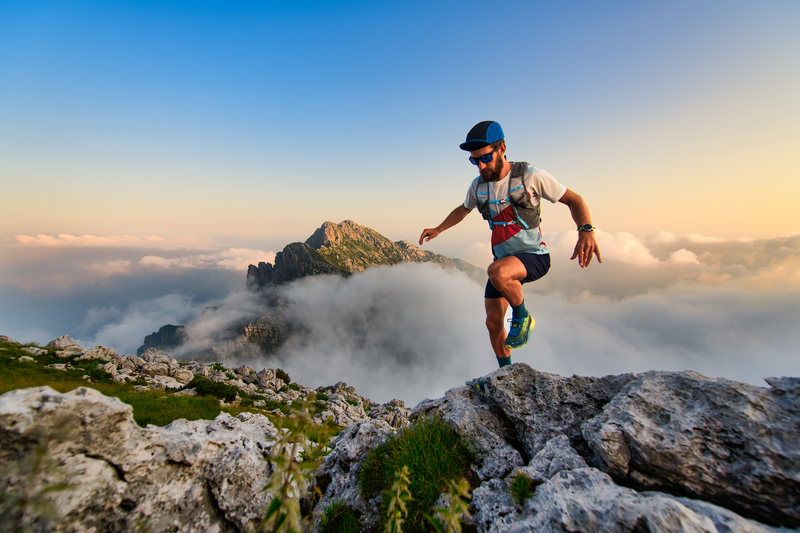
94% of researchers rate our articles as excellent or good
Learn more about the work of our research integrity team to safeguard the quality of each article we publish.
Find out more
ORIGINAL RESEARCH article
Front. Virtual Real. , 03 June 2022
Sec. Technologies for VR
Volume 3 - 2022 | https://doi.org/10.3389/frvir.2022.910379
This article is part of the Research Topic Electrical Stimulation for Immersive Virtual and Augmented Reality View all 5 articles
Haptic perception is a vital part of the human experience that enriches our engagement with the world, but the ability to provide haptic information in virtual reality (VR) environments is limited. Neurostimulation-based sensory feedback has the potential to enhance the immersive experience within VR environments by supplying relevant and intuitive haptic feedback related to interactions with virtual objects. Such feedback may contribute to an increase in the sense of presence and realism in VR and may contribute to the improvement of virtual reality simulations for future VR applications. This work developed and evaluated xTouch, a neuro-haptic platform that extends the sense of touch to virtual environments. xTouch is capable of tracking a user’s grasp and manipulation interactions with virtual objects and delivering haptic feedback based on the resulting grasp forces. Seven study participants received haptic feedback delivered via multi-channel transcutaneous electrical stimulation of the median nerve at the wrist to receive the haptic feedback. xTouch delivered different percept intensity profiles designed to emulate grasp forces during manipulation of objects of different sizes and compliance. The results of a virtual object classification task showed that the participants were able to use the active haptic feedback to discriminate the size and compliance of six virtual objects with success rates significantly better than the chance of guessing it correctly (63.9 ± 11.5%, chance = 16.7%, p < 0.001). We demonstrate that the platform can reliably convey interpretable information about the physical characteristics of virtual objects without the use of hand-mounted devices that would restrict finger mobility. Thus, by offering an immersive virtual experience, xTouch may facilitate a greater sense of belonging in virtual worlds.
The ability to navigate the tactile world we live in through haptic perception provides us with an experience that facilitates engagement with our surrounding environment. Haptic cues allow us to interpret physical properties of our surroundings and use the knowledge in unison with other senses to formulate interactive approaches with objects in our environment. We also use haptics to form a sense of connectedness with our physical environment, which includes other living organisms such as pets and plants as well as with other human beings. Social distancing conditions such as those imposed by a pandemic limit social haptics and interpersonal interactions. As we shift towards more virtual settings and remote operations (e.g., Metaverse (Dionisio et al., 2013; Mystakidis, 2022)), we risk losing the sense of connection we receive through touch. The lack of haptic feedback in the virtual reality (VR) environment prevents full interaction with the virtual environment which can have limiting effects on immersion and user connection (Price et al., 2021). Including haptic feedback in the VR environment may allow more meaningful interactions in the virtual world, which can have implications for stronger user engagement and task performance.
Typically, VR environments are limited to providing the user with an audio-visual experience. The addition of haptic feedback could enhance the sensorial experience by allowing users to navigate the virtual environment through the sense of touch (Preusche and Hirzinger, 2007). Haptic feedback has been delivered to users in a virtual environment through several types of haptic interfaces, with the most common being handheld and wearable devices. Handheld devices most commonly integrate vibrotactile actuators into handheld controllers to track and simulate hand movements. Handheld devices such the Haptic Revolver (Whitmire et al., 2018) provide users with the experience of touch, shear forces and motion in the virtual environment by using an interchangeable actuated wheel underneath the fingertip that spins and moves up and down to render various haptic sensations. Other handheld devices such as the CLAW (Choi et al., 2018) provide kinesthetic and cutaneous haptic feedback while grasping virtual objects. While functional and easy to mount, these devices occupy the hands and fail to consider the role our fingers play in our ability to dexterously manipulate and interact with objects in virtual environments.
On the other hand, wearable haptic systems (Pacchierotti et al., 2017; Yem and Kajimoto, 2017) provide feedback to the hand and fingertips through hand-mounted actuators. These technologies can take the form of exoskeletons (Hinchet et al., 2018; Wang et al., 2019) and fingertip devices (Maereg et al., 2017; Yem and Kajimoto, 2017) to provide cutaneous and/or kinesthetic feedback to the hands while allowing dexterous movement of the fingers. Wearable devices such as the Grabity (Choi et al., 2017) simulate the grasping and weight of virtual objects by providing vibrotactile feedback and weight force feedback during lifting. Maereg et al. developed a fingertip haptic device which allows users to discriminate stiffness during virtual interactions. While these devices may be cost-effective and wireless with good mounting stability, these wearable systems are often bulky, cumbersome and limit user mobility.
Consideration has also been given to the use of non-invasive sensory substitution techniques such as mechanical (Colella et al., 2019; Pezent et al., 2019) and electro-tactile (Hummel et al., 2016; Kourtesis et al., 2021; Vizcay et al., 2021) stimulation, which activate cutaneous receptors in the user’s skin, to convey information about touch and grasping actions in virtual environments. But these approaches are not intuitive, can restrict finger mobility if mounted on the hands or fingers, and often require remapping and learning due to percept modality and location mismatch. A non-restrictive wearable haptic system that is capable of providing relevant and intuitive feedback to facilitate user interaction with virtual environments or remote systems may address these shortcomings.
We explored the use of ExtendedTouch (xTouch), a novel Neuro-Haptics Feedback Platform, to enable meaningful interactions with virtual environments. xTouch utilizes transcutaneous electrical nerve stimulation (TENS), a non-invasive approach that targets the sensory pathways in peripheral nerves to evoke distally-referred sensations in the areas innervated by those nerves (Jones and Johnson, 2009). TENS has previously been combined with VR as an intervention for treating neuropathic pain (Preatoni et al., 2021) and as a tool to reduce spatial disorientation in a VR-based motion task for flying (Yang et al., 2012). Outside of VR applications, TENS of the peripheral nerves is frequently used in prostheses research to convey sensations from prosthetic hands about hand grasp force (D’Anna et al., 2017) and physical object characteristics (Vargas et al., 2020). D’Anna et al. showed that prosthesis users were able to feel distally-referred sensations in their phantom hand with TENS at the residual median and ulnar nerves and that they were able to use the information to perceive stimulation profiles designed to emulate the sensations felt during manipulation of objects with varying shapes and compliances. However, with their stimulation approach localized sensations in the region of the electrode were found to increase with increasing current, which can be distracting and irritable to the user, thereby restricting the discernability of distinct object characteristics. On the contrary, xTouch implements a novel stimulation strategy that was previously developed by Pena et al. (2021), which has been shown to elicit intuitive distally-referred tactile percepts without the localized discomfort associated with conventional transcutaneous stimulation methods (Li et al., 2017; Stephens-Fripp et al., 2018). With this approach, peripheral nerves can be activated by delivering electrical stimuli from surface electrodes placed proximal to the hand (i.e., the wrist). This means that haptic feedback can be provided to the hand and fingers without restrictive finger-mounted hardware, allowing for enhanced manipulation and movement.
In this work, we aimed to investigate the capabilities of xTouch to deliver interpretable information about the physical characteristics of virtual objects. xTouch integrates contactless motion tracking of the hand with a custom software program to calculate grasp forces and deliver haptic feedback related to interactions with virtual objects. The objective of this study was to evaluate the extent to which haptic feedback provided by this platform enables users to classify virtual objects by their size and compliance. We also evaluated the capability of the xTouch to provide a wide range of intensities users could perceive as they manipulated the object. We hypothesized that participants would be able to characterize the size and compliance of virtual objects and classify them with success rates significantly better than the chance of guessing them correctly. A haptic interface that could provide such information during virtual object interactions would have the potential to improve remote work engagement, telepresence and social haptics, and object manipulation during teleoperation.
Written informed consent was obtained from seven right-handed adult study participants (five males, two females, mean age ±SD: 24.6 ± 3.0) in compliance with the Institutional Review Board of Florida International University (FIU) which approved this study protocol. All prospective study participants were screened prior to the study to determine eligibility. All participants were able-bodied, with no sensory disorders or any self-reported condition listed as a contraindication for transcutaneous electrical stimulation (pregnancy, epilepsy, lymphedema, and or cardiac pacemaker) (Rennie, 2010).
An a priori power analysis was conducted on preliminary data obtained in a pilot study (Pena, 2020) to determine the effective sample size for this study at α = 0.05 and β = 0.2. The pilot study indicated that a sample size of four was sufficient to determine if subjects could identify the virtual objects at rates significantly better than chance (16.7%). To account for participant errors and potential censor data, seven participants were recruited.
The xTouch haptic feedback platform (Figure 1) has three functional blocks: contactless motion capture to track the hand as user’s interact with a virtual environment, a haptic rendering module to generate haptic feedback profiles based on calculated forces resulting from user interaction with virtual objects, and non-invasive peripheral nerve stimulation via surface electrodes on the wrist, to deliver the haptic feedback profiles (Jung and Pena, 2021; Pena et al., 2021).
FIGURE 1. xTouch: a neuro-haptic feedback platform for virtual object interaction. During virtual object exploration, measurements of hand aperture are used by a haptic rendering module with information from an object properties database to calculate forces resulting from user interaction. The haptic feedback profiles are mapped to determine parameters of the stimulation delivered by the neurostimulation system.
Contactless motion tracking was performed using a Leap Motion Controller (Ultraleap, Mountain View CA United States), a video-based hand-tracking unit. The hand aperture tracker was used to capture the movements of the right hand during the virtual object classification studies. The computational module consisted of a custom MATLAB® program (v2020b, MathWorks® Inc., Natick, MA) interfaced with the Leap software (Orion 3.2.1 SDK) and was used to process the tracking data and determine hand aperture distance by calculating the linear distance between the thumb pad and the average of the index, middle, and ring finger pad locations (Figure 2C). This distance was used to determine virtual object contact, compute the resulting interaction forces, and generate haptic feedback profiles matching the virtual interaction. The MATLAB® program was also interfaced with a custom stimulation control module, developed on the Synapse Software (version 96, Tucker-Davis Technologies (TDT), Alachua FL United States), and running on the TDT RZ5D base processor. This enabled real-time modulation of electrical stimulation parameters based on the haptic feedback profiles.
FIGURE 2. Schematics of the experimental setup for stimulation parameter fitting and virtual object classification task. (A) During the stimulation parameter fitting procedure, custom response buttons and a control knob were used. (B) During practice trials, the visual display was used to show changes in compression of the object as hand aperture changed. (C) The hand aperture tracker (Leap Motion Controller) was placed on the right side of the table.
Electrical stimulation was delivered transcutaneously through four small self-adhesive hydrogel electrodes (Rhythmlink International LLC, Columbia, SC) strategically located around the participant’s right wrist to activate their median nerve sensory fibers. Two small stimulating electrodes (15 × 20 mm) were placed on the ventral aspect of the wrist and two large return electrodes (20 × 25 mm) were placed on the opposite (dorsal) side. An optically isolated, multi-channel bio-stimulator (TDT IZ2-16H, Tucker-Davis Technologies, Alachua FL United States) was used to deliver charge-balanced, current-controlled biphasic rectangular pulses following the channel-hopping interleaved pulse scheduling (CHIPS) strategy (Pena et al., 2021). The interleaved current pulses delivered in CHIPS produce electric field profiles that activate underlying nerve fibers without activating those close to the surface of the skin, thus eliciting comfortable referred sensations in the hand while avoiding localized discomfort near the electrodes.
Study participants sat in a chair with their arms resting on a table facing a computer monitor used for displaying instructions (Figure 2). The right forearm rested on an elevated support pad with the hand resting on the ulnar side. Electrodes were placed around the right wrist using the approach described by Pena et al. (2021) to find the electrode locations that elicited distal percepts for each channel. Participants used custom response buttons to provide percept responses, and a control knob to explore different stimulation parameters during the stimulation fitting procedure. The hand aperture tracker was placed on the right side of table. A computer monitor was placed on the table to display task instructions.
A participant-controlled calibration routine was utilized to streamline the stimulation parameter fitting process. Strength-duration (SD) profiles were derived using the Lapicque-Weiss’s theoretical model (Weiss, 1901; Lapicque, 1909). After comfortable placement of the electrodes, pulse amplitude (PA) thresholds were obtained from all participants at five different pulse width (PW) values (300–700 µs, at 100 μs intervals) in randomized order (Forst et al., 2015). Participants interacted with a custom MATLAB® interface designed to control the delivery of electrical stimuli and collect the participant’s responses. The “Go” button was pressed to trigger the delivery of a pulse train with a constant 5 Hz pulse frequency (PF). To find the lowest possible current pulse amplitude that evoked a percept, perception threshold (PAth), participants adjusted the PA (from 0 to 3000 μA) using the custom control knob. Throughout this study, the stimulation PA was set to 50% above the percept threshold (1.5 × PAth) at a PW of 500 µs This duration was selected to allow for a wide range of PW to be used at this PA, as it lays beyond the nonlinear region of the SD profile.
A similar participant-controlled calibration routine was implemented to determine the lower and upper limits for the operating ranges of PW and PF at 1.5 × PAth using the custom control knob. First, stimulation was delivered at a fixed PF of 100 Hz while participants explored a wide range of PW (from 100 to 800 µs) to find the lowest possible level that evoked a reliable percept, and the highest possible level that did not cause discomfort. From pilot studies, 100 Hz was determined as a frequency that lied between the fusion and saturation points for most participants, therefore it was chosen as the test PF value so that participants did not feel pulsating stimulation and focused on the PW range. Next, the stimulation PW was set to the midpoint of the recently obtained PW range, and the participants used the knob to explore a wide range of PF (from 30 to 300 Hz) to find the lowest possible frequency that was not perceived as pulsating (fusion), and the level at which the perceived stimulation intensity did not change (saturation).
The activation charge rate (ACR) model (Graczyk et al., 2016) was used to predict the perceived intensity as a combination of PF and charge per pulse (Q). The ACR model was found to be a strong predictor for graded intensity perception during extraneural neurostimulation with cuff electrodes (Graczyk et al., 2016), and during pilot studies with transcutaneous neurostimulation. PW and PF were adjusted simultaneously along their operating ranges to modulate activation charge-rate and convey a wide range of graded percept intensities. For each participant, the pulse charge at perception threshold (Qth) was derived from their SD profile and was used with the ACR model (ACR=(Q − Qth) × PF where Q = PA × PW) to calculate the equivalent ACR range values that would result from each PF and PW adjustment.
Magnitude estimation tasks were performed to assess the span of evoked percept intensities perceived within the ACR range. This task followed a protocol consistent with the one used during the extraneural neurostimulation study with the ACR model (Graczyk et al., 2016). For each magnitude estimation trial, a 1-second-long stimulation burst was delivered, and the participant was asked to state a number that represented the perceived intensity or magnitude of the evoked sensation by comparing it with the previous burst. Each burst was calculated using the ACR model, while varying the PF and PW parameters over the ranges collected in the fitting procedure. An open-ended scale was used to allow relative comparison of the perceived strength levels. For example, if one stimulus felt twice or half as intense as the previous one, it could be given a score that is half or twice as large (Stevens, 1956; Banks and Coleman, 1981). A score of 0 was used when no sensation was perceived. All participants completed 3 experimental blocks, each consisting of 10 randomized trials. Ratings were normalized by dividing the values by the grand mean rating on their respective blocks.
Virtual object classification tasks were performed to test the ability to distinguish different percept intensity profiles designed to emulate grasping forces during manipulation of various objects of different physical characteristics. Six unique virtual object profiles were created from all possible combinations of 2 sizes (small, large) and 3 compliance levels (soft, medium, and hard) (Figure 3).
FIGURE 3. Virtual object profiles used during the classification task. Six profiles were created from combinations of two sizes and three compliance levels. The maximum compression distance of each object, which is dependent on the compliance of the object, is presented.
Virtual object grasping trials began with the participant’s right hand placed within the hand aperture tracking space as shown in Figure 2C, and open to an aperture greater than 100 mm. Once the hand was detected in place, participants were instructed to slowly close their hand until they began to perceive the stimulation, indicating they had contacted the virtual object (i.e., their hand aperture was equal or less than the uncompressed size of the virtual object). The participants explored the compressive range of the object by “squeezing” it and paying attention to how the resulting percept intensity changed (Figure 4). For instance, squeezing a hard object would ramp up the grasp force faster than a more compressible, softer object. Based on the compressive ranges shown in Figure 3, the rate of intensity changes for large-medium and large-soft objects were 3.7%/mm and 1.6%/mm respectively, while small-medium and small-soft objects had rates of 5.6%/mm and 2.4%/mm, respectively. For hard objects, the stimulation parameters were increased over 2 mm of compression, allowing for a rapid increase in percept intensity at a rate of 50%/mm. To indicate size, percepts were initiated at greater hand apertures for large objects relative to small objects. If the hand went beyond the object’s maximum compression limit, breakage of the object was emulated by cutting off stimulation. Object exploration after breakage restarted when the hand reopened to an aperture greater than 100 mm. For all virtual grasp trials, participants were blindfolded to prevent the use of visual feedback of hand aperture. A 60 s limit was enforced for each grasp trial, with no restrictions on the number of times the hand could be opened and closed. Participants then verbally reported the perceived size and compliance of the virtual object. For example, if the perceived object was a small and hard object, they would state, “small and hard”.
FIGURE 4. Hand aperture tracking from the start (T0) to the end (T4) of a virtual object exploration attempt. The hand aperture (red dashed trace; left y-axis) was tracked during exploration to determine when the hand made contact with the object (T2) up to the maximum compression of the object (T3). The hand aperture data was used to estimate the resulting grasping force as the user interacted with the object (blue solid trace; right y-axis). The full compressive range of the virtual object (shaded region) was linearly mapped to the full range of stimulation intensities.
A practice block was presented at the onset of the experiment to introduce and allow participants to become familiar with each unique profile. Participants were instructed to pay attention to the hand aperture at which the percept began and the rate at which the intensity increased, indicating that contact was made with the object and the compliance of the object, respectively. The practice block included a 2D virtual interactive display as shown in Figure 2B, to provide participants with a visualization of the object during exploration. The purpose of this practice phase was to induce learning so that the participants felt confident enough to be able to identify the profiles without visual feedback. There were no time constraints for exploration or limits to the number of presentations of the objects in this phase. Participants then completed 2 experimental blocks of 18 non-repeating, randomized grasp trials each (6 repetitions per profile), resulting in a total of 36 double-blinded presentations. The virtual object profile that was presented was compared to the participant’s response for each trial. The performance measure was the frequency of correct responses. Throughout the experiment, they were encouraged to stretch during breaks and frequently asked about their comfort levels or if additional breaks were needed after each task to prevent discomfort.
One-sample t-tests at significance level 0.05 were performed to determine if the success rate of identifying the virtual object profile was significantly greater than chance. The chance of correctly identifying the object size or compliance alone was 50 and 33.3% respectively, while the chance of correctly identifying size and compliance together was 16.7%. The perceived intensity as a function of charge-rate is presented for all participants. A post-hoc test was conducted to assess the statistical power achieved.
For all seven participants, comfortable distally referred sensations were evoked in the general areas of the hand innervated by the sensory fibers in the median nerve (palmar surface, index, middle, and part of the ring finger). The participant-controlled calibration routine allowed participants to select appropriate stimulation amplitude levels and operating ranges for PW and PF. No local sensations or side effects like irritation or redness of the skin were observed in any of the participants.
Participants reported an operating range for PW spanning from (mean ± SD) 360 ± 70 µs to 550 ± 100 µs, while the operating range for PF spanned from 73.9 ± 35.1 Hz (fusion) to 213.6 ± 65.1 Hz (saturation). Intensity ratings given by the participants were normalized to the grand mean of their respective blocks for comparison. Figure 5 shows the normalized perceived intensity range as a function of the ACR range used for each participant.
FIGURE 5. A wide range of intensities were perceived by all participants. The individual markers represent the percept intensities reported by each participant across the chosen ACR range, normalized to the grand mean rating of their respective blocks. The solid blue line and blue shade represent the average of the normalized perceived intensities and SEM respectively, across all participants.
Participants were able to integrate percept intensity information delivered by xTouch as they grasped virtual objects (Figure 6A) to successfully determine their size and compliance, (Figure 6B). Most participants spent an average of 8 min exploring and learning cues for the different virtual objects during the practice block. The most one participant spent in this block was 16 min. During an experimental session, each of six virtual object profiles were presented six times, for 36 double-blinded presentations. Participants were able to differentiate between large and small objects with an average success rate (mean ± SD) of 93.7 ± 7.6%, p < 0.001. Participants successfully classified virtual objects by their compliance with success rates significantly greater than chance for large objects (70.6 ± 10.0%, p < 0.001) and small objects (67.5 ± 14.9%, p < 0.001). Participants were most successful in identifying Small-Hard and Large-Hard objects (Figure 6B).
FIGURE 6. Feedback of grasp force profiles enables identification of virtual object size and compliance. (A) Example of hand aperture (blue) and virtual grasp force profile (red) traces when grasping a Small-Hard object (left) and a Large-Soft object (right). The shaded regions represent the compressive range of the object. (B) Confusion matrix quantifying the perceived size and compliance combined (left-right), in relation with the presented profile (up-down) across 36 trials per participant. Each block represents the frequency of responses provided by all participants when they were presented with a profile (actual) and classified it (perceived). The first letter indicates the size (small/large) and the second letter indicates the compliance (soft/medium/hard). Successful identification of both size and compliance (diagonal) by chance is 16.7%.
On average, participants were more successful in classifying some virtual object characteristics more frequently than other characteristics. Participants were most successful in identifying hard objects (88.1 ± 13.5%) and small objects (98.4 ± 4.2%) (Figure 7). Overall participants identified both the size and compliance of objects together with success rates significantly better than chance (63.9 ± 11.5%, p < 0.001).
FIGURE 7. Frequency of classifying objects by their size independent of compliance or compliance independent of size. All classification combinations of size and compliance were assessed, with 4 and 9 possible combinations of classification in size (left) and compliance (right), respectively. Successful identification of size or compliance by chance are 50% and 33%, respectively.
The response rates for identifying both size and compliance of the virtual object correctly were utilized to perform a post-hoc power analysis. Results of the analysis indicated that the effect size (d = 4.11) is large, giving a low Type II error (β < 0.01) and high statistical power. This suggests that the sample size of seven participants is sufficient to detect an effect.
This study sought to evaluate the extent to which a novel neuro-haptic feedback platform enables users to classify virtual objects by their size and compliance. Study participants received relevant and intuitive haptic feedback based on virtual grasping actions, facilitated by a novel stimulation strategy that evoked comfortable sensations in the hand, with a wide range of graded intensities. This study demonstrates that this platform can facilitate feedback with virtual objects that are interpretable by users. The integration of xTouch with the visual and auditory feedback provided through current VR systems can improve the realism of the simulation, which can enhance immersion and user experience in the simulation.
Similar to the reports in Pena et al., 2021, participants subjectively reported sensations in the hand regions innervated by the median nerve. To ensure congruency, the electrodes were adjusted as needed at the beginning of the experiment until the reported percept areas included the fingertips. After the participant-controlled calibration procedures, a magnitude estimation task was performed to identify the perceived ranges of intensity. Modulation of charge-rate was accomplished along the participant-calibrated operating ranges by adjusting pulse width and pulse frequency simultaneously. Generally, participants perceived greater intensities with increasing ACR (Figure 5), showing that several discriminable levels of intensities were perceivable within the ACR range. These different levels of intensity are also likely to be usable for conveying information about different object characteristics. Therefore, the full compressive range of each unique virtual object was linearly mapped to the full range of percept intensities.
The grasping action performed while exploring the object’s characteristics was guided by the feedback received from xTouch. The object’s full compressive range contained the full range of percept intensities, which encoded the size and compliance of different virtual objects by the intensity of the percepts during changes in the grasping action. When exploring the full compressive range of a soft or medium compliance object, participants typically reversed course and began to open the hand (Figure 6A), suggesting that they perceived the object as fully compressed. Participants were allowed to squeeze the virtual object as many times as needed to identify the perceived size and compliance.
Participants successfully recognized virtual objects by their size and compliance combined about 64% of the time, indicating that they were able to distinguish the virtual object profiles through the haptic feedback they received. Object compliance was recognized correctly in about 70% of the trials using the xTouch. These results are better than those obtained to correctly classify objects of three compliances (60% accuracy) using traditional TENS (D’Anna et al., 2017) or to classify objects with four compliances (60% accuracy) when using vibrotactile feedback (Witteveen et al., 2014). Our results suggest that the xTouch system can provide interpretable information on the compliance of virtual objects which users can accurately identify better than other haptic feedback methods.
To achieve the best precision with the Leap Motion Controller, routine maintenance was performed to ensure the sensor was properly calibrated and that the sensor surface was clean. The Leap software version used (Orion 3.2.1 SDK) featured improved hand reconstruction and tracking stability over previous versions. To further reduce tracking error, participants were instructed to close the hand slowly while keeping the hand at a fixed distance from the sensor. Whether hand-closing speed may have played a role in classification performance is unclear. During preliminary performance testing, we observed end-point tracking errors that were <5 mm under more dynamic conditions (worst-case) than the conditions tested in this study.
The highest percentages of misclassification were due to incorrect perception of compliance. The differences in object compliance were determined by several factors, including the compression distances and the rate at which the intensity ramped up during grasping. While each compliance profile consisted of unique characteristics, it was frequently reported that the soft and medium compliant objects were the most challenging to differentiate between. Of the soft compliant objects presented, 27.4% were misidentified as medium compliance and 34.5% of the medium compliant objects were misidentified as soft (Figure 7), making up the highest percentages of misidentification. This may be due to the perceived similarity in sensation intensity provided at the beginning of the stimulus. Participants often reported that hard objects were significantly easier to identify due to the high intensity felt immediately after contacting the object. However, the soft and medium compliant objects felt similar upon contact to them, and hence they required more time and exploration to provide a response. Perhaps an approach where the intensity at contact for each compliance is noticeably different would make it easier to differentiate between the two compliances. Fewer objects were misclassified by size. Of the large objects presented, 11.1% were perceived as small objects (Figure 7). This may be due to a sequencing order effect on perception, as these misidentifications of size for large objects mostly occurred when a large-hard or large-medium object was presented in the trial before.
Additionally, all participants performed a practice block where they were introduced to the six unique profiles and provided cues to assist in identifying the physical characteristics. It was observed during the experiment that one participant was only reporting objects of two compliances—soft and hard. After the block, the participant was reminded that there were 6 object profiles to differentiate between; here the participant reported that they were not aware that there were objects with medium compliance. This error may have contributed to the lower success rates in identifying objects of medium compliance.
The magnitude estimation performance could have been masked by potentially narrow pulse frequency operating ranges due to the fusion-saturation limits. Other studies have shown that participants often use individual pulse timings as supplementary cues. Because of this, frequency discrimination performance is often better with low frequency references (George et al., 2020). Since the intent of the study was to pay attention to the perceived intensity and not the frequency, participants were instructed to pick the point where individual pulses were no longer detectable (fusion) as the lower end of the operational frequency range. This may have increased the difficulty of the task by avoiding the presence of low frequency references and test bursts during the magnitude estimation trials.
In this study we focused on percept intensity only. Percept modality is also an important dimension of artificial feedback. While intensity is encoded by rate and population recruitment, modality seems to be encoded by the spatiotemporal patterning of this activity (Tan et al., 2014). Sensations delivered by conventional surface stimulation methods have often been reported as artificial or unnatural electrical tingling, or paresthesia. Synchronous activation within a population of different fibers is believed to cause these sensations (Ochoa and Torebjork, 1980; Mogyoros et al., 1996), which contrast with the more complex spatiotemporal patterns recognized during natural sensory perception (Weber et al., 2013). Future advancements to xTouch could incorporate ability to study percept modalities contribution to more intuitive and natural sensations.
When grasping objects, there is often a physical restraint on the distance our hands can close upon contact. This resistance provides haptic feedback that restrains us from going beyond the compression limits of objects, thereby preventing breakage. With virtual objects, there is no physical resistance at the fingertips and therefore, nothing preventing participants from closing their hands beyond the compressive range of each object. Taking this into account, the experience of breakage was emulated by stopping stimulation when the hand closed beyond the maximum compressive range for respective objects. In some instances, participants reported a response after breaking the object, which was most notably used when classifying hard objects, despite size. However, the participants were strongly discouraged from using this tactic. Perhaps a method incorporating physical resistance such as in augmented reality applications could be explored to prevent participants from using similar tactics.
In the interest of time, the just noticeable difference (JND) was not evaluated. Instead, we relied on results from a series of intensity discrimination tasks during a previous study (Pena, 2020) with ten participants which suggested that intensity mapping along the ACR range could potentially allow for an average of ten noticeably different intensity levels. The full compressive range of each unique virtual object was linearly mapped to the full range of percept intensities, which are achieved by simultaneously modulating PW and PF along the ACR range. Prior to performing the virtual object classification task, participants completed multiple magnitude estimation trials over ten different ACR levels equally distributed along the full ACR range. The magnitude estimation tasks showed that participants perceived a wide range of intensities within their fitted ACR range. However, whether participants could reliably discriminate between adjacent levels was not determined.
To reliably classify virtual objects with complex characteristics, xTouch must be able to convey detectable changes with a high enough intensity resolution. Assuming that we could achieve ten noticeably different intensity levels with this approach, a participant would need to change their aperture by more than 10% of the object’s compressive range as depicted in Figure 3 to experience a distinguishably different percept intensity. For instance, large-soft objects would require the most change in aperture (>6.3 mm) to produce a detectable change in intensity based on its 63 mm compressive range. The relatively coarse intensity change makes these objects less sensitive to aperture tracking errors. In contrast, a small-medium object would require the least change in aperture (>1.8 mm) making it the most sensitive to aperture tracking errors. The ability to convey multiple levels of discriminable intensities is not as critical for hard objects as it is for more compressible objects. Future work should include JND determination tasks to determine the smallest detectable increments in intensities that can be used. The effect of tracking accuracy on the JND should also be explored for different tracking approaches.
Additionally, participants had the opportunity to explore the object as many times as needed before providing a response. If the object was grasped beyond the compressive range and breakage was initiated, participants were able to restart exploration by opening the hand to a distance greater than the objects uncompressed width (>100 mm). This may not be representative of typical daily life activities, where reaching force targets with one attempt is often required. Future studies could use a single attempt method in which participants are instructed to approach the target from one direction and stop once they feel they have reached compressive bounds.
Throughout the study participants were encouraged to take breaks and stretch when needed to mitigate discomfort. However, it was not considered whether stretching could have affected the perceived intensity ranges. Ultrasound studies have shown that stretching the wrist can cause displacement of the median nerve (Nakamichi and Tachibana, 1992; Martínez-Payá et al., 2015). Previous studies with transcutaneous stimulation (Forst et al., 2015; Shin et al., 2018) at the arm suggest that some degree of position dependence exists and influences the perceived sensations, suggesting that potential displacement of the nerve does affect the perceived sensations. A potential solution to this problem is the adoption of an electrode array design [patent pending]. The haptic-feedback platform has the potential to utilize an array design which can create unique touch experiences to better match the environment. The array design may mitigate substantial changes in stimulation due to movements of the nerve, as different electrode locations within the array may activate similar populations of the nerve. Additionally, the information delivered by the feedback system could be further expanded by implementing multi-channel stimulation schemes where multiple electrode pairs target different parts of the nerve to evoke percepts in different areas of the hand. Potential combinations of electrode pairs within the array can be used to simultaneously stimulate percept locations, opening the opportunity to elicit whole hand sensations (Shin et al., 2018). These enhanced percepts could be used to replicate a variety of interactions with different types of objects, providing more realistic cues that go beyond size and compliance (Saal and Bensmaia, 2015). Development of this platform in an array form may also be used to better replicate complex interactions of event cues such as object slippage, thus enabling users to execute virtual or remote manipulation tasks with high precision.
VR is often characterized by visual dominance and previous work has shown that sense of agency in VR can be altered when the visual feedback provided is incongruent with the participant’s actual movements (Salomon et al., 2016). However, more recent work challenges this visual dominance theory with haptic feedback manipulation during multisensory conflicts in VR (Boban et al., 2022). Boban et al. found that active haptic feedback paired with congruent proprioceptive and motor afferent signals and incongruent visual feedback can reduce visual perception accuracy in a changing finger movement task in VR. When subjects were provided haptic feedback inconsistent with the finger they saw moving, they were more uncertain about which finger they saw moving, thereby challenging the visual-dominance theory. This phenomenon could be examined using the xTouch system in the future. Here, we understand how the information from the xTouch system can be utilized in isolation. Hence, the haptic feedback was provided by the xTouch system without confounding effects of visual feedback of hand aperture, which may have given away the size of the object. These data lay the groundwork for investigating the use of xTouch in more complex situations such as in the presence of other forms of feedback.
In this study, the electrical stimulation provided by the xTouch platform is driven by a benchtop stimulation system and controlled by the experiment computer. This imposes obvious mobility constraints on the user. Recent advances in portable neuromodulation technology could enable the development of a portable xTouch system for real-world VR/AR applications capable of delivering neuro-haptic feedback without restricting user mobility. The types of electronics needed to stimulate peripheral nerves have become much smaller in recent years and have demonstrated safety and reliability in widely used commercial systems. For example, commercially available TENS units are portable battery-powered devices that are slightly larger than most smartphones. These devices can deliver enough current to safely evoke muscle contractions (between 10 and 30 mA depending on the application). For comparison, the amount of current used to elicit the kinds of sensations provided by xTouch were no higher than 6 mA and therefore compatible with a portable smaller form-factor stimulator design.
A desired feature of VR experiences is interaction transparency. This is when users cannot distinguish between operating in a local (or real) environment, and a distant (or virtual) environment (Preusche and Hirzinger, 2007). While it was out of the scope of this study to evaluate immersion and realism, future studies should investigate how haptic feedback affects realism and presence in VR simulations. It has been shown that the quality of realism of virtual environments promotes a greater sense of presence in VR (Hvass et al., 2018; Newman et al., 2022). A previous study (Huffman et al., 1998) investigated how realism of a virtual environment was influenced by tactile augmentation and found that the group that received tactile feedback was likely to predict more realistic physical properties of other objects in the virtual environment. This shows that presenting stimuli that match real physical characteristics will lead users’ expectations in the environment to be congruent with their expectation of reality, which in turn may induce users to interact, perform and interpret virtual interactions more like how they would in real environments. In other words, providing users with stimuli similar in quality and experience to real environments has the potential to enhance realism of VR simulations. Perhaps the addition of xTouch can also enhance the user’s perception of the environment, creating a more realistic experience for the user and greater sense of presence, ultimately promoting interaction transparency.
This work evaluated the use of a novel neuro-haptics feedback platform that integrates contactless hand tracking and non-invasive electrical neurostimulation to provide relevant and intuitive haptic information related to user interactions with virtual objects. This approach was used to simulate object manipulation cues such as object contact, grasping forces, and breakage during a virtual object classification task, without restrictive hand-mounted hardware.
VR has been used in a variety of research applications and has been growing in interest to live up to our ideas of existing in a virtual world, such as in the Metaverse (Dionisio et al., 2013; Mystakidis, 2022). With the translatable use of virtual reality across multiple sectors (i.e. gaming, telehealth, and defense, etc.), this haptic interface has the potential for use in a variety of research and social applications. For example, the presented platform can be used to enhance immersion of simulated environments in gaming applications, facilitate stronger interactions with rehabilitative tasks in telehealth applications, advance non-invasive applications of neuroprosthetics, and allow haptic communication with other users in collaborative virtual environments. Since the hands of the user are not restrained by the wearable device, xTouch has potential uses within augmented reality applications, allowing the user to experience augmented virtual sensations while freely manipulating physical objects.
Intuitive sensory feedback is crucial to enhancing the experience of virtual reality. This study demonstrates that this platform can be used to facilitate meaningful interactions with virtual environments. While additional studies are required to investigate whether additional sensory channels can be added (e.g., delivering proprioceptive feedback to the ulnar nerve), this study demonstrated that the artificial sensory feedback delivered by xTouch may enable individuals to execute virtual or remote manipulation tasks with high precision without relying solely on visual or auditory cues. In future work, we will consider the neural mechanisms by which we interpret touch in VR and how the interactive engagement with objects in VR affects realism, immersion, haptic perception, and user performance. This may have implications for the benefits of xTouch as an addition to VR and provide insight into the mechanisms our brains use to translate haptic perception in virtual reality simulations. Identifying xTouch’s capability to provide interpretable haptic information in virtual environments may potentially improve virtual reality simulations for future research and industry applications.
The raw data supporting the conclusion of this article will be made available by the authors, without undue reservation.
The studies involving human participants were reviewed and approved by Institutional Review Board of Florida International University. The patients/participants provided their written informed consent to participate in this study.
Development of xTouch, AP. Data collection and analyses, AS Writing—original draft preparation, AS. Figures, AP and AS. Writing, figure review and editing, AS, AP, JA, and RJ. Supervision, JA and RJ. All authors have read and agreed to the published version of the manuscript.
This work was funded by the FIU Wallace H Coulter Eminent Scholars Endowment to RJ. AP and AS were supported in part by the Institute for Integrative and Innovative Research (I3R). AS was supported by the Florida Education Fund McKnight Doctoral Fellowship, the I3R Graduate Research Assistantship, and the Paul K. Kuroda Endowed Graduate Fellowship in Engineering.
The authors declare that the research was conducted in the absence of any commercial or financial relationships that could be construed as a potential conflict of interest.
All claims expressed in this article are solely those of the authors and do not necessarily represent those of their affiliated organizations, or those of the publisher, the editors and the reviewers. Any product that may be evaluated in this article, or claim that may be made by its manufacturer, is not guaranteed or endorsed by the publisher.
We thank Heriberto A. Nieves for characterizing the hand aperture tracker and conducting pilot studies with the xTouch. In addition, we thank Sathyakumar S. Kuntaegowdanahalli for his help in conducting the experiments.
Banks, W. P., and Coleman, M. J. (1981). Two Subjective Scales of Number. Percept. Psychophys. 29, 95–105. doi:10.3758/BF03207272
Boban, L., Pittet, D., Herbelin, B., and Boulic, R. (2022). Changing Finger Movement Perception: Influence of Active Haptics on Visual Dominance. Front. Virtual Real. 3. doi:10.3389/frvir.2022.860872
Choi, I., Culbertson, H., Miller, M. R., Olwal, A., and Follmer, S. (2017). “Grabity,” in Proceedings of the 30th Annual ACM Symposium on User Interface Software and Technology. UIST 2017. doi:10.1145/3126594.3126599
Choi, I., Ofek, E., Benko, H., Sinclair, M., and Holz, C. (2018). “Claw,” in Conference on Human Factors in Computing Systems - Proceedings. doi:10.1145/3173574.3174228
Colella, N., Bianchi, M., Grioli, G., Bicchi, A., and Catalano, M. G. (2019). A Novel Skin-Stretch Haptic Device for Intuitive Control of Robotic Prostheses and Avatars. IEEE Robot. Autom. Lett. 4, 1572–1579. doi:10.1109/LRA.2019.2896484
D’Anna, E., Petrini, F. M., Artoni, F., Popovic, I., Simanić, I., Raspopovic, S., et al. (2017). A Somatotopic Bidirectional Hand Prosthesis with Transcutaneous Electrical Nerve Stimulation Based Sensory Feedback. Sci. Rep. 7. doi:10.1038/s41598-017-11306-w
Dionisio, J. D. N., Iii, W. G. B., and Gilbert, R. (2013). 3D Virtual Worlds and the Metaverse. ACM Comput. Surv. 45, 1–38. doi:10.1145/2480741.2480751
Forst, J. C., Blok, D. C., Slopsema, J. P., Boss, J. M., Heyboer, L. A., Tobias, C. M., et al. (2015). Surface Electrical Stimulation to Evoke Referred Sensation. J. Rehabil. Res. Dev. 52. doi:10.1682/JRRD.2014.05.0128
George, J. A., Brinton, M. R., Colgan, P. C., Colvin, G. K., Bensmaia, S. J., and Clark, G. A. (2020). Intensity Discriminability of Electrocutaneous and Intraneural Stimulation Pulse Frequency in Intact Individuals and Amputees. Annu. Int. Conf. IEEE Eng. Med. Biol. Soc. 2020, 3893–3896. doi:10.1109/EMBC44109.2020.9176720
Graczyk, E. L., Schiefer, M. A., Saal, H. P., Delhaye, B. P., Bensmaia, S. J., and Tyler, D. J. (2016). The Neural Basis of Perceived Intensity in Natural and Artificial Touch. Sci. Transl. Med. 8. doi:10.1126/scitranslmed.aaf5187
Hinchet, R., Vechev, V., Shea, H., and Hilliges, O. (2018). “DextrES,” in Proceedings of the 31st Annual ACM Symposium on User Interface Software and Technology. UIST 2018. doi:10.1145/3242587.3242657
Hoffman, H. G., Hollander, A., Schroder, K., Rousseau, S., and Furness, T. (1998). Physically Touching and Tasting Virtual Objects Enhances the Realism of Virtual Experiences. Virtual Real. 3, 226–234. doi:10.1007/bf01408703
Hummel, J., Dodiya, J., Center, G. A., Eckardt, L., Wolff, R., Gerndt, A., et al. (2016). “A Lightweight Electrotactile Feedback Device for Grasp Improvement in Immersive Virtual Environments,” in Proceedings - IEEE Virtual Reality. doi:10.1109/VR.2016.7504686
Hvass, J., Larsen, O., Vendelbo, K., Nilsson, N., Nordahl, R., and Serafin, S. (2017). “Visual Realism and Presence in a Virtual Reality Game,” in Proceedings of the 3DTV-Conference. doi:10.1109/3DTV.2017.8280421
Jones, I., and Johnson, M. I. (2009). Transcutaneous Electrical Nerve Stimulation. Continuing Educ. Anaesth. Crit. Care & Pain 9, 130–135. doi:10.1093/BJACEACCP/MKP021
Jung, R., and Pena, A. E. (2021). Systems and Methods for Providing Haptic Feedback when Interacting with Virtual Objects. 199903–b1. U.S. Patent 11
Kourtesis, P., Argelaguet, F., Vizcay, S., Marchal, M., and Pacchierotti, C. (2021). Electrotactile Feedback for Hand Interactions:A Systematic Review, Meta-Analysis,and Future Directions. doi:10.48550/arxiv.2105.05343
Li, K., Fang, Y., Zhou, Y., and Liu, H. (2017). Non-Invasive Stimulation-Based Tactile Sensation for Upper-Extremity Prosthesis: A Review. IEEE Sensors J. 17, 2625–2635. doi:10.1109/JSEN.2017.2674965
Maereg, A. T., Nagar, A., Reid, D., and Secco, E. L. (2017). Wearable Vibrotactile Haptic Device for Stiffness Discrimination during Virtual Interactions. Front. Robot. AI 4. doi:10.3389/frobt.2017.00042
Martínez-Payá, J. J., Ríos-Díaz, J., del Baño-Aledo, M. E., García-Martínez, D., de Groot-Ferrando, A., and Meroño-Gallut, J. (2015). Biomechanics of the Median Nerve during Stretching as Assessed by Ultrasonography. J. Appl. Biomechanics 31, 439–444. doi:10.1123/jab.2015-0026
Mogyoros, I., Kiernan, M. C., and Burke, D. (1996). Strength-duration Properties of Human Peripheral Nerve. Brain 119, 439–447. doi:10.1093/brain/119.2.439
Nakamichi, K., and Tachibana, S. (1992). Transverse Sliding of the Median Nerve beneath the Flexor Retinaculum. J. Hand Surg. Br. 17, 213–216. doi:10.1016/0266-7681(92)90092-G
Newman, M., Gatersleben, B., Wyles, K. J., and Ratcliffe, E. (2022). The Use of Virtual Reality in Environment Experiences and the Importance of Realism. J. Environ. Psychol. 79, 101733. doi:10.1016/j.jenvp.2021.101733
Ochoa, J. L., and Torejök, H. E. (1980). Paræsthesiæ from Ectopic Impulse Generation in Human Sensory Nerves. Brain 103, 835–853. doi:10.1093/brain/103.4.835
Pacchierotti, C., Sinclair, S., Solazzi, M., Frisoli, A., Hayward, V., and Prattichizzo, D. (2017). Wearable Haptic Systems for the Fingertip and the Hand: Taxonomy, Review, and Perspectives. IEEE Trans. Haptics 10, 580–600. doi:10.1109/TOH.2017.2689006
Pena, A. E., Abbas, J. J., and Jung, R. (2021). Channel-hopping during Surface Electrical Neurostimulation Elicits Selective, Comfortable, Distally Referred Sensations. J. Neural Eng. 18, 055004. doi:10.1088/1741-2552/abf28c
Pena, A. E. (2020). Enhanced Surface Electrical Neurostimulation (eSENS): A Non-invasive Platform for Peripheral Neuromodulation. Dissertation. Florida International University.
Pezent, E., Israr, A., Samad, M., Robinson, S., Agarwal, P., Benko, H., et al. (2019). “Tasbi: Multisensory Squeeze and Vibrotactile Wrist Haptics for Augmented and Virtual Reality”, in Proceeding of the IEEE World Haptics Conference(WHC). doi:10.1109/WHC.2019.8816098
Preatoni, G., Bracher, N. M., and Raspopovic, S. (2021). “Towards a Future VR-TENS Multimodal Platform to Treat Neuropathic Pain,” in Proceeding of the International IEEE/EMBS Conference on Neural Engineering(NER). doi:10.1109/NER49283.2021.9441283
Preusche, C., and Hirzinger, G. (2007). Haptics in Telerobotics. Vis. Comput. 23. 273–284. doi:10.1007/s00371-007-0101-3
Price, S., Jewitt, C., Chubinidze, D., Barker, N., and Yiannoutsou, N. (2021). Taking an Extended Embodied Perspective of Touch: Connection-Disconnection in iVR. Front. Virtual Real. 2. doi:10.3389/frvir.2021.642782
Rennie, S. (2010). ELECTROPHYSICAL AGENTS - Contraindications and Precautions: An Evidence-Based Approach to Clinical Decision Making in Physical Therapy. Physiother. Can. 62, 1–80. Physiotherapy Canada. doi:10.3138/ptc.62.5
Saal, H. P., and Bensmaia, S. J. (2015). Biomimetic Approaches to Bionic Touch through a Peripheral Nerve Interface. Neuropsychologia 79, 344–353. doi:10.1016/j.neuropsychologia.2015.06.010
Salomon, R., Fernandez, N. B., van Elk, M., Vachicouras, N., Sabatier, F., Tychinskaya, A., et al. (2016). Changing Motor Perception by Sensorimotor Conflicts and Body Ownership. Sci. Rep. 6. doi:10.1038/srep25847
Shin, H., Watkins, Z., Huang, H., Zhu, Y., and Hu, X. (2018). Evoked Haptic Sensations in the Hand via Non-invasive Proximal Nerve Stimulation. J. Neural Eng. 15, 046005. doi:10.1088/1741-2552/aabd5d
Stephens-Fripp, B., Alici, G., and Mutlu, R. (2018). A Review of Non-invasive Sensory Feedback Methods for Transradial Prosthetic Hands. IEEE Access 6, 6878–6899. doi:10.1109/ACCESS.2018.2791583
Stevens, S. S. (1956). The Direct Estimation of Sensory Magnitudes: Loudness. Am. J. Psychol. 69, 1. doi:10.2307/1418112
Tan, D. W., Schiefer, M. A., Keith, M. W., Anderson, J. R., Tyler, J., and Tyler, D. J. (2014). A Neural Interface Provides Long-Term Stable Natural Touch Perception. Sci. Transl. Med. 6. doi:10.1126/scitranslmed.3008669
Vargas, L., Shin, H., Huang, H., Zhu, Y., and Hu, X. (2020). Object Stiffness Recognition Using Haptic Feedback Delivered Through Transcutaneous Proximal Nerve Stimulation. J. Neural Eng.. doi:10.1088/1741-2552/ab4d99
Vizcay, S., Kourtesis, P., Argelaguet, F., Pacchierotti, C., and Marchal, M. (2021). Electrotactile Feedback for Enhancing Contact Information in Virtual Reality. arXiv preprint arXiv:2102.00259.
Wang, D., Guo, Y., Liu, S., Zhang, Y., Xu, W., and Xiao, J. (2019). Haptic Display for Virtual Reality: Progress and Challenges. Virtual Real. Intelligent Hardw. 1, 136–162. doi:10.3724/SP.J.2096-5796.2019.0008
Weber, A. I., Saal, H. P., Lieber, J. D., Cheng, J.-W., Manfredi, L. R., Dammann, J. F., et al. (2013). Spatial and Temporal Codes Mediate the Tactile Perception of Natural Textures. Proc. Natl. Acad. Sci. U.S.A. 110, 17107–17112. doi:10.1073/pnas.1305509110
Weiss, G. (1901). Sur la possibilité de rendre comparable entre eux les appareils servant à l’excitation électrique. Arch. Ital. Biol. 35 (1), 413–445. doi:10.4449/AIB.V35I1.1355
Whitmire, E., Benko, H., Holz, C., Ofek, E., and Sinclair, M. (2018). “Haptic Revolver,” in Proceeding of the Conference on Human Factors in Computing Systems - Proceedings. doi:10.1145/3173574.3173660
Witteveen, H. J. B., Luft, F., Rietman, J. S., and Veltink, P. H. (2014). Stiffness Feedback for Myoelectric Forearm Prostheses Using Vibrotactile Stimulation. IEEE Trans. Neural Syst. Rehabil. Eng. 22, 53–61. doi:10.1109/TNSRE.2013.2267394
Yang, S.-R., Shi-An Chen, S-A., Shu-Fang Tsai, S-F., and Lin, C.-T. (2012). “Transcutaneous Electrical Nerve Stimulation System for Improvement of Flight Orientation in a VR-Based Motion Environment,” in ISCAS 2012 - 2012 IEEE International Symposium on Circuits and Systems. doi:10.1109/ISCAS.2012.6271686
Keywords: non-invasive electrical stimulation, peripheral nerve stimulation, transcutaneous electrical stimulation, haptic feedback, neuromodulation, virtual interaction, neuro-haptics
Citation: Shell AK, Pena AE, Abbas JJ and Jung R (2022) Novel Neurostimulation-Based Haptic Feedback Platform for Grasp Interactions With Virtual Objects. Front. Virtual Real. 3:910379. doi: 10.3389/frvir.2022.910379
Received: 01 April 2022; Accepted: 19 May 2022;
Published: 03 June 2022.
Edited by:
Pedro Lopes, The University of Chicago, United StatesReviewed by:
Florian Daiber, German Research Center for Artificial Intelligence (DFKI), GermanyCopyright © 2022 Shell, Pena, Abbas and Jung. This is an open-access article distributed under the terms of the Creative Commons Attribution License (CC BY). The use, distribution or reproduction in other forums is permitted, provided the original author(s) and the copyright owner(s) are credited and that the original publication in this journal is cited, in accordance with accepted academic practice. No use, distribution or reproduction is permitted which does not comply with these terms.
*Correspondence: Andres E. Pena, YW5kcmVzcEB1YXJrLmVkdQ==
Disclaimer: All claims expressed in this article are solely those of the authors and do not necessarily represent those of their affiliated organizations, or those of the publisher, the editors and the reviewers. Any product that may be evaluated in this article or claim that may be made by its manufacturer is not guaranteed or endorsed by the publisher.
Research integrity at Frontiers
Learn more about the work of our research integrity team to safeguard the quality of each article we publish.