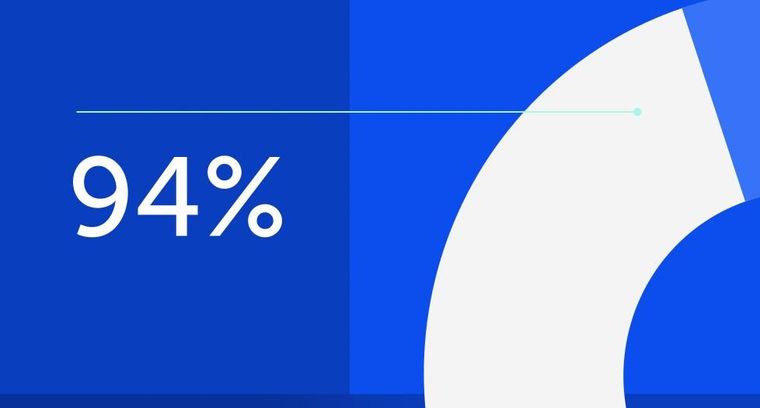
94% of researchers rate our articles as excellent or good
Learn more about the work of our research integrity team to safeguard the quality of each article we publish.
Find out more
ORIGINAL RESEARCH article
Front. Virtual Real., 14 September 2021
Sec. Virtual Reality and Human Behaviour
Volume 2 - 2021 | https://doi.org/10.3389/frvir.2021.739038
This article is part of the Research TopicSimulating Virtual Humans and Crowds for Virtual RealityView all 4 articles
Care for chronic disease requires patient adherence to treatment advice. Nonadherence worsens health outcomes and increases healthcare costs. When healthcare professionals are in short supply, a virtual physician could serve as a persuasive technology to promote adherence. However, acceptance of advice may be hampered by the uncanny valley effect—a feeling of eeriness elicited by human simulations. In a hypothetical virtual doctor consultation, 441 participants assumed the patient’s role. Variables from the stereotype content model and the heuristic–systematic model were used to predict adherence intention and behavior change. This 2 × 5 between-groups experiment manipulated the doctor’s bedside manner—either good or poor—and virtual depiction at five levels of realism. These independent variables were designed to manipulate the doctor’s level of warmth and eeriness. In hypothesis testing, depiction had a nonsignificant effect on adherence intention and diet and exercise change, even though the 3-D computer-animated versions of the doctor (i.e., animation, swapped, and bigeye) were perceived as eerier than the others (i.e., real and cartoon). The low-warmth, high-eeriness doctor prompted heuristic processing of information, while the high-warmth doctor prompted systematic processing. This pattern contradicts evidence reported in the persuasion literature. For the stereotype content model, a path analysis found that good bedside manner increased the doctor’s perceived warmth significantly, which indirectly increased physical activity. For the heuristic–systematic model, the doctor’s eeriness, measured in a pretest, had no significant effect on adherence intention and physical activity, while good bedside manner increased both significantly. Surprisingly, cognitive perspective-taking was a stronger predictor of change in physical activity than adherence intention. Although virtual characters can elicit the uncanny valley effect, their effect on adherence intention and physical activity was comparable to a video of a real person. This finding supports the development of virtual consultations.
There is growing interest in adopting virtual characters to persuade people to change their health-related behaviors. Strategies that people adopt to persuade others, such as arguments and social cues, could also be employed in virtual characters (André et al., 2011). This could offer a novel and efficient way to increase adherence to treatment advice and improve health literacy more generally. Consulting with conversational agents has been found to increase users’ physical activity (Yin et al., 2010) and guide older adults toward healthy behaviors (Looije et al., 2010). However, virtual characters, depending on their level of realism, could appear eerie—an effect called the uncanny valley (Mori, 2012). Thus, we must consider how eeriness influences persuasion in virtual characters.
Persuasion can be used socially to encourage behaviors that enhance health and discourage those that harm it (Umberson, 1987; Lewis and Rook, 1999). Adherence measures how much a patient’s behavior follows the program they have established with their healthcare provider, such as taking prescribed medications on time and making recommended lifestyle changes (McDonald et al., 2002). Adherence intention is the patient’s strength of determination to follow the program.
This work adopts as its use case scenario a virtual consultation involving a possible diabetes diagnosis. Diabetes and other chronic diseases pose a growing threat to global health. By 2030, the number of people with type II diabetes is projected to hit 578 million (Saeedi et al., 2019). Diabetes reduces life expectancy by 5 years, and its complications reduce quality of life (Zhuo et al., 2013). To stay healthy, diabetes patients must follow their doctors’ diet and exercise advice (Nelson et al., 2002). Although a sedentary lifestyle and poor dietary habits are risk factors, a healthy diet and regular exercise can delay or prevent type II diabetes and its associated health complications (Eriksson and Lindgärde, 1991; Tuomilehto et al., 2001; Rejeski et al., 2012; Sami et al., 2017).
Increasing adherence is a major public health challenge. In the United States, nonadherence to medication affects 40–50% of patients with chronic diseases like diabetes and hypertension, leading to 100,000 preventable deaths each year (Kleinsinger, 2018). For diabetic patients, nonadherence to medication increases adverse health outcomes, hospitalization, and mortality (Ho et al., 2006). The rate of nonadherence can exceed 70% when the physician advises significant or complex changes in behavior (Martin et al., 2005). Nonadherence results in increased hospitalization and mortality rates, especially in older adults (Walsh et al., 2019).
The cost of nonadherence to healthcare systems is staggering. In the United States, estimates ranged from $100 billion to $300 billion per year (Chisholm-Burns and Spivey, 2012; McGuire and Iuga, 2014; Morello and Hirsh 2017). Per patient, they ranged from $949 to $44,190 in 2015, depending on the disease (Cutler et al., 2018).
Physician bedside manner influences the physician–patient relationship, which, in turn, influences adherence (Miller, 1997; Safran et al., 1998; Ettner, 1999). For example, a poor physician–patient relationship lowered long-term adherence in patients with hypertension (Waeber et al., 2000). However, a European study found that a good physician–patient relationship encouraged patients to follow recommendations (Stavropoulou, 2011). A good relationship also improved adherence for HIV-positive patients (Schneider et al., 2004).
The United States faces a shortage of clinicians and other healthcare professionals (McKechnie, 2016; Kirch and Petelle, 2017; Marć et al., 2019). Researchers estimate that nearly 52,000 more primary care physicians will be needed by 2025 (Petterson et al., 2012). A virtual clinical consultation could address the scarcity of healthcare experts. However, creating credible virtual characters remains a challenge for researchers and designers, in part because factors like the uncanny valley could negatively influence the persuasiveness of their advice (McDonnell and Breidt, 2010; Wang et al., 2013).
The uncanny valley effect, proposed in 1970 by Mori (2012), is a negative affective reaction toward objects that imperfectly resemble human beings, such as android robots or computer-animated characters. A large meta-analysis has confirmed the effect (Diel et al., 2022), which could hinder the acceptance of virtual characters and their advice. The uncanny valley effect can occur when a character’s features are atypical, appear less real than others, or deviate from a familiar configuration (Chattopadhyay and MacDorman, 2016; Diel and MacDorman, 2021). This can elicit in viewers a feeling of eeriness and revulsion. For example, eeriness could be elicited by atypical proportions in a photorealistic human face (Green et al., 2008), such as enlarged eyes, or inconsistent realism among facial features (MacDorman et al., 2009; Stein and Ohler, 2018). Eeriness has been considered detrimental to persuasion because of its negative effect on a virtual character’s credibility (Patel and MacDorman, 2015). Increasing a human character’s overall realism makes subtle nonhuman imperfections in its features more noticeable and disturbing (McDonnell et al., 2012). Different stylizations of computer-generated characters could affect the perception of eeriness, trustworthiness, attractiveness, and realism (Zell et al., 2015; Schindler et al., 2017).
MacDorman (2019) proposed that the uncanny valley effect could disrupt feelings of empathy for a virtual character. It could, therefore, have a greater effect on a high-warmth character than a low-warmth character because a high-warmth character elicits more empathy. Thus, in a virtual doctor’s consultation, it would be useful to examine both realism and bedside manner to check for interaction effects.
Virtual characters have been found to be at least as persuasive as real people (Bickmore et al., 2009; Dai and MacDorman, 2018; Ogawa et al., 2018). Their ability to persuade has been used to improve patient adherence (Brown et al., 2016; Richards and Caldwell, 2016). Thus, we hypothesize that (H1) the depiction of the virtual physician affects adherence. In our previous results, the animated physician was more persuasive than a video recording of a real person because interacting with the animated character was more enjoyable (Dai and MacDorman, 2018).
In the literature on persuasion, the heuristic–systematic model (HSM) explains how people process information (Chaiken, 1980; Chaiken and Eagly, 1983; Chen and Chaiken, 1999; Todorov, Chaiken et al., 2002). Systematic processing demands cognitive effort to understand and evaluate the content of a message. Thus, for systematic processing, message-related thoughts mediate persuasion. By contrast, heuristic processing relies on rules of thumb or peripheral cues, which reduce cognitive effort. For heuristic processing, persuasion is mediated by thoughts unrelated to the message, such as features of the message’s source. These could include, for example, a speaker’s interpersonal warmth, physical attractiveness, novelty, and realism (Miller et al., 1976; Chaiken, 1980).
People may pay more attention to a virtual character than a real human because its visual novelty stimulates curiosity, discovery, and learning (Sokolov, 1963; Patel et al., 2014). For example, people stared longer at the faces of eerie digital human characters than real humans (Carter et al., 2013). Virtual environments have used behavioral measures like proximity (i.e., the minimum distance between a person and a virtual character). People moved closer to eerie, zombielike virtual characters and paid more attention to them than to more realistic characters (Zibrek et al., 2018). Thus, we hypothesize that by increasing the heuristic processing of information, (H2a) the high-eeriness virtual physician elicits more thoughts related to the message source than the low-eeriness virtual physician and that (H2b) the low-eeriness virtual physician elicits more thoughts related to the message itself than the high-eeriness physician.
Fiske and colleagues proposed the stereotype content model (SCM), which describes the formation of interpersonal impressions and group stereotypes on two dimensions: warmth and competence (Fiske et al., 2002). Warmth encompasses traits indicating whether a person intends to help or harm, such as the presence or absence of friendliness, helpfulness, morality, sincerity, and trustworthiness. Competence encompasses traits indicating the ability or inability to act on that intention, such as the presence or absence of creativity, efficacy, intelligence, and skill (Fiske et al., 2002, 2007). A person viewed as warm and competent elicits positive emotions and behaviors, whereas a person viewed as lacking these traits elicits negative emotions and behaviors. These responses confer selective advantage to individuals and groups. Warmth is the primary dimension of interpersonal perception. It accounts for 53% of the variance in global impressions, while competence accounts for 29% (Wojciszke et al., 1998). Warmth and competence have been used to explain interpersonal and intergroup social cognition, especially as related to competition and status.
The physician’s warmth helps support the physician–patient relationship (Buller and Street, 1992). In clinical settings, it is exhibited by such affiliative behavior as attention to patients, empathy, and kindness. Affiliative behavior motivates patient adherence in real (Willson and McNamara, 1982; Kim et al., 2004) and virtual contexts (Dai and MacDorman, 2018). However, affiliative behavior does not ensure that patients will continue to follow the recommended treatment (O’Hair, 1986; Street, 1990; Buller and Street, 1992). We hypothesize (H3) greater adherence to the advice of a high-warmth physician than a low-warmth physician.
Warmth and competence are positively correlated when patients interact with physicians in clinical settings (Kraft-Todd et al., 2017). A rude, unfriendly physician may attract more attention than a polite, friendly one. Moreover, sarcasm and dark humor could make communication more entertaining and memorable (Ziegele and Jost, 2020). We hypothesize that (H4a) the low-warmth virtual physician elicits more source-related thoughts than the high-warmth virtual physician by encouraging the heuristic processing of information, and (H4b) the high-warmth virtual physician elicits more message-related thoughts than the low-warmth virtual physician by encouraging the systematic processing of information.
Our assumption may appear to run counter to findings in the persuasion literature, namely that warm, competent, or attractive sources of information elicit more heuristic processing than cold, incompetent, or unattractive ones (Chaiken, 1980, 1987; DeBono and Harnish, 1988; Wood and Kallgren, 1988). According to HSM, if a source is judged positively, less cognitive effort must be expended to evaluate the message. We justify our assumption based on the context of the clinical scenario: Patients expect their doctor to be warm and competent by default. This expectation is implied by the Hippocratic Oath. Behavior deviating from it is surprising, thus drawing attention away from the message to its source.
People’s attitudes shape their intentions, which, in turn, predict behavior change (Austin and Vancouver, 1996; Abraham et al., 1998; Webb and Sheeran, 2006; Montano and Kasprzyk, 2015). In general, a shift in attitudes resulting solely from heuristic processing will be less stable and less resistant to counterarguments and have less of an effect on behavior change than a shift resulting from systematic processing (Chaiken, 1980). Prochaska and DiClemente (1983) proposed the transtheoretical model of behavior change, which has been used to increase physical activity (Prochaska et al., 1994; Prochaska et al., 2015; Stonerock and Blumenthal, 2017). In their model, adherence intention is a major predictor of behavior change (Austin and Vancouver, 1996; Abraham et al., 1998; Webb and Sheeran, 2006; Montano and Kasprzyk, 2015).
This study investigates the effect of bedside manner on people’s behavior change in diet and physical activity. Good bedside manner in a virtual physician has been found to increase patients’ adherence and satisfaction (Schmid Mast et al., 2007; Dai and MacDorman, 2018). Based on these findings, we hypothesize that (H5a) the consultation with the high-warmth physician will improve diet and (H5b) physical activity more than the consultation with the low-warmth physician. Based on the literature on adherence, we hypothesize that (H6a) those reporting greater adherence intention after the virtual consultation will improve their diet and (H6b) increase their physical activity more than others.
To the best of our knowledge, the effect of a virtual character on persuasion has not yet been fully explored in a virtual clinical setting. In particular, the extent to which the physician’s warmth and eeriness affect heuristic and systematic processes and how these processes affect adherence intention and behavior change has not been considered. Our experiment manipulated a virtual doctor’s bedside manner and depiction to determine how these variables influence perceived warmth and eeriness, heuristic and systematic processing, adherence intention, and behavior change. We further explored these variables of the stereotype content model and the heuristic–systematic model in a path analysis to predict adherence intention and behavior change. Thus, our overarching research question is this: How does a physician’s bedside manner and depiction influence adherence intention and behavior change through the stereotype content model and heuristic–systematic model?
Our previous study examined how bedside manner, outcome (i.e., being awarded a fellowship or sued for malpractice), and virtual depiction affected adherence intention in a virtual clinical scenario (Dai and MacDorman, 2018). Its findings revealed two main threats to interval validity resulting from the experimental design. First, poor bedside manner caused the doctor to be rated significantly higher in eeriness than good bedside manner. The eeriness index was not designed as a posttest for comparing characters whose responses differ greatly in warmth or other traits between scenarios. To eliminate the effect of bedside manner on eeriness, this study measured the doctor’s eeriness in a neutral setting as a pretest (see Procedure). The second threat to internal validity was that our previous study failed to separate the computer-animated doctor’s positive effect on consultation enjoyment and adherence intention from the uncanny valley’s negative effect. To address this threat, this study used multiple versions of the computer-animated doctor to measure eeriness. Finally, our previous study did not measure behavior change. This study measured dietary and exercise change at least 1 week after the virtual consultation.
The experiment centered on an online virtual consultation. Participants took on the role of a patient in a doctor’s consultation regarding a possible diabetes diagnosis. The virtual doctor was manipulated by bedside manner and depiction. Bedside manner had two stimulus conditions: either good or poor. Depiction had five stimulus conditions that varied in their level of realism: cartoon, bigeye, swapped, animation, and real (Figure 1).
FIGURE 1. The doctor in the experiment was presented with five styles of depiction. These were, from left to right, (A) cartoon, (B) bigeye, (C) swapped, (D) animation, and (E) real. Real was a video of a real person. Animation was a 3-D computer animation. Swapped was a face replacement of animation using deep learning. Bigeye was also a face replacement but with eyes enlarged 50%. Cartoon was a cartoon version of animation. The props and background for bigeye and swapped were identical to animation.
These five conditions were selected to manipulate the virtual physician’s level of eeriness to measure the effect of eeriness on adherence intention and behavior change, as mediated by the stereotype content model and the heuristic–systematic model. The real condition used a video of a male actor playing the part of the doctor. This video was used as a reference by a professional animator to create the animation condition. A realistic computer model was first developed from high-resolution reference photos of the human actor. In a previous study, the same model had been rated significantly eerier than the real actor (Patel and MacDorman, 2015).
The animation condition served as a reference for the remaining three conditions. We created a cartoon character as a low-eeriness condition with flat shading and fewer textural features. This approach has been used to avoid the uncanny valley in game development (McDonnell and Breidt, 2010; McDonnell et al., 2012). FaceSwap, a deep learning neural network program, was used to generate characters with a swapped face (swapped) and a swapped face with eyes enlarged 50% (bigeye). The bigeye condition was motivated by the fact that viewers are prone to be sensitive to atypical features in human faces, especially when they involve the eyes (Kätsyri et al., 2015; Schein and Gray, 2015). Increasing the size of the eyes or making their level of realism inconsistent with other facial features causes the face to appear eerier (MacDorman et al., 2009; Seyama and Nagayama, 2009; MacDorman and Chattopadhyay, 2016; Feng et al., 2018).
Undergraduate and graduate students randomly selected from a public university system in the Midwestern United States comprised the sample. Each was randomly assigned to one of 10 groups.
A total of 441 participants completed the experiment and first survey (72% female, n = 318) with between 36 and 52 in each group. Among those participants, 329 completed a second follow-up survey, with between 28 and 38 in each group (73% female, n = 244). One participant’s responses were removed for not completing the thought-listing task. Participants ranged in age from 18 to 70 (Mdn = 22, IQR = [19, 28]), and most had grown up in the United States (89%, n = 394).
The power analysis aimed to determine how many participants each condition would require for the effect of depiction on pretest Eeriness to be sufficient to have a significant effect on Adherence Intention. In MacDorman et al. (2009), a 50% increase in eye size significantly increased eeriness, m1 = −1.60, SD1 = 1.22, m2 = 1.37, SD2 = 1.23,
In preparation for this experiment, 30 participants rated a virtual physician on Eeriness. The virtual physician was presented at three levels of realism in 20-s video clips. At the lowest level, the eyes were enlarged 50%. The effect of realism on Eeriness was significant and large, F (2, 58) = 15.34,
The experiment adopted a 2 × 5 between-groups posttest design, although the doctor’s perceived Eeriness was also pretested. The 10 treatment groups had five different stylizations of the virtual doctor, who had either good or poor bedside manner.
Participants first completed informed consent. To measure the eeriness of the virtual physician, Dr. Richards, independently from the bedside manner manipulation, participants rated the doctor on Eeriness in a short, 7-s clip. Dr. Richards was presented on the screen holding a clipboard. He wore a white lab coat over a white shirt with a red tie.
Participants were then introduced to the doctor–patient scenario and their role as patient. They began the consultation with a test result indicating higher-than-normal blood sugar.
Eight hypothetical doctor–patient interactions comprised the virtual consultation. For all but the last, the participant chose a preferred question from four options. For experimental control, the doctor provided the same response, regardless of the question selected, and the questions and responses were designed to create the impression of a logically flowing conversation. The doctor closed the consultation by providing the patient with a recommendation concerning diet and exercise in audio and text.
Next, the participant completed the indices (see Dependent variables) and a demographics questionnaire. The indices were presented in the following order: a thought-listing task; a fully randomized block of scales composed of human realism, eeriness, warmth, and competence; cognitive perspective-taking; adherence intention; diet; and physical activity. After at least 1 week, the diet and physical activity indices were administered a second time to measure behavior change.
The experiment was conducted from October 10 to November 8, 2019. The median duration of the experiment and first survey was 21 min 39 s (25th percentile = 18 min 2 s, 75th percentile = 29 min 35 s). For the virtual consultation, the duration of the physician’s part of the physician–patient interactions was 3 min 26 s for the physician with good bedside manner and 2 seconds longer for the physician with poor bedside manner. The average duration of the user’s part was 2 min 18 s for a total virtual consultation time of 5 min 45 s.
The independent variables were bedside manner (good or poor) and depiction (five levels of realism). Depiction was recoded as real (two levels) and 3-D animation (two levels) for planned contrasts. A transcript of the interactions including the bedside manner manipulation and diet and exercise recommendation is provided in Supplementary Material. All videos including the bedside manner and depiction manipulations are available at https://doi.org/10.6084/m9.figshare.13337267.
Bedside manner had two conditions (i.e., good or poor), which were designed to exhibit high warmth or low warmth, respectively. The doctor’s bedside manner was depicted mainly via dialogue (40% of the total), nonverbal expressions, and gestures. The high-warmth doctor treated the patient with care, empathy, and patience, responded to questions positively, provided reassurance and emotional support, and expressed a willingness to be available. By contrast, the low-warmth doctor was rude and impatient, joked about the patient’s diagnosis, and tried to end the consultation early. The low-warmth doctor showed little empathy for the patient, interacted paternalistically, and implied that the patient’s questions were ill-informed. These are the same qualities encountered in real physicians with good and poor bedside manner (Hickson, 1994; Levinson et al., 1997; Schmid Mast et al., 2007).
The depiction of the character was presented in five different rendering styles: cartoon, bigeye, swapped, animation, and real. The five styles were a video of a real person, a 3-D computer animation modeled on the video using Maya and ZBrush, a software-generated cartoon version of the animation, and face replacements of the animation generated by FaceSwap, which contained two versions (i.e., the face replacement with an animated face, and the same face with eyes enlarged 50%). Apart from character stylization, all five high-warmth conditions had the same narrative content, and all five low-warmth conditions had the same narrative content. The doctor’s mouth movements were synchronized to his voice.
Indices were used to measure the dependent variables. They are discussed below and listed in the Supplementary Material. Source- and message-related thoughts were determined by two coders who labeled and counted each participant’s thoughts. Dietary and exercise change were quantified by the participant who counted servings of food or hours of activity during the prior week. All other indices were composed of semantic differential scales (e.g., strongly agree–strongly disagree or creepy–ordinary). Each scale was depicted with a horizontal bar with a term on one end and its antonym on the other (see Reips and Funke, 2008; Funke and Reips, 2012). For each scale, the participant used a slider to position an indicator on the bar. The indicator’s position was recorded as a decimal value between –1.00 and 1.00.
To evaluate the appearance of the message source, Dr. Richards’ human realism was measured using the combined scales of the realism and humanness indices; eeriness was measured using the eeriness index’s eerie subindex (Ho and MacDorman, 2017).
Dr. Richards’ perceived warmth and competence were measured using a warmth index (Ho and MacDorman, 2010) and a competence index (McCroskey and Teven, 1999).
A thought-listing task was used to analyze the heuristic and systematic processing of information for persuasion (Chaiken, 1980; Pallak et al., 1983; Koh and Sundar, 2010). Chaiken and Maheswaran (1994) used the number of source-related thoughts to measure heuristic processing and the number of message-related thoughts to measure systematic processing. In the experiment, participants listed their thoughts about consultation content and Dr. Richards’ appearance. They were given 3 min and 10 text input boxes to record their thoughts. Two coders, blind to the conditions, independently assigned each thought to one of seven categories: positive, neutral, or negative source-related, positive, neutral, or negative health-related, or other. An index for source-related thoughts was calculated by adding up the number of thoughts in this category. The process was repeated for message-related thoughts, which were further divided into negative and nonnegative thoughts.
Participants’ ability to imagine Dr. Richards’ mental state (Davis, 1983; Leslie, 1987; Frith, 2001) was measured using a cognitive perspective-taking index designed for the virtual consultation’s specific clinical scenario (Dai and MacDorman, 2018). The index was composed of statements about Dr. Richards’ mental state paired with the semantic differential scale strongly agree–strongly disagree. Either strongly agree or strongly disagree corresponded to the correct answer. In that sense, the index was a disguised test. This variable was included as a second measure of information processing (DeBono and Harnish, 1988).
The intention to adhere to the doctor’s advice was measured using an adherence intention index designed for the clinical scenario (Dai and MacDorman, 2018).
Behavior change was measured by a survey on diet and physical activities, inspired by a lifestyle change survey for diabetic patients (Chong et al., 2017). Participants reported their consumption of vegetables, fruits, fatty and processed foods, and foods with added sugar for the prior week. These four items comprised a diet index. They also reported how many hours they spent walking and doing moderate and vigorous physical activities for the prior week. These three items comprised an index on physical activity. The indices were administered immediately after the experiment and at least 1 week later. The difference between their first and second administration constitutes the dietary change and exercise change indices.
For reliability thresholds, Cronbach’s α was interpreted with acceptable = 0.7, good = 0.8, and excellent = 0.9. For effect size thresholds, Cohen’s κ was interpreted with fair = 0.21, moderate = 0.41, substantial = 0.61, and perfect = 0.81 (Cohen, 1960; Landis and Koch, 1977), and partial eta squared (
The cognitive perspective-taking index was an unweighted average of its scales. To maximize variance explained, all other indices were averages of their respective scales weighted by their component loadings.
Test statistics were interpreted at the significance level p ≤ 0.05. For the manipulation checks and directional hypotheses, planned contrasts were one-tailed. All other tests were two-tailed. Linear mixed-effects models were fitted by maximum-likelihood estimation. Mixed-effects models were used instead of ANOVA and ANCOVA because they account for variability among groups. Planned contrasts were orthogonal and used type III sum of squares.
For path models, the standard for acceptable global fit required meeting the following criteria: p > 0.05 for model χ2, the root mean square error of approximation (RMSEA)
All indices were reliable (0.72 ≤ α ≤ 0.93). Item 7 was removed from the adherence intention index to increase its reliability.
In the thought-listing task, the two coders had substantial agreement in assigning one of the seven labels to each thought, κ = 0.73, agreeing on the labels for 75% of the thoughts. Labeling discrepancies were resolved through discussion.
The psychometric properties of the final indices are listed in Table 1. The initial indices and their factor loadings appear in the Supplementary Material. Correlations among independent and dependent variables (indices) are listed in Figure 2.
FIGURE 2. Pearson’s correlation between the dependent and independent variables. All correlations were significant except those indicated by white text on a gray background.
A two-way MANOVA indicated a significant effect of bedside manner, Pillai’s trace = 0.49, F (4, 432) = 105.00, p < 0.001, and depiction, V = 0.19, F (16, 1740) = 5.28, p < 0.001, on the manipulation check variables. Two-way mixed-effects models confirmed that bedside manner × depiction had only nonsignificant interaction effects on Human Realism, pretest Eeriness, Eeriness, Warmth, and Competence. Mixed-effects models with planned contrasts were used for the manipulation checks.
After controlling for the effect of depiction, bedside manner had a nonsignificant effect on Human Realism and pretest Eeriness, but a significant effect on posttest Eeriness, Warmth, and Competence (Table 2, n = 441). Planned contrasts revealed that the doctor with good bedside manner was rated significantly less eerie, warmer, and more competent than the doctor with poor bedside manner.
TABLE 2. Mixed-effects model of bedside manner and depiction on Human Realism, pretest Eeriness, Eeriness, Warmth, and Competence with planned contrasts.
After controlling for the effect of bedside manner, depiction had a significant effect on Human Realism and pretest Eeriness, but a nonsignificant effect on posttest Eeriness, Warmth, and Competence. The fact that bedside manner, not depiction, had a significant effect on posttest Eeriness indicates that not only is bedside manner a confounding variable, but that it renders nonsignificant the effect of depiction.
A planned contrast revealed that the real human doctor (i.e., real) was rated significantly higher in Human Realism than the others (i.e., cartoon, bigeye, swapped, and animation). Another planned contrast revealed that 3-D animation (i.e., bigeye, swapped, and animation) was rated significantly higher in pretest Eeriness than the others (i.e., cartoon, real). Figure 3 plots pretest Eeriness by depiction. Increasing levels of realism reproduced the characteristic U-shape of the uncanny valley (Mori, 2012; Ho and MacDorman, 2017).
Women have been found to be more responsive than men to specific persuasion strategies and scenarios (MacDorman et al., 2009; Orji et al., 2015). Their satisfaction is also more likely to increase when the physician exhibits good bedside manner (Schmid Mast et al., 2007). Thus, to ensure the generalizability of our results given that the participants were 72% female, we tested the effect of participant gender on all dependent variables used in the manipulation checks and in hypothesis testing. A MANOVA revealed that the effect of gender on these variables was nonsignificant.
A two-way mixed-effects model confirmed that bedside manner × depiction had only nonsignificant interaction effects on Adherence Intention, number of source- and message-related thoughts, Dietary Change, and Exercise Change. Mixed-effects models with planned contrasts were used in hypothesis testing. The results are shown in Table 3.
TABLE 3. Mixed-effects model of bedside manner and depiction on Adherence Intention, number of source- and message-related thoughts, Dietary Change, and Exercise Change with planned contrasts.
H1 states that the physician’s depiction affects the intention to adhere to his advice. After controlling for the effect of bedside manner, depiction had a nonsignificant effect on Adherence Intention. Thus, H1 was not supported.
H2a states that the high-eeriness physician elicits more source-related thoughts than the low-eeriness physician. High eeriness is operationalized as 3-D animation (i.e., bigeye, swapped, and animation) and low eeriness as cartoon and real. After controlling for the effect of bedside manner, depiction had a significant effect on the number of source-related thoughts. A planned contrast revealed that 3-D animation elicited significantly more source-related thoughts than cartoon and real (Table 3; Figure 4). Thus, H2a was supported.
FIGURE 4. Mean and 95% confidence interval of the number of source-related thoughts and number of message-related thoughts by bedside manner and depiction.
H2b states that the low-eeriness physician elicits more message-related thoughts than the high-eeriness physician. After controlling for the effect of bedside manner, depiction had a nonsignificant effect on the number of message-related thoughts. Thus, H2b was unsupported.
H3 states that patients would be more likely to adhere to the advice of the high-warmth physician than the low-warmth physician. After controlling for the effect of depiction, bedside manner had a significant effect on Adherence Intention. A planned contrast revealed that Adherence Intention was significantly higher for the doctor with good bedside manner (Table 3; Figure 5). Thus, H3 was supported.
H4a states that the low-warmth physician elicits more source-related thoughts than the high-warmth physician. After controlling for the effect of depiction, bedside manner had a significant effect on the number of source-related thoughts. A planned contrast revealed that the doctor with poor bedside manner elicited significantly more source-related thoughts. Thus, H4a was supported.
H4b states that the high-warmth physician elicits more message-related thoughts than a low-warmth physician. After controlling for the effect of depiction, bedside manner had a significant effect on the number of message-related thoughts. A planned contrast revealed that the doctor with good bedside manner elicited significantly more message-related thoughts. Thus, H4b was supported.
H5a states that the consultation with the high-warmth physician will improve diet more than the consultation with the low-warmth physician, and H5b states that it will also improve physical activity more than the consultation with the low-warmth physician. To operationalize behavior change, diet and exercise activity were measured immediately after the consultation and at least 1 week later. After controlling for the effect of depiction, bedside manner had a nonsignificant effect on Dietary Change (Table 3). However, after controlling for the effect of depiction, bedside manner had a significant effect on Exercise Change. A planned contrast revealed that good bedside manner increased Exercise Change more than poor bedside manner. Thus, although H5a was not supported, H5b was supported.
H6a states that those reporting greater adherence intention after the virtual consultation will improve their diet more than others, and H6b states that they will also increase their physical activity more than others. After controlling for the effect of bedside manner and depiction, Adherence Intention had a nonsignificant effect on Dietary Change and Exercise Change. Thus, neither H6a nor H6b were supported.
A regression analysis revealed that 3-D animation was a nonsignificant predictor of Adherence Intention and Exercise Change. Bedside manner was a significant predictor of Adherence Intention with a medium-to-large effect size, β = 0.48, t (439) = 11.39, p < 0.001, and explained a significant proportion of the variance, R2 = 0.23, adj. R2 = 0.23, F (1, 439) = 129.67. Bedside manner was a significant predictor of Exercise Change with a small effect size, β = 0.11, t (326) = 2.05, p = 0.042, and explained a significant proportion of the variance, R2 = 0.01, adj. R2 = 0.01, F (1, 326) = 4.18.
We next develop path models to address our overarching research question: How does a physician’s bedside manner and depiction influence adherence intention and behavior change through the stereotype content model and heuristic–systematic model?
The stereotype content model predicts that adherence intention and behavior change will be greater for the doctor perceived as having more interpersonal warmth because warmth indicates a willingness to help. Moreover, the uncanny valley effect predicts that a 3-D animated doctor elicits the perception of greater eeriness and less warmth than a real or cartoon doctor, which could decrease adherence intention and behavior change, owing to an aversive response. Hypothesis testing confirmed that over the course of a week, the doctor with good bedside manner increased participant’s physical activity significantly more than the doctor with poor bedside manner.
In a path model, a direct effect is a hypothesized directional relation between two variables, represented by an arrow. The relation’s strength is a parameter estimated by model identification. If no relation is hypothesized, there is no arrow, and the parameter is fixed to zero. By the parsimony principle, model specification begins with the simplest model with the highest priority effect and proceeds by adding the next highest priority effects incrementally (Kline, 2016).
We start with Exercise Change—the effect we seek to predict. Among the dependent variables, only cognitive perspective-taking had a significant direct effect on Exercise Change. Adherence Intention and Warmth were added in Model 1, followed by Competence in Model 2, and Warmth’s direct effect on Competence in Model 3. In Model 4—our final SCM model—the independent variables bedside manner and 3-D animation had significant direct effects on Warmth (Figure 6).
FIGURE 6. Model 4: Path model of Exercise Change with SCM variables. The blue arrow indicates a positive direct effect, and the red arrow indicates a negative direct effect. Next to each arrow, the standardized estimate of the parameter (β, γ, ζ, φ, ψ) indicates the strength of the direct effect, and the number of asterisks indicates its significance level: *p ≤ 0.05, **p ≤ 0.01, and ***p ≤ 0.001. The independent variables bedside manner (good or poor) and 3-D animation (two levels: either bigeye, swapped, and animation or cartoon and real) are the exogenous variables. The model had acceptable local and global fit (Table 4).
Models 1–4 had acceptable local fit (p ≤ 0.05 for β's and γ's). Table 4 shows that Models 1–4 also had acceptable global fit (p > 0.05 for model χ2, RMSEA
FIGURE 7. Model 4a: Path model of Exercise Change with SCM variables and pretest Eeriness. Pretest Eeriness was incorporated into Model 4. The model had acceptable local and global fit (Table 4).
The heuristic–systematic model predicts that the high-eeriness doctor is processed more heuristically, eliciting more source-related thoughts owing to its visual novelty. By contrast, the low-eeriness doctor is processed more systematically, eliciting more message-related thoughts. Thus, the low-eeriness doctor is predicted to promote adherence more because persuasion increases more and endures longer with the number and favorability of message-related thoughts (Greenwald, 1968). As before, we proceed by adding significant direct effects, this time to Model 4. Model 7—our final hybrid model—had acceptable local and global fit (Figure 8; Table 4).
FIGURE 8. Model 7: Path model of Exercise Change with both SCM and HSM variables. The number of source-related thoughts and message-related thoughts were incorporated into Model 4.
Based on the heuristic–systematic model, we found that number of source-related thoughts had a negative direct effect on Adherence Intention. We wanted to explore these effects in more detail but could not add more free parameters given the sample size. The N:q rule stipulates that the sample size should exceed the number of free parameters by a factor of at least 20 (Jackson, 2003). Given 441 participants, this set the upper bound on the number of free parameters at 22. So, we removed variables related to the stereotype content model, and instead focused on the heuristic–systematic model, dividing source- and message-related thoughts into negative or nonnegative ones. Model 11 was our final model using HSM variables (Figure 9). The model had acceptable local and global fit (Table 4). Model 11 shows that good bedside manner had a strong negative direct effect on number of negative source-related thoughts, which had a negative direct effect on Adherence Intention.
FIGURE 9. Model 11: Path model of Exercise Change with HSM variables. The model includes the number of negative and nonnegative source-related thoughts and the number of nonnegative message-related thoughts.
This research investigates how a character’s warmth and eeriness in a virtual clinical scenario impact adherence intention and behavior change. The 3-D computer-animated versions of the doctor in the virtual consultation had a nonsignificant effect on adherence intention (H1) and change in physical activity as compared with the real and cartoon versions; however, the doctor’s bedside manner significantly increased adherence intention (H3) and physical activity (H5b). These results indicate that a good bedside manner, which is perceived as warm and competent, has a much stronger effect on persuasion than the uncanny valley effect.
In the path analysis of Model 4, derived from the stereotype content model’s variables, 3-D animation slightly decreased warmth, while good bedside manner increased warmth significantly with a large effect size. This in turn increased perceived competence, adherence intention, cognitive perspective-taking, and physical activity (Figure 6). In Model 11, derived from the heuristic–systematic model’s variables, 3-D animation significantly increased negative source-related thoughts with a small effect size, which significantly decreased adherence intention, while good bedside manner significantly increased adherence intention (Figure 9).
We found roughly comparable adherence intention and exercise change in a virtual consultation using a 3-D computer animation as using a cartoon or real human, even though the 3-D rendering styles elicited the uncanny valley effect. The effect of the doctor’s bedside manner washed out that of the uncanny valley to such an extent that the effect of depiction on eeriness was nonsignificant in the posttest. This bodes well for the acceptance of virtual characters in clinical settings as compared with videos of real humans. Virtual characters have the added advantage of efficiency and controllability (Johnson et al., 2000; Kenny et al., 2007; Bickmore et al., 2010b). Interactions can be scripted and subsequently enhanced by artificial intelligence (AI) systems like IBM Watson Health. It would be worthwhile to determine a virtual character’s effect on persuasion in other clinical scenarios.
Adherence intention, however, was not an ideal predictor of exercise change. We found that cognitive perspective-taking instead directly predicted exercise change. Cognitive perspective-taking measured participants’ ability to infer the doctor’s mental state, including his thoughts, feelings, and beliefs. Most behavior change theories, including the most popular ones, share the idea that intention determines behavior. These include the theory of planned behavior, the theory of reasoned action, theories of attitude–behavior relations, goal theories, models of health behavior, and the technology acceptance model and its extensions (Ajzen, 1991; Eagly and Chaiken, 1993; Austin and Vancouver, 1996; Conner and Norman, 1996; Gollwitzer and Moskowitz, 1996; Abraham et al., 1998; Maddux, 1999; Hale et al., 2002; Webb and Sheeran, 2006; Tamilmani et al., 2020). In the path models, however, adherence intention only predicted exercise change indirectly, mediated by cognitive perspective-taking.
SCM variables like warmth and competence were far more predictive of adherence intention than HSM variables like the number of source- and message-related thoughts. This is surprising because SCM is not a theory of persuasion.
Source credibility (McGuire, 1978), which is determined by the source’s trustworthiness and competence (Kelman and Hovland, 1953), is crucial for persuasion (Petty and Wegener, 1998; Pornpitakpan, 2004; Patel and MacDorman, 2015). Positive and neutral thoughts increase persuasion, while negative thoughts reduce it (Greenwald, 1968; Petty et al., 2014). Thus, source- and message-related thoughts should increase persuasion as long as they are not negative (Chaiken, 1980; Chen and Chaiken, 1999; Griskevicius et al., 2010). Model 11 aligns with this characterization (Figure 9).
The doctor with poor bedside manner elicited significantly more source-related thoughts than the doctor with good bedside manner (H4a), while the doctor with good bedside manner elicited significantly more message-related thoughts (H4b, Table 3). This pattern also emerged in the path analysis (Figures 8, 9; Table 4). These results run counter to findings in the persuasion literature. HSM predicts that interpersonal warmth prompts heuristic processing, indicated by an increase in source-related thoughts (Chaiken, 1980, 1987; DeBono and Harnish, 1988; Wood and Kallgren, 1988; Horcajo et al., 2010) and that less warm, attractive, and competent sources prompt systematic processing (Chaiken, 1979; Pallak et al., 1983; Brownlow, 1992; Messner et al., 2008; Koh and Sundar, 2010). However, in a clinical setting, patients may expect good bedside manner; thus, a doctor who breaks this expectation could draw attention. Likewise, patients may not expect the doctor to appear eerie; thus, an eerie appearance could also draw attention.
The doctor’s interpersonal warmth increased his perceived competence and shifted thoughts from the source to the message, contrary to most HSM studies. In HSM, both warmth and competence are deemed heuristic cues, which prompt people to make decisions by rules of thumb learned from experience, such as trusting a source that looks and sounds authoritative (Abelson, 1976; Lin et al., 2016; Lin and Spence, 2018). The effects of heuristic cues tend to be fleeting because they are unlikely to elicit systematic processing of the message. They may increase adherence intention temporarily but without a lasting effect on behavior change. This aligns with Model 11, which shows that message-related thoughts have a stronger effect on adherence intention and exercise change than source-related thoughts.
In the medical literature, however, there is a close link between warmth and competence. When patients perceive their physicians as friendly and helpful, they also perceive them as competent, and they are less likely to sue them for malpractice (Charles et al., 1985; Shapiro et al., 1989; Hickson, 1994; Levinson et al., 1997). The physician’s warmth has a major influence on the patient–physician relationship. It prevents patient dissatisfaction, mistrust, and nonadherence (Cousin et al., 2013). Determining how SCM variables influence heuristic and systematic processing is crucial to the study of adherence because systematic processing tends to result in more enduring changes in attitude and intention—as well as changes in behavior that are more resistant to relapse (Cacioppo and Petty, 1984).
The results confirmed our earlier suspicion that interpersonal warmth has a confounding effect on measuring eeriness (Dai and MacDorman, 2018). Depiction affected pretest eeriness, but not posttest eeriness, which was instead affected by the doctor’s bedside manner, even when controlling for this variable. The manipulation check of pretest eeriness revealed that the 3-D computer-animated doctor, rendered in three different styles, was eerier than the real and cartoon doctors. Thus, eeriness should be measured in a pretest before an interactive scenario that manipulates warmth instead of in a posttest with other dependent variables.
This study builds on past research on how a relational agent’s characteristics influence credibility and trust in health counseling (Bickmore et al., 2005; Bickmore et al., 2010a; Bickmore and Gruber, 2010; Sillice et al., 2018) and pedagogy (Baylor and Kim, 2005). However, we varied warmth and realism systematically by using the same character interacting in the same way while controlling for other aspects of the scenario. By eliminating the effect of using virtual agents based on different characters, we could isolate the effect of warmth and realism on adherence.
Virtual consultations offer a cost-effective way to improve patient adherence and health literacy (Bickmore et al., 2009b; 2010c). Recent advances in AI, computer modeling and animation, and immersive games will enable the rapid development and deployment of virtual consultations via the Internet to computers and mobile devices. The advantages of AI-enabled virtual consultations include the ease of applying them to different conditions and treatments, speed of revision, and internationalization and localization for language, culture, and demographic group (DeSmet et al., 2014).
Concerning the effect of depiction on Adherence Intention, the study was underpowered. MacDorman et al. (2009) found that a 50% increase in eye size significantly increased Eeriness with a large effect size,
Cognitive perspective-taking was a better predictor of exercise change than adherence intention. It is unclear why this should be the case. Was understanding the situation from the doctor’s perspective predictive, or was this variable simply a correlate for understanding the message? Further research is needed. In addition, behavior change only occurred for physical activity, not diet. This pattern, however, is not uncommon in the literature (e.g., Anderson et al., 2010).
The use of self-reported scales to measure behavior change in participants could be inaccurate for various reasons, such as the fabricated purpose of the virtual consultation, the participants’ poor recollection of it, or their tendency to respond in a socially desirable way. Exercise change can be measured more accurately by taking a different approach, such as using a monitoring device for physical activity. These include accelerometers, pedometers, and heart rate monitors (Bravata et al., 2007; Rogers et al., 2014; Patel et al., 2015; Stonerock and Blumenthal, 2017; Jelen et al., 2020).
Although patients’ emotional responses to their health conditions can influence their attitudes and intention to follow health-related advice and their actual behavior change (Dillard and Shen, 2005; Krakow et al., 2018), variables like fear and anger were not measured in the current study. Emotions like fear, anxiety, and disgust are also known to correlate with the uncanny valley effect (Ho et al., 2008). Future research could examine how these variables affect the persuasiveness of virtual agents.
A major finding in the literature on persuasion is that a message will be less convincing if its source is evaluated negatively. Likewise, a major finding of the literature on the uncanny valley effect is that virtual characters that resemble humans will be evaluated negatively because of their eerie appearance. Therefore, it stands to reason that a human-looking virtual character should be less convincing than a real human. However, this study found no significant difference. Instead, it found that, in the context of a virtual doctor’s consultation, good bedside manner increased the intention to adhere to health advice and actual physical activity. The study also showed how bedside manner—a form of interpersonal warmth—had such an overriding effect on perceptions of the virtual character that a standard measure of eeriness was rendered invalid for use in a posttest. Although in the persuasion literature warmth typically elicits more thoughts about the message source, in the virtual consultation, the doctor with poor bedside manner or an eerie depiction elicited more source-related thoughts. Finally, contrary to the literature on behavior change, adherence intention was a poor predictor of exercise change compared with the ability to take the doctor’s perspective.
In 1970, Mori (2012) proposed that human replicas—like android robots—could appear eerie. This effect, known as the uncanny valley, has been confirmed by a meta-analysis (Diel et al., 2022). The present study reproduced this effect by using a 3-D computer-animated doctor in a virtual consultation with three different rendering styles. However, the impact of the uncanny valley on adherence intention and exercise change was negligible compared with bedside manner. Bedside manner also had a pronounced effect on two SCM variables, warmth and competence, and on the HSM variables number and valence of source- and message-related thoughts. Thus, although virtual characters can elicit the uncanny valley effect, in this study they were comparable to a video of a real human in their effect on adherence intention and exercise change.
The present study provides empirical findings that extend the theory and practice of persuasion to virtual clinical settings. SCM variables like warmth and competence were found to be important dimensions in designing persuasive computer-animated characters. Contrary to the literature, a physician with good bedside manner prompted greater systematic processing in HSM, which increased adherence intention and exercise change. Physician warmth predicted competence. Surprisingly, both warmth and competence were better predictors of adherence intention than HSM variables. Cognitive perspective-taking performed better in predicting exercise change than adherence intention. Depiction had much less of an impact on persuasion than the physician’s warmth and competence.
The analysis was performed in the R statistical computing environment (packages: effectsize, jmv, lavaan, lavaanPlot, psy, psych, pwr2, multcomp, nlme, performance). The dataset and R scripts for the analyses are available as Supplementary Material.
The studies involving human participants were reviewed and approved by Indiana University’s Office of Research Administration. Written informed consent for participation was not required for this study in accordance with the national legislation and the institutional requirements.
ZD and KM conceptualized and designed the experiments, and ZD conducted them. ZD and KM analyzed the data, prepared the tables and figures, and wrote and revised the manuscript. ZD reviewed the literature on persuasion and adherence. KM wrote the final R scripts and supervised the research.
The study was supported by the National Institutes of Health (P20 GM066402/GM/NIGMS NIH HHS/United States), an IUPUI Signature Center grant, Research Investment Funds grant, and Open Access Fund.
The authors declare that the research was conducted in the absence of any commercial or financial relationships that could be construed as a potential conflict of interest.
All claims expressed in this article are solely those of the authors and do not necessarily represent those of their affiliated organizations, or those of the publisher, the editors and the reviewers. Any product that may be evaluated in this article, or claim that may be made by its manufacturer, is not guaranteed or endorsed by the publisher.
We are grateful to Himalaya Patel, Lino Stephen, and Zebulun M. Wood for their help with producing the virtual consultation: Patel filmed and edited the consultation; Stephen modeled the doctor, his office and props and animated the doctor; and Wood shot reference photographs in preparation for computer modeling. We would also like to thank Danni Yang for coding data from the thought-listing task.
The Supplementary Material for this article can be found online at: https://www.frontiersin.org/articles/10.3389/frvir.2021.739038/full#supplementary-material
Abelson, R. P. (1976). “Script Processing in Attitude Formation and Decision Making,” in Cognition and Social Behavior (Oxford, England: Lawrence Erlbaum), 31–45.
Abraham, C., Sheeran, P., and Johnston, M. (1998). From Health Beliefs to Self-Regulation: Theoretical Advances in the Psychology of Action Control. Psychol. Health 13, 569–591. doi:10.1080/08870449808407420
Ajzen, I. (1991). The Theory of Planned Behavior. Organ. Behav. Hum. Decis. Process. 50, 179–211. doi:10.1016/0749-5978(91)90020-T
Anderson, R., Anderson, D., and Hurst, C. (2010). Modeling Factors that Influence Exercise and Dietary Change Among Midlife Australian Women: Results from the Healthy Aging of Women Study. Maturitas 67, 151–158. doi:10.1016/j.maturitas.2010.06.007
André, E., Bevacqua, E., Heylen, D., Niewiadomski, R., Pelachaud, C., Peters, C., et al. (2011). “Non-verbal Persuasion and Communication in an Affective Agent,” in Emotion-Oriented Systems: The Humaine Handbook. Editors R. Cowie, C. Pelachaud, and P. Petta (Berlin, Heidelberg: Springer), 585–608. doi:10.1007/978-3-642-15184-2_30
Austin, J. T., and Vancouver, J. B. (1996). Goal Constructs in Psychology: Structure, Process, and Content. Psychol. Bull. 120, 338–375. doi:10.1037/0033-2909.120.3.338
Baylor, A. L., and Kim, Y. (2005). Simulating Instructional Roles through Pedagogical Agents. Int. J. Artif. Intell. Edu. 15, 95–115. doi:10.1007/s40593-015-0055-y
Bickmore, T., and Gruber, A. (2010). Relational Agents in Clinical Psychiatry. Harv. Rev. Psychiatry 18, 119–130. doi:10.3109/10673221003707538
Bickmore, T., Schulman, D., and Yin, L. (2010a). Maintaining Engagement in Long-Term Interventions with Relational Agents. Appl. Artif. Intell. 24, 648–666. doi:10.1080/08839514.2010.492259
Bickmore, T. W., Caruso, L., Clough-Gorr, K., and Heeren, T. (2005). ‘It's Just like You Talk to a Friend' Relational Agents for Older Adults. Interact. Comput. 17, 711–735. doi:10.1016/j.intcom.2005.09.002
Bickmore, T. W., Pfeifer, L. M., Byron, D., Forsythe, S., Henault, L. E., Jack, B. W., et al. (2010b). Usability of Conversational Agents by Patients with Inadequate Health Literacy: Evidence from Two Clinical Trials. J. Health Commun. 15, 197–210. doi:10.1080/10810730.2010.499991
Bickmore, T. W., Pfeifer, L. M., and Jack, B. W. (2009b). “Taking the Time to Care,” in Proceedings of the SIGCHI Conference on Human Factors in Computing Systems, Boston, Massachusetts, April 4–9, 2009 (New York: ACM), 1265–1274. doi:10.1145/1518701.1518891
Bickmore, T. W., Pfeifer, L. M., and Paasche-Orlow, M. K. (2009a). Using Computer Agents to Explain Medical Documents to Patients with Low Health Literacy. Patient Edu. Couns. 75, 315–320. doi:10.1016/j.pec.2009.02.007
Bickmore, T. W., Puskar, K., Schlenk, E. A., Pfeifer, L. M., and Sereika, S. M. (2010c). Maintaining Reality: Relational Agents for Antipsychotic Medication Adherence. Interact. Comput. 22, 276–288. doi:10.1016/j.intcom.2010.02.001
Bravata, D. M., Smith-Spangler, C., Sundaram, V., Gienger, A. L., Lin, N., Lewis, R., et al. (2007). Using Pedometers to Increase Physical Activity and Improve Health. JAMA 298, 2296–2304. doi:10.1001/jama.298.19.2296
Brown, M., O'Neill, N., van Woerden, H., Eslambolchilar, P., Jones, M., and John, A. (2016). Gamification and Adherence to Web-Based Mental Health Interventions: A Systematic Review. JMIR Ment. Health 3, e39. doi:10.2196/mental.5710
Brownlow, S. (1992). Seeing Is Believing: Facial Appearance, Credibility, and Attitude Change. J. Nonverbal Behav. 16, 101–115. doi:10.1007/BF00990325
Buller, D. B., and Street, R. L. (1992). “Physician–patient Relationships,” in Applications of Nonverbal Behavioral Theories and Research. Editor R. S. Feldman (New York: Psychology Press), 119–142.
Cacioppo, J. T., and Petty, R. E. (1984). The Elaboration Likelihood Model of Persuasion. Adv. Consumer Res. 11, 673–675.
Carter, E. J., Mahler, M., and Hodgins, J. K. (2013). “Unpleasantness of Animated Characters Corresponds to Increased Viewer Attention to Faces,” in Proceedings of the ACM Symposium on Applied Perception, Dublin, Ireland: August 22–23, 2013, 35–40. doi:10.1145/2492494.2502059
Chaiken, S. (1987). “The Heuristic Model of Persuasion,” in Social Influence: The Ontario Symposium. Editors M. P. Zanna, J. M. Olson, and C. P. Herman (New York: Lawrence Erlbaum), 5, 3–39. doi:10.1037/0022-3514.66.3.460
Chaiken, S. (1979). Communicator Physical Attractiveness and Persuasion. J. Personal. Soc. Psychol. 37, 1387–1397. doi:10.1037/0022-3514.37.8.1387
Chaiken, S., and Eagly, A. H. (1983). Communication Modality as a Determinant of Persuasion: The Role of Communicator Salience. J. Personal. Soc. Psychol. 45, 241–256. doi:10.1037/0022-3514.45.2.241
Chaiken, S. (1980). Heuristic versus Systematic Information Processing and the Use of Source versus Message Cues in Persuasion. J. Personal. Soc. Psychol. 39, 752–766. doi:10.1037/0022-3514.39.5.752
Chaiken, S., and Maheswaran, D. (1994). Heuristic Processing Can Bias Systematic Processing: Effects of Source Credibility, Argument Ambiguity, and Task Importance on Attitude Judgment. J. Personal. Soc. Psychol. 66, 460–473. doi:10.1037/0022-3514.66.3.460
Charles, S. C., Wilbert, J. R., and Franke, K. J. (1985). Sued and Nonsued Physicians' Self-Reported Reactions to Malpractice Litigation. Am. J. Psychiatry 142, 437–440. doi:10.1176/ajp.142.4.437
Chattopadhyay, D., and MacDorman, K. F. (2016). Familiar Faces Rendered Strange: Why Inconsistent Realism Drives Characters into the Uncanny Valley. J. Vis. 16 (7), 7–25. doi:10.1167/16.11.7
Chen, S., and Chaiken, S. (1999). “The Heuristic-Systematic Model in its Broader Context,” in Dual–process Theories in Social Psychology. Editors S. Chaiken, and Y. Trope (New York: The Guilford Press), 73–96.
Chisholm-Burns, M. A., and Spivey, C. A. (2012). The ‘Cost' of Medication Nonadherence: Consequences We Cannot Afford to Accept. J. Am. Pharm. Assoc. 52, 823–826. doi:10.1331/JAPhA.2012.11088
Chong, S., Ding, D., Byun, R., Comino, E., Bauman, A., and Jalaludin, B. (2017). Lifestyle Changes after a Diagnosis of Type 2 Diabetes. Diabetes Spectr. 30, 43–50. doi:10.2337/ds15-0044
Cohen, J. (1960). A Coefficient of Agreement for Nominal Scales. Educ. Psychol. Meas. 20, 37–46. doi:10.1177/001316446002000104
Cohen, J. (1973). Eta-squared and Partial Eta-Squared in Fixed Factor ANOVA Designs. Educ. Psychol. Meas. 33, 107–112. doi:10.1177/001316447303300111
Conner, M., and Norman, P. (1996). The Role of Social Cognition in Health Behaviours. Maidenhead, England: Open University Press.
Cousin, G., Schmid Mast, M., and Jaunin-Stalder, N. (2013). Finding the Right Interactional Temperature: Do Colder Patients Need More Warmth in Physician Communication Style?. Soc. Sci. Med. 98, 18–23. doi:10.1016/j.socscimed.2013.08.034
Cutler, R. L., Fernandez-Llimos, F., Frommer, M., Benrimoj, C., and Garcia-Cardenas, V. (2018). Economic Impact of Medication Non-adherence by Disease Groups: A Systematic Review. BMJ Open 8, e016982. doi:10.1136/bmjopen-2017-016982
Dai, Z., and MacDorman, K. F. (2018). The Doctor's Digital Double: How Warmth, Competence, and Animation Promote Adherence Intention. PeerJ Comput. Sci. 4, e168. doi:10.7717/peerj-cs.168
Davis, M. H. (1983). Measuring Individual Differences in Empathy: Evidence for a Multidimensional Approach. J. Personal. Soc. Psychol. 44, 113–126. doi:10.1037/0022-3514.44.1.113
DeBono, K. G., and Harnish, R. J. (1988). Source Expertise, Source Attractiveness, and the Processing of Persuasive Information: A Functional Approach. J. Personal. Soc. Psychol. 55, 541–546. doi:10.1037/0022-3514.55.4.541
DeSmet, A., Van Ryckeghem, D., Compernolle, S., Baranowski, T., Thompson, D., Crombez, G., et al. (2014). A Meta-Analysis of Serious Digital Games for Healthy Lifestyle Promotion. Prev. Med. 69, 95–107. doi:10.1016/j.ypmed.2014.08.026
Diel, A., and MacDorman, K. F. (2021). Creepy Cats and Strange High Houses: Support for Configural Processing in Testing Predictions of Nine Uncanny Valley Theories. J. Vis. 21, 1–20. doi:10.1167/jov.21.4.1
Diel, A., Weigelt, S., and MacDorman, K. F. (2022). A Meta-Analysis of the Uncanny Valley's Independent and Dependent Variables. ACM Trans. Human Robot Interact. 11, 1–38. doi:10.1145/3470742
Dillard, J. P., and Shen, L. (2005). On the Nature of Reactance and its Role in Persuasive Health Communication. Commun. Monogr. 72, 144–168. doi:10.1080/03637750500111815
Eagly, A. H., and Chaiken, S. (1993). The Psychology of Attitudes. Orlando, FL: Harcourt Brace Jovanovich.
Eriksson, K.-F., and Lindgärde, F. (1991). Prevention of Type 2 (Non-insulin-dependent) Diabetes Mellitus by Diet and Physical Exercise: The 6-year Malmo Feasibility Study. Diabetologia 34, 891–898. doi:10.1007/BF00400196
Ettner, S. L. (1999). The Relationship Between Continuity of Care and the Health Behaviors of Patients. Med. Care 37, 547–555. doi:10.1097/00005650-199906000-00004
Feng, S., Wang, X., Wang, Q., Fang, J., Wu, Y., Yi, L., et al. (2018). The Uncanny Valley Effect in Typically Developing Children and its Absence in Children with Autism Spectrum Disorders. PLOS ONE 13, e0206343. doi:10.1371/journal.pone.0206343
Fiske, S. T., Cuddy, A. J. C., and Glick, P. (2007). Universal Dimensions of Social Cognition: Warmth and Competence. Trends Cogn. Sci. 11, 77–83. doi:10.1016/j.tics.2006.11.005
Fiske, S. T., Cuddy, A. J. C., Glick, P., and Xu, J. (2002). A Model of (Often Mixed) Stereotype Content: Competence and Warmth Respectively Follow from Perceived Status and Competition. J. Personal. Soc. Psychol. 82, 878–902. doi:10.1037/0022-3514.82.6.878
Frith, U. (2001). Mind Blindness and the Brain in Autism. Neuron 32, 969–979. doi:10.1016/S0896-6273(01)00552-9
Funke, F., and Reips, U.-D. (2012). Why Semantic Differentials in Web-Based Research Should Be Made from Visual Analogue Scales and Not from 5-point Scales. Field Methods 24, 310–327. doi:10.1177/1525822X12444061
Gollwitzer, P. M., and Moskowitz, G. B. (1996). “Goal Effects on Action and Cognition,” in Social Psychology: Handbook of Basic Principles (New York: The Guilford Press), 361–399.
Green, R. D., MacDorman, K. F., Ho, C.-C., and Vasudevan, S. (2008). Sensitivity to the Proportions of Faces that Vary in Human Likeness. Comput. Hum. Behav. 24, 2456–2474. doi:10.1016/j.chb.2008.02.019
Greenwald, A. G. (1968). Cognitive Learning, Cognitive Response to Persuasion, and Attitude Change. New York: Academic Press.
Griskevicius, V., Shiota, M. N., and Neufeld, S. L. (2010). Influence of Different Positive Emotions on Persuasion Processing: A Functional Evolutionary Approach. Emotion 10, 190–206. doi:10.1037/a0018421
Hale, J. L., Householder, B. J., and Greene, K. L. (2002). “The Theory of Reasoned Action,” in The Persuasion Handbook: Developments in Theory and Practice. Editors J. P. Dillard, and L. Shen (Thousand Oaks, CA: Sage Publication), 14, 259–286.
Hickson, G. B. (1994). Obstetricians' Prior Malpractice Experience and Patients' Satisfaction with Care. JAMA 272, 1583–1587. doi:10.1001/jama.1994.03520200039032
Ho, C.-C., and MacDorman, K. F. (2017). Measuring the Uncanny Valley Effect. Int. J. Soc. Robot. 9, 129–139. doi:10.1007/s12369-016-0380-9
Ho, C.-C., MacDorman, K. F., and Pramono, Z. A. D. D. (2008). “Human Emotion and the Uncanny Valley,” in Proceedings of the 3rd ACM/IEEE International Conference on Human Robot Interaction, Amsterdam, March 12–15, 2008 (New York: ACM), 169–176. doi:10.1145/1349822.1349845
Ho, C.-C., and MacDorman, K. F. (2010). Revisiting the Uncanny Valley Theory: Developing and Validating an Alternative to the Godspeed Indices. Comput. Hum. Behav. 26, 1508–1518. doi:10.1016/j.chb.2010.05.015
Ho, P. M., Rumsfeld, J. S., Masoudi, F. A., McClure, D. L., Plomondon, M. E., Steiner, J. F., et al. (2006). Effect of Medication Nonadherence on Hospitalization and Mortality Among Patients with Diabetes Mellitus. Arch. Intern. Med. 166, 1836–1841. doi:10.1001/archinte.166.17.1836
Horcajo, J., Petty, R. E., and Briñol, P. (2010). The Effects of Majority versus Minority Source Status on Persuasion: A Self-Validation Analysis. J. Personal. Soc. Psychol. 99, 498–512. doi:10.1037/a0018626
Hu, L. t., and Bentler, P. M. (1999). Cutoff Criteria for Fit Indexes in Covariance Structure Analysis: Conventional Criteria versus New Alternatives. Struct. Equ. Model. A Multidis. J. 6, 1–55. doi:10.1080/10705519909540118
Jackson, D. L. (2003). Revisiting Sample Size and Number of Parameter Estimates: Some Support for the N:q Hypothesis. Struct. Equ. Model. A Multidis. J. 10, 128–141. doi:10.1207/S15328007SEM1001_6
Jelen, B., Richards, O. K., Whitman, S. A., Ongwere, T., Kresnye, K. C., and Siek, K. A. (2020). “Exploring the Use of Electronics to Customize Pervasive Health Technologies with Older Adult Crafters,” in Proceedings of the 14th EAI International Conference on Pervasive Computing Technologies for Healthcare, October 6–8, 2020 (New York: ACM), 166–178. doi:10.1145/3421937.3421976
Johnson, W. L., Rickel, J. W., and Lester, J. C. (2000). Animated Pedagogical Agents: Face-To-Face Interaction in Interactive Learning Environments. Int. J. Artif. Intell. Edu. 11, 47–78.
Kätsyri, J., Forger, K., Mäkäräinen, M., and Takala, T. (2015). A Review of Empirical Evidence on Different Uncanny Valley Hypotheses: Support for Perceptual Mismatch as One Road to the Valley of Eeriness. Front. Psychol. 6, 390. doi:10.3389/fpsyg.2015.00390
Kelman, H. C., and Hovland, C. I. (1953). “Reinstatement” of the Communicator in Delayed Measurement of Opinion Change. J. Abnormal Soc. Psychol. 48, 327–335. doi:10.1037/h0061861
Kenny, P., Parsons, T. D., Gratch, J., Leuski, A., and Rizzo, A. A. (2007). “Virtual Patients for Clinical Therapist Skills Training,” in International Workshop on Intelligent Virtual Agents (Berlin: Springer), 197–210. doi:10.1007/978-3-540-74997-4_19
Kim, S. S., Kaplowitz, S., and Johnston, M. V. (2004). The Effects of Physician Empathy on Patient Satisfaction and Compliance. Eval. Health Prof. 27, 237–251. doi:10.1177/0163278704267037
Kirch, D. G., and Petelle, K. (2017). Addressing the Physician Shortage. JAMA 317, 1947–1948. doi:10.1001/jama.2017.2714
Kleinsinger, F. (2018). The Unmet Challenge of Medication Nonadherence. Perm J. 22, 83–85. doi:10.7812/TPP/18-033
Kline, R. B. (2016). Principles and Practice of Structural Equation Modeling. New York: Guilford Press.
Koh, Y. J., and Sundar, S. S. (2010). Heuristic Versus Systematic Processing of Specialist Versus Generalist Sources in Online Media. Hum. Commun. Res. 36, 103–124. doi:10.1111/j.1468-2958.2010.01370.x
Kraft-Todd, G. T., Reinero, D. A., Kelley, J. M., Heberlein, A. S., Baer, L., and Riess, H. (2017). Empathic Nonverbal Behavior Increases Ratings of Both Warmth and Competence in a Medical Context. PLOS ONE 12, e0177758. doi:10.1371/journal.pone.0177758
Krakow, M. M., Yale, R. N., Jensen, J. D., Carcioppolo, N., and Ratcliff, C. L. (2018). Comparing Mediational Pathways for Narrative- and Argument-Based Messages: Believability, Counterarguing, and Emotional Reaction. Hum. Commun. Res. 44, 299–321. doi:10.1093/hcr/hqy002
Landis, J. R., and Koch, G. G. (1977). The Measurement of Observer Agreement for Categorical Data. Biometrics 33, 159–174. doi:10.2307/2529310
Leslie, A. M. (1987). Pretense and Representation: The Origins of "theory of mind.". Psychol. Rev. 94, 412–426. doi:10.1037/0033-295X.94.4.412
Levinson, W., Roter, D. L., Mullooly, J. P., Dull, V. T., and Frankel, R. M. (1997). Physician-patient Communication. The Relationship with Malpractice Claims Among Primary Care Physicians and Surgeons. JAMA 277, 553–559. doi:10.1001/jama.1997.0354031005103410.1001/jama.277.7.553
Lewis, M. A., and Rook, K. S. (1999). Social Control in Personal Relationships: Impact on Health Behaviors and Psychological Distress. Health Psychol. 18, 63–71. doi:10.1037/0278-6133.18.1.63
Lin, X., and Spence, P. R. (2018). Identity on Social Networks as a Cue: Identity, Retweets, and Credibility. Commun. Stud. 69, 461–482. doi:10.1080/10510974.2018.1489295
Lin, X., Spence, P. R., and Lachlan, K. A. (2016). Social Media and Credibility Indicators: The Effect of Influence Cues. Comput. Hum. Behav. 63, 264–271. doi:10.1016/j.chb.2016.05.002
Looije, R., Neerincx, M. A., and Cnossen, F. (2010). Persuasive Robotic Assistant for Health Self-Management of Older Adults: Design and Evaluation of Social Behaviors. Int. J. Human Comp. Stud. 68, 386–397. doi:10.1016/j.ijhcs.2009.08.007
MacCallum, R. C., Browne, M. W., and Sugawara, H. M. (1996). Power Analysis and Determination of Sample Size for Covariance Structure Modeling. Psychol. Methods 1, 130–149. doi:10.1037/1082-989X.1.2.130
MacDorman, K. F., and Chattopadhyay, D. (2016). Reducing Consistency in Human Realism Increases the Uncanny Valley Effect; Increasing Category Uncertainty Does Not. Cognition 146, 190–205. doi:10.1016/j.cognition.2015.09.019
MacDorman, K. F., Green, R. D., Ho, C.-C., and Koch, C. T. (2009). Too Real for comfort? Uncanny Responses to Computer Generated Faces. Comput. Hum. Behav. 25, 695–710. doi:10.1016/j.chb.2008.12.026
MacDorman, K. F. (2019). In the Uncanny Valley, Transportation Predicts Narrative Enjoyment More Than Empathy, but Only for the Tragic Hero. Comput. Hum. Behav. 94, 140–153. doi:10.1016/j.chb.2019.01.011
Maddux, J. E. (1999). “Expectancies and the Social-Cognitive Perspective: Basic Principles, Processes, and Variables,” in How Expectancies Shape Experience. Editor I. Kirsch (Washington, DC: APA), 17–39. doi:10.1037/10332-001
Marć, M., Bartosiewicz, A., Burzyńska, J., Chmiel, Z., and Januszewicz, P. (2019). A Nursing Shortage – A Prospect of Global and Local Policies. Int. Nurs. Rev. 66, 9–16. doi:10.1111/inr.12473
Martin, L. R., Williams, S. L., Haskard, K. B., and DiMatteo, M. R. (2005). The Challenge of Patient Adherence. Ther. Clin. Risk Manag. 1, 189–199.
McCroskey, J. C., and Teven, J. J. (1999). Goodwill: A Reexamination of the Construct and its Measurement. Commun. Monogr. 66, 90–103. doi:10.1080/03637759909376464
McDonald, H. P., Garg, A. X., and Haynes, R. B. (2002). Interventions to Enhance Patient Adherence to Medication Prescriptions. JAMA 288, 2868–2879. doi:10.1001/jama.288.22.2868
McDonnell, R., Breidt, M., and Bülthoff, H. H. (2012). Render Me Real?. ACM Trans. Graph. 31, 1–11. doi:10.1145/2185520.2185587
McDonnell, R., and Breidt, M. (2010). “Face Reality,” in ACM SIGGRAPH ASIA 2010 Sketches, Seoul, Korea, December 15–18, 2010 (New York: ACM), 1–2. doi:10.1145/1899950.1899991
McGuire, M., and Iuga, M. J. (2014). Adherence and Health Care Costs. Risk Manag. Healthc. Policy 7, 35–44. doi:10.2147/RMHP.S19801
McGuire, W. J. (1978). “An Information-Processing Model of Advertising Effectiveness,” in Behavioral and Management Science in Marketing (New York: Ronald Press), 156–180.
McKechnie, T. (2016). Call for Nurses: The Multi-Dimensional Manifestation of Nursing Shortage on Patient Care. QIHI J. Healthc. Improvement Patient Saf. 2 (2).
Messner, M., Reinhard, M. A., and Sporer, S. L. (2008). Compliance through Direct Persuasive Appeals: The Moderating Role of Communicator's Attractiveness in Interpersonal Persuasion. Soc. Influ. 3, 67–83. doi:10.1080/15534510802045261
Miller, N. H. (1997). Compliance with Treatment Regimens in Chronic Asymptomatic Diseases. Am. J. Med. 102, 43–49. doi:10.1016/S0002-9343(97)00467-1
Miller, N., Maruyama, G., Beaber, R. J., and Valone, K. (1976). Speed of Speech and Persuasion. J. Personal. Soc. Psychol. 34, 615–624. doi:10.1037/0022-3514.34.4.615
Montano, D. E., and Kasprzyk, D. (2015). “Theory of Reasoned Action, Theory of Planned Behavior, and the Integrated Behavioral Model,” in Health Behavior: Theory, Research and Practice (San Francisco: Jossey-Bass), 95–124.
Morello, C. M., and Hirsch, J. D. (2017). Strategies for Addressing the Cost of Nonadherence in Diabetes. Am. J. Manag. Care 23, S247–S252.
Mori, M., MacDorman, K. F., and Kageki, N. (2012). The Uncanny Valley [From the Field]. IEEE Robot. Automat. Mag. 19, 98–100. doi:10.1109/MRA.2012.2192811
Nelson, K. M., Reiber, G., and Boyko, E. J. (2002). Diet and Exercise Among Adults with Type 2 Diabetes: Findings from the Third National Health and Nutrition Examination Survey (NHANES III). Diabetes Care 25, 1722–1728. doi:10.2337/diacare.25.10.1722
Ogawa, K., Bartneck, C., Sakamoto, D., Kanda, T., Ono, T., and Ishiguro, H. (2018). “Can an Android Persuade You?,” in Geminoid Studies: Science and Technologies for Humanlike Teleoperated Androids (Singapore: Springer), 235–247. doi:10.1007/978-981-10-8702-8_14
Orji, R., Mandryk, R. L., and Vassileva, J. (2015). “Gender, Age, and Responsiveness to Cialdini's Persuasion Strategies,” in Persuasive Technology, Lecture Notes in Computer Science. Editors T. MacTavish, and S. Basapur (Cham, Switzerland: Springer), 147–159. doi:10.1007/978-3-319-20306-5_14
Pallak, S. R., Murroni, E., and Koch, J. (1983). Communicator Attractiveness and Expertise, Emotional versus Rational Appeals, and Persuasion: A Heuristic versus Systematic Processing Interpretation. Soc. Cogn. 2, 122–141. doi:10.1521/soco.1983.2.2.122
Patel, H., Bayliss, L. C., Ivory, J. D., Woodard, K., McCarthy, A., and MacDorman, K. F. (2014). Receptive to Bad Reception: Jerky Motion Can Make Persuasive Messages More Effective. Comput. Hum. Behav. 32, 32–39. doi:10.1016/j.chb.2013.11.012
Patel, H., and MacDorman, K. F. (2015). Sending an Avatar to Do a Human's Job: Compliance with Authority Persists Despite the Uncanny Valley. Presence. Teleop. Vir. Environ. 24, 1–23. doi:10.1162/PRES_a_00212
Patel, M. S., Asch, D. A., and Volpp, K. G. (2015). Wearable Devices as Facilitators, Not Drivers, of Health Behavior Change. JAMA 313, 459–460. doi:10.1001/jama.2014.14781
Petterson, S. M., Liaw, W. R., Phillips, R. L., Rabin, D. L., Meyers, D. S., and Bazemore, A. W. (2012). Projecting US Primary Care Physician Workforce Needs: 2010-2025. Ann. Fam. Med. 10, 503–509. doi:10.1370/afm.1431
Petty, R. E., and Wegener, D. T. (1998). Matching versus Mismatching Attitude Functions: Implications for Scrutiny of Persuasive Messages. Pers Soc. Psychol. Bull. 24, 227–240. doi:10.1177/0146167298243001
Petty, R., Ostrom, T. M., and Brock, T. C. (2014). Cognitive Responses in Persuasion. New York: Psychology Press.
Pornpitakpan, C. (2004). The Persuasiveness of Source Credibility: A Critical Review of Five Decades' Evidence. J. Appl. Soc. Pyschol. 34, 243–281. doi:10.1111/j.1559-1816.2004.tb02547.x
Prochaska, J. O., and DiClemente, C. C. (1983). Stages and Processes of Self-Change of Smoking: Toward an Integrative Model of Change. J. Consult. Clin. Psychol. 51, 390–395. doi:10.1037/0022-006X.51.3.390
Prochaska, J. O., and Marcus, B. H. (1994). “The Transtheoretical Model: Applications to Exercise,” in Advances in Exercise Adherence. Editor R. K. Dishman (Champaign, Illinois: Human Kinetics), 161–180.
Prochaska, J. O., Redding, C. A., and Evers, K. E. (2015). “The Transtheoretical Model and Stages of Change,” in Health Behavior: Theory, Research, and Practice (San Francisco: Jossey-Bass), 125–148.
Reips, U.-D., and Funke, F. (2008). Interval-level Measurement with Visual Analogue Scales in Internet-Based Research: VAS Generator. Behav. Res. Methods 40, 699–704. doi:10.3758/BRM.40.3.699
Rejeski, W. J., Ip, E. H., Bertoni, A. G., Bray, G. A., Evans, G., Gregg, E. W., et al. (2012). Lifestyle Change and Mobility in Obese Adults with Type 2 Diabetes. N. Engl. J. Med. 366, 1209–1217. doi:10.1056/NEJMoa1110294
Richards, D., and Caldwell, P. H. (2016). “Gamification to Improve Adherence to Clinical Treatment Advice: Improving Adherence to Clinical Treatment,” in Handbook of Research on Holistic Perspectives in Gamification for Clinical Practice (Hershey, PA: IGI Global), 47–77.
Rogers, T., Milkman, K. L., and Volpp, K. G. (2014). Commitment Devices. JAMA 311, 2065–2066. doi:10.1001/jama.2014.3485
Saeedi, P., Petersohn, I., Salpea, P., Malanda, B., Karuranga, S., Unwin, N., et al. (2019). Global and Regional Diabetes Prevalence Estimates for 2019 and Projections for 2030 and 2045: Results from the International Diabetes Federation Diabetes Atlas, 9th Edition. Diabetes Res. Clin. Pract. 157, 107843. doi:10.1016/j.diabres.2019.107843
Safran, D. G., Taira, D. A., Rogers, W. H., Kosinski, M., Ware, J. E., and Tarlov, A. R. (1998). Linking Primary Care Performance to Outcomes of Care. J. Fam. Pract. 47, 213–220.
Sami, W., Ansari, T., Butt, N. S., and Hamid, M. R. A. (2017). Effect of Diet on Type 2 Diabetes Mellitus: A Review. Int. J. Health Sci. (Qassim) 11, 65–71. doi:10.1002/dmrr.2515
Schein, C., and Gray, K. (2015). The Unifying Moral Dyad. Pers Soc. Psychol. Bull. 41, 1147–1163. doi:10.1177/0146167215591501
Schindler, S., Zell, E., Botsch, M., and Kissler, J. (2017). Differential Effects of Face-Realism and Emotion on Event-Related Brain Potentials and Their Implications for the Uncanny Valley Theory. Sci. Rep. 7, 45003. doi:10.1038/srep45003
Schmid Mast, M., Hall, J. A., and Roter, D. L. (2007). Disentangling Physician Sex and Physician Communication Style: Their Effects on Patient Satisfaction in a Virtual Medical Visit. Patient Edu. Couns. 68, 16–22. doi:10.1016/j.pec.2007.03.020
Schneider, J., Kaplan, S. H., Greenfield, S., Li, W., and Wilson, I. B. (2004). Better Physician-Patient Relationships are Associated with Higher Reported Adherence to Antiretroviral Therapy in Patients with HIV Infection. J. Gen. Intern. Med. 19, 1096–1103. doi:10.1111/j.1525-1497.2004.30418.x
Seyama, J., and Nagayama, R. S. (2007). The Uncanny Valley: Effect of Realism on the Impression of Artificial Human Faces. Presence Teleop. Vir. Environ. 16, 337–351. doi:10.1162/pres.16.4.337
Shapiro, R. S., Simpson, D. E., Lawrence, S. L., Talsky, A. M., Sobocinski, K. A., and Schiedermayer, D. L. (1989). A Survey of Sued and Nonsued Physicians and Suing Patients. Arch. Intern. Med. 149, 2190–2196. doi:10.1001/archinte.149.10.2190
Sillice, M. A., Morokoff, P. J., Ferszt, G., Bickmore, T., Bock, B. C., Lantini, R., et al. (2018). Using Relational Agents to Promote Exercise and Sun Protection: Assessment of Participants' Experiences with Two Interventions. J. Med. Internet Res. 20, e48. doi:10.2196/jmir.7640
Stavropoulou, C. (2011). Non-adherence to Medication and Doctor-Patient Relationship: Evidence from a European Survey. Patient Edu. Couns. 83, 7–13. doi:10.1016/j.pec.2010.04.039
Stein, J.-P., and Ohler, P. (2018). Uncanny... but Convincing? Inconsistency Between a Virtual Agent's Facial Proportions and Vocal Realism Reduces its Credibility and Attractiveness, but Not its Persuasive Success. Interact Comput. 30, 480–491. doi:10.1093/iwc/iwy023
Stonerock, G. L., and Blumenthal, J. A. (2017). Role of Counseling to Promote Adherence in Healthy Lifestyle Medicine: Strategies to Improve Exercise Adherence and Enhance Physical Activity. Prog. Cardiovasc. Dis. 59, 455–462. doi:10.1016/j.pcad.2016.09.003
Street, R. L. (1990). Communication in Medical Consultations: A Review Essay. Q. J. Speech 76, 315–332. doi:10.1080/00335639009383926
Tamilmani, K., Rana, N. P., Wamba, S. F., and Dwivedi, R. (2021). The Extended Unified Theory of Acceptance and Use of Technology (UTAUT2): A Systematic Literature Review and Theory Evaluation. Int. J. Inf. Manage. 57, 102269. doi:10.1016/j.ijinfomgt.2020.102269
Todorov, A., Chaiken, S., and Henderson, M. D. (2002). “The Heuristic-Systematic Model of Social Information Processing,” in The Persuasion Handbook: Developments in Theory and Practice (Thousand Oaks, CA: Sage Publications), 195–211.
Tuomilehto, J., Lindström, J., Eriksson, J. G., Valle, T. T., Hämäläinen, H., Ilanne-Parikka, P., et al. (2001). Prevention of Type 2 Diabetes Mellitus by Changes in Lifestyle Among Subjects with Impaired Glucose Tolerance. N. Engl. J. Med. 344, 1343–1350. doi:10.1056/NEJM200105033441801
Umberson, D. (1987). Family Status and Health Behaviors: Social Control as a Dimension of Social Integration. J. Health Soc. Behav. 28, 306–319. doi:10.2307/2136848
Waeber, B., Burnier, M., and Brunner, H. R. (2000). How to Improve Adherence with Prescribed Treatment in Hypertensive Patients?. J. Cardiovasc. Pharmacol. 35 (Suppl. 3), S23–S26. doi:10.1097/00005344-200035063-00006
Walsh, C. A., Cahir, C., Tecklenborg, S., Byrne, C., Culbertson, M. A., and Bennett, K. E. (2019). The Association between Medication Non‐adherence and Adverse Health Outcomes in Ageing Populations: A Systematic Review and Meta‐analysis. Br. J. Clin. Pharmacol. 85, 2464–2478. doi:10.1111/bcp.14075
Wang, Y., Geigel, J., and Herbert, A. (2013). “Reading Personality: Avatar vs. Human Faces,” in Proceedings of the 2013 Humaine Association Conference on Affective Computing and Intelligent Interaction, Geneva, September 2–5, 2013, 479–484. doi:10.1109/ACII.2013.85
Webb, T. L., and Sheeran, P. (2006). Does Changing Behavioral Intentions Engender Behavior Change? A Meta-Analysis of the Experimental Evidence. Psychol. Bull. 132, 249–268. doi:10.1037/0033-2909.132.2.249
Willson, P., and McNamara, J. R. (1982). How Perceptions of a Simulated Physician-Patient Interaction Influence Intended Satisfaction and Compliance. Soc. Sci. Med. 16, 1699–1704. doi:10.1016/0277-9536(82)90095-8
Wojciszke, B., Bazinska, R., and Jaworski, M. (1998). On the Dominance of Moral Categories in Impression Formation. Pers Soc. Psychol. Bull. 24, 1251–1263. doi:10.1177/01461672982412001
Wood, W., and Kallgren, C. A. (1988). Communicator Attributes and Persuasion. Pers Soc. Psychol. Bull. 14, 172–182. doi:10.1177/0146167288141017
Yin, L., Bickmore, T., and Cortés, D. E. (2010). “The Impact of Linguistic and Cultural Congruity on Persuasion by Conversational Agents,” in Intelligent Virtual Agents, Lecture Notes in Computer Science.. Editors J. Allbeck, N. Badler, T. Bickmore, C. Pelachaud, and A. Safonova (Berlin, Heidelberg: Springer), 343–349. doi:10.1007/978-3-642-15892-6_36
Zell, E., Aliaga, C., Jarabo, A., Zibrek, K., Gutierrez, D., McDonnell, R., et al. (2015). To Stylize or Not to Stylize?. ACM Trans. Graph. 34, 1–12. doi:10.1145/2816795.2818126
Zhuo, X., Zhang, P., and Hoerger, T. J. (2013). Lifetime Direct Medical Costs of Treating Type 2 Diabetes and Diabetic Complications. Am. J. Prev. Med. 45, 253–261. doi:10.1016/j.amepre.2013.04.017
Zibrek, K., Kokkinara, E., and McDonnell, R. (2018). The Effect of Realistic Appearance of Virtual Characters in Immersive Environments - Does the Character's Personality Play a Role?. IEEE Trans. Vis. Comput. Graph. 24, 1681–1690. doi:10.1109/TVCG.2018.2794638
Keywords: adherence, anthropomorphism, doctor–patient communication, heuristic–systematic model, interactive narratives, persuasive technologies, stereotype content model, uncanny valley effect
Citation: Dai Z and MacDorman KF (2021) Creepy, but Persuasive: In a Virtual Consultation, Physician Bedside Manner, Rather than the Uncanny Valley, Predicts Adherence. Front. Virtual Real. 2:739038. doi: 10.3389/frvir.2021.739038
Received: 09 July 2021; Accepted: 18 August 2021;
Published: 14 September 2021.
Edited by:
Funda Durupinar, University of Massachusetts Boston, United StatesReviewed by:
Matias Volonte, Northeastern University, United StatesCopyright © 2021 Dai and MacDorman. This is an open-access article distributed under the terms of the Creative Commons Attribution License (CC BY). The use, distribution or reproduction in other forums is permitted, provided the original author(s) and the copyright owner(s) are credited and that the original publication in this journal is cited, in accordance with accepted academic practice. No use, distribution or reproduction is permitted which does not comply with these terms.
*Correspondence: Karl F. MacDorman, a21hY2Rvcm1AaW5kaWFuYS5lZHU=
Disclaimer: All claims expressed in this article are solely those of the authors and do not necessarily represent those of their affiliated organizations, or those of the publisher, the editors and the reviewers. Any product that may be evaluated in this article or claim that may be made by its manufacturer is not guaranteed or endorsed by the publisher.
Research integrity at Frontiers
Learn more about the work of our research integrity team to safeguard the quality of each article we publish.