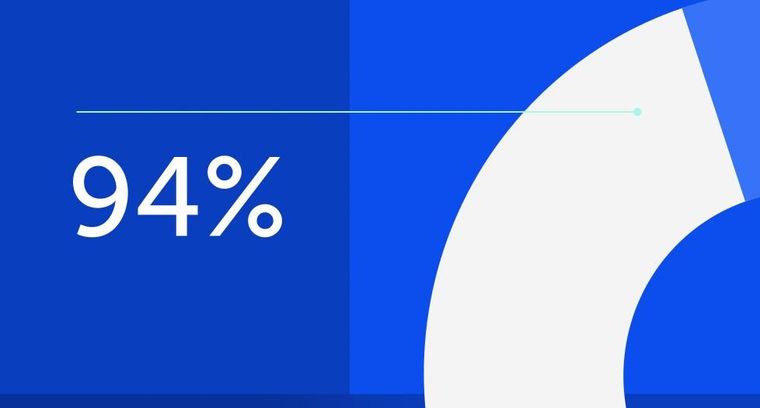
94% of researchers rate our articles as excellent or good
Learn more about the work of our research integrity team to safeguard the quality of each article we publish.
Find out more
ORIGINAL RESEARCH article
Front. Virtual Real., 12 April 2021
Sec. Virtual Reality and Human Behaviour
Volume 2 - 2021 | https://doi.org/10.3389/frvir.2021.619811
A high realism of avatars is beneficial for virtual reality experiences such as avatar-mediated communication and embodiment. Previous work, however, suggested that the usage of realistic virtual faces can lead to unexpected and undesired effects, including phenomena like the uncanny valley. This work investigates the role of photographic and behavioral realism of avatars with animated facial expressions on perceived realism and congruence ratings. More specifically, we examine ratings of photographic and behavioral realism and their mismatch in differently created avatar faces. Furthermore, we utilize these avatars to investigate the effect of behavioral realism on perceived congruence between video-recorded physical person’s expressions and their imitations by the avatar. We compared two types of avatars, both with four identities that were created from the same facial photographs. The first type of avatars contains expressions that were designed by an artistic expert. The second type contains expressions that were statistically learned from a 3D facial expression database. Our results show that the avatars containing learned facial expressions were rated more photographically and behaviorally realistic and possessed a lower mismatch between the two dimensions. They were also perceived as more congruent to the video-recorded physical person’s expressions. We discuss our findings and the potential benefit of avatars with learned facial expressions for experiences in virtual reality and future research on enfacement.
Humanlike avatars are designed to be our proxies in virtual reality (VR). In the last decade, they became increasingly realistic and the ability to animate their bodies facilitates a large range of actions and interactions in the virtual environment. For instance, the animation of gestures provides important nonverbal cues in avatar-mediated communication (Bente and Krämer, 2011; Nowak and Fox, 2018). The technical advancement of real-time VR systems facilitates tracking of the user’s body and the animation of its avatar with a high degree of realism (Achenbach et al., 2017; Waltemate et al., 2018). Recent works indicate that also facial expression tracking will soon become possible with head-mounted displays (Lombardi et al., 2018; Thies et al., 2018). On the one hand, this provides great opportunities to enrich avatars with nonverbal facial behavior (Gonzalez-Franco et al., 2020; Herrera et al., 2020; Kruzic et al., 2020). On the other hand, the animation of the avatar’s face is required. It is well known that the creation of realistic facial expressions in humanlike avatars is challenging (Lewis et al., 2014; Dobs et al., 2018). A large variety of readily prepared avatar faces is available through avatar creation software and online stores. Most of these characters were designed by artistic experts. Since such a design procedure is rather subjective, the avatar’s realism is to be explored more closely.
The realism of an avatar can be characterized along different dimensions (Nowak and Fox, 2018; Oh et al., 2018). The most prominent dimension is photographic realism. It usually pertains to the characteristics of the avatar’s static appearance (i.e., shape and texture) and the synthesis of its display (e.g., shading). A high degree of photorealism was found to modulate the intensity of embodiment (Kilteni et al., 2015; Latoschik et al., 2017). It also increases emotion contagion on humans in avatar-mediated communication (Volante et al., 2016). The avatar’s behavioral realism is another crucial dimension and is basically associated with the dynamic properties of an avatar. For instance, the term has been used by Blascovich et al. (2002) to describe the degree to which an avatar appears to behave as it would do in the physical world. More pronounced behavioral realism was shown to increase the perceived social potential of an avatar (Breazeal, 2003) and to induce the illusion of interacting with a human (Blascovich et al., 2002). Avatars behaving more humanlike seem to be perceived as more persuasive (Bailenson and Yee, 2005; Guadagno et al., 2007) and also foster the nonverbal behavior of users (Herrera et al., 2020). Previous works have interpreted behavioral realism differently, ranging from physical to social properties of the avatar’s behavior, which can thus be regarded as a multifaceted construct (Nowak and Fox, 2018). In this study, we specifically focus on the physical properties of an avatar’s animated facial expression, i.e., the naturalness of motion of the facial surface.
Achievement of a high photographic and behavioral realism of avatars is valuable for many applications in VR. However, changing an avatar toward high photographic realism can lead to undesired effects, such as the uncanny valley. Previous work indicated that both dimensions of realism interact in the perception of expression intensity in static faces (Mäkäräinen et al., 2014). Research on the uncanny valley hypothesis showed that a mismatch between photographic and behavioral realism can cause disturbance of visual face processing (de Borst and de Gelder, 2015; Kätsyri et al., 2015; Dobs et al., 2018). This supports similar findings with avatars in VR, where such a mismatch negatively affected experiences like presence and embodiment (Garau et al., 2003; Bailenson and Yee, 2005; Zibrek and McDonnell, 2019). In such works, manipulation of photographic realism was commonly achieved by changes in the avatar’s shape, texture, or shading. However, the variation of behavioral realism of an avatar’s face in the sense of naturalness of motion was limited so far, for instance, to different intensities along the spectrum from neutral to exaggerated expressions (Mäkäräinen et al., 2014) or differences in eye gaze behavior (Garau et al., 2003; Bailenson and Yee, 2005). Previous research also primarily utilized static images of manipulated expressions (Dobs et al., 2018). Until today, only little is known about how animated facial expressions in different human-like avatars affect behavioral realism and its interaction with photographic realism. This generally challenges the creation of realistic avatars.
The goal of the present work is to focus on the behavioral realism of differently created but similarly animated avatar faces. To assess how their behavioral realism can be varied, we initially discuss common methods for the creation of avatar faces with facial expressions. As a more objective alternative to the design of faces and expressions by artistic experts, we motivate the usage of statistical shape analysis of a large 3D facial expression database. We established a statistical model of facial identity and expressions that we call the Facial Expression Morphable Model (FexMM) and developed a method for the creation of a new type of avatar faces. The resulting avatars are compared to avatars with artistically designed facial expressions. Both types of avatars were similarly animated by tracking a video-recorded physical person’s facial expressions. We investigated the photographic and behavioral realism of the animated avatars in an online rating study. Although a virtual face itself might be perceived as realistic, its expressions can be incongruent to an equivalent expression displayed by a physical face. In our case, for example, an observer might perceive a mismatch in the smile of the video-recorded person and the smile of the imitating avatar with respect to its intensity or emotional connotation. As another aspect of behavioral realism, we thus investigate the effect of behavioral realism on perceived congruence between the physical and virtual faces. Since previous experience with avatars might have a moderating effect on this relationship (Busselle and Bilandzic, 2012; Jeong et al., 2012; Zhang et al., 2015; Manaf et al., 2019), we also examined if this experience will explain individual differences in the effect of behavioral realism on perceived congruence.
Today, avatars are available from a large variety of sources. Avatar creation software, such as MakeHuman, Character Generator, or Poser, and several online stores offer characters that are readily prepared for usage in VR. Most of these avatars are designed by artistic experts and often contain stylized or fictitious features. For instance, it can be observed that they resemble stereotypes shaped by the game and entertainment industries. However, an assessment of their realism is challenging. An alternative for the creation of avatars with a high degree of photographic realism is the usage of 3D scanning techniques (Grewe and Zachow, 2016; Achenbach et al., 2017). Unfortunately, 3D scanning is still expensive and elaborate postprocessing is often needed before the created avatars can be used in VR. A more practical approach is the reconstruction of avatars from a few conventional photographs. Specifically for faces, this method has been greatly advanced more recently (Ichim et al., 2015; Thies et al., 2016; Huber et al., 2016). For instance, the software FaceGen (FG)1 can create an individualized avatar from a frontal and two lateral photographs only. The individualized avatars provide a high degree of photographic realism, are readily prepared for VR, and became well-established in psychology (e.g., Todorov et al., 2013; Gilbert et al., 2018; Soto and Ashby, 2019; Hays et al., 2020).
Due to technical challenges, methods for avatar individualization from photographs currently only reconstruct the static neutral shape and the texture of the face (Egger et al., 2019 for a recent review). Such a static avatar is often equipped with nonverbal facial behavior by the transfer of a predefined facial expression model. This model preferably contains independent expression components like Action Units (AUs) (Ekman et al., 2002) in order to maximize its expressiveness (Lewis et al., 2014). The AUs are typically designed by artistic experts, like in the expression model, which is used in FG. However, the design of realistic AUs is a subjective procedure and particularly difficult if they should be applicable for a large variety of faces. Further, the combination of multiple AUs easily leads to undesired artifacts (see Figure 2). Given humans’ sensitivity toward faces, even the most subtle implausibility can lead to disturbances of visual face processing and potentially impedes the behavioral realism of the avatar.
Digital facial morphometry provides an alternative approach to the design of expression models by means of statistical shape analysis (Egger et al., 2019). For instance, dimension reduction techniques such as Principal Component Analysis (PCA) or tensor decomposition can be applied to extract the most representative expressions from a large 3D face database. They can then be combined into a statistical expression model. By learning such a model from physical data, the above issues with designed expression models can potentially be avoided. Digital facial morphometry has been previously used to establish statistical models of facial identity and expressions, like the Basel Face Model 2017 (Gerig et al., 2018) and the FLAME (Li et al., 2017). Unfortunately, most current statistical expression models are limited in their expressiveness. The reason lies in the facial expression databases (Egger et al., 2019 for an overview). Almost all databases contain characteristic AU combinations, which typically appear in spontaneous and posed expressions of the same type. For instance, in an expression of fear, the opening of the eyes and mouth often goes along with the lifting of the eyebrows. Dimension reduction techniques are usually unable to decompose these correlations when they are inherently present in the entire database. In consequence, they also appear as components of current statistical expression models. For instance, a typical model contains a component that corresponds to the average expression of fear. Decomposition methods have been proposed in order to further split them into independent expression components (Blanz and Vetter, 1999; Li et al., 2010; Tena et al., 2011; Cao et al., 2013; Neumann et al., 2013); however, to our knowledge, no statistical expression model has yet been published that a) contains independent AUs components, b) was statistically learned from a large and diverse database of 3D face scans, c) did not rely on designed expression models as priors during construction, and d) is suitable to create avatars for VR.
To improve the realism of avatar faces, we investigated the benefit of data-driven methods by training of a statistical model of facial identity and expressions. We call this new model the FexMM. It can be used to create faces across a large range of identities and allows flexible animation of expressions. Based on the FexMM, we also describe an automated method that allows convenient individualization of avatar faces from a few portrait photographs, which have been acquired from different perspectives.
For our statistical face analysis, we used the Binghamton University 3D Facial Expression database (BU3DFE) (Yin et al., 2006). It contains 2,500 high-quality stereophotogrammetric scans of 100 individuals (56% female) of various ethnicities. In addition to neutral faces, each person was scanned in six posed expressions of four intensity levels according to the basic expression categories, such as anger, disgust, fear, happiness, sadness, and surprise (Ekman et al., 2002). The faces in the database consist of textured surface meshes with a varying amount of vertices, but statistical analysis requires a fixed amount across all scans. A common surface mesh with 1,827 vertices and 1,750 quads is transferred to all scans using the approach proposed by Grewe et al. (2018). As shown in Figure 4, we used the face mesh of the MakeHuman2 project since it is suitable for a broad range of applications, including VR. Also, it has been previously used in psychological research (Hays et al., 2020). Compatibility of the face mesh facilitates combination with the full body avatars created with MakeHuman. With our model, we focus on the part of the face that is primarily affected by expressions and commonly captured across all scans in the BU3DFE.
Face scans usually differ in head pose since persons are free to move in front of the scanner. Prior to statistical analysis, pose variation needs to be removed by rigid alignment to a common coordinate system. In contrast to existing statistical face models, we aligned all scans to a cranial coordinate system (CCS). A few anthropometric landmarks were used that remain stable even under large expression deformations, such as a wide opening of the mouth (Grewe et al., 2018). An alignment within a CCS is particularly beneficial to facilitate the attachment of additional facial details like the eyes, teeth, or hair.
The faces in the BU3DFE vary within and between persons. Prior to statistical analysis, we separated variation in expressions from identity by computing differences between the neutral scan of a person and its six basic expressions. These differences were transferred to the average neutral face such that a new set of 2,400 facial expressions, i.e., without the neutral cases, with a constant identity was generated. The BU3DFE contains bilateral and thus mainly symmetric expressions. We symmetrized the expressions between both hemifaces using the approach of Klingenberg et al. (2002). However, asymmetry in expressions can still be easily achieved by constraining the motion differently for each half.
Similar to previous expression models, we used PCA for dimension reduction. The resulting principal components (PCs) typically describe characteristic combinations of multiple AU that are mainly related to the posed expressions in the BU3DFE. In other words, the entire face is always deformed by each PC. Figure 1 shows a PC that is related to an expression of sadness together with the respective magnitude of facial deformation. To split up the global deformation into locally concentrated components, we further transform the PCAs by application of Varimax rotation (Kaiser, 1958). Figure 1 illustrates that the rotated components still contain minor deformations in other areas. In order to obtain an ideal AU, undesired deformation was manually removed using simple mesh editing tools. This leads to AUs that only locally deform the face as intended.
FIGURE 1. Comparison of the surface deformation and deformation magnitude, intensity-coded from white (low) to black (high), between a PC of a sad expression, the varimax-rotated component including depression of the lip corners, and the final edited AU.
Finally, the magnitude of deformation per AU should remain within the range of realistic facial motion. This is particularly important if multiple AUs are combined such that an imbalance becomes apparent. For instance, as shown in Figure 2, the combination of AUs 1, 2, 5, 20, and 26 is characteristic of a prototypic expression of fear. We rescaled the AU into units of intensity as defined by the Facial Action Coding System (FACS), i.e., six increasing steps of intensity from the face in rest to maximum activation. This was achieved by determining the distribution of intensities over all expressions in BU3DFE. We used nonnegative least squares (Mullen and van Stokkum, 2012) to project each scan onto the obtained set of AUs. The 90th percentile intensity was considered full intensity and the AUs were rescaled accordingly. The final expression model is shown along with expert-designed AUs in Figure 2.
FIGURE 2. Comparison of AU activations between the FexMM and FG expression models. Artifacts arise for combination of multiple AUs in the designed FG model, like the folding on the forehead in fear illustrated on the right.
To create a morphable avatar face that also describes interindividual variation, we learned a statistical identity model from the neutral scans in BU3DFE. As described in Weiss et al. (2020), we analyzed symmetric and asymmetric shape variation separately using PCA. This results in symmetric and asymmetric identity components that can be varied independently. We additionally regressed facial shape onto sex and ethnicity. Some components of the resulting model are shown in Figure 3. By specifying a weight for each of the components, the respective characteristics can be combined and various new facial identities can be generated. We also determined the distribution of faces in the BU3DFE along the components. This allows to quantify plausibility of the resulting faces and therefore ensure realism. The ability to specifically manipulate faces enhances the scope of research that can be addressed (e.g., Todorov et al., 2013; Ma et al., 2018; Hays et al., 2020).
FIGURE 3. Identity components of the FexMM rendered with an average texture. The first two components are obtained via regression analysis, the latter two via PCA. Please note that texture related facial details are missing due to averaging.
The facial identity and expression models are combined into our FexMM. Since only the shape of the surface was analyzed statistically, we added additional models for facial details like the eyes, teeth, and the inner of the mouth (see Figure 4) to increase visual realism. By attaching these models to the CCS, facial details can be manipulated in accordance with changes in the identity features. The position of eyes, teeth, etc., can easily be changed on demand, e.g., to create distinct faces or compensate for minor inaccuracies. Once the identity of an avatar is specified, all additional models for facial details of the upper third of the face will remain in place. However, the lower jaw needs to follow the motion of the facial surface, which is defined by the expression model, e.g., AU 26 for jaw drop. We approximate the jaw axis within the CCS and apply a rotation to the respective parts of the mouth (lower teeth, gums, and tongue) relative to the motion of a landmark that is centrally located on the chin, i.e., pogonion. To enhance real-time capabilities of the virtual face, such joint motion can be precomputed can be precomputed.
FIGURE 4. The FexMM with eyes, teeth, tongue, and gums, showing a happy expression through activation of the AUs 10, 12, and 26.
For convenient individualization of the FexMM, we implemented an automated method that reconstructs the individual 3D shape and texture from a few conventional photographs or a selfie video, as shown in Figure 5. Initially, all pictures are fed into the OpenFace toolkit for landmark detection (Baltrusaitis et al., 2018). We employ the method of Huber et al. (2016) for the estimation of camera geometry and reconstruction of identity coefficients of the FexMM. A high-resolution photographic texture (e.g., 2,0482 or 4,0962 pixels, depending on the quality of input images) is generated by merging patches from best viewing perspectives. For each texture patch, low-frequency components of diffuse scene light are removed using spherical harmonics for light estimation (Ichim et al., 2015). Disturbing stitching artifacts may arise when seams between different patches pass through primary facial structures like eyes and mouth. Hence, we force seams to be in less significant areas using a predefined segmentation of the face mesh by means of vertex correspondence. The final texture is composed of all patches via Poisson blending. The result of the individualization pipeline is an animatable avatar face with a high degree of photographic realism.
FIGURE 5. Individualized avatar automatically reconstructed from several photographs. After merging of patches, the photographic texture is nonrigidly aligned with the template to accurately match the outlines of facial structures.
The goal of the user study was a) to investigate photographic and behavioral realism and their mismatch of differently created avatars and b) to examine the effect of behavioral realism onto perceived congruence of avatars as they imitate the facial expressions of physical persons. We compared two types of avatars in our study. In addition to our newly developed FexMM avatars, we selected the avatar creation suite FaceGen Modeller Core 3.18 (FG) since this software a) is widely established in behavioral sciences (e.g., Todorov et al., 2013; Gilbert et al., 2018; Soto and Ashby, 2019; Hays et al., 2020), b) uses an artist-designed AU expression model, and c) supports individualization from photographs. Consequently, the FexMM and FG avatars can be created from the same portraits of individuals such that they show the same photorealistic identity features. This specifically facilitates the analysis of differences in behavioral realism.
We developed two tasks for the comparison of the avatars in an online study. Firstly, each avatar was rated with respect to its photographic and behavioral realism (realism rating task). The goal of the first task was to evaluate the differences in ratings of realism between the two avatar types and the mismatch between the two dimensions of realism. Secondly, the behavioral similarity between the facial motion of a physical person and an imitating avatar was rated (simultaneous similarity rating task). With the second task, we aimed to analyze perceived congruence for different avatar types and to test the previous experience of the participants with avatars as a moderator of the relationship between behavioral realism and perceived congruence.
We chose two female and two male faces from our photographic database. For each face, four stereo photographs of the neutral expression were captured with our stereophotogrammetric setup (Grewe and Zachow, 2016). All individuals gave their consent for the use of their data in further research projects.
The reconstruction method in the FG software requires a frontal photograph and two lateral photographs. It determines the individual shape and photographic texture from a couple of facial landmarks that are interactively located in the pictures by an operator. The four FG avatars were reconstructed with a full set of AUs and standardized mouth interiors, including teeth, tongue, and gums. Individualized FexMM avatars were created for the same four individuals as described in sec:animatable_face_model. In contrast to FG, with our approach, the textured 3D shape was obtained fully automatically. Since the FexMM includes a standard eye model, individual iris color in the photographs was matched to one of eight template textures from Wood et al. (2016). In total, eight avatars were created (2 types × 4 identities, see Figures 5, 6, for examples).
FIGURE 6. A frame of the input video showing the video-taped person along with individualized and animated avatars. Please note that the person in the input video differs intentionally from the individualized avatar to avoid an effect of identity in the similarity rating task.
The animation of facial expressions plays a crucial role in comparing the behavioral realism of both avatar types. We therefore let the avatars imitate the facial motion of physical persons. For our study, video-recorded performance was used. We chose clips of different actors from the MMI Facial Expression database (Valstar and Pantic, 2010), which mimic one of the prototypical expressions (anger, disgust, fear, sadness, surprise, and happiness). We chose actors with different identities to avoid confounding effects in the behavioral similarity rating task (e.g., comparison of identity). A female and a male actor were selected for each expression category. Actors and avatars were matched by sex. The clip’s length ranged from 2 to 4 s. The faces were tracked with the OpenFace toolkit (Baltrusaitis et al., 2018), yielding frame-wise prediction of head pose and AU intensities. The noisy predictions were smoothed in the time domain such that the animation parameters describe continuous and uniform motion. We employed exponential smoothing with αAU = 0.4 and αpose = 0.7 fall-off per frame. This produces smooth animations of the avatar faces, which were still in synchrony with the input videos.
The avatars were animated and rendered with Eevee in Blender3 2.82. Initially, the FG and FexMM avatars were aligned to the same head pose. Photographic textures were displayed using the Principled BSDF shader. All pose and AUs that were tracked by OpenFace were transferred to the avatars. An animation was rendered into a sequence of uncompressed images of size 1,0802 and finally composed into a web-compatible video file. An input frame showing the actor and the correspondingly rendered avatars can be seen in Figure 6. In total, 48 sequences were rendered (2 avatar types × 4 identities × 6 expressions). Since the FG and FexMM avatars were animated with the same parameters, differences can be fully attributed to photographic and behavioral realism produced by the models.
All data in the user study were collected online with SoSci Survey.4 The animated avatars were presented to human participants in two tasks, a realism rating task and a simultaneous similarity rating task. Each task included 48 experimental trials (50% FexMM avatars), presented in a randomized order. Prior to the tasks, participants were asked to report on their experience with avatars on a Likert scale ranging from 1 = “not at all” to 5 = “very much” experienced.
In the realism rating task, participants saw video sequences for all of the animated facial avatars, one at a time. They rated the avatars with respect to their behavioral realism (“How realistic is the expression of the avatar?”) and their photographic realism (“How realistic is the avatar with respect to its appearance?”) on a visual analog scale ranging from 0 = “definitely not realistic” to 100 = “definitely realistic.” There were 48 experimental trials for each video sequence. In each trial, participants had the chance to replay the video clips as many times as desired before providing their ratings. In order to standardize the scale definition across participants, we provided a short definition of the realism dimensions in the instruction. To let participants be familiarized with the rating procedure, they had a practice trial at the beginning of the experiment. The practice trial showed the same item format under the same conditions as previously described with stimuli that were not used in the main study.
In the simultaneous similarity rating task, each avatar was presented along with the corresponding clip of the physical person’s expression. In detail, we had the participants watch each pair of video clips side by side. Note that in every pair, the facial motion tracked from the physical person’s face was used to animate the avatar. However, depending on the type of avatar, its behavioral similarity to the physical person varied across the 48 experimental trials. Again, participants had the chance to replay the video clips as many times as they desired before providing their ratings. They were asked to evaluate the proportions of behavioral similarity (“How well does the avatar mimic the facial expression of the real person?”) between the two on a visual analog scale ranging from 0 = “definitely not in line” to 100 = “perfectly in line.”
To control the data quality in our online study, we included attention checks in the congruence rating task. In these trials, completely mismatching facial expressions between the video-recorded physical face and the avatar were displayed (e.g., human stimulus displaying a happy facial expression and the avatar a sad one). The mismatch was easily recognizable given attentive task processing such that a definite disagreement was expected as a response. Participants who failed these trials were excluded from the data analyses. One hundred participants were recruited by advertising the study on the mailing lists of the university. Overall, after removing six participants who failed the attention check, the final sample consisted of N = 94 participants, age range 18–57 years, M = 23.20, SD = 2.96. About 67.02% of the participants were female.
First, we conducted repeated measures ANOVA (rmANOVA) separately for photographic and behavioral realism ratings as dependent variables. A third rmANOVA was performed with the dependent variable representing the person-specific absolute difference between photographic and behavioral realism ratings for each avatar. We included the within-person factors avatar type (two levels) and facial expression (six levels) as independent variables, as well as their interaction. These models test whether one of the avatar types was perceived as more realistic than the other and whether these differences are specific for expression categories. Due to violation of the assumptions for rmANOVA, i.e., normally distributed variables, and variance homogeneity, we used a robust, rank-based ANOVA-type statistic [nparLD package in R, Noguchi et al. (2012)]. As a nonparametric method, ANOVA-type statistics perform well with non-Gaussian data and heteroscedasticity (Brunner et al., 2017).
Second, given the above design, the acquired data are nested for stimuli and participants. To investigate whether the perceived behavioral realism depending on the avatar type was mediated by photographic realism (see Figure 7), we applied a Linear Mixed-effects Model (LMM), including random effects for persons. We expected photographic realism to partly, but not fully, explain the effect of avatar type on behavioral realism. This is to ask whether differences in behavioral realism between FG and FexMM go beyond potential differences in photographic realism. The direction of influence between both dimensions of realism might, however, be two-sided. We, therefore, first explore their directional dependence with the method proposed by Sungur (2005).
FIGURE 7. The mediation model with persons as level two units. The LMM was estimated to study whether differences in avatar type with respect to behavioral realism are driven by differences in photographic realism.
Third, because stimulus-related variation is relevant for perceived congruence, we next applied LMMs, including a random intercept for stimuli to capture between stimulus variability. As Figure 8 illustrates, we aimed to 1) test the effect of the two avatar types on perceived congruence between human and avatar expression (β1). 2) The effect was expected to be mediated by behavioral realism (β2 × β3) and the mediation to depend on users’ experience with avatars. Statistical significance of fixed effects was evaluated by using type III Wald F-tests with Kenward-Roger degrees of freedom (Kenward and Roger, 1997). A backward model selection procedure was applied, starting with a full model including all covariates and second-order interactions (Bliese and Ployhart, 2002). For LMM analysis, we used the lme4 package in R (Bates et al., 2007).
FIGURE 8. The LMM with moderated mediation and stimulus as level two units. It was estimated to study whether differences in avatar type with respect to perceived congruence are driven by differences in behavioral realism and whether this effect differs depending on the amount of the user experience with avatars. β, regression weights; ϵ, residual variance.
There was a significant main effect of the type of avatar
FIGURE 9. Boxplot of photographic realism ratings comparing the two avatar types. Level of significance is indicated by ****
Similarly, there was a significant main effect of the type of avatar
FIGURE 10. Boxplot of behavioral realism ratings comparing the two avatar types. Level of significance is indicated by **
Furthermore, we investigated differences related to the type of avatar on the within-person absolute difference between photographic and behavioral realism ratings. This is a realism mismatch indicator of facial avatars. Same as for photographic and behavioral realism ratings, there was a significant main effect of the avatar type
FIGURE 11. Boxplot of realism mismatch computed as absolute differences between the single ratings. The boxes denote the interquartile range and the median. Level of significance is indicated by *
First, we conducted a directional dependence analysis between the two realism dimensions. To this purpose, two LMMs with different effect directions were estimated. In the first model, the photographic realism was set as a dependent variable, whereas the direction was inverted in the second model. According to a bootstrapping analysis with 10,000 resamplings, the third central moment of the regression residuals in the first model was significantly larger than in the second model
Figure 7 illustrates the LMM mediation model including a random effect for persons. The total effect
Perceived congruence to the video-taped peoples’ facial expressions was predicted by avatar type, behavioral realism, previous experience with avatars, and their two-way interactions. Photographic realism was controlled for in the model. The intraclass correlation (ICC = 0.24) indicated that a considerable amount of variance was at the second level of the data structure, motivating the mixed-effects model. The results revealed higher similarity ratings for the FexMM avatars
FIGURE 12. Moderation effect of previous experience on the relationship between behavioral realism and perceived congruence.
To further understand the moderation effect of previous experience with avatars, we followed up this interaction by estimating regions of significance using the Johnson-Neyman technique (Bauer and Curran, 2005). Regions of significance indicate values of measured experience for which the slope of behavioral realism on perceived congruence turned out to be significant. The results revealed that the effect of behavioral realism was significant for experience scores below 5.97, thus exceeding the Likert scale, which ranged from 1 to 5 (see Figure 13). In other words, for very high levels of previous experience, the effect of behavioral realism on congruence perceptions seems dispensable.
FIGURE 13. The Johnson-Neyman plot shows the threshold of previous experience (blue dashed line) for a significant effect of behavioral realism on perceived congruence.
The influence of avatar type on perceived congruence was examined by conducting an LMM moderated mediation analysis with behavioral realism as mediator and experience as moderator (see Figure 8). According to a bootstrap confidence interval with 10,000 resamplings, the conditional indirect effect of the moderator excluded zero (95% CI [−0.2, −0.005). This indicates that the indirect effect differed depending on the level of previous experience with avatars. Specifically, the indirect effect of behavioral realism on perceived congruence was stronger when the previous experience was 1 SD below the mean (
Many experiences in VR, such as avatar-mediated communication and embodiment, can profit from the animation of nonverbal behavior of avatars (Oh et al., 2018). Especially, the creation of facial expressions is difficult due to the sensitivity of face perception in humans (Dobs et al., 2018). Even subtle implausibilities and artifacts can impede the perceived realism (Kätsyri et al., 2015). Further, an animated facial expression of an avatar might be perceived as incongruent to the same expression that is displayed by a physical person since its intensity or emotional connotation can differ (Mäkäräinen et al., 2014). We investigated the behavioral realism of two types of avatars with either statistically learned or designed facial expressions. We employed statistical shape analysis of a large 3D face and expression database to establish our FexMM, and described how it could be used to create realistic avatar faces. Virtual faces created with our FexMM were compared to avatars comprising designed expressions as created by FG. Both avatars were created from photographs of four individuals, which ensures that each pair of avatars shares similar identity features. Each pair of avatars was similarly animated by tracking the facial motions from video-recorded physical individuals. Their differences can consequently be attributed to the level of either photographic or behavioral realism. This allowed us to specifically compare the differences between the subjectively designed and statistically learned expression models.
The results of our study show that animated avatar faces being created with the FexMM were rated more photographically and behaviorally realistic. The mismatch between the two dimensions was also reduced for this type of avatars. Because all other components were similar and we controlled for differences in photographic realism, the increase in behavioral realism can basically be related to the usage of the statistically learned expression model. In line with the suggestions of Lewis et al. (2014) and common usage of 3D scanning and motion capture in high-end entertainment productions, our results provide first empirical indicators of the advantage of measurement-based creation of avatar faces and expressions over subjective design processes and the impact on psychological experiments.
The realism ratings also revealed the potential for improvement of the FexMM. For instance, the mismatch analysis indicates a need for refinement of the AUs, which are primarily involved in expressions of anger. Further, an outlier analysis pointed to certain animated sequences of FexMM avatars that received low ratings in behavioral realism by some participants in our study. Remarkably, the same statistical expression model was used in all avatars of this type, such that it remains to be investigated why this applies only to a few identity-expression combinations. One potential technical explanation could be that the avatars differed in the attachment of facial details like the inner of the mouth. Specific animations might have produced subtle artifacts, for example, implausible teeth motion during jaw drop. The integration of an anatomically more plausible model of the temporomandibular joint may be an alternative to improve realism.
We also demonstrated that the perceived congruence between the physical and the virtual expressions was larger for avatar faces with a higher behavioral realism. The congruence ratings might have suffered from the imperfection of the employed face tracking method; however, both avatar types were animated using the same tracked motion parameters. Further, the identities of the physical and the virtual faces were different such that an effect of similarity in identity features on congruence ratings can be excluded. This supports our conclusion that the improvements in perceived congruence were due to the higher behavioral realism of FexMM avatars. We further discovered that previous experience in dealing with avatars moderates the relationship between behavioral realism and perceived congruence. Especially participants who were highly experienced with avatars rated the facial expressions of the video-recorded individual and the imitating virtual face similarly, regardless of its level of realism. Such an adaptation to virtual environments is in line with previous studies suggesting that a user’s previous experience has an effect on the intensity of embodiment (Ferri et al., 2013; Liepelt et al., 2017) and is also consistent with research showing that individual affinity toward characters affects their perception in movies and games (Busselle and Bilandzic, 2012; Jeong et al., 2012; Zhang et al., 2015; Manaf et al., 2019). For the generalizability of our results, it would be worthwhile to conduct a future study on this adaptation effect with a more diverse sample, e.g., in age or culture.
Over all applied measures, our study shows that the behavioral realism of animated facial expressions depends on photographic realism and correlates with the perceived congruence of virtual and physical expressions. We demonstrated that avatars based on learned expressions received higher ratings of photographic and behavioral realism over avatars with artistically designed expressions as provided with FG. The FexMM thus provides a valuable tool for many applications in VR. For instance, the FexMM is beneficial in avatar-mediated communication since it can imitate expressions with high behavioral realism and congruence (Bente and Krämer, 2011; Nowak and Fox, 2018). Previous work also suggested that a high degree of realism in avatars strengthens experiences of embodiment (Kilteni et al., 2015). It is reasonable to assume that a similar relationship also exists for faces, but only a few works have investigated enfacement in VR so far (Serino et al., 2015; Estudillo and Bindemann, 2016; Ma et al., 2017). The effects were weak and visuomotor stimulation was rather limited (Porciello et al., 2018). For example, the avatars only mimicked the user’s head pose, i.e., the position and orientation of the head. The combination of advanced face tracking with the realistic animation of an avatar’s facial expressions holds great potential to strengthen the sense of agency and, therewith, enfacement experiences (Gonzalez-Franco et al., 2020). A goal of our future research is to investigate the benefit of realistic avatars created with the FexMM for enfacement research using virtual mirror experiments.
The raw data supporting the conclusions of this article will be made available by the authors, without undue reservation.
The studies involving human participants were reviewed and approved by the Ethic Commission of the Deutsche Gesellschaft für Psychologie (DGPs). The participants provided their written informed consent to participate in this study. Written informed consent was obtained from the individual(s) for the publication of any potentially identifiable images or data included in this article.
All authors conceptualized the research endeavor. CMG and TL wrote the manuscript. CMG, CK, and SZ conducted the statistical shape analysis, developed the software, and created the stimuli. TL, AH, CMG, and SZ designed the user study. TL and AH collected the data and performed the statistical analyses. AH and SZ edited the manuscript. All authors contributed to the article in a considerable amount to deserve coauthorship and approved the published version.
This research was funded by the Deutsche Forschungsgemeinschaft (DFG, German Reserach Foundation) within the SPP 2134, projects HI 1780/5-1 and ZA 592/5-1.
The authors declare that the research was conducted in the absence of any commercial or financial relationships that could be construed as a potential conflict of interest.
The authors wish to thank Anna Engels for her valuable support in the preparation of the animated avatars and the setup of the online study. We also kindly thank Gabriel le Roux for his contribution in the creation of our Morphable Face Models.
1Singular Inversion, Canada, https://facegen.com/
2http://www.makehumancommunity.org/
3The Blender Foundation, Netherlands, https://www.blender.org/
4SoSci Survey GmbH, Germany, https://www.soscisurvey.de/
Achenbach, J., Waltemate, T., Latoschik, M. E., and Botsch, M. (2017). “Fast generation of realistic virtual humans,” in Proceedings of the 23rd ACM symposium on virtual reality software and technology, November 2017, Gothenburg Sweden. 1–10. doi:10.1145/3139131.3139154
Bailenson, J. N., and Yee, N. (2005). Digital chameleons: automatic assimilation of nonverbal gestures in immersive virtual environments. Psychol. Sci. 16, 814–819. doi:10.1111/j.1467-9280.2005.01619.x
Baltrusaitis, T., Zadeh, A., Lim, Y. C., and Morency, L.-P. (2018). “Openface 2.0: facial behavior analysis toolkit,” in Automatic face & gesture recognition (FG 2018), 2018 13th IEEE international conference on (IEEE), Xi'an, China, May 15–19, 2018 (IEEE), 59–66.
Bates, D., Sarkar, D., Bates, M. D., and Matrix, L. (2007). The lme4 package. R Package Version 2, 74.
Bauer, D. J., and Curran, P. J. (2005). Probing interactions in fixed and multilevel regression: inferential and graphical techniques. Multivar. Behav. Res. 40, 373–400. doi:10.1207/s15327906mbr4003_5
Bente, G., and Krämer, N. C. (2011). “Virtual gestures: embodiment and nonverbal behavior in computer-mediated communication,”in Studies in emotion and social interaction (Cambridge: Cambridge University Press) 176–210. doi:10.1017/CBO9780511977589.010
Blanz, V., and Vetter, T. (1999). “A morphable model for the synthesis of 3D faces,”in Proceedings of the 26th annual conference on computer graphics and interactive techniques (ACM), 187–194. doi:10.1145/311535.311556
Blascovich, J., Loomis, J., Beall, A. C., Swinth, K. R., Hoyt, C. L., and Bailenson, J. N. (2002). Immersive virtual environment technology as a methodological tool for social psychology. Psychol. Inq. 13, 103–124. doi:10.1207/s15327965pli1302_01
Bliese, P. D., and Ployhart, R. E. (2002). Growth modeling using random coefficient models: model building, testing, and illustrations. Organ. Res. Methods 5, 362–387. doi:10.1177/109442802237116
Breazeal, C. (2003). Emotion and sociable humanoid robots. Int. J. Human-Computer Stud. 59, 119–155. doi:10.1016/s1071-5819(03)00018-1
Brunner, E., Konietschke, F., Pauly, M., and Puri, M. L. (2017). Rank-based procedures in factorial designs: hypotheses about non-parametric treatment effects. J. R. Stat. Soc. B 79, 1463–1485. doi:10.1111/rssb.12222
Busselle, R., and Bilandzic, H. (2012). “Cultivation and the perceived realism of stories,”in Living with television now: advances in cultivation theory and research, NY: Peter Lang. 168–186.
Cao, C., Weng, Y., Zhou, S., Tong, Y., and Zhou, K. (2013). Facewarehouse: a 3D facial expression database for visual computing. IEEE Trans. Vis. Comput. Graphics 20, 413–425. doi:10.1109/TVCG.2013.249
de Borst, A. W., and de Gelder, B. (2015). Is it the real deal? perception of virtual characters versus humans: an affective cognitive neuroscience perspective. Front. Psychol. 6, 576. doi:10.3389/fpsyg.2015.00576
Dobs, K., Bülthoff, I., and Schultz, J. (2018). Use and usefulness of dynamic face stimuli for face perception studies—a review of behavioral findings and methodology. Front. Psychol. 9, 1355. doi:10.3389/fpsyg.2018.01355
Egger, B., Smith, W. A., Tewari, A., Wuhrer, S., Zollhoefer, M., Beeler, T., et al. (2019). 3D morphable face models–past, present and future. arXiv preprint arXiv:1909.01815.
Ekman, P., Friesen, W. V., and Hager, J. C. (2002). Facs investigator’s guide. CD-ROM [Dataset]. Salt Lake City: Research Nexus, a subsidiary of Network Information Research Corporation
Estudillo, A. J., and Bindemann, M. (2016). Can gaze-contingent mirror-feedback from unfamiliar faces alter self-recognition? Q. J. Exp. Psychol. (Hove) 70, 944–958. doi:10.1080/17470218.2016.1166253
Ferri, F., Chiarelli, A. M., Merla, A., Gallese, V., and Costantini, M. (2013). The body beyond the body: expectation of a sensory event is enough to induce ownership over a fake hand. Proc. R. Soc. B. 280, 20131140. doi:10.1098/rspb.2013.1140
Garau, M., Slater, M., Vinayagamoorthy, V., Brogni, A., Steed, A., and Sasse, M. A. (2003). “The impact of avatar realism and eye gaze control on perceived quality of communication in a shared immersive virtual environment,” in Proceedings of the SIGCHI conference on human factors in computing systems, ACM, Ft. Lauderdale Florida US. 529–536. doi:10.1145/642611.642703
Gerig, T., Morel-Forster, A., Blumer, C., Egger, B., Luthi, M., Schönborn, S., et al. (2018). “Morphable face models-an open framework (IEEE),” in 2018 13th IEEE international conference on automatic face & gesture recognition (FG 2018), Xi’an, China, May 15–19, 2018 (IEEE), 75–82.
Gilbert, M., Demarchi, S., and Urdapilleta, I. (2018). “Facshuman a software to create experimental material by modeling 3D facial expression,” in Proceedings of the 18th international conference on intelligent virtual agents, November 2018, Sydney Australia. 333–334.
Gonzalez-Franco, M., Steed, A., Hoogendyk, S., and Ofek, E. (2020). Using facial animation to increase the enfacement illusion and avatar self-identification. IEEE Trans. Vis. Comput. Graphics 26, 2023–2029. doi:10.1109/tvcg.2020.2973075
Grewe, C. M., Le Roux, G., Pilz, S.-K., and Zachow, S. (2018). “Spotting the details: the various facets of facial expressions,” in 2018 13th IEEE international conference on automatic face & gesture recognition (FG 2018), Xi’an, China, May 15–19, 2018 (IEEE), 286–293.
Grewe, C. M., and Zachow, S. (2016). “Fully automated and highly accurate dense correspondence for facial surfaces,” in European Conference on Computer Vision, Amsterdam, Netherlands: Springer, 552–568. doi:10.1007/978-3-319-48881-3_38
Guadagno, R. E., Blascovich, J., Bailenson, J. N., and Mccall, C. (2007). Virtual humans and persuasion: the effects of agency and behavioral realism. Media Psychol. 5, 1–22. doi:10.1080/15213260701300865
Hays, J., Wong, C., and Soto, F. (2020). Faret: a free and open-source toolkit of three-dimensional models and software to study face perception. Behav. Res. Methods 52, 2604–2622. doi:10.3758/s13428-020-01421-4
Herrera, F., Oh, S. Y., and Bailenson, J. N. (2020). Effect of behavioral realism on social interactions inside collaborative virtual environments. PRESENCE: Teleoperat. Virtual Environ. 27, 163–182. doi:10.1162/pres_a_00324
Huber, P., Hu, G., Tena, R., Mortazavian, P., Koppen, P., Christmas, W. J., et al. (2016). “A multiresolution 3D morphable face model and fitting framework,” in Proceedings of the 11th international joint conference on computer vision, imaging and computer graphics theory and applications, February 2016, Rome Italy. doi:10.5220/0005669500790086
Ichim, A. E., Bouaziz, S., and Pauly, M. (2015). Dynamic 3D avatar creation from hand-held video input. ACM Trans. Graphics 34, 1–14. doi:10.1145/2766974
Jeong, E. J., Biocca, F. A., and Bohil, C. J. (2012). Sensory realism and mediated aggression in video games. Comput. Hum. Behav. 28, 1840–1848. doi:10.1016/j.chb.2012.05.002
Kaiser, H. F. (1958). The varimax criterion for analytic rotation in factor analysis. Psychometrika 23, 187–200. doi:10.1007/bf02289233
Kätsyri, J., de Gelder, B., and de Borst, A. W. (2015). Amygdala responds to direct gaze in real but not in computer-generated faces. NeuroImage 204, 1–(12.)
Kenward, M. G., and Roger, J. H. (1997). Small sample inference for fixed effects from restricted maximum likelihood. Biometrics 53. 983–997. doi:10.2307/2533558
Kilteni, K., Maselli, A., Kording, K. P., and Slater, M. (2015). Over my fake body: body ownership illusions for studying the multisensory basis of own-body perception. Front. Hum. Neurosci. 9, 1–20. doi:10.3389/fnhum.2015.00141
Klingenberg, C. P., Barluenga, M., and Meyer, A. (2002). Shape analysis of symmetric structures: quantifying variation among individuals and asymmetry. Evolution 56, 1909–1920. doi:10.1111/j.0014-3820.2002.tb00117.x
Kruzic, C. O., Kruzic, D., Herrera, F., and Bailenson, J. (2020). Facial expressions contribute more than body movements to conversational outcomes in avatar-mediated virtual environments. Sci. Rep. 10, 1–23. doi:10.1038/s41598-020-76672-4
Latoschik, M. E., Roth, D., Gall, D., Achenbach, J., Waltemate, T., and Botsch, M. (2017). “The effect of avatar realism in immersive social virtual realities,” in Proceedings of the 23rd ACM symposium on virtual reality software and technology, November 2017, Gothenburg Sweden. 1–10.
Lewis, J. P., Anjyo, K., Rhee, T., Zhang, M., Pighin, F. H., and Deng, Z. (2014). Practice and theory of blendshape facial models” in Eurographics (State of the art reports) , Geneve Switzerland: The Eurographics Association Vol. 1, 2.
Li, H., Weise, T., and Pauly, M. (2010). Example-based facial rigging. ACM Trans. Graphics 29, 1–6. doi:10.1145/1778765.1778769
Li, T., Bolkart, T., , Black, M. J., , Li, H., , and Romero, J., (2017). Learning a model of facial shape and expression from 4D scans. Trans. Graphics 36, 1–17. doi:10.1145/3130800.3130813
Liepelt, R., Dolk, T., and Hommel, B. (2017). Self-perception beyond the body: the role of past agency. Psychol. Res. 81, 549–559. doi:10.1007/s00426-016-0766-1
Lombardi, S., Saragih, J., Simon, T., and Sheikh, Y. (2018). Deep appearance models for face rendering. Trans. Graphics 37, 68. doi:10.1145/3197517.3201401
Ma, K., Lippelt, D. P., and Hommel, B. (2017). Creating virtual-hand and virtual-face illusions to investigate self-representation. J. Vis. Exp. 121, e54784. doi:10.3791/54784
Ma, K., Sellaro, R., and Hommel, B. (2018). Personality assimilation across species: enfacing an ape reduces own intelligence and increases emotion attribution to apes. Psychol. Res. 83, 373–(383.) doi:10.1007/s00426-018-1048-x
Mäkäräinen, M., Kätsyri, J., and Takala, T. (2014). Exaggerating facial expressions: a way to intensify emotion or a way to the uncanny valley? Cogn. Comput. 6, 708–721. doi:10.1007/s12559-014-9273-0
Manaf, A. A. A., Ismail, S. N. F., and Arshad, M. R. (2019). Perceived visual cgi familiarity towards uncanny valley theory in film. Int. J. Appl. Creat. Arts 2, 7–16. doi:10.33736/ijaca.1575.2019
Mullen, K. M., and van Stokkum, I. H. M. (2012). nnls: the Lawson-Hanson algorithm for non-negative least squares (NNLS). R Package Version 1.4.
Neumann, T., Varanasi, K., Wenger, S., Wacker, M., Magnor, M., and Theobalt, C. (2013). Sparse localized deformation components. ACM Trans. Graphics 32, 1–10. doi:10.1145/2508363.2508417
Noguchi, K., Gel, Y. R., Brunner, E., and Konietschke, F. (2012). nparld: an r software package for the nonparametric analysis of longitudinal data in factorial experiments. J. Stat. Softw. 50, 14539. doi:10.18637/jss.v050.i12
Nowak, K. L., and Fox, J. (2018). Avatars and computer-mediated communication: a review of the definitions, uses, and effects of digital representations. Rev. Commun. Res. 6, 30–53. doi:10.12840/issn.2255-4165.2018.06.01.015
Oh, C. S., Bailenson, J. N., and Welch, G. F. (2018). A systematic review of social presence: definition, antecedents, and implications. Front. Robot. AI 10, 1–35. doi:10.3389/frobt.2018.00114
Porciello, G., Bufalari, I., Minio-Paluello, I., Di Pace, E., and Aglioti, S. M. (2018). The ‘Enfacement’ illusion: a window on the plasticity of the self. Cortex 104, 261–275. doi:10.1016/j.cortex.2018.01.007
Serino, A., Sforza, A. L., Kanayama, N., van Elk, M., Kaliuzhna, M., Herbelin, B., et al. (2015). Tuning of temporo-occipital activity by frontal oscillations during virtual mirror exposure causes erroneous self-recognition. Eur. J. Neurosci. 42, 2515–2526. doi:10.1111/ejn.13029
Soto, F. A., and Ashby, F. G. (2019). Novel representations that support rule-based categorization are acquired on-the-fly during category learning. Psychol. Res. 83, 544–566. doi:10.1007/s00426-019-01157-7
Sungur, E. A. (2005). A note on directional dependence in regression setting. Commun. Stat. - Theor. Methods 34, 1957–1965. doi:10.1080/03610920500201228
Tena, J. R., De la Torre, F., and Matthews, I. (2011). “Interactive region-based linear 3D face models,” in Transaction on Graphics. 1–10. doi:10.1145/2010324.1964971
Thies, J., Zollhofer, M., Stamminger, M., Theobalt, C., and Nießner, M. (2016). “Face2face: real-time face capture and reenactment of rgb videos,” in International conference on computer vision and pattern recognition, June 2016, Las Vegas. 2387–2395.
Thies, J., Zollhöfer, M., Stamminger, M., Theobalt, C., and Nießner, M. (2018). Facevr: real-time gaze-aware facial reenactment in virtual reality. Trans. Graphics 37, 1–15. doi:10.1145/3182644
Todorov, A., Dotsch, R., Porter, J. M., Oosterhof, N. N., and Falvello, V. B. (2013). Validation of data-driven computational models of social perception of faces. Emotion 13, 724. doi:10.1037/a0032335
Valstar, M., and Pantic, M. (2010). “Induced disgust, happiness and surprise: an addition to the mmi facial expression database,” Proceeding 3rd intern. workshop on EMOTION (satellite of LREC): corpora for research on emotion and affect. Vol. 65. (Paris, France).
Volante, M., Babu, S. V., Chaturvedi, H., Newsome, N., Ebrahimi, E., Roy, T., et al. (2016). Effects of virtual human appearance fidelity on emotion contagion in affective inter-personal simulations. IEEE Trans. Vis. Comput. Graphics 22, 1326–1335. doi:10.1109/TVCG.2016.2518158
Waltemate, T., Gall, D., Roth, D., Botsch, M., and Latoschik, M. E. (2018). The impact of avatar personalization and immersion on virtual body ownership, presence, and emotional response. IEEE Trans. Vis. Comput. Graphics 24, 1643–1652. doi:10.1109/tvcg.2018.2794629
Weiss, S., Grewe, C. M., Olderbak, S., Goecke, B., Kaltwasser, L., and Hildebrandt, A. (2020). Symmetric or not? a holistic approach to the measurement of fluctuating asymmetry from facial photographs. Pers. Individ. Differ. 166, 110137. doi:10.1016/j.paid.2020.110137
Wood, E., Baltrušaitis, T., Morency, L.-P., Robinson, P., and Bulling, A. (2016). “A 3D morphable eye region model for gaze estimation,” in European conference on computer vision, Springer, 297–313. doi:10.1007/978-3-319-46448-0_18
Yin, L., Wei, X., Sun, Y., Wang, J., and Rosato, M. J. (2006). ” A 3D facial expression database for facial behavior research,” in 7th international conference on automatic face and gesture recognition (FGR06), Southampton, UK, April 10–12, 2006 (IEEE), 211–216.
Zhang, J., Ma, K., and Hommel, B. (2015). The virtual hand illusion is moderated by context-induced spatial reference frames. Front. Psychol. 6, 1659. doi:10.3389/fpsyg.2015.01659
Keywords: behavioral realism, animated avatar faces, avatar creation, statistical face models, photographic realism
Citation: Grewe CM, Liu T, Kahl C, Hildebrandt A and Zachow S (2021) Statistical Learning of Facial Expressions Improves Realism of Animated Avatar Faces. Front. Virtual Real. 2:619811. doi: 10.3389/frvir.2021.619811
Received: 21 October 2020; Accepted: 26 January 2021;
Published: 12 April 2021.
Edited by:
Mar Gonzalez-Franco, Microsoft Research, United StatesReviewed by:
Katja Zibrek, Inria Rennes - Bretagne Atlantique Research Center, FranceCopyright © 2021 Grewe, Liu, Kahl, Hildebrandt and Zachow. This is an open-access article distributed under the terms of the Creative Commons Attribution License (CC BY). The use, distribution or reproduction in other forums is permitted, provided the original author(s) and the copyright owner(s) are credited and that the original publication in this journal is cited, in accordance with accepted academic practice. No use, distribution or reproduction is permitted which does not comply with these terms.
*Correspondence: C. Martin Grewe, Z3Jld2VAemliLmRl
Disclaimer: All claims expressed in this article are solely those of the authors and do not necessarily represent those of their affiliated organizations, or those of the publisher, the editors and the reviewers. Any product that may be evaluated in this article or claim that may be made by its manufacturer is not guaranteed or endorsed by the publisher.
Research integrity at Frontiers
Learn more about the work of our research integrity team to safeguard the quality of each article we publish.