: 03 February 2023.
Edited by:
Elisa Galofaro, Heidelberg University, GermanyReviewed by:
Valay Shah, University of Florida, United StatesGionata Salvietti, University of Siena, Italy
Atsushi Takagi, NTT Communication Science Laboratories, Japan
Copyright © 2023 Takahashi, Nagano, Tazaki and Yokokohji. This is an open-access article distributed under the terms of the Creative Commons Attribution License (CC BY). The use, distribution or reproduction in other forums is permitted, provided the original author(s) and the copyright owner(s) are credited and that the original publication in this journal is cited, in accordance with accepted academic practice. No use, distribution or reproduction is permitted which does not comply with these terms.
*Correspondence: Hikaru Nagano, bmFnYW5vQG1lY2gua29iZS11LmFjLmpw