- Department of Psychology, University of Utah, Salt Lake City, UT, United States
Navigational tools are relied on to traverse unfamiliar grounds, but their use may come at a cost to situational awareness and spatial memory due to increased cognitive load. In order to test for a cost-benefit trade off in navigational cues, we implemented a variety of navigation cues known to facilitate target search and spatial knowledge acquisition of an urban virtual environment viewed through an HTC VIVE Pro as a simulation of cues that would be possible using Augmented Reality (AR). We used a Detection Response Task (DRT) during the navigation task to measure cognitive load and situational awareness. Participants searched for targets in the city with access to a map that did or did not have a “you are here” indicator showing the viewer’s location as they moved. In addition, navigational beacons were also present or absent in the environment as well as a compass and street name indicator. Participants searched for three separate target objects and then returned back to their starting location in the virtual world. After returning home, as a measure of spatial knowledge acquisition, they pointed toward each target from the home location and pointed to home and to the other targets from each target location. Results showed that the navigational cues aided spatial knowledge without increasing cognitive load as assessed with the DRT. Pointing error was lowest when all navigational cues were present during navigation and when pointing was done from home to the target objects. Participants who received the “you are here” indicator on their map consulted the map more often, but without detrimental effects for the acquisition of spatial knowledge compared to a map with no indicator. Taken together, the results suggest that navigational cues can help with spatial learning during navigation without additional costs to situational awareness.
1 Introduction
Navigation allows for interaction with the world that can lead to the acquisition of spatial knowledge. Spatial knowledge is acquired and refined by building up representations of the environment from different sources (Siegel and White, 1975) in likely parallel or continuous processes (Montello, 1998; Ishikawa and Montello, 2006; Chrastil and Warren, 2012). These representations are made stronger with the presence of landmarks–or distinct visual locations within the environment. Landmark knowledge is obtained when traversing a new environment by observing and learning the appearance of landmarks that are highly visible from many viewpoints. Identifying landmarks can help in understanding and updating one’s location in an environment as navigation occurs. Landmarks also contribute to route knowledge, which is gained from internalizing the sequential order of directional changes that encompass a route; and graph or survey knowledge that represents multiple interconnected routes with local or global metric information supporting a “cognitive map” of the environment and more flexible route planning. Recent technologies, such as GPS or mobile Augmented Reality (AR), also now provide navigational aids (e.g., landmarks) outside of the environmental structure itself that could help attain spatial knowledge. However, if these navigational aids become distracting, they may deter the acquisition of spatial knowledge. Thus, a better understanding of the type of information that aids the formation of spatial knowledge without overly distracting the navigator is needed in order to better understand the development of effective cognitive maps as well as technologies to best aid navigation.
The goal of this paper is to investigate how certain cues may aid navigation to form survey spatial knowledge of the traversed environment, while also ensuring that attention is not distracted by the presence of such cues. We are particularly interested in simulating AR cues that can be superimposed on the environment to provide additional information for the development of spatial knowledge. To understand which cues may be most effective, we first review the literature on cueing for navigation in the real world before then turning to which cues and recent technologies have started to be investigated in different types of virtual environments. Finally, we discuss work suggesting that cueing may drive attention to particular locations, which could cause navigators to lose awareness of other situations unfolding in an environment (e.g., situational awareness). We then outline the experiment conducted to assess potential benefits of navigational cueing using the approach of simulated AR in a controlled, immersive virtual environment while also measuring potential costs incurred for continuous situational awareness during navigation.
1.1 Cues in real world navigation
A large body of work has investigated whether and how navigational cues may help to learn the layout of an environment. These cues can be divided into proximal cues (those that are considered near to observers) as compared to distal cues (those that are farther away) (O’Keefe and Nadel, 1978). Proximal cues provide more positional information in closer spaces by establishing relative reference points for spatial representations (e.g., a unique storefront or street sign). Distal cues are large distinctive landmarks that can be seen from a distance as one explores an environment (e.g., a large mountain range or a tall building) (Epstein and Vass, 2014). Effective navigation can involve using both of these types of landmark cues. Proximal elements such as signs are used to ascertain relative positions within the environment, while distal cues such as the mountains are more effective for orientation (Knierim and Hamilton, 2011). However, distal cues may be especially beneficial in the acquisition of survey spatial knowledge (Credé et al., 2020).
In addition to landmarks and cues within the environment, navigators often also rely on aids such as maps, signs, and compasses to guide wayfinding and form cognitive representations of the environment (Thorndyke and Hayes-Roth, 1982; Richardson et al., 1999). These navigational aids are useful because they help to orient navigators within a particular reference frame. In the case of large, outdoor city environments, a global reference frame (i.e., one that allows for orientation, localization or objects, and ordering of elements in the space (Roskos-Ewoldsen et al., 1998)) can even be developed to include an understanding of which direction is north. But, if these directions are not well understood by navigators, compasses can be provided to aid the formation of cognitive representations that are reliably oriented. Weisberg et al. (2018) implemented a compass that emitted a continuous vibrotactile sensation in the direction of true north that updated as navigators moved around and changed orientation. Allowing for vibrotactile stimulation to denote north led to even blind navigators understanding directional cues that aided in the ability to localize and point to external global landmarks over indoor landmarks.
In addition to the use of a compass, GPS is widely popular in leading navigators along optimal routes to a chosen location. It does so often by supplementing information provided by a compass with other cues such as maps and street names (along with detailed route instructions). The benefits of GPS for navigation have been shown when directly comparing navigation performance with GPS to a compass alone (Young et al., 2008). Similarly, Holscher et al. (2007) found that both maps and signs were useful in guiding wayfinding within an indoor environment with the best performance exhibited when both were present. However, the type of map and the amount of information it provides may matter in terms of superior navigation performance. Using GPS can be helpful in getting a navigator to their destination, but it could also negatively affect the acquisition of spatial knowledge (Ishikawa et al., 2008; Münzer et al., 2012; Brügger et al., 2019). Ishikawa et al. (2008) found that participants who were asked to use GPS to navigate made more stops and also traveled farther distances than those who used a traditional map when navigating routes to targets. This difference in navigation efficiency also translated to a poorer ability to draw maps of the routes. Other work also suggests that the use of maps through systems like GPS can affect the acquisition of spatial knowledge, likely because GPS redirects attentional resources to the system rather than the environment (Gardony et al., 2015). GPS users are also worse in their ability to relate learned virtual routes in order to potentially take shortcuts (Ruginski et al., 2019) and in their ability to travel more direct routes based on spatial knowledge (Hejtmánek et al., 2018).
1.2 Cues in virtual navigation
Although many studies have identified that landmarks and other navigational aids are used to guide wayfinding in the real world, the literature has also shown that the effectiveness of these aids can be tested in virtual environments (Chen and Stanney, 1999). Virtual and augmented reality provide a unique tool for manipulating cues in the environment to test the extent to which they are relied upon for successful navigation. For example, virtual reality (VR) has been used to overlay beacons on important objects that need to be found. When precisely aligned to the object, these beacons are shown to be effective in helping users find their targets more quickly (Brunyé et al., 2016). Bolton et al. (2015) showed that overlaying markers for landmarks as in an augmented reality (AR) display also improved navigation performance. Others have manipulated the presence of global and local landmarks in virtual environments displayed on a large projection screen and showed that both types of landmarks were used to make route decisions, but that individuals relied on them differently when the cues were put in conflict with each other (Steck and Mallot, 2000). Recently, Liu et al. (2022) also developed a “virtual global landmark (VGL)” system that constantly displayed a global landmark through a head-worn AR system during navigation. They found better incidental spatial learning with the VGL system as assessed by a map drawing task when compared to a control (auditory instruction only) condition. Further, no additional cognitive workload was present for the VGL system. If the goal of navigation is to build survey spatial knowledge of an environment, then navigation in virtual reality could allow one to experience perspectives not possible in real world navigation that could aid the formation of stronger cognitive maps. Brunyé et al. (2012) tested this by allowing participants to experience a simulated route from a vehicle with the camera positioned either on the front of the vehicle or above it (allowing for a survey perspective). Afterwards, they were asked to navigate to targets experienced along the route. The study measured the extent of spatial knowledge, the time it took to navigate to successive targets, and the heading participants took. Survey perspective allowed for a representation that was larger and more accurate, as well as one that could better compensate for unexpected detours.
Virtual environments allow for unique representations of maps to be implemented that can also be continuously displayed to aid the navigator. This is readily experienced in current video games that implement markers to help the user to know where they are, such as games with a compass with markers as in Skyrim or trails in the environment as in Fable 2 (Johanson et al., 2017). Some of the first studies on virtual navigation compared two different configurations of a virtual map: one with the environment in a fixed orientation (north-up) and the other with the user’s heading constantly facing up (forward-up) (Darken and Cevik, 1999). Findings suggested that forward-up was preferred in targeted search tasks, but that north-up was preferred in free-roaming exploration tasks. Further, Ruddle et al. (1999) tested participants in a large-scale desktop virtual environment and asked them to find targets with both a global and local map, but only the local map represented target positions. They found that searching with both maps was most effective, but that over time use of just the global map became possible. More recently, König et al. (2021) conducted an experiment that tested spatial knowledge acquisition when navigators were able to actively explore a European virtual village compared to when they were only able to use an interactive map. The results showed that judgments for directions to targets were better after exploration than map use, but that knowledge of cardinal direction and relative associations between buildings was improved with map use. In another navigation study through a virtual village, Münzer et al. (2020) compared performance between different map visualization conditions that showed a small map fragment relating self-position to targets or a comprehensive allocentric map. They also manipulated whether the map always provided a north-up frame of reference or heading-up, which rotated with the heading of the navigator. Wayfinding benefited from visualizations that aligned with current heading, and while spatial knowledge acquisition was helped by both types of visualizations, the comprehensive map better supported object-to-object learning. Manipulations of maps so that local or global landmarks are highlighted during wayfinding in a virtual environment has also shown that accentuating local landmarks facilitates route knowledge whereas accentuating global landmarks facilitates survey knowledge (Löwen et al., 2019).
1.3 Potential costs for navigational cues
Although implementing cues for navigation in real and virtual environments has been effective in improving spatial learning and wayfinding, there may be a limit to the benefits these cues have for navigation or a sweet spot in terms of the number of cues that can be added to aid performance. In other words, placing too many cues in the environment could clutter the environment and distract attention from information needed to maintain situational awareness and build spatial knowledge. Indeed, work from other domains has shown that providing cues to users can lead to negative consequences under certain circumstances (Drew et al., 2012, 2020).
Clinicians such as radiologists routinely face the daunting task of finding small abnormalities in complex medical images that may contain hundreds of slices of volumetric images and millions of pixels. To help detect abnormalities, many different computer vision approaches have been used to try to cue locations that may contain cancer or other diagnostically relevant information Doi (2007). In the medical image perception literature there is growing recognition that the effectiveness of these cues greatly depends on how this information is conveyed to the clinician. For instance, cues that appear contemporaneously with the onset of the medical image appear to drive exogenous attention to the cued location. In some of our prior work, this led to less attention and fewer fixations on locations that were not cued (Drew et al., 2012, 2020). Cueing also greatly increased miss rates when the cueing system did not highlight the location of a target item. As a result of these costs, some have suggested that in order to avoid driving spatial attention, cues produced through artificial intelligence will be more effective if they are only revealed when the user queries a specific location–called “interactive Computer Aided Detection” (Hupse et al., 2013; Drew et al., 2020). Ultimately, this literature suggests that providing cues is very effective at driving attention, but it is important to consider the downstream consequences of the method of cueing.
An open question for the current work is how to measure a loss of situational awareness of the environment in a spatial navigation task with cueing included or not. Gardony et al. (2013) had participants navigate a desktop virtual environment with a verbal or tonal aid that presented spatial perspective information and also without an aid. Although both aids improved navigation slightly, this improvement came at a cost to spatial memory, possibly due to the costs associated with attending to the aids. But, a continuous measure of the load associated with attending to the aids was not provided in their study. To continuously assess one’s available resources during navigation, a task could be adapted that has been extensively used to measure cognitive load, or the amount of resources available to attend to many different things in a given time period, known as the Detection Response Task, or DRT (Bruyas et al., 2013). This task has been shown to reliably quantify the cognitive costs of multi-tasking in other naturalistic tasks such as driving or interruptions. This task involves giving auditory or tactile stimuli in short, random intervals with the user being tasked with responding as quickly as possible. When used alongside another task that is cognitively demanding, users tend to take longer to respond or to fail to respond altogether. Thus, this task is useful for measuring cognitive load in a variety of different tasks. For instance, it has been used to measure cognitive load from different modes of message transmissions in tactical military simulations (Hollands et al., 2019). It has also been used to measure cognitive load in driving simulators (Engström et al., 2013; Stojmenova and Sodnik, 2018). For example, Strayer et al. (2015) conducted a study that showed the number of distractions occurring in tasks like driving are directly proportional to cognitive workload. Specifically, they showed the negative effects of voice-activated vehicle systems.
In the context of navigation, several studies have used a task that is conceptually similar to the DRT to assess attentional engagement during route learning using a secondary auditory probe task (Allen and Kirasic, 2003; Hartmeyer et al., 2017; Hilton et al., 2020). These studies found increased response times to an auditory stimulus at navigationally relevant locations around a route, such as decision points, quantifying attentional engagement. Prior work has shown that spatial knowledge is improved when users’ attention is redirected away from looking at a map to looking directly at the environment itself (Lu et al., 2021) and conversely, spatial memory is impaired when navigational aids divide attention (Gardony et al., 2015). Relatedly, as described above, when people actively use GPS in order to navigate their environments, it also hinders their ability to learn environmental layout (Ruginski et al., 2019), as well as their spatial memory for the environment (Hejtmánek et al., 2018; Dahmani and Bohbot, 2020). In the current study, we use the DRT to assess ongoing cognitive load while navigators are searching for targets in an immersive virtual environment either with or without cues present to aid their navigation. The DRT response times allow for assessment of situational awareness given the assumption that when navigators are experiencing less cognitive load, they can be more situationally aware.
A second consideration that could affect the loss of situational awareness in our current study is the manipulation of the presentation of cues as either overlaid on the main field of view of the navigator or outside of it. By its very nature, a secondary map is usually outside of the field of view. However, newer technology, such as immersive VR does allow for presentation of cues within the field of view. For example, a study that assessed navigational cueing while driving showed that users preferred heads-up displays that showed cues in the navigator’s field of view (Jose et al., 2016). Similarly, Gerber et al. (2020) tested whether a heads-up display led drivers to experience less of an attentional switch cost than a mobile phone, and showed that the heads-up display did improve situational awareness compared to a mobile, screen-based display. Cao et al. (2018) also showed that an AR cueing system powered by a mobile device in a vehicle could be an effective system for improving navigation. Important cues were overlaid on video feed of the real environment as driving progressed and taxi drivers rated it as easier to use and less distracting than more traditional systems, such as Google maps. Taken together, all of these studies suggest that displaying cues within the field of view of navigators may be effective at increasing navigation performance as well as reducing costs to situational awareness. Thus, we further test this question in the current study by providing some cues overlaid in the environment, as well as more traditional navigation aids, such as a map and a compass.
2 Overview of current study
The goal of the current experiment was to determine whether adding navigational cues that simulate augmented reality would affect the trade-off between spatial learning and situational awareness in a virtual environment. The key applications for this study are to be found in AR. However, we could not implement AR cues and virtual maps using current AR technologies in a real environment, so we simulated these cues in VR. Doing so also necessitated that we utilize a locomotion method that allowed participants to traverse city-sized spaces in the laboratory. So, participants translated using a joystick and physically turned in the experimental chair. We developed virtual city environments that were displayed to participants either with navigational cues that simulate what one would see in augmented reality (e.g., with beacons, landmarks, cardinal directions) or with no additional cues. In addition, all participants were shown a map depicting the layout of the environments, but some participants were given a positional indicator on their map that updates as they move while others were not. In this way, we were able to test the role of virtual environmental cues that could be displayed overlaid in the environment, such as the landmarks and beacons, as well as more traditional navigational aids, such as a map, on spatial learning and situational awareness. Spatial learning was assessed based on how long it took to return to the initial starting location and with a pointing task after participants had successfully searched for all targets in the virtual city. Participants were asked to locate all the targets from the home position and from the other targets. Situational awareness was assessed with the Detection Response Task (DRT) as well as other behavioral measures during navigation to quantify any potential costs incurred from the added cues.
We had the following hypotheses:
• H1: Spatial knowledge will improve when more environmental navigation cues are present in the city.
• H2: Spatial knowledge will be hindered by maps that give dynamic positional information.
• H3: Situational awareness is expected to decline when environmental navigation cues are present in the city due to increases in cognitive load needed to attend to those cues.
• H4: Situational awareness is expected to decrease as maps present more information (e.g., dynamic positional information), due to their likely being consulted more for navigational search.
3 Experiment
3.1 Participants
We consented a total of 45 participants. Five participants withdrew from the experiment due to simulator sickness. Thus, 40 participants were analyzed with 10 in each combination of cues and map (as listed in Table 1 and described in detail in Section 3.4). Gaming experience (self-reported hours played per week) was similar across participants in the two map conditions (Dynamic: M = 8.78, SD = 13.14, Median = 4.25; Static: M = 10.16, SD = 12.90, Median = 4.0). All participants gave informed consent for their participation and were not aware of the hypotheses.
3.2 Apparatus
The virtual environment was simulated using a Dell PC with an nVidia GTX 3080 graphics card. The virtual environment was displayed to participants via an HTC Vive Pro head-mounted display (HMD). The Vive Pro has a 110° diagonal field of view and weighs 555 g. The VR program was created using Unity (version 2019.2.3f1) and Steam VR (version 1.14.16) and ran as a standalone application on a Windows 10 computer. In order to aid in rotational capability of the participants, all cabling for the HMD was secured to the ceiling with an ability to move with the participant. The participant was seated in a rotating chair so that they could turn freely. In addition, to measure situational awareness, we used the Detection Response Task (DRT), which attached to the participant’s collarbone and provided a vibrotactile cue at random intervals. There was a button device that was attached to the user’s index finger on their non-dominant hand so that they could respond to the vibrotactile stimulation by pressing this finger together with their thumb on the same hand. They were asked to respond as quickly as possible when they felt a vibrotactile stimulus. The participants held a controller from the HTC Vive in their dominant hand in order to control their locomotion and to access information in the task.
3.3 Interaction scenario
We placed participants in a virtual city environment where users could explore and acquaint themselves with the layout of the streets. The streets were laid out in a grid setting that was 3 blocks by 3 blocks of navigable terrain (seen in Figure 1). The length and width of this three-block grid was 660 feet (around 200 m). The dimensions of these blocks were chosen to mirror block lengths in Salt Lake City, which are larger than in most other cities. The blocks visually extended further than the roads, but the extended areas were blocked off with barricades. The objective of the task was to navigate throughout the streets to find three targets that were indicated in the instructions. Because this was a VR space whose city environment is much larger than testing environment would allow, we decided that the main form of locomotion would be visual movement (under active control of the user) in the indicated direction (similar to joystick locomotion in other virtual environments) while they sat in place in a chair. In most virtual navigation systems, when the user presses forward on the touchpad or analog stick, they will move forward in the direction that they are currently looking, and their movement direction changes depending on where they look. For our task, we implemented a different method of locomotion in order to allow the user to look freely without having to consider how that looking affected their movement or possibly induced simulator sickness. For our locomotion method, when the user pressed a direction on the Vive controller touchpad, the system stored the user’s heading and kept that direction as forward regardless of where they looked after that. The forward looking direction stayed the same until the user released the touchpad entirely.
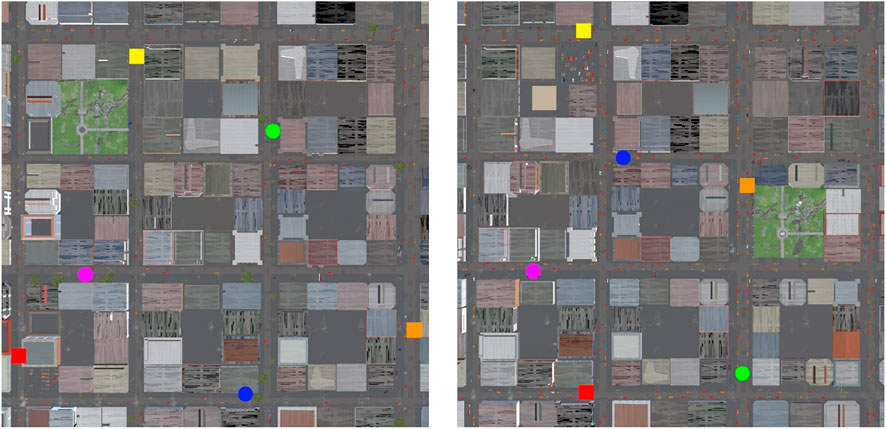
FIGURE 1. Two maps showing City 1 (left) and City 2 (right). The blue and green beacons show the permanent cues that were always visible from the start of navigation, whereas the other beacons are the targets that were shown once users found and marked them. The pink circle is the home location.
We implemented two distinct virtual cities, referred to as City 1 and City 2 to allow for generalization of effects across two environments (see Figure 1, see supplementary materials for list of assets used in cities). Both cities were 3 × 3 blocks and had a very similar overall design with implementation of distal cues in both worlds (i.e., mountains in the distance in certain directions). Both cities also had distinct areas positioned near two of the three targets in the form of a park and a parking lot. The return to home route that was considered most optimal in both cities consisted of a single turn. One city had a return route of 2.75 block lengths and the other had a return route of 3 block lengths (see Figure 1). Both city conditions were populated with cars lining the roads and various inanimate objects on the sidewalks. The types of targets, their placements, and the overall layout of the city differed between city version, as described above. The targets in City 1 were a sports car, an ambulance, and a school bus. City 2 contained a cop car, a news van, and a garbage truck as the targets for search. In addition to the three primary targets the participant was asked to find, there were two distinctive proximal landmarks present in the world in both cue conditions. City 1 contained a gazebo and a helicopter as these stable and distinct proximal landmarks. City 2 contained a helicopter and a tipped over semi-truck as the proximal landmarks.
In one of the conditions of the experiment, the Cues-on condition, there were colored cylinders superimposed on top of these landmarks. These beacon cues were visible through buildings and walls, suggesting that if the buildings were not there, participants could walk directly to the beacon. The beacon cues acted as reference points to provide a relative spatial orientation to the navigator (as well as an indication of distance given their size changed based on relative distance) and were intended to aid survey knowledge acquisition and spatial memory. Similar colored cube overlays appeared on targets after they were located and remained visible through walls, along with a line of the same color attaching the participant to the located target as they moved around. Located near the target cues was information about the name of the located target and the distance (in meters) the participant was from the target (see Figure 2). Whenever an object was found in the cues condition, the cue and the line for it stayed active until the user reached the pointing task. For this experiment, duffel bags, mailboxes, and guns (12 in each city) were randomly placed on the sidewalks of both cities and identified to the participants as BOLO (be on the lookout) objects. Participants were told to try to identify and collect as many of these BOLO objects as they saw while searching for their primary targets. The search for BOLO items provided an additional measure for quantifying situational awareness while navigating. Additionally, both cities displayed street names on the sidewalk at each intersection, along with reasonably sized road signs.
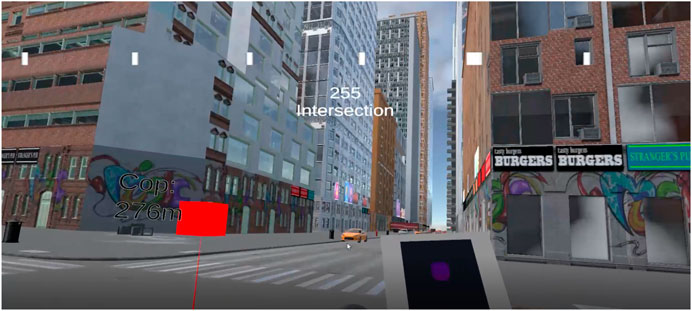
FIGURE 2. An example of the AR cue attached to a found target (police car) that remains visible through the building as one navigates. The visible line also shows the distance and direction between the cue and the navigator.
In order to aid navigation given the size of the virtual environment, participants were also given a virtual map of the city layout, which was a 3 × 3 city block navigable area of the virtual environment (omitting the extended road or the background terrain). We implemented two versions of the map, referred to as the dynamic map and the static map. The dynamic map showed an indicator of the user’s current position and heading that dynamically updated as the user traversed the environment; whereas, the static map had no such indicator.
3.4 Experimental design and procedure
In order to evaluate the effect of the presence of these navigational cues in a VR environment, we designed a between-participants experiment with 2 primary factors that varied across participants: map type (Dynamic or Static) and cue type (Cues-on or Cues-off). Participants were randomly assigned to one of four groups: Dynamic/Cues-on, Dynamic/Cues-off, Static/Cues-on or Static/Cues-off (as listed in Table 1). The city that the participant was assigned to (City 1 or City 2) was randomly assigned, but half of participants navigated City 1 and the other half City 2. In the Dynamic map conditions, the map that participants used had a “you are here” indicator that updated as they moved, and in the Static map conditions, their map did not have that indicator. In the Cues-off conditions, participants could see no more than what they would normally be able to see when navigating in a real-world setting, including street signs and the fixed distal cues (trees and mountains). In the Cues-on conditions, participants had access to three types of cues to augment their navigation beyond those in the Cues-off conditions. The first was a heading indicator that covered the top part of the field of view and functioned as a compass. The second cue was an indicator of what street the participant was currently navigating or the streets that crossed if they were at an intersection. The last cue was two stable beacons in the environment that were present from the start of the experimental trial, as well as cubes that appeared on each of the targets after they were found by the user, as described in more detail below (see Figure 3).
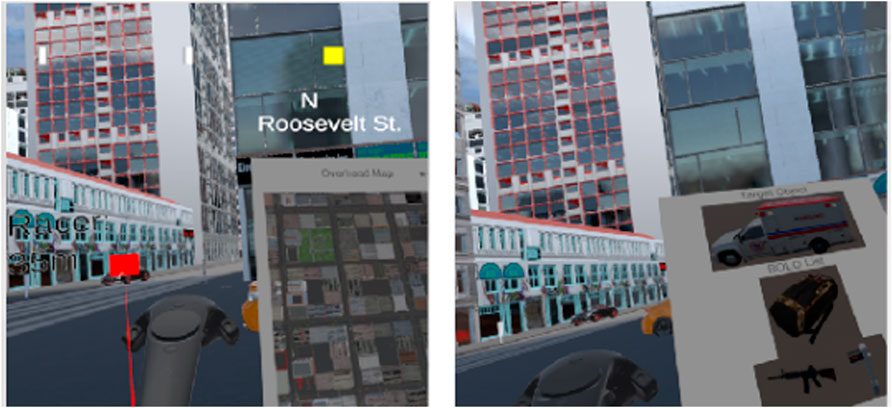
FIGURE 3. Two images showing the same view of the city, the clipboard, and a target (race car) in the Cues-on (left) and Cues-off (right) conditions. The Cues-on condition has a compass on the top of the screen that shows heading and current street location. In addition, the red square on the left side of the Cues-on image shows the target that was previously marked by the user. The clipboard in each image (to the right of the pictured controller) shows examples of the map (left) and the target and BOLO objects to search for (right).
Prior to starting the navigation task, participants completed a few brief questionnaires. These included the Simulator Sickness Questionnaire (SSQ) that addressed their overall comfort level in VR (Saredakis et al., 2020), the Wayfinding Strategy Scale (Lawton and Kallai, 2002), and a set of questions about participants’ prior video game experience. Once the participant completed these surveys, their interpupillary distance (IPD) was measured in order to calibrate the HMD appropriately. They were given a thorough explanation of how they were to interact with the equipment’s controls and a brief overview of their objectives for the task before donning the HMD and being connected to the DRT device. The participant was then placed in a training city where they were able to acclimate to the virtual environment and practice the navigation technique with the controller while responding to the DRT stimulus (by pressing their index finger and thumb together on their non-dominant hand). In this training environment, participants also saw an example target and the BOLO objects displayed on their controller’s virtual clipboard. The clipboard was always attached to the VR controller, and when the user pressed the grip button on the controller, they could access a map of the city. After it was clear that participants understood the task and could effectively control their own locomotion and access the clipboard, we started them in the experimental scenario by teleporting them to the City they were assigned to navigate to search for the targets.
In the actual city, the participant was instructed to look down at their clipboard to view the picture of the first target they should search for along with the pictures of the BOLO objects they were instructed to note if seen during the search task (by clicking the hand controller’s menu button standing within a certain distance from the object), see Figure 3 (right) for an example of the clipboard and items. They were also told to remember the location where they started navigating from in the world (marked as a purple pedestal on the sidewalk) because they would be asked to navigate back to that home position after successfully finding all three targets. They were also told that their task involved not only finding the targets, but also trying to remember where the targets were located as well because they would be tested on their memory for all locations at the end of the experiment. After reiterating that they were able to utilize the map (either static or dynamic depending on condition), the participant was instructed to start searching for their first target on the main roads.
Once the participant found a target, they pulled the trigger on the controller and a virtual cube appeared on the target. They were encouraged to look at their clipboard to view the new picture of their next target. When all targets were located, the participant was instructed to return back to the place where they started (in this phase, they did not have to mark BOLO objects along the way). Time to return home after finding the third target was used as one of the dependent variables to assess spatial knowledge. Once home, the virtual cubes on the targets disappeared, the DRT was turned off, and the participant was instructed on how to perform a point-to-origin task to assess their spatial memory among the targets they found. The participant was prompted to point at each target’s relative location by directing an augmented line from their controller to where they remember the target being. After the participant pointed at all three targets from home, they were teleported to the location of one of the targets, where they pointed to all three of the other points of interest (two targets and the home location). This final step was repeated for the other two targets, resulting in 12 pointing trials. Pointing accuracy was measured by the degree of error from their augmented line to the actual location of the target and was used as our second dependent variable assessing spatial knowledge. After completion of the pointing task and removing the HMD and DRT, the participant was asked to complete the second part of the SSQ. Finally, the participant was debriefed and compensated for their time.
3.5 Results: Spatial knowledge
Our first two hypotheses concerned the effects of Cues and Map Type on spatial knowledge. We predicted that (H1) spatial knowledge would improve when environmental cues were present (Cues-on) and that (H2) spatial knowledge would be hindered by maps that give dynamic positional information (Dynamic Map). We quantified spatial knowledge in two ways. First, we analyzed Time to Return Home, the time between when the participant marked the final target and when they arrived at the initial start location. Second, we analyzed Pointing Error, the absolute value of the angular difference between the direction that the user pointed and the actual direction to the target. Before running our primary analyses, we assessed the possible influence of the two different city environments with independent samples t-tests run on time to return home and average pointing error. There was no difference between the cities for either measure of performance (time to return: t (38) = 0.96, p = 0.35; pointing error: t (38) = 0.81, p = 0.42), so subsequent analyses do not include city as a factor.
3.5.1 Time to return home
A 2 (Cues) X 2 (Map Type) univariate analysis of variance (ANOVA) with gaming hours added as a covariate (run as GLM in SPSS) on time to return home showed no effect of cue F (1, 35) = 2.66, p = 0.11, η2 = 0.07 or map type F (1, 35) = 0.11, p = 0.74, η2 = 0.003, and no interaction between cue and map type, F (1, 35) = 0.14, p = 0.71, η2 < 0.004 (see Figure 4).
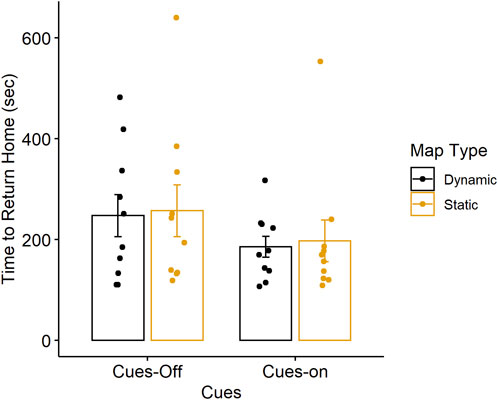
FIGURE 4. Average time to return to home for each cue and map type condition. Error bars represent ± 1 standard error. Individual points represent time to return home for each participant.
3.5.2 Pointing error
Participants completed 12 pointing trials as a measure of their spatial memory. These included pointing from home to each target object found while navigating as well as from each target object to each of the other targets and to home. We calculated the absolute value of the angular difference between the participant’s pointing direction and the actual direction to the target and then averaged the absolute pointing error across trials for each pointing location (3 home-to-target, 3 target-to-home and 6 target-to-target).
We ran a 3 (Pointing Location) x 2 (Cues) x 2 (Map Type) repeated-measures ANOVA (run as GLM in SPSS) on absolute pointing error, with pointing location as a within-participants variable and cues and map type as between-participants variables, and gaming hours as a covariate. There was no effect of gaming hours, F (1, 35) = 0.008, p = 0.93, η2 = 0.0001. We found an effect of cues, F (1, 35) = 4.23, p < 0.05, η2 = 0.10, with lower error in the Cues-on (M = 34.82) compared to the Cues-off condition (M = 50.58). There was no effect of map type, F (1, 35) = 0.01, p = 0.92, η2 = 0.0001 and no cues x map type interaction, F (1, 35) = 0.82, p = 0.37, η2 = 0.02. There was no effect of pointing location, F (2, 70) = 0.60, p = 0.55, η2 = 0.02 but planned within-subjects contrasts revealed an interaction between pointing location and cues (for home to target vs target to home), F (1, 35) = 4.13, p = 0.05, η2 = 0.10. To further examine this interaction, we ran a 3 (Pointing Location) x 2 (Map Type) ANOVA on the averaged absolute pointing error for the Cues-on and Cues-off conditions separately. The Cues-off condition did not show a significant effect of location, but the Cues-on condition did exhibit a significant effect of pointing location, F (1, 36) = 3.30, p < 0.05, η2 = 0.15. Planned contrasts showed that error was lower when pointing from home-to-target (M = 28.55), F (1, 18) = 6.23, p < 0.05, η2 = 0.25 versus target-to-home (M = 40.77), but there was no difference between target-to-target (M = 35.27) and home-to-target, F (1, 18) = 1.34, p = 0.26, η2 = 0.08 (see Figure 5).
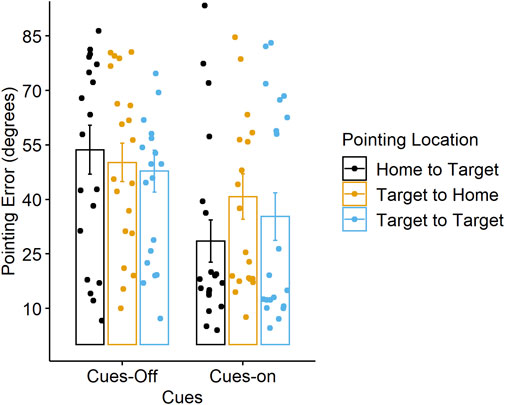
FIGURE 5. Average pointing error for each cue condition and pointing location. Error bars represent ± 1 standard error. Individual points represent averaged pointing error for each participant.
3.6 Results: Situational awareness
Our third and fourth hypotheses concerned the effects of Cues and Map Type on situational awareness. We predicted that (H3) situational awareness would decline when environmental navigation cues were present (Cues-on) and that (H4) situational awareness would decrease as maps present more positional information (Dynamic map), due to being consulted more for navigational search. We quantified situational awareness in several ways. First, we assessed response time and accuracy on the DRT. Increased response time and decreased accuracy in responding (missed stimuli) is associated with an increase in cognitive load and a decline in situational awareness. Second, we assessed use of the map by analyzing number of calls to the map during the search and return tasks as well as the percentage of time spent looking at the map. Our assumption was that more time spent looking at the map meant less time looking directly at the environment, which is necessary to maintain situational awareness. Third, we analyzed success in finding BOLOs as a measure of whether participants were able to attend to other parts of the environment while navigating.
As in the spatial knowledge analyses, we first assessed the possible influence of the two different city environments with independent samples t-tests run on the situational awareness measures. There was no difference between the cities for DRT average response time during the search phase (t (36) = 0.76, p = 0.45) or the return phase (t (36) = 1.39, p = 0.17, DRT accuracy during search (t (36) = 0.13, p = 0.90) or return (t (36) = 0.43, p = 0.67, calls to the map during the search phase (t (38) = 0.43, p = 0.66) or the return phase (t (38) = −0.46, p = 0.65), time spent on the map during search (t (38) = 0.01, p = 0.99) or during return (t (38) = −0.87, p = 0.39), so subsequent analyses do not include city as a factor.
3.6.1 DRT response time and accuracy
Our software recorded the DRT data for each participant separately from the recording of the other data files and was timestamped to allow for syncing with the navigation data. Two participants’ DRT data were not recorded due to an unknown error, resulting in 38 participants analyzed. The DRT data was first screened for outliers, and responses that were less than 200 msec or greater than 3,00 msec were removed. This resulted in the removal of 1.98% of the search phase and 0.48% of the trials in the return phase.
Response Time. A linear mixed effects model is appropriate for modeling the DRT response time data because of the repeated-measures design involving many trials per participant that also varied in number (due to the differences in overall search and return time between individuals and differences in accuracy in responding to the probes). Mixed effects modeling allows for partitioning of variance both within and between participants. The response time distributions were positively skewed and non-normal, as is typical of response time data. Thus, we ran a generalized Gaussian mixed effects linear model with log link, using the glmer function from the lme4 package in R. The dependent variable was response time. The fixed effects of Cues (Cues-on = 1, Cues-off = 0) and Map Type (dynamic = 1, static = 0), the interaction between Cues and Map Type, and gaming hours were included along with a participant random intercept to account for differences between participants. We tested for the fixed effects using likelihood ratio tests (model comparisons with the full model reported as X2). The analysis on the Search Phase showed no effects of cues (B = 0.22, SE = 0.18, X2 (2) = 1.74, p = 0.42) or map type (B = 0.17, SE = 0.19, X2 (2) = 1.51, p = 0.47), and no cue x map type interaction (B = −0.34, SE = 0.27, X2 (1) = 1.51, p = 0.22). There was an effect of gaming hours (B = −0.01, SE = 0.01, X2 (1) = 4.28, p = 0.04) such that DRT response time decreased with increasing gaming experience. We also performed this same analysis for the Return Phase. Similarly, there was no effect of cues (B = 0.19, SE = 0.18, X2 (2) = 2.12, p = 0.35) or map type (B = 0.20, SE = 0.18, X2 (2) = 2.11, p = 0.35), and no cue x map type interaction (B = −0.40, SE = 0.27, X2 (1) = 2.11, p = 0.15). There was an effect of gaming hours (B = −0.01, SE = 0.01, X2 (1) = 4.92, p = 0.03); response time decreased with increased gaming hours, consistent with the performance in the search phase.
Accuracy. Accuracy was calculated for each participant as their number of responses to the vibrotactile stimuli divided by the total number of stimuli (after the outlier removal). For the Search Phase, a 2 (Cues) X 2 (Map Type) ANOVA on accuracy, with gaming hours as a covariate, showed no effect for cues, F (1, 33) = 0.009, p = 0.92, η2 = 0.001, or map type, F (1, 33) = 2.12, p = 0.15, η2 = 0.06, and no cue x map type interaction, F (1, 33) = 0.12, p = 0.73, η2 = 0.004. There was also no effect of gaming hours, F (1, 33) = 2.58, p = 0.11, η2 = 0.07. For the Return Phase, a 2 (Cues) X 2 (Map Type) ANOVA on accuracy, with gaming hours as a covariate, also showed no effect of cues, F (1, 33) = 0.28, p = 0.60, η2 = 0.01, or map type F (1, 33) = 0.96, p = 0.33, η2 = 0.02, and no cue x map type interaction, F (1, 33) = 0.26, p = 0.61, η2 = 0.008. There was also no effect of gaming hours, F (1, 33) = 1.60, p = 0.21, η2 = 0.04. See Table 2 for DRT response times and accuracy means and standard deviations for each condition.
3.6.2 Cognitive load at different stages of navigation with DRT
Given the lack of predicted DRT effects as a function of cue presence and map type, we conducted further analyses on the DRT to assess whether it would reveal other differences in cognitive load that would be expected during navigation and search. For example, responses made at intersections where turn decisions are necessary should reveal increases in cognitive load as compared to responses outside of intersections, as seen in prior work (Hartmeyer et al., 2017; Hilton et al., 2020).
Search Phase. We ran similar generalized linear mixed effects models as described above to test the effect of responses made at intersections versus along a street on DRT response time, but with fixed effects of response Location (intersection = 1, street = 0) and gaming hours, along with a participant random intercept. There was a significant effect of Location (B = 0.07, SE = 0.01, X2 (1) = 54.83, p < 0.0001). Response times were longer for the probes at intersections (M = 991.81 ms, SD = 371.19) compared to along the streets (M = 899.85 ms, SD = 396.26) (see Figure 6). There was no effect of gaming hours (B = −0.01, SE = 0.01, X2 = 3.26, p = 0.07). Likewise, a paired t-test comparing location effects on accuracy revealed significantly lower accuracy at intersections (M = 80.9%, SD = 17) compared to the street (M = 89.4%, SD = 10), t (37) = −5.18, p < 0.001, Cohen’s d = −0.84.
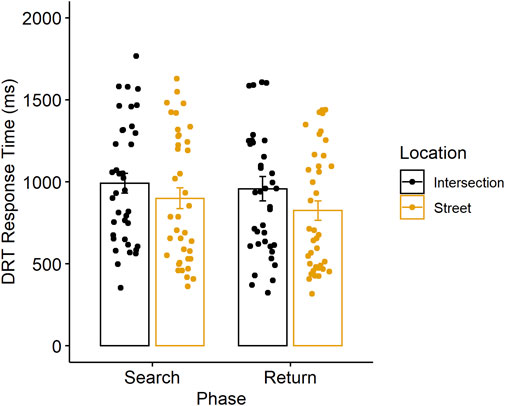
FIGURE 6. Average response time for the DRT at intersections versus along the street in the search and return phases. Error bars represent ± 1 standard error. Individual points are averaged response times for each participant.
We also assessed DRT differences as a result of target proximity with the rationale that attentional resources are needed when identifying a target, so responses made close to a target should be affected more than those made when farther away. We coded being “near to a target” as being within 20 feet from the target, and anything farther than that was not considered to be near. This decision was made based on the design of the search task where we determined that the participant could truly identify the target at a distance of 20 m or less, but not farther. The generalized linear mixed effect model used fixed effects of Target Proximity (near = 1, not near = 0) and gaming hours and a participant random intercept. There was a significant effect of Target Proximity (B = 0.07, SE = 0.02, X2 (1) = 6.57, p < 0.01). Response times were longer when near the target (M = 1008.05 ms, SD = 398.74) compared to far from from the target (M = 921.57 ms, SD = 385.84). There was no effect of gaming hours (B = −0.01, SE = 0.01, X2 (1) = 3.23, p = 0.07). Similarly, a paired t-test showed lower DRT accuracy near the target (M = 61.34%, SD = 24) compared to away from a target (M = 88.15%, SD = 11), t (37) = −9.29, p < 0.001, Cohen’s d = −1.51).
Return Phase. The same models were run for the Return Phase as in the Search Phase to test the effects of Location on DRT response time and accuracy. There was a significant effect of Location (B = 0.11, SE = 0.02, X2 (1) = 21.40, p < 0.0001). DRT response times were longer at intersections (M = 957.98 ms, SD = 455.84) versus on a street (M = 824.14 ms, SD = 369.81) (see Figure 6). Although numerically lower in percentage, there was no difference in accuracy at intersections (M = 83.6%, SD = 20) compared to along the street (M = 88.0%, SD = 9) (p = 0.10). We did not analyze differences as a function of target proximity given that the return task did not involve searching for targets. Together, these results validate the use of the DRT as an effective measure of cognitive load during navigation, despite the lack of expected effect from additional environmental cues or a dynamic map.
3.6.3 Use of the map: Number of calls and time in use
We analyzed differences in using the static and dynamic map in two ways. First, we analyzed number of calls to the map during the Search Phase with a 2 (Cue) x 2 (Map Type) ANOVA with gaming hours as a covariate. Number of calls to the map were greater when using the dynamic map (M = 21.65) compared to the static map (M = 6.00), F (1, 35) = 32.89, p < 0.001, η2 = 0.48. Number of calls to the map during the Return Phase also were greater with the dynamic map (M = 4.60) compared to the static map (M = 1.70), F (1, 35) = 9.16, p < 0.01, η2 = 0.20. There were no effects gaming hours, cues, and no cue x map type interaction.
Second, we analyzed the percentage of time spent looking at each map. A 2 (Cue) x 2 (Map Type) ANOVA with gaming as a covariate showed no effect of cues, map type or interactions on time looking at the map during the Search Phase. However, during the Return Phase, we found a cue x map type interaction, F (1, 35) = 13.26, p < 0.001, η2 = 0.27. Although percentage of time was overall low, post-hoc t-tests on map type for each cue condition revealed that for the Cues-on condition, percentage of time using the dynamic map was higher (M = 5.47%) than the static map (M = 0.15%), t (18) = 4.43, p < 0.001, Cohen’s d = 1.98. There was no difference between dynamic and static map time in the Cues-off condition (p = 0.21).
3.6.4 BOLO search success
Finally, we compared the number of BOLO objects found, which was a measure of whether participants were able to attend to the environment as they navigated through it. In order to avoid having the total length of time spent navigating confound the analysis, we calculated a ratio of the number of BOLOs that the user found to the total number that they passed. On average, participants found 58% of BOLOs in the Cues-off condition and 66% in the Cues-on condition; 64% with the Dynamic map and 60% with the Static map. We ran a 2 (Cue) x 2 (Map Type) ANOVA with gaming hours as a covariate on the ratios of BOLOs found. We found no effect of cues F (1, 35) = 1.90, p = 0.17, η2 = 0.05, or map type F (1, 35) = 0.51, p = 0.48, η2 = 0.01, no cue x map type interaction, F (1, 35) = 0.38, p = 0.54, η2 = 0.01, and no effect of gaming hours, F (1, 35) = 2.41, p = 0.13, η2 = 0.06.
3.7 Results: SSQ
We also analyzed ratings on the SSQ before and after the virtual navigation task. Table 3 shows the mean ratings for current experience of each symptom using the following scale: 0 = none, 1 = slight, 2 = moderate, 3 = severe). Although there were slight increases in mean ratings for some of the symptoms in the post-test compared to the pre-test, all means were below 1, suggesting little evidence of simulator sickness due to the task.
4 Discussion
Augmented Reality (AR) has the potential to improve navigation efficiency and spatial learning of an environment by overlaying cues in the environment to guide wayfinding and the development of accurate cognitive maps. These navigational cues were simulated in VR in the current study due to an inability to fully implement them in AR. However, in either technology, the addition of cues could impose additional costs to situational awareness. Prior work in medical imaging and driving suggests that while cueing is effective at driving attention to specific locations, it can also distract attention from other locations, thereby reducing awareness of the rest of the scene or environment. In navigation, there is a growing body of work suggesting that navigational aids in many forms (e.g., GPS directions, maps, highlighting of landmarks) can help with wayfinding tasks but may also negatively influence spatial learning and memory due to a misallocation of attention. In the current study, we investigated the question of how providing additional cues for navigation would affect both spatial learning and situational awareness in the same experimental paradigm. Because we could not implement AR cues and virtual maps using current AR technologies in a real environment, we simulated an AR-like application in VR. Given the range of navigational aids possible, we examined traditional aids, such as maps, that are inherently outside of the environment as well as virtual cues that were placed directly in the urban virtual environment. We predicted that spatial knowledge would be improved with the addition of the embedded environmental navigation cues, but possibly hindered by a reliance on maps that gave dynamic positional information (i.e, due to these maps likely drawing attention away from the environment more). We also implemented a measure of cognitive load during navigation, the DRT, to objectively address whether attentional load is increased with the addition of cues, which could affect situational awareness. We expected that situational awareness would decrease with the additional environmental cues–due to increased attention to those cues–and with a dynamic map–due to the tendency to rely more on the map during search. Our results partially supported our hypotheses, finding that the virtual environmental cues did improve spatial learning, but we did not find negative effects of the dynamic map. Further, we were surprised that additional environmental cueing had little effect on situational awareness as measured by the DRT. Despite this, the DRT provided a reliable measure of cognitive load associated with other dimensions of navigation that require attention. We discuss each of these findings below, along with some limitations of the current study and future directions for research.
Our first hypothesis was that spatial knowledge would improve with the additional cues overlaid on the city environment. We added a compass, an indicator that displayed the current street, and virtual beacons that were overlaid on stable large objects or targets after they were found. All of these cues provided additional information for spatial orientation and some also provided information about the distance to targets. The compass provided a cardinal direction for current heading, the street name emphasized the grid street layout, and the beacons served as distal landmarks that could be used to triangulate other target locations and to inform an allocentric representation of the spatial layout of the city environment. The virtual cubes that were attached to the targets also could serve as proximal landmarks, used to update spatial location as the participant passed by. The greater pointing accuracy that resulted in the Cues-on condition compared to the Cues-off condition suggests that together, this set of simulated AR cues facilitated the acquisition of survey spatial knowledge of the city environment. These results replicate and extend prior work that found virtual cues to be effective landmarks (Bolton et al., 2015; Brunyé et al., 2016; Liu et al., 2022). We also found that pointing accuracy was best when pointing from home to the targets as compared to targets to home. This pattern of performance is consistent with what has been found in other large-scale spatial learning tasks (Gagnon et al., 2018) and suggests that even though participants show that they have formed target-to-target spatial representations, there may still be an advantage for memories formed from one’s initial starting location.
Our second hypothesis stated that spatial knowledge would be worse after navigating with the dynamic map compared to the static map. This prediction was based on the growing evidence for impaired survey spatial knowledge when using GPS directions (Ishikawa et al., 2008; Münzer et al., 2012; Brügger et al., 2019) and the notion that the dynamic map would engage more attention than the static map. Although we did find that participants accessed (with the controller) and spent more time looking at the dynamic map compared to the static map, there was no effect on spatial knowledge. This result has implications for choices of navigational aids to assist navigational tasks. At least based on the current scenario, dynamic maps can provide more information with little cost. The lack of an effect of the dynamic map on spatial learning also suggests that it is not as detrimental to performance as has been found with GPS, suggesting that dynamic maps may be able to aid navigation with less distraction than GPS (as discussed further below). There are numerous ways to measure survey spatial knowledge and the current study used only two–pointing accuracy from multiple locations and time to return home. It is possible that other measures, such as sketch maps or landmark placement tasks, would have been more sensitive to our manipulations, and these measures could be incorporated into future work.
Our third and fourth hypotheses focused on measures of situational awareness. We predicted that attending to the virtual cues would increase cognitive load and result in a decline in situational awareness. We also predicted that use of the dynamic map would have similar effects. Neither of these hypotheses was supported. The DRT, our primary measure of cognitive load, showed no difference in response time or accuracy as a function of the cue condition or map condition. Given prior work showed a direct, positive relationship between distractions while driving and cognitive load as assessed with the DRT, we were surprised that our task did not also show a detriment in load based on cue type (Strayer et al., 2015). We deliberately chose to use a vibrotactile stimulus given that we were attempting to simulate AR where it would be difficult to present a visual probe for the DRT and visual AR cues at the same time without cluttering the visible display area. It is possible that using a visual stimulus instead would have shown an effect of cues on attentional load. However, multiple simple stimuli (visual, auditory, vibrotactile) are standardized for the DRT as a measure of cognitive workload (ISO DIS 17488, 2016) and a recent analysis by Strayer et al. (2022) argues that visual and vibrotactile probes similarly reveal cognitive load. It is even more important that we were able to demonstrate that performance on the DRT could be manipulated–response time increased and accuracy decreased in situations that would be expected to require more cognitive resources or divided attention, such as at intersections where wayfinding decisions were made (Brunyé et al., 2018; Hilton et al., 2020) and in close proximity to targets as they were identified. Thus, we consider these findings to be strong evidence that the DRT does reflect changes in cognitive load during navigation, and that our cue and map manipulations did not have strong detrimental effects on situational awareness. Prior work using a similar auditory probe during navigation used a passive route following task where slides or video recordings of virtual environments were presented (Allen and Kirasic, 2003; Hartmeyer et al., 2017; Hilton et al., 2020). We have extended this work to demonstrate the DRT as a feasible method to assess cognitive load during actively controlled virtual navigation tasks, and encourage future use of it as an assessment of load in both virtual and real environments.
The current study provides an evaluation of the effectiveness of visual cues in a VR environment that simulated what could be possible in future AR technologies. For example, AR devices could overlay highlighting and beacons on environments in real-time, outdoor navigation tasks to aid navigators during wayfinding and search. Understanding whether and how AR cues can aid spatial learning while maintaining situational awareness could inform a variety of applied domains, such as military applications to aid efficient and safe navigation in novel environments or assistive technologies for those with cognitive or sensory impairment. We acknowledge that our initial evaluation was conducted completely in VR, which is obviously different from overlaying AR cues on a real environment. Further, our virtual environment task necessarily relied on controller-based navigation that limited the body-based cues available for translation during travel, such as proprioceptive and vestibular information for path integration and orientation. Rotational cues that provided some body-based information were present, but prior work suggests that translational information may also be important for understanding orientation and distance traveled (Ruddle and Lessels, 2006; Ruddle et al., 2011). Ongoing work using AR displays for navigation in the real world will help to address this difference.
Our results showing improvement in spatial learning without cost to situational awareness are intriguing but also must be interpreted only in the context of the current experimental design using five beacons and a relatively large city area. We did not parametrically vary the number of cues, the amount of clutter, or the size of the environment to be traversed. Future work could certainly continue the investigation of how many cues as well as where and how they are implemented are best for aiding navigation to promote spatial learning. It is possible (and likely, if pushed to the extreme) that adding too many cues would lead to attentional distraction and a potential loss of situational awareness. The question of how many and which types of cues lead to distraction will be important to address in future work. We must also acknowledge that we used a between-subjects design with a relatively small sample size and between-subjects variability could have contributed to the lack of effects of cues found on situational awareness. Finally, while we can confidently claim that our virtual cues helped in spatial learning, our “all-or-none” cue manipulation does not allow us to isolate which of our cues were used or most helpful given the current results. Use of eye tracking methodology could more precisely identify which cues are most effective in these tasks and further quantify attentional costs of cueing during navigation in future work as well.
Data availability statement
The raw data supporting the conclusions of this article will be made available by the authors, without undue reservation.
Ethics statement
The study involving human participants was reviewed and approved by University of Utah Institutional Review Board (study number 00132736). Written informed consent to participate in this study was provided by all participants.
Author contributions
DB helped to write and edit the manuscript, made the figures and tables, and analyzed data. HF developed the virtual environment and experimental procedure and helped to collect the data. EW collected the data and contributed to writing the Method section. JS, SC-R, and TD conceptualized the project, analyzed the data, and wrote and edited the manuscript.
Funding
This work was funded by the U.S. Army Combat Capabilities Development Command Soldier Center Measuring and Advancing Soldier Tactical Readiness and Effectiveness (MASTR-E) program under contract #W911NF2020268 and by the Office of Naval Research under contract #N00014-21-1-2583.
Acknowledgments
We thank Tyler Labov for his help in collecting data for these experiments.
Conflict of interest
The authors declare that the research was conducted in the absence of any commercial or financial relationships that could be construed as a potential conflict of interest.
Publisher’s note
All claims expressed in this article are solely those of the authors and do not necessarily represent those of their affiliated organizations, or those of the publisher, the editors and the reviewers. Any product that may be evaluated in this article, or claim that may be made by its manufacturer, is not guaranteed or endorsed by the publisher.
Supplementary Material
The Supplementary Material for this article can be found online at: https://www.frontiersin.org/articles/10.3389/frvir.2022.971310/full#supplementary-material
References
Allen, G. L., and Kirasic, K. C. (2003). “Visual attention during route learning: A look at selection and engagement,” in International Conference on Spatial Information Theory (Springer), 390–398.
Bolton, A., Burnett, G., and Large, D. R. (2015). “An investigation of augmented reality presentations of landmark-based navigation using a head-up display,” in Proceedings of the 7th International Conference on Automotive User Interfaces and Interactive Vehicular Applications, 56–63.
Brügger, A., Richter, K.-F., and Fabrikant, S. I. (2019). How does navigation system behavior influence human behavior? Cogn. Res. 4, 5–22. doi:10.1186/s41235-019-0156-5
Brunyé, T. T., Gardony, A. L., Holmes, A., and Taylor, H. A. (2018). Spatial decision dynamics during wayfinding: Intersections prompt the decision-making process. Cogn. Res. 3, 13–19. doi:10.1186/s41235-018-0098-3
Brunyé, T. T., Gardony, A., Mahoney, C. R., and Taylor, H. A. (2012). Going to town: Visualized perspectives and navigation through virtual environments. Comput. Hum. Behav. 28, 257–266. doi:10.1016/j.chb.2011.09.008
Brunyé, T. T., Moran, J. M., Houck, L. A., Taylor, H. A., and Mahoney, C. R. (2016). Registration errors in beacon-based navigation guidance systems: Influences on path efficiency and user reliance. Int. J. Human-Computer Stud. 96, 1–11. doi:10.1016/j.ijhcs.2016.07.008
Bruyas, M.-P., Dumont, L., and Bron, F. (2013). “Sensitivity of Detection Response Task (drt) to the driving demand and task difficulty,” in Proceedings of the seventh international driving symposium on human factors in driver assessment, training, and vehicle design (Iowa City: University of Iowa), 64–70.
Cao, C., Li, Z., Zhou, P., and Li, M. (2018). Amateur: Augmented reality based vehicle navigation system. Proc. ACM Interact. Mob. Wearable Ubiquitous Technol. 2, 1–24. doi:10.1145/3287033
Chen, J. L., and Stanney, K. M. (1999). A theoretical model of wayfinding in virtual environments: Proposed strategies for navigational aiding. Presence. (Camb). 8, 671–685. doi:10.1162/105474699566558
Chrastil, E. R., and Warren, W. H. (2012). Active and passive contributions to spatial learning. Psychon. Bull. Rev. 19, 1–23. doi:10.3758/s13423-011-0182-x
Credé, S., Thrash, T., Hölscher, C., and Fabrikant, S. I. (2020). The advantage of globally visible landmarks for spatial learning. J. Environ. Psychol. 67, 101369. doi:10.1016/j.jenvp.2019.101369
Dahmani, L., and Bohbot, V. D. (2020). Habitual use of gps negatively impacts spatial memory during self-guided navigation. Sci. Rep. 10, 6310–6314. doi:10.1038/s41598-020-62877-0
Darken, R. P., and Cevik, H. (1999). “Map usage in virtual environments: Orientation issues,” in Proceedings IEEE virtual reality (cat. no. 99CB36316 (IEEE), 133–140.
Doi, K. (2007). Computer-aided diagnosis in medical imaging: Historical review, current status and future potential. Comput. Med. imaging Graph. 31, 198–211. doi:10.1016/j.compmedimag.2007.02.002
Drew, T., Cunningham, C., and Wolfe, J. M. (2012). When and why might a computer-aided detection (cad) system interfere with visual search? An eye-tracking study. Acad. Radiol. 19, 1260–1267. doi:10.1016/j.acra.2012.05.013
Drew, T., Guthrie, J., and Reback, I. (2020). Worse in real life: An eye-tracking examination of the cost of cad at low prevalence. J. Exp. Psychol. Appl. 26, 659–670. doi:10.1037/xap0000277
Engström, J., Larsson, P., and Larsson, C. (2013). “Comparison of static and driving simulator venues for the tactile detection response task,” in Proceedings of the seventh international driving symposium on human factors in driver assessment, training, and vehicle design, 369–375.
Epstein, R. A., and Vass, L. K. (2014). Neural systems for landmark-based wayfinding in humans. Phil. Trans. R. Soc. B 369, 2012053333. doi:10.1098/rstb.2012.0533
Gagnon, K. T., Thomas, B. J., Munion, A., Creem-Regehr, S. H., Cashdan, E. A., and Stefanucci, J. K. (2018). Not all those who wander are lost: Spatial exploration patterns and their relationship to gender and spatial memory. Cognition 180, 108–117. doi:10.1016/j.cognition.2018.06.020
Gardony, A. L., Brunyé, T. T., Mahoney, C. R., and Taylor, H. A. (2013). How navigational aids impair spatial memory: Evidence for divided attention. Spatial Cognition Comput. 13, 319–350. doi:10.1080/13875868.2013.792821
Gardony, A. L., Brunyé, T. T., and Taylor, H. A. (2015). Navigational aids and spatial memory impairment: The role of divided attention. Spatial Cognition Comput. 15, 246–284. doi:10.1080/13875868.2015.1059432
Gerber, M. A., Schroeter, R., Xiaomeng, L., and Elhenawy, M. (2020). “Self-interruptions of non-driving related tasks in automated vehicles: Mobile vs head-up display,” in Proceedings of the 2020 CHI Conference on Human Factors in Computing Systems, 1–9.
Hartmeyer, S., Grzeschik, R., Wolbers, T., and Wiener, J. M. (2017). The effects of attentional engagement on route learning performance in a virtual environment: An aging study. Front. Aging Neurosci. 9, 235. doi:10.3389/fnagi.2017.00235
Hejtmánek, L., Oravcová, I., Motỳl, J., Horáček, J., and Fajnerová, I. (2018). Spatial knowledge impairment after gps guided navigation: Eye-tracking study in a virtual town. Int. J. Human-Computer Stud. 116, 15–24. doi:10.1016/j.ijhcs.2018.04.006
Hilton, C., Miellet, S., Slattery, T. J., and Wiener, J. (2020). Are age-related deficits in route learning related to control of visual attention? Psychol. Res. 84, 1473–1484. doi:10.1007/s00426-019-01159-5
Hollands, J. G., Spivak, T., and Kramkowski, E. W. (2019). Cognitive load and situation awareness for soldiers: Effects of message presentation rate and sensory modality. Hum. Factors 61, 763–773. doi:10.1177/0018720819825803
Holscher, C., Buchner, S. J., Brosamle, M., Meilinger, T., and Strube, G. (2007). “Signs and maps–cognitive economy in the use of external aids for indoor navigation,” in Proceedings of the Annual meeting of the Cognitive Science Society.29.
Hupse, R., Samulski, M., Lobbes, M. B., Mann, R. M., Mus, R., den Heeten, G. J., et al. (2013). Computer-aided detection of masses at mammography: Interactive decision support versus prompts. Radiology 266, 123–129. doi:10.1148/radiol.12120218
Ishikawa, T., Fujiwara, H., Imai, O., and Okabe, A. (2008). Wayfinding with a gps-based mobile navigation system: A comparison with maps and direct experience. J. Environ. Psychol. 28, 74–82. doi:10.1016/j.jenvp.2007.09.002
Ishikawa, T., and Montello, D. R. (2006). Spatial knowledge acquisition from direct experience in the environment: Individual differences in the development of metric knowledge and the integration of separately learned places. Cogn. Psychol. 52, 93–129. doi:10.1016/j.cogpsych.2005.08.003
ISO DIS 17488 (2016). Road vehicles–transport information and control systems–detection response task (DRT) for assessing attentional effects of cognitive load in driving. ISO TC 22/SC39/WG8.
Johanson, C., Gutwin, C., and Mandryk, R. L. (2017). “The effects of navigation assistance on spatial learning and performance in a 3d game,” in Proceedings of the Annual Symposium on Computer-Human Interaction in Play, 341–353.
Jose, R., Lee, G. A., and Billinghurst, M. (2016). “A comparative study of simulated augmented reality displays for vehicle navigation,” in Proceedings of the 28th Australian conference on computer-human interaction, 40–48.
Knierim, J. J., and Hamilton, D. A. (2011). Framing spatial cognition: Neural representations of proximal and distal frames of reference and their roles in navigation. Physiol. Rev. 91, 1245–1279. doi:10.1152/physrev.00021.2010
König, S. U., Keshava, A., Clay, V., Rittershofer, K., Kuske, N., and König, P. (2021). Embodied spatial knowledge acquisition in immersive virtual reality: Comparison to map exploration. Front. Virtual Real. 4. doi:10.3389/frvir.2021.625548
Lawton, C. A., and Kallai, J. (2002). Gender differences in wayfinding strategies and anxiety about wayfinding: A cross-cultural comparison. Sex. roles 47, 389–401. doi:10.1023/a:1021668724970
Liu, J., Singh, A. K., and Lin, C. T. (2022). Using virtual global landmark to improve incidental spatial learning. Sci. Rep. 12 (1), 6744. doi:10.1038/s41598-022-10855-z
Löwen, H., Krukar, J., and Schwering, A. (2019). Spatial learning with orientation maps: The influence of different environmental features on spatial knowledge acquisition. ISPRS Int. J. Geoinf. 8, 149. doi:10.3390/ijgi8030149
Lu, J., Han, Y., Xin, Y., Yue, K., and Liu, Y. (2021). “Possibilities for designing enhancing spatial knowledge acquirements navigator: A user study on the role of different contributors in impairing human spatial memory during navigation,” in Extended Abstracts of the 2021 CHI Conference on Human Factors in Computing Systems, 1–6.
Montello, D. R. (1998). A new framework for understanding the acquisition of spatial knowledge in large-scale environments. Spatial temporal Reason. Geogr. Inf. Syst., 143–154.
Münzer, S., Lörch, L., and Frankenstein, J. (2020). Wayfinding and acquisition of spatial knowledge with navigation assistance. J. Exp. Psychol. Appl. 26, 73–88. doi:10.1037/xap0000237
Münzer, S., Zimmer, H. D., and Baus, J. (2012). Navigation assistance: A trade-off between wayfinding support and configural learning support. J. Exp. Psychol. Appl. 18, 18–37. doi:10.1037/a0026553
Richardson, A. E., Montello, D. R., and Hegarty, M. (1999). Spatial knowledge acquisition from maps and from navigation in real and virtual environments. Mem. cognition 27, 741–750. doi:10.3758/bf03211566
Roskos-Ewoldsen, B., McNamara, T. P., Shelton, A. L., and Carr, W. (1998). Mental representations of large and small spatial layouts are orientation dependent. J. Exp. Psychol. Learn. Mem. Cognition 24, 215–226. doi:10.1037/0278-7393.24.1.215
Ruddle, R. A., and Lessels, S. (2006). For efficient navigational search, humans require full physical movement, but not a rich visual scene. Psychol. Sci. 17, 460–465. doi:10.1111/j.1467-9280.2006.01728.x
Ruddle, R. A., Payne, S. J., and Jones, D. M. (1999). The effects of maps on navigation and search strategies in very-large-scale virtual environments. J. Exp. Psychol. Appl. 5, 54–75. doi:10.1037/1076-898x.5.1.54
Ruddle, R. A., Volkova, E., and Bülthoff, H. H. (2011). Walking improves your cognitive map in environments that are large-scale and large in extent. ACM Trans. Comput. Hum. Interact. 18, 1–20. doi:10.1145/1970378.1970384
Ruginski, I. T., Creem-Regehr, S. H., Stefanucci, J. K., and Cashdan, E. (2019). Gps use negatively affects environmental learning through spatial transformation abilities. J. Environ. Psychol. 64, 12–20. doi:10.1016/j.jenvp.2019.05.001
Saredakis, D., Szpak, A., Birckhead, B., Keage, H. A., Rizzo, A., and Loetscher, T. (2020). Factors associated with virtual reality sickness in head-mounted displays: A systematic review and meta-analysis. Front. Hum. Neurosci. 14, 96. doi:10.3389/fnhum.2020.00096
Siegel, A. W., and White, S. H. (1975). The development of spatial representations of large-scale environments. Adv. Child. Dev. Behav. 10, 9–55. doi:10.1016/s0065-2407(08)60007-5
Steck, S. D., and Mallot, H. A. (2000). The role of global and local landmarks in virtual environment navigation. Presence. (Camb). 9, 69–83. doi:10.1162/105474600566628
Stojmenova, K., and Sodnik, J. (2018). Detection Response Task—Uses and limitations. Sensors 18, 594. doi:10.3390/s18020594
Strayer, D. L., Castro, S. C., Turrill, J., and Cooper, J. M. (2022). The persistence of distraction: The hidden costs of intermittent multitasking. J. Exp. Psychol. Appl. 28, 262–282. doi:10.1037/xap0000388
Strayer, D. L., Turrill, J., Cooper, J. M., Coleman, J. R., Medeiros-Ward, N., and Biondi, F. (2015). Assessing cognitive distraction in the automobile. Hum. Factors 57, 1300–1324. doi:10.1177/0018720815575149
Thorndyke, P. W., and Hayes-Roth, B. (1982). Differences in spatial knowledge acquired from maps and navigation. Cogn. Psychol. 14, 560–589. doi:10.1016/0010-0285(82)90019-6
Weisberg, S. M., Badgio, D., and Chatterjee, A. (2018). Feel the way with a vibrotactile compass: Does a navigational aid aid navigation? J. Exp. Psychol. Learn. Mem. Cognition 44, 667–679. doi:10.1037/xlm0000472
Keywords: virtual reality, augmented reality, navigation, spatial memory, situational awareness, cognitive load
Citation: Stefanucci JK, Brickler D, Finney HC, Wilson E, Drew T and Creem-Regehr SH (2022) Effects of simulated augmented reality cueing in a virtual navigation task. Front. Virtual Real. 3:971310. doi: 10.3389/frvir.2022.971310
Received: 16 June 2022; Accepted: 06 September 2022;
Published: 29 September 2022.
Edited by:
Stephen Palmisano, University of Wollongong, AustraliaReviewed by:
Sebastien Miellet, University of Wollongong, AustraliaElizabeth Chrastil, University of California, United States
Copyright © 2022 Stefanucci, Brickler, Finney, Wilson, Drew and Creem-Regehr. This is an open-access article distributed under the terms of the Creative Commons Attribution License (CC BY). The use, distribution or reproduction in other forums is permitted, provided the original author(s) and the copyright owner(s) are credited and that the original publication in this journal is cited, in accordance with accepted academic practice. No use, distribution or reproduction is permitted which does not comply with these terms.
*Correspondence: Sarah H. Creem-Regehr, sarah.creem@psych.utah.edu