- 1Institute of Psychology, Polish Academy of Sciences, Warsaw, Poland
- 2Central Institute for Labor Research, National Research Institute, Warsaw, Poland
This study investigated the efficacy of training a complex skill within an immersive virtual environment. We constructed a sensorimotor workstation task and trained participants to operate it with either a non-interactive (text, video) method or with a virtual equivalent of the workstation. Our results indicate that virtual reality trained participants had significantly higher accuracy and produced fewer timeouts in the workstation task than those trained using non-interactive methods, demonstrating a successful transfer of skills between virtual reality and the real world. Further, comparing younger and older adults in their performance, we found that older adults did not differ in their ability to benefit from virtual reality training, and that the extent of this benefit was not significantly affected by existing cognitive deficits (as measured using the Operation Span and AX-Continuous Performance tasks). These findings suggest that virtual reality technologies can find application in a wide range of skill training contexts and across diverse age groups.
Introduction
Virtual reality (VR) has long been used as a platform for skill training. The most notable and widely implemented example is likely the use of flight simulators for pilot training, with favorable evidence as to its efficacy (for meta-analysis see: Hays, Jacobs, Prince, and Salas, 1992). More recently, the use of VR for laparoscopic training has seen wide adoption and study, with a growing body of evidence for its ability to lower error rates and operating times for novice surgeons as well as its significant advantages over video-based training (Gurusamy et al., 2008; Nagendran et al., 2013; Alaker et al., 2016). Virtual reality-based training is also increasingly present in industry fields, such as for mining operations (Stothard et al., 2015) and manufacturing procedures (Gavish, et al., 2015; Westerfield et al., 2015).
Based on the yet limited empirical evidence, it appears that virtual reality can be used to successfully teach individuals tasks that are demanding of both manual and cognitive abilities. Further, past research (such as that cited above) also suggests that virtual reality training is as or more effective than more traditional training methods, ranging from training manuals to hands-on practice. This factor is of special importance, as it means that skills which typically imply some level of threat to the trainee or subject can be trained in a risk-free environment. Coupled with the practicality of being able to deploy virtual reality solutions almost anywhere, it seems likely that VR will continue to increase its adoption rate as the technology matures and solutions become more affordable.
Despite its promise, there is currently very little empirical data on just how generally and widely virtual reality can be implemented in the training of complex skills. One known barrier to the implementation of any learning or training paradigm is that of transfer, or the ability for improvements in one domain to be reflected in other domains. It is generally held that training in a task will improve performance at that task (and other nearly identical tasks), but that these improvements typically do not carry over to other related tasks (Stine-Morrow and Basak, 2011; Noack et al., 2014; Simons et al., 2016). In the case of virtual reality as a training medium, some work has already been done to describe the types of transfer that can be elicited in virtual environments, and the conditions that promote it (Bossard et al., 2008; Buhr et al., 2019). However, experimental data is mostly limited to the previously described contexts of laparoscopic surgery (for study of transfer in this context see: Lehmann et al., 2005) and flight simulation, with sparse and mixed evidence in other domains (e.g. Rose et al., 2000; Gavish, et al., 2015).
A second possible barrier to the mass adoption of VR technology for skill training may also be the limited knowledge of how the use of such methods interacts with age. Given the rapidly aging populations of most industrialized nations (e.g. in the European Union, 28.5% of the population is expected to be over the age of 65 within the next 3 decades: Eurostat, 2019), it is inevitable that older adults will come into contact with VR technology. This is particularly likely in the form of job retraining, as the workforce adapts to the inevitable loss of historically prevalent jobs to automation (Czaja and Sharit, 2009; Campos et al., 2020). As such, the question of how well older adults can adapt to such technologies is highly relevant.
It is well known that cognitive abilities decline with age, beginning as soon as the early 20s (Salthouse, 2004). This is seen in a linear decline in processing speed with age, and an increasing curvilinear decline in memory and reasoning abilities (Salthouse, 2019). However, cognitive aging is also accompanied by a shift from direct novel information processing strategies to those relying more heavily on available knowledge (Jones and Conrad, 1933). This has been suggested as a natural compensatory mechanism to aging (Kanfer and Ackerman, 2004), and may help to explain the frequent lack of accompanying decreases in real-world accomplishment or competency (Salthouse, 2010).
Although there are multiple models explaining the process of cognitive aging (for overview see: Anderson and Craik, 2017), one that seems most relevant in the case of virtual reality applications is the Context Processing Theory of Cognitive Control (Braver et al., 2001). The authors posit that impairments to the ability to process context, i.e. “any task-relevant information that is internally represented in such a form that it can bias processing in the pathways responsible for performance”, play a central role in explaining the cognitive declines that come with age. In other words, older adults struggle with maintaining task relevant information (context) in their working memory, and this explains many of the deficits seen in different aspects of cognitive control. At the same time, there are studies showing that older adults perform significantly better at tasks when they are conducted within ecological/naturalistic contexts, i.e. those they were more familiar with (Garden et al., 2001; Henry et al., 2004; Bordin et al., 2006).
Considering these factors, it is unclear if the efficacy of VR-based skill training is equivalent across age groups. On the one hand, VR provides greatly improved ecological validity over traditional mediums such as manuals and video-based instructions. The possibility of creating training environments that are virtually identical to real-world equivalents is a seemingly positive facet when considering the benefits of familiarity to older adult performance. Further, a high degree of similarity between training and task environment is considered by some theories of transfer to be the fundamental basis for successful transfer (Thorndike’s theory of transfer by identical elements: Thorndike, 1906). On the other hand, older adults are unlikely to have had any previous experience with virtual reality. This adds an additional layer of difficulty to the experience in the form of an unfamiliar medium and interface. In older adults with context processing deficits, this factor may lead to the further constraint of already limited cognitive resources, which in turn can exacerbate existing deficits.
We were unable to identify any research comparing the relative effectiveness of VR-based skill training on real-world performance between younger and older adults. Most research involving older adults and VR is focused on its use in various forms of rehabilitation (Optale et al., 2010; Lee et al., 2016; Micarelli et al., 2019) and in improving physical health (for review see: Molina et al., 2014). One study was also able to demonstrate the transfer of improvements in attentional control training to performance in a VR “virtual car ride” in older adults (Bier et al., 2018), which offers some evidence that older adults are able to carry over real-world abilities into the context of complex tasks in virtual reality. This is a promising result, as transfer of training gains has previously been reported as reduced in older adults when compared to younger adults (at least in the case of working memory training: Brehmer et al., 2012; Zinke et al., 2014). Further, studies of the feasibility of using VR technology in populations of older adults generally indicate that older adults tolerate the use of head-mounted displays well, report minimal cybersickness, and tend to report positive attitudes towards VR technology after experiencing it (Appel et al., 2019; Huygelier et al., 2019).
The paucity of available data on how the efficacy of VR training solutions is affected by age motivated us to design an experiment in which the performance of younger and older adults in a real-world task is measured after undergoing training in either an immersive virtual reality (IVR) version of the task or after training using non-interactive methods (multimedia, written materials). Further, as the experimental evidence for transfer between virtual tasks and real-world tasks is still very limited, our experiment also serves to test the extent to which skills learned in an IVR training can transfer into an analogous real-world task. Based on the principle of identical elements in theories of transfer and previous (albeit limited) research, we expect to find a significant advantage in the training efficacy of IVR when compared to non-interactive media. In the case of older adults, given the suspected crucial role of context processing deficits in the manifestation of age-related cognitive decline, we expect to find that older adults will see the same relative benefit of IVR training over non-interactive training after accounting for context processing and working memory performance.
Material and Methods
Participants
One hundred eighteen participants (69 women) took part in this experiment. Participants were divided into two groups based on age: younger adults (n = 60, M = 23.95, SD = 2.71, Min = 20, Max = 30) and older adults (n = 58, M = 59.05, SD = 5.82, Min = 50, Max = 77). Additionally, participants were randomly assigned to one of two training groups: the non-interactive methods group, which consisted of a manual and training video, and the IVR training group, which consisted of a virtual simulation of the real-world task. All participants had good or corrected vision. Informed consent was obtained from all participants prior to the study, and 120 PLN compensation (approx. 30 USD) was paid upon study completion. The study plan received ethical committee approval from the local ethics committee.
Cognitive Tasks and Questionnaires
The well-known Automated Operation Span task (Unsworth et al., 2005) was used to obtain measures of working memory performance, and the AX Continuous Performance Test (Rush et al., 2006) was used to measure the context processing abilities of participants (see Supplementary materials for full task descriptions). All older adults were screened using the Mini-Mental State Examination (Folstein et al., 1975). Prior to the experiment, all participants reported a simple yes/no answer to whether they had any previous experience with virtual reality. After the training, we tested the recall of participants by asking them questions about the number of objects and the functions of the workstation screens and buttons. The Simulator Sickness Questionnaire (Kennedy et al., 1993) was applied to participants in the IVR training groups. Finally, after the real-world task execution, participants were given the Training and Work assessment questionnaire which asked about the subjectively perceived level of control over the task, self-assessment of performance, received training impact, and the quality of training materials.
Real-World Task
The task being trained in the reported study was comprised of two interconnected subtasks. The primary task was inspired by an electronic assembly task used in previous VR training studies (Gavish et al., 2015; Westerfield et al., 2015) and the "Symbol Digit Substitution Test" (Thorndike, 1919), which is broadly used in cognitive psychology to measure processing speed ability. Participants were asked to match three-dimensional (3D printed) simple geometric objects to the correct slots on a 3 by 3 matrix, while simultaneously maintaining the level of a gauge by pressing a button (see Figure 1). Eight objects in total were used, differing in shape and color. The distribution of objects was initially randomly assigned for each trial, and then these trials and their compositions were fixed across participants. Six blocks of five trials were administered, with each consecutive block representing a higher difficulty level. The difficulty was varied by manipulating object load (i.e. number of objects to place), with possible loads of four to nine objects (see Supplementary Material for more details). Participants had up to 10 s to place each object and were informed that they were not being judged on their speed, so long as the task was completed within the limit. At the same time, they needed to constantly respond to the secondary task by pressing a button to keep a gauge at an optimal level (see Supplementary Figure S2 for depiction). If the gauge reached its minimum or maximum, the trial was considered a timeout. The primary performance metrics in this task are the number of correctly placed objects within a given load level (accuracy) and the number of secondary task timeouts. We also measures the completion time for each trial, though this was not a performance metric and instead serves as a behavioral descriptive.
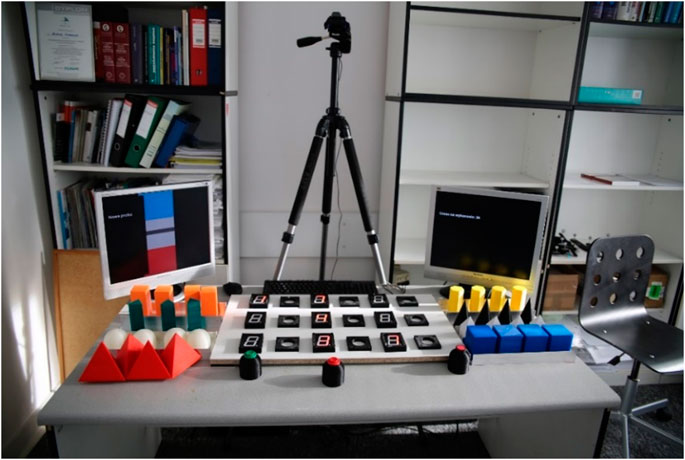
FIGURE 1. Sample setup of the task. Object matrix in the center. Monitor on left displays the secondary task, monitor on right the remaining time or object mapping on request. The button on left corrects secondary task position, middle button ends/begins the trial, and right button displays object-slot mapping.
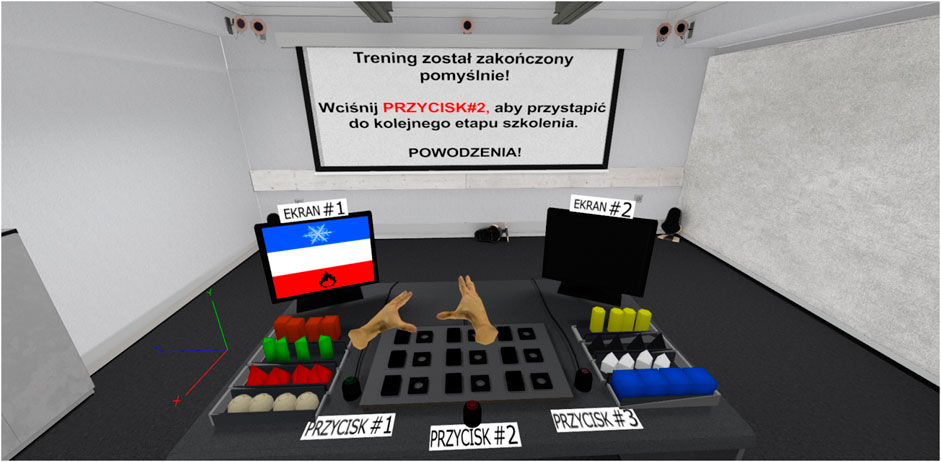
FIGURE 2. Virtual training environment. The elements of the workstation are labeled. Hand avatars are visible in the foreground.
Training Procedures
The non-interactive approach to training that we set to emulate consisted of a training video guiding the viewer through the elements of the workstation and procedures, accompanied by a 4-page long paper brochure repeating in a large extent the information presented in video form. Participants were encouraged to take their time, but could not spend longer than 20 min in total viewing the materials. The video material was presented on the 17-inch laptop screen. No audio track was played, as instructions were presented in the form of captions. The brochure was printed with color images.
The IVR based training was built around the concept of a hands-on approach, encouraging participants to perform the task virtually before the real-world test. The simulation environment and the task elements were created based on 3D scans of the physical elements and 3D computer models prepared previously for printing (see Figure 2). Before training commenced, participants went through technical setup (mounting and adjusting head and hands interface devices) and a 5-min procedure introducing them to functioning in a simulated environment. The training itself guided participants through the individual elements of the workstation, demonstrated their functionality, and ended with a free training session with all elements of the workstation. Participants were again encouraged to take their time, but the whole IVR simulation session lasted no longer than 20 min in total. The environment was created using the Unity 3D real-time graphics engine, presented via a Head Mounted Display (SONY HMZ-T3), and interfaced using custom-made data gloves. No audio track was played, but instructions were presented in the form of slides on a projector screen within the virtual environment.
Experiment Procedure
The experimental procedure took on average two and a half hours to complete, and consisted of the following stages: 1) basic questionnaire, 2) MMSE administration, 3) cognitive tests administration (AX-CPT, OSPAN), 4) mandatory 15-min break, 5) non-interactive or IVR training, 6) post-training test of knowledge and SSQ (in case of IVR training), and 7) real-world task execution. After completion of the study, participants were asked about their experience and afforded a place to rest before leaving.
Results
Questionnaires Data. All older participants scored at least 21 points (out of 30 possible) on the MMSE (M = 22.87, SD = 1.12), with no significant difference between older adults in the two training groups (p = 0.563). One-way ANOVA comparing the four groups (Non-Interactive/IVR x Younger/Older adults) on total SSQ score indicated no significant effect of group (M = 340.17, SD = 333.48), p = 0.507. A Kruskal-Wallis test of self-report VR experience data indicated no significant differences between groups (p = 0.068).
Cognitive Data. Impaired context processing is primarily reflected in the AX-CPT task by accuracy on AY trials, or trials in which the cue A is followed by a non-target letter. Older adults display a counterintuitive decrease in errors on such trials (Rush et al., 2006), theoretically because the impairment of context processing leads to degradation of the response cue (here the letter A), which in turn makes them less likely to false alarm. Unexpectedly, independent samples t-test comparison of our older and younger adults indicated no significant difference in accuracy on AY trials (Younger Adults: M = 85.02%, SD = 12.92; Older Adults: M = 87.64%, SD = 12.41), t(113) = -1.10, p = 0.270. As for working memory capacity (OSPAN task), the expected group differences were found to be significant: t(99) = 8.06, p < 0.001. Younger adults had significantly higher working memory capacity (M = 42.23, SD = 16.72) than did older adults (M = 17.80, SD = 13.42).
Real Task Data. The following behavioral indicators were extracted from the real-world performance task: number of correctly placed objects, trial completion time (average number of seconds needed to complete a block of trials), and the number of secondary task timeouts. Results from the post-training test of knowledge were also included in this analysis.
A RM ANOVA (Greenhouse-Geisser corrected where necessary) was conducted on the average number of correctly placed objects, with difficulty level (1–6) as a within-subjects factor. Training Type (Traditional vs. Virtual Reality) and Age Group (Younger Adults, Older Adults) were the between subject factors. The between-subject comparisons indicated a significant overall effect of Training Type [F(1, 104) = 5.40, p = 0.022, ηp2 = 0.049), a significant overall effect of Age Group [F(1,104) = 21.21, p < 0.001, ηp2 = 0.169, and no significant Training Type x Age Group interaction (p = 0.069). The non-interactive training approach resulted in significantly lower task accuracy than did the virtual reality training (see Figure 3), and younger adults performed significantly better than older adults (see Figure 4). The trend-level Training Type x Age Group interaction indicates that older adults benefited more from IVR training than did younger adults (see Supplementary Figure S5). Although there was an expected main effect of difficulty level [F(5, 434.03, p < 0.001, ηp2 = 0.883), this factor did not interact with Training Type (p = 0.260) or Age Group (p = 0.789), and there was no significant three-way interaction (p = 0.756).
The same analysis was conducted for data on trial time completion. On the between-subject level, there was a significant overall effect of both Training Type [F(1, 114) = 6.73, p = 0.011, ηp2 = 0.056] and Age Group [F(1, 114) = 110.37, p < 0.001, ηp2 = 0.492], with no Training Type x Age Group interaction (p = 0.814). The non-interactive training approach resulted in significantly lower task completion time than did the virtual reality training, and younger adults performed significantly faster than older adults. Difficulty again showed an expected main affect, with more difficult trials taking more time to complete: F(3.96, 452.40) = 62.36, p < 0.001, ηp2 = 0.354. There was no Difficulty x Training Type interaction (p = 0.304), though Difficulty did interact with Age Group significantly: F(3.96, 452.40) = 3.25, p = 0.012, ηp2 = 0.028. This interaction was not based on differences in group performance, and instead consisted of differences in comparisons of difficulty levels within each group. The three-way interaction was non-significant (p = 0.377).
Due to the generally low number of timeouts caused by the secondary task, we decided to sum them across all of the trials into a single variable. Univariate ANOVA revealed the significant effects of both Training Type [F(1, 114) = 7.39, p = 0.008, ηp2 = 0.061] and Age Group [F(1, 114) = 17.87, p < 0.001, ηp2 = 0.136], with no Training Type x Age Group interaction (p = 0.067). IVR trained participants had significantly fewer timeouts than did non-interactive trained participants, and younger adults had significantly fewer timeouts than did older adults. Our test of task knowledge results were analyzed using the model, again resulting in significant main effects of Training Type [F(1, 114) = 5.49, p = 0.021, ηp2 = 0.046] and Age Group [F(1, 114) = 14.60, p < 0.001, ηp2 = 0.114], and no Training Type x Age Group interaction (p = 0.923). IVR trained participants scored significantly higher on the test of knowledge than did non-interactive trained participants (87.63% vs 77.50%, respectively), and younger adults scored significantly higher than did older adults (90.83% vs 74.30%, respectively).
Finally, we repeated the four above analyses while controlling for the factors of context processing performance and working memory capacity. Neither factor was a significant predictor of any of the tested dependent variables. Further, this inclusion did not change the significance of any of the Training Type x Age Group interactions. However, in the case of the average number of correctly placed objects, the main effect of Training Type became non-significant (p = 0.095).
Discussion
The results of our experiment provide empirical evidence for the successful transfer of complex skills learned in an immersive virtual reality training to an analogous real-word task. Participants trained in IVR achieved higher accuracy rates and fewer timeouts at the real “assembly” task than participants trained using non-interactive methods. Further, they were significantly more knowledgeable about the task immediately after training. This finding is expected given the hypothetical dependence of learning transfer on the similarity between the trained task and the actual task, and the properties of the immersive virtual training used here.
However, such near-transfer has only rarely been demonstrated experimentally. Our results are in line with those reported by Rose et al. (2000) in a non-immersive virtual training of a simple sensorimotor task, where skills trained solely in virtual reality task carried over into the real version of that task. The conditions of the experiment itself are most closely matched by the research of Gavish et al. (2015), in which post-training performance on a real maintenance and assembly task was compared after undergoing either VR training or a film demonstration control condition. In contrast to our study, they were unable to find significant performance differences between such conditions.
One unexpected result is that IVR trained participants had longer completion times than the non-interactive group. Previous research has found that VR training of other complex tasks (e.g. Torkington et al., 2001) can result in increased movement speed and greater efficiency. It is apparent from the object placing accuracy data that the task we used was not particularly challenging to our participants, and it seems to be the case that IVR-trained participants were better able to fully utilize their time to increase task accuracy (while maintaining lower rates of timeouts). Our participants were only informed of a 10 s time limit for placing each object; there was no benefit to placing the object significantly faster.
There were several promising findings regarding our different age groups. First of all, our data show no evidence that younger and older adults significantly differed in their ability to benefit from IVR training in comparison to non-interactive training, as demonstrated by the lack of training type x age group interactions in our analyses. Both age groups benefited significantly more from IVR training in their later behavioral performance and in terms of task knowledge. Furthermore, older adults reported similar levels of simulator sickness symptoms to that of younger adults, though it should be mentioned that the overall duration of exposure was quite short (<20 min). Despite the younger adults broadly outperforming the older adults in real task performance, as is expected given the effects of cognitive aging (Salthouse, 2004), it seems that age did not play a strong role in determining the efficacy of IVR training.
We were surprised to find that our expectation of older adults gaining similar benefit from IVR training as younger adults held even without adjusting for context processing or working memory capacity, both of which are hallmarks of cognitive aging (Braver et al., 2001; Braver and West, 2008). The working memory results are typical in that older adults have significantly lower capacity than younger adults, but no significant differences were found in context processing as measured by the AX-CPT. Older adults did have slightly higher accuracy on the representative trials (AY), which would be expected in the case of deficient context processing, but not of the scale found in other studies (e.g. Braver and West, 2008). It may be the case that the task was not difficult enough to strain older participants to an extent that would elicit deficits related to working memory and context processing. However, it should be noted that the main effect of training type (for placement data) became non-significant after correcting for those two factors, which suggests that at least some of the benefit derived from IVR training is related to working memory/context processing ability.
In conclusion, our study shows that IVR training of a complex skill can transfer into a real-world analog, is significantly more effective than video and text-based methods, and can be equally beneficial to both younger and older adults. The latter result is the first comparison of its kind in the current context, and the former two add experimental evidence to a sparse and mixed literature. Together, these results offer a novel experimental contribution to the study IVR-based learning methods. Both age groups were able to adapt to the IVR interface given a short introduction and both groups reported similar levels of simulator sickness, demonstrating that older adults are capable of significant learning even in completely new contexts and through previously unknown interfaces. Further, we did not find any direct evidence that the effectiveness of IVR training was related to the levels of working memory/context processing in our participants. One flaw of our study is the lack of a real-task training group, which would provide the best test of equivalence between VR and real-world training. We encourage future studies to include such control groups where feasible.
Data Availability Statement
The raw data supporting the conclusions of this article will be made available by the authors, without undue reservation.
Ethics Statement
Written informed consent was obtained from the individual(s) for the publication of any potentially identifiable images or data included in this article.
Author Contributions
PD, MS, GP, MM, and AG designed and planned the experiment. MS and MM gathered the data and recruited participants. PD and AG prepared the tasks. PD analyzed the data, and PD and MS wrote the manuscript.
Funding
This work was supported by the National Science Center (grant number 2012/05/N/HS6/04018) and the Ministry of Science and Higher Education (grant number I.P.23/TSB).
Conflict of Interest
The authors declare that the research was conducted in the absence of any commercial or financial relationships that could be construed as a potential conflict of interest.
Supplementary Material
The Supplementary Material for this article can be found online at: https://www.frontiersin.org/articles/10.3389/frvir.2020.604008/full#supplementary-material.
References
Alaker, M., Wynn, G. R., and Arulampalam, T. (2016). Virtual reality training in laparoscopic surgery: a systematic review and meta-analysis. Int. J. Surg. 29, 85–94. doi:10.1016/j.ijsu.2016.03.034
Anderson, N. D., and Craik, F. I. (2017). 50 Years of cognitive aging theory. J. Gerontol. B Psychol. Sci. Soc. Sci. 72, 1–6. doi:10.1093/geronb/gbw108
Appel, L., Appel, E., Bogler, O., Wiseman, M., Cohen, L., Ein, N., et al. (2019). Older adults with cognitive and/or physical impairments can benefit from immersive virtual reality experiences: a feasibility study. Front. Med. 6, 329. doi:10.3389/fmed.2019.00329
Bier, B., Ouellet, É., and Belleville, S. (2018). Computerized attentional training and transfer with virtual reality: effect of age and training type. Neuropsychology. 32, 597–614. doi:10.1037/neu0000417
Bordin, L. H., Kliegel, M., and Martin, M. (2006). Age and planning tasks: the influence of ecological validity. Int. J. Aging Hum. Dev. 62, 175–184. doi:10.2190/EM1W-HAYC-TMLM-WW8X
Bossard, C., Kermarrec, G., Buche, C., and Tisseau, J. (2008). Transfer of learning in virtual environments: a new challenge?. Virtual Real. 12, 151–161. doi:10.1007/s10055-008-0093-y
Braver, T. S., Barch, D. M., Keys, B. A., Carter, C. S., Cohen, J. D., Kaye, J. A., et al. (2001). Context processing in older adults: evidence for a theory relating cognitive control to neurobiology in healthy aging. J. Exp. Psychol. Gen. 130, 746–763. doi:10.1037/0096-3445.130.4.746
Braver, T. S., and West, R. (2008). “Working memory, executive control, and aging.” in The handbook of aging and cognition. 3rd Edn, Editor F. I. M. Craik, and T. A. Salthouse (Hove, East Sussex: Psychology Press), Chap. 7, Vol. 3, 311–372.
Brehmer, Y., Westerberg, H., and Bäckman, L. (2012). Working-memory training in younger and older adults: training gains, transfer, and maintenance. Front. Hum. Neurosci. 6, 63. doi:10.3389/fnhum.2012.00063
Buhr, D. E., Huber, M. E., and Sternad, D. (2019). Learning and transfer of complex motor skills in virtual reality: a perspective review. J. NeuroEng. Rehabil. 16, 121. doi:10.1186/s12984-019-0587-8
Campos, M. E., Torres, W. J., and Beal, D. J. (2020). “Workplace aging and jobs in the twenty-first century,” in Current and emerging trends in aging and work. Editors S. J. Czaja, J. Sharit, and J. B. James (Cham, Switzerland: Springer International Publishing), 13–32. doi:10.1007/978-3-030-24135-3_2
Czaja, S. J., and Sharit, J. (2009). “Preparing organizations and workers for current and future employment: training and retraining,” in In Aging and work: issues and implications in a changing landscape. Editor S. J. Czaja (Baltimore, MD: Johns Hopkins University Press), 259–278.
Folstein, M. F., Folstein, S. E., and McHugh, P. R. (1975). “Mini-mental state”. A practical method for grading the cognitive state of patients for the clinician. J. Psychiatr. Res. 12, 189–198. doi:10.1016/0022-3956(75)90026-6
Garden, S. E., Phillips, L. H., and MacPherson, S. E. (2001). Midlife aging, open-ended planning, and laboratory measures of executive function. Neuropsychology 15, 472–482. doi:10.1037//0894-4105.15.4.472
Gavish, N., Gutiérrez, T., Webel, S., Rodríguez, J., Peveri, M., Bockholt, U., et al. (2015). Evaluating virtual reality and augmented reality training for industrial maintenance and assembly tasks. Interact. Learn. Environ. 23, 778–798. doi:10.1080/10494820.2013.815221
Gurusamy, K., Aggarwal, R., Palanivelu, L., and Davidson, B. R. (2008). Systematic review of randomized controlled trials on the effectiveness of virtual reality training for laparoscopic surgery. Br. J. Surg. 95, 1088–1097. doi:10.1002/bjs.6344
Henry, J. D., MacLeod, M. S., Phillips, L. H., and Crawford, J. R. (2004). A meta-analytic review of prospective memory and aging. Psychol. Aging 19, 27–39. doi:10.1037/0882-7974.19.1.27
Huygelier, H., Schraepen, B., van Ee, R., Vanden Abeele, V., and Gillebert, C. R. (2019). Acceptance of immersive head-mounted virtual reality in older adults. Sci. Rep. 9, 4519. doi:10.1038/s41598-019-41200-6
Jones, H. E., and Conrad, H. S. (1933). The growth and decline of intelligence: a study of a homogeneous group between the ages of ten and sixty. Genet. Psychol. Monogr. 13, 223–298.
Kanfer, R., and Ackerman, P. L. (2004). Aging, adult development, and work motivation. AMRO. 29, 440–458. doi:10.5465/amr.2004.13670969
Kennedy, R. S., Lane, N. E., Berbaum, K. S., and Lilienthal, M. G. (1993). Simulator Sickness Questionnaire: an enhanced method for quantifying simulator sickness. Int. J. Aviat. Psychol. 3, 203–220. doi:10.1207/s15327108ijap0303_3
Lee, M., Son, J., Kim, J., Pyun, S.-B., Eun, S.-D., and Yoon, B. (2016). Comparison of individualized virtual reality- and group-based rehabilitation in older adults with chronic stroke in community settings: a pilot randomized controlled trial. Eur. J. Integr. Med. 8, 738–746. doi:10.1016/j.eujim.2016.08.166
Lehmann, K. S., Ritz, J. P., Maass, H., Cakmak, H. K., Kuehnapfel, U. G., Germer, C. T., et al. (2005). A prospective randomized study to test the transfer of basic psychomotor skills from virtual reality to physical reality in a comparable training setting. Ann. Surg. 241, 442–449. doi:10.1097/01.sla.0000154552.89886.91
Micarelli, A., Viziano, A., Micarelli, B., Augimeri, I., and Alessandrini, M. (2019). Vestibular rehabilitation in older adults with and without mild cognitive impairment: effects of virtual reality using a head-mounted display. Arch. Gerontol. Geriatr. 83, 246–256. doi:10.1016/j.archger.2019.05.008
Molina, K. I., Ricci, N. A., de Moraes, S. A., and Perracini, M. R. (2014). Virtual reality using games for improving physical functioning in older adults: a systematic review. J. NeuroEng. Rehabil. 11, 156. doi:10.1186/1743-0003-11-156
Nagendran, M., Gurusamy, K. S., Aggarwal, R., Loizidou, M., and Davidson, B. R. (2013). Virtual reality training for surgical trainees in laparoscopic surgery, Cochrane Database Syst. Rev. 2013 (8), CD006575. doi:10.1002/14651858.CD006575.pub3
Noack, H., Lövdén, M., and Schmiedek, F. (2014). On the validity and generality of transfer effects in cognitive training research. Psychol. Res. 78, 773–789. doi:10.1007/s00426-014-0564-6
Optale, G., Urgesi, C., Busato, V., Marin, S., Piron, L., Priftis, K., et al. (2010). Controlling memory impairment in elderly adults using virtual reality memory training: a randomized controlled pilot study. Neurorehabilitation Neural Repair. 24, 348–357. doi:10.1177/1545968309353328
Rose, F. D., Attree, E. A., Brooks, B. M., Parslow, D. M., Penn, P. R., and Ambihaipahan, N. (2000). Training in virtual environments: transfer to real world tasks and equivalence to real task training. Ergonomics. 43, 494–511. doi:10.1080/001401300184378
Rush, B. K., Barch, D. M., and Braver, T. S. (2006). Accounting for cognitive aging: context processing, inhibition or processing speed?. Neuropsychol. Dev. Cogn. B Aging Neuropsychol. Cogn. 13, 588–610. doi:10.1080/13825580600680703
Salthouse, T. A. (2004). What and when of cognitive aging, Curr. Dir. Psychol. Sci. 13, 140–144. doi:10.1111/j.0963-7214.2004.00293.x
Salthouse, T. A. (2010). Major issues in cognitive aging. Oxford, England: Oxford University Press 13, 140–144. doi:10.1093/acprof:oso/9780195372151.001.0001
Salthouse, T. A. (2019). Trajectories of normal cognitive aging. Psychol. Aging 34, 17–24. doi:10.1037/pag0000288
Simons, D. J., Boot, W. R., Charness, N., Gathercole, S. E., Chabris, C. F., Hambrick, D. Z., et al. (2016). Do "Brain-Training" programs work?. Psychol. Sci. Publ. Interest 17, 103–186. doi:10.1177/1529100616661983
Stine-Morrow, E. A. L., and Basak, C. (2011). “Chapter 10 - cognitive interventions,” in Handbook of the psychology of aging. 7th Edn, Editors K. W. Schaie, and S. L. Willis (San Diego: Academic Press), 153–171. doi:10.1016/B978-0-12-380882-0.00010-3
Stothard, P., Laurence, D., and Squelch, A. (2015). “Interactive virtual reality simulation--A tool for improving understanding of safety and environmental risk relating to sustainable mining practices,” in Africa Australia technical mining conference 2015, Adelaide, Australia, June 11–12, 2015, 139–144.
Thorndike, E. L. (1919). A standardized group examination of intelligence independent of language. J. Appl. Psychol. 3, 13–32. doi:10.1037/h0070037
Thorndike, E. L. (1906). The principles of teaching: based on psychology, Dietikon, Switzerland: AG Seiler. doi:10.1037/11487-000
Torkington, J., Smith, S. G., Rees, B. I., and Darzi, A. (2001). Skill transfer from virtual reality to a real laparoscopic task. Surg. Endosc. 15, 1076–1079. doi:10.1007/s004640000233
Unsworth, N., Heitz, R. P., Schrock, J. C., and Engle, R. W. (2005). An automated version of the operation span task. Behav. Res. Methods 37, 498–505. doi:10.3758/bf03192720
Westerfield, G., Mitrovic, A., and Billinghurst, M. (2015). Intelligent augmented reality training for motherboard assembly. Int. J. Artif. Intell. Educ. 25, 157–172. doi:10.1007/s40593-014-0032-x
Keywords: immersive virtual reality, transfer, skill training, work training, cognitive aging
Citation: Dobrowolski P, Skorko M, Pochwatko G, Myśliwiec M and Grabowski A (2021) Immersive Virtual Reality and Complex Skill Learning: Transfer Effects After Training in Younger and Older Adults. Front. Virtual Real. 1:604008. doi: 10.3389/frvir.2020.604008
Received: 08 September 2020; Accepted: 08 December 2020;
Published: 27 January 2021.
Edited by:
Michael Madary, University of the Pacific, United StatesReviewed by:
Marcos Serrano, Université Toulouse III Paul Sabatier, FranceJoan Llobera, University of Barcelona, Spain
Copyright © 2021 Dobrowolski, Skorko, Pochwatko, Myśliwiec and Grabowski.. This is an open-access article distributed under the terms of the Creative Commons Attribution License (CC BY). The use, distribution or reproduction in other forums is permitted, provided the original author(s) and the copyright owner(s) are credited and that the original publication in this journal is cited, in accordance with accepted academic practice. No use, distribution or reproduction is permitted which does not comply with these terms.
*Correspondence: Paweł Dobrowolski, pawel.dobrowolski@psych.pan.pl