- Faculty of Computer Science, Otto von Guericke University Magdeburg, Magdeburg, Germany
Virtual reality technologies are broadly used in medicine, including medical educational tasks like surgery training. Annotations are an inseparable part of many medical research and educational tasks. In this systematic review, we investigate the potential of VR for medical tasks with focus on annotation. The questions we pursue are as follows. (Q1) For which healthcare-associated tasks do we find VR-associated investigations and which involve a crowd worker-based annotation? (Q2) To what extent are there gender-specific differences in the usage of VR? To address these questions, we formulated a keyword list and inclusion/exclusion criteria for the collection of recent scientific articles according to the PRISMA Statement 2020. We queried the Medline database and included 59 free full articles available in English and published from 2017 upward. We inspected the abstracts of the retained articles and organized them into 6 categories that referred to VR in the medical context. We identified categories of medicine-related tasks, for which VR is used, and one category associated to cybersickness. We traced technologies used with a higher priority for some tasks, and we found that gender-related investigations are more widespread for some categories than for others. The main findings of our investigation on the role of VR for medical annotation tasks are as follows: VR was used widely for tasks associated with medicine, including medical research and healthcare, but the use of VR for annotation purposes in that context was very limited. Many of the relevant studies concerned VR in education, where annotations may refer to labeling or other enhancements of materials or may refer to exercises. The investigation of gender-related aspects was typically found in studies that encompassed the usage of VR on patients and controls, or on healthy participants in order to assess the potential and limitations of VR for specific tasks/medical assessments or treatments. To fully exploit the VR potential for tasks of medical annotation, especially for the creation of ground truth datasets and similar resources, more research is needed, especially on the interplay of annotator demographics and accessibility to VR technologies.
1 Introduction
Virtual Reality (VR) technologies are widely used in applications associated to medicine. Next to tasks associated with the analysis and visualization of medical data (as, e.g., in Azkue, 2013; Legetth et al., 2021), and to studies using VR to acquire insights on human behavior (see, e.g., Lier et al., 2018; Matsuda et al., 2021), medical education broadly exploits the potential of VR, for example, for surgery training (Chheang et al., 2019). COVID-19 forced universities to consider virtual and online solutions for teaching, whereby online solutions for medical courses, especially those demanding haptics (e.g., anatomy and surgery), place a large challenge. Moro et al. (2021) have shown that student performance is not significantly lower when using VR—a very encouraging finding on the potential of VR for medical education.
Crowdsourcing is increasingly used in medicine and healthcare applications. Wazny (2018) identified 8 areas where crowdsourcing has been used, including diagnosis (e.g., by scoring tumor markers), surveillance (e.g., by getting information on mosquitoes’ locations from citizens), and prediction (e.g., by acquiring filled questionnaires and assessing the likelihood of a disease from them). Tucker et al. (2019) stressed the aspect of collective intelligence in crowdsourcing and observed it as a means of acquiring shared solutions in an open space. Wang et al. (2020) performed a systematic review “to summarize quantitative evidence on crowdsourcing to improve health,” and collected evidence from several application fields, including works on the evaluation of surgical skills and studies on the particular task of “inform[ing] artificial intelligence projects, most often related to the annotation of medical data.” In this systematic review, we focus on medical annotation and investigate the potential of VR for this task.
Annotations are an inseparable part of many medical research works and educational tasks. The annotation of medical content for the acquisition of ground truth datasets is widespread, whereby the term “ground truth” includes the assignment of class labels to instances, but goes well beyond it. For example, Peitek et al. (2018) reported on synchronizing EyeTracker recordings with fMRI, while Joshi et al. (2018) recruited “12 observers [who] independently annotated emotional episodes regarding their temporal location and duration.” Annotation tasks appear in some of the studies in the area “Diagnosis” of Wazny (2018), but medical annotations are also used beyond diagnosis, for example, for data curation and for the evaluation of medical skills in education programs.
Some of these medical annotation tasks naturally lend themselves to the usage of VR technologies. Huaulmé et al. (2019) elaborated on the potential of VR for the annotation of surgical activities. Moro et al. (2021) stated that “Upon review of the literature … anatomy.” However, is it appropriate to assume that VR technologies, such as those listed by Azkue, (2013) for the visualization and annotation in anatomy learning, are available at the desks of crowdworkers? An indirect answer is provided by Johnson et al. (2021), who pointed out that “in other disciplines, data collection tool … ” and highlighted “the ability … studies online.” However, the potential of VR for medical annotation tasks performed by crowdworkers has not been investigated yet. In our study, we investigate the following research question:
[Q1:] For which healthcare-associated tasks do we find VR-associated investigations and which involve crowdworker-based annotations?
Since studies on the demographics in crowdsourcing indicate a large proportion of female crowdworkers [(Ross et al., 2010)] (f: 55%, m: 45%), [(Sun et al., 2022)] (“young, well-educated, and predominantly female”), we want to win insights on whether this gender imbalance could lead to higher opportunities or risks in deploying VR for medical tasks. This could be the case, as an example, for VR technologies where female users report cybersickness more frequently than male users. We therefore also investigate the role of gender in VR for medical annotation tasks, leading to the following research question:
[Q2:] To what extent are there gender-specific differences in the usage of VR?
2 Methods
This systematic review is based on the updated PRISMA statement by Page et al. (2021a), with respect to the specification of information sources, search strategy, eligibility criteria, selection and data collection process, synthesis, and analysis thereof.
2.1 Information Sources and Search Strategy
As data sources, we queried the Medline database (PubMed) on October 10, 2021. We used keywords and combinations of keywords related to “crowdsourcing,” “gender differences,” “virtual reality,” and “medical annotation.” The specific search criteria can be found in Supplementary Materials. In addition to the full search strategy, we used “general browsing” as in the PRISMA extension for searching as described by Rethlefsen et al. (2021). Both the full search strategy of PubMed and our results of general browsing can be found in Supplementary Materials.
2.2 Eligibility Criteria
We included only free full-text articles available in English and those published from 2017 upward. Furthermore, we directly included articles related to the medical area (task XOR study participants) and with a focus on technologies such as HMD, eye trackers, or physiological sensors. We excluded systematic reviews, meta-analyses, and surveys; articles on Deep Learning, crowdfunding, and augmented reality; articles reporting on frameworks; and articles on reading medical documents.
2.3 Selection and Data Collection Process
According to the explanation of Page et al. (2021b) in “Box 3: Study selection methods,” we used “Assessment of each record by one reviewer” in the first steps and “Assessment of records by more than one reviewer” for the remaining articles. Both authors of this systematic review worked independently. Furthermore, we did not use any automation tools for removing duplicates or screening articles (Abstract and Title). We created the PRISMA 2020 flow diagram for new systematic reviews which included searches of databases, registers, and other sources (Figure 1) with the software by Neal R Haddaway (2020), last accessed on October 10th, 2021.
2.4 Synthesis for Q1
We inspected the abstracts of the retained articles and organized them into categories that referred to VR in the medical context. Then, we processed the articles within each category and across the categories as follows:
2.4.1 Description of Each Category With a Set of Keyterms
We invoked functions of the R text mining package “tm” by R Core Team (2021) to remove numbers, stopwords, extra spaces, and words like “Figure” and “Table.” Over the sanitized abstracts with the TermDocumentMatrix() function to derive a document matrix that contains the frequency of each word (row) inside each document (column), we invoked the function wordcloud() with arguments min.freq = 1 (lower boundary on frequency: words with the lower frequency are not plotted) and max.words = 50 (upper boundary on the number of words to be plotted) to plot a wordcloud for the category. As “keyterms” of each category, we chose the top-5 words.
2.4.2 Identification of Within-Category Words Associated With Selected Keyterms
For a small selection of keyterms, we invoked the function findAssoc() of the “tm” package of R by Feinerer et al. (2008) to acquire words that are correlated with the keyterm inside each category. The selected keyterms were the top-1 for each category; if more than one keyterms shared the top-1 position, we considered all of them. The output was a table with one row per keyterm, one column per category, and a list of words within each cell; these words were correlated to the keyterm, subject to the argument corlimit = 0.8 (lower boundary on the correlation coefficient).
2.4.3 Identification of Technologies
Within each category, we inspected the whole articles and identified all used technologies. We ignored the individual product specifications (e.g., specific cameras or head-mount devices). We summarized components and auxiliary devices into generic terms (e.g., “sensor”). We summarized several technologies that were used in one or two articles only into “Other.”
2.5 Synthesis for Q2
We used the categories identified under Q1 and refined them on gender awareness as follows:
2.5.1 Identification of Gender-Related Expressions
We inspected the abstracts and identified termini associated to gender. These terms were “gender” and “sex,” “female” and “male” (also in plural form), “women” and “men.”
2.5.2 Marking Gender Awareness and Gender-Specific Differences
We inspected all articles that contained any of the aforementioned termini and determined whether the reported study contained a gender-related investigation. If yes, we set the gender-awareness flag to Yes. For studies thus flagged, we checked whether the study identified gender-specific differences in the reported outcomes, whereupon we set the gender-specific-differences-found flag to Yes. For studies that contained more than one investigation, we set the flag to Yes, if there was at least one investigation that satisfied the two criteria.
2.5.3 Identification of Within-Category Words Associated With Gender-Related Keyterms
We extended the table built under Q1—task 3 by adding the keyterms “gender,” “female,” “male” and invoking findAssoc() with corlimit = 0.8. We further marked all gender-associated words in the table in orange for better readability.
3 Results
3.1 Study Selection
As shown in Figure 1, we identified 159 records from the Medline database. After removing duplicates (127 records) and screening the remained records (Abstract and Title), we excluded records as described previously. After that, 54 records remained. Additional to the 159 identified records via the Medline database, we identified 5 other potentially records via “General browsing” as described in “Methods.” All in all, this systematic review comprises 59 studies.
3.2 Risk of Bias in Studies
Not applicable. According to Marshall et al. (2017), the risk of bias refers to participant recruitment in the individual studies. The recruitment process in these studies is not of relevance for our investigation because we do not aggregate the results at the participant level.
3.3 Results of Synthesis
The manual inspection of the abstracts led to the following 6 categories:
• Category 1: educational tasks for med students and task improvements (28 studies).
• Category 2: motion and tactile tasks for patients (6 studies).
• Category 3: recognition and navigation tasks for patients (6 studies).
• Category 4: recognition tasks for healthy participants (4 studies).
• Category 5: investigations concerning sickness during the interaction with the VR environment (includes simulation sickness and motion sickness) (4 studies).
• Category 6: medical annotation for crowdsourcing (11 studies).
We report on the VR-associated topics characterizing each category, that is, the technologies used inside each category and across categories (cf. Q1). Then, we describe to what extent gender was considered within each category and in the context of each technology, and we elaborate on the cases where gender-related differences were found (cf. Q2).
3.3.1 Results for Q1: VR-Related Categories for Healthcare Tasks
The keyterms of each category were extracted from the category-specific wordclouds of the 6 categories (cf. Synthesis method 1 for Q1), which we depict in Figure 2. The colors serve to distinguish among words of different frequences; the choice of colors itself has no semantics.
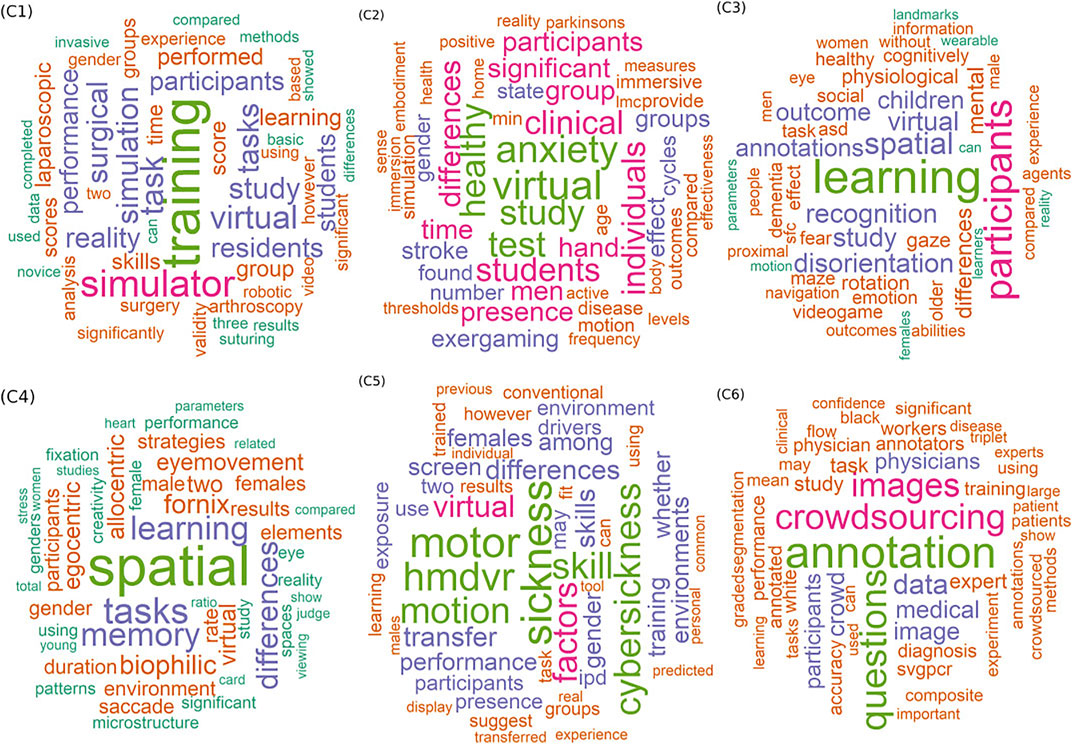
FIGURE 2. Wordclouds for 6 categories: words are ordered on the frequency of appearance in the abstracts of the category; more frequent words appear in a larger font.
The top-5 words of each wordcloud are the “keyterms” of the corresponding category. These words, together with the number of appearances of each one, are shown in Table 1. We also show the top-6th word, to highlight the fact that the frequency of words decreases slowly, and also the fact that the likelihood of seeing the same top word in more than one category decreases as we move down the list. The top-1 word of each category appears in boldface. One category (C5) has two top-1 words of equal frequency.

TABLE 1. Top-5 + 1 words inside the wordcloud of each category; top-5 words serve as “keyterms” to describe the category.
As can be seen from the keyterms in Table 1, category C1 refers to training tasks (1st keyterm), performed with virtual technologies (3rd keyterm), whereupon simulator technologies (2nd keyterm), and other forms of simulation were used. Category C2 also refers to virtual technologies (1st keyterm), but here, the emphasis is not on training but rather on study and test conductment (3rd and 4th keyterms) for healthy individuals (5th keyterm), whereby anxiety (2nd keyterm) is a central aspect; indeed, the 6th most frequent word indicates that the studies in this category are of clinical nature. Categories C3 and C4 are close to each other, both referring to learning and to spatial tasks in studies, whereby C3 refers to recognition tasks and C4 to tasks associated with remembering (cf. memory as keyterm). C5 and C6 are categories on more specialized tasks: C5 is on studies about sickness when performing motoric tasks with VR technologies, prominently with head-mount devices (cf. hmdvr as keyterm). C6 is on annotation tasks (cf. also questions) associated to crowdsourcing, involving images and other forms of data.
Table 2 depicts the concrete healthcare tasks, medical objectives, and technologies that appear inside each category in association with the top-1 keyterm (s) of all categories (cf. Synthesis method 2. for Q1). Note that gender-related words are marked in orange; these are discussed under Results for Q2 later on.
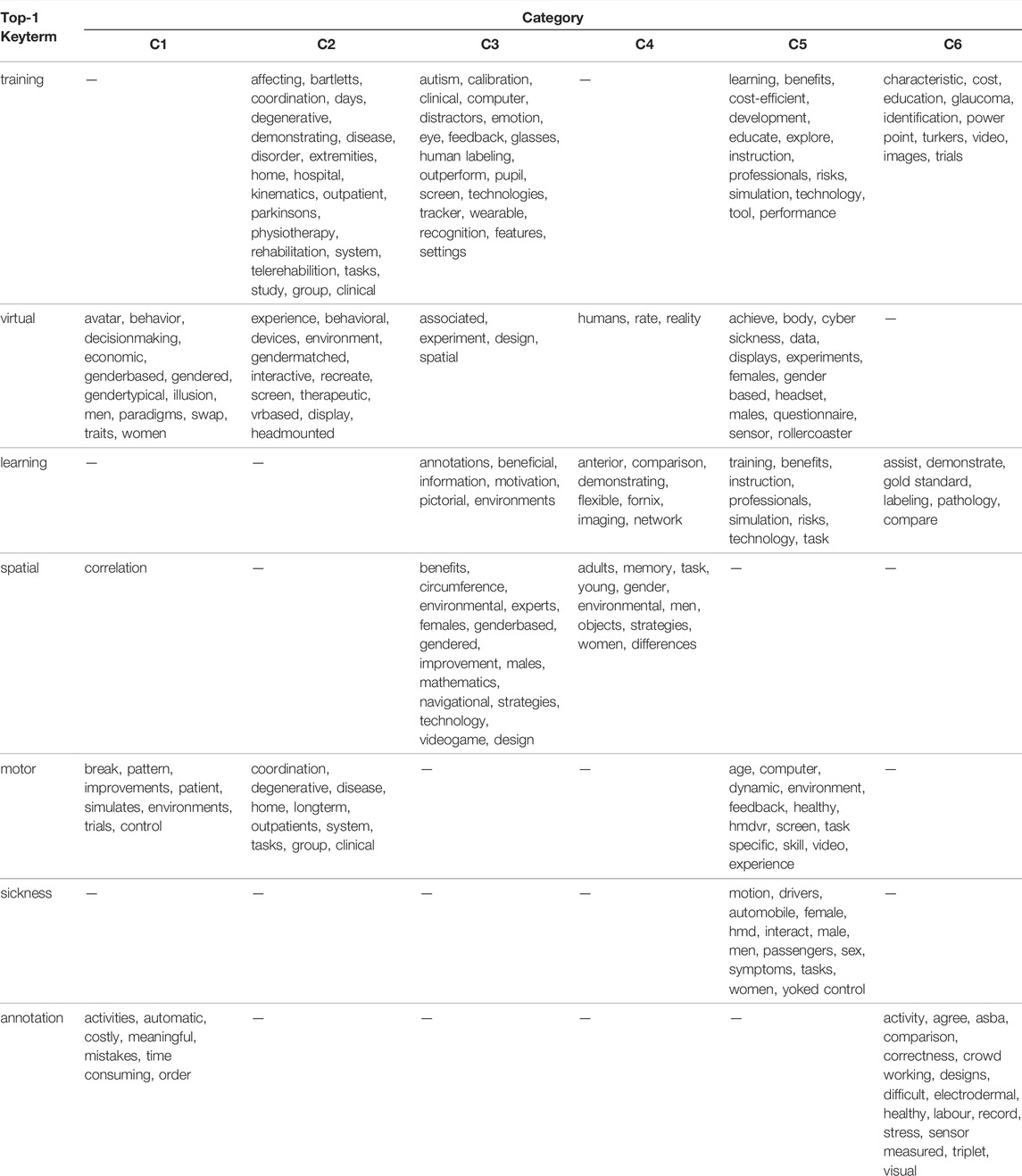
TABLE 2. Associated terms for each category based on all abstracts for the most frequently used words; gender-specific words are marked in orange.
In Table 2, an empty cell indicates that there were no frequent words associated with this keyterm inside the category. Hence, some keyterms are peculiar to one category only, as is the case for sickness and category C5, while other keyterms (like training) are used in multiple categories, however for different purposes. For example, training is associated with VR for rehabilitation and physiotherapy within C2, and with education and with annotations (cf. turkers, identification) within C6; virtual is associated with avatars and decision-making within C1, and with displays, headsets, and sensors within the cybersickness studies’ category C5. Keyterm motor is associated with simulations within C1, with HMD VR, screens, and other devices within C5, and with (body) coordination tasks in cases of degenerative diseases within C2.
Table 2 also shows some ambiguous keyterm–word associations inside the categories. For example, learning and training appear within C5—possibly in the context of instructions for educational experiments or exercises. Keyterm annotation appears also within C1—possibly in the context of educational tasks. The absence of words associated with training within C1 is also remarkable despite the fact that training is the most frequent word in C1; an explanation is that training is associated with many words within C1, but with none of them frequently enough.
Despite these ambiguities, the automatically identified keyterms and the keyterm–word associations highlight the differences and the overlaps among the categories, agreeing and refining the original manual characterization:
C1: category on VR for educational tasks in healthcare, involving training with the help of simulators and avatars, and also encompassing annotation tasks.
C2: category of VR usage for patients, including patient training and rehabilitation, highlighting also patient anxiety as a key issue.
C3 and C4: partially overlapping categories concerning spatial tasks with VR for patients, whereby C3 covers recognition tasks, while C4 covers memory-related tasks.
C5: clear-cut category devoted to the study of cybersickness in VR tasks.
C6: category associated with medical annotation tasks in the context of crowdworking and in the context of education/training with VR.
3.3.2 Results for Q1: VR Technologies for Healthcare Tasks
The 6 categories of healthcare tasks were found to differ on the tasks investigated (training, learning, and cybersickness) and on the study participants (healthy, patients, students, and annotators). However, the used technologies are frequently mentioned only for some of the categories (C1 and C5). A manual inspection of the articles (cf. Synthesis method 3 for Q1) led to the identification of the following technologies depicted in the first row of Table 3: HMD (head-mounted devices), simulators, screens (also displays), sensors of any kind, and other technologies. For each category, the number of studies referring to a given technology is depicted in the column “t_a,” where a study may refer to more than one technology. The acronyms of the columns are explained in Table 4; note that gender-related results are presented in the next subsection.
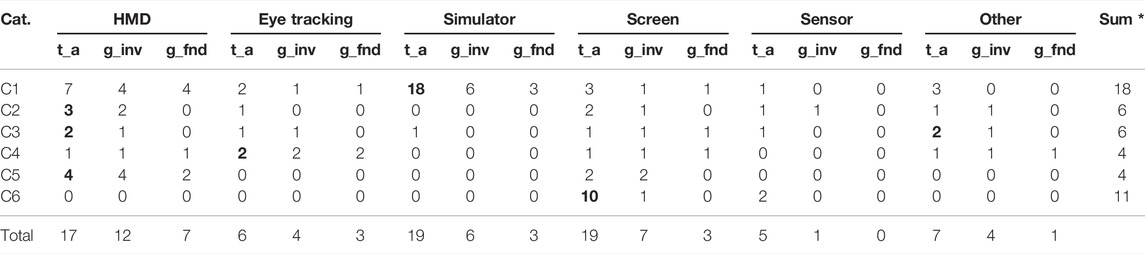
TABLE 3. Results of synthesis—summary of used technologies and gender differences; * Note: some studies cover more than one technology.
For each category listed in Table 3, we marked in boldface the technology most often used: all studies in C1 use simulators. HMD is the most intensively investigated technology in cybersickness category C5, while the EyeTracker technology does not appear in C5 at all. The educational purposes’ category C1 is the one where most of the technologies are used. In contrast, the studies in annotation category C6 concentrate on rudimentary technologies: 9 out of 11 studies only consider screens, and only two of the studies consider sensors, one of them in addition to screens. This indicates a large disparity in the exploitation of the VR-potential among the categories of healthcare tasks, which translates into an unexploited potential of VR for patient support (categories C2 and C3) and for annotation purposes (category C6).
When studying the cross-category spread of technologies on Table 3, we see that technologies HMD and EyeTracking are considered in multiple categories, whereby HMD is the most widespread one. Next to it come sensors. In contrast, simulators are used mainly in C1, that is, for educational purposes. Cybersickness category C5 focuses exclusively on HMD. This implies that the awareness about side effects and risks of other technologies, for example, EyeTrackers and sensors (especially in wearables), should be increased so that these technologies can be fully and safely exploited.
Q2: To what extent are there gender-specific differences in the usage of VR? We presented in Table 3 in detail whether gender-specific differences were investigated. In the following, we give a rough overview, summarized per study, technology, and category. In contrast to Table 3, where multiple technologies could be used per study and thus gender-specific differences were counted multiple times, in the following, only g_inv individual per study is counted.
Investigation of gender differences among categories: When juxtaposing categories to each other, we see that investigation of gender differences is done frequently for some categories (C4 and C5: all studies included an investigation), while for C6, such an investigation was an exception (1 out of 11). For categories C1, C2, and C3, no more than half of the studies contained such an investigation. When investigations were done, the results on the importance of gender varied.
Under C1: Only 11 out of the 28 studies investigated gender aspects. The investigations concerned almost all studied technologies, namely, HMD, Eye Tracking, Simulator, and Screen usage. For Simulator usage, 3 out of the 6 investigations found gender differences; for the other three technologies, each investigation did find some gender difference.
Under C2, C3, and C4: These studies concern VR for patients, although some involve only healthy participants. The role of gender was investigated in most of them: in 5 of the 6 C2 studies, in 4 out of the 6 C3 studies, and all of the 4 C4 studies. While gender-related differences were found only in one C2 study and one C3 study, all of the C4 studies identified gender-related differences. This indicates that the existence of differences associated to gender is due rather to the medical task being investigated rather than to the technology used.
Under C5: All of the four studies in the cybersickness category investigated gender-related aspects, and two of them found gender-related differences. It is not possible to generalize from this small number, but the presence of gender-related aspects in the other categories indicate that more investigations are needed here since the technology may play a gender-dependent negative role on the participant’s experience with VR.
Under C6: Only one of the 11 studies investigated the role of gender, and this one found no differences. Since the VR technologies considered in this category are rather rudimentary (cf. Results on Q1), we can expect that gender-related investigations will become relevant once more when advanced VR technologies, like HMD and EyeTracking, are used for the annotation tasks.
The presence but not prominence of gender-related investigation in most of the 6 categories is also reflected in the associations depicted on Table 5. As explained under “Results for Q1,” Table 5 lists the words appearing frequently together with the keyterms of each category (1st column), subject to a corrlimit of 0.8. We see (in orange color) that words like “female,” “male,” and “gender” (possibly followed by a word suffix like “typical” or “based”) appear frequently together with the keyterm of each category in all categories but C6. Independently of the category, these words appear most often with the keyterms “virtual” (in three categories) and “spatial” (in two categories) but are fairly rare. This agrees with the results on the technologies’ Table 3, where gender-related aspects were investigated often for some categories (like C4: all studies) and rarely for others (like C6: 1 out of 11), summing up in only 26 out of 59 studies with gender-related aspects.
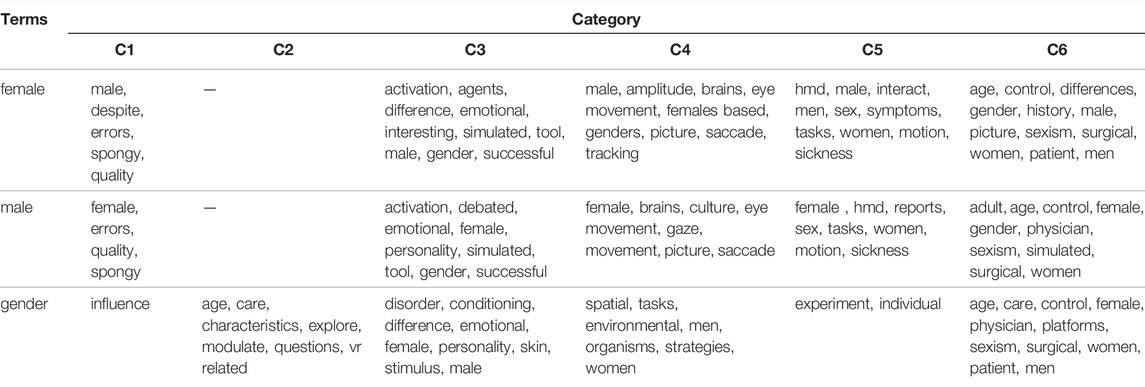
TABLE 5. Associated terms for each category based on all abstracts for gender; gender-specific words are marked in orange.
To identify the aspects associated with gender in the studies inside each of the 6 categories, we have used the words “gender,” “female,” and “male” as keyterms and extracted from the wordcloud of each category the words frequently associated with these keyterms (corrlimit = 0.8). We depicted these words in Table 5: Under cybersickness category C5, these keyterms are indeed associated with sickness, motion, and HMD in experiments. Under categories C3 and C4, we find words describing the specific tasks performed by the study participants. The same holds for annotation category C6, where the keyterms are associated with surgical tasks and with pictures (to be annotated). Under categories C2 and C1, keyterms do not appear frequently and are associated with words like “age” (in C2) and with generic words like “quality” and “influence.” In Table 6, we depict the articles where gender-related differences were found; as expected, gender was used often as the subject of investigation (studying, e.g., whether women perform better than men in some task), as an explaining variable (e.g., women scored higher/lower in questionnaires associated to emotion, interest, etc.), and less frequently as a confounder. Since this covers only 26 out of the 59 studies, there is much space for further investigations on the role of gender in VR studies.
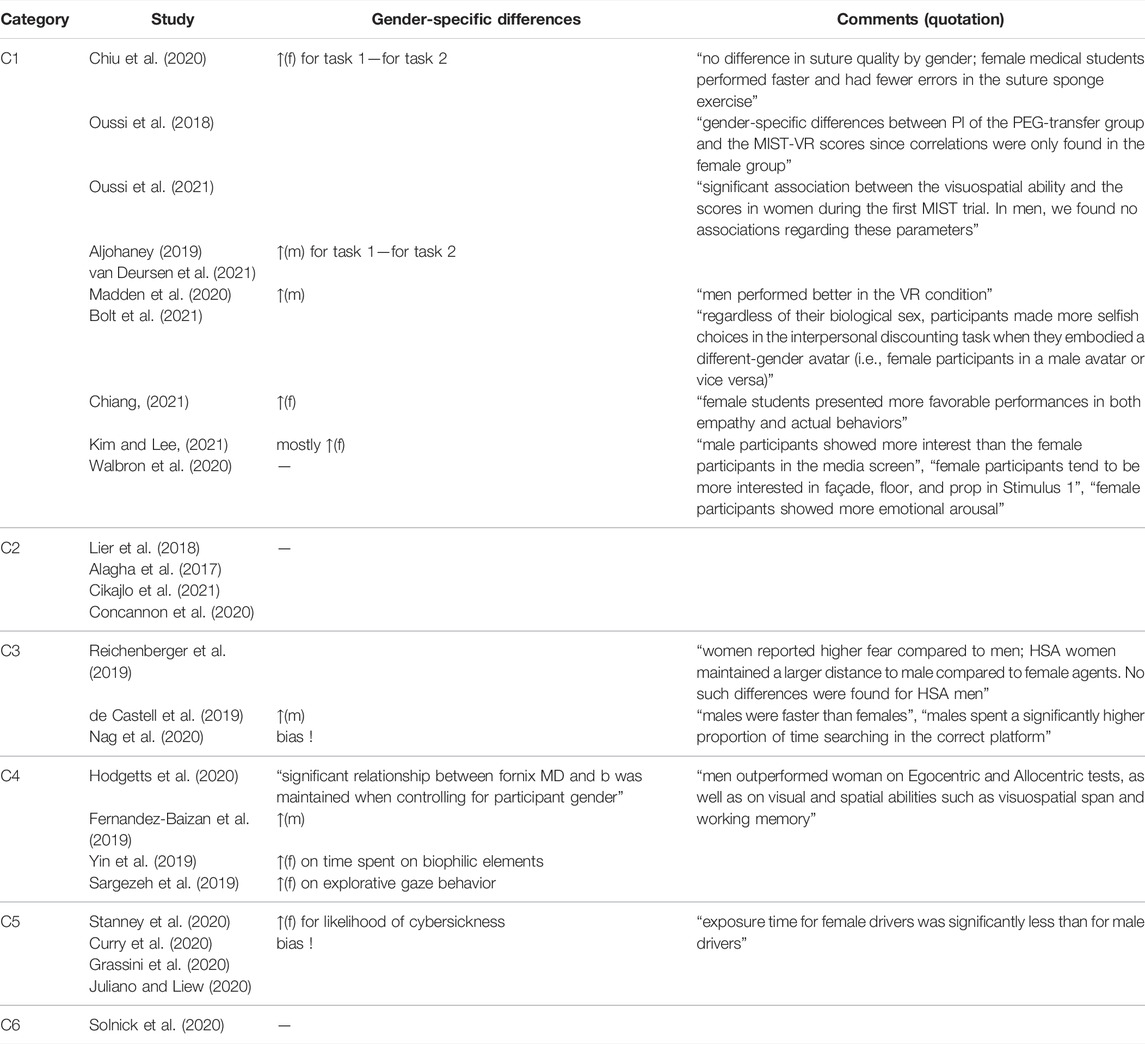
TABLE 6. Gender-specific differences within each category for the 26 (out of the 59) studies that investigated the role of gender. Legend: ↑(f), resp. ↑(m) for ‘higher’ quantity (e.g., performance) or ‘higher’ likelihood (of observing an event) by female, resp. male;—for “no difference”; empty space if differences unclear.
In Table 6, we focused on gender-specific differences within each category. For category C1, 10 studies investigated the role of gender, sometimes with several tasks. Four studies found no gender-specific differences. Women performed better on three tasks and men on two tasks. No gender-specific differences were found in whole category C2, where four studies were represented, and in category C6. In contrast to category C3, where in the three studies investigated, it was very mixed. In category C4, 2 out of 4 studies found better performance among women. In one study, men performed better, and in another study, it was unclear. Furthermore, category C5 includes four studies. Two out of four studies found no differences. In one study, women performed better, and in another study, there was a bias.
4 Discussion
The main findings of our investigation on the role of VR for medical annotation tasks are as follows. VR was used widely for tasks associated with medicine, including medical research and healthcare, but the use of VR for annotation purposes in that context was very limited. Many of the relevant studies concerned VR in education, where annotations may refer to labeling or other enhancements of materials or may refer to exercises. While studies with VR for educational purposes exploited a wide variety of technologies for core educational tasks, the technologies used for annotation purposes were much more limited—mainly conventional screens and sensors.
VR technologies were used in further types of studies; some of them include studies on healthy participants and patients: some of these studies were on tasks of cognition and orientation, whereupon VR technologies were tailored to the task being investigated. Finally, the effects of VR devices constituted a separate category of studies, mainly associated with cybersickness and concerning specific devices like HMD.
The investigation of gender-related aspects was typically found in studies that encompassed the usage of VR on patients and controls, or on healthy participants, in order to assess the potential and limitations of VR for specific tasks/medical assessments or treatments. Therefore, the findings on gender-specific differences were most likely to depend on concrete medical research questions. Studies on VR for educational purposes often addressed the role of gender. Some studies on cybersickness, especially those on HMD, did address the role of gender, though with inconclusive results. Studies on VR for annotations very rarely addressed gender aspects.
4.1 Discussion on Q1—VR for Medical Annotation Tasks
The studies in our collection exhibited much diversity with respect to VR technologies. head-mounted displays (HMD) were the dominant technology, appearing in studies of 4 out of the 5 categories of VR tasks we introduced. However, most studies in all 5 categories also employed further VR technologies, tailored to the task investigated in each article. The VR technologies used for medical annotations were very simple though.
A possible explanation for the limited exploitation of VR for medical annotations might be found in the increasing spread of crowdsourcing for annotation tasks in diagnostics, education, and surveillance, as reflected in the systematic reviews of Wazny (2018), Tucker et al. (2019), and Wang et al. (2020). Some of the studies cited by them involved games, but these games do not seem to have been technology-demanding. Wazny (2018) indicated that the participants in some of the studies owned smartphones and thus had access to mHealth apps. This indicates that crowdworking is currently perceived as an assignment of tasks to workers who own rather rudimentary technologies, while VR demands elaborate hardware.
A further possible explanation for the limited role of VR for medical annotations by crowdworkers can be found in the study of Kourtesis et al. (2019), who reported on the importance of “technological competence.” Kourtesis et al. (2019) investigated the usage of VR as a means of assisting in interventions and of acquiring assessments in neuroscience. Albeit their goals were different from ours, and albeit the studies they collected are closer to those in our categories C2, C3, and C4 but not to C1, we believe that their conclusion on the importance of an ergonomic interaction also applies to C1 on annotation tasks. They stressed that “the VR software should include an ergonomic interaction and navigation system, as well as tutorials, in-game instructions, and prompts.” While tutorials are widespread in crowdworking, the design of games and the focus on ergonomic interaction demand investment—both on the side of the crowdworkers and on the side of the crowdworking task providers. It seems that for medical annotation tasks, we need to separate between annotators that are crowdworkers and those that have access to all the facilities of the medical site. The suggestions of Kourtesis et al. (2019) may be implemented for the latter. For the former, it must be investigated whether there is willingness to perform such investments. Taking the crowdworkers’ profiles reported in the study Vanhove et al. (2021) into account, it seems that the cost of VR might be a barrier at the crowdworkers’ side.
Nonetheless, we can expect that the accessibility to higher-end technologies may increase, also as part of the health measures taken against COVID-19. Next to medical education conducted online (see, e.g., Nimavat et al., 2021), VR technologies are being used for group discussions on diagnostics (Kim et al., 2020); even studies conducted online with the use of VR have become reality (Mottelson et al., 2021). Hence, it can be expected that medical annotation tasks will capitalize more on VR technologies, as these become more broadly available. Future investigations should also take costs and accessibility to VR-related infrastructure since VR may demand hardware and software [Azkue (2013); Kpokiri et al. (2021)] that is not available off the shelf to crowdworkers. Crowdworker demographics should also be considered, since not all technologies are equally available (including “inexpensive enough”) in all countries.
4.2 Discussion on Q2—Gender-Specific Differences
Gender-related aspects were investigated in all categories of articles in our collection, although the portion of studies with such investigations varied substantially among technologies. Many studies involving head-mounted devices contained investigations on the role of gender; gender-specific differences were often identified. As with many other studies on the role of gender in VR or immersive reality, cf (Czerwinski et al., 2002; Ausburn et al., 2009; Dirin et al., 2019; Grassini and Laumann, 2020), there is no clear conclusion on whether there are gender-dependent differences or not. As in the early work of Czerwinski et al. (2002), some studies in our collection found that female participants behaved differently or, for the educational category C1, performed differently than male participants. This indicates that gender-specific differences might be task-dependent.
For medical annotation tasks involving VR, the investigation of gender-related aspects was limited to one single study. When we observe medical annotation tasks from the perspective of crowdsourcing, we do identify studies related to the role of gender, including insights on gender distribution among the crowdworkers themselves, cf (Ross et al., 2010) and (Sun et al., 2022). As VR technologies become more accessible and start being used online Mottelson et al. (2021), we expect that their potential for VR-based crowdsourcing will increase and the need for gender-aware usage of VR components will become paramount.
Since the accessibility to VR equipment in home offices may depend on many demographic factors, it may be worth distinguishing between the role of gender in VR-based annotation tasks conducted in labs and the role of crowdworker genders for VR-based crowdsourcing tasks. The surveys of Tucker et al. (2019) and Sun et al. (2022) point to concrete profiles of crowdworkers, going beyond gender and including family state, role within family, employment, education, and age distribution. These aspects may affect the affinity to VR and the willingness to invest on VR at a home–office; hence, they should be included, next to gender, in investigations toward VR for medical annotation tasks.
4.3 Limitations
One limitation of our systematic review is the rather small and heterogeneous set of collected articles. This is due to our focus on VR for medical tasks with an emphasis on annotation: while there are studies on annotation as part of medical education tasks and studies on learning, the mainstream of VR seems to be still on other usages, including games. Our work shows that there is potential in this domain, but there are also risks associated to gender bias.
We built our set of articles by issuing queries to the Medline database. Other literature collections, for example, Google scholar1, would have delivered additional hits, as indicated by the literature cited in the Introduction. However, we wanted to concentrate on reports about the impact of VR on human participants of studies rather than technological advances and software validation of VR components, and we expected that studies on VR-exposure to humans are most likely to be registered with Medline, even if they also appear in other collections. A comparative study between the topical areas of articles in Medline and articles indexed by Google scholar, ACM, IEEE Digital Library, DBLP, etc., is needed in order to figure out whether studies associated with VR exposure are mostly indexed in Medline or not. Notwithstanding the need for such a study, the coverage of the subject in Medline seems rather limited. This implies that medical researchers and practitioners may need more time to “discover” cutting edge VR technologies of relevance to them—keeping also in mind that the terminology in engineering journals is rather different than in medicine-oriented journals.
Furthermore, we excluded publications that appeared earlier than 2017. Since VR is a very dynamic domain, we decided to concentrate on the most recent technologies. However, it is possible that we missed insights on older technologies that are still in use. Despite these limitations, our systematic review delivered a first insight on the as-yet-limited role of VR technologies for annotation tasks. To capitalize on the VR potential for annotations in the lab by crowdworkers, it is important to consider technology accessibility (including technology costs) in dependence of annotator demographics.
Data Availability Statement
The original contributions presented in the study are included in the article/Supplementary Material; further inquiries can be directed to the corresponding author.
Author Contributions
AR and MS developed the concept of this systematic review. AR wrote the initial draft and performed the literature search/analyses. MS reviewed and edited the manuscript.
Funding
The student assistant position of author AR has been financed through the Equal Opportunities Fund of the Faculty of Computer Science, Otto von Guericke University Magdeburg.
Conflict of Interest
The authors declare that the research was conducted in the absence of any commercial or financial relationships that could be construed as a potential conflict of interest.
Publisher’s Note
All claims expressed in this article are solely those of the authors and do not necessarily represent those of their affiliated organizations, or those of the publisher, the editors, and the reviewers. Any product that may be evaluated in this article, or claim that may be made by its manufacturer, is not guaranteed or endorsed by the publisher.
Supplementary Material
The Supplementary Material for this article can be found online at: https://www.frontiersin.org/articles/10.3389/frvir.2022.717383/full#supplementary-material
Footnotes
References
Alagha, M. A., Alagha, M. A., Dunstan, E., Sperwer, O., Timmins, K. A., and Boszczyk, B. M. (2017). Development of a New Assessment Tool for Cervical Myelopathy Using Hand-Tracking Sensor: Part 2: Normative Values. Eur. Spine J. 26, 1298–1304. doi:10.1007/s00586-017-4949-2
Aljohaney, A. A. (2019). Predictors of Virtual Reality Simulation Bronchoscopy Performance Among Novice Bronchoscopists. Adv. Med. Educ. Pract. 10, 63–70. doi:10.2147/amep.s186275
Ausburn, L. J., Martens, J., Washington, A., Steele, D., and Washburn, E. (2009). A Cross-Case Analysis of Gender Issues in Desktop Virtual Reality Learning Environments. J. STEM Teach. Educ. 46, 6.
Azkue, J.-J. (2013). A Digital Tool for Three-Dimensional Visualization and Annotation in Anatomy and Embryology Learning. Eur. J. Anat. 17, 146–154.
Bolt, E., Ho, J. T., Roel Lesur, M., Soutschek, A., Tobler, P. N., and Lenggenhager, B. (2021). Effects of a Virtual Gender Swap on Social and Temporal Decision-Making. Sci. Rep. 11, 15376–15415. doi:10.1038/s41598-021-94869-z
Chheang, V., Saalfeld, P., Huber, T., Huettl, F., Kneist, W., Preim, B., et al. (2019). “Collaborative Virtual Reality for Laparoscopic Liver Surgery Training,” in 2019 IEEE International Conference on Artificial Intelligence and Virtual Reality (AIVR) (IEEE), 1–17. doi:10.1109/aivr46125.2019.00011
Chiang, T. H. (2021). Investigating Effects of Interactive Virtual Reality Games and Gender on Immersion, Empathy and Behavior into Environmental Education. Front. Psychol. 12, 2900. doi:10.3389/fpsyg.2021.608407
Chiu, H.-Y., Kang, Y.-N., Wang, W.-L., Tong, Y.-S., Chang, S.-W., Fong, T.-H., et al. (2020). Gender Differences in the Acquisition of Suturing Skills with the da Vinci Surgical System. J. Formos. Med. Assoc. 119, 462–470. doi:10.1016/j.jfma.2019.06.013
Cikajlo, I., Hukić, A., and Zajc, D. (2021). Exergaming as Part of the Telerehabilitation Can Be Adequate to the Outpatient Training: Preliminary Findings of a Non-Randomized Pilot Study in Parkinson’s Disease. Front. Neurology 12, 280. doi:10.3389/fneur.2021.625225
Concannon, B. J., Esmail, S., and Roduta Roberts, M. (2020). Immersive Virtual Reality for the Reduction of State Anxiety in Clinical Interview Exams: Prospective Cohort Study. JMIR Serious Games 8, e18313. doi:10.2196/18313
Curry, C., Li, R., Peterson, N., and Stoffregen, T. A. (2020). Cybersickness in Virtual Reality Head-Mounted Displays: Examining the Influence of Sex Differences and Vehicle Control. Int. J. Human-Computer Interact. 36, 1161–1167. doi:10.1080/10447318.2020.1726108
Czerwinski, M., Tan, D. S., and Robertson, G. G. (2002). “Women Take a Wider View,” in Proceedings of the SIGCHI conference on Human factors in computing systems, 195–202. doi:10.1145/503376.503412
de Castell, S., Larios, H., and Jenson, J. (2019). Gender, Videogames and Navigation in Virtual Space. Acta Psychol. 199, 102895. doi:10.1016/j.actpsy.2019.102895
Dirin, A., Alamäki, A., and Suomala, J. (2019). Gender Differences in Perceptions of Conventional Video, Virtual Reality and Augmented Reality. Available at: https://www.learntechlib.org/p/216491/
Feinerer, I., Hornik, K., and Meyer, D. (2008). Text Mining Infrastructure in R. J. Stat. Softw. 25, 1–54. doi:10.18637/jss.v025.i05
Fernandez-Baizan, C., Arias, J. L., and Mendez, M. (2019). Spatial Memory in Young Adults: Gender Differences in Egocentric and Allocentric Performance. Behav. brain Res. 359, 694–700. doi:10.1016/j.bbr.2018.09.017
Grassini, S., and Laumann, K. (2020). Are Modern Head-Mounted Displays Sexist? a Systematic Review on Gender Differences in Hmd-Mediated Virtual Reality. Front. Psychol. 11, 1604. doi:10.3389/fpsyg.2020.01604
Grassini, S., Laumann, K., and Rasmussen Skogstad, M. (2020). The Use of Virtual Reality Alone Does Not Promote Training Performance (But Sense of Presence Does). Front. Psychol. 11, 1743. doi:10.3389/fpsyg.2020.01743
[Dataset] Haddaway, N. R. (2020). PRISMA2020: R Package and ShinyApp for Producing PRISMA 2020 Compliant Flow Diagrams. Zenodo. doi:10.5281/zenodo.4287835
Hodgetts, C. J., Stefani, M., Williams, A. N., Kolarik, B. S., Yonelinas, A. P., Ekstrom, A. D., et al. (2020). The Role of the Fornix in Human Navigational Learning. Cortex 124, 97–110. doi:10.1016/j.cortex.2019.10.017
Huaulmé, A., Despinoy, F., Perez, S. A. H., Harada, K., Mitsuishi, M., and Jannin, P. (2019). Automatic Annotation of Surgical Activities Using Virtual Reality Environments. Int. J. CARS 14, 1663–1671. doi:10.1007/s11548-019-02008-x
Johnson, B. P., Dayan, E., Censor, N., and Cohen, L. G. (2021). Crowdsourcing in Cognitive and Systems Neuroscience. The Neuroscientist. Boston: RStudio Inc. Available at: https://www.organizingcreativity.com/2020/08/citing-r-and-rstudio/.
Joshi, A. A., Chong, M., Li, J., Choi, S., and Leahy, R. M. (2018). Are You Thinking what I'm Thinking? Synchronization of Resting fMRI Time-Series across Subjects. NeuroImage 172, 740–752. doi:10.1016/j.neuroimage.2018.01.058
Juliano, J. M., and Liew, S. L. (2020). Transfer of Motor Skill between Virtual Reality Viewed Using a Head-Mounted Display and Conventional Screen Environments. J. Neuroeng Rehabil. 17, 48–13. doi:10.1186/s12984-020-00678-2
Kim, N., and Lee, H. (2021). Assessing Consumer Attention and Arousal Using Eye-Tracking Technology in Virtual Retail Environment. Front. Psychol. 12, 2861. doi:10.3389/fpsyg.2021.665658
Kim, B., Loke, Y.-H., Mass, P., Irwin, M. R., Capeland, C., Olivieri, L., et al. (2020). A Novel Virtual Reality Medical Image Display System for Group Discussions of Congenital Heart Disease: Development and Usability Testing. JMIR cardio 4, e20633. doi:10.2196/20633
Kourtesis, P., Collina, S., Doumas, L. A. A., and MacPherson, S. E. (2019). Technological Competence Is a Pre-Condition for Effective Implementation of Virtual Reality Head Mounted Displays in Human Neuroscience: a Technological Review and Meta-Analysis. Front. Hum. Neurosci. 13, 342. doi:10.3389/fnhum.2019.00342
Kpokiri, E. E., John, R., Wu, D., Fongwen, N., Budak, J. Z., Chang, C. C., et al. (2021). Crowdsourcing to Develop Open-Access Learning Resources on Antimicrobial Resistance. BMC Infect. Dis. 21, 914–917. doi:10.1186/s12879-021-06628-0
Legetth, O., Rodhe, J., Lang, S., Dhapola, P., Wallergård, M., and Soneji, S. (2021). CellexalVR: A Virtual Reality Platform to Visualize and Analyze Single-Cell Omics Data. iScience 24, 103251. doi:10.1016/j.isci.2021.103251
Lier, E. J., Harder, J., Oosterman, J. M., De Vries, M., and Van Goor, H. (2018). Modulation of Tactile Perception by Virtual Reality Distraction: The Role of Individual and Vr-Related Factors. Plos one 13, e0208405. doi:10.1371/journal.pone.0208405
Madden, J., Pandita, S., Schuldt, J. P., Kim, B., S. Won, A. A., and Holmes, N. G. (2020). Ready Student One: Exploring the Predictors of Student Learning in Virtual Reality. Plos One 15, e0229788. doi:10.1371/journal.pone.0229788
Marshall, I. J., Kuiper, J., Banner, E., and Wallace, B. C. (2017). Automating Biomedical Evidence Synthesis: RobotReviewer. Proc. Conf. Assoc. Comput. Linguist. Meet. 2017, 7–12. doi:10.18653/v1/P17-4002
Matsuda, Y., Nakamura, J., Amemiya, T., Ikei, Y., and Kitazaki, M. (2021). Enhancing Virtual Walking Sensation Using Self-Avatar in First-Person Perspective and Foot Vibrations. Front. Virtual Real. 2, 26. doi:10.3389/frvir.2021.654088
Moro, C., Birt, J., Stromberga, Z., Phelps, C., Clark, J., Glasziou, P., et al. (2021). Virtual and Augmented Reality Enhancements to Medical and Science Student Physiology and Anatomy Test Performance: A Systematic Review and Meta-Analysis. Anat. Sci. Educ. 14, 368–376. doi:10.1002/ase.2049
Mottelson, A., Petersen, G. B., Lilija, K., and Makransky, G. (2021). Conducting Unsupervised Virtual Reality User Studies Online. Front. Virtual Real. 2, 66. doi:10.3389/frvir.2021.681482
Nag, A., Haber, N., Voss, C., Tamura, S., Daniels, J., Ma, J., et al. (2020). Toward Continuous Social Phenotyping: Analyzing Gaze Patterns in an Emotion Recognition Task for Children with Autism through Wearable Smart Glasses. J. Med. Internet Res. 22, e13810. doi:10.2196/13810
Nimavat, N., Singh, S., Fichadiya, N., Sharma, P., Patel, N., Kumar, M., et al. (2021). Online Medical Education in India - Different Challenges and Probable Solutions in the Age of COVID-19. Adv. Med. Educ. Pract. 12, 237–243. doi:10.2147/amep.s295728
Oussi, N., Loukas, C., Kjellin, A., Lahanas, V., Georgiou, K., Henningsohn, L., et al. (2018). Video Analysis in Basic Skills Training: a Way to Expand the Value and Use of Blackbox Training? Surg. Endosc. 32, 87–95. doi:10.1007/s00464-017-5641-7
Oussi, N., Renman, P., Georgiou, K., and Enochsson, L. (2021). Baseline Characteristics in Laparoscopic Simulator Performance: The Impact of Personal Computer (PC)-gaming Experience and Visuospatial Ability. Surg. Open Sci. 4, 19–25. doi:10.1016/j.sopen.2020.06.002
Page, M. J., McKenzie, J. E., Bossuyt, P. M., Boutron, I., Hoffmann, T. C., Mulrow, C. D., et al. (2021a). The Prisma 2020 Statement: an Updated Guideline for Reporting Systematic Reviews. BMJ 372, n71. doi:10.1136/bmj.n71
Page, M. J., Moher, D., Bossuyt, P. M., Boutron, I., Hoffmann, T. C., Mulrow, C. D., et al. (2021b). Prisma 2020 Explanation and Elaboration: Updated Guidance and Exemplars for Reporting Systematic Reviews. BMJ 372, n160. doi:10.1136/bmj.n160
Peitek, N., Siegmund, J., Parnin, C., Apel, S., and Brechmann, A. (2018). “Toward Conjoint Analysis of Simultaneous Eye-Tracking and Fmri Data for Program-Comprehension Studies,” in Proceedings of the Workshop on Eye Movements in Programming, 1–5. doi:10.1145/3216723.3216725
R Core Team (2021). R: A Language and Environment for Statistical Computing. Vienna, Austria: R Foundation for Statistical Computing.
Reichenberger, J., Pfaller, M., Forster, D., Gerczuk, J., Shiban, Y., and Mühlberger, A. (2019). Men Scare Me More: Gender Differences in Social Fear Conditioning in Virtual Reality. Front. Psychol. 10, 1617. doi:10.3389/fpsyg.2019.01617
Rethlefsen, M. L., Kirtley, S., Waffenschmidt, S., Ayala, A. P., Moher, D., Page, M. J., et al. (2021). Prisma-s: an Extension to the Prisma Statement for Reporting Literature Searches in Systematic Reviews. Syst. Rev. 10, 39–19. doi:10.1186/s13643-020-01542-z
Ross, J., Irani, L., Silberman, M. S., Zaldivar, A., and Tomlinson, B. (2010). “Who Are the Crowdworkers? Shifting Demographics in Mechanical Turk,” in CHI’10 extended abstracts on Human factors in computing systems, 2863–2872.
Sargezeh, B. A., Tavakoli, N., and Daliri, M. R. (2019). Gender-Based Eye Movement Differences in Passive Indoor Picture Viewing: An Eye-Tracking Study. Physiology Behav. 206, 43–50. doi:10.1016/j.physbeh.2019.03.023
Solnick, R. E., Peyton, K., Kraft-Todd, G., and Safdar, B. (2020). Effect of Physician Gender and Race on Simulated Patients' Ratings and Confidence in Their Physicians: A Randomized Trial. JAMA Netw. Open 3, e1920511. doi:10.1001/jamanetworkopen.2019.20511
Stanney, K., Fidopiastis, C., and Foster, L. (2020). Virtual Reality Is Sexist: but it Does Not Have to Be. Front. Robot. AI 7, 4. doi:10.3389/frobt.2020.00004
Sun, Y., Ma, X., Ye, K., and He, L. (2022). Investigating Crowdworkers' Identify, Perception and Practices in Micro-Task Crowdsourcing. Proc. ACM Hum.-Comput. Interact. 6, 1–20. doi:10.1145/3492854
Tucker, J. D., Day, S., Tang, W., and Bayus, B. (2019). Crowdsourcing in Medical Research: Concepts and Applications. PeerJ 7, e6762. doi:10.7717/peerj.6762
van Deursen, M., Reuvers, L., Duits, J. D., de Jong, G., van den Hurk, M., and Henssen, D. (2021). Virtual Reality and Annotated Radiological Data as Effective and Motivating Tools to Help Social Sciences Students Learn Neuroanatomy. Sci. Rep. 11, 12843–12910. doi:10.1038/s41598-021-92109-y
Vanhove, A. J., Miller, A. D., and Harms, P. D. (2021). Understanding Subpopulations on Mechanical Turk. J. Personnel Psychol. 20 (4), 176–186. doi:10.1027/1866-5888/a000281
Walbron, P., Common, H., Thomazeau, H., Hosseini, K., Peduzzi, L., Bulaid, Y., et al. (2020). Virtual Reality Simulator Improves the Acquisition of Basic Arthroscopy Skills in First-Year Orthopedic Surgery Residents. Orthop. Traumatology Surg. Res. 106, 717–724. doi:10.1016/j.otsr.2020.03.009
Wang, C., Han, L., Stein, G., Day, S., Bien-Gund, C., Mathews, A., et al. (2020). Crowdsourcing in Health and Medical Research: a Systematic Review. Infect. Dis. Poverty 9, 8–9. doi:10.1186/s40249-020-0622-9
Wazny, K. (2018). Applications of Crowdsourcing in Health: an Overview. J. Glob. Health 8, 010502. doi:10.7189/jogh.08.010502
Keywords: immersive virtual reality (VR), medical annotation, eye movement monitoring, wearable sensors, eye-tracking, head-mounted display (HMD), crowdsourcing, gender-specific differences
Citation: Rother A and Spiliopoulou M (2022) Virtual Reality for Medical Annotation Tasks: A Systematic Review. Front. Virtual Real. 3:717383. doi: 10.3389/frvir.2022.717383
Received: 30 May 2021; Accepted: 19 May 2022;
Published: 29 June 2022.
Edited by:
Panagiotis Germanakos, SAP SE, GermanyReviewed by:
Panagiotis Kourtesis, Inria Rennes - Bretagne Atlantique Research Centre, FranceAbraham Galbraith Campbell, University College Dublin, Ireland
Manuela Chessa, University of Genoa, Italy
Copyright © 2022 Rother and Spiliopoulou. This is an open-access article distributed under the terms of the Creative Commons Attribution License (CC BY). The use, distribution or reproduction in other forums is permitted, provided the original author(s) and the copyright owner(s) are credited and that the original publication in this journal is cited, in accordance with accepted academic practice. No use, distribution or reproduction is permitted which does not comply with these terms.
*Correspondence: Anne Rother , YW5uZS5yb3RoZXJAb3ZndS5kZQ==