- 1Faculty of Society and Design, Bond University, Gold Coast, QLD, Australia
- 2Business School, Bond University, Gold Coast, QLD, Australia
- 3Marshall Day Acoustics, Sydney, VIC, Australia
Irrelevant ambient noise can have profound effects on human performance and wellbeing. Acoustic interventions (e.g., installation of sound absorbing materials) that reduce intelligible noise (i.e., sound unrelated to the relevant speech, including noise from other talkers within the space) by reducing room reverberation, have been found to be an effective means to alleviate the negative effects of noise on cognitive performance. However, these interventions are expensive, and it is difficult to evaluate their impact in the field. Virtual reality (VR) provides a promising simulation platform to evaluate the likely impact of varied acoustic interventions before they are chosen and installed. This study employed a virtual classroom environment to evaluate whether an intervention to reduce reverberation can be simulated successfully in VR and mitigate the effects of ambient noise on cognitive performance, physiological stress, and mood. The repeated-measures experimental design consisted of three acoustic conditions: no ambient noise, typical open-plan classroom ambient noise without acoustic treatment, and the same ambient noise with acoustic treatment to reduce reverberation. Results revealed that ambient noise negatively affected participants’ cognitive performance but had no measurable effect on physiological stress or self-reported mood. Importantly, the negative effect of ambient noise was completely ameliorated by the acoustic treatment (i.e. indistinguishable from performance in the no noise condition). The study shows that VR provides an effective and efficient means to evaluate the cognitive effects of acoustic interventions.
Introduction
Acoustical properties of buildings are still often overlooked despite the fact that negative effects of noise (i.e., background chatter and environmental sounds) on performance have been well established (e.g., Dockrell and Shield, 2006; Schlittmeier et al., 2008; Klatte et al., 2010a; Clark and Sörqvist, 2012; Reinten et al., 2017). Research has demonstrated that irrelevant speech (i.e. background chatter) constitutes the most disturbing form of noise, resulting in difficulties in maintaining concentration (Di Blasio et al., 2019). Specifically, working-memory processes are negatively impacted (Salamé and Baddeley, 1982). Furthermore, poor acoustics have been shown to trigger physiological stress responses (Ising and Kruppa, 2004; Tiesler et al., 2015) and decreases in self-reported wellbeing (Klatte et al., 2010a; Scannell et al., 2015).
Noise in schools can have deleterious effects on student learning outcomes (Berglund and Lindvall, 1995). Poor classroom acoustics contribute to poorer speech intelligibility, and lower levels of self-reported happiness amongst students (Astolfi et al., 2019). Research by Prodi et al., (2019) found that different types of noise (i.e., quiet, traffic noise, classroom noise) reduced students’ task performance and increased listening effort.
Thus, acoustic interventions, such as the installation of sound absorbing materials, constitute an important avenue to improve cognitive performance and well-being. Seddigh et al., (2014) compared the acoustic and psychological impacts of three different types of sound absorbents: 1) reflective ceiling tiles, 2) absorbent ceiling tiles, and 3) absorbent ceiling tiles plus wall panels within an office building. They found that improved room acoustics were associated with perceived lower levels of stress and cognitive disturbance among office workers.
Given that acoustic interventions can be expensive, it is important to determine the appropriate type of acoustic intervention for different settings, users, and tasks. It is, however, often not practical to comprehensively compare the effects of multiple acoustic interventions in real-world settings. Instead, dynamic high-fidelity simulations of acoustic environments in VR may provide a cost effective means to evaluate the cognitive and well-being effects of differing acoustic scenarios and interventions (Begault and Trejo, 2000; Imran et al., 2019). The availability of low-cost VR has recently enhanced interest in immersive sound studies (Serafin et al., 2018) especially in the built environment (Zhang et al., 2020). Specifically, a recent study by Muhammad et al. (2019) has provided promising results, by successfully using VR to evaluate effects of isolating sound from adjacent spaces on cognitive performance (i.e., working memory performance) and producing comparable results to real laboratory settings. In addition to sound isolation, efforts to reduce the overall noise level should also consider the reverberation characteristics of buildings (how much soundwaves are reflected by surfaces). Excessive reverberation has been established as an important psychoacoustical factor which negatively impacts speech perception and memory performance (Beaman and Holt, 2007; Ljung and Kjellberg, 2009; Klatte et al., 2010b; Braat-Eggen et al., 2019).
The aim of the current study is to test whether an acoustic intervention focused on reducing reverberation can be simulated successfully in VR, and to ascertain its effects on cognitive performance and well-being. Our specific predictions were that firstly, participants’ cognitive performance would be significantly reduced (indicated by slower response times) in the presence of irrelevant ambient noise compared to a control condition without irrelevant ambient noise. Secondly, we predicted that ambient noise would negatively impact well-being, as measured by objective physiological measures of stress (heart rate, heart rate variability, and skin conductance), and by self-reported mood. Most importantly, we predicted that the simulated acoustic intervention to reduce reverberation would mitigate the effects of noise on cognitive performance and well-being.
Materials and Methods
Design
The experiment utilized a 3 × 2 repeated-measures design, with the factors of Acoustics (No Background Ambient Noise, Untreated with Noise, Treated with Noise) and Cognitive Load (low, high). There were three classes of dependent variables: cognitive performance, physiological stress, and mood. Cognitive performance was assessed using response time measured by an n-back task (Monk et al., 2011), Physiological stress was measured by heart rate, heart rate variability and skin conductance). Positive and negative mood were assessed using the PANAS scale. All measures are described in detail in Measures of Cognitive Performance–Positive and Negative Mood. The acoustic conditions were counterbalanced to control for practice and fatigue effects.
Participants
Using G*power Version 3.1.9.3 it was estimated that to detect a medium effect (Cohen’s d = 0.5) with a probability of 80%, a minimum of 34 participants were required. To cater for potential data loss, 43 participants were recruited using Facebook, email, and the university’s psychology student participation pool. Inclusion criteria required participants to have normal, or corrected to normal vision, normal hearing, and to be at least 17 years of age. One participant was excluded from data analysis because their response pattern was indicative of task disengagement. The remaining sample comprised 42 participants of which 35 were female (83.7%) and 7 (16.3%) were male. Participants ranged in age from 18 to 29 years (M = 20.69, SD = 2.44) and 81% of them were undergraduate university students. Due to technical faults (i.e. fully or partially missing signal), there are only 39 data sets for the physiological measures.
Virtual Environment
The VR headset allowed the participant to have a six degrees of freedom 360-degree view of a simulated indoor space, in this case an open-plan classroom (see Figures 1A,B). The virtual environment was calibrated to dynamically match the participants’ position in space using a high-fidelity sound spatialization. The visual environment of the virtual classroom was developed in the Unity game engine. The acoustic rendering of the environment was prepared by creating the space in ODEON Auditorium Room Acoustic Software Version 14, with 3D impulse responses calculated from each speaker location, including instructional n-back talker location and other children and teachers in the space. Figure 1C provides a schematic layout of the environment and the virtual location of speaker, participant and noise sources. MAX MSP Version 8 software was then used to convolve anechoic (echo removal) and quasi-anechoic (sound reflection removal) recordings of human speech as well as computer generated n-back speech with the 3D impulse responses in order to generate ambisonic (high-fidelity directional) audio at the participant location. A separate MAX MSP Version 8 was then used to administer the acoustic conditions and the working memory task whilst also recording participant responses. The VR headset provided head tracking information to MAX MSP such that the sound field would rotate to match the participants head orientation. Harpex Version 1.6 software plugin for MAX 8 was used to decode the ambisonic audio to a binaural signal for the stereo headphones. The participant remained in the virtual classroom for the entirety of the experiment, and the cognitive performance task, physiological measures, and mood rating tasks were undertaken in this setting.
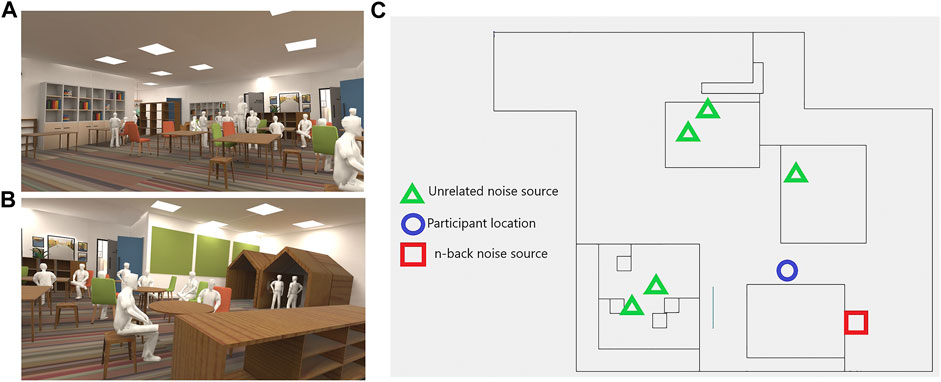
FIGURE 1. Virtual classroom environment (A,B) First-person view of the virtual environment (C) Schematic top-down view of the environment indicating the virtual positions of the speaker, participants and noise sources.
Hardware
The computer used for the VR environment was a DELL Precision 5820 computer, with an Intel Xeon 4GHz CPU, 32GB RAM and NVIDI GeForce GTX1080 Graphics Card. A HTC VIVO-Pro Eye VR Headset with 2880 × 1600-pixel resolution was used to immerse participants into the simulated classroom environment. The virtual soundscapes were presented via calibrated stereo headphones, using Beyerdynamic DT 860 with an open-back design.
Acoustic Conditions
In all three conditions the virtual soundscapes were presented via headphones. The virtual room was of an irregular shape (please see Figure 1C) with maximum dimensions of approximately 18 by 14 m in plan and a ceiling height of 2.8 m. The room volume was 560 m3. The virtual room included simulated plasterboard walls with 31 m2 of acoustically absorbent wall panels as well as a thin carpet. Table 1 provides the sound absorption coefficients for the key materials used in the virtual environment. In the untreated scenario the ceiling was modelled as plasterboard, which is inefficient at absorbing noise. For the condition with simulated reverberation-reducing treatment, the ceiling (approx. 196 m2) was changed to an acoustically absorbent ceiling tile.
The no ambient noise condition served as the control condition, and only contained the speaker presenting the n-back task (please see description in Measures of Cognitive Performance) without additional ambient noise. The untreated with noise condition simulated a classroom with ambient noise, i.e., background chatter. In the treated with noise condition, the effects of the same ambient noise were mitigated by simulated acoustically absorbent ceiling tiles to reduce reverberation. The untreated room had a reverberation time (T30 mid frequency) of 0.9 s, which reduced to 0.5 s in the treated scenario. In addition, clarity measured as C50 of the untreated room was 4dB, which rose to 13dB in the treated scenario. C50 is a measure of the ratio of early energy, within 50 ms of the direct signal, to late energy, received more than 50 ms after the direct signal (Ballou, 2013). The C50 is a commonly used to assess the influence of room acoustics on the clarity and intelligibility of speech (Bradley et al., 1999). The reduction in reverberation was predicted to cause an increase in the intelligibility of the speaker and decreased perceptual and cognitive demands placed on the individual listener (Rantala and Sala, 2015). It is important to clarify that the ambient noise was predicted to not prevent understanding the speaker (i.e., no reduction in task accuracy), but rather make it cognitively more challenging to attend to (i.e., increase in task response time). In terms of overall loudness, the three conditions were presented at comfortable listening level, with an equivalent continuous sound pressure levels of 52–56 dB LAeq. Sound pressure was measured inside the headphone using a Bruel & Kjaer type 2250 Sound Level Analyzer (see Table 2 for more detailed results).
Measures of Cognitive Performance
To measure cognitive performance, we used the popular n-back task (Monk et al., 2011). Participants are asked to respond if the current stimulus matches the stimulus presented n back in the sequence (Monk et al., 2011). A key component of the n-back task is that the task difficulty can be systematically adjusted by increasing the size of n (i.e., 0-back, 2-back, 3-back etc.; Jaeggi et al., 2010). Given that in the current study participants were required to wear the VR headset, an auditory version of the n-back task was employed. The stimuli were nine English numbers (1, 2, 3, 4, 5, 6, 7, 8, 9) spoken by a female voice presented via the headphones. Participants were required to indicate the presence of target stimuli by pressing a response button as quickly as possible. In the 2-back condition, participants had to respond, whenever the current stimulus matched the one from two steps earlier. For example, if the sequence was ‘8, 4, 2, 4, 3, 7’, ‘4’ would be the target number to which the participant must respond. The 2-back task therefore requires continuous encoding and updating of items in memory in a rule-based fashion (Owen et al., 2005). We also employed a control condition (i.e., the 0-back condition), to gauge effects of noise on a task with minimal working-memory requirements. In the 0-back condition participants were simply required to respond whenever the number ‘5’ was presented. In both conditions, there were 120 stimuli per task, 30 were target stimuli and 90 were distractors. The stimuli were separated by interstimulus intervals of 1500 ms. Each condition of the n-back task lasted for a total of 3 min. Participants’ average response times were calculated based only on correct responses (hits). Lower response times indicate greater performance and higher response times indicate poorer performance (Meule, 2017). For response accuracy, hits, misses, and false positives were analyzed using Ziemus et al. (2007) discrimination score technique: {1−[(false positives + misses)/(targets + distractors)]}×100. The discrimination score ranges from 0% (all responses are misses or false positives) to 100% (all responses are hits or correctly rejected).
Psychophysiological Measures of Stress
Three psychophysiological measures were acquired for this study: skin conductance level, heart rate, and heart rate variability. Skin conductance level is a tonic (slow changing) galvanic skin response over time (Fontanella et al., 2012) measured in micro-Siemens (μS), and is widely used indicator of measuring physiological stress (Zhang et al., 2017; Birenboim et al., 2019; Reeves et al., 2019; ). Heart rate represents heartbeats per minute (bpm; Solhjoo et al., 2019). Previous studies have shown that elevated heart rate can serve as an objective measure of psychological stress (Solhjoo et al., 2019). Heart rate variability is a measure of the variation in time between heartbeats (Thayer et al., 2012). More specifically, in this study heart rate variability was calculated as the root mean square of successive differences between normal heart beats (RMSSD), which is recommended for use as a short-term time domain measure (Malik et al., 1996; Stapelberg et al., 2018). Higher levels of psychophysiological stress are indicated by decreased levels of heart rate variability, i.e. are associated with more steady and predictable heartbeat patterns (Thayer et al., 2012; Kim et al., 2018). Skin conductance level and heart rate measures were acquired using a Shimmer3 GSR + device. Heart rate was measured via a photoplethysmogram from the participants’ earlobe. Skin conductance level was recorded via two silver-chloride electrodes attached to the participant’s fingers of the nondominant hand. The software ConsensysPRO version 1.6.0 was used to monitor and record the physiological data. The physiological measures were acquired during the n-back task, and lasted for a total of 3 min per each condition.
Positive and Negative Mood
To measure the self-reported mood associated with the different acoustic conditions, the PANAS scale was employed (Watson et al., 1988). The PANAS is a self-report questionnaire developed to measure an individual’s mood via two dimensions: negative mood and positive mood (Watson et al., 1988). The questionnaire was administered verbally since participants were wearing a VR-headset. The PANAS is a psychometrically sound measure demonstrating high reliability and validity (Leue and Lange, 2011). To avoid testing fatigue, the PANAS scale was only administered once per acoustic condition (i.e., after the 2-back task).
Procedure
To ensure standardization, laboratory conditions were consistent throughout the experiments including room temperature and layout for all participants. To ensure all changes in data were attributable to the acoustic conditions, the study was conducted in an isolated windowless laboratory. Each participant completed at least one practice trial of the 2-back task to ensure that performance was at least at 90% accuracy. This was done to avoid large practice effects during the experiment. After the practice, the Shimmer device was set up and relevant sensors attached. The headphones were then adjusted to fit the individual. Lastly, the VR headset was placed on the participant’s head and adjusted accordingly. The participants were then allowed to explore the virtual classroom to satisfy their curiosity. Following equipment set up, the participant’s dominant hand was placed on the response button and they were informed to press it for a correct answer. When the participants were ready to begin, the first acoustic condition was selected based on the counterbalancing scheme. Given that the focus of the study was on the effects of the acoustic conditions and since the difficulty effects of the 2-back vs the 0-back task are well established (Heinzel et al., 2014; Kuschpel et al., 2015), the order of the two conditions was not counterbalanced, but instead a blocked task structure was employed. Participants were always presented first with the 0-back condition followed by the 2-back task. Thus, participants always started the experiment with the low-load cognitive task. After each block (including both the 0-back and 2-back condition) of the acoustic condition, the PANAS was administered. Following this, the process was repeated for the remaining two sound conditions.
Results
A custom-built Python script was used to compute heart rate variability and to calculate averages for heart rate and skin conductance. To assess statistical significance an alpha level of 0.05 was adopted unless specified otherwise. The analyses consisted of four Analyses of Variance (ANOVA) and one Multivariate Analysis of Variance (MANOVA) to enable investigation into the effect of Acoustics (No Ambient Noise, Untreated with Noise, Treated with Noise) on working memory performance (response time), physiological measures of stress, and self-reported mood. Due to the multiple comparisons, the Bonferroni adjustment was used in post hoc analysis to ensure appropriate statistical power whilst adjusting for type I error (Crawley, 2005).
Effects of Task and Noise on Working Memory Performance
Accuracy
The descriptive statistics for response accuracy are depicted in Table 3. As expected, participants scored more accurately in the 0-back condition than in the 2-back condition. Accuracy in the 0-back condition was at ceiling level, therefore no inferential statistical analysis was conducted. This is also in line with earlier literature employing the n-back task, in which statistical analysis commonly focused on response time (Heinzel et al., 2014; Meule, 2017).
Response Time
To examine the effects of the acoustic condition on response time in the n-back task, a repeated measures ANOVA was conducted. The results revealed a significant main effect of acoustics, F (2,82) = 5.38, p = 0.006, η2 partial = 0.12. A statistically significant main effect of cognitive load (i.e. 2-back vs. 0-back) was also found, F (1,41) = 44.57, p > 0.001, η2 partial = 0.52. A nonsignificant interaction of Acoustics × Cognitive Load was revealed, F (2,82) = 1.15, p = 0.321. The significant main effects are shown in Figure 2. The simple main effect of acoustics was investigated using post hoc pairwise comparisons (Bonferroni corrected). The results indicated that the no background sound condition led to significantly faster response times (M = 788.97, SE = 14.58) than the untreated sound condition (M = 817.73, SE = 16.28; p = 0.042). Further, the untreated sound condition presented significantly slower response times (M = 817.73, SE = 16.28) than the treated sound condition (M = 789.55, SE = 15.76; p = 0.012). There was no significant difference between the no background sound condition (M = 788.97, SE = 14.58) and the treated sound condition (M = 789.55, SE = 15.76; p = 1.00) response times. These results indicate the acoustical treatment successfully reduced the negative impact of background sound on cognitive performance, for tasks with low as well as high cognitive load.
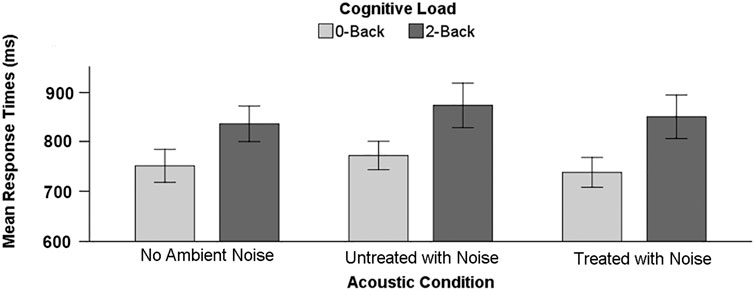
FIGURE 2. Mean Response Time For all Conditions of Acoustics and Cognitive Load. Note. Error bars = 95% Confidence Intervals (CI).
Physiological Indicators of Stress
Skin Conductance Level
To examine the effects of acoustic condition and cognitive load on skin conductance, a repeated measures ANOVA was conducted. No significant main effect of acoustics was found, F (2,76) = 0.17, p = 0.840. Further, no significant interactive effect of Acoustics × Cognitive Load was revealed, F (1.68, 63.96 Greenhouse-Geisser) = 0.34, p = 0.677. The results revealed, however, a significant main effect of cognitive load, F (1,38) = 5.03, p =0.031, η2 partial=0.12.
Heart Rate
To examine the effects of acoustic condition and cognitive load on heart rate, a repeated measures ANOVA was conducted. The results revealed a nonsignificant effect of acoustic condition F (2,76) = 0.42, p = 0.660, and a further nonsignificant Acoustics × Cognitive Load interaction F (2, 76) = 0.77, p = 0.467. The results revealed, however, a significant effect of cognitive load on heart rate, F (1,38) = 16.50, p < 0.001, η2 partial = 30.
Heart Rate Variability
To examine the effects of acoustic condition and cognitive load on heart rate variability (RMSSD), a repeated measures ANOVA was conducted. There was no statistically significant main effect of acoustics on heart rate variability, F (2,76) = 1.33, p = 0.270. The results further revealed the Acoustics × Cognitive Load interaction was nonsignificant, F (2,76 Greenhouse-Geisser) = 3.30, p = 0.050, η2 partial = 0.08. The results revealed, however, a significant main effect of cognitive load, F (1,38) = 24.45, p < 0.001, η2 partial = 0.39.
Mood
A repeated measure MANOVA was used to investigate if there were significant differences in positive and negative mood across the three levels of the independent variable (Acoustics; No Ambient Noise, Untreated with Noise, Treated with Noise). The use of a multivariate analysis is appropriate as the two dependent variables are moderately negatively correlated. The assumption of sphericity was tested using Mauchly’s test of sphericity, which revealed that both dependent variables met the assumption; Positive Mood, χ2(2) = 2.65, p = 0.265, Negative Mood, χ2(2) = 5.20, p = 0.074. The one-way repeated measures MANOVA revealed that overall the factors of acoustics had a nonsignificant effect on mood, F (4,164) = 2.20, p = 0.071.
Discussion
The current study aimed to investigate if the psychological effects of an acoustic intervention focused on reducing reverberation can be evaluated in VR. Although there is growing interest in the use of VR applications for the built environment (Zhang et al., 2020) and immersive sound simulation (Serafin et al., 2018), there is still only limited research being conducted on using VR for evaluating building acoustics and their effect on cognitive performance (Muhammad et al., 2019). Results of the current study showed that cognitive performance, irrespective of working-memory demands, was negatively affected by ambient noise, and that simulated acoustic interventions that reduced reverberation were effective in alleviating those impairments. In contrast, the physiological measures and mood were not affected by the presence of background noise and the simulated room acoustics. The consistent significant effect of cognitive load on the physiological measures indicates that the equipment and the task duration were in principle sufficient to detect physiological effects of cognitive strain. Given that earlier studies have found detrimental effects of irrelevant speech noise on self-reported wellbeing (Klatte et al., 2010a; Scannell et al., 2015) and physiological indicators of stress (Ising and Kruppa, 2004; Tiesler et al., 2015), the most parsimonious explanation for the lack of effects of noise on physiology and mood, and hence a limitation of the current study, is that the noise was not disruptive enough (i.e., low intelligibility of background chatter), or that the task duration (i.e., 3 min) per condition was too short to cause robust physiological and affective changes.
Moreover, the participant sample in the current study consisted primarily of female university students, who are an academically high performing group, used to working on demanding cognitive tasks in the presence of irrelevant sounds and poor acoustics. Younger children, the elderly, or individuals not attending university may find the situation more stressful and therefore more likely to display changes in psychophysiological and self-reported stress responses. The study by Prodi et al. (2019) found an interaction effect on performance between type of noise, age and task, in a quite homogenous group (i.e., 11–13 year old high-school students), suggesting that these effects could be further amplified in more diverse samples.
Nevertheless, the fact that high levels of reverberation produced a significant impact on cognitive performance highlights that the manipulations were strong enough to affect the participants. It also suggests that participants can be impaired in their cognitive performance in the short term without immediate effects on their well-being. Longer term exposure, as is typical in a work or school day, may well have physiological and affective consequences. Future research should explore the effects of longer-term exposure to typical work and classroom environment noise and various treatments to mitigate it. A further limitation of our study was that the control condition of no background noise is likely to be impossible to achieve in shared work and study settings, hence the need to assess acoustic treatments to ameliorate the effects of otherwise unavoidable ambient noise. Finally, since we did not compare the VR with a real-life classroom, our study could not determine whether the effects of noise are identical. This is important since an evaluation of current acoustic modeling algorithms showed that they can generate plausible but not entirely authentic simulations, i.e. human observers could distinguish simulations from recordings (Brinkmann et al., 2019). Nevertheless, our study shows that the cognitive effects of acoustic inventions can be successfully obtained in VR, as highlighted by earlier work that successfully measured the cognitive effects of simulated acoustic insulation (Muhammad et al., 2019).
In conclusion, our study highlights that high-fidelity-dynamic VR simulations of the built environment hold great promise for more efficient evaluation of acoustic interventions, and will ultimately allow more people to work and study in environments that provide optimal conditions for performance and well-being.
Data Availability Statement
The datasets presented in this study can be found in online repositories. The names of the repository/repositories and accession numbers can be found below: The data is available at Open Science Framework (DOI 10.17605/OSF.IO/X5ZWR).
Ethics Statement
The studies involving human participants were reviewed and approved by the Human Research Ethics Committee of Bond University. The patients/participants provided their written informed consent to participate in this study.
Author Contributions
All authors contributed to conception and design of the study. RD performed the data acquisition and analysis. All authors wrote and revised sections of the manuscript, read and approved the submitted version.
Funding
The study was funded internally by Bond University (Faculty of Society and Design & Business School).
Conflict of Interest
MO was employed by the company Marshal Day Acoustics.
The remaining authors declare that the research was conducted in the absence of any commercial or financial relationships that could be construed as a potential conflict of interest.
Acknowledgments
We also thank Christopher Nolan for writing software for extraction, processing and averaging the behavioural and physiological data. We also thank Yuxiao Chen for assisting with development of the MAX and ODEON acoustic models.
References
Astolfi, A., Puglisi, G. E., Murgia, S., Minelli, G., Pellerey, F., Prato, A., et al. (2019). Influence of classroom acoustics on noise disturbance and well-being for first graders. Front. Psychol. 10, 2736. doi:10.3389/fpsyg.2019.02736
Beaman, C. P., and Holt, N. J. (2007). Reverberant auditory environments: the effects of multiple echoes on distraction by 'irrelevant' speech. Appl. Cognit. Psychol. 21, 1077–1090. doi:10.1002/acp.1315
Begault, D. R., and Trejo, L. J. (2000). 3-D sound for virtual reality and multimedia. San Diego, CA: Academic Press.
Birenboim, A., Dijst, M., Scheepers, F. E., Poelman, M. P., and Helbich, M. (2019). Wearables and location tracking technologies for mental-state sensing in outdoor environments. The Prof. Geogra. 71, 449–461. doi:10.1080/00330124.2018.1547978
Braat-Eggen, E., Poll, M. K. V. D., Hornikx, M., and Kohlrausch, A. (2019). Auditory distraction in open-plan study environments: effects of background speech and reverberation time on a collaboration task. Appl. Acoust. 154, 148–160. doi:10.1016/j.apacoust.2019.04.038
Bradley, J. S., Reich, R., and Norcross, S. G. (1999). A just noticeable difference in C 50 for speech. Appl. Acoust. 58, 99–108. doi:10.1016/S0003-682X(98)00075-9
Brinkmann, F., Aspöck, L., Ackermann, D., Lepa, S., Vorländer, M., and Weinzierl, S. (2019). A round robin on room acoustical simulation and auralization. The J. Acoust. Soc. America 145, 2746–2760. doi:10.1121/1.5096178
Clark, C., and Sörqvist, P. (2012). A 3 year update on the influence of noise on performance and behavior. Noise Health 14, 292–296. doi:10.4103/1463-1741.104896
Di Blasio, S., Shtrepi, L., Puglisi, G., and Astolfi, A. (2019). A cross-sectional survey on the impact of irrelevant speech noise on annoyance, mental health and well-being, performance and occupants' behavior in shared and open-plan offices. Int. J. Environ. Res. Public Health 16, 280. doi:10.3390/ijerph16020280
Dockrell, J. E., and Shield, B. M. (2006). Acoustical barriers in classrooms: the impact of noise on performance in the classroom. Br. Educ. Res. J. 32, 509–525. doi:10.1080/01411920600635494
Fontanella, L., Ippoliti, L., and Merla, A. (2012). Multiresolution karhunen loéve analysis of galvanic skin response for psycho-physiological studies. Metrika 75, 287–309. doi:10.1007/s00184-010-0327-3
Heinzel, S., Lorenz, R. C., Brockhaus, W.-R., Wustenberg, T., Kathmann, N., Heinz, A., et al. (2014). Working memory load-dependent brain response predicts behavioral training gains in older adults. J. Neurosci. 34, 1224–1233. doi:10.1523/jneurosci.2463-13.2014
Imran, M., Heimes, A., and Vorländer, M. (2019). Real-time building acoustics noise auralization and evaluation of human cognitive performance in virtual reality. Proc. DAGA. 18–21.
Ising, H., and Krupa, B. (2004). Health effects caused by noise: evidence in the literature from the past 25 years. Noise Health 6, 5–13.
Jaeggi, S. M., Buschkuehl, M., Perrig, W. J., and Meier, B. (2010). The concurrent validity of theN-back task as a working memory measure. Memory 18, 394–412. doi:10.1080/09658211003702171
Kim, H.-G., Cheon, E.-J., Bai, D.-S., Lee, Y. H., and Koo, B.-H. (2018). Stress and heart rate variability: a meta-analysis and review of the literature. Psychia. Inves. 15, 235–245. doi:10.30773/pi.2017.08.17
Klatte, M., Hellbrück, J., Seidel, J., and Leistner, P. (2010a). Effects of classroom acoustics on performance and well-being in elementary school children: a field study. Environ. Behav. 42, 659–692. doi:10.1177/0013916509336813
Klatte, M., Lachmann, T., and Meis, M. (2010b). Effects of noise and reverberation on speech perception and listening comprehension of children and adults in a classroom-like setting. Noise Health 12, 270–282. doi:10.4103/1463-1741.70506
Kuschpel, M., Liu, S., Schad, D., Heinzel, S., Heinz, A., Rapp, M., et al. (2015). Differential effects of wakeful rest, music and video game playing on working memory performance in the n-back task. Front. Psychol. 17, 354–381. doi:10.3389/fpsyg.2015.01683
Leue, A., and Lange, S. (2011). Reliability generalization. Assessment 18, 487–501. doi:10.1177/1073191110374917
Ljung, R., and Kjellberg, A. (2009). Long reverberation time decreases recall of spoken information. Building Acoust. 16, 301–311. doi:10.1260/135101009790291273
Malik, M., Bigger, J. T., Camm, A. J., Kleiger, R. E., Malliani, A., Moss, A. J., et al. (1996). Heart rate variability: standards of measurement, physiological interpretation, and clinical use. Eur. Heart J. 17, 354–381. doi:10.1093/oxfordjournals.eurheartj.a014868
Meule, A. (2017). Reporting and interpreting working memory performance in n-back tasks. Front. Psychol. 8, 352. doi:10.3389/fpsyg.2017.00352
Monk, A. F., Jackson, D., Nielsen, D., Jefferies, E., and Olivier, P. (2011). N-backer: an auditory n-back task with automatic scoring of spoken responses. Behav. Res. 43, 888–896. doi:10.3758/s13428-011-0074-z
Muhammad, I., Vorländer, M., and Schlittmeier, S. J. (2019). Audio-video virtual reality environments in building acoustics: an exemplary study reproducing performance results and subjective ratings of a laboratory listening experiment. J. Acoust. Soc. America 146, EL310. doi:10.1121/1.5126598
Owen, A. M., McMillan, K. M., Laird, A. R., and Bullmore, E. (2005). N-back working memory paradigm: a meta-analysis of normative functional neuroimaging studies. Hum. Brain Mapp. 25, 46–59. doi:10.1002/hbm.20131
Prodi, N., Visentin, C., Borella, E., Mammarella, I. C., and Di Domenico, A. (2019). Noise, age, and gender effects on speech intelligibility and sentence comprehension for 11- to 13-year-old children in real classrooms. Front. Psychol. 10, 2166. doi:10.3389/fpsyg.2019.02166
Rantala, L. M., and Sala, E. (2015). Effects of classroom Acoustics on teachers' voices. Building Acoust. 22, 243–258. doi:10.1260/1351-010X.22.3-4.243
Reeves, J. P., Knight, A. T., Strong, E. A., Heng, V., Neale, C., Cromie, R., et al. (2019). The application of wearable technology to quantify health and wellbeing Co-benefits from urban wetlands. Front. Psychol. 10, 1–10. doi:10.3389/fpsyg.2019.01840
Reinten, J., Braat-Eggen, P. E., Hornikx, M., Kort, H. S. M., and Kohlrausch, A. (2017). The indoor sound environment and human task performance: a literature review on the role of room acoustics. Building Envi. 123, 315–332. doi:10.1016/j.buildenv.2017.07.005
Salamé, P., and Baddeley, A. (1982). Disruption of short-term memory by unattended speech: implications for the structure of working memory. J. Verbal Learn. Verbal Behav. 21, 150–164. doi:10.1016/s0022-5371(82)90521-7
Scannell, L., Hodgson, M., García Moreno Villarreal, J., and Gifford, R. (2015). The role of acoustics in the perceived suitability of, and well-being in, informal learning spaces. Environ. Behav. 48, 769–795. doi:10.1177/0013916514567127
Schlittmeier, S. J., Hellbrück, J., Thaden, R., and Vorländer, M. (2008). The impact of background speech varying in intelligibility: effects on cognitive performance and perceived disturbance. Ergonomics 51, 719–736. doi:10.1080/00140130701745925
Seddigh, A., Berntson, E., Bodin Danielsson, C., and Westerlund, H. (2014). Concentration requirements modify the effect of office type on indicators of health and performance. J. Environ. Psychol. 38, 167–174. doi:10.1016/j.jenvp.2014.01.009
Serafin, S., Geronazzo, M., Erkut, C., Nilsson, N. C., and Nordahl, R. (2018). Sonic interactions in virtual reality: state of the art, current challenges, and future directions. IEEE Comput. Grap. Appl. 38, 31–43. doi:10.1109/MCG.2018.193142628
Solhjoo, S., Haigney, M. C., McBee, E., van Merrienboer, J. J. G., Schuwirth, L., Artino, A. R., et al. (2019). Heart rate and heart rate variability correlate with clinical reasoning performance and self-reported measures of cognitive load. Sci. Rep. 9, 14668–14669. doi:10.1038/s41598-019-50280-3
Stapelberg, N. J. C., Neumann, D. L., Shum, D. H. K., McConnell, H., and Hamilton‐Craig, I. (2018). The sensitivity of 38 heart rate variability measures to the addition of artifact in human and artificial 24‐hr cardiac recordings. Ann. Noninvasive Electrocardiol. 23, e12483. doi:10.1111/anec.12483
Thayer, J. F., Åhs, F., Fredrikson, M., Sollers, J. J., and Wager, T. D. (2012). A meta-analysis of heart rate variability and neuroimaging studies: implications for heart rate variability as a marker of stress and health. Neurosci. Biobehavioral Rev. 36, 747–756. doi:10.1016/j.neubiorev.2011.11.009
Tiesler, G., Machner, R., and Brokmann, H. (2015). Classroom acoustics and impact on health and social behaviour. Energ. Proced. 78, 3108–3113. doi:10.1016/j.egypro.2015.11.765
Watson, D., Clark, L. A., and Tellegen, A. (1988). Development and validation of brief measures of positive and negative affect: the PANAS scales. J. Personal. Soc. Psychol. 54, 1063–1070. doi:10.1037//0022-3514.54.6.106310.1037/0022-3514.54.6.1063
Zhang, X., Lian, Z., and Wu, Y. (2017). Human physiological responses to wooden indoor environment. Physiol. Behav. 174, 27–34. doi:10.1016/j.physbeh.2017.02.043
Zhang, Y., Liu, H., Kang, S.-C., and Al-Hussein, M. (2020). Virtual reality applications for the built environment: research trends and opportunities. Automation in Construction 118, 103311. doi:10.1016/j.autcon.2020.103311
Keywords: virtual reality, acoustics, psychoacoustics, cognitive performance, well-being, heartrate, working memory, noise
Citation: Doggett R, Sander EJ, Birt J, Ottley M and Baumann O (2021) Using Virtual Reality to Evaluate the Impact of Room Acoustics on Cognitive Performance and Well-Being. Front. Virtual Real. 2:620503. doi: 10.3389/frvir.2021.620503
Received: 23 October 2020; Accepted: 19 February 2021;
Published: 12 April 2021.
Edited by:
Frank Steinicke, University of Hamburg, GermanyReviewed by:
Arianna Astolfi, Politecnico di Torino, ItalyRichard Skarbez, La Trobe University, Australia
Copyright © 2021 Doggett, Sander, Birt, Ottley and Baumann. This is an open-access article distributed under the terms of the Creative Commons Attribution License (CC BY). The use, distribution or reproduction in other forums is permitted, provided the original author(s) and the copyright owner(s) are credited and that the original publication in this journal is cited, in accordance with accepted academic practice. No use, distribution or reproduction is permitted which does not comply with these terms.
*Correspondence: Oliver Baumann, b2JhdW1hbm5AYm9uZC5lZHUuYXU=