- 1Neuroscience and Experimental Virtual Reality Lab, Graduate Program in Rehabilitation Sciences, Federal University of Health Sciences of Porto Alegre (UFCSPA), Porto Alegre, Brazil
- 2KITE, Toronto Rehabilitation Institute, University Health Network, Toronto, ON, Canada
- 3Department of Psychology, Federal University of Health Sciences of Porto Alegre (UFCSPA), Porto Alegre, Brazil
Parkinson's disease (PD) is a neurodegenerative condition that is often associated with movement impairments, such as reduced balance and gait control. Virtual reality (VR) is a promising intervention for enhancing rehabilitation efficiency and may assist in overcoming functional limitations imposed by the disability. The objective of this study was to evaluate the feasibility, safety, and efficacy of a 5-weeks VR intervention in increasing mobility smoothness in participants with PD. Participants were assessed using functional mobility tasks—Timed Up and Go (TUG) and 10-m walking test (10MWT) and cognitive function tests. A total of 23 participants with a diagnosis of PD were randomly assigned to groups of VR or control interventions. In the VR group, for each session, participants received a VR-based balance training, i.e., tightrope simulator, for 20 min, preceded by 8 min of total body warm-up (total of 10 sessions over 5 weeks). Participants in the control group received 20 min of psychoeducation without a structured exercise program (twice a week). All screened participants engaged and completed a total of 10 VR-based intervention sessions. VR-based balance training caused no significant improvement in mobility smoothness for TUG or 10MWT (p > 0.05). Similarly, cognitive function was not affected by the VR intervention (p > 0.05). The outcomes of this study suggest that 10–20 min of VR-based intervention is a feasible and safe rehabilitation activity. However, it was insufficient to promote improvement in mobility smoothness and cognitive function in participants with PD. A combination of task-specific training in the virtual and physical environments in a more intensive approach is warranted for future study designs.
Introduction
Parkinson's disease is one of the most common neurodegenerative disorders worldwide (Bellou et al., 2016), which is associated with motor and cognitive impairments. Such motor deficits may negatively impact balance, gait, and movement quality (Fox et al., 2018), and non-motor manifestations (e.g., depression, loss of motivation/initiation, and anxiety), ultimately affecting normal function and quality of life (Rizos et al., 2014; Weiss et al., 2014). Parkinson's disease (PD) progression and response to rehabilitation are based on assessments of motor and non-motor symptoms, such as gait and mood disorders, respectively (Kassubek, 2014). Motor impairments of gait involve slower walking velocity, reduced stride length, and reduced cadence and proportion of swing time to stance time (Sofuwa et al., 2005; Mirek et al., 2016; Shah et al., 2018). Gait training in the environment of the clinical setting may lack real-life challenges or novelty, reducing the transfer to real-life situations.
Virtual reality (VR) is a relatively new technology used by researchers to explore novel and optimized rehabilitation and training strategies (Imam and Jarus, 2014; Weiss et al., 2014; Flores et al., 2018). In such VR-based rehabilitation, patients are immersed in interactive scenarios mimicking real-world environments, and this immersion may offer a more straightforward transfer from the rehabilitation to activities of daily living (Rose et al., 2000; Schultheis and Rizzo, 2001). VR has been used for rehabilitation following stroke (Souza Barbosa de et al., 2011; Imam and Jarus, 2014), cerebral palsy (Ravi et al., 2017), and several neurodegenerative disorders (Dockx et al., 2016; Doniger et al., 2018; Maggio et al., 2019), including PD.
Although multiple studies have reported positive results of VR on gait and balance (Ghai and Ghai, 2019; Kim et al., 2019; Maggio et al., 2019), there is still limited evidence of improvements in balance for persons with PD (van den Heuvel et al., 2014; Liao et al., 2015; Wang et al., 2019).
The addition of VR-based training may help in motivating and providing gait rehabilitation in other contexts. An optimal VR-based balance rehabilitation protocol should challenge individuals with PD to maintain balance during walking, with potential implications to challenging tasks (e.g., turns). However, the feasibility of providing such VR-based training in persons with PD is unknown. Another consideration is the use of functional mobility tests to mimic real-life situations, such as the Timed Up and Go (TUG), which may increase the sensitivity to detect effects of rehabilitation (Stack et al., 2004; Mancini et al., 2012, 2018; El-Gohary et al., 2013). Usually, TUG and 10-m walking test (10MWT) assessments are based on the total time to complete the task. However, motor control changes in balance may go undetected if these traditional task duration measurements are employed, given the lack of a movement quality metric (Pinto et al., 2019). The use of sensor-based performance analysis has attracted attention over the past few years, especially given the lightweight, portable nature that makes inertial sensors a useful tool to be implemented in clinical context with an ecological approach (Patel et al., 2020). Studies suggest that sensor-based motor performance assessments provide objective, fast, reliable, and sensitive measurements of mobility (Salarian et al., 2010; Mancini et al., 2018; Miller Koop et al., 2018). Here, we used a wearable sensor [i.e., inertial measurement unit (IMU)] to assess movement quality in persons with PD over a VR-based balance training. Aligned with this technology, we opted to use state-of-the-art movement smoothness metrics to quantify movement quality, the spectral arc length (SPARC) (Balasubramanian et al., 2012, 2015; Beck et al., 2018). SPARC has essential advantages in evaluating movement smoothness if compared to other metrics, such as the number of velocity peaks (Gulde and Hermsdörfer, 2018) or jerk (Hogan and Sternad, 2009; Balasubramanian et al., 2012). In a previous study, we used an IMU system to evaluate and quantify mobility smoothness of persons with PD and freezing of gait (FOG) during a functional mobility test (Pinto et al., 2019). We found that participants with PD and FOG presented reduced smoothness compared to healthy controls.
Interestingly, not only motor deficits contribute to FOG and fall risk, but cognitive dysfunction may also contribute to such episodes (Weiss et al., 2014; Walton et al., 2018). Specifically, executive function (i.e., working memory, planning, and inhibition) and attention are critical for mobility. Frequently, persons with PD present cognitive dysfunction that affects the ability to appropriately respond to complex situations, hindering the capacity to walk efficiently and safely (Peterson et al., 2016). Given the cognitive and mobility profiles of individuals with PD, it may be useful to develop targeted rehabilitation strategies to improve both cognitive function and functional mobility in this population.
A VR-based intervention challenging balance should induce changes of motor control related to maintenance of function during gait and turning, which would be captured if movement quality outcomes are employed. Here, in this study, we report on the safety, feasibility, and efficacy of using a 5-weeks VR-based intervention in promoting changes in mobility smoothness in participants with PD. We hypothesized that VR-based intervention focused on balance activity would improve the movement quality and non-motor performance of participants with PD. To this end, we evaluated mobility smoothness during the performance of TUG and 10MWT tests at baseline and following 5 weeks of VR-based intervention. We also described non-motor performance using the State–Trait Anxiety Inventory (STAI) and Rivermead Behavioral Memory Test, third edition (RBMT-3) for accounting for possible cognitive improvement with the VR-based intervention. The bulk of our findings provides essential information for future work in terms of estimating sample size and adherence to VR interventions and may assist in designing randomized clinical trials.
Methods
Participants and Recruitment
This study was a double-blinded randomized controlled trial conducted from January 2017 until November 2017 in the Movement Analysis and Rehabilitation Laboratory at the Federal University of Health Sciences of Porto Alegre (UFCSPA). Volunteers were recruited through a database of the neurology service of Santa Casa de Misericordia Hospital of Porto Alegre. Individuals preidentified as meeting our inclusion criteria were informed about our study over phone calls. During this initial contact, the study coordinator explained details of the research and prescreened against eligibility criteria. Ethical approval for this study was received from the UFCSPA Research Ethics Committee (approval number 1.972.062). Written informed consent was obtained from all the participants before randomization. The study was powered based on previous data using a VR-based balance intervention; nonetheless, the primary mobility outcome did not encompass movement quality, but task duration—often used to score the TUG test in the clinical setting (Liao et al., 2015).
To be considered eligible, participants must have received a diagnosis of idiopathic PD (according to the London Brain Bank Criteria) and be able to understand the study requirements, to communicate sufficiently and ambulate independently. Individuals with a previous history of other neurological or orthopedic diseases and severe vision deficits that would interfere with participation in the study were excluded. Twenty-three participants with PD were randomly assigned to either the intervention group (PD VR, n = 11) or the control group (PD control n = 12). The general characteristics of the participants are listed in Table 1. Participants allocated in the control group kept their regular physical activities (walking, stretching exercises) and received no VR or other physical therapy intervention. Additionally, they received, twice a week, a 20-min session of psychoeducation delivered by researchers involved in the present study—on topics relevant to PD (Walton et al., 2018) including cognition, sleep, mood, fall risk, and rehabilitation. This approach was used to improve self-management, providing the knowledge and skills to better manage the symptoms and modifiable risk factors of their condition (Jones and Riazi, 2011).
Assessment and Intervention
All participants underwent two assessments: (1) pre-intervention (baseline) measures collected at study enrollment and before randomization and (2) post-intervention, after 5 weeks of control or VR-based intervention. Both assessments were performed by a physiotherapist blinded to group allocation. Additionally, at the pre-intervention visit, all participants underwent psychological/cognitive and motor assessments, such as Mini-Mental State Examination (MMSE), STAI, RBMT-3, motor part of the Unified Parkinson's Disease Rating Scale (UPDRS III), Hoehn & Yahr stage (H&Y), and self-reported physical activity status [International Physical Activity Questionnaire (IPAQ)] (Figure 1B).
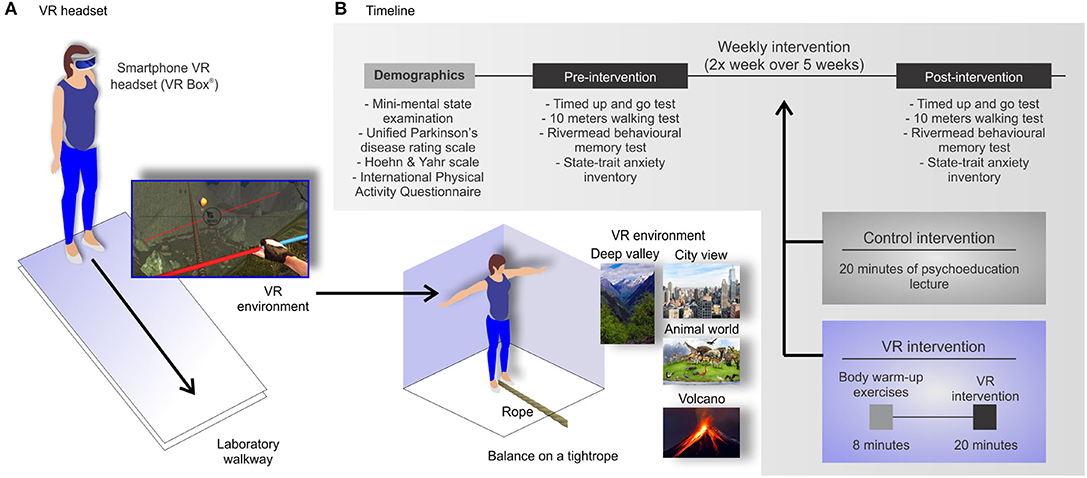
Figure 1. Virtual reality (VR)-based intervention. (A) The VR Box® smartphone headset and laboratory environment. In the VR environment, participants had to tilt the head left and right during walking over four different scenarios. (B) Experiment timeline: all participants underwent pre-intervention (baseline) and post-intervention assessments, after 5 weeks of control or VR-based intervention.
The virtual environment was a tightrope simulator (The Rope Crossing Adventure VR 1.8 for IOS), using a smartphone VR headset (VR Box©). In this VR task, participants had to balance on a tightrope walking over four different scenarios (deep valley, city view, animal world, and volcano, Figure 1A). The rope crossing in the virtual environment required participants to tilt head left and right during walking. First, participants had a familiarization session with the VR headset and were instructed on the movements required to perform the task. During the VR experience, participants were standing and were allowed to walk on the laboratory walkway. The evaluator walked on the participants' side to prevent loss of balance and eventual falls. The TUG, 10MWT, RBMT-3, and STAI were assessed before the VR intervention (pre-intervention) and after the 10th session of intervention (post-intervention) (Figure 1B). All assessments and interventions were performed during the on-medication phase. Participants from the intervention group received 8 min of total body warm-up exercises and 20 min of VR training (in each session) twice a week for 5 weeks.
Outcome Measures
Two primary outcomes were recorded: (1) functional mobility and (2) gait speed, both assessed using a wearable device. The Bluetooth-compatible commercial IMU system (BTS G-Walk BTS Bioengineering Corporation, Italy) was attached to the participants' waist with a semielastic belt. For the TUG test, participants were asked to rise from sitting on a standard chair, walk 3 m at a self-selected speed, turn around a cone, walk back to the chair, and sit down (Salarian et al., 2010). The test initiated when the evaluator said “go” and ended when the participant sat down with the back resting (Figure 2A). For 10MWT, participants were instructed to walk at a self-selected speed (comfortable) on a flat surface with 10 m (Figure 3A) (Batten et al., 2019). Raw acceleration data from the IMU system were extracted using the G-sensor® software and exported in ASCII format. Participants performed the tests mentioned above three times, and the total time (s) and speed (m/s) were recorded. The average of three trials was used in the analysis.
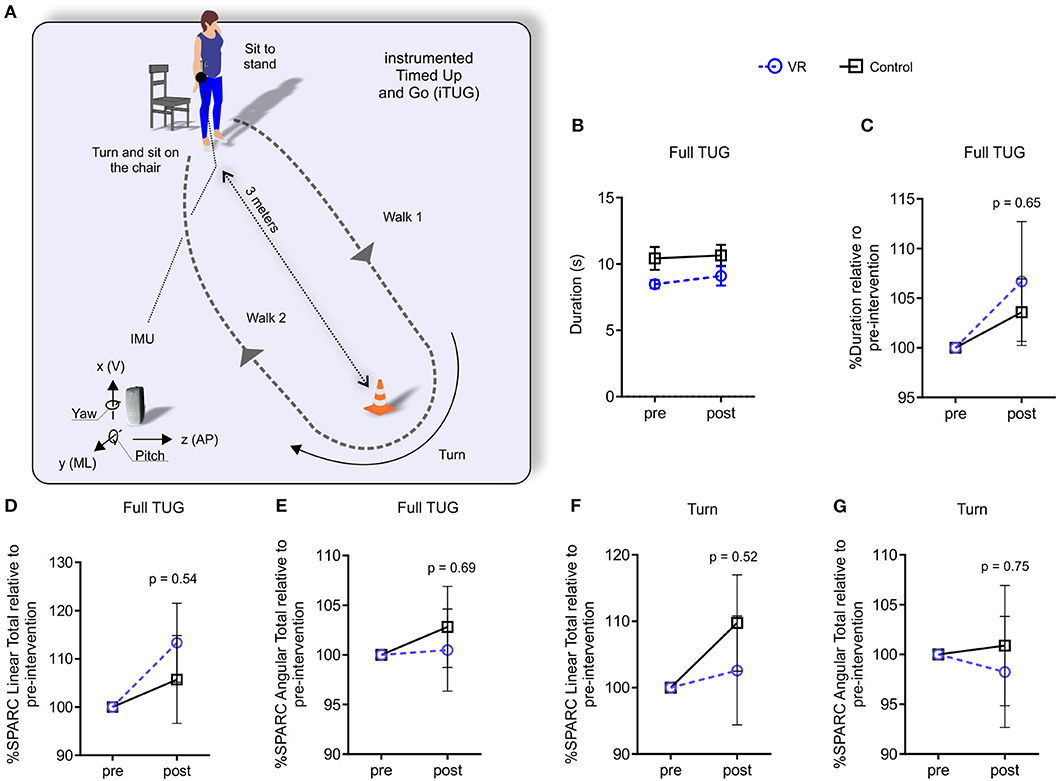
Figure 2. VR-based intervention did not improve performance or movement quality in the TUG test. (A) The TUG test was performed using standard protocols; participants were wearing an IMU unit to record movement quality data. (B,C) Treatment did not improve test performance or (D–G) movement quality (SPARC). Data are expressed as mean ± SEM. Unpaired t-tests (PD VR, n = 11, PD control, n = 12). TUG, Timed Up and Go; IMU, inertial measurement unit; SPARC, spectral arc length; VR, virtual reality.
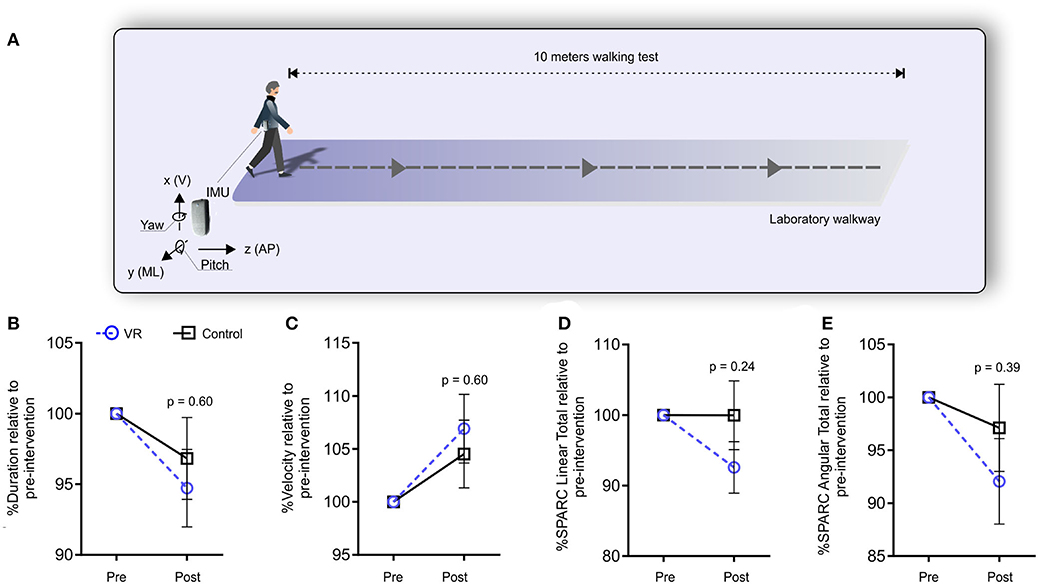
Figure 3. VR-based intervention did not improve performance or movement quality in the 10-m walking test (10MWT). (A) The 10MWT test was performed using standard protocols; participants were wearing an IMU unit to record movement quality data. (B,C) Treatment did not significantly improve test performance or (D,E) movement quality (SPARC). Data are expressed as mean ± SEM. Unpaired t-tests (PD VR, n = 11, PD control, n = 12). IMU, inertial measurement unit; SPARC, spectral arc length; VR, virtual reality.
Secondary outcome measures focused on psychological assessments, including STAI and RBMT-3. STAI measures two different components of anxiety: state and trait, with 20 items, each one answered on a 4-point Likert scale (Fioravanti-Bastos et al., 2011). RBMT-3 includes 14 subtests assessing aspects of visual, verbal, recall, recognition, and immediate and delayed everyday memory (Koltai et al., 1996; Wilson et al., 2008). Here, we used the general memory index to represent the overall memory performance.
Data Analysis
The signals acquired by the IMU system were the following: three linear acceleration axes, vertical acceleration (V), mediolateral acceleration (ML), anterior–posterior acceleration (AP); three angular velocity axes, vertical angular velocity (V), mediolateral angular velocity (ML), anterior–posterior angular velocity (AP); and three angles, yaw, pitch, and roll used to detect the TUG phases—following a methodology described by Pinto et al. (2019) and Figueiredo et al. (2020). The signals were recorded at a sampling frequency of 100 Hz and later transferred to a personal computer for offline analysis. LabVIEW® 8.5 custom software routines were developed to analyze the data. We used the movement smoothness based on the spectral arc length, described as SPARC elsewhere (Balasubramanian et al., 2015; Beck et al., 2018; Pinto et al., 2019). We calculated the average SPARC from three TUG trials and three 10MWT trials. SPARC was calculated for full TUG and also for the individual phases of TUG, such as (i) sit-to-stand, (ii) walk 1, (iii) turn, (iv) walk 2, and (v) turn and stand-to-sit (Pinto et al., 2019). For each phase of the TUG test, the linear accelerations and angular velocities were summed to yield the SPARC linear total and SPARC angular total, respectively (Beck et al., 2018). As previously described (Beck et al., 2018; Gulde and Hermsdörfer, 2018), SPARC metrics follow the assumption that less smooth movements are more complex in terms of their frequency composition. Therefore, less movement smoothness indicates lower SPARC values.
Feasibility was measured using the adherence of participants to complete 10 sessions of 20 min of VR intervention. Safety was assessed by participants' reports of adverse events, such as cybersickness, dizziness, nausea, or falls in each session. Efficacy was evaluated by an improvement of smoothness metrics before and after a 5-weeks VR-based intervention. All the above-mentioned events were recorded in an intervention logs worksheet every session.
Statistical Analysis
Descriptive statistics were used to report sample characteristics. Repeated-measures two-way ANOVAs were used to compare groups (time: pre-intervention × post-intervention; group: VR × control) followed by post-hoc using the Sidak correction when appropriate. The first exploratory data analysis revealed that VR and control groups were discrepant in the baseline outcomes for all evaluated variables. Therefore, data were reported as the proportional change relative to pre-intervention (% pre-intervention). Data were then tested for mean comparisons using unpaired t-tests (PD VR vs. PD control). A correlation analysis, Pearson moment product, was performed between the clinical scores (UPDRS-III, H&Y, STAI, RBMT-3) and quantitative metrics to determine if a significant relationship existed. As we had a small sample size for this feasibility study, the smallest real differences (SRD) were calculated for the control group, according to Beckerman et al. (2001) and Zariffa et al. (2018). This analysis was conducted to understand the measurement error and the ability of SPARC to capture subtle rehabilitation effects. For the SRD calculation, an ANOVA was conducted with the control participants modeled as a random variable and the pre- and post-interventions as a time variable (fixed variable). The pre-intervention time point was duplicated to allow ANOVA calculations. The within-participant variance was obtained from the sum-of-squares results of the one-way ANOVA (within-subject variance). SRD was computed according to Equation (1).
Overall, data were expressed as the mean and standard error of the mean (SEM); the significance level was set at α < 0.05. Data were analyzed using GraphPad Prism® version 6.01 (GraphPad Software, San Diego, CA, USA) and LabVIEW® (National Instruments, Austin, TX, USA).
Results
We had 30 eligible participants who participated in the pre-intervention (baseline) assessments. However, seven of them declined to participate due to problems to commute twice a week to the University or time constraints. A total of 23 individuals with PD participated in the study. In terms of the feasibility of the intervention, there was no withdraw across the study duration. Overall, participants in the intervention group tolerated well 20 min of VR exercise; none of the participants reported any adverse events (cybersickness, dizziness, nausea, or falls). No significant differences were found in demographics and clinical characteristics after stratification in groups (Table 1).
All variables extracted from the TUG test showed the absence of time, group, or interaction effects (Supplementary Figures 2E–I and Supplementary Table 3). After correcting for baseline performance, the results also indicated no significant difference between groups for all TUG variables (Figures 2B–G). We also investigated mobility smoothness—SPARC—in individual subtasks of the TUG test and, similarly, report a lack of differences between groups (Supplementary Table 1).
Significant time and group effects were evident in the 10MWT duration, indicating that walking duration decreased across time; i.e., both groups walked faster at the post-intervention time point (Supplementary Figures 2A,B, Supplementary Table 3). For SPARC metrics, we found a group effect only for SPARC linear total, where VR participants presented greater smoothness compared to the control group (Supplementary Figure 2C, Supplementary Table 3). Nevertheless, when correcting for baseline performance, there was no significant difference in 10MWT duration (PD VR, 94.72% ± 2.7 and PD control, 96.83% ± 2.8, p = 0.60, Figure 3B) or velocity (PD VR, 106.9% ± 3.23 and PD control, 104.5% ± 3.19, p = 0.60, Figure 3C). Similarly, linear and angular SPARC values for the 10MWT were not significantly different at post-intervention; only small trends were evident. These trends indicated movement quality improvement with the VR-based intervention (linear, 92.57% ± 3.65 and 99.97% ± 4.8, respectively, p = 0.24 and angular, 92.06% ± 4.03 and 97.11% ± 4.11, p = 0.39, Figures 3D, E).
We found moderate correlations between SPARC parameters and UPDRS III, H&Y, STAI, and RBMT-3 (Supplementary Table 2). There were consistent negative correlations between the sit to stand TUG phase with UPDRS III and H&Y (r = −0.50, p = 0.02 and r = −0.45, p = 0.03; respectively), indicating that a less smooth movement is associated with disease severity. On the other hand, SPARC during the 10MWT moderately correlated with cognitive function parameters (STAI-T: r = 0.45, p = 0.03; RBMT-3: r = 0.41, p < 0.05). This positive correlation suggests that participants with lower trait–anxious profile had a better movement quality outcome.
Discussion
This study investigated the feasibility, safety, and efficacy of a short-term VR-based balance intervention. We hypothesized the VR-based intervention could improve mobility smoothness (SPARC) and cognitive function in participants with PD. All participants were evaluated during the on-medication phase, and data were compared with the post-intervention time point. Adherence to the VR-based intervention program was high, and no adverse events were reported. The 5-weeks VR-based intervention was not able to induce any significant improvement in the mobility smoothness. In addition, no significant differences were found between groups on the secondary outcomes related to psychological/cognitive assessments. Nevertheless, this short-term VR-based intervention was safe, feasible, and well-accepted by the participants.
Our results show the first evidence of SPARC measurements after a VR-based intervention in participants with PD. The overall SPARC metrics were not affected by the intervention in either walk or TUG tasks. SPARC magnitude was similar to previously reported elsewhere for participants with PD (Beck et al., 2018; Pinto et al., 2019). Moreover, we found some moderate correlations between questionnaires and SPARC values in specific cases, such as arising from a chair with UPDRS III and H&Y, turn and stand to sit with H&Y and STAI-state and the turn phase of the TUG test. These findings also corroborate our previous study in which correlations also involved the turn phase of the TUG test (Pinto et al., 2019).
Turning is a challenging task for PD persons and considered a trigger for FOG and falls risk (El-Gohary et al., 2013). Hence, the quality of turning movement (i.e., smoothness) has been a target for rehabilitation assessment and intervention (Mancini et al., 2018). This critical aspect of turning is also reflected in the present study by the negative correlation between mobility smoothness while turning and STAI-state, relating higher anxiety behavior with a less smooth turning movement (Supplementary Table 2). Given this crucial role, we suggest that the turn phase of the TUG test should be better explored in future studies.
Previous studies indicated VR-based training benefits on balance (Lee et al., 2015; Liao et al., 2015; Wang et al., 2019), executive, and visuospatial (Maggio et al., 2018) abilities of participants with PD. Nevertheless, the effects on gait velocity and distance are less evident (Wang et al., 2019). The positive effects of VR-based training were also observed in combined intervention protocols, for example, VR and treadmill training (Liao et al., 2015) or VR combined with neurodevelopment treatment and functional electrical stimulation (Lee et al., 2015). Importantly, these above-mentioned studies reported VR-based interventions lasting from 20 to 100 min, for 4–12 weeks, and the frequency of interventions ranging from 2 to 5 days/week—of higher intensity and duration compared to the present study. Here, we used a 20-min VR-based balance training twice a week for 5 weeks. Another important aspect is the combination of task-specific training in the virtual and physical environments, for example, by providing balance training using obstacle crossing (Liao et al., 2015). This combination strategy may increase task complexity and optimize functional balance improvements; these factors may have accounted for the findings of the present study.
Thus, the lessons learned for further study design should consider the evaluation of movement quality following a task-specific VR-based balance training, including additions to the physical environment, to enhance retention and facilitate transfer to the tasks in the physical environment (Birkenmeier et al., 2010; Imam and Jarus, 2014; Weiss et al., 2014). Another consideration of our VR intervention also includes the low-tech VR equipment used in the present study (i.e., smartphone-based headset). This VR headset relies on built-in sensors of the smartphone and may not provide the most accurate spatial tracking—of importance for balance training. The rope crossing in the virtual environment required participants to tilt the head left and right during walking. In this case, visual–motor localization discrepancy could affect balance training effectiveness (Fuchs, 2017). To this end, more sophisticated headset systems, i.e., with 6 degrees of freedom, high frame rate, and low latency, could be a better solution to improve balance training. Moreover, a custom VR environment can be developed to progressively incorporate advanced mobility functions, such as obstacle crossing, dynamic balance, breaks, and turns during the VR-based rehabilitation program. Performance scores can be added to the game/activity; thus, working to achieve a goal may enhance attention and concentration in therapy, increasing the efficacy of rehabilitation intervention (Weiss et al., 2014). Finally, a broader recruitment strategy using posters, word-of-mouth, and recruitment cards placed at collaborating organizations may add to the representation of the study population.
Another essential methodological consideration for future studies is the performance of additional baseline assessments to account for measurement errors [e.g., using the smallest real difference (SRD) approach]. Understanding the measurement error is important in follow-up studies since it can hamper the detection of small motor control changes with VR-based interventions, similar to post-stroke robotic rehabilitation (Zariffa et al., 2018). Here, we performed the SRD calculation to understand how the measurement error could have affected our findings (Supplementary Figure 1). Indeed, the outcome with lower SRD, SPARC linear total during the 10MWT, displayed the most robust trend toward a positive VR-based intervention effect. SPARC has been first introduced to analyze point-to-point reach using kinematics (Balasubramanian et al., 2012). This metric has been adapted to IMUs to assess gait; studies have shown a less smooth walking and mobility pattern in PD compared to healthy control participants, indicating that SPARC is sensitive to PD movement impairments (Beck et al., 2018; Pinto et al., 2019). Here, we showed preliminary results on the effects of short-term VR training on SPARC metrics. Future studies may employ repeated baseline measurements to understand the between-day variability and the SRD of the proposed approach. This understanding will advance the use of IMUs and SPARC to provide reliable and sensitive outcomes in studying motor changes following rehabilitation, especially using VR-based interventions.
VR-based interventions can be a valuable tool in providing new experiences to improve mobility and balance control. Another aspect strengthening the VR application in the clinical settings is related to program adherence, which may ultimately facilitate better clinical outcomes compared to traditional rehabilitation programs (Adamovich et al., 2009). We highlight the feasibility of using VR technology as an additional rehabilitative resource to be combined with another motor (and non-motor) interventions, which are potentially beneficial to improve functional mobility and to reduce the incidence of falls in PD.
Limitations of the present study include the following: (i) the control group maintained their regular activities, such as walking and stretching exercises, which could have affected the comparison of movement quality outcomes; (ii) the lack of measurement other than questionnaires to better distinguish motor impairments and stratify PD participants homogeneously; (iii) the sample size calculation was based on traditional outcomes (i.e., task duration); future studies should consider SPARC metrics to attend the specific variances; and (iv) inclusion of safety harness during VR intervention to prevent falls and fear of falling.
Conclusion
A short-term VR-based balance training is a safe and feasible intervention but did not improve movement quality and cognitive function in participants with PD. SPARC is a reliable measurement to estimate mobility smoothness; it correlated with motor and non-motor clinical assessments and showed the ability to quantify small real differences. The adherence to the program was high, and no adverse events were reported. Based on our findings, we recommend immersive VR training for persons with PD; however, we emphasize the need for a combination of task-specific training in the virtual and physical environments to optimize functional mobility gains.
Data Availability Statement
The raw data supporting the conclusions of this article will be made available by the authors, without undue reservation.
Ethics Statement
The studies involving human participants were reviewed and approved by Federal University of Health Sciences of Porto Alegre (UFCSPA) Research Ethics Committee (approval number 1.972.062). The patients/participants provided their written informed consent to participate in this study.
Author Contributions
MB and AO contributed to the conception or design of the work. MB and AG contributed to data acquisition. CS and GB analyzed the data, wrote the first draft of the manuscript, and contributed to the interpretation of data. GB was responsible for the figures. CS, GB, MB, AG, and AO revised the manuscript for final submission. All authors approved the version to be published.
Funding
This work was supported by CAPES—Coordenação de Aperfeiçoamento de Pessoal de Nível Superior, Brazil.
Conflict of Interest
The authors declare that the research was conducted in the absence of any commercial or financial relationships that could be construed as a potential conflict of interest.
Supplementary Material
The Supplementary Material for this article can be found online at: https://www.frontiersin.org/articles/10.3389/frvir.2020.00007/full#supplementary-material
References
Adamovich, S. V., Fluet, G. G., Tunik, E., and Merians, A. S. (2009). Sensorimotor training in VR: a review. Neuro Rehabil. 25, 1–21. doi: 10.3233/NRE-2009-0497
Balasubramanian, S., Melendez-Calderon, A., and Burdet, E. (2012). A robust and sensitive metric for quantifying movement smoothness. IEEE Trans. Biomed. Eng. 59, 2126–2136. doi: 10.1109/TBME.2011.2179545
Balasubramanian, S., Melendez-Calderon, A., Roby-Brami, A., and Burdet, E. (2015). On the analysis of movement smoothness. J. Neuroeng. Rehabil. 12, 1–11. doi: 10.1186/s12984-015-0090-9
Batten, H. R., McPhail, S. M., Mandrusiak, A. M., Varghese, P. N., and Kuys, S. S. (2019). Gait speed as an indicator of prosthetic walking potential following lower limb amputation. Prosthet. Orthot. Int. 43, 196–203. doi: 10.1177/0309364618792723
Beck, Y., Herman, T., Brozgol, M., Giladi, N., Mirelman, A., and Hausdorff, J. M. (2018). SPARC: a new approach to quantifying gait smoothness in patients with Parkinson's disease. J. Neuroeng. Rehabil. 15, 1–9. doi: 10.1186/s12984-018-0398-3
Beckerman, H., Roebroeck, M. E., Lankhorst, G. J., Becher, J. G., Bezemer, P. D., and Verbeek, A. L. (2001). Smallest real difference, a link between reproducibility and responsiveness. Qual. Life Res. 10, 571–578. doi: 10.1023/A:1013138911638
Bellou, V., Belbasis, L., Tzoulaki, I., Evangelou, E., and Ioannidis, J. P. (2016). Environmental risk factors and Parkinson's disease: an umbrella review of meta-analyses. Parkinsonism Relat. Disord. 23, 1–9. doi: 10.1016/j.parkreldis.2015.12.008
Birkenmeier, R. L., Prager, E. M., and Lang, C. E. (2010). Translating animal doses of task-specific training to people with chronic stroke in 1-hour therapy sessions: a proof-of-concept study. Neurorehabil. Neural Repair. 24, 620–635. doi: 10.1177/1545968310361957
Dockx, K., Bekkers, E. M., Van den Bergh, V., Ginis, P., Rochester, L., Hausdorff, J. M., et al. (2016). Virtual reality for rehabilitation in Parkinson's disease. Cochrane Database Syst. Rev. 12:CD010760. doi: 10.1002/14651858.CD010760.pub2
Doniger, G. M., Beeri, M. S., Bahar-Fuchs, A., Gottlieb, A., Tkachov, A., Kenan, H., et al. (2018). Virtual reality-based cognitive-motor training for middle-aged adults at high alzheimer's disease risk: a randomized controlled trial. Alzheimers Dement. 4, 118–129. doi: 10.1016/j.trci.2018.02.005
El-Gohary, M., Pearson, S., McNames, J., Mancini, M., Horak, F., Mellone, S., et al. (2013). Continuous monitoring of turning in patients with movement disability. Sensors 14, 356–369. doi: 10.3390/s140100356
Figueiredo, A. I., Balbinot, G., Brauner, F. O., Schiavo, A., Baptista, R. R., Pagnussat, A. S., et al. (2020). SPARC metrics provide mobility smoothness assessment in oldest-old with and without a history of falls: a case control study. Front. Physiol. 11:540. doi: 10.3389/fphys.2020.00540
Fioravanti-Bastos, A. C. M., Cheniaux, E., and Landeira-Fernandez, J. (2011). Development and validation of a short-form version of the brazilian state-trait anxiety inventory. Psicol. Reflexão Crít. 24, 485–494. doi: 10.1590/S0102-79722011000300009
Flores, A., Linehan, M. M., Todd, S. R., and Hoffman, H. G. (2018). The use of virtual reality to facilitate mindfulness skills training in dialectical behavioral therapy for spinal cord injury: a case study. Front. Psychol. 9:531. doi: 10.3389/fpsyg.2018.00531
Fox, S. H., Katzenschlager, R., Lim, S. Y., Barton, B., de Bie, R. M. A., Seppi, K., et al. (2018). International Parkinson and movement disorder society evidence-based medicine review: update on treatments for the motor symptoms of Parkinson's disease. Mov. Disord. 33, 1248–1266. doi: 10.1002/mds.27372
Ghai, S., and Ghai, I. (2019). Virtual reality enhances gait in cerebral palsy: a training dose-response meta-analysis. Front. Neurol. 10:236. doi: 10.3389/fneur.2019.00236
Gulde, P., and Hermsdörfer, J. (2018). Smoothness metrics in complex movement tasks. Front. Neurol. 9:615. doi: 10.3389/fneur.2018.00615
Hogan, N., and Sternad, D. (2009). Sensitivity of smoothness measures to movement duration, amplitude, and arrests. J. Mot. Behav. 41, 529–534. doi: 10.3200/35-09-004-RC
Imam, B., and Jarus, T. (2014). Virtual reality rehabilitation from social cognitive and motor learning theoretical perspectives in stroke population. Rehabil. Res. Pract. 2014:594540. doi: 10.1155/2014/594540
Jones, F., and Riazi, A. (2011). Self-efficacy and self-management after stroke: a systematic review. Disabil. Rehabil. 33, 797–810. doi: 10.3109/09638288.2010.511415
Kassubek, J. (2014). Diagnostic procedures during the course of Parkinson's disease. Basal Ganglia 4, 15–18. doi: 10.1016/j.baga.2014.02.001
Kim, O., Pang, Y., and Kim, J.-H. (2019). The effectiveness of virtual reality for people with mild cognitive impairment or dementia: a meta-analysis. BMC Psychiatry 19:219. doi: 10.1186/s12888-019-2180-x
Koltai, D. C., Bowler, R. M., and Bowler, R. M. (1996). The rivermead behavioural memory test and wechsler memory scale-revised: relationship to everyday memory impairment. Assessment 3, 443–448. doi: 10.1177/107319119600300410
Lee, N. Y., Lee, D. K., and Song, H. S. (2015). Effect of virtual reality dance exercise on the balance, activities of daily living, and depressive disorder status of Parkinson's disease patients. J. Phys. Ther. Sci. 27, 145–147. doi: 10.1589/jpts.27.145
Liao, Y. Y., Yang, Y. R., Cheng, S. J., Wu, Y. R., Fuh, J. L., and Wang, R. Y. (2015). Virtual reality-based training to improve obstacle-crossing performance and dynamic balance in patients with Parkinson's disease. Neurorehabil. Neural Repair. 29, 658–667. doi: 10.1177/1545968314562111
Maggio, M. G., De Cola, M. C., Latella, D., Maresca, G., Finocchiaro, C., La Rosa, G., et al. (2018). What about the role of virtual reality in Parkinson disease's cognitive rehabilitation? Preliminary findings from a randomized clinical trial. J. Geriatr. Psychiatry Neurol. 31, 312–318. doi: 10.1177/0891988718807973
Maggio, M. G., Russo, M., Cuzzola, M. F., Destro, M., La Rosa, G., Molonia, F., et al. (2019). Virtual reality in multiple sclerosis rehabilitation: a review on cognitive and motor outcomes. J. Clin. Neurosci. 65, 106–111. doi: 10.1016/j.jocn.2019.03.017
Mancini, M., Priest, K. C., Nutt, J. G., and Horak, F. B. (2012). “Quantifying freezing of gait in Parkinson's disease during the instrumented timed up and go test. Conf. Proc. IEEE Eng. Med. Biol. Soc. 23, 1198–1201. doi: 10.1109/EMBC.2012.6346151
Mancini, M., Weiss, A., Herman, T., and Hausdorff, J. M. (2018). Turn around freezing: community-living turning behavior in people with Parkinson's disease. Front. Neurol. 9:18. doi: 10.3389/fneur.2018.00018
Miller Koop, M., Ozinga, S. J., Rosenfeldt, A. B., and Alberts, J. L. (2018). Quantifying turning behavior and gait in Parkinson's disease using mobile technology. IBRO Rep. 5, 10–16. doi: 10.1016/j.ibror.2018.06.002
Mirek, E., Kubica, J. L., Szymura, J., Pasiut, S., Rudzinska, M., and Chwala, W. (2016). Assessment of gait therapy effectiveness in patients with Parkinson's disease on the basis of three-dimensional movement analysis. Front. Neurol. 7:102. doi: 10.3389/fneur.2016.00102
Patel, M., Pavic, A., and Goodwin, V. A. (2020). Wearable inertial sensors to measure gait and posture characteristic differences in older adult fallers and non-fallers: a scoping review. Gait Posture 76, 110–121. doi: 10.1016/j.gaitpost.2019.10.039
Peterson, D. S., King, L. A., Cohen, R. G., and Horak, F. B. (2016). Cognitive contributions to freezing of gait in Parkinson disease: implications for physical rehabilitation. Phys. Ther. 96, 659–670. doi: 10.2522/ptj.20140603
Pinto, C., Schuch, C. P., Balbinot, G., Salazar, A. P., Hennig, E. M., Kleiner, A. F. R., et al. (2019). Movement smoothness during a functional mobility task in subjects with Parkinson's disease and freezing of gait–an analysis using inertial measurement units. J. Neuroeng. Rehabil. 16:110. doi: 10.1186/s12984-019-0579-8
Ravi, D. K., Kumar, N., and Singhi, P. (2017). Effectiveness of virtual reality rehabilitation for children and adolescents with cerebral palsy: an updated evidence-based systematic review. Physiotherapy 103, 245–258. doi: 10.1016/j.physio.2016.08.004
Rizos, A., Martinez-Martin, P., Odin, P., Antonini, A., Kessel, B., Kozul, T. K., et al. (2014). Characterizing motor and non-motor aspects of early-morning off periods in Parkinson's disease: an international multicenter study. Parkinsonism Relat. Disord. 20, 1231–1235. doi: 10.1016/j.parkreldis.2014.09.013
Rose, F. D., Attree, E. A., Brooks, B. M., Parslow, D. M., Penn, P. R., and Ambihaipahan, N. (2000). Training in virtual environments: transfer to real world tasks and equivalence to real task training. Ergonomics 43, 494–511. doi: 10.1080/001401300184378
Salarian, A., Horak, F. B., Zampieri, C., Carlson-Kuhta, P., Nutt, J. G., and Aminian, K. (2010). ITUG, a sensitive and reliable measure of mobility. IEEE Trans. Neural Syst. Rehabil. Eng. 18, 303–310. doi: 10.1109/TNSRE.2010.2047606
Schultheis, M. T., and Rizzo, A. (2001). The application of virtual reality technology in rehabilitation. Rehabil. Psychol. 46, 296–311. doi: 10.1037/0090-5550.46.3.296
Shah, J., Pillai, L., Williams, D. K., Doerhoff, S. M., Larson-Prior, L., Garcia-Rill, E., et al. (2018). Increased foot strike variability in Parkinson's disease patients with freezing of gait. Parkinsonism Relat. Disord. 53, 58–63. doi: 10.1016/j.parkreldis.2018.04.032
Sofuwa, O., Nieuwboer, A., Desloovere, K., Willems, A. M., Chavret, F., and Jonkers, I. (2005). Quantitative gait analysis in Parkinson's disease: comparison with a healthy control group. Arch. Phys. Med. Rehabil. 86, 1007–1013. doi: 10.1016/j.apmr.2004.08.012
Souza Barbosa de, L., da Rosa Paino Paim, C., Imamura, M., and Alfieri, F. M. (2011). Use of interactive video game for stroke rehabilitation. Acta Fisiátrica 18, 217–221. doi: 10.5935/0104-7795.20110010
Stack, E., Jupp, K., and Ashburn, A. (2004). Developing methods to evaluate how people with Parkinson's disease turn 180°: an activity frequently associated with falls. Disabil. Rehabil. 26, 478–484. doi: 10.1080/09638280410001663085
van den Heuvel, M. R., Kwakkel, G., Beek, P. J., Berendse, H. W., Daffertshofer, A., and van Wegen, E. E. (2014). Effects of augmented visual feedback during balance training in Parkinson's disease: a pilot randomized clinical trial. Parkinsonism Relat. Disord. 20, 1352–1358. doi: 10.1016/j.parkreldis.2014.09.022
Walton, C. C., Mowszowski, L., Gilat, M., Hall, J. M., O'Callaghan, C., Muller, A. J., et al. (2018). Cognitive training for freezing of gait in Parkinson's disease: a randomized controlled trial. NPJ Parkinson's Dis. 4:15. doi: 10.1038/s41531-018-0052-6
Wang, B., Shen, M., Wang, Y. X., He, Z. W., Chi, S. Q., and Yang, Z. H. (2019). Effect of virtual reality on balance and gait ability in patients with Parkinson's disease: a systematic review and meta-analysis. Clin. Rehabil. 33, 1130–1138. doi: 10.1177/0269215519843174
Weiss, P. L. T., Keshner, E. A., and Levin, M. F. (2014). Virtual Reality for Physical and Motor Rehabilitation. New York, NY: Springer New York.
Wilson, B. A., Greenfield, E., Clare, L., Baddeley, A., Cockburn, J., Watson, P., et al. (2008). The Rivermead Behavioural Memory Test. 3rd Edn. London: Pearson Education.
Keywords: Parkinson's disease, mobility, smoothness, virtual reality, cognitive function, spectral arc length
Citation: Schuch CP, Balbinot G, Bonilla MN, Guedes Machado A and de Oliveira AA (2020) Feasibility of a Short-Term Virtual Reality Balance Intervention to Improve Mobility Smoothness in Parkinson's Disease. Front. Virtual Real. 1:7. doi: 10.3389/frvir.2020.00007
Received: 15 April 2020; Accepted: 30 June 2020;
Published: 26 August 2020.
Edited by:
David Joseph Brown, Nottingham Trent University, United KingdomReviewed by:
P. J. Standen, University of Nottingham, United KingdomPhilip Breedon, Nottingham Trent University, United Kingdom
James Lewis, Nottingham Trent University, United Kingdom
Copyright © 2020 Schuch, Balbinot, Bonilla, Guedes Machado and Oliveira. This is an open-access article distributed under the terms of the Creative Commons Attribution License (CC BY). The use, distribution or reproduction in other forums is permitted, provided the original author(s) and the copyright owner(s) are credited and that the original publication in this journal is cited, in accordance with accepted academic practice. No use, distribution or reproduction is permitted which does not comply with these terms.
*Correspondence: Clarissa Pedrini Schuch, clarissa.schuch84@gmail.com; clarissaps@ufcspa.edu.br