- 1Department of Mechanical Engineering, University of Maryland College Park, College Park, MD, United States
- 2Center for Devices and Radiological Health, Office of Science and Engineering Laboratories, U. S. Food and Drug Administration Center for Devices and Radiological Health, Silver Spring, MD, United States
- 3ORISE Research Fellow, Oak Ridge Institute for Science and Education, Oak Ridge, TN, United States
Virtual reality is being used to aid in prototyping of advanced limb prostheses with anthropomorphic behavior and user training. A virtual version of a prosthesis and testing environment can be programmed to mimic the appearance and interactions of its real-world counterpart, but little is understood about how task selection and object design impact user performance in virtual reality and how it translates to real-world performance. To bridge this knowledge gap, we performed a study in which able-bodied individuals manipulated a virtual prosthesis and later a real-world version to complete eight activities of daily living. We examined subjects' ability to complete the activities, how long it took to complete the tasks, and number of attempts to complete each task in the two environments. A notable result is that subjects were unable to complete tasks in virtual reality that involved manipulating small objects and objects flush with the table, but were able to complete those tasks in the real world. The results of this study suggest that standardization of virtual task environment design may lead to more accurate simulation of real-world performance.
Introduction
IT was estimated in 2005 that there were two million amputees in the United States, and this number was expected to double by 2050 (Ziegler-Graham et al., 2008; McGimpsey and Bradford, 2017). The prosthesis rejection rate for upper limb (UL) amputees has been reported to be as high as 40% (Biddiss E. A. and Chau T. T., 2007). Among the reasons for prosthesis rejection is difficultly when attempting to use the prosthesis to complete activities of daily living (ADLs), such as grooming and dressing (Biddiss E. and Chau T., 2007). The prosthesis control scheme plays an important role in object manipulation, preventing objects from slipping out of or being crushed in a prosthetic hand. Improving the response time of the device, the control scheme (i.e., body-powered vs. myoelectric control), and how the device signal is recorded (external vs. implanted electrodes) will help with ensuring that amputees can complete ADLs with less difficulty (Harada et al., 2010; Belter et al., 2013). Programs such as the Defense Advanced Research Projects Agency (DARPA) Hand Proprioceptive and Touch Interfaces (HAPTIX) program have been investigating how to improve UL prosthesis designs (Miranda et al., 2015).
Building advanced prostheses is expensive and time consuming (Hoshigawa et al., 2015; Zuniga et al., 2015), requiring customization for each individual and integration of advanced sensors and robotics (Biddiss et al., 2007; van der Riet et al., 2013; Hofmann et al., 2016). To efficiently study advanced UL prostheses in a well-controlled environment prior to physical prototyping, a virtual version can be used (Armiger et al., 2011). The virtual version can be programmed and calibrated in a manner similar to a physical prosthesis and can be used to allow amputees to practice device control schemes with simulated objects (Pons et al., 2005; Lambrecht et al., 2011; Resnik et al., 2011; Kluger et al., 2019).
Virtual reality (VR) has also been used to aid in clinical prosthesis training and rehabilitation. A prosthetist can load a virtual version of an amputee's prosthesis to allow him/her to practice using the control scheme of the prosthesis (e.g., muscle contractions for a myoelectric device or foot movements for inertial measurement units) (Lambrecht et al., 2011; Resnik et al., 2012; Blana et al., 2016). A variety of VR platforms exist for this purpose, but there is a gap in the literature about what tasks and object characteristics need to be replicated in VR to predict real world (RW) performance. A better understanding of how to design and translate results from VR to RW is needed to inform clinical practice. This paper presents a study comparing performance of virtual ADLs with a virtual prosthesis with RW ADL using a physical prosthesis. We examined what factors affect performance in VR to determine if these factors translate to RW performance. This work will inform the design of VR ADLs for training and transfer to RW performance.
Background
Clinical Outcome Assessments
Clinical outcome assessments (COAs) are used to evaluate an individual's progress through training or rehabilitation with their prosthetic device. Research has shown that motor control learning is highly activity specific (Latash, 1996; Giboin et al., 2015; van Dijk et al., 2016); therefore, selecting training activities is important to help a new prosthesis user return to a normal routine. However, few COAs have been developed to assess upper limb prosthesis rehabilitation progress; therefore, activities for assessing function with other medical conditions, such as stroke or traumatic brain injury (TBI), are used (Wang et al., 2018). One such test is the Box and Blocks Test (BBT) (Mathiowetz et al., 1985; Lin et al., 2010), in which subjects complete a simple activity that is not truly reflective of an activity that a prosthesis user would perform in daily life. The goal of the BBT is to move as many blocks as possible from one side of a box over a partition to the other side in 60 s. Researchers have made modifications to the BBT to assess an individual's ability to perform basic movements with their prosthesis (Hebert and Lewicke, 2012; Hebert et al., 2014; Kontson et al., 2017).
Another clinical outcome assessment that has been used to assess UL prosthetic devices is the Jebsen–Taylor Hand Function Test (JTHFT). The JTHFT is a series of standardized activities designed to assess an individual's ability to complete ADLs following a stroke, TBI, or hand surgery (Sears and Chung, 2010). The seven activities in the JTHFT are simulated feeding, simulated page turning, stacking checkers, writing, picking up large objects, picking up large heavy objects, and picking up small objects. Individuals are timed as they complete each activity, and their results are compared with normative data (Sears and Chung, 2010). Studies have been performed with the UL amputee population to validate the use of the JTHFT as a tool to assess prosthetic device performance (Wang et al., 2018). This assessment's use of simulated ADLs makes it a better candidate than the BBT for assessing how a person would use a prosthesis in daily life.
Research has also been performed to develop COAs specifically to assess upper limb prosthesis rehabilitation progress. The Activities Measure for Upper Limb Amputees (AM-ULA) (Resnik et al., 2013) and Capacity Assessment of Prosthetic Performance for the Upper Limb (CAPPFUL) (Kearns et al., 2018) were designed to test an amputee's ability to complete ADLs with their device. These two COAs consist of 18 and 11 ADLs, respectively, and assess a person's ability to complete the activity, time to completion, and movement quality.
While these activities can be completed with a physical prosthetic device, training in a virtual environment has shown to be an effective way to train amputees to use their device (Phelan et al., 2015; Nakamura et al., 2017; Perry et al., 2018; Nissler et al., 2019). Training in a virtual environment can be a cost effective way for clinics to perform rehabilitation (Phelan et al., 2015; Nakamura et al., 2017) and help prosthesis users learn how to manipulate their device using its particular control scheme (Blana et al., 2016; Woodward and Hargrove, 2018), and gamifying rehabilitation has been shown to increase a prosthesis user's desire to complete the program (Prahm et al., 2017, 2018).
Virtual Reality Prosthesis Testing and Training Environments
Several VR testbeds have been created or adapted to evaluate different aspects of prosthesis development. The Musculoskeletal Modeling Software (MSMS) was originally developed to aid with musculoskeletal modeling (Davoodi et al., 2004), but was later adapted for training, development, and modeling of neural prosthesis control (Davoodi and Loeb, 2011). The Hybrid Augmented Reality Multimodal Operation Neural Integration Environment (HARMONIE) was developed to support the study of human assistive robotics and prosthesis operations (Katyal et al., 2013). Users that interact with the HARMONIE system control their device through surface electromyography (sEMG), neural interfaces (EEG), or other control signals (Katyal et al., 2013, 2014; McMullen et al., 2014; Ivorra et al., 2018). Another tool, Multi-Joint dynamics with Contact (MuJoCo), is a physics engine that was originally designed to facilitate research and development in robotics, biomechanics, graphics, and animation (Todorov et al., 2012). MuJoCo HAPTIX was created to model contacts and provide sensory feedback to the user through the VR environment (Kumar and Todorov, 2015). Studies are being performed to improve the contact forces applied to objects in MuJoCo HAPTIX (Kim and Park, 2016; Lim et al., 2019; Odette and Fu, 2019). These testbeds aid in training and studying of prosthesis control in VR, but little is known about how VR object characteristics impact performance.
User Performance Assessment
Simulations should require visual and cognitive resources similar to those needed to complete the activity in the real world (Stone, 2001; Gamberini, 2004; Stickel et al., 2010). While previous studies evaluated VR testbeds or activities implemented in them (Carruthers, 2008; Cornwell et al., 2012; Blana et al., 2016), none have identified the characteristics of the tasks that make an activity easy or difficult to complete in VR. Subjects in these studies did not complete ADLs from COAs that have been validated with a UL population, which could limit the ability to replicate and retest these tasks for RW study.
Study Objectives
The purpose of this study is to provide preliminary validation for a VR system to test advanced prostheses through comparison with similar RW activity outcomes. In addition, this study aims to gain a better understanding of how activity design affects an individual's ability to complete virtual activities with a virtual prosthetic hand. The activities used in this study are derived from existing, validated UL prosthesis outcome measures that are used to evaluate prosthesis control. Motion capture hardware and software were used to collect normative data from able-bodied individuals to determine how activity selection and virtual design affects the completion rate, completion time, and number of attempts to complete the activity. By replicating validated outcome measures in VR, the results from the VR performance was then compared with RW task performance to assess how VR performance translates to RW performance.
Methods
Task Development
MuJoCo HAPTIX (Roboti, Seattle, Washington) is a VR simulator that has been adapted to the needs of the DARPA HAPTIX program by adding an interactive graphical user interface (GUI) and integrating real-time motion capture to control a virtual hand's placement in space (Kumar and Todorov, 2015) (Figure 1). MuJoCo is open source and can be used to test other limb models as well. Four tasks were designed in the MuJoCo HAPTIX environment to study movement quality: (1) hand pose matching, (2) stimulation identification and use of proprioceptive feedback and (3) sensory feedback to identify characteristics of an object, and (4) object manipulation. This research focuses on the MuJoCo object manipulation task, which is based on existing COAs, the JTHFT and the AM-ULA.
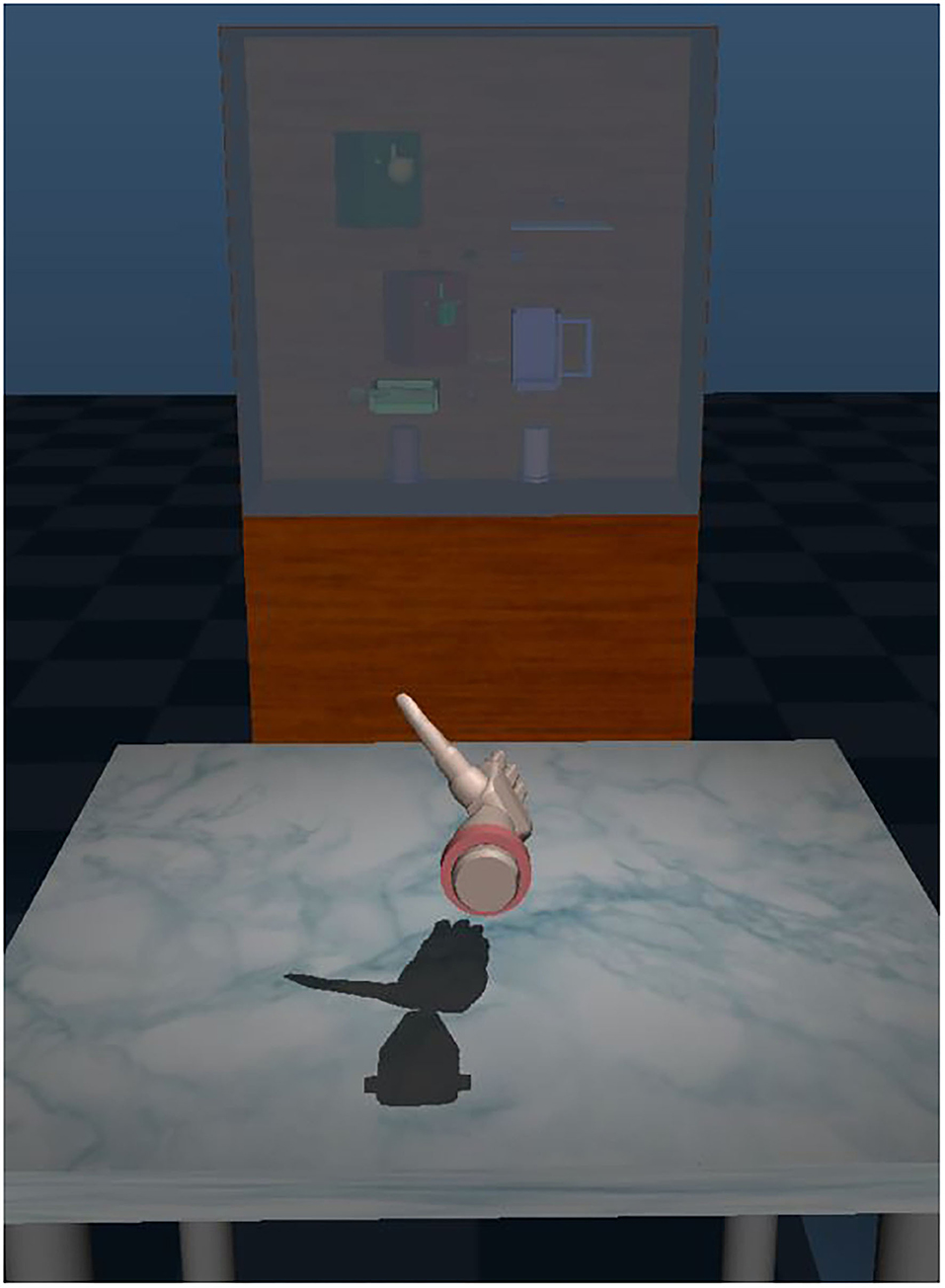
Figure 1. The virtual environment, Multi-Joint dynamics with Contact (MuJoCo) Hand Proprioceptive, and Touch Interfaces (HAPTIX).
Task Selection and Analysis
Eight ADLs from the AM-ULA (Resnik et al., 2013) and JHFT (Sears and Chung, 2010) were completed in VR and in RW (Figure 2 and Table 1). The tasks selected for replication from the JHFT and AM-ULA were chosen for their capacity to assess both prosthesis dexterity and representative ADLs such as food preparation and common object interaction. The moving cylinders (Move Cyl.) task is representative of activities that require subjects to move a relatively large object. The place sphere in cup (Sphere cup), lock/key (Lock Key), and stack checkers (Checkers) tasks are representative of activities that require precise manual manipulation to move a small object. The spoon transfer (Spoon Tran.) and writing tasks required rotation and precise targeting. Research has shown that tasks requiring small objects to be manipulated require more dexterous movement, while tasks where large objects are manipulated require more power and less dexterity (Park and Cheong, 2010; Zheng et al., 2011).
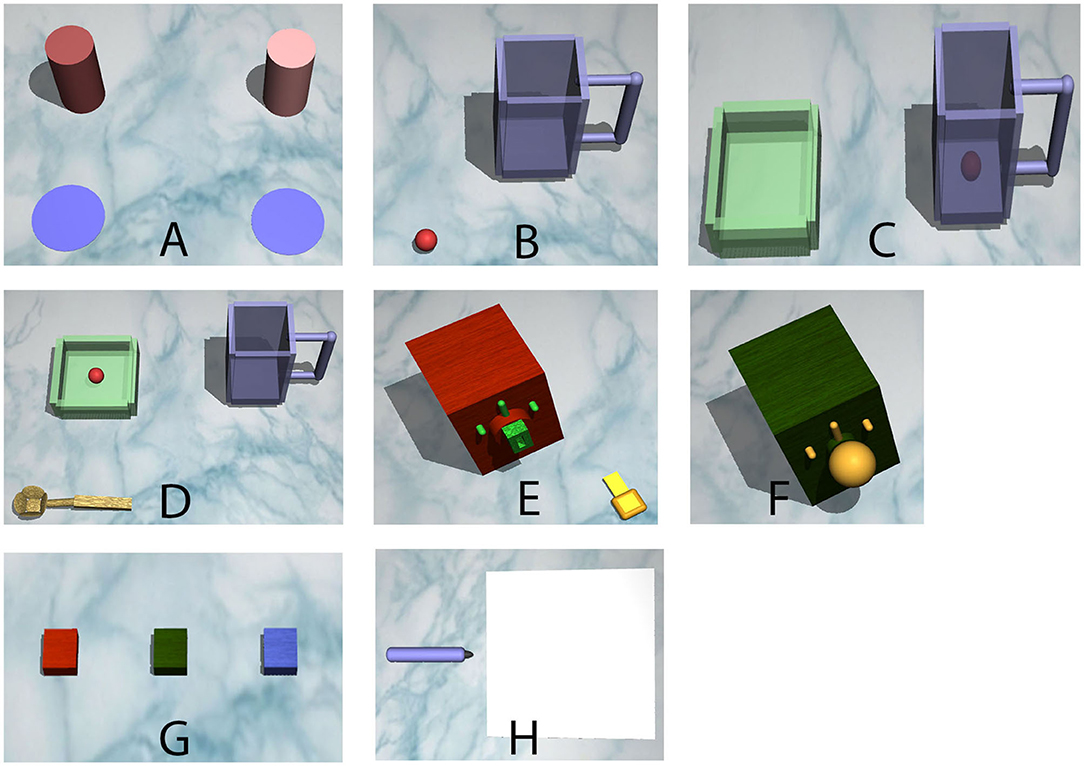
Figure 2. The tasks that subjects completed. In order: (A) Task 1: move cans to targets, (B) Task 2: put ball in pitcher, (C) Task 3: pour ball in bowl, (D) Task 4: transfer ball with spoon, (E) Task 5: insert key and turn, (F) Task 6: turn knob, (G) Task 7: stack squares, and (H) Task 8: simulated writing.
A hierarchical task analysis (HTA) was performed on each of the ADLs to understand what steps or subtasks need to be completed in order to complete the ADL high-level goals. An HTA is a process used by human factor engineers to decompose a task into subtasks necessary for completion, which can help to identify use difficulty or use failure for product users (Patrick et al., 2000; Salvendy, 2012; Hignett et al., 2019). The HTA used for this research focused on the observable physical actions that a person must complete. To ensure that the number of steps presented in the HTA provided sufficient depth for understanding necessary components of the tasks, the instructions for the AM-ULA and the JHFT were referenced to inform the ADL subtask decomposition.
The descriptions of the subtasks utilized seven action verbs: reach, grasp, pick up, place, release, move, and rotate (Supplementary Table 1). These action verbs were picked due to their use in describing the steps to complete tasks in the AM-ULA (Resnik et al., 2013). Reach consists of moving the hand toward an object by extension of the elbow and protraction of the shoulder. Grasp involves flexion of the fingers of the hand around an object. Pick up includes flexion of the shoulder and potentially the elbow to lift the object from the table. Move consists of medial or lateral rotation of the arm to align the primary object toward a secondary object or shifting the hand away from one object and aligning it with another. Place involves extension of the elbow to lower the object onto its target. Release involves extension of the fingers to let go of the object. Rotation consists of pronation or supination of the arm to rotate an object.
Subjects
Able-bodied individuals were recruited for this study due to limited availability of upper limb amputees. Prior studies have used able-bodied individuals, with the use of a bypass or simulator prosthesis, to assess the ability to complete COAs and ADLs with different prosthesis control schemes (Haverkate et al., 2016; Bloomer et al., 2018). These studies showed that the use of able-bodied subjects allows the experimenter to control for levels of experience with a prosthetic device and that performance between the able-bodied group and amputee group is comparable.
Twenty-two individuals (10 females, average age of all subjects 35 ± 17 years) completed the VR experiments, and 22 individuals (eight females, average age of all subjects 38 ± 16 years) completed the RW experiments. The VR experiment was completed first, followed by the RW experiment to provide a comparative evaluation of virtual task performance and its utility for this application. Only two subjects overlapped between the two groups due to the amount of time between completing the VR experiment and being given access to the physical prosthesis. Because participants learned techniques for completing tasks that could generalize across RW/VR environments, and we intended to measure naïve performance, our study design did not include completion of the tasks in both environments. All subjects were right-handed. No subjects reported upper limb disabilities. Subject participation was approved by the FDA IRB (RIHSC #14-086R).
Materials
Virtual Reality Equipment
The VR software used was MuJoCo HAPTIX v1.4 (Roboti, Seattle, Washington), with MATLAB (Mathworks, Natick, MA) to control task presentation. Computer and motion capture (mocap) component specifications can be found on mujoco.org/book/haptix.html. Subjects manipulated the position of the virtual hand with Motive software (OptiTrack, Corvallis, OR), mocap markers, and an OptiTrack V120: Trio camera (OptiTrack, Corvallis, OR) while using a right-handed CyberGlove III (CyberGlove Systems LLC, San Jose, CA) to control the fingers.
Real-World Equipment
The RW experiments were performed with the DEKA LUKE arm (Mobius Bionics, Manchester, NH) attached to a bypass harness. The bypass harness allowed able-bodied subjects to wear the prosthetic device. Inertial measurement units (IMUs), worn on the subject's feet, controlled the manipulation of the wrist and grasping (Resnik and Borgia, 2014; Resnik et al., 2014a,b; Resnik et al., 2018a,b; George et al., 2020). The objects used in the RW experiment were modeled after the ones manipulated in VR (Supplementary Figure 1).
Experimental Setup and Procedure
Virtual Reality Experiment
Mocap setup was performed before starting each experiment. Reflective markers were placed on the monitor, and subjects were assisted with donning the CyberGlove III and a mocap wrist component (Supplementary Figure 2). Subjects could only use their right hand to manipulate the virtual prosthesis. The height and spacing of the OptiTrack camera were adjusted to ensure that the subject could reach all of the virtual table (Figure 3A). A series of calibration movements was performed to align the subject's hand movements with the virtual hand on the screen. The movements required the subject to flex and extend his or her wrist and fingers maximally. Once the series of movements was completed, the subject moved his or her hand and observed how the virtual hand responded. If the subject was satisfied with the hand movement, then the experiment could begin.
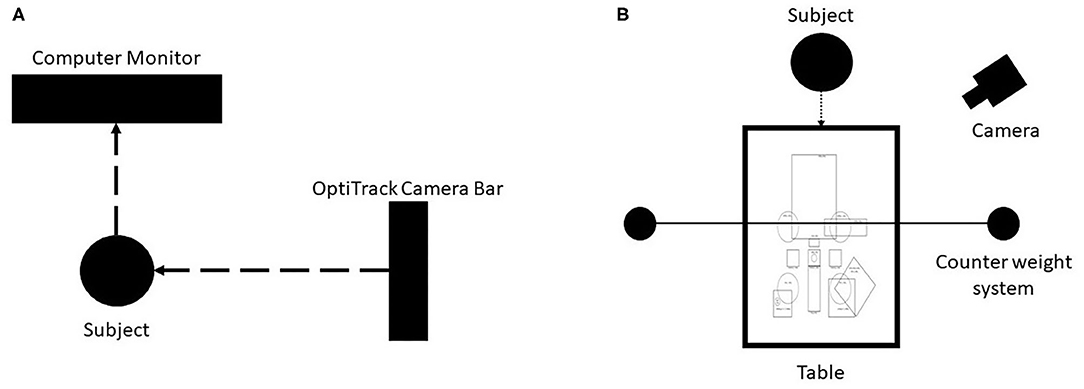
Figure 3. Virtual reality (VR) and real-world (RW) experiment setups. (A) VR setup: Subjects were seated in front of a computer monitor, and a motion capture camera was placed to their right. The height and placement of the camera was adjusted to allow subjects to interact with the virtual table. (B) RW experimental setup. The subject sat in front of the table with a camera to their left to capture their performance for later review. A template was placed on the table to match where the objects would appear in the virtual environment. A counter-weight system was used to offset the torque placed on the subject's arm by the DEKA Arm bypass attachment.
The task environment was opened in MuJoCo, and operation scripts were loaded in MATLAB. MuJoCo recorded the subject's virtual performance for analysis. MATLAB scripts controlled when the tasks started, progressed the experiment through the tasks, and created a log file for analysis. Log files contained the task number and time remaining when the subject completed or moved on to the next task.
Task objects were presented to the subjects one at a time. Instructions were printed on the upper-right hand corner for 3 s and then replaced with a 60-s countdown timer signifying the start of the task. If the subject completed the task before time ran out, then he or she could click the next button to move on. Each task is completed twice in immediate succession. If the subject was unable to complete the task before time ran out, then the program automatically moved on to the next task. Analysis was performed on task completion, number of attempts to complete the task, and time to complete tasks.
Real-World Experiment
This experiment was performed following the VR experiment. Subjects tended to struggle with various aspects of completing task in VR. The VR tasks were replicated in RW based on the virtual models provided, and a physical version of the prosthetic was used for the experiments. This real-world follow-up experiment was performed to better understand which task characteristics need to be improved in the virtual design for more realistic comparison to its real-world counterparts.
Subjects were given a brief training session on how to manipulate the prosthesis before starting the experiment. Training was done to familiarize subjects with the control schema of the device and would be insufficient to affect the task success rates (Bloomer et al., 2018). The training began with device orientation, which included safety warnings, arm componentry, and arm control (Figure 4). The IMUs were then secured to the subject's shoes, and the prosthetist software for training amputees was displayed to the subjects to allow them to practice the manipulation motions. The left foot controlled the opening and closing of a hand grasp (plantarflexion and dorsiflexion movements, respectively) as well as grasp selection (inversion and eversion movements, respectively). The right foot controlled wrist movements: flexion and extension (plantarflexion and dorsiflexion movements, respectively), as well as pronation and supination (inversion and eversion movements, respectively). The speed of the hand and wrist movement was proportional to the steepness of the foot angle; the steeper the angle, the faster the motion. A reference sheet displaying foot controls and the different grasps was placed on the table for subjects to reference throughout training and the experiment.
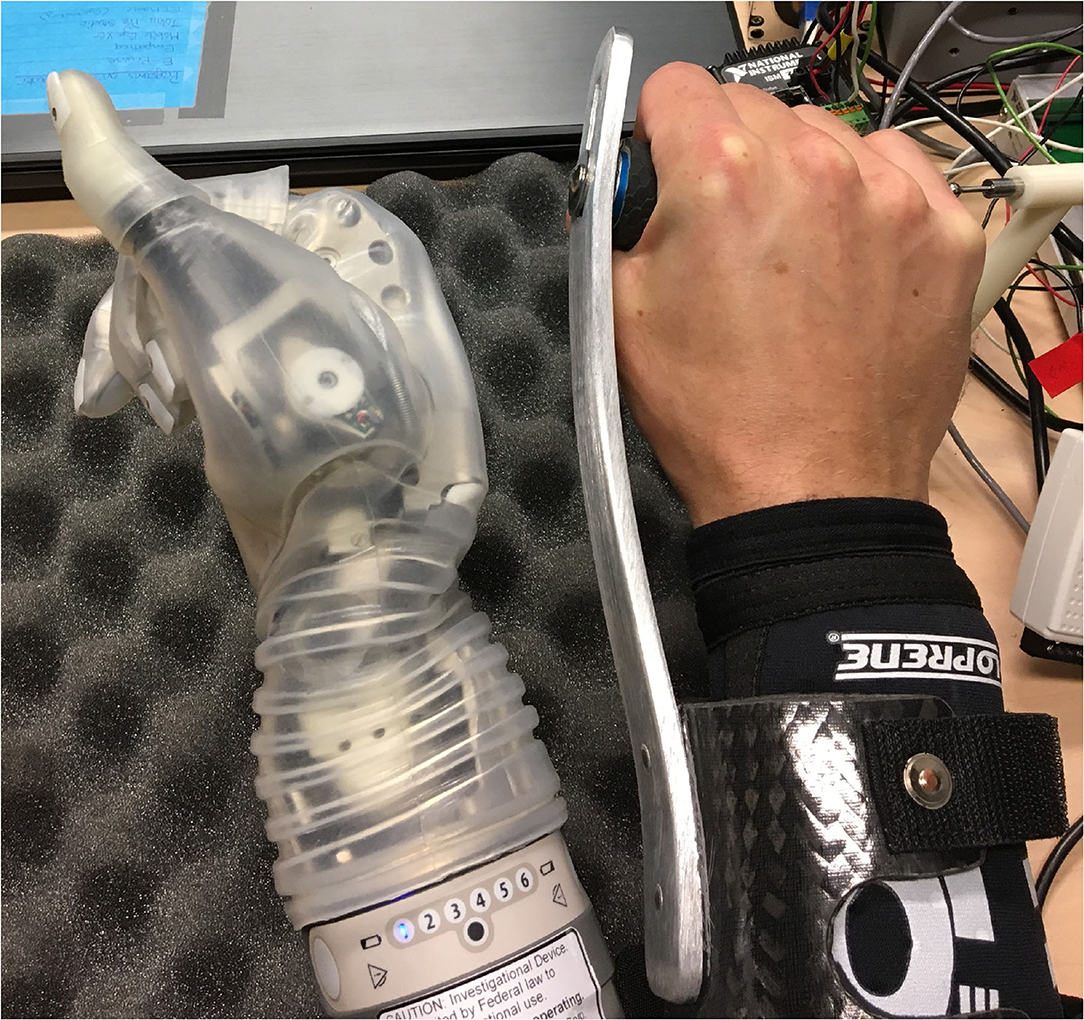
Figure 4. The DEKA Arm was attached to a bypass to allow able-bodied individuals to wear the prosthesis.
Subjects were given a total of 10 min to practice the device control scheme. The first 5 min was used to practice controlling a virtual version of the device in the prosthetist software, and the next 5 min was used to practice wearing the device and performing RW object manipulation.
Training objects were removed from the table at the end of training, and the task objects were brought out. A camera captured subjects' task completion attempts for later analysis. For each task, objects were placed on the table in the locations in which they would appear in VR (Figure 3B). Subjects could select the grasp they wanted to use and ask any questions after hearing the explanation of the task. Grasps could be changed during the attempt to complete the task, but the task timer would not be stopped. The experimenter started the camera after confirming with the subject that they were ready to begin. Task completion, attempts, time to complete, and additional observations were recorded by the experimenter as the subject attempted to complete the task (Supplementary Figure 3).
The primary differences between the VR and RW setups were the control schemes used and training. This study focused on examining what characteristics can make a task difficult to complete in VR where subjects can manipulate the virtual device with their hand. This was done to show a best-case scenario control scheme. In the VR setup, subjects used a CyberGlove to control the virtual prosthetic. This allowed subjects to use their hand in a manner that replicated normal motion to complete object manipulation tasks; therefore, no training was necessary. The RW experiment used a different control scheme because the only marketed configuration of the DEKA limb uses foot control. Since the subjects were able-bodied individuals with no UL, impairment training was provided on device operation.
Virtual Reality and Real World Data Analysis
Task completion rate, number of attempts, task completion time, and movement quality were examined to evaluate task design in VR and compare against RW results. These attributes were chosen because they could provide a comparative measure of task difficulty. A task analysis was performed to decompose the tasks into subtasks that must be completed to complete the task. Task completion is binary; if a subject partially completed a task, then it was marked as incomplete. Completion rate was calculated by summing the total number of completions and dividing it by the total number of attempts across all subjects. Subtasks were also rated on a binary scale for completion to better understand what parts of a task posed the most difficulty. This information, paired with object characteristics and interactions, provided insight into each activity and the motion requirements.
Task attempts were defined as the number of times a subject picked up or began interacting with an object and began movement toward task completion. Attempts at each of the subtasks was examined as well. Since there were numerous techniques a subject could use to complete the tasks, each subject's recording of their performance was reviewed.
Time remaining for the VR tasks was converted to completion time by subtracting the time remaining from the total time. Completion time, a continuous variable, was defined by how much time it took subjects to complete a task. Completion time for the subtasks and the tasks as a whole was compared to understand whether object characteristics and interactions affected task difficulty.
Movement quality was defined by the amount of awkwardness and compensatory movements a subject used during their attempts to complete a task (Resnik et al., 2013; van der Laan et al., 2017). Compensatory movements are atypical movements that are used to complete tasks, e.g., exaggerated trunk flexion to move an object (Resnik et al., 2013). These compensatory movements, along with adding extra steps toward subtask completion such as repeatedly putting an object back on the table to reposition it in the hand add awkwardness to how a subject moves (Levin et al., 2015). The amount of awkwardness and compensatory movements are expected to negatively impact movement quality. A scale, based on the one developed in the AM-ULA, was used to quantify movement quality for each subtask. In the AM-ULA, a five-point Likert scale is used where 0 points are given if a subject is unable to complete a task and four points are given if the subject completes the task with no awkwardness. The lowest score received for a subtask in the AM-ULA is the score given for the entire task. Reducing a task score down to one value was not performed in this experiment to provide granularity and insight into which subtasks caused the most difficulty for subjects. A modified version of this scale was used to assess the subtasks of each task. This modified scale rated movement quality on a four-point numerical scale; 1, meaning the subject moved very awkwardly with many compensatory movements, to 4, meaning excellent movement quality with no awkwardness or compensatory movement. A score of N/A was recorded if a subject did not progress to the subtask before running out of time.
To analyze the data, log files were run through a custom MATLAB script (publicly available at github.com/dbp-osel/DARPA-HAPTIX-VR-Analysis), and the VR recordings were played in an executable included with MuJoCo. The VR recordings were inspected to verify that the task was completed and to identify the number of attempts to complete a task. The task log file was exported at the end of each experiment containing the task completion time for off-line analysis. Statistical analysis was performed with a custom script written in R. A McNemar test was used compare completion rate differences. A Mann–Whitney U test was used to compare attempt rate and completion time. All statistical tests were run with α = 0.05 and with Bonferroni correction. The tasks were compared to determine whether there was a significant difference in task difficulty based on task design. Subtasks scores and values (e.g., time in seconds) were averaged across all subjects for each of the high-level tasks. This provided a quick view of which subtasks were the most difficult for subjects to complete.
Results
Virtual Reality Task Completion Rate
Tasks Sphere Cup, Spoon Tran., Lock Key, and Checkers could not be completed by the subjects (p = 1), as shown in Tables 2, 3 (statistical comparison of task completion rate in VR for all tasks; p-values produced from the McNemar test where α = 0.05). Values with an * and highlighted in gray were found to be statistically significant. The completion rate for Move Cyl was not significantly different from the aforementioned tasks (p = 0.0625). Tasks Sphere Bowl, Doorknob, and Writing had the highest completion rates and were found to have a statistically significant difference (p < 0.05) from tasks Sphere Cup, Spoon Tran., Lock Key, and Checkers. Of the seven subtask actions (reach, grasp, pick up, place, release, move, and rotate), the reach action had the highest completion rate regardless of the high-level task (82.73%) (Tables 4, 5).
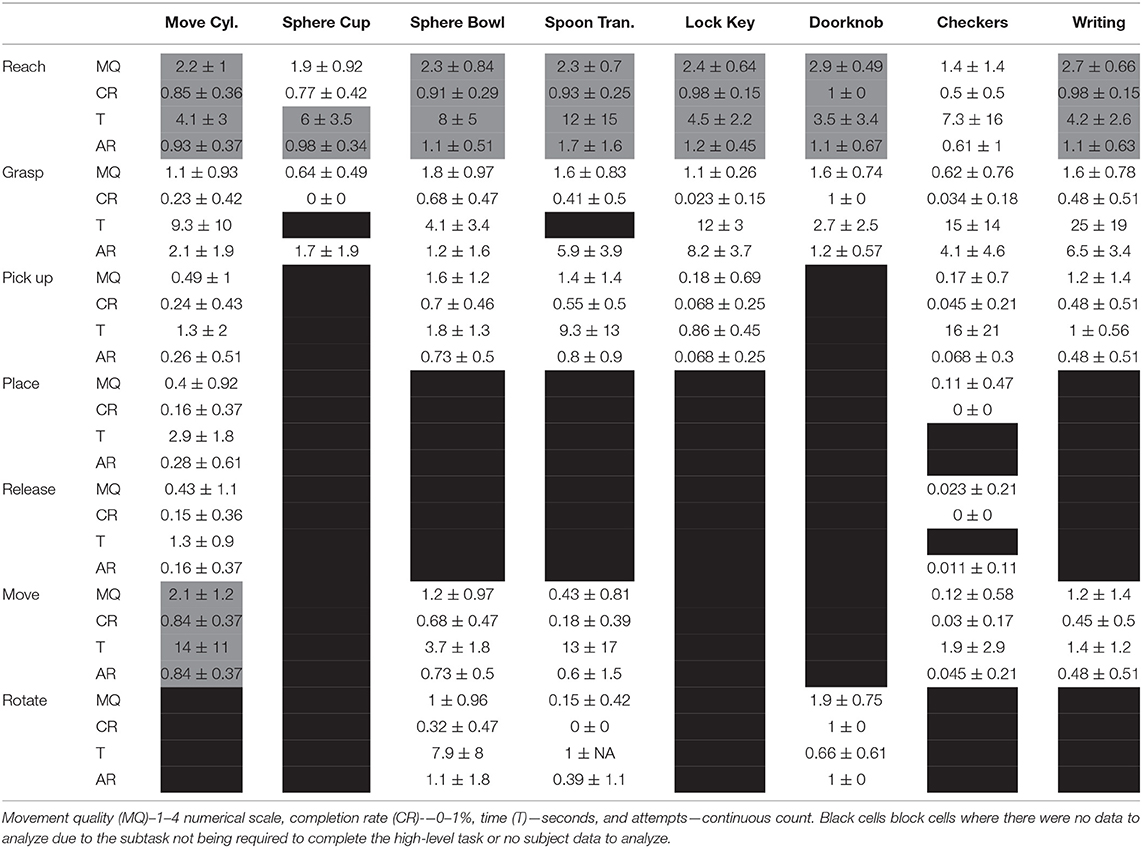
Table 5. Average and standard deviation for VR characteristic values across subtasks and their high-level tasks.
Virtual Reality Task Completion Time
Since tasks Sphere Cup, Spoon Tran., Lock Key, and Checkers could not be completed by the subjects, there was no completion time data to compare between them resulting in no p-values to report. The remaining tasks were all found to have a statistically significant difference in completion time (p < 0.05) (Table 6). On average, subjects took the longest to complete the reach and move actions; taking 5.96 ± 8.55 s and 8.3 ± 10.83 s, respectively (Tables 4, 5).
Virtual Reality Task Attempt Rate
The average number of attempts at a task can be seen in Figure 7. Tasks that had a higher average attempt rate were most often found to have a lower completion rate. Tasks Sphere Cup, Sphere Bowl, and Doorknob had no statistical difference in attempt rates (p > 0.05) due to their low attempt rate. Tasks Lock Key, Checkers, and Writing had no statistical difference due to their high attempt rates (p > 0.05). All remaining tasks varied in the number of attempts and were found to have a statistically significant difference in attempt rate from one another (Table 7). Subjects used the most attempts to complete the Grasp action with an average of 4.48 ± 3.83 attempts. The pick up, release, and rotate actions all had less than one attempt on average due to subjects not making it to these subtasks often (0.3 ± 0.55, 0.07 ± 0.25, and 0.58 ± 1.13 attempts, respectively) (Tables 4, 5).
Real-World Task Completion Rate
Task completion rate varied between the two task environments (Figure 5). As mentioned previously, Sphere Bowl, Sphere Tran., Lock Key, and Checkers could not be completed in VR Table 2. The Doorknob task was the only task that could be completed 100% of the time in VR and RW. Subjects were able to complete all seven subtask actions with over 95% accuracy regardless of the high-level task (Tables 4, 8).
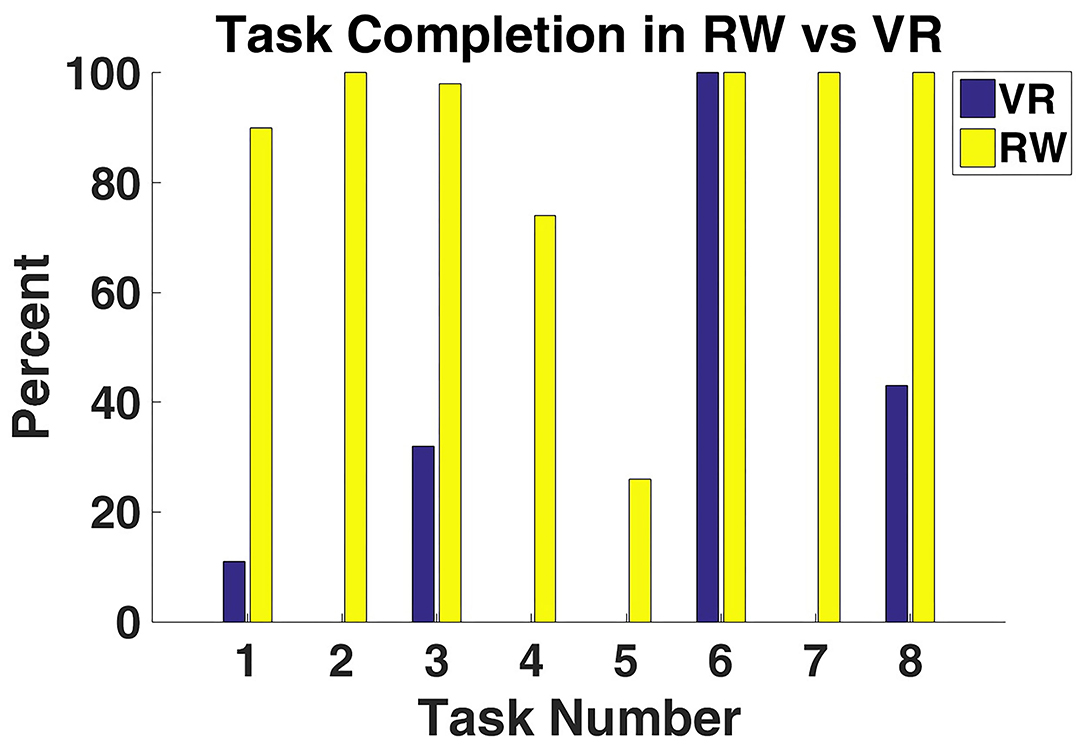
Figure 5. VR and RW task completion percentage for all subjects. Subjects were only able to complete a subset of the tasks in VR, while they were able to complete all the tasks in RW.
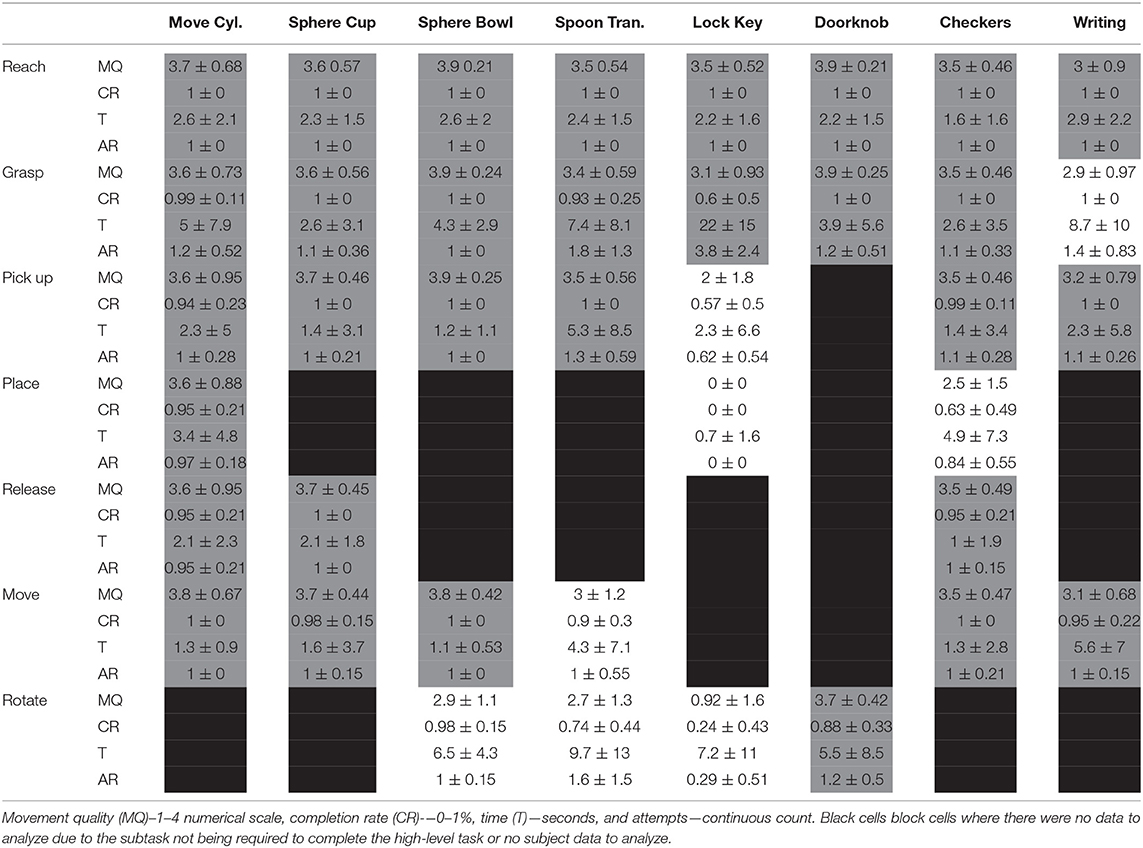
Table 8. Average and standard deviation RW characteristic values across sub-tasks and their high-level tasks.
Real-World Task Completion Time
On average, subjects were able to complete the majority of the tasks faster in RW than in VR (Figure 6). The Doorknob task was the only task that subjects were able to complete faster in VR than in RW. If a task could not be completed, then the data were excluded from the summary statistics. Subjects were able to complete all seven subtask actions in <1 s on average, regardless of the high-level task (Tables 4, 8).
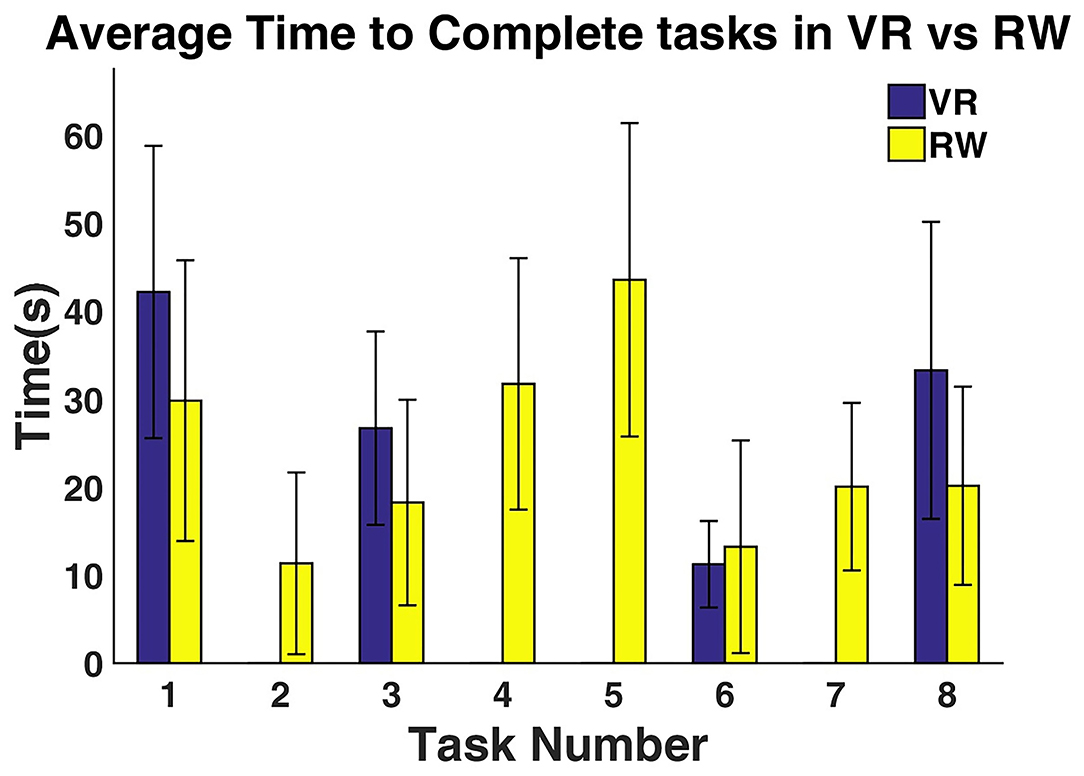
Figure 6. Average time it took subjects to complete tasks in VR vs. RW. Tasks 2, 4, 5, and 7 do not have an average completion time in VR because they could not be completed. Task 6 was the only task that subjects were able to complete faster in VR than in RW. Error bars display standard deviation of the data.
Real-World Task Attempt Rate
On average, subjects required more attempts to complete tasks in VR than in RW (Figure 7). The Lock Key and Checkers tasks took the most attempts to complete in VR. The Spoon Tran. and Lock Key tasks required the most attempts in RW. Most subtask actions took an average of approximately one attempt to complete (Tables 4, 8).
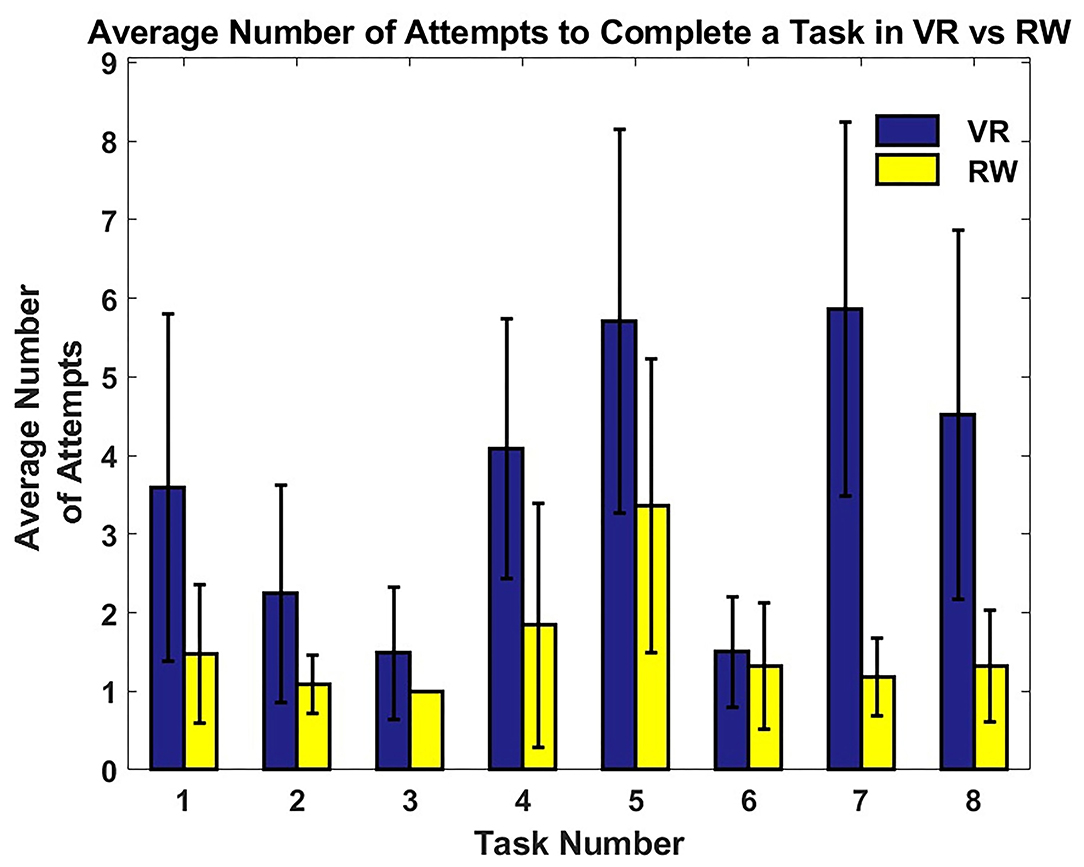
Figure 7. Average number of attempts subjects made while trying to complete a task in VR vs. RW. All tasks required fewer attempts in RW than in VR. The characteristics of the items in the tasks (e.g., small size) had a more marked effect on number of attempts in VR than in RW. Error bars display standard deviation of the data.
Motion Quality and Subtask Analysis
Tables 5, 8 present the average and standard deviations for motion quality (MQ), completion rate (CR), time (T), and attempt rate (AR) for VR and RW, respectively. All subtask actions were not required across all tasks, and in some cases, subjects did not attempt to complete the subtask; these areas are marked with “NA” on the table. Across all tasks in VR, the reach action had the highest average motion quality (>2 points), denoted in green on the table. Completion rate was above 80% for subtasks with a motion quality score greater than two points in VR. Subtask actions that had a motion quality score of less than two points (denoted in red on the table) had a completion rate that was <50% on average.
In the RW environment, the only subtask action to have an average motion quality score <1 was rotate during the Lock and Key task with an average score of 0.917 ± 1.58 (Table 8). Tasks with a motion quality score above tow points had an average completion rate above 50%.
Discussion
Virtual Reality and Real-World Task Completion Rate
Tasks with a low completion rate were difficult due to task characteristics and potential object interactions (Supplementary Table 2). Subjects' task performance varied greatly between the two used environments. In VR, subjects struggled to complete Move Cyl., Sphere Bowl, and Writing tasks while being completely unable to complete Sphere Cup, Spoon Trans., Lock Key, and Checkers tasks. In the RW, subjects were able to complete all the tasks, but struggled the most with the Lock Key task. The differences in performance can be attributed to the contact modeling in VR and object occlusion. Subjects reported an experience of “inaccurate friction,” which caused objects to slip out of the virtual hand more often than they would have in RW. Unrealistic physics in object interactions in VR has been shown to have a negative impact on a user's experience (Lin et al., 2016; McMahan et al., 2016; Höll et al., 2018). This lack of accurate physics causes a mismatch between the user's perception of what should happen and what they are seeing. Improvements are being made to physics calculations to more accurately calculate how an object should respond to touch (Todorov et al., 2012; Höll et al., 2018).
In VR, it was more difficult for subjects to see around their virtual hand to interact with the objects on the table. Because head tracking was not used in this experiment, the only way for them to see the task items from a different perspective was to use a mouse to turn the VR world camera, but this approach would provide a view that could be disorienting if it did not reflect the orientation of the hand. Object contact and occlusion also affected RW performance. In the Lock Key task, subjects tended to have difficulty picking the key up from the table and would occasionally apply too much force to the key. This would cause the key to fly off the table. The prosthetic hand would also block the subject's view of the key, thus leading the subject to lean from side to side to get a better view. There were cases where the subjects would accidently slide the key off the table when the key was occluded.
The subtask action that inhibited completion rate the most in the both environments was the grasp action (Tables 5, 8). If subjects were unable to grasp an object, then they could not progress through the rest of the task. Grasp failure was caused by the object falling out of the prosthetic hand causing the subject to start over or the object falling off the table. Grasping, flexion of the fingers around an object is a necessary action to perform many ADLs (Polygerinos et al., 2015; Raj Kumar et al., 2019). Grasping requires precise manipulation of the fingers to form a grasp and apply enough force to keep an object from slipping free as well as deformation of the soft tissue in the hands around an object (Ciocarlie et al., 2005; Iturrate et al., 2018). Researchers are developing methods to allow prosthetic devices to detect object slippage as well as the design of the prosthetic itself to allow for more human-like motion or finger deformation (Odhner et al., 2013; Stachowsky et al., 2016; Wang and Ahn, 2017). The ability to grasp reliably with a prosthetic device is of high importance to amputees that use prostheses, and the lack of this ability can result in amputees choosing not to use a prosthetic device (Biddiss et al., 2007; Cordella et al., 2016).
Virtual Reality and Real-World Task Completion Time
Subjects on average were able to complete the tasks faster in RW than in VR. Object contact and occlusion affected these results as well. With each failure to maintain object contact in the RW and VR environments, subjects were required to restart the object manipulation attempt. When objects were occluded while attempting object interactions, it would take time to realize missed object pickups, or time was spent to manipulate objects into high-visibility locations to ease interactions. The door knob task was the only task subjects completed faster in VR than in RW because it was easier to turn the virtual door knob. The resistance to turn the door knob was very low; thus, minimal contact was needed. The control scheme for the RW prosthesis could have slowed down the completion time for this task as well. The rotation speed of the RW prosthesis wrist was proportional to the tilt angle of the subject's foot. For example, the Doorknob task could be completed faster if the subject used a steeper inversion angle to make the wrist rotate faster.
Virtual Reality and Real-World Task Attempt Rate
Attempt rate and completion rate were negatively correlated for most of the tasks. Tasks Lock Key and Stacking Checkers had the highest attempt rates out of all the tasks and the lowest completion rates due to small object manipulation and occlusion. This is also reflected in the increased number of attempts at the grasp subtask action in these tasks (Tables 5, 8). In comparison, Tasks Sphere Bowl and Doorknob had the lowest attempt rates and high completion rates due to the manipulation of large objects or objects locked onto the table. However, Tasks Sphere Cup and Writing did not show the same negative relationship. Task Sphere Cup had a low attempt rate due to its early exclusion action that also contributed to the low completion rate. Task Writing had a high attempt rate due to the round pen being flush with the table causing it to roll away from the subjects as they attempted to pick it up. However, the subjects were able to prevent the pen from rolling off the table, allowing them to complete the task.
Repeated, ineffective attempts at completing a task can negatively impact a person's willingness to use a prosthetic device. Gamification of prosthesis training is intended to make prosthesis training more enjoyable and provide a steady stream of feedback (Tabor et al., 2017; Radhakrishnan et al., 2019), though these training games need to be designed appropriately to avoid unnecessary frustration. Training and device use frustration has been shown to cause people to stop using their device (Dosen et al., 2015).
Effect of Motion Quality on Completion Rate
Motion quality scores were positively correlated with task completion rate in both environments. Object view obstruction contributed to the decrease in motion quality scores. Subjects would flex and abduct their shoulders or perform lateral bending of their torso in an effort to view around the prosthetic device they were using. Subjects were also more likely to use compensatory movements when they knew they were running out of time to complete the task. Between the two environments, VR had lower motion quality scores, which is due to the slow movement of subjects while attempting to complete these tasks and the rushed reactions to objects moving away from them. Compensatory movements are known to put extra strain on the musculoskeletal system (Carey et al., 2009; Hussaini et al., 2017; Reilly and Kontson, 2020; Valevicius et al., 2020). This strain can eventually lead to injuries that could cause an individual to stop using their prosthesis. It is important for prosthetists to identify compensatory movements and help train amputees to avoid habitually relying on these types of motions.
Study Limitations
The lack of RW-like friction, object occlusion, and prosthesis control issues all negatively affected the results. These factors made it difficult for subjects to complete tasks, increased the amount of time needed to complete a task, and required subjects to make multiple attempts to complete the task. While task completion strategies positively impacted the results, the tactics that could be applied in one environment were not always compatible with the other environment. In RW, subjects would slide objects to the edge of the table to give themselves access to another side of the object to interact with or to make it easier to get their prosthesis under the object. This tactic could not be applied in VR due to the placement of motion capture cameras and the inability of the hand to go beneath the plane of the table top. Future VR environments should allow subjects to practice all possible RW object manipulation tactics and control in restricting possible tactics to prosthetists for training purposes. Future work will need to explore the use of within-subject design to study the translatability of findings between the two environments.
Another limitation is the difference in training between the two environments. Subjects in the VR experiment were not given training or time to practice picking up objects. The use of the CyberGlove allowed subjects to use their hand to manipulate the virtual prosthetic, therefore reducing, the need to train on device control, but subjects did not know how the virtual prosthesis and objects would interact. Practicing object manipulation on non-task-related items may have improved performance outcomes in VR. While subjects in the RW experiment were given training, it was not significant enough to impact performance. In a study by Bloomer et al., they showed that it would take several days of training to improve performance with a bypass prosthetic (Bloomer et al., 2018). The training given to subjects in this experiment was meant to provide them with baseline knowledge on how to use the device. Future work should provide light training for subjects in VR and RW to ensure that subjects have comparable baseline knowledge.
Conclusions
The results showed that performance between the two used environments can vary greatly depending on task design in VR and the used environment in RW. VR could be used to help device users practice multiple methods to complete a task to later inform strategy testing in RW.
Given the results of this study, virtual task designers should avoid placing objects flush with a table and requiring subjects to manipulate very small objects, and ensure that contact modeling is sufficient for object interactions to feel “natural.” Objects that are flush with the table and small can be easily occluded. Task objects would be less likely to fall out of the virtual hand with improved contact modeling when subjects are attempting different grasps. These factors make it difficult to manipulate objects in VR, causing inaccurately poor results that limit the translatability of the training and progress tracking. The results of the move cyl., sphere bowl, doorknob, and writing tasks were most similar between the VR and RW environments, suggesting that these tasks may be the most useful for VR training and assessment.
Prosthetists using VR to assist with training should use VR environments in intervals and assess frustration with the training. Performing VR training in intervals would provide time for both the prosthetist and amputee to assess how this style of training is working. Reducing the amount of frustration will improve training and help reduce the chance of the amputee forgoing his/her prosthetic.
Additional research is needed using the same prosthesis control schemes between the two environments. Two different control schemes were used in this study, one natural control (“best-case”) scenario and one with the actual prosthetic device control scheme. Even with the best-case scenario control scheme, subjects were unable to complete half of the tasks due to the aforementioned issues. A comparison of performance in VR and RW with the same control scheme would provide more insight into what types of tasks prosthetists could have amputees practice virtually. The ability to virtually practice could help amputees feel comfortable with their devices' control mechanisms and open the door for completely virtual training sessions.
Data Availability Statement
The raw data supporting the conclusions of this article will be made available by the authors, without undue reservation.
Ethics Statement
The studies involving human participants were reviewed and approved by Food and Drug Administration IRB. The patients/participants provided their written informed consent to participate in this study.
Author Contributions
HB and JJ conceptualized the study, methodology, and developed the methodology. JJ, MV-C, and HB validated the study, wrote, and reviewed, and edited the article. JJ made the formal analysis, conducted the investigation and data curation, prepared and wrote the original draft, and conducted the visualization. HB provided the resources and acquired the funding. MV-C and HB supervised the study and handled the project administration. All authors contributed to the article and approved the submitted version.
Funding
This work was sponsored by the Defense Advanced Research Projects Agency (DARPA) BTO through the DARPA-FDA IAA No. 224-14-6009.
Disclaimer
The views, opinions and/or findings expressed are those of the author and should not be interpreted as representing the official views or policies of the Department of Defense or the U.S. Government. The mention of commercial products, their sources, or their use in connection with material reported herein is not to be construed as either an actual or implied endorsement of such products by the Department of Health and Human Services.
Conflict of Interest
The authors declare that the research was conducted in the absence of any commercial or financial relationships that could be construed as a potential conflict of interest.
Acknowledgments
We thank Emo Todorov and his team at Roboti for the development of MuJoCo HAPTIX and contributions to the experiments.
Supplementary Material
The Supplementary Material for this article can be found online at: https://www.frontiersin.org/articles/10.3389/frvir.2021.599274/full#supplementary-material
References
Armiger, R. S., Tenore, F. V., Bishop, W. E., Beaty, J. D., Bridges, M. M., Burck, J. M., et al. (2011). A real-time virtual integration environment for neuroprosthetics and rehabilitation. Johns Hopkins APL Technical Digest. Retrieved from: https://www.semanticscholar.org/paper/A-Real-Time-Virtual-Integration-Environment-for-and-Armiger-Tenore/0352fc07333f6baa6cfa4e49a7c1ab15132f0392
Belter, J. T., Segil, J. L., Dollar, A. M., and Weir, R. F. (2013). Mechanical design and performance specifications of anthropomorphic prosthetic hands: a review. J. Rehabil. Res. Dev. 50, 599–618. doi: 10.1682/JRRD.2011.10.0188
Biddiss, E., Beaton, D., and Chau, T. (2007). Consumer design priorities for upper limb prosthetics. Disabil. Rehabil. Assist. Technol. 2, 346–357. doi: 10.1080/17483100701714733
Biddiss, E., and Chau, T. (2007). Upper-limb prosthetics: critical factors in device abandonment. Am. J. Phys. Med. Rehabil. 86, 977–987. doi: 10.1097/PHM.0b013e3181587f6c
Biddiss, E. A., and Chau, T. T. (2007). Upper limb prosthesis use and abandonment: A survey of the last 25 years. Prosthet. Orthot. Int. 31, 236–257. doi: 10.1080/03093640600994581
Blana, D., Kyriacou, T., Lambrecht, J. M., and Chadwick, E. K. (2016). Feasibility of using combined EMG and kinematic signals for prosthesis control: a simulation study using a virtual reality environment. J. Electromyogr. Kinesiol. 29, 21–27. doi: 10.1016/j.jelekin.2015.06.010
Bloomer, C., Wang, S., and Kontson, K. (2018). Creating a standardized, quantitative training protocol for upper limb bypass prostheses. Phys. Med. Rehabil. Res. 3, 1–8. doi: 10.15761/PMRR.1000191
Carey, S. L., Dubey, R. V., Bauer, G. S., and Highsmith, M. J. (2009). Kinematic comparison of myoelectric and body powered prostheses while performing common activities. Prosthet. Orthot. Int. 33, 179–186. doi: 10.1080/03093640802613229
Carruthers, G. (2008). Types of body representation and the sense of embodiment. Conscious. Cogn. 17, 1302–1316. doi: 10.1016/j.concog.2008.02.001
Ciocarlie, M., Miller, A., and Allen, P. (2005). Grasp Analysis Using Deformable Fingers. Edmonton, AB: IEEE. doi: 10.1109/IROS.2005.1545525
Cordella, F., Ciancio, A. L., Sacchetti, R., Davalli, A., Cutti, A. G., Guglielmelli, E., et al. (2016). Literature review on needs of upper limb prosthesis users. Front. Neurosci. 10:209. doi: 10.3389/fnins.2016.00209
Cornwell, A. S., Liao, J. Y., Bryden, A. M., and Kirsch, R. F. (2012). A standard set of upper extremity tasks for evaluating rehabilitation interventions for individuals with complete arm paralysis. J. Rehabil. Res. Dev. 49, 395–403. doi: 10.1682/JRRD.2011.03.0040
Davoodi, R., and Loeb, G. E. (2011). MSMS software for VR simulations of neural prostheses and patient training and rehabilitation. Stud. Health Technol. Inform. 163, 156–162. doi: 10.3233/978-1-60750-706-2-156
Davoodi, R., Urata, C., Todorov, E., and Loeb, G. E. (2004). “Development of clinician-friendly software for musculoskeletal modeling and control,” in Paper Presented at the The 26th Annual International Conference of the IEEE Engineering in Medicine and Biology Society (San Francisco, CA).
Dosen, S., Markovic, M., Somer, K., Graimann, B., and Farina, D. (2015). EMG Biofeedback for online predictive control of grasping force in a myoelectric prosthesis. J. Neuroeng. Rehabil. 12:55. doi: 10.1186/s12984-015-0047-z
Gamberini, L. (2004). Virtual reality as a new research tool for the study of human memory. CyberPsychol. Behav. 3, 337–342. doi: 10.1089/10949310050078779
George, J. A., Davis, T. S., Brinton, M. R., and Clark, G. A. (2020). Intuitive neuromyoelectric control of a dexterous bionic arm using a modified Kalman filter. J. Neurosci. Methods 330:108462. doi: 10.1016/j.jneumeth.2019.108462
Giboin, L. S., Gruber, M., and Kramer, A. (2015). Task-specificity of balance training. Hum. Mov. Sci. 44, 22–31. doi: 10.1016/j.humov.2015.08.012
Harada, A., Nakakuki, T., Hikita, M., and Ishii, C. (2010). “Robot finger design for myoelectric prosthetic hand and recognition of finger motions via surface EMG,” in Paper Presented at the IEEE International Conference on Automation and Logistics (Hong Kong, Macau). doi: 10.1109/ICAL.2010.5585294
Haverkate, L., Smit, G., and Plettenburg, D. H. (2016). Assessment of body-powered upper limb prostheses by able-bodied subjects, using the box and blocks test and the nine-hole peg test. Prosthet. Orthot. Int. 40, 109–116. doi: 10.1177/0309364614554030
Hebert, J. S., and Lewicke, J. (2012). Case report of modified Box and Blocks test with motion capture to measure prosthetic function. J. Rehabil. Res. Dev. 49, 1163–1174. doi: 10.1682/JRRD.2011.10.0207
Hebert, J. S., Lewicke, J., Williams, T. R., and Vette, A. H. (2014). Normative data for modified box and blocks test measuring upper-limb function via motion capture. J. Rehabil. Res. Dev. 51, 918–932. doi: 10.1682/JRRD.2013.10.0228
Hignett, S., Hancox, G., Pillin, H., Silmäri, J., O'Leary, A., and Brodrick, E. (2019). Integrating Macro and Micro Hierarchical Task Analyses to Embed New Medical Devices in Complex Systems. Available online at: https://pdfs.semanticscholar.org/0d61/0cd6d5876525410bc2a83cd5015915969848.pdf?_ga=2.169610215.732966907.1610459164-22837427.1555078550
Hofmann, M., Harris, J., Hudson, S. E., and Mankoff, J. (2016). “Helping hands,” in Paper Presented at the Proceedings of the 2016 CHI Conference on Human Factors in Computing Systems. https://dl.acm.org/ doi: 10.1145/2858036.2858340
Höll, M., Oberweger, M., Arth, C., and Lepetit, V. (2018). Efficient Physics-Based Implementation for Realistic Hand-Object Interaction in Virtual Reality. Reutlingen: IEEE Virtual Reality. doi: 10.1109/VR.2018.8448284
Hoshigawa, S., Jiang, Y., Kato, R., Morishita, S., Nakamura, T., Yabuki, Y., et al. (2015). “Structure design for a Two-DoF myoelectric prosthetic hand to realize basic hand functions in ADLs,” in Paper Presented at the Engineering in Medicine and Biology Society (EMBC) (Milan). doi: 10.1109/EMBC.2015.7319463
Hussaini, A., Zinck, A., and Kyberd, P. (2017). Categorization of compensatory motions in transradial myoelectric prosthesis users. Prosthet. Orthot. Int. 41, 286–293. doi: 10.1177/0309364616660248
Iturrate, I., Chavarriaga, R., Pereira, M., Zhang, H., Corbet, T., Leeb, R., et al. (2018). Human EEG reveals distinct neural correlates of power and precision grasping types. Neuroimage 181, 635–644. doi: 10.1016/j.neuroimage.2018.07.055
Ivorra, E., Ortega, M., Alcaniz, M., and Garcia-Aracil, N. (2018). Multimodal Computer Vision Framework for Human Assistive Robotics. Retrieved from: https://ieeexplore.ieee.org/stamp/stamp.jsp?tp=&arnumber=8428330 doi: 10.1109/METROI4.2018.8428330
Katyal, K. D., Johannes, M. S., Kellis, S., Aflalo, T., Klaes, C., McGee, T. G., et al. (2014). A Collaborative BCI Approach to Autonomous Control of a Prosthetic Limb System. Retrieved from: https://ieeexplore.ieee.org/stamp/stamp.jsp?tp=andarnumber=6974124 doi: 10.1109/SMC.2014.6974124
Katyal, K. D., Johannes, M. S., McGee, T. G., Harris, A. J., Armiger, R. S., Firpi, A. H., et al. (2013). “HARMONIE: a multimodal control framework for human assistive robotics,” in Paper Presented at the 2013 6th International IEEE/EMBS Conference on Neural Engineering (NER) (San Diego, CA). doi: 10.1109/NER.2013.6696173
Kearns, N. T., Peterson, J. K., Smurr Walters, L., Jackson, W. T., Miguelez, J. M., and Ryan, T. (2018). Development and psychometric validation of capacity assessment of prosthetic performance for the upper limb (CAPPFUL). Arch. Phys. Med. Rehabil. 99, 1789–1797. doi: 10.1016/j.apmr.2018.04.021
Kim, J. S., and Park, J. M. (2016). “Direct and realistic handover of a virtual object,” in Paper Presented at the International Conference on Intelligent Robots and Systems (IROS) (Daejeon). doi: 10.1109/IROS.2016.7759170
Kluger, D. T., Joyner, J. S., Wendelken, S. M., Davis, T. S., George, J. A., Page, D. M., et al. (2019). Virtual reality provides an effective platform for functional evaluations of closed-loop neuromyoelectric control. IEEE Trans. Neural Syst. Rehabil. Eng. 27, 876–886. doi: 10.1109/TNSRE.2019.2908817
Kontson, K., Marcus, I., Myklebust, B., and Civillico, E. (2017). Targeted box and blocks test: normative data and comparison to standard tests. PLoS ONE 12:15. doi: 10.1371/journal.pone.0177965
Kumar, V., and Todorov, E. (2015). “MuJoCo HAPTIX: a virtual reality system for hand manipulation,” in Paper Presented at the 2015 IEEE-RAS 15th International Conference on Humanoid Robots (Humanoids). doi: 10.1109/HUMANOIDS.2015.7363441
Lambrecht, J. M., Pulliam, C. L., and Kirsch, R. F. (2011). Virtual reality environment for simulating tasks with a myoelectric prosthesis: an assessment and training tool. J. Prosthet. Orthot. 23, 89–94. doi: 10.1097/JPO.0b013e318217a30c
Latash, M. L. (1996). “Change in movement and skill learning, retention, and transfer,” in Dexterity and Its Development, eds M. L. Latash, M. T. Turvey, and N. A. Bernshtein (Mahwah, NJ: Erlbaum), 393–430.
Levin, M. F., Magdalon, E. C., Michaelsen, S. M., and Quevedo, A. A. (2015). Quality of grasping and the role of haptics in a 3-D immersive virtual reality environment in individuals with stroke. IEEE Trans. Neural Syst. Rehabil. Eng. 23, 1047–1055. doi: 10.1109/TNSRE.2014.2387412
Lim, J. H., Pinheiro, P. O., Rostamzadeh, N., Pal, C., and Ahn, S. (2019). “Neural multisensory scene inference,” in Paper Presented at the Conference on Neural Information Processing Systems (Vancouver, BC).
Lin, J., Guo, X., Shao, J., Jiang, C., Zhu, Y., and Zhu, S. C. (2016). “A virtual reality platform for dynamic human-scene interaction,” in Paper Presented at the SIGGRAPH ASIA 2016 Virtual Reality meets Physical Reality: Modelling and Simulating Virtual Humans and Environments (New York, NY). doi: 10.1145/2992138.2992144
Lin, K. C., Chuang, L. L., Wu, C. Y., Hsieh, Y. W., and Chang, W. Y. (2010). Responsiveness and validity of three dexterous function measures in stroke rehabilitation. J. Rehabil. Res. Dev. 47, 563–571. doi: 10.1682/JRRD.2009.09.0155
Mathiowetz, V., Volland, G., Kashman, N., and Weber, K. (1985). Adult norms for the box and block test of manual dexterity. Am. J. Occup. Ther. 39, 386–391. doi: 10.5014/ajot.39.6.386
McGimpsey, G., and Bradford, T. (2017). Limb Prosthetics Services and Devices Critical Unmet Need: Market Analysis. Retrieved from: https://www.nist.gov/system/files/documents/2017/04/28/239_limb_prosthetics_services_devices.pdf
McMahan, R. P., Lai, C., and Pal, S. K. (2016). Interaction Fidelity: The Uncanny Valley of Virtual Reality Interactions. Cham: Springer International Publishing. doi: 10.1007/978-3-319-39907-2_6
McMullen, D. P., Hotson, G., Katyal, K. D., Wester, B. A., Fifer, M. S., McGee, T. G., et al. (2014). Demonstration of a semi-autonomous hybrid brain-machine interface using human intracranial EEG, eye tracking, and computer vision to control a robotic upper limb prosthetic. IEEE Trans. Neural Syst. Rehabil. Eng. 22, 784–796. doi: 10.1109/TNSRE.2013.2294685
Miranda, R. A., Casebeer, W. D., Hein, A. M., Judy, J. W., Krotkov, E. P., Laabs, T. L., et al. (2015). DARPA-funded efforts in the development of novel brain-computer interface technologies. J. Neurosci. Methods 244, 52–67. doi: 10.1016/j.jneumeth.2014.07.019
Nakamura, G., Shibanoki, T., Kurita, Y., Honda, Y., Masuda, A., Mizobe, F., et al. (2017). A virtual myoelectric prosthesis training system capable of providing instructions on hand operations. Int. J. Adv. Robot. Syst. 14. doi: 10.1177/1729881417728452
Nissler, C., Nowak, M., Connan, M., Buttner, S., Vogel, J., Kossyk, I., et al. (2019). VITA-an everyday virtual reality setup for prosthetics and upper-limb rehabilitation. J. Neural Eng. 16:026039. doi: 10.1088/1741-2552/aaf35f
Odette, K., and Fu, Q. (2019). A Physics-based Virtual Reality Environment to Quantify Functional Performance of Upper-limb Prostheses. Berlin: IEEE.
Odhner, L. U., Ma, R. R., and Dollar, A. M. (2013). Open-loop precision grasping with underactuated hands inspired by a human manipulation strategy. IEEE Trans. Automat. Sci. Eng. 10, 625–633. doi: 10.1109/TASE.2013.2240298
Park, J., and Cheong, J. (2010). “Analysis of collective behavior and grasp motion in human hand,” in Paper Presented at the International Conference on Control, Automation and Systems, (Gyeonggi-do).
Patrick, J., Gregov, A., and Halliday, P. (2000). Analysing and training task analysis. Instruct. Sci. 28, 51–79. doi: 10.1023/A:1003583420137
Perry, B. N., Armiger, R. S., Yu, K. E., Alattar, A. A., Moran, C. W., Wolde, M., et al. (2018). Virtual integration environment as an advanced prosthetic limb training platform. Front. Neurol. 9:785. doi: 10.3389/fneur.2018.00785
Phelan, I., Arden, M., Garcia, C., and Roast, C. (2015). “Exploring virtual reality and prosthetic training,” in Paper Presented at the 2015 IEEE Virtual Reality (VR) (Arles). doi: 10.1109/VR.2015.7223441
Polygerinos, P., Galloway, K. C., Sanan, S., Herman, M., and Walsh, C. J. (2015). EMG Controlled Soft Robotic Glove for Assistance During Activities of Daily Living. Singapore: IEEE. doi: 10.1109/ICORR.2015.7281175
Pons, J. L., Ceres, R., Rocon, E., Levin, S., Markovitz, I., Saro, B., et al. (2005). Virtual reality training and EMG control of the MANUS hand prosthesis. Robotica 23, 311–317. doi: 10.1017/S026357470400133X
Prahm, C., Kayali, F., Sturma, A., and Aszmann, O. (2018). Playbionic: game-based interventions to encourage patient engagement and performance in prosthetic motor rehabilitation. PM R 10, 1252–1260. doi: 10.1016/j.pmrj.2018.09.027
Prahm, C., Vujaklija, I., Kayali, F., Purgathofer, P., and Aszmann, O. C. (2017). Game-Based Rehabilitation for Myoelectric Prosthesis Control. JMIR Ser. Games 5:e3. doi: 10.2196/games.6026
Radhakrishnan, M., Smailagic, A., French, B., Siewiorek, D. P., and Balan, R. K. (2019). Design and Assessment of Myoelectric Games for Prosthesis Training of Upper Limb Amputees. Kyoto: IEEE.
Raj Kumar, A., Bilaloglu, S., Raghavan, P., and Kapila, V. (2019). “Grasp rehabilitator: a mechatronic approach,” in Paper Presented at the 2019 Design of Medical Devices Conference (Minneapolis, MN). doi: 10.1115/DMD2019-3242
Reilly, M., and Kontson, K. (2020). Computational musculoskeletal modeling of compensatory movements in the upper limb. J. Biomech. 108:109843. doi: 10.1016/j.jbiomech.2020.109843
Resnik, L., Acluche, F., Lieberman Klinger, S., and Borgia, M. (2018a). Does the DEKA Arm substitute for or supplement conventional prostheses. Prosthet. Orthot. Int. 42, 534–543. doi: 10.1177/0309364617729924
Resnik, L., Adams, L., Borgia, M., Delikat, J., Disla, R., Ebner, C., et al. (2013). Development and evaluation of the activities measure for upper limb amputees. Arch. Phys. Med. Rehabil. 94, 488–494.e484. doi: 10.1016/j.apmr.2012.10.004
Resnik, L., and Borgia, M. (2014). User ratings of prosthetic usability and satisfaction in VA study to optimize DEKA arm. J. Rehabil. Res. Dev. 51, 15–26. doi: 10.1682/JRRD.2013.02.0056
Resnik, L., Etter, K., Klinger, S. L., and Kambe, C. (2011). Using virtual reality environment to facilitate training with advanced upper-limb prosthesis. J. Rehabil. Res. Dev. 48, 707–718. doi: 10.1682/JRRD.2010.07.0127
Resnik, L., Klinger, S. L., and Etter, K. (2014a). User and clinician perspectives on DEKA arm: results of VA study to optimize DEKA arm. J. Rehabil. Res. Dev. 51, 27–38. doi: 10.1682/JRRD.2013.03.0068
Resnik, L., Latlief, G., Klinger, S. L., Sasson, N., and Walters, L. S. (2014b). Do users want to receive a DEKA Arm and why? Overall findings from the Veterans Affairs Study to optimize the DEKA Arm. Prosthet. Orthot. Int. 38, 456–466. doi: 10.1177/0309364613506914
Resnik, L., Meucci, M. R., Lieberman-Klinger, S., Fantini, C., Kelty, D. L., Disla, R., et al. (2012). Advanced upper limb prosthetic devices: implications for upper limb prosthetic rehabilitation. Arch. Phys. Med. Rehabil. 93, 710–717. doi: 10.1016/j.apmr.2011.11.010
Resnik, L. J., Borgia, M. L., Acluche, F., Cancio, J. M., Latlief, G., and Sasson, N. (2018b). How do the outcomes of the DEKA Arm compare to conventional prostheses? PLoS ONE 13:e0191326. doi: 10.1371/journal.pone.0191326
Salvendy, G. (2012). Handbook of Human Factors and Ergonomics (G. Salvendy Ed. Fourth ed.). Hoboken, NJ: John Wiley & Sons, Inc. doi: 10.1002/9781118131350
Sears, E. D., and Chung, K. C. (2010). Validity and responsiveness of the Jebsen-Taylor hand function test. J. Hand Surg. Am. 35, 30–37. doi: 10.1016/j.jhsa.2009.09.008
Stachowsky, M., Hummel, T., Moussa, M., and Abdullah, H. A. (2016). A slip detection and correction strategy for precision robot grasping. IEEE/ASME Trans. Mechatron. 21, 2214–2226. doi: 10.1109/TMECH.2016.2551557
Stickel, C., Ebner, M., and Holzinger, A. (2010). “The XAOS metric – understanding visual complexity as measure of usability,” in HCI in Work and Learning, Life and Leisure, eds G. Leitner, M. Hitz, and A. Holzinger (Berlin; Heidelberg: Springer), 278–290. doi: 10.1007/978-3-642-16607-5_18
Stone, R. J. (2001). “Haptic feedback: a brief history from telepresence to virtual reality,” in Paper Presented at the Haptic HCI 2000: Haptic Human-Computer Interaction (Berlin; Heidelberg). doi: 10.1007/3-540-44589-7_1
Tabor, A., Bateman, S., Scheme, E., Flatla, D. R., and Gerling, K. (2017). “Designing game-based myoelectric prosthesis training,” in Paper Presented at the Proceedings of the 2017 CHI Conference on Human Factors in Computing Systems (Denver, CO). doi: 10.1145/3025453.3025676
Todorov, E., Erez, T., and Tassa, Y. (2012). “MuJoCo: a physics engine for model-based control,” in Paper presented at the 2012 IEEE/RSJ International Conference on Intelligent Robots and Systems (Vilamoura-Algarve). doi: 10.1109/IROS.2012.6386109
Valevicius, A. M., Boser, Q. A., Chapman, C. S., Pilarski, P. M., Vette, A. H., and Hebert, J. S. (2020). Compensatory strategies of body-powered prosthesis users reveal primary reliance on trunk motion and relation to skill level. Clin. Biomech. 72, 122–129. doi: 10.1016/j.clinbiomech.2019.12.002
van der Laan, T. M. J., Postema, S. G., Reneman, M. F., Bongers, R. M., and van der Sluis, C. K. (2017). Development and reliability of the rating of compensatory movements in upper limb prosthesis wearers during work related tasks. J. Hand Ther. 32, 368–374. doi: 10.1016/j.jht.2017.12.003
van der Riet, D., Stopforth, R., Bright, G., and Diegel, O. (2013). “An overview and comparison of upper limb prosthetics,” in Paper presented at the Africon, Pointe-Aux-Piments, Mauritius (Pointe aux Piments). doi: 10.1109/AFRCON.2013.6757590
van Dijk, L., van der Sluis, C. K., van Dijk, H. W., and Bongers, R. M. (2016). Task-oriented gaming for transfer to prosthesis use. IEEE Trans. Neural Syst. Rehabil. Eng. 24, 1384–1394. doi: 10.1109/TNSRE.2015.2502424
Wang, S., Hsu, C. J., Trent, L., Ryan, T., Kearns, N. T., Civillico, E. F., et al. (2018). Evaluation of performance-based outcome measures for the upper limb: a comprehensive narrative review. PM R 10, 951–962 e953. doi: 10.1016/j.pmrj.2018.02.008
Wang, W., and Ahn, S. H. (2017). Shape memory alloy-based soft gripper with variable stiffness for compliant and effective grasping. Soft Robot. 4, 379–389. doi: 10.1089/soro.2016.0081
Woodward, R. B., and Hargrove, L. J. (2018). Robust Pattern Recognition Myoelectric Training for Improved Online Control within a 3D Virtual Environment. Honolulu, HI: IEEE. doi: 10.1109/EMBC.2018.8513183
Zheng, J. Z., De La Rosa, S., and Dollar, A. M. (2011). “An investigation of grasp type and frequency in daily household and machine shop tasks,” in Paper presented at the IEEE International Conference on Robotics and Automation (Shanghai). doi: 10.1109/ICRA.2011.5980366
Ziegler-Graham, K., MacKenzie, E. J., Ephraim, P. L., Travison, T. G., and Brookmeyer, R. (2008). Estimating the prevalence of limb loss in the United States: 2005 to 2050. Arch. Phys. Med. Rehabil. 89, 422–429. doi: 10.1016/j.apmr.2007.11.005
Keywords: activities of daily living, performance metrics, virtual task environment, upper limb prosthesis, functional performance
Citation: Joyner JS, Vaughn-Cooke M and Benz HL (2021) Comparison of Dexterous Task Performance in Virtual Reality and Real-World Environments. Front. Virtual Real. 2:599274. doi: 10.3389/frvir.2021.599274
Received: 26 August 2020; Accepted: 22 February 2021;
Published: 20 April 2021.
Edited by:
Anat Vilnai Lubetzky, New York University, United StatesReviewed by:
Savita G. Bhakta, University of California, San Diego, United StatesTal Krasovsky, University of Haifa, Israel
Copyright © 2021 Joyner, Vaughn-Cooke and Benz. This is an open-access article distributed under the terms of the Creative Commons Attribution License (CC BY). The use, distribution or reproduction in other forums is permitted, provided the original author(s) and the copyright owner(s) are credited and that the original publication in this journal is cited, in accordance with accepted academic practice. No use, distribution or reproduction is permitted which does not comply with these terms.
*Correspondence: Janell S. Joyner, ampveW5lcjImI3gwMDA0MDt0ZXJwbWFpbC51bWQuZWR1