- 1Human-Technology-Systems Group, University of Würzburg, Würzburg, Germany
- 2Human-Computer Interaction Group, University of Würzburg, Würzburg, Germany
Artificial Intelligence (AI) covers a broad spectrum of computational problems and use cases. Many of those implicate profound and sometimes intricate questions of how humans interact or should interact with AIs. Moreover, many users or future users do have abstract ideas of what AI is, significantly depending on the specific embodiment of AI applications. Human-centered-design approaches would suggest evaluating the impact of different embodiments on human perception of and interaction with AI. An approach that is difficult to realize due to the sheer complexity of application fields and embodiments in reality. However, here XR opens new possibilities to research human-AI interactions. The article’s contribution is twofold: First, it provides a theoretical treatment and model of human-AI interaction based on an XR-AI continuum as a framework for and a perspective of different approaches of XR-AI combinations. It motivates XR-AI combinations as a method to learn about the effects of prospective human-AI interfaces and shows why the combination of XR and AI fruitfully contributes to a valid and systematic investigation of human-AI interactions and interfaces. Second, the article provides two exemplary experiments investigating the aforementioned approach for two distinct AI-systems. The first experiment reveals an interesting gender effect in human-robot interaction, while the second experiment reveals an Eliza effect of a recommender system. Here the article introduces two paradigmatic implementations of the proposed XR testbed for human-AI interactions and interfaces and shows how a valid and systematic investigation can be conducted. In sum, the article opens new perspectives on how XR benefits human-centered AI design and development.
Introduction
Artificial Intelligence (AI) today covers a broad spectrum of application use cases and the associated computational problems. Many of those implicate profound and sometimes intricate questions of how humans interact or should interact with AIs. The continuous proliferation of AIs and AI-based solutions into more and more areas of our work and private lives also significantly extends the potential range of users in direct contact with these AIs.
There is an open and ongoing debate on the necessity of required media competencies or, even more, on required computer science competencies for users of computer systems. This digital literacy (competencies needed to use computational devices (Bawden and others, 2008) and computational literacy (the ability to use code to express, explore, and communicate ideas (DiSessa, 2001)), lately has been extended to also include AI literacy to denote competencies that enable individuals to critically evaluate AI technologies; communicate and collaborate effectively with AI; and use AI as a tool online, at home, and in the workplace (Long and Magerko, 2020). This debate roots deep into the progress of the digital revolution for some decades now. AI brings in an exciting flavor to this debate since it risks significantly amplifies the digital divide for certain (groups of) individuals just by the implicit connotation of the term. For one, AI’s implicit claim to replicate human intelligence can be attributed to the term “Artificial Intelligence” itself, as John McCarthy proposed at the famous Dartmouth conference in 1956. Some researchers still consider the term ill-posed to begin with, and the history of AI records alternatives with less implicate associations, see contemporary textbooks on AI, e.g., by Russel and Norvig (Russell and Norvig, 2020).
Nevertheless, now AI-applications are omnipresent, the term AI is commonly used for the field, and the term certainly implies far-reaching connotations for many users not experts in the specific field of AI or in computer science in general. Additionally, the reception and presentation of AI by mainstream media, e.g., in movies and other works of fiction, has undoubtedly contributed to shaping a very characteristic AI profile (Kelley et al., 2019; Zhang and Dafoe, 2019). This public understanding often sketches at least a skewed image of the principles and the potentials and risks of AI (see Figure 1). As Clarke has pointed out in his often quoted third law, “Any sufficiently advanced technology is indistinguishable from magic” (Clarke, 1962). Suppose the latter could already be observed for such simple computing applications like a spread-sheet, or more complex examples of Information Technology (IT) like “the Internet”. In that case, it seems even more likely to be true for assistive devices that listen to our voices and speak to us in our native tongue, robots that operate in the same physical realm with us and which—for a naïve observer—seem to be alive, or self-driving cars, or many more incarnations of modern AI systems. From a Human-Computer Interaction (HCI) perspective, it is of utmost importance to understand and investigate if, and if, how the human user perceives the AI she is interacting with. Besides, most AI-systems will incorporate a human-computer interface. With interface we here denote the space where the interaction between human and machine takes place, including all hardware and software components and the underlying interaction concepts and styles.
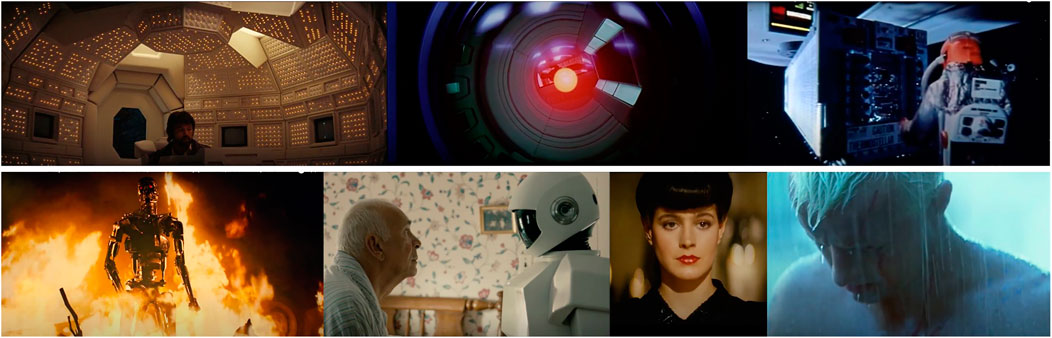
FIGURE 1. Examples of movie presentations of AI embodiments. From upper left to lower right: Dallas (Tom Skerrit) talking to mother, the ship’s AI (all around him in the background) via a terminal in Alien (Scott, 1979); The famous red eye of H.A.L. 9,000, the AI in 2001: A Space Odessey (Kubrick, 1968), which later follows its own agenda; Philosophical debate between Doolittle (Brian Narelle) and reeled-out bomb 20, a star killing device, why not to detonate on potentially false evidence in Dark Star (Carpenter, 1974); The threatening T 800 stepping out of the fire to hunt down its human prey in the dystopian fiction The Terminator (Cameron, 1984); Frank talking to his domestic robot who later becomes his partner in crime in Robot & Frank (Schreier, 2012); Two replicants, bio-engineered synthethic humans, in Blade Runner (Scott, 1982), Rachel (Sean Young), who does not know about her real form of existence and operating/lifetime expecation, and Roy Batty (Ruttger Hauer), philosphing about the essence of life before he dies at the end of his operating/lifetime expecation. Screenshots made by the authors.
The appearance of an AI at the interface can range from simple in general pervasive effects like the execution of a requested operation, such as switching on a lamp in a smart home appliance, to simple text displays, to humanoid and human looking robots or virtual agents trying to mimic real persons with communicative behaviors typically associated with real humans. Often, AIs will appear to the user at the interface with some sort of embodiment, such as a specific device like a smart speaker, as a self-driving car, or as a humanoid robot or a virtual agent appearing in Virtual, Augmented, or Mixed Reality (VR, AR, MR: XR, for short). The latter is specifically interesting since the embodied aspect of the interface is mapped to the virtual world in contrast to the real physical environment. Embodiment itself has interesting effects on the user such as the well-known uncanny valley effect (Mori et al., 2012) or the Proteus effect (Yee and Bailenson, 2007). The latter describes a change of behavior caused by a modified and perceived self-representation, such as the appearance of a self-avatar. First results indicate that this effect also exists regarding the perception of others (Latoschik et al., 2017) which can be hypothesized to also apply to the human-AI interface.
The media equation postulates that the sheer interaction itself already contributes significantly to the perception and, more specifically, an anthropomorphization of technical systems by users (Reeves and Nass, 1996a). In combination with an already potentially shallow or even skewed understanding of AI by some users, i.e., a sketchy AI literacy, or an unawareness of interacting with an AI by others, and the huge design space of potential human-AI interfaces, it seems obvious that we need a firm understanding of the potential effects the developers’ choice of the appearance of human-AI interfaces has on the users. Human-centered-design approaches would suggest evaluating the impact of different embodiments on human perception of and interaction with AIs and identifying the effects these manipulations would have on users. This approach, which is central to the principles in HCI, however, is difficult to realize due to the large design space of embodiments in, and the sheer complexity of many fields of applications of the real physical world. Another rising technology cluster, XR, accounts for control and systematic manipulations of complex interactions (Blascovich et al., 2002; Wienrich and Gramlich, 2020; Wienrich et al., 2021a). Hence, XR provides much potential to increase the investigability of human-AI interactions and interfaces.
In sum, a real-world embodiment of an AI might need considerable resources, e.g., when we think of humanoid robots or self-driving cars. In turn, systematic investigations are essential for an evidence-based human-centered AI design, the design and evaluation of explainable AIs and tangible training modules, and basic research of human-AI interfaces and interactions. The present paper suggests and discusses XR as a new perspective on the XR-AI combination space and as a new testbed for human-AI interactions and interfaces by raising the question:
How can we establish valid and systematic investigation procedures for human-AI interfaces and interactions?
Four sub-questions structure the first part of the article. Theoretical examinations about these questions contribute to a new perspective on the XR-AI combination space on the one hand and a new testbed for human-AI interactions and interfaces on the other hand (Table 1).

TABLE 1. Summarizes the research questions and corresponding contributions of the article’s first part.
The second part of the present article introduces two paradigmatic implementations of the proposed XR testbed for human-AI interactions and interfaces. An XR environment simulated interactive and embodied AIs (Experiment 1: a conversational robot, Experiment 2: recommender system) to evaluate the perception of the AI and the interaction in dependency of various AI embodiments (Table 2).
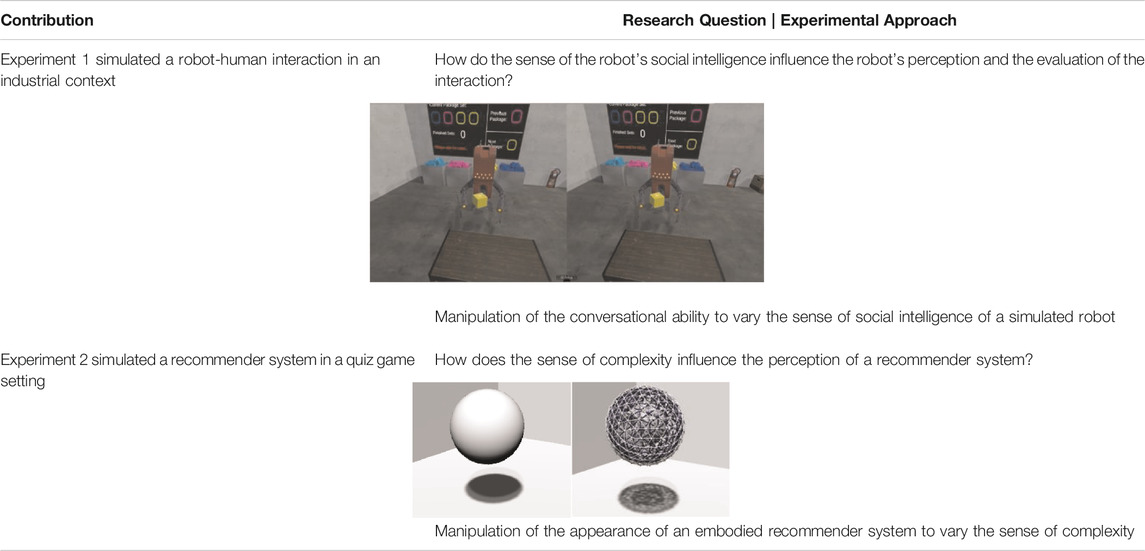
TABLE 2. Summarizes the experimental approach and corresponding contributions of the article’s second part.
A New Perspective on the XR-Artificial Intelligence Combination Space
How Is Human-Artificial Intelligence Interaction Defined?
Human-AI interaction (HAII) has its roots in the more general concept of HCI, since we here assume that some sort of computing machinery realizes an artificial intelligence. Hence human-AI interaction is a special form of HCI where the AI is a special incarnation of a computer system. Note that this definition does not distinguish between a hardware and a software layer, but uses the term computer system in the general sense, combining both aspects of hardware and software together to constitute a system that interacts with the user.
The second aspect to clarify is what precisely we mean by the term Artificial Intelligence or AI. As we have briefly noted in the introduction, the term has a long history going back at least to the Dartmouth conference in 1956. Typical definitions of AI usually incorporate some definition of the aspect of artificiality referring to a machine or computer system as the executor of some sort of simulation or process which can be attributed to some type of intelligence either in its relationship between input and output or in its internal functioning or model of operation, which might try to mimic the internal functioning of a biological entity one considers intelligent. Note that in these types of definitions, the term intelligence is not defined but often implicitly refers to human cognitive behaviors.
Russell and Norvig demarcate AI as a separate field of research and application from other fields like mathematics, control theory, or operations research by two descriptions (Russell and Norvig, 2020): First, they state that from the beginning, AI included the concept to replicate human capabilities like creativity, self-dependent learning, or utilization of speech. Second, they point to the employed methods, which first and centrally are rooted in computer science: AI uses computing machines as the executor of some creative or intelligent processes, i.e., processes that allow the machine to autonomously operate in complex, continuously changing environments. Both descriptions cover a good number of AI use cases. However, they are not comprehensive enough to cover all use cases, unless one interprets complex, continuously changing environments very broadly. We want to extend this circumscription of AI with processes that adopt computational models of intelligent behavior to solve complex problems that humans are not able to solve due to the sheer quantity and/or complexity of input data, i.e., as typical in big data and data mining.
To further clarify what we mean by human-AI interaction, we use the term AI in human-AI to denote the incarnation of a computing system incorporating AI capabilities as described above. For the remainder of this paper, the context should clarify if we talk about AI as the field of research or AI as such an incarnation of an intelligent computing system, and we will precisely specify what we mean where it might be ambiguous. Note that this ambiguity between AI as a field or AI as a system already indicates the tendency to attribute particular capabilities and human-like attributes to such a system and potentially see it as an independent entity that we can interact with. The latter is not specific to an AI but can already be noticed for non-AI computer systems where users tend to attribute independent behavior to the system, specifically if something is going wrong. Typical examples from user reactions calling a support line like “He doesn’t print” or “He is not letting me do this” indicate this tendency and usually are interpreted as examples of the media equation (Reeves and Nass, 1996b; Nass and Gong, 2000; Wienrich et al., 2021b). However, we want to stress the point that such effects might be amplified by AI-systems due to their, in principle, rather complex behaviors and, as we will discuss in the next sections, due to the general character and appearance of an AI’s embodiment.
How to Classify Combined XR With Artificial Intelligence? The XR-Artificial Intelligence Continuum
As we pointed out, there are various use-cases for and also incarnations of AIs, and in this context, we do not want to restrict the kinds of AIs in human-AI interaction any further. As we have seen, AI are specialized computer systems either by their internal workings or by their use case, and capabilities. As such, if they do not operate 100% independently from humans but will serve a role, task, or function, there will be the need to interact with human users. For some AI-applications, it seems more straightforward to think about forms of an embodiment for an AI, e.g., robots, conversational virtual agents, or smart speakers (examples Figure 2). However, if there is a benefit of AI-embodiment for the user, e.g., if it helps to increase the usability or user experience (UX), or if it helps the user to gain a deeper understanding of an AI’s function and capabilities, then we should consider extending the idea of AI-embodiment to more use cases of AIs, from expert systems to data science to self-driving cars. However, appearance matters, i.e., the kind of embodiment impacts significantly on human perception and acceptance. From an HCI perspective, it is of utmost importance to understand and investigate how an AI’s appearance influences the human counterpart. Only when we understand this influence we can scientifically contribute to a user-centered AI design process, considering responsively different user groups. Note, the term embodiment is not restricted to self-avatars representing humans in a virtual environment. We follow the common understanding of embodiment addressing also “others” in social encounters (also in conjunction with the term other-avatar). The complexity of human-AI interactions and interfaces challenges such investigations (see below). XR offers promising potentials to meet these challenges.
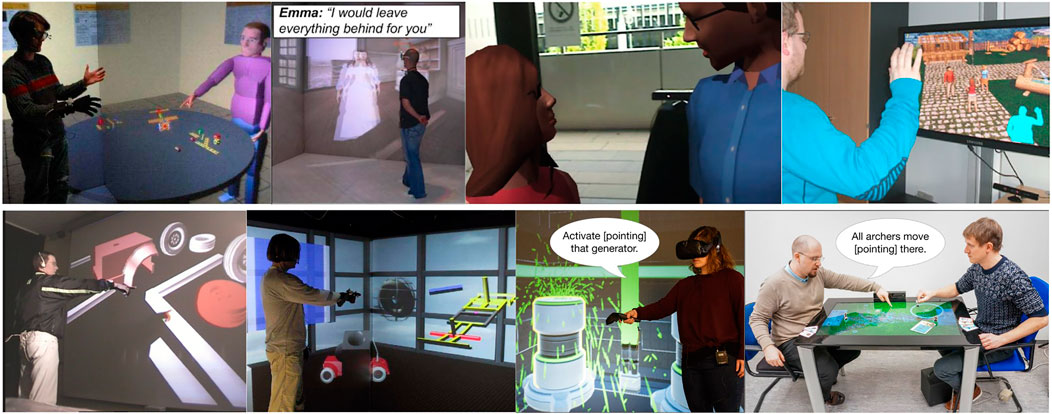
FIGURE 2. Examples of XR-AI integrations. From upper left to lower right: A user is interacting with an intelligent virtual agent to solve a construction task (Latoschik, 2005) and interaction with an agent actor in Madam Bovary, an interactive intelligent story telling piece (Cavazza et al., 2007), both in a CAVE (Cruz-Neira et al., 1992); Virtual agents in an Augmented Reality (AR) (Obaid et al., 2012) and in a Mixed Reality (MR) (Kistler et al., 2012); Speech and gesture interaction in a virtual construction scenario in front of a power wall (Latoschik and Wachsmuth, 1998) and in a CAVE (Latoschik, 2005). Multimodal interactions in game-like scenarios full-immersed using a Head-Mounted Display (HMD) (Zimmerer et al., 2018b) and placed at an MR tabletop (Zimmerer et al., 2018a).
Intelligent Graphics is about visually representing the world and visually representing our ideas. Artificial intelligence is about symbolically representing the world, and symbolically representing our ideas. And between the visual and the symbolic, between the concrete and the abstract, there should be no boundary. (Lieberman, 1996)
Lieberman’s quote describes a central paradigm that combines AI with computer graphics (CG). Its focus on symbolic AI methods seems limited today. However, in the late nineties of the last century, the surge and success of machine learning and deep learning approaches was yet to come. Hence the quote should be seen as a general statement about the combination of AI and CG. Today, intelligent graphics or synonymously smart graphics refers to a wide variety of application scenarios. These range from the intelligent and context-sensitive arrangement of graphical elements in 2D desktop systems to speech-gesture interfaces or intelligent agents in virtual environments as assistants to users. All these approaches have in common that a graphical human-computer interface is adapted to the user’s cognitive characteristics with the help of AI processes to improve the operation (Latoschik, 2014).
The combination of AI and XR, more specifically of Artificial Intelligence and artificial life techniques with those of virtual environments, has been denoted by Aylett and Luck (2000) as Intelligent Virtual Environments. They specifically concentrated on autonomous, physical, and cognitive agents and argued that “embodiment may be as significant for virtual agents as they are for real agents.” They proposed a spectrum between physical and cognitive and identified autonomy as an important quality for such virtual entities. We here argue that the combination of XR and AI is significantly broader and propose the XR-AI continuum (see Figure 3).
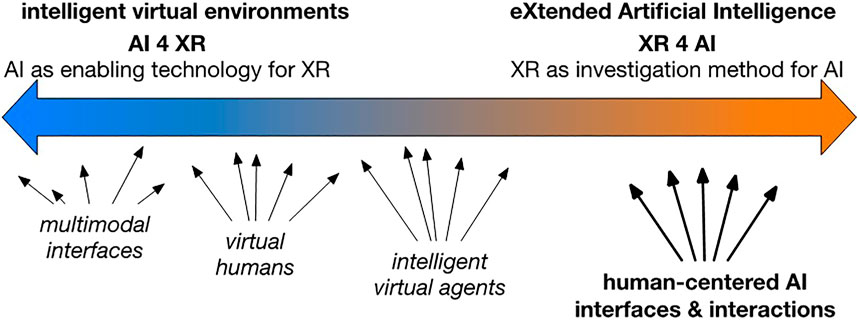
FIGURE 3. The XR-AI Continuum classifies potential XR-AI combination approaches with respect to the main epistemological perspective motivating the combination. The scale’s poles denote approaches that purely target AI as the object of investigation (XR 4 AI) or that target AI as an enabling technology (AI 4 XR). Well-known research areas which utilize XR-AI approaches are depiced with their potential mapping onto the scale. Notably, approaches will often serve both perspectives to various degrees, mapping them to the respective positions between the poles. The established fields and terms of intelligent virtual environments and smart graphics/intelligent graphics will mostly cover the left spectrum of the continuum. In contrast, the right spectrum, which concentrates on AI as the object of investigation, is covered by approaches we denote as eXtended Artificial Intelligence.
The XR-AI continuum is spanned between two endpoints defined by the overall epistemological perspective and goal of a given XR-AI combination. The continuum ranks XR-AI combinations with respect to the general question of what we want to achieve by a given XR-AI combination, i.e. the epistemological motive. Are we using AI as an enabling technology to improve an XR system, e.g., to realize certain AI-supported functionalities, user interfaces, and/or to improve the overall usability? Or do we use XR as a tool to investigate AI? The first perspective is on-trend typical for the majority of early approaches of XR-AI combinations, e.g., as described by intelligent virtual environments, intelligent real-time interactive systems, or, with less focus on immersive and highly interactive displays, as described by smart or intelligent graphics (Latoschik, 2014). While Aylett and Luck mainly focused on AI as an enabling technology to improve the virtual environment (Aylett and Luck, 2000), the XR-AI continuum also highlights how XR technologies provide a new investigability of HAII.
The proposed XR-AI continuum with its explicit perspective of the investigability of HAII circumscribed by the eXtended AI approaches combines current developments and results from XR and AI. Recent work has motivated to investigate how humans react to AIs during interaction. These approaches include investigations of verbal interaction (Fraser et al., 2018; Rieser, 2019) and its influence on the tendency to anthropomorphize (Strathmann et al., 2020), and behavior design (Azmandian et al., 2019) including emotional intelligence (Fan et al., 2017). (Kulms and Kopp, 2016) explicitly target embodiment effects. There are some early examples motivating the methodological aspects of investigations of HAII, though they concentrate on emulating investigability by desktop applications (Zhang et al., 2010; Bickmore et al., 2013; Mattar et al., 2015). However, none of the approaches motivate to utilize the increased design and effect space of XR, e.g., to provide situated and/or embodied interactions in a simulated real-like context. There is strong evidence that media-related characteristics like interaction, immersion (inclusive, extensive, surrounding, and vivid) (Slater and Wilbur, 1997), or plausibility (Slater, 2009; Skarbez et al., 2017; Latoschik and Wienrich, 2021) have a huge impact on investigated and expected target effects, for example in terms of emotional response and/or embodiment (Yee and Bailenson, 2007; Gall and Latoschik, 2018; Waltemate et al., 2018).
More recently, Antakli et al. (2018) presented a virtual simulation for testing human-robot interaction (Antakli et al., 2018). However, the authors only briefly illustrated the entire design space and the advantages of an XR testbed proposed in the present paper. Very recently, Sterna et al. (2021) pointed to the lack of pretesting in the VR community (Sterna et al., 2021). They presented preliminary work on a web-based tool for pretesting virtual agents. Again, the application does not allow situated or embodied interaction in a real-like context.
Two currently published contributions point to a lack of research referring to the right side of our continuum. Ospina-Bohórquez et al. (2021) analyzed in a structured literature review the interplay between VR and multi-agent systems (Ospina-Bohórquez et al., 2021). It answered two research questions: “What applications have been developed with Multi-agent systems in the field of Virtual Reality?” and “How does Virtual Reality benefit from the use of Multi-agent systems?”. The analysis revealed fruitful combination and application areas. Remarkable, the search also demonstrated that most combinations are intended to make the virtual environment more intelligent referring to the left side of our continuum. The authors conclude that there is a lack of research investigating more complex simulations to examine the user-avatar relationship on the one hand. On the other hand, they stated that “[…] it would be necessary to include the mental state of agents (emotions, personality, etc.) as part of the agent’s perception process, since these factors influence human attention processes in the real world” (p. 17). Both desiderates hints to the significance of the right sight of our continuum. Further, Fitrianie et al. (2020) pointed to a methodological crisis on the evaluation of artificial social agents (Fitrianie et al., 2020). They discuss that most studies use different approaches to investigate human-agent interactions, resulting in a lack of comparability and replicability. They contributed to a solution by looking for constructs and questionnaire items to make the research on virtual agents more comparable and replicable.
In sum, many research can be classified to the left side of the XR-AI Continuum, while using XR as an investigation method for AI needs further research.
The following sections discuss why the extension is necessary and how it contributes to a better understanding of human-AI interactions and interfaces.
XR as a New Testbed for Human-AI Interactions and Interfaces
What can We Learn From the Challenges and XR Solutions Concerning the Investigability of Human-Human Interaction?
In psychology, interaction is defined as a dynamic sequence of social actions between individuals (or groups) who modify their actions and reactions due to actions by their interaction partner(s) (Jonas et al., 2014).
Researchers studying individual differences in human-human social interactions face the challenge of keeping constant or changing systematically the behavior and appearance of the interaction partner across participants (Hatfield et al., 1992). Even slightly different behaviors and appearances influence participants’ behavior (Congdon and Schober, 2002; Topál et al., 2008; Kuhlen and Brennan, 2013). For investigating social interactions between humans, the potentials of XR are already recognized (Blascovich, 2002; Blascovich et al., 2002). Using virtual humans provides high ecological validity and high standardization (Bombari et al., 2015; Pan and Hamilton, 2018). In addition, using a virtual simulation of interaction enables researchers to easily replicate the studies, which is essential for social psychology, in which replication is lacking (Blascovich et al., 2002; Bombari et al., 2015; Pan and Hamilton, 2018). Another advantage of using XR to study human-human interactions is that situations and manipulations that would be impossible in real life can be created (Bombari et al., 2015; Pan and Hamilton, 2018). Many studies substantiated XR’s applicability and versatility to simulate and investigate social interaction between (virtual) humans (Blascovich, 2002; Bombari et al., 2015; Wienrich et al., 2018a, 2018b). Many of them showed the significant impact of different self-embodiments on self-perception, known as the Proteus effect (Yee and Bailenson, 2007; Latoschik et al., 2017; Ratan et al., 2019). Recent results show the Proteus effect caused by self-avatars also applies to the digital counterparts (the avatars) of others. Others demonstrated how XR potentials are linked to psychological variables (Wienrich and Gramlich, 2020; Wienrich et al., 2021a).
Which Challenges and Solutions Arise for the Systematic Investigation of Human-AI Interaction and Interfaces?
In HCI and hence in HAII, at least one interacting partner is a human, and at least one partner is constituted by a computing system or an AI, respectively. However, the focus has traditionally less been on the social aspects but a task level or the pragmatic quality of interaction. Notably, social aspects of the interaction are recently becoming more and more found interesting in HCI (Carolus and Wienrich, 2019). This trend is becoming even more relevant for HAII due to the close resemblance of certain HAII properties with human intelligence (Carolus et al., 2019).
Consequently, AI applications, becoming increasingly interactive and embodied, leads up to essential changes from an HCI perspective:
1) Interactive embodied AI changes the interface conceptualization from an artificial tool/device into an artificial (social) counterpart.
2) Interactive embodied AI changes the usage by skilled experts into diverse users usage.
3) Interactive embodied AI changes the applications in specific domains into diverse (every day) domain applications.
4) Additionally, the penetration of AI in almost every domain of life also changes the consequences of lacking acceptance and misperceptions. While a lack of acceptance and misperception resulted in usage avoidance in the past, avoidance passes into incorrect usage with considerable consequences in the future.
Thus, AI applications are becoming increasingly interactive and embodied, leading to the question of how researchers can study individual differences in human-AI interactions and the impact of different AI embodiments on human perception of and interaction with AI. Similar to human-human interaction, we face the challenge of keeping constant or changing systematically the behavior and appearance of the artificial interaction partner across participants. Such an approach is challenging to realize due to the sheer complexity of application fields and embodiments in reality. Similar to human-human interaction, interactive and embodied AIs lead up to considerable challenges for the systematic investigability of human-AI interactions. However, XR opens four essential potentials shown in Table 3.
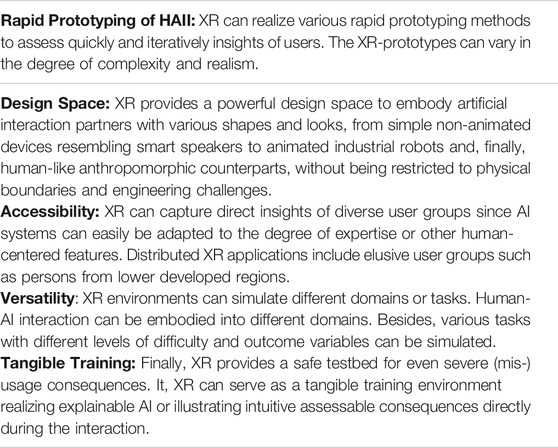
TABLE 3. Describes the four potentials of XR as a new testbed for human-AI interactions and interfaces. Each potential can be realized by more or less complex and realistic prototypes of HAII.
From an HCI perspective, eXtended AI (left side of the XR-AI continuum) constitutes a variant of rapid prototyping for HAII (Table 3). Rapid prototyping includes methods to fabricate a scale model of a physical part or to show the function of a software product quickly (Yan and Gu, 1996; Pham and Gault, 1998). The clue is that users interact with a prestage or a simulation instead of the fully developed product. Such methods are essential for iterative user-centered design processes because they supply user insights in the early stages of development processes (Razzouk and Shute, 2012). Computer aided design (CAD), Wizard-of-Oz, mock-ups, darkhorse prototyping, or the Eliza principle are established rapid prototyping methods. XR as a testbed for HAII allows for rapid prototyping of interactive and embodied AIs, for complex interactions, and in different development stages to understand user’s mental models about AI, predict interaction paths and reactions. Besides, multimodel interactions and analyzes can be quickly realized and yield interesting results for the design, accessibility, versatility, and training effects. Of course, XR technology faces some challenges regarding the accessibility of the technology itself (Peck et al., 2020). However, XR technology becomes increasingly mobile and cheaper (e.g., Oculus Quest, Pico neo) and easier to developed (e.g., with Unity). Accessibility and versability refer instead to the advantage of XR reaching regions or user groups that are often cut off from industrial centers or innovation hubs. Moreover, situated interaction in different contexts can be realized facilitating participative design approaches and contextual learning.
In sum, the first part presented theoretical examinations about four sub-questions revealing a new perspective on the XR-AI combination space on the one hand and a new testbed for human-AI interactions and interfaces on the other hand. Hence, the first part show why the combination of XR and AI fruitfully contribute to a valid and systematic investigation of human-AI interactions and interfaces.
The following second part of the present article introduces two paradigmatic implementations of the proposed XR testbed for human-AI interactions and interfaces and show how a valid and systematic investigation can be conducted.
Paradigmatic Implementations of an XR Testbed for Human-Artifical Intelligence Interactions and Interfaces
In the following, we outline two experiments simulating a human-AI interaction in XR. The first experiment simulated a human-robot interaction in an industrial context. The second one resembled an interaction with an embodied recommender system in a quiz game context. The experiments serve as an illustration to elucidate the four potentials of XR mentioned above. Thus, only a few pieces of information are presented related to the scope of the article leading to a deviation from a typical method and results presentation. Please refer to the authors for more detailed information regarding the experiments. In the discussion section, the possibilities and limits of such an XR testbed for AI-human interactions, in general, are illustrated on the paradigmatic implementations.
Paradigmatic Experiment 1: Simulated Human-Robot Interaction
Background
Robots reflect an embodied AI. In industrial contexts, robots and humans already work side by side. Mainly, robots operate within a security zone to ensure the safety of human co-workers. However, collaboration or cooperation, including contact and interaction with robots, will gain importance. As mentioned above, investigating collaborative or cooperative human-robot interactions are complex due to myriads of gestalt variants, tasks, and security reasons. Besides, many different user groups with different needs and motives will work with robots. One crucial aspect of interaction is the sense of social intelligence in the artificial co-worker (Biocca, 1999). The scientific literature describes different cues implying the social intelligence of an artificial counterpart, such as starting a conversation, adaptive answering, or sharing personal experiences (Aragon, 2003; Terry and Garrison, 2007).
The present experiment manipulated the conversational ability to vary the sense of social intelligence of a simulated robot. The experiment asks: How does the sense of the robot’s social intelligence influence the perception of the robot and the evaluation of the interaction?
Method
Thirty-five participants (age in years: M = 22.00, SD = 1.91; 24 females) interacted with a simulated robot in an industrial XR environment (see Figure 4). All participants were students and received course credit for participation. The environment was created in Unity Engine Version 2019.2.13f1. The player interactions are pre-made and imported through the Steam-VR plugin. All assets (tools and objects for use in Unity, like 3D-models) were available in the Unity Asset Store. The Vive pro headset was used.
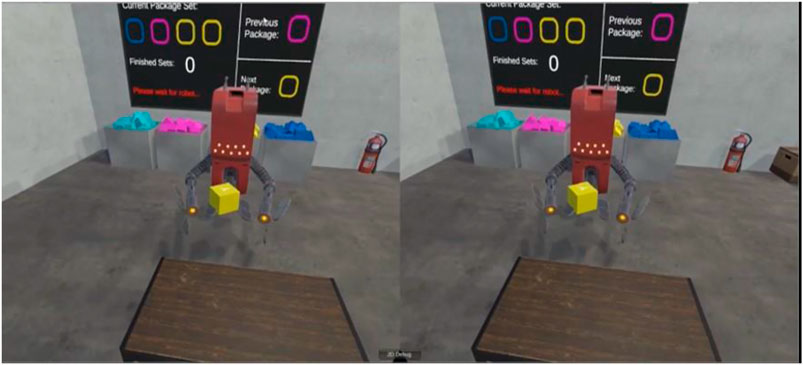
FIGURE 4. shows the virtual environment and the embodiment of the robot (stereoscopic view of a user wearing a Head-Mounted Display—HMD).
Collaboratively, they sorted packages with different colors and letters as fast and accurately as possible. First, the robot sorted the packages by color (yellow, cyan, pink, dark blue). Second, the robot delivered the packages with the right color to the participant. Third, the participant threw the packages into one of two shafts, either into the shaft for the letters A to M or into that for N to Z’s letters. A gauge showed the number of correct sorted packages. The performance of the robot was the same in all interactions.
Participants conducted two conditions in a within subject design while the order of the conditions was balanced. In the conversational condition (short: CR), the robot showed cues of social intelligence by starting a conversation, adaptive answering, and sharing personal preferences about small-talk topics (e.g., “Hi, I am Roni, we are working together today!”; “How long do you live here?”; What is your favorite movie?”). In a Wizard-of-Oz scenario, the robot answered adaptively to the reaction of the participants. The conversation ran alongside the tasks. In the control condition, the robot did not talk to the participant (short: nCR). The experimenter was present during the whole experiment.
To assess the perception of the robot, we measured the uncanniness on a five-point Likert-scale (Ho and MacDorman, 2010) with the subscales humanness, eeriness, and attractiveness. Furthermore, the sense of social presence was measured with the subscale social of the Bailenson social presence scale (Bailenson et al., 2004). Finally, the valence of the robot evaluation was assessed by the negative attitude towards robots scale (short: NARS), including the subscales S1 negative attitudes towards situations of interactions with robots, S2 negative attitudes toward the social influence of robots, S3 negative attitudes toward emotions in interaction with robots (Nomura et al., 2006). Participants gave their answers on a five-point Likert-scale. For data analyses, an overall score as the average of the subscales was built.
To assess the evaluation of the interaction, the user experience of the participants was measured. Four items assessed the pragmatic (e.g., “The interaction fulfilled my seeking for simplicity.”) and hedonic (e.g., “The interaction fulfilled my seeking for pleasure.”) quality based on the short version of the AttrackDiff mini (Hassenzahl and Monk, 2010). The eudaimonic quality was measured by four items (e.g., “The interaction fulfilled my seeking to do what you believe in.”) adapted from (Huta, 2016). Besides, a the social quality was assessed by four items (e.g., “The interaction fulfilled my seeking for social contact.”) based on (Hassenzahl et al., 2015). Additionally, NASA-TLX with the subscales mental demand, physical demand, temporal demand, performance, effort, and frustration was measured on a scale ranging from 0 to 100 (Hart and Staveland, 1988).
Since the experiment serves as an example, we followed an explorative data analysis by comparing the two conditions with an undirected paired t-Test. Further, some explorative moderator analyses, including participant’s gender as a moderator, were analyzed. For each analysis, the alpha level was set up to 0.05 to indicate the significance and to 0.2 to indicate significance by trend (Field, 2009).
Results
Table 4 summarizes the results. The robot with conversational abilities was evaluated as more human-like, attractive, social present, and positive. The evaluation of the interaction yielded mixed results. The pragmatic quality and the mental effort indicated a more negative evaluation of the conversational robot than the non-conversational robot. In contrast, the hedonic, and social quality, the perceived performance were evaluated more positively after interacting with the conversational robot than the non-conversational robot.
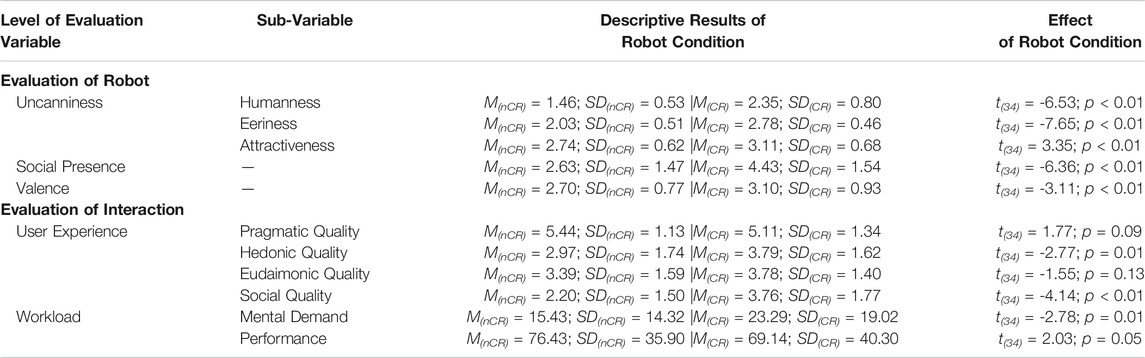
TABLE 4. shows the descriptive and t-test results for the robot condition. M refers to the mean and SD to the standard deviation in the corresponding condition. The t-value represents the test statistic resulting from the t-Test of dependent samples and includes the degrees of freedom. The p-value indicates to the significance of the test. nCR refers to the interaction with the non-conversational robot, i.e., the control condition. CR refers to the robot talking to the participants during the interaction, i.e., the conversational robot.
Furthermore, the results showed that gender matters in human-robot interaction (see Figure 5 and Figure 6). Women rated the conversational robot as more positive (significant) and attractive (by trend) than the non-conversational robot, while men did not show any differences. Regarding the interaction evaluation, men showed lower values of the pragmatic quality after interacting with the conversational robot, while women showed no difference for the conversational conditions. Moreover, women rated the interaction with the conversational robot as more hedonic, while men did not show any differences. Finally, the gradient regarding the social quality for the conversational robot was stronger for women than for men. In general, men showed higher ratings for the robot. Women only showed similar high ratings in the conversational robot condition.
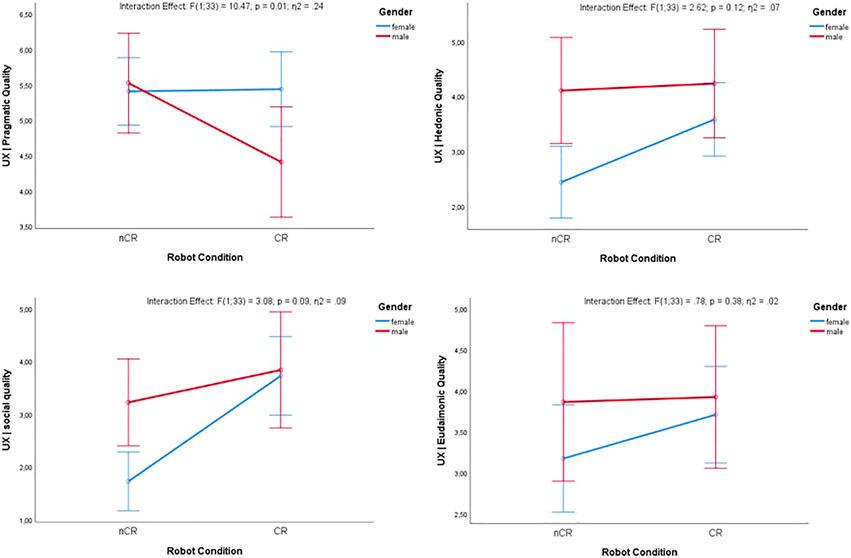
FIGURE 5. shows the interaction effect between robot condition and gender of participants regarding the UX ratings. nCR refers to the non-conversational robot. CR refers to the conversational robot. The results demonstrate that men decreased the pragmatic rating for the conversational robot compared to the non-conversational robot. In contrast, women increased their hedonic, social, and eudaimic rating for the conversational robot (by trend).
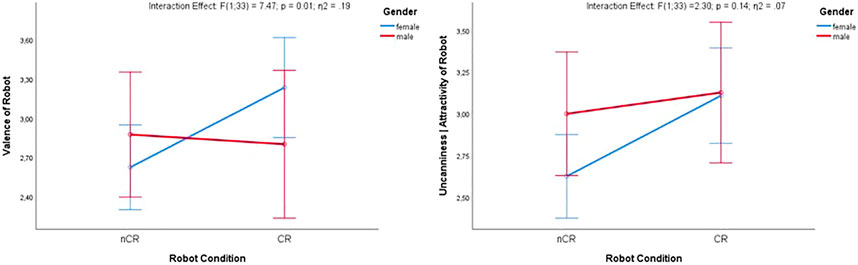
FIGURE 6. shows the interaction effect between robot condition and gender of participants regarding some of the robot ratings. nCR refers to the non-conversational robot. CR refers to the conversational robot. The results demonstrate that women rated the conversational robot more positive than the non-conversational robot while men did not. Similar, but not significant, women rated the conversational robot more attractive than the non-conversational robot while men did not.
Paradigmatic Experiment 2: Simulated Recommender System
Background
Besides robots, recommender systems are another important application of human-AI interaction. Two effects might influence the perception of such a system. The Eliza effect occurs when a system uses simple technical operations but produces effects that appear complex. Then, humans attribute more intelligence and competence than the system provides (Wardrip-Fruin, 2001; Long and Magerko, 2020). The Tale-Spin effect occurs when a system uses complex internal operations but produces effects that appear less complex (Wardrip-Fruin, 2001; Long and Magerko, 2020). However, systematic investigations of these effects are rarely.
The second experiment manipulated the appearance of an embodied recommender system to vary the sense of complexity. The experiment asks: How does the sense of complexity influence the perception of the recommender system?
Method
Thirty participants (age in years: M = 23.07, SD = 2.03; 13 females) interacted with a simulated recommender system in a virtual quiz game environment (see Table 3). All participants were students and received course credit for participation. The environment was created in Unity Engine Version 2019.2.13f1. The player interactions are pre-made and imported through the Steam-VR plugin. All assets (tools and objects for use in Unity, like 3D-models) were available in the Unity Asset Store. The Vive pro headset was used.
Participants answered 40 difficult quiz questions (Figure 7). They could choose from four answers. Participants decided under uncertainty. First, they had 10 s to guess and log in the answer. Then, an embodied AI recommended via voice output which answer possibility might be correct. The participant could decide whether they want to correct their initial choice. After the final selection, feedback about the correctness was given. Participants passed through a tutorial to learn the procedure, including ten test questions and interactions that the AI recommender system (conditions: correct, complex, and simple, see below). The experimenter was present during the whole experiment.
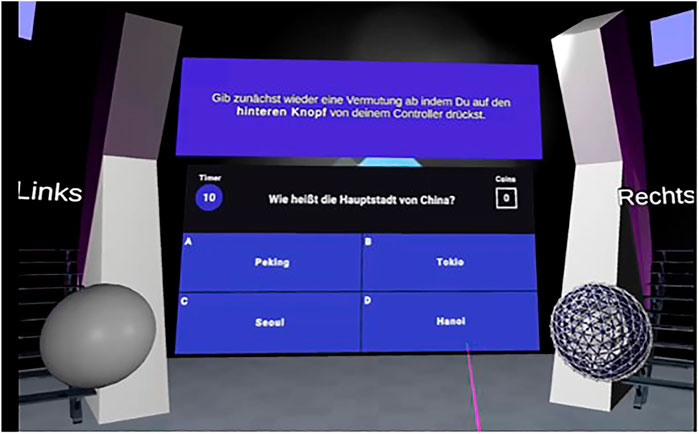
FIGURE 7. shows the virtual quiz game environment. Participants can log in their answer by pointing to one of the four answer possibilities. A sphere embodied the recommender system, either with a simple or complex surface. During the experiment, only one of the recommender systems was present. The recommender system interacted via voice output with the participant.
The recommender system was prescripted and split into two factors correctness (between-subject) and appearance (within-subject). The recommender system answered either 100% correct (high correctness, i.e., AI correct) or 75% correct (low correctness, i.e., AI incorrect). A sphere embodied the recommender system (see Figure 7). The sphere’s surface was either white plain, referring to a simple appearance, or metallic patterned, referring to a complex appearance. A pretest ascertained the distinction. The combination of the factors resulted in four conditions (see Figure 8).
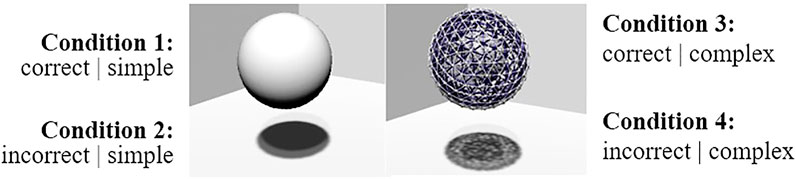
FIGURE 8. shows the embodied recommender system. Left illustrates the simple appearance with the white plain surface. Right illustrates the complex appearance with the metallic patterned surface.
To assess the perception of the recommender systems, participants rated the perceived competence (e.g., The AI was competent) and friendliness (e.g., The AI was friendly). The 7-point Likert-scales based on (Fiske et al., 2002). Further, they rated the perceived trustworthiness based on (Bär et al., 2011), including the subscales perceived risk (e.g., It is a risk to interact with the AI.), benevolence (e.g., I believe, the AI act to my good.), and trust (e.g., I can trust the information given by the AI.). To evaluate the interaction, the user experience of the participants was measured. Four items assessed the pragmatic (e.g., “The interaction fulfilled my seeking for simplicity.”) and hedonic (e.g., “The interaction fulfilled my seeking for pleasure.”) quality based on the short version of the AttrackDiff mini (Hassenzahl and Monk, 2010).
Since the experiment serves as an example, we followed an explorative data analysis by testing the main and interaction effects with a 2 × 2 MANOVA. For each analysis, the alpha level was set up to 0.05 to indicate significance and to 0.2 to indicate significance by trend (Field, 2009).
Results
Table 5 summarizes the results. Participants interacting with the 100% correct AI perceived more competence, trust, and less risk, independently of its appearance. The complex appearance led up to less perceived risk (a sign of higher trustworthiness). However, the simple appearance was rated friendlier, and more benevolent (in the correct condition). Finally, the interaction with the simple appearance rated as more hedonic (when interacting in the correct condition). Figure 9 and Figure 10 illustrate the results.
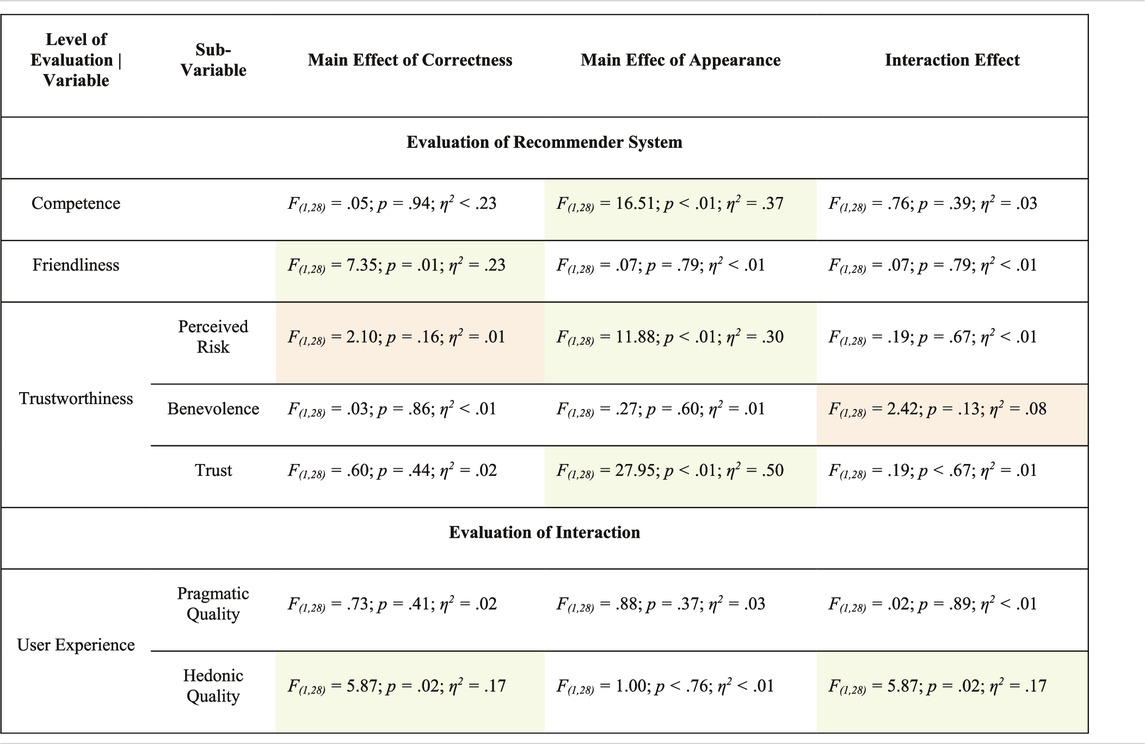
TABLE 5. shows the MANOVA-test results. Green indicates significant results and orange significance by trend (p < 0.20). F refers to the test statistic, p indicates the significance, and η2 represents the effect size.
Discussion
AI-applications are omnipresent, and AI as a term certainly implies far-reaching connotations for many users, not experts. HCI approaches would suggest evaluating the impact of different AI applications, appearances, and operations on human perception of and interaction with AIs and identifying the effects these manipulations would have on users (Wienrich et al., 2021b). However, this approach, which is central to HCI principles, is challenging to realize due to the sheer complexity of many fields of applications of the real physical world. Another rising technology cluster, XR, accounts for control and systematic manipulations of complex interactions (Blascovich et al., 2002; Wienrich and Gramlich, 2020; Wienrich et al., 2021a). Hence, XR provides much potential to increase the investigability of human-AI interactions and interfaces.
The present paper suggests and discusses new perspective on the XR-AI combination space and XR as a new testbed for human-AI interactions and interfaces to establish a valid and systematic investigation procedures for human-AI interfaces and interactions. Four sub-questions structured the article’s first, theoretical, part (see Table 6). The second part presented two paradigmatic implementations of an XR testbed for human-AI interactions (see Table 7).
Contribution 1: A New Perspective on the XR-Artificial Intelligence Combination Space
Lieberman motivated an integrated combination of AI and computer graphics (Lieberman, 1996). Aylett and Luck (Aylett and Luck, 2000) denoted, more specifically, combinations of AI and XR technologies as Intelligent Virtual Environments. In both views, AI is conceptualized as enabling technology to improve the computer graphics or virtual environments. The XR-AI continuum extends the XR-AI combination space by introducing XR as a new investigability of HAII. It includes two perspectives on XR-AI combinations: Does the combination target AI as an object of investigation, e.g., to learn about the effects of a prospective AI embodiment on users? Or does the combination use AI as an enabling technology for XR, e.g., to provide techniques for multimodal interfaces? Often, XR-AI combinations will serve both tasks to some specific degree which allows to map them to the XR-AI continuum.
The XR-AI continuum provides a frame to classify XR-AI combinations. Such a classification serves multiple scientific purposes: First, it allows to systematically evaluate and rate different approaches of XR-AI combinations and hence it helps to structure the overall field. Second, it hopefully leads to an identification of best practices for certain approaches depending on the general perspective taken, and hence, third, that these best practices provide useful guidance, e.g., for replicating or reevaluating work and results. XR-AI combinations can drastically vary in terms of the required development efforts and complexities (Latoschik and Blach, 2008; Fischbach et al., 2017). However, many approaches targeting the eXtended AI range of the XR-AI continuum seem—in principle—to be realizable either by favorable loose couplings of the AI components or to require a rather limited completeness or development effort of the AI part. Regarding the latter, XR technology allows to adopt lightweight AI-mockups based on the Eliza principle or on Wizard-of-Oz scenarios to various degrees and to extensively investigate the relationship between humans and AIs as well as important usability and user experience aspects before an AI is fully functional. Hence, these investigations can be performed without the necessity to first develop a full-blown AI system and, in addition, an AI development process can be integrated with a user-centered design process to continuously optimize the human-AI interface. These rapid prototyping prospects lay the cornerstone to render XR as an ideal testbed to research human-AI interaction and interfaces.
Contribution 2: A New Testbed for Human-Artificial Intelligence Interactions and Interface
Results stemming from human-human interactions have shown that XR provides high ecological validity and high standardization for investigating social interactions between humans (Blascovich et al., 2002; Bombari et al., 2015; Pan and Hamilton, 2018; Wienrich and Gramlich, 2020; Wienrich et al., 2021a). AI applications, becoming increasingly interactive and embodied, lead up to essential changes from an HCI perspective and challenges of a valid and systematic investigability. XR as a new testbed for human-AI interactions and interfaces provides four potentials (see also Table 3) discussed along with the results of the paradigmatic implementations (see Table 7):
1) Design Space: XR provides a powerful design space. Relatively simple and easy to develop XR environments brought insights into the impact of different social intelligence cues and appearances on a robot and recommender evaluation. Instead of test situations asking participants to imagine the AI interaction with different robots (recommender systems) or showing pictures or vignettes, participants interacted with the embodied AI increasing the results’ validity. Furthermore, such simple XR applications can serve as a starting point for systematic variations such as other cues of social intelligence, other appearances, other embodiments of interactive AIs, and combinations of these factors. The effort-cost trade-off is more worthwhile than producing new systems for each variation. Finally, features becoming possible or essential in the future or which are unrealizable in the present can be simulated. In turn, creative design solutions can be developed, keeping pace with the time and fast-changing requirements.
2) Accessibility: XR can capture direct insights of diverse user groups. The paradigmatic experiment investigated student participants. However, the setup can easily be transferred to a testing room in a real industrial or leisure environment and be replicated with users who will collaborate with robots or recommender systems in the future. In addition, distributed VR systems are getting more and more available. Participants from different regions with different profiles, various degrees of expertise, or other human-centered factors can be tested with the same standardized scenario. Thus, a XR testbed can contribute to comparable results revealing individual preferences and needs during a human-AI interaction. As we could see in experiment 1, women rated the robot and interaction differently from men, particularly concerning the need fulfillment. Moreover, interactions between variations of the AI or the human-AI interaction and various users can be investigated systematically.
3) Versatility: XR environments can simulate different domains or tasks such as industry or game. The current experiments simulated simple tasks in two settings. The tasks and the environments can easily be varied in XR. From the perspective of a human-centered design process, the question arises, which AI features are domain-specific and which general design guidelines can be deduced. By testing the same AI in different environments, the question can be answered. Further senseful fields of application can be identified—the same AI can be accepted by users in an industrial setting but not at home, for example. The task difficulty can also impact the evaluation of the AI. The presented results of experiment 1, for example, are valid for easy tasks where conversational abilities of the robot was rated positively. However, during more difficult tasks, conversation might be inappropriate due to distraction and consequences on the task performance. In reality, investigation of such impacts bears many risks while XR provides a safe testbed.
4) Tangible Training: XR can serve as a tangible training environment. The presented experiment did not include a training. However, the operational principles of such embodied AIs can be illustrated directly during the interaction. Users can learn step by step how to interact with AIs, what they can expect from AI applications, what abilities they have, and what limits AIs possess. In other words, users can get a realistic picture of the potentials and limits of AI applications. Again, operations becoming possible and essential in the future can already be simulated in XR. That possibility offers a user-centered design and training approaches keeping pace with the time and fast-changing requirements. Acceptance, expertise, and experience with AI systems can be developed proactively instead of retrospectively.
Limitations
Although, we have many indicators stemming from investigating human-human interactions in XR (Blascovich, 2002; Bombari et al., 2015; Pan and Hamilton, 2018) and product testing in XR, simulations only resemble real human-AI interactions. Hence, we cannot be sure that the results stemming from XR interactions precisely predict human-AI interactions in real settings. For establishing XR as valid testbed, comparative studies testing real interaction settings with XR interactions would be necessary. Additionally, the easiness of producing different variants of features might mislead researchers into engendering myriad results stemming from neglectable variation. The significance of the outcome would blow out of proportion. Since XR simulations can be set up in each laboratory, results might increasingly be collected with student samples instead of real users of the corresponding AI application. Thus, it seems important to make XR testbeds attractive and available for practical stakeholders and diverse user groups.
Conclusion
AI covers a broad spectrum of computational problems and use cases. At the same time, AI applications are becoming increasingly interactive. Consequently, AIs will exhibit a certain form of embodiment to users at the human-AI interface. This embodiment is manifold and can, e.g., range from simple feedback systems, to text or graphical terminals, to humanoid agents or robots of various appearances. Simultaneously, human-AI interactions gain massively in importance for diverse user groups and in various fields of application. However, the outer appearance and behavior of an AI result in profound differences on how an AI is perceived by users, e.g., what they think about the AI’s potential capabilities, competencies or even dangers, i.e., how user’s attitude towards an AI are shaped. Moreover, many users or future users do have abstract ideas of what AI is, significantly increasing the embodiment effects and hence the importance of the specific embodiment of AI applications. These circumstances raise intricate questions of why and how humans interact or should interact with AIs.
From a human-computer interaction and human-centered design perspective, it is essential to investigate the acceptance of AI applications and the consequences of interacting with an AI. However, the sheer complexity of application fields and AI embodiments, in reality, face researchers with enormous challenges regarding valid and systematic investigations. This article proposes eXtended AI as a method for such a systematic investigation. We provided a theoretical treatment and model of Human-AI interaction based in the introduced XR-AI continuum as a framework for and a perspective of different approaches of XR-AI combinations. We motivate eXtended AI as a specific XR-AI combination capable of helping us to learn about the effects of prospective human-AI interfaces and to show why the combination of XR and AI fruitfully contributes to a valid and systematic investigation of human-AI interactions and interfaces.
The article also provided two exemplary experiments investigating aforementioned approach for two distinct AI-systems. The first experiment reveals an interesting gender effect in human-robot interaction, while the second experiment reveals an Eliza effect of a recommender system. These two examples of paradigmatic implementations of the proposed XR testbed for human-AI interactions and interfaces show how a valid and systematic investigation can be conducted. It additionally reports on two interesting findings of embodied human-AI interfaces supporting the proposed idea to use XR as a testbed to investigate human-AI interaction. From an HCI perspective, eXtended AI (left side of the XR-AI continuum) constitutes a variant of rapid prototyping for HAII. The clue is that users interact with a prestage or a simulation instead of the fully developed product. In sum, the article opens new perspectives on how XR benefits for systematic investigations are essential for an evidence-based human-centered AI design, the design and evaluation of explainable AIs and tangible training modules, and basic research of human-AI interfaces and interactions.
Data Availability Statement
The original contributions presented in the study are included in the article/Supplementary Material, further inquiries can be directed to the corresponding author.
Ethics Statement
Ethical review and approval was not required for the study on human participants in accordance with the local legislation and institutional requirements. The patients/participants provided their written informed consent to participate in this study.
Author Contributions
All authors listed have made a substantial, direct, and intellectual contribution to the work and approved it for publication.
Funding
This publications was supported by the Open-Access Publication Fund of the University of Wuerzburg.
Conflict of Interest
The authors declare that the research was conducted in the absence of any commercial or financial relationships that could be construed as a potential conflict of interest.
Publisher’s Note
All claims expressed in this article are solely those of the authors and do not necessarily represent those of their affiliated organizations, or those of the publisher, the editors and the reviewers. Any product that may be evaluated in this article, or claim that may be made by its manufacturer, is not guaranteed or endorsed by the publisher.
References
Antakli, A., Hermann, E., Zinnikus, I., Du, H., and Fischer, K. (2018). “Intelligent Distributed Human Motion Simulation in Human-Robot Collaboration Environments,” in Proceedings of the 18th International Conference on Intelligent Virtual Agents, IVA 2018 (New York: ACM), 319–324. doi:10.1145/3267851.3267867
Aragon, S. R. (2003). Creating Social Presence in Online Environments. New Dir. Adult Cont. Edu. 2003, 57–68. doi:10.1002/ace.119
Aylett, R., and Luck, M. (2000). Applying Artificial Intelligence to Virtual Reality: Intelligent Virtual Environments. Appl. Artif. Intell. 14, 3–32. doi:10.1080/088395100117142
Azmandian, M., Arroyo-Palacios, J., and Osman, S. (2019). “Guiding the Behavior Design of Virtual Assistants,” in Proceedings of the 19th ACM International Conference on Intelligent Virtual Agents, 16–18.
Bailenson, J., Aharoni, E., Beall, A., Guadagno, R., Dimov, A., and Blascovich, J. (2004). “Comparing Behavioral and Self-Report Measures of Agents’ Social Presence in Immersive Virtual Environments,” in Proceedings of the 7th Annual International Workshop on PRESENCE, 1864–1105.
Bär, N., Hoffmann, A., and Krems, J. (2011). “Entwicklung von Testmaterial zur experimentellen Untersuchung des Einflusses von Usability auf Online-Trust,” in Reflexionen und Visionen der Mensch-Maschine-Interaktion – Aus der Vergangenheit lernen, Zukunft gestalten. Editors S. Schmid, M. Elepfandt, J. Adenauer, and A. Lichtenstein, 627–631.
Bawden, D. (2008). “Origins and Concepts of Digital Literacy,” in Digital Literacies: Concepts, Policies and Practices Editors LankshearC. KnobelM. New York: Peter Lang. 30, 17–32.
Bickmore, T., Vardoulakis, L., Jack, B., and Paasche-Orlow, M. (2013). “Automated Promotion of Technology Acceptance by Clinicians Using Relational Agents,” in Lecture Notes in Computer Science (including subseries Lecture Notes in Artificial Intelligence and Lecture Notes in Bioinformatics), 68–78. doi:10.1007/978-3-642-40415-3_6
Biocca, F. (1999). The Cyborg's Dilemma. Hum. Factors Inf. Technol. 113, 113–144. doi:10.1016/s0923-8433(99)80011-2
Blascovich, J., Loomis, J., Beall, A. C., Swinth, K. R., Hoyt, C. L., and Bailenson, J. N. (2002). TARGET ARTICLE: Immersive Virtual Environment Technology as a Methodological Tool for Social Psychology. Psychol. Inq. 13, 103–124. doi:10.1207/s15327965pli1302_01
Blascovich, J. (2002). “Social Influence within Immersive Virtual Environments,” in The social life of avatars (Springer), 127–145. doi:10.1007/978-1-4471-0277-9_8
Bombari, D., Schmid Mast, M., Canadas, E., and Bachmann, M. (2015). Studying Social Interactions through Immersive Virtual Environment Technology: Virtues, Pitfalls, and Future Challenges. Front. Psychol. 6, 869. doi:10.3389/fpsyg.2015.00869
Cameron, J. (1984). The Terminator. Hendale Film Corpoperation, Pacific Western Productions, Euro Film Funding, Cinema '84.
Carolus, A., Binder, J. F., Muench, R., Schmidt, C., Schneider, F., and Buglass, S. L. (2019). Smartphones as Digital Companions: Characterizing the Relationship between Users and Their Phones. New Media Soc. 21, 914–938. doi:10.1177/1461444818817074
Carolus, A., and Wienrich, C. (2019). How Close Do You Feel to Your Devices? Visual Assessment of Emotional Relationships with Digital Devices. dl.gi.de.. doi:10.18420/muc2019-ws-652
Carpenter, J. (1974). Dark Star. Jack H. Harris Enterprise. Los Angeles, California: University of Southern California. doi:10.5962/bhl.title.84512
Cavazza, M., Lugrin, J.-L., Pizzi, D., and Charles, F. (2007). “Madame Bovary on the Holodeck: Immersive Interactive Storytelling,” in Proceedings of the 15th international conference on Multimedia MULTIMEDIA ’07 (New York, NY, USA: ACM), 651–660.
Congdon, S. P., and Schober, M. F. (2002). “How Examiners’ Discourse Cues Affect Scores on Intelligence Tests,” in Kansas City, MO: 43th Annual Meeting of the Psychonomic Society.
Cruz-Neira, C., Sandin, D. J., DeFanti, T. A., Kenyon, R. V., and Hart, J. C. (1992). The CAVE: Audio Visual Experience Automatic Virtual Environment. Commun. ACM 35, 64–72. doi:10.1145/129888.129892
Fan, L., Scheutz, M., Lohani, M., McCoy, M., and Stokes, C. (2017). “Do we Need Emotionally Intelligent Artificial Agents? First Results of Human Perceptions of Emotional Intelligence in Humans Compared to Robots,” in International Conference on Intelligent Virtual Agents, 129–141. doi:10.1007/978-3-319-67401-8_15
Field, A. (2009). Discopering Statistics Using SPSS. Thrid Edition. Thousand Oaks, California: SAGE Publications.
Fischbach, M., Wiebusch, D., and Latoschik, M. E. (2017). Semantic Entity-Component State Management Techniques to Enhance Software Quality for Multimodal VR-Systems. IEEE Trans. Vis. Comput. Graphics 23, 1342–1351. doi:10.1109/TVCG.2017.2657098
Fiske, S. T., Cuddy, A. J. C., Glick, P., and Xu, J. (2002). A Model of (Often Mixed) Stereotype Content: Competence and Warmth Respectively Follow from Perceived Status and Competition. J. Personal. Soc. Psychol. 82, 878–902. doi:10.1037/0022-3514.82.6.878
Fitrianie, S., Bruijnes, M., Richards, D., Bönsch, A., and Brinkman, W.-P. (2020). “The 19 Unifying Questionnaire Constructs of Artificial Social Agents,” in Proceedings of the 20th ACM International Conference on Intelligent Virtual Agents, IVA 2020 (New York: Association for Computing Machinery, Inc), 1–8. doi:10.1145/3383652.3423873
Fraser, J., Papaioannou, I., and Lemon, O. (2018). “Spoken Conversational Ai in Video Games: Emotional Dialogue Management Increases User Engagement,” in Proceedings of the 18th International Conference on Intelligent Virtual Agents, 179–184.
Gall, D., and Latoschik, M. E. (2018). “Immersion and Emotion: On the Effect of Visual Angle on Affective and Cross-Modal Stimulus Processing,” in Proceedings of the 25th IEEE Virtual Reality Conference (IEEE VR). doi:10.1109/vr.2018.8446153
Hart, S. G., and Staveland, L. E. (1988). “Development of NASA-TLX (Task Load Index): Results of Empirical and Theoretical Research,” in Human Mental Workload. Editors P. A. Hancock, and N. Meshkati (Amsterdam: Elsevier), 139–183. doi:10.1016/s0166-4115(08)62386-9
Hassenzahl, M., and Monk, A. (2010). The Inference of Perceived Usability from beauty. Human-comp. Interaction 25, 235–260. doi:10.1080/07370024.2010.500139
Hassenzahl, M., Wiklund-Engblom, A., Bengs, A., Hägglund, S., and Diefenbach, S. (2015). Experience-Oriented and Product-Oriented Evaluation: Psychological Need Fulfillment, Positive Affect, and Product Perception. Int. J. Human-Computer Interaction 31, 530–544. doi:10.1080/10447318.2015.1064664
Hatfield, E., Cacioppo, J. T., and Rapson, R. L. (1992). Primitive Emotional Contagion. Emot. Soc. Behav. 14, 151–177.
Ho, C.-C., and MacDorman, K. F. (2010). Revisiting the Uncanny valley Theory: Developing and Validating an Alternative to the Godspeed Indices. Comput. Hum. Behav. 26, 1508–1518. doi:10.1016/j.chb.2010.05.015
Huta, V. (2016). “Eudaimonic and Hedonic Orientations: Theoretical Considerations and Research Findings,” in Handbook of eudaimonic well-being (Springer), 215–231. doi:10.1007/978-3-319-42445-3_15
Jonas, K., Stroebe, W., and H., M. (2014). Sozialpsychologie. 6th ed. Berlin, Germany: Springer-Verlag.
Kelley, P. G., Yang, Y., Heldreth, C., Moessner, C., Sedley, A., Kramm, A., et al. (2019). “Happy and Assured that Life Will Be Easy 10years from now.”: Perceptions of Artificial Intelligence in 8 Countries. Arxiv Prepr. arXiv2001.00081. doi:10.1145/3461702.3462605
Kistler, F., Endrass, B., Damian, I., Dang, C. T., and André, E. (2012). Natural Interaction with Culturally Adaptive Virtual Characters. J. Multimodal User Inter. 6, 39–47. doi:10.1007/s12193-011-0087-z
Kuhlen, A. K., and Brennan, S. E. (2013). Language in Dialogue: When Confederates Might Be Hazardous to Your Data. Psychon. Bull. Rev. 20, 54–72. doi:10.3758/s13423-012-0341-8
Kulms, P., and Kopp, S. (2016). “The Effect of Embodiment and Competence on Trust and Cooperation in Human-Agent Interaction,” in International Conference on Intelligent Virtual Agents, 75–84. doi:10.1007/978-3-319-47665-0_7
Latoschik, M. E. (2005). “A User Interface Framework for Multimodal {VR} Interactions,” in Proceedings of the IEEE seventh International Conference on Multimodal Interfaces (Trento, Italy: ICMI 2005). doi:10.1145/1088463.1088479 Available at: http://trinity.inf.uni-bayreuth.de/download/pp217-latoschik.pdf
Latoschik, M. E., and Blach, R. (2008). “Semantic Modelling for Virtual Worlds – A Novel Paradigm for Realtime Interactive Systems?,” in Proceedings of the ACM VRST 2008, 17–20. Available at: http://trinity.inf.uni-bayreuth.de/download/semantic-modeling-for-VW-VRST08.pdf.
Latoschik, M. E., Roth, D., Gall, D., Achenbach, J., Waltemate, T., and Botsch, M. (2017). “The Effect of Avatar Realism in Immersive Social Virtual Realities,” in 23rd ACM Symposium on Virtual Reality Software and Technology (VRST), 39:1–39:10.
Latoschik, M. E. (2014). Smart Graphics/Intelligent Graphics. Informatik Spektrum 37, 36–41. doi:10.1007/s00287-013-0759-z
Latoschik, M. E., and Wachsmuth, I. (1998). “Exploiting Distant Pointing Gestures for Object Selection in a Virtual Environment,” in Gesture and Sign Language in Human-Computer Interaction, September 17-19, 1997 (Bielefeld, Germany: International Gesture Workshop), 185–196. doi:10.1007/BFb0052999
Latoschik, M. E., and Wienrich, C. (2021). Coherence and Plausibility, Not Presence?! Pivotal Conditions for XR Experiences and Effects, a Novel Model. arXiv. Available at: https://arxiv.org/abs/2104.04846.
Long, D., and Magerko, B. (2020). “What Is AI Literacy? Competencies and Design Considerations,” in Proceedings of the 2020 CHI Conference on Human Factors in Computing Systems, 1–16.
Mattar, N., Van Welbergen, H., Kulms, P., and Kopp, S. (2015). “Prototyping User Interfaces for Investigating the Role of Virtual Agents in Human-Machine Interaction,” in Lecture Notes in Computer Science (including subseries Lecture Notes in Artificial Intelligence and Lecture Notes in Bioinformatics) (Springer-Verlag), 356–360. doi:10.1007/978-3-319-21996-7_39
Mori, M., MacDorman, K., and Kageki, N. (2012). The Uncanny valley [from the Field]. IEEE Robot. Automat. Mag. 19, 98–100. doi:10.1109/mra.2012.2192811
Nass, C., and Gong, L. (2000). Speech Interfaces from an Evolutionary Perspective. Commun. ACM 43, 36–43. doi:10.1145/348941.348976
Nomura, T., Suzuki, T., Kanda, T., and Kato, K. (2006). Measurement of Negative Attitudes toward Robots. Is 7, 437–454. doi:10.1075/is.7.3.14nom
Obaid, M., Damian, I., Kistler, F., Endrass, B., Wagner, J., and André, E. (2012). “Cultural Behaviors of Virtual Agents in an Augmented Reality Environment,” in 12th International Conference on Intelligent Virtual Agents (IVA 2012) LNCS. Editors Y. I. Nakano, M. Neff, A. Paiva, and M. A. Walker (Berlin, Germany: Springer), 412–418. doi:10.1007/978-3-642-33197-8_42
Ospina-Bohórquez, A., Rodríguez-González, S., and Vergara-Rodríguez, D. (2021). On the Synergy between Virtual Reality and Multi-Agent Systems. Sustainability 13, 4326. doi:10.3390/su13084326
Pan, X., and Hamilton, A. F. d. C. (2018). Why and How to Use Virtual Reality to Study Human Social Interaction: The Challenges of Exploring a New Research Landscape. Br. J. Psychol. 109, 395–417. doi:10.1111/bjop.12290
Peck, T. C., Sockol, L. E., and Hancock, S. M. (2020). Mind the Gap: The Underrepresentation of Female Participants and Authors in Virtual Reality Research. IEEE Trans. Vis. Comput. Graphics 26, 1945–1954. doi:10.1109/TVCG.2020.2973498
Pham, D. T., and Gault, R. S. (1998). A Comparison of Rapid Prototyping Technologies. Int. J. Machine Tools Manufacture 38, 1257–1287. doi:10.1016/S0890-6955(97)00137-5
Ratan, R., Beyea, D., Li, B. J., and Graciano, L. (2019). Avatar Characteristics Induce Users' Behavioral Conformity with Small-To-Medium Effect Sizes: a Meta-Analysis of the proteus Effect. Media Psychol. 23, 1–25. doi:10.1080/15213269.2019.1623698
Razzouk, R., and Shute, V. (2012). What Is Design Thinking and Why Is it Important? Rev. Educ. Res. 82, 330–348. doi:10.3102/0034654312457429
Reeves, B., and Nass, C. (1996a). The media Equation: How People Treat Computers, Television, and New media like Real People. Cambridge, UK: Cambridge University Press.
Reeves, B., and Nass, C. (1996b). The media Equation: How People Treat Computers, Television, and New media like Real People and Places. Cambridge, UK: Cambridge University Press.
Rieser, V. (2019). “Let’s Chat! Can Virtual Agents Learn How to Have a Conversation?,” in Proceedings of the 19th ACM International Conference on Intelligent Virtual Agents, 5–6.
Russell, S. J., and Norvig, P. (2020). Artificial Intelligence: A Modern Approach. 4th ed.. Hoboken, New Jersey: Prentice-Hall.
Schreier, J. (2012). Robot & Frank. Stage 6 Films, Park Pictures, White Hat Entertainment, Dug Run Pictures New York: Park Pictures.
Scott, R. (1979). Alien. Brandywine Productions. Los Angles: Brandywine productions. doi:10.6028/nbs.sp.480-12
Scott, R. (1982). Blade Runner. The Ladd Company, Shaw Brothers, Blade Runner Partnership. Los Angles: The Ladd Company.
Skarbez, R., Brooks, F. P., and Whitton, M. C. (2018). A Survey of Presence and Related Concepts. ACM Comput. Surv. 50, 96:1–39. doi:10.1145/3134301
Slater, M. (2009). Place Illusion and Plausibility Can lead to Realistic Behaviour in Immersive Virtual Environments. Phil. Trans. R. Soc. B 364, 3549–3557. doi:10.1098/rstb.2009.0138
Slater, M., and Wilbur, S. (1997). A Framework for Immersive Virtual Environments (FIVE): Speculations on the Role of Presence in Virtual Environments. Presence: Teleoperators & Virtual Environments 6, 603–616. doi:10.1162/pres.1997.6.6.603
Sterna, R., Cybulski, A., Igras-Cybulska, M., Pilarczyk, J., and Kuniecki, M. (2021). “Pretest or Not to Pretest? A Preliminary Version of a Tool for the Virtual Character Standardization,” in 2021 IEEE Conference on Virtual Reality and 3D User Interfaces Abstracts and Workshops (Danvers: The Institute of Electrical and Electronics Engineers), 123–126. doi:10.1109/vrw52623.2021.00030
Strathmann, C., Szczuka, J., and Krämer, N. (2020). “She Talks to Me as if She Were Alive: Assessing the Social Reactions and Perceptions of Children toward Voice Assistants and Their Appraisal of the Appropriateness of These Reactions,” in Proceedings of the 20th ACM International Conference on Intelligent Virtual Agents, 1–8.
Terry, L. R., and Garrison, A. D. R. (2007). Assessing Social Presence in Asynchronous Text-Based Computer Conferencing J. J. Distance Educ. 14, 1–18.
Topál, J., Gergely, G., Miklósi, A., Erdohegyi, A., and Csibra, G. (2008). Infants' Perseverative Search Errors Are Induced by Pragmatic Misinterpretation. Science 321, 1831–1834. doi:10.1126/science.111363410.1126/science.1161437
Waltemate, T., Gall, D., Roth, D., Botsch, M., and Latoschik, M. E. (2018). The Impact of Avatar Personalization and Immersion on Virtual Body Ownership, Presence, and Emotional Response. IEEE Trans. Vis. Comput. Graphics 24, 1643–1652. doi:10.1109/tvcg.2018.2794629
Wienrich, C., Döllinger, N., and Hein, R. (2021a). Behavioral Framework of Immersive Technologies (BehaveFIT): How and Why Virtual Reality Can Support Behavioral Change Processes. Front. Virtual Real. 2, 84. doi:10.3389/frvir.2021.627194
Wienrich, C., and Gramlich, J. (2020). appRaiseVR - an Evaluation Framework for Immersive Experiences. i-com 19, 103–121. doi:10.1515/icom-2020-0008
Wienrich, C., Gross, R., Kretschmer, F., and Müller-Plath, G. (2018b). “Developing and Proving a Framework for Reaction Time Experiments in VR to Objectively Measure Social Interaction with Virtual Agents,” in 2018 IEEE Conference on Virtual Reality and 3D User Interfaces (Danvers: The Institute of Electrical and Electronics Engineers), 191–198.
Wienrich, C., Reitelbach, C., and Carolus, A. (2021b). The Trustworthiness of Voice Assistants in the Context of Healthcare Investigating the Effect of Perceived Expertise on the Trustworthiness of Voice Assistants, Providers, Data Receivers, and Automatic Speech Recognition. Front. Comput. Sci. 3, 53. doi:10.3389/fcomp.2021.685250
Wienrich, C., Schindler, K., Döllinger, N., and Kock, S. (2018a). “Social Presence and Cooperation in Large-Scale Multi-User Virtual Reality-The Relevance of Social Interdependence for Location-Based Environments,” in IEEE Conference on Virtual Reality and 3D User Interfaces (Danvers: The Institute of Electrical and Electronics Engineers), 207–214.
Yan, X., and Gu, P. (1996). A Review of Rapid Prototyping Technologies and Systems. Computer-Aided Des. 28, 307–318. doi:10.1016/0010-4485(95)00035-6
Yee, N., and Bailenson, J. (2007). The Proteus Effect: The Effect of Transformed Self-Representation on Behavior. Hum. Comm. Res. 33, 271–290. doi:10.1111/j.1468-2958.2007.00299.x
Zhang, B., and Dafoe, A. (2019). Artificial Intelligence: American Attitudes and Trends. SSRN J. doi:10.2139/ssrn.3312874
Zhang, H., Fricker, D., and Yu, C. (2010). “A Multimodal Real-Time Platform for Studying Human-Avatar Interactions,” in Lecture Notes in Computer Science (including subseries Lecture Notes in Artificial Intelligence and Lecture Notes in Bioinformatics), 49–56. doi:10.1007/978-3-642-15892-6_6
Zimmerer, C., Fischbach, M., and Latoschik, M. E. (2018b). “Space Tentacles - Integrating Multimodal Input into a VR Adventure Game,” in Proceedings of the 25th IEEE Virtual Reality (VR) conference (IEEE), 745–746. doi:10.1109/vr.2018.8446151 Available at: https://downloads.hci.informatik.uni-wuerzburg.de/2018-ieeevr-space-tentacle-preprint.pdf
Keywords: human-artificial intelligence interface, human-artificial intelligence interaction, XR-artificial intelligence continuum, XR-artificial intelligence combination, research methods, human-centered, human-robot, recommender system
Citation: Wienrich C and Latoschik ME (2021) eXtended Artificial Intelligence: New Prospects of Human-AI Interaction Research. Front. Virtual Real. 2:686783. doi: 10.3389/frvir.2021.686783
Received: 27 March 2021; Accepted: 23 June 2021;
Published: 06 September 2021.
Edited by:
Athanasios Vourvopoulos, Instituto Superior Técnico (ISR), PortugalReviewed by:
Tomas Trescak, Western Sydney University, AustraliaRichard Skarbez, La Trobe University, Australia
Copyright © 2021 Wienrich and Latoschik. This is an open-access article distributed under the terms of the Creative Commons Attribution License (CC BY). The use, distribution or reproduction in other forums is permitted, provided the original author(s) and the copyright owner(s) are credited and that the original publication in this journal is cited, in accordance with accepted academic practice. No use, distribution or reproduction is permitted which does not comply with these terms.
*Correspondence: Carolin Wienrich, carolin-wienrich@uni.wuerzburg.de
†These authors have contributed equally to this work and share first authorship