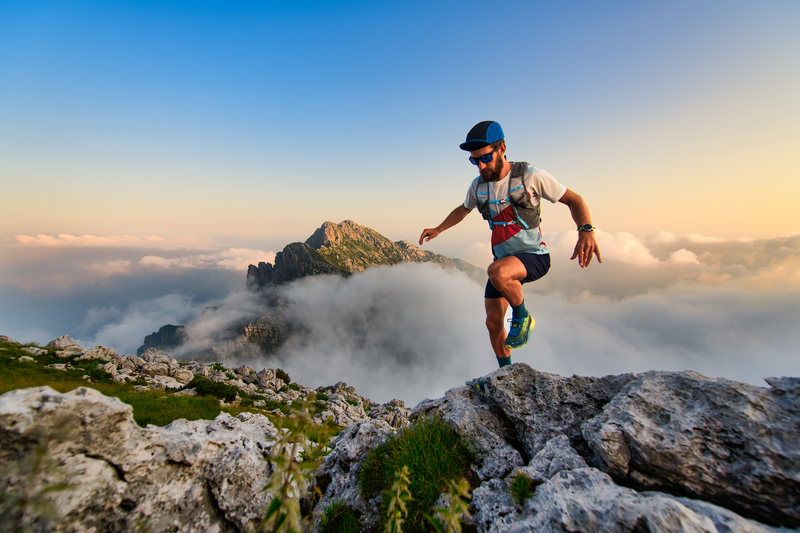
95% of researchers rate our articles as excellent or good
Learn more about the work of our research integrity team to safeguard the quality of each article we publish.
Find out more
MINI REVIEW article
Front. Transplant. , 24 November 2023
Sec. Transplantation Immunology
Volume 2 - 2023 | https://doi.org/10.3389/frtra.2023.1286881
This article is part of the Research Topic Precision Therapeutics Using Next Generation Technologies in Transplantation View all 7 articles
Scarcity of high-quality organs, suboptimal organ quality assessment, unsatisfactory pre-implantation procedures, and poor long-term organ and patient survival are the main challenges currently faced by the solid organ transplant (SOT) field. New biomarkers for assessing graft quality pre-implantation, detecting, and predicting graft injury, rejection, dysfunction, and survival are critical to provide clinicians with invaluable prediction tools and guidance for personalized patients' treatment. Additionally, new therapeutic targets are also needed to reduce injury and rejection and improve transplant outcomes. Proteins, which underlie phenotypes, are ideal candidate biomarkers of health and disease statuses and therapeutic targets. A protein can exist in different molecular forms, called proteoforms. As the function of a protein depends on its exact composition, proteoforms can offer a more accurate basis for connection to complex phenotypes than protein from which they derive. Mass spectrometry-based proteomics has been largely used in SOT research for identification of candidate biomarkers and therapeutic intervention targets by so-called “bottom-up” proteomics (BUP). However, such BUP approaches analyze small peptides in lieu of intact proteins and provide incomplete information on the exact molecular composition of the proteins of interest. In contrast, “Top-down” proteomics (TDP), which analyze intact proteins retaining proteoform-level information, have been only recently adopted in transplantation studies and already led to the identification of promising proteoforms as biomarkers for organ rejection and dysfunction. We anticipate that the use of top-down strategies in combination with new technological advancements in single-cell and spatial proteomics could drive future breakthroughs in biomarker and therapeutic target discovery in SOT.
Solid organ transplantation (SOT) is the standard-of-care treatment for patients with end-stage organ disease (1). Despite considerable progresses made in the field to improve transplant outcomes, there are still significant challenges that must be overcome, including scarcity of high-quality organs suitable for transplant, suboptimal assessment of organ quality pre-transplantation, and unsatisfactory long-term organ and patient survival (2, 3).
Most grafts derive from brain-dead (BD) donors. However, their usage results in higher rejection rates and worse transplant outcomes compared to those obtained from living donors that are less damaged in pre-implantation (4–6). Organs are subjected to damage from the pre- and peri-implantation procedures (i.e., Ischemia reperfusion injury, IRI) that can trigger higher recipient immune response (7, 8). Higher immunoactivation increases the risk of T-cell and antibody mediated rejections (TCMR and ABMR), graft dysfunction, and graft loss (9–12). While immunosuppressive (IS) therapies are used to prevent and treat rejection by dampening the recipient immune system, those cause toxicity and make the recipient more susceptible to infections, malignancies, and graft injury resulting in diminished graft survival (13) (Figure 1A).
Figure 1. Translational MS-based proteomics for identifying novel biomarkers and therapeutic targets in SOT research. (A) Overview of mechanisms contributing to SOT outcomes. (B) Workflow of translational bottom-up and top-down MS-based proteomics approaches in SOT.
Therefore, to increase the amount and quality of donor supply, avoid rejection, and improve long-term transplant outcomes, it is crucial to identify accurate and reliable biomarkers to (1) assess donor organ quality pre-implantation, (2) early detect immunoactivation and rejection, (3) monitor rejection and disease progression and the response of patients to immunotherapies. In addition, the transplant field lacks prognostic biomarkers that can predict (1) transplant outcomes before implantation based on organ quality, (2) graft rejection, dysfunction, and failure before their occurrence, and (3) the response of patients to immunosuppression. Such biomarkers could guide allocation, decision-making, patient risk stratification, and personalized treatments (14).
Mechanisms behind graft injury and rejection are not well understood. Their characterization is fundamental for developing new therapeutic strategies aimed to minimize injury due to graft origin, IRI and its sequelae to improve graft quality, function, and survival. More targeted graft pre-treatment would be particularly beneficial to expand the pool of organs suitable for transplant and improve long-term graft survival.
Proteins are directly responsible for the function, structure, and regulation of cells, tissues, and organs (15–17). Therefore, the identification and characterization of proteins involved in injury and rejection is crucial to advance transplant research.
Mass spectrometry (MS)-based proteomics can elucidate biological processes in depth (18, 19) and has been extensively used to analyze the protein composition of a variety of clinical samples from transplant recipients (20). There are two general approaches for performing MS-based proteomics analysis: Bottom-up (BUP) and Top-down proteomics (TDP). In BUP, proteins are digested into peptides prior to MS analysis, while in TDP, the analysis is conducted directly at intact protein level (Figure 1B) (21, 22). Here, we discuss the application of both approaches in SOT research.
BUP is the strategy traditionally used for proteomics studies (23–25). In BUP (Figure 2A), proteins are extracted from the clinical sample, enriched by immunoprecipitation or fractionation when needed, and digested with trypsin into peptides (26). Peptides are then seperated by liquid chromatography (LC) or capillary electrophoresis (CE), ionized by electrospray ionization (ESI) (27), and injected into the mass spectrometer for producing precursor ion (MS1) spectra. Next, individual peptides are fragmented via higher-energy collisional dissociation (HCD) or collision-induced dissociation (CID) to obtain the fragment ion (MS2) (28) spectrum in either untargeted or targeted mode. In untargeted mode, fragmentation is performed based on top-intensity peptides or MS1 mass-to-charge ratio (m/z)-selected ranges. In targeted mode, fragmentation targets are specific peptides with known sequence and m/z information. Data from MS1 and MS2 spectra is combined and used to sequence peptides, infer proteins, identify posttranslational modifications (PTMs), and quantify peptides/proteins present in the sample using a variety of computational engines (29–32). Aside from label-free quantitative approach that relies on the intensities of the peptides obtained in MS1 (33), labeling approaches are commonly used in BUP for relative and absolute quantitation. In particular, Tandem Mass Tags (TMT) and Isobaric tags (iTRAQ), use multiplex isobaric tags to label peptides and base the quantitation analysis on the intensity of reporter ions from the tag found in MS2 spectra (34, 35).
Figure 2. BUP and TDP strategies for biomarker and therapeutic target identification. Workflows of BUP (A), TDP (B) and spatial proteomics (C) using mass spectrometry imaging approaches.
Most BUP studies aimed at identifying biomarkers to detect, monitor, and predict acute rejection, TCMR, ABMR, injury, and dysfunction while uncovering mechanisms behind injury and rejection to find novel therapeutic targets. Such studies have been conducted both on solid tissue biopsies (gold standard in the field but invasive), and the less invasive body fluids, including urine, blood, bronchoalveolar lavage (BAL) fluid, and perfusates. Importantly, the combined analysis of different sample types can help elucidating pathophysiological scenarios. For example, solid tissue biopsies can inform on the outset of the disease process (i.e., immune cell infiltration and allograft injury), while body fluids on the presence of circulating blood immunological proteins (i.e., antibodies) migrating into the site of injury (36) or proteins secreted from the allograft in the urine (37).
Kidney is the most transplanted organ (38). Given its physiological role, urine has been largely investigated for biomarker discovery (39). Sigdel et al. identified urine protein panels able to distinguish different conditions after kidney transplant, including acute rejection (AR), chronic allograft nephropathy, BK virus nephritis, and stable grafts (40). Other studies found potential urinary biomarkers for diagnosing acute tubulointerstitial renal allograft rejection (41) and chronic allograft nephropathy (i.e., beta-2-microglobulin) (42, 43); exosomal tetraspanin-1 and hemopexin for early detection of TCMR (44); collagen peptides and matrix metalloproteinase-8 as reporters for clinical and subclinical TCMR (45); matrix metalloproteinase-8 as indicator of renal allograft inflammation and injury (46); and a 10-protein panel distinguishing ABMR vs. no-ABMR (47).
BUP analysis of plasma, serum, and peripheral blood mononuclear cells (PBMCs) led to the identification of (1) several proteins with AR diagnostic value, including properdin, keratin 1, titin, kininogen-1, and lipopolysaccharide-binding protein (48, 49); (2) serum aminoacylase-1 as a potential diagnostic biomarker of delayed graft function (DGF) severity with moderate outcome predictive power (50); and (3) protein profiles with high predictive accuracies of Chronic Allograft Nephropathy severity (51).
BUP analysis of kidney biopsies identified signatures distinguishing healthy from injured tissues caused by TCMR or polyomavirus BK nephropathy (52); differentially expressed proteins in chronic rejection, including the alpha-1 chain of collagen type IV and Integrin alpha-1; and several prognostic candidates of fibrosis (53). Additionally, combining laser microdissection of glomeruli from biopsies with BUP has allowed for determining proteome profiles of active and chronic active ABMR and identified three potential ABMR biomarkers (54). Finally, various studies were conducted on tissue biopsies and perfusates of kidney from living or deceased donors after IRI, preservation, and DGF to elucidate injury mechanism and guide the development of improved organ preservation strategies to elevate graft quality (55–60).
Liver is the second most transplanted organ (38). Liver transplant BUP research mainly focused on mechanisms of IRI and acute rejection. Studies on biopsies showed that IRI alters proteins involved in lipid and energy metabolism, metabolic pathways, redox signaling, oxidative-stress response, and cytoskeleton remodeling and indicated Ras GTPase-activating-like protein IQGAP1 and Cytoplasmic protein NCK1 as potential targets to reduce IRI (61, 62).
The comparison of global proteomes of liver tissues from acute cellular rejection (ACR) and non-ACR transplant recipients showed that proteins in ACR were mainly involved in immune response and inflammation while those downregulated implicated in metabolism dysfunction. Heme oxygenase-1 was indicated as a candidate biomarker for ACR (63). Another study found 41 differential expressed proteins in sera of ACR patients, including complement component 4q and 1q that could also predict ACR (64).
BUP studies in lung transplantation focused on determining biomarkers and mechanisms of chronic lung allograft dysfunction (CLAD), a major contributor to poor long-term transplant outcomes. BAL fluid analysis showed that the ratio of Clara cell protein to lysozyme has diagnostic power (65). In addition, the analysis of BAL from CLAD patients and controls by parallel reaction monitoring (PRM) assays targeting Angiotensin II-regulated proteins showed that these assays could diagnose patients with graft inflammation and predict chronic graft dysfunction (66).
In heart transplantation, BUP sought to identify potential diagnostic and prognostic biomarkers of AR and graft dysfunction in blood. It was found that (1) a set of exosomal serum proteins could distinguish acute ACR and ABMR from no rejection patients (67); (2) BD alters plasma proteome causing alterations in several cellular processes, including coagulation and gluconeogenesis among others; (3) expression levels of lysine-specific demethylase 3A and Kallikrein (KLKB1) could predict graft dysfunction, and those of myosin Va and proteasome activator subunit 2 acute rejection (68). The potential outcome predictive value of KLKB1 was also suggested in serum macrovesicles by others (69).
Protein complexity is much broader than the amino acid sequence determined by the genetic code. A single gene can produce different forms of proteins called “proteoforms” deriving from genetic variation, alternative splicing, and PTMs (70). Different proteoforms can have different functions and contribute to different phenotypes. Therefore, they can be more accurate biomarkers of health and disease statuses and therapeutic targets than proteins and their identification advance SOT research (71, 72).
Several examples corroborate this hypothesis: (1) proteoform-level information of histones, which are DNA binding proteins regulating gene expression through combinations of PTMs (73), correlate better to the biological outcome than the overall abundance of histones or individual sites (74–77); (2) proteoforms better distinguish cell types than proteins in PBMCs (78); (3) serum apolipoproteins A-I and A-II proteoforms specifically associate with cardiometabolic indices while no significant differences were detected at protein level (79); and (4) proteoforms of KRAS have distinct signaling functions in colorectal cancer (80).
TDP is able to thoroughly characterize proteoforms informing on exact mass, unambiguous isoform assignment, abundance, and high-value PTM information (i.e., stoichiometry, types, and sites). While BUP identifies and quantifies protein groups with great sensitivity and dynamic range, it has a big limitation in PTMs and proteoform identification due to the inaccurate “inference” of proteoform from peptide-level data (81). An example is illustrated in Figure 2A. If a hypothetical protein has three phosphorylation sites on different tryptic peptides, BUP can neither determine the proteoform composition of the original protein group, whether all three phosphorylations occur together on one proteoform or a mixture of mono- to triple-phosphorylated proteoforms or quantify their stoichiometries. TDP overcomes this issue by analyzing intact proteins. In TDP, which is often coupled with LC, proteoforms are separated and directly ionized into mass spectrometers where the intact mass spectra (MS1) are collected, and ions further fragmented (MS2) for sequencing and PTM site identification (Figure 2B). Further advantages and limitations of TDP and BUP were reviewed elsewhere (20, 82).
TDP has been applied in transplantation studies aimed at identifying novel proteoform diagnostic and prognostic biomarkers of graft rejection and dysfunction. A TDP study conducted on PBMCs from 10 transplant recipients with and without acute rejection (AR) identified and quantified 3,000 unique proteoforms (83). Of these, 111 showed significant differential expression in the two conditions, including stress-associated ER protein 1 and 60S ribosomal protein L35a (84, 85).
Another study analyzed PBMCs from a small cohort of liver-transplanted patients to identify biomarkers of different transplant outcome: transplant excellence (TX), acute dysfunction with no rejection (ADNR), and AR. The analysis revealed 82 differentially expressed proteoforms and indicated that the most significant variations were associated with chemokine/cytokine signaling and cytoskeletal regulation (86).
In a follow-up “discovery mode” TDP study conducted on a larger single-center cohort (n = 75), 61 differentially expressed proteoforms were identified (78). Validation was conducted on patients from another multicenter cohort using a “targeted” method with narrower MS1 scan window focusing on the 61 proteoforms of interest. Among these proteoforms, 24 were confirmed to be biomarker candidates for liver-transplant dysfunction or rejection. For more stringent validation, Huang et al. recently described the proteoform reaction monitoring (PfRM) workflow, which resembles the BUP targeted MS2 quantification. In PfRM, the selected 24 proteoforms were fragmented and the resulted ions used for a more sensitive and specific quantification to femtomolar magnitude (87). We anticipate that future studies will likely validate these proteoforms on a larger scale.
TDP was also used in heart allograft evaluation studies. Zhang et al. identified the phosphorylation of cardiac troponin I (cTnI) as a candidate biomarker for chronic heart failure (CHF). Specifically, they found that the relative abundances of total phosphorylated cTnI forms in postmortem heart tissues decreased from those with normal cardiac function to end-stage CHF. Similar studies conducted on heart transplant tissues revealed a significant loss of phosphorylation on cTnI Ser22/23 sites in end-stage failing hearts compared to those from non-failing donors (88). Profiling of other proteoform targets in heart tissues are currently ongoing (89–91). Notably, while only a few studies applied TDP to transplant research, TDP has shown promising translational values in other clinical areas (92) and is expected to make a similar impact in the transplantation field in the future (93).
TDP has several technical challenges that need to be overcome to reach its full potential. One challenge is associated with limitations in the size of the protein analytes due to difficulties in separation (94, 95). Drown et al. recently demonstrated that coupling capillary zone electrophoresis (CZE) with TDP further increases proteoform identification by 1.7-fold across five different tissue types compared to using LCMS only (96). This technological improvement could be applied to graft evaluation in the future. Another TDP challenge is the overlapping of different species, charge states and isotopic distributions in the limited m/z space of mass spectra. To address this, Kafader et al. developed Individual Ion Mass Spectrometry (I2MS) that was recently used for analyzing antibody repertoires against SARS-CoV-2 in COVID-19 patients and vaccinated individuals (97–99).
MS-based proteomics is a promising approach for SOT biomarker and therapeutic target discovery by providing global profiling and quantitative readout of proteoform changes (20, 100). However, current proteomic workflows in SOT research focus on the analysis of bulk clinical samples. Those fall short in reporting proteomic changes in specific cell types and populations and lose information on the spatial origin of the cells in solid tissues.
Protein distributions and changes in tissues and cells have been traditionally probed by antibody-based approaches in SOT research (101–103). These studies have been focused on proteins with prior knowledge and have limitations in the discovery of novel protein-based biomarkers. In recent years, emerging spatial and single cell proteomics technologies have enabled the discovery of protein signatures specific to functional tissue units, cellular neighborhoods, and cell types. As infiltrating immune cell populations are heterogeneous in allograft rejection, single cell-resolved proteome measurement is crucial to elucidate transplant rejection pathology and targeted cell therapy (104). BUP-based single cell proteomic (SCP) technologies such as SCoPE-MS and NANOPOTS have enabled the characterization of single cells in heterogeneous cell populations (105, 106). These techniques emerged from advances in high-throughput cell isolation, preparation, and automated small volume cell processing. By interfacing isobaric tag-based high-sensitivity LC-MS workflows, thousands of proteins from single cells were quantified, opening new avenues for SOT protein biomarker discovery (107–114) (Figure 2A). In one example applied to organ transplantation research, despite not single cells, Clotet-Freixas et al. collected glomerular and tubulointerstitial compartments of transplant kidney biopsies using laser capture microdissection that identified protein signatures responsible for extracellular matrix remodeling in antibody-mediated kidney allograft rejection (115). However, despite significant advances in SCP, current technologies have limitations in experimental throughput and rare cell profiling, and proteoform-level measurement is yet to be achieved.
Spatial mapping of proteins directly in SOT biopsies preserves the origin of cells of interest and their surrounding microenvironment (116). Immunostaining-based multiplexed protein imaging techniques have enabled dozens of proteins to be imaged simultaneously in a tissue section. For example, Imaging Mass Cytometry (IMC) (117) has been applied to kidney imaging and discovered spatial heterogeneity of immune cells in cortex and medulla and a rare subset of proximal tubule cell population representing regenerating cells (118). Despite significant advances in the number of imaged proteins, these techniques rely on pre-defined antibody panels for proteins with prior knowledge. Imaging multiple protein markers in one tissue sample in solid organ transplant biopsies significantly benefits SOT research where availability and quantity of tissue biopsies are extremely limited. Recently, protein and proteoform imaging has been enabled by spatially resolved MS imaging (MSI) approaches. Aside from MSI workflows utilizing spatial tissue sampling coupled to LC-MS BUP proteomics (119), laser and liquid extraction-based scanning MS imaging probes have enabled spatial mapping of dozens to hundreds of intact proteins and proteoforms (120–122). Specifically, liquid sampling probes (nano-DESI) coupled with single molecule MS detection have allowed imaging of intact proteoforms and native protein complexes directly from tissue sections in a recently introduced technique called proteoform imaging MS (PiMS) (114, 123–127) (Figure 2C). In the first report, PiMS detected ∼400 proteoforms from healthy human kidney tissues with many of them showing localizations to different kidney tissue compartments and cellular neighborhoods. These techniques can be readily adapted to tissue biopsies in SOT research for protein and proteoform biomarker discovery that were previously obscured by bulk measurements.
Although several promising biomarkers of graft injury and rejection have been identified (14, 128, 129), robust diagnostic and prognostic are still unavailable. In addition, there are no efficacious treatments to block injury and rejection.
Proteins are the direct drivers of biological functions and phenotypes, therefore, represent ideal biomarkers and druggable targets. New advances in MS-based proteomics have enabled the analysis of proteomes at unprecedented levels of granularity by increasing the proteome coverage and providing information at proteoform, single-cell and spatial resolution. Such information could elucidate mechanisms underlying injury and rejection and lead to novel biomarkers and therapeutic strategies. However, technical, and economic challenges still prevent the transition of these new technologies from discovery to clinical applications (20, 93, 130).
Finally, the adoption of a multi-omics approach integrating data generated by those powerful proteomics technologies, genomics, transcriptomics, and metabolomics will help to unveil mechanisms linking genotype and phenotype in SOT and drive the identification of multi-biomolecules biomarker signatures, the development of new therapeutics, and the advancement of precise medicine (131).
C-FH: Conceptualization, Investigation, Visualization, Writing – original draft, Writing – review & editing. PS: Conceptualization, Investigation, Visualization, Writing – original draft, Writing – review & editing. TF: Investigation, Writing – review & editing. JL: Conceptualization, Writing – review & editing. NK: Conceptualization, Funding acquisition, Supervision, Writing – review & editing. EF: Conceptualization, Funding acquisition, Investigation, Project administration, Supervision, Visualization, Writing – original draft, Writing—review & editing.
The author(s) declare financial support was received for the research, authorship, and/or publication of this article.
This study was supported by the National Institute of General Medical Sciences of the National Institutes of Health under P41GM108569 awarded to NK. The content is solely the responsibility of the authors and does not necessarily represent the official views of the National Institutes of Health. Additional funding was received from Northwestern Medicine Dr. Michael M. Abecassis Transplant Innovation Endowment Grant (NK and EF).
The authors declare the following competing financial interest: NLK is involved in entrepreneurial activities in top-down proteomics and consults for Thermo Fisher Scientific.
The remaining authors declare that the research was conducted in the absence of any commercial or financial relationships that could be construed as a potential conflict of interest.
All claims expressed in this article are solely those of the authors and do not necessarily represent those of their affiliated organizations, or those of the publisher, the editors and the reviewers. Any product that may be evaluated in this article, or claim that may be made by its manufacturer, is not guaranteed or endorsed by the publisher.
1. Abecassis M, Bartlett ST, Collins AJ, Davis CL, Delmonico FL, Friedewald JJ, et al. Kidney transplantation as primary therapy for end-stage renal disease: a national kidney foundation/kidney disease outcomes quality initiative (NKF/KDOQITM) conference. Clin J Am Soc Nephrol. (2008) 3(2):471–80. doi: 10.2215/CJN.05021107
2. Tucker EL, Smith AR, Daskin MS, Schapiro H, Cottrell SM, Gendron ES, et al. Life and expectations post-kidney transplant: a qualitative analysis of patient responses. BMC Nephrol. (2019) 20(1):175. doi: 10.1186/s12882-019-1368-0
3. Giwa S, Lewis JK, Alvarez L, Langer R, Roth AE, Church GM, et al. The promise of organ and tissue preservation to transform medicine. Nat Biotechnol. (2017) 35(6):530–42. doi: 10.1038/nbt.3889
4. Floerchinger B, Oberhuber R, Tullius SG. Effects of brain death on organ quality and transplant outcome. Transplant Rev (Orlando). (2012) 26(2):54–9. doi: 10.1016/j.trre.2011.10.001
5. Saidi RF, Elias N, Kawai T, Hertl M, Farrell ML, Goes N, et al. Outcome of kidney transplantation using expanded criteria donors and donation after cardiac death kidneys: realities and costs. Am J Transplant. (2007) 7(12):2769–74. doi: 10.1111/j.1600-6143.2007.01993.x
6. Watts RP, Thom O, Fraser JF. Inflammatory signalling associated with brain dead organ donation: from brain injury to brain stem death and posttransplant ischaemia reperfusion injury. J Transplant. (2013) 2013:521369. doi: 10.1155/2013/521369
7. Zhai Y, Petrowsky H, Hong JC, Busuttil RW, Kupiec-Weglinski JW. Ischaemia-reperfusion injury in liver transplantation–from bench to bedside. Nat Rev Gastroenterol Hepatol. (2013) 10(2):79–89. doi: 10.1038/nrgastro.2012.225
8. Fernandez AR, Sanchez-Tarjuelo R, Cravedi P, Ochando J, Lopez-Hoyos M. Review: ischemia reperfusion injury-A translational perspective in organ transplantation. Int J Mol Sci. (2020) 21(22). doi: 10.3390/ijms21228549
9. Duneton C, Winterberg PD, Ford ML. Activation and regulation of alloreactive T cell immunity in solid organ transplantation. Nat Rev Nephrol. (2022) 18(10):663–76. doi: 10.1038/s41581-022-00600-0
10. Loupy A, Lefaucheur C. Antibody-mediated rejection of solid-organ allografts. N Engl J Med. (2018) 379(12):1150–60. doi: 10.1056/NEJMra1802677
11. Chong AS. Mechanisms of organ transplant injury mediated by B cells and antibodies: implications for antibody-mediated rejection. Am J Transplant. (2020) 20(Suppl 4):23–32. doi: 10.1111/ajt.15844
12. Levitsky J, Goldberg D, Smith AR, Mansfield SA, Gillespie BW, Merion RM, et al. Acute rejection increases risk of graft failure and death in recent liver transplant recipients. Clin Gastroenterol Hepatol. (2017) 15(4):584–93.e2. doi: 10.1016/j.cgh.2016.07.035
13. Harrington CR, Yang GY, Levitsky J. Advances in rejection management: prevention and treatment. Clin Liver Dis. (2021) 25(1):53–72. doi: 10.1016/j.cld.2020.08.003
14. Merola J, Emond JC, Levitsky J. Novel noninvasive biomarkers in liver transplantation: a tool on the doorstep of clinical utilization. Transplantation. (2023) 107:2120–25. doi: 10.1097/TP.0000000000004580
15. Kim MS, Pinto SM, Getnet D, Nirujogi RS, Manda SS, Chaerkady R, et al. A draft map of the human proteome. Nature. (2014) 509(7502):575–81. doi: 10.1038/nature13302
16. Anderson NL, Matheson AD, Steiner S. Proteomics: applications in basic and applied biology. Curr Opin Biotechnol. (2000) 11(4):408–12. doi: 10.1016/s0958-1669(00)00118-x
17. Pandey A, Mann M. Proteomics to study genes and genomes. Nature. (2000) 405(6788):837–46. doi: 10.1038/35015709
18. Walther TC, Mann M. Mass spectrometry-based proteomics in cell biology. J Cell Biol. (2010) 190(4):491–500. doi: 10.1083/jcb.201004052
19. Gygi SP, Aebersold R. Mass spectrometry and proteomics. Curr Opin Chem Biol. (2000) 4(5):489–94. doi: 10.1016/s1367-5931(00)00121-6
20. Farkona S, Pastrello C, Konvalinka A. Proteomics: its promise and pitfalls in shaping precision medicine in solid organ transplantation. Transplantation. (2023) 107:2126–42. doi: 10.1097/TP.0000000000004539
21. Han X, Aslanian A, Yates JR 3rd. Mass spectrometry for proteomics. Curr Opin Chem Biol. (2008) 12(5):483–90. doi: 10.1016/j.cbpa.2008.07.024
22. Tipton JD, Tran JC, Catherman AD, Ahlf DR, Durbin KR, Kelleher NL. Analysis of intact protein isoforms by mass spectrometry. J Biol Chem. (2011) 286(29):25451–8. doi: 10.1074/jbc.R111.239442
23. Bantscheff M, Lemeer S, Savitski MM, Kuster B. Quantitative mass spectrometry in proteomics: critical review update from 2007 to the present. Anal Bioanal Chem. (2012) 404(4):939–65. doi: 10.1007/s00216-012-6203-4
24. Zhang Y, Fonslow BR, Shan B, Baek MC, Yates JR 3rd. Protein analysis by shotgun/bottom-up proteomics. Chem Rev. (2013) 113(4):2343–94. doi: 10.1021/cr3003533
25. Peng J, Gygi SP. Proteomics: the move to mixtures. J Mass Spectrom. (2001) 36(10):1083–91. doi: 10.1002/jms.229
26. Shuken SR. An introduction to mass spectrometry-based proteomics. J Proteome Res. (2023) 22(7):2151–71. doi: 10.1021/acs.jproteome.2c00838
27. Fenn JB, Mann M, Meng CK, Wong SF, Whitehouse CM. Electrospray ionization for mass spectrometry of large biomolecules. Science. (1989) 246(4926):64–71. doi: 10.1126/science.2675315
28. Hunt DF, Yates JR 3rd, Shabanowitz J, Winston S, Hauer CR. Protein sequencing by tandem mass spectrometry. Proc Natl Acad Sci U S A. (1986) 83(17):6233–7. doi: 10.1073/pnas.83.17.6233
29. Aebersold R, Mann M. Mass-spectrometric exploration of proteome structure and function. Nature. (2016) 537(7620):347–55. doi: 10.1038/nature19949
30. Tyanova S, Temu T, Cox J. The MaxQuant computational platform for mass spectrometry-based shotgun proteomics. Nat Protoc. (2016) 11(12):2301–19. doi: 10.1038/nprot.2016.136
31. Eng JK, Searle BC, Clauser KR, Tabb DL. A face in the crowd: recognizing peptides through database search. Mol Cell Proteomics. (2011) 10(11):R111 009522. doi: 10.1074/mcp.R111.009522
32. Tabb DL. The SEQUEST family tree. J Am Soc Mass Spectrom. (2015) 26(11):1814–9. doi: 10.1007/s13361-015-1201-3
33. Al Shweiki MR, Monchgesang S, Majovsky P, Thieme D, Trutschel D, Hoehenwarter W. Assessment of label-free quantification in discovery proteomics and impact of technological factors and natural variability of protein abundance. J Proteome Res. (2017) 16(4):1410–24. doi: 10.1021/acs.jproteome.6b00645
34. Wiese S, Reidegeld KA, Meyer HE, Warscheid B. Protein labeling by iTRAQ: a new tool for quantitative mass spectrometry in proteome research. Proteomics. (2007) 7(3):340–50. doi: 10.1002/pmic.200600422
35. Thompson A, Schafer J, Kuhn K, Kienle S, Schwarz J, Schmidt G, et al. Tandem mass tags: a novel quantification strategy for comparative analysis of complex protein mixtures by MS/MS. Anal Chem. (2003) 75(8):1895–904. doi: 10.1021/ac0262560
36. Malone AF. Transplant biopsy assessment in 21st century. J Am Soc Nephrol. (2021) 32(8):1827–8. doi: 10.1681/ASN.2021060804
37. Wu L, Boer K, Woud WW, Udomkarnjananun S, Hesselink DA, Baan CC. Urinary extracellular vesicles are a novel tool to monitor allograft function in kidney transplantation: a systematic review. Int J Mol Sci. (2021) 22(19):10499. doi: 10.3390/ijms221910499
38. United Network of Organ Sharing-Transplant Trends. (2023). Available at: https://unos.org/data/transplant-trends/ (Cited August 16, 2023).
39. Oliveira Arcolino F, Tort Piella A, Papadimitriou E, Bussolati B, Antonie DJ, Murray P, et al. Human urine as a noninvasive source of kidney cells. Stem Cells Int. (2015) 2015:362562. doi: 10.1155/2015/362562
40. Sigdel TK, Gao Y, He J, Wang A, Nicora CD, Fillmore TL, et al. Mining the human urine proteome for monitoring renal transplant injury. Kidney Int. (2016) 89(6):1244–52. doi: 10.1016/j.kint.2015.12.049
41. Schaub S, Wilkins JA, Antonovici M, Krokhin O, Weiler T, Rush D, et al. Proteomic-based identification of cleaved urinary beta2-microglobulin as a potential marker for acute tubular injury in renal allografts. Am J Transplant. (2005) 5(4 Pt 1):729–38. doi: 10.1111/j.1600-6143.2005.00766.x
42. Cassidy H, Slyne J, O'Kelly P, Traynor C, Conlon PJ, Johnston O, et al. Urinary biomarkers of chronic allograft nephropathy. Proteomics Clin Appl. (2015) 9(5–6):574–85. doi: 10.1002/prca.201400200
43. Johnston O, Cassidy H, O'Connell S, O'Riordan A, Gallagher W, Maguire PB, et al. Identification of beta2-microglobulin as a urinary biomarker for chronic allograft nephropathy using proteomic methods. Proteomics Clin Appl. (2011) 5(7–8):422–31. doi: 10.1002/prca.201000160
44. Lim JH, Lee CH, Kim KY, Jung HY, Choi JY, Cho JH, et al. Novel urinary exosomal biomarkers of acute T cell-mediated rejection in kidney transplant recipients: a cross-sectional study. PLoS One. (2018) 13(9):e0204204. doi: 10.1371/journal.pone.0204204
45. Metzger J, Chatzikyrkou C, Broecker V, Schiffer E, Jaensch L, Iphoefer A, et al. Diagnosis of subclinical and clinical acute T-cell-mediated rejection in renal transplant patients by urinary proteome analysis. Proteomics Clin Appl. (2011) 5(5–6):322–33. doi: 10.1002/prca.201000153
46. Ho J, Rush DN, Krokhin O, Antonovici M, Gao A, Bestland J, et al. Elevated urinary matrix metalloproteinase-7 detects underlying renal allograft inflammation and injury. Transplantation. (2016) 100(3):648–54. doi: 10.1097/TP.0000000000000867
47. Mertens I, Willems H, Van Loon E, Schildermans K, Boonen K, Baggerman G, et al. Urinary protein biomarker panel for the diagnosis of antibody-mediated rejection in kidney transplant recipients. Kidney Int Rep. (2020) 5(9):1448–58. doi: 10.1016/j.ekir.2020.06.018
48. Zhang Y, Ou M, Lin H, Lai L, Chen H, Chen J, et al. Proteomic analysis of differentially expressed proteins in the serum of patients with acute renal allograft rejection using iTRAQ labelling technology. Mol Med Rep. (2020) 22(3):2329–41. doi: 10.3892/mmr.2020.11299
49. Freue GV, Sasaki M, Meredith A, Gunther OP, Bergman A, Takhar M, et al. Proteomic signatures in plasma during early acute renal allograft rejection. Mol Cell Proteomics. (2010) 9(9):1954–67. doi: 10.1074/mcp.M110.000554
50. Welberry Smith MP, Zougman A, Cairns DA, Wilson M, Wind T, Wood SL, et al. Serum aminoacylase-1 is a novel biomarker with potential prognostic utility for long-term outcome in patients with delayed graft function following renal transplantation. Kidney Int. (2013) 84(6):1214–25. doi: 10.1038/ki.2013.200
51. Kurian SM, Heilman R, Mondala TS, Nakorchevsky A, Hewel JA, Campbell D, et al. Biomarkers for early and late stage chronic allograft nephropathy by proteogenomic profiling of peripheral blood. PLoS One. (2009) 4(7):e6212. doi: 10.1371/journal.pone.0006212
52. Song L, Fang F, Liu P, Zeng G, Liu H, Zhao Y, et al. Quantitative proteomics for monitoring renal transplant injury. Proteomics Clin Appl. (2020) 14(4):e1900036. doi: 10.1002/prca.201900036
53. Mortensen LA, Svane AM, Burton M, Bistrup C, Thiesson HC, Marcussen N, et al. Proteomic analysis of renal biomarkers of kidney allograft fibrosis-A study in renal transplant patients. Int J Mol Sci. (2020) 21(7):2371. doi: 10.3390/ijms21072371
54. Chauveau B, Raymond AA, Di Tommaso S, Visentin J, Vermorel A, Dugot-Senant N, et al. The proteome of antibody-mediated rejection: from glomerulitis to transplant glomerulopathy. Biomedicines. (2022) 10(3):569. doi: 10.3390/biomedicines10030569
55. Moser MAJ, Sawicka K, Arcand S, O'Brien P, Luke P, Beck G, et al. Proteomic analysis of perfusate from machine cold perfusion of transplant kidneys: insights into protection from injury. Ann Transplant. (2017) 22:730–9. doi: 10.12659/aot.905347
56. Van Erp AC, Rebolledo RA, Hoeksma D, Jespersen NR, Ottens PJ, Norregaard R, et al. Organ-specific responses during brain death: increased aerobic metabolism in the liver and anaerobic metabolism with decreased perfusion in the kidneys. Sci Rep. (2018) 8(1):4405. doi: 10.1038/s41598-018-22689-9
57. Zaza G, Neri F, Bruschi M, Granata S, Petretto A, Bartolucci M, et al. Proteomics reveals specific biological changes induced by the normothermic machine perfusion of donor kidneys with a significant up-regulation of latexin. Sci Rep. (2023) 13(1):5920. doi: 10.1038/s41598-023-33194-z
58. Thorne AM, Huang H, O'Brien DP, Eijken M, Krogstrup NV, Norregaard R, et al. Subclinical effects of remote ischaemic conditioning in human kidney transplants revealed by quantitative proteomics. Clin Proteomics. (2020) 17(1):39. doi: 10.1186/s12014-020-09301-x
59. van Leeuwen LL, Spraakman NA, Brat A, Huang H, Thorne AM, Bonham S, et al. Proteomic analysis of machine perfusion solution from brain dead donor kidneys reveals that elevated complement, cytoskeleton and lipid metabolism proteins are associated with 1-year outcome. Transpl Int. (2021) 34(9):1618–29. doi: 10.1111/tri.13984
60. Snoeijs MG, Pulinx B, van Dieijen-Visser MP, Buurman WA, van Heurn LW, Wodzig WK. Characterization of the perfusate proteome of human donor kidneys. Ann Clin Biochem. (2013) 50(Pt 2):140–6. doi: 10.1258/acb.2012.011144
61. Emadali A, Muscatelli-Groux B, Delom F, Jenna S, Boismenu D, Sacks DB, et al. Proteomic analysis of ischemia-reperfusion injury upon human liver transplantation reveals the protective role of IQGAP1. Mol Cell Proteomics. (2006) 5(7):1300–13. doi: 10.1074/mcp.M500393-MCP200
62. Emadali A, Metrakos PP, Kalantari F, Boutros T, Boismenu D, Chevet E. Proteomic analysis of tyrosine phosphorylation during human liver transplantation. Proteome Sci. (2007) 5:1. doi: 10.1186/1477-5956-5-1
63. Jia J, Nie Y, Geng L, Li J, Liu J, Peng Y, et al. Identification of HO-1 as a novel biomarker for graft acute cellular rejection and prognosis prediction after liver transplantation. Ann Transl Med. (2020) 8(5):221. doi: 10.21037/atm.2020.01.59
64. Massoud O, Heimbach J, Viker K, Krishnan A, Poterucha J, Sanchez W, et al. Noninvasive diagnosis of acute cellular rejection in liver transplant recipients: a proteomic signature validated by enzyme-linked immunosorbent assay. Liver Transpl. (2011) 17(6):723–32. doi: 10.1002/lt.22266
65. Zhang Y, Wroblewski M, Hertz MI, Wendt CH, Cervenka TM, Nelsestuen GL. Analysis of chronic lung transplant rejection by MALDI-TOF profiles of bronchoalveolar lavage fluid. Proteomics. (2006) 6(3):1001–10. doi: 10.1002/pmic.200500105
66. Berra G, Farkona S, Mohammed-Ali Z, Kotlyar M, Levy L, Clotet-Freixas S, et al. Association between the renin-angiotensin system and chronic lung allograft dysfunction. Eur Respir J. (2021) 58(4). doi: 10.1183/13993003.02975-2020
67. Kennel PJ, Saha A, Maldonado DA, Givens R, Brunjes DL, Castillero E, et al. Serum exosomal protein profiling for the non-invasive detection of cardiac allograft rejection. J Heart Lung Transplant. (2018) 37(3):409–17. doi: 10.1016/j.healun.2017.07.012
68. Lukac J, Dhaygude K, Saraswat M, Joenvaara S, Syrjala SO, Holmstrom EJ, et al. Plasma proteome of brain-dead organ donors predicts heart transplant outcome. J Heart Lung Transplant. (2022) 41(3):311–24. doi: 10.1016/j.healun.2021.11.011
69. Giangreco NP, Lebreton G, Restaino S, Jane Farr M, Zorn E, Colombo PC, et al. Plasma kallikrein predicts primary graft dysfunction after heart transplant. J Heart Lung Transplant. (2021) 40(10):1199–211. doi: 10.1016/j.healun.2021.07.001
70. Smith LM, Kelleher NL, Consortium for Top Down P. Proteoform: a single term describing protein complexity. Nat Methods. (2013) 10(3):186–7. doi: 10.1038/nmeth.2369
71. Smith LM, Kelleher NL. Proteoforms as the next proteomics currency. Science. (2018) 359(6380):1106–7. doi: 10.1126/science.aat1884
72. Aebersold R, Agar JN, Amster IJ, Baker MS, Bertozzi CR, Boja ES, et al. How many human proteoforms are there? Nat Chem Biol. (2018) 14(3):206–14. doi: 10.1038/nchembio.2576
73. Young NL, DiMaggio PA, Plazas-Mayorca MD, Baliban RC, Floudas CA, Garcia BA. High throughput characterization of combinatorial histone codes. Mol Cell Proteomics. (2009) 8(10):2266–84. doi: 10.1074/mcp.M900238-MCP200
74. Phanstiel D, Brumbaugh J, Berggren WT, Conard K, Feng X, Levenstein ME, et al. Mass spectrometry identifies and quantifies 74 unique histone H4 isoforms in differentiating human embryonic stem cells. Proc Natl Acad Sci U S A. (2008) 105(11):4093–8. doi: 10.1073/pnas.0710515105
75. Pesavento JJ, Mizzen CA, Kelleher NL. Quantitative analysis of modified proteins and their positional isomers by tandem mass spectrometry: human histone H4. Anal Chem. (2006) 78(13):4271–80. doi: 10.1021/ac0600050
76. Tran JC, Zamdborg L, Ahlf DR, Lee JE, Catherman AD, Durbin KR, et al. Mapping intact protein isoforms in discovery mode using top-down proteomics. Nature. (2011) 480(7376):254–8. doi: 10.1038/nature10575
77. Sigismondo G, Papageorgiou DN, Krijgsveld J. Cracking chromatin with proteomics: from chromatome to histone modifications. Proteomics. (2022) 22(15–16):e2100206. doi: 10.1002/pmic.202100206
78. Melani RD, Gerbasi VR, Anderson LC, Sikora JW, Toby TK, Hutton JE, et al. The blood proteoform atlas: a reference map of proteoforms in human hematopoietic cells. Science. (2022) 375(6579):411–8. doi: 10.1126/science.aaz5284
79. Wilkins JT, Seckler HS, Rink J, Compton PD, Fornelli L, Thaxton CS, et al. Spectrum of apolipoprotein AI and apolipoprotein AII proteoforms and their associations with indices of cardiometabolic health: the CARDIA study. J Am Heart Assoc. (2021) 10(17):e019890. doi: 10.1161/JAHA.120.019890
80. Adams LM, DeHart CJ, Drown BS, Anderson LC, Bocik W, Boja ES, et al. Mapping the KRAS proteoform landscape in colorectal cancer identifies truncated KRAS4B that decreases MAPK signaling. J Biol Chem. (2023) 299(1):102768. doi: 10.1016/j.jbc.2022.102768
81. Huang T, Wang J, Yu W, He Z. Protein inference: a review. Brief Bioinform. (2012) 13(5):586–614. doi: 10.1093/bib/bbs004
82. Gregorich ZR, Chang YH, Ge Y. Proteomics in heart failure: top-down or bottom-up? Pflugers Arch. (2014) 466(6):1199–209. doi: 10.1007/s00424-014-1471-9
83. Toby TK, Fornelli L, Srzentic K, DeHart CJ, Levitsky J, Friedewald J, et al. A comprehensive pipeline for translational top-down proteomics from a single blood draw. Nat Protoc. (2019) 14(1):119–52. doi: 10.1038/s41596-018-0085-7
84. Savaryn JP, Toby TK, Catherman AD, Fellers RT, LeDuc RD, Thomas PM, et al. Comparative top down proteomics of peripheral blood mononuclear cells from kidney transplant recipients with normal kidney biopsies or acute rejection. Proteomics. (2016) 16(14):2048–58. doi: 10.1002/pmic.201600008
85. Kurian SM, Williams AN, Gelbart T, Campbell D, Mondala TS, Head SR, et al. Molecular classifiers for acute kidney transplant rejection in peripheral blood by whole genome gene expression profiling. Am J Transplant. (2014) 14(5):1164–72. doi: 10.1111/ajt.12671
86. Toby TK, Abecassis M, Kim K, Thomas PM, Fellers RT, LeDuc RD, et al. Proteoforms in peripheral blood mononuclear cells as novel rejection biomarkers in liver transplant recipients. Am J Transplant. (2017) 17(9):2458–67. doi: 10.1111/ajt.14359
87. Huang C KJ, Negrão F, Robey M, Toby T, Durbin K, Fellers R, et al. Targeted quantification of proteoforms in Complex samples by proteoform reaction monitoring. ChemRxiv. (2023). doi: 10.26434/chemrxiv-2023-g5986
88. Zhang J, Guy MJ, Norman HS, Chen YC, Xu Q, Dong X, et al. Top-down quantitative proteomics identified phosphorylation of cardiac troponin I as a candidate biomarker for chronic heart failure. J Proteome Res. (2011) 10(9):4054–65. doi: 10.1021/pr200258m
89. Tucholski T, Cai W, Gregorich ZR, Bayne EF, Mitchell SD, McIlwain SJ, et al. Distinct hypertrophic cardiomyopathy genotypes result in convergent sarcomeric proteoform profiles revealed by top-down proteomics. Proc Natl Acad Sci U S A. (2020) 117(40):24691–700. doi: 10.1073/pnas.2006764117
90. Chen B, Brown KA, Lin Z, Ge Y. Top-down proteomics: ready for prime time? Anal Chem. (2018) 90(1):110–27. doi: 10.1021/acs.analchem.7b04747
91. Peng Y, Ayaz-Guner S, Yu D, Ge Y. Top-down mass spectrometry of cardiac myofilament proteins in health and disease. Proteomics Clin Appl. (2014) 8(7–8):554–68. doi: 10.1002/prca.201400043
92. Smith LM, Agar JN, Chamot-Rooke J, Danis PO, Ge Y, Loo JA, et al. The human proteoform project: defining the human proteome. Sci Adv. (2021) 7(46):eabk0734. doi: 10.1126/sciadv.abk0734
93. Brown KA, Melby JA, Roberts DS, Ge Y. Top-down proteomics: challenges, innovations, and applications in basic and clinical research. Expert Rev Proteomics. (2020) 17(10):719–33. doi: 10.1080/14789450.2020.1855982
94. Compton PD, Zamdborg L, Thomas PM, Kelleher NL. On the scalability and requirements of whole protein mass spectrometry. Anal Chem. (2011) 83(17):6868–74. doi: 10.1021/ac2010795
95. Li Y, Compton PD, Tran JC, Ntai I, Kelleher NL. Optimizing capillary electrophoresis for top-down proteomics of 30-80 kDa proteins. Proteomics. (2014) 14(10):1158–64. doi: 10.1002/pmic.201300381
96. Drown BS, Jooss K, Melani RD, Lloyd-Jones C, Camarillo JM, Kelleher NL. Mapping the proteoform landscape of five human tissues. J Proteome Res. (2022) 21(5):1299–310. doi: 10.1021/acs.jproteome.2c00034
97. Kafader JO, Melani RD, Durbin KR, Ikwuagwu B, Early BP, Fellers RT, et al. Multiplexed mass spectrometry of individual ions improves measurement of proteoforms and their complexes. Nat Methods. (2020) 17(4):391–4. doi: 10.1038/s41592-020-0764-5
98. Melani RD, Des Soye BJ, Kafader JO, Forte E, Hollas M, Blagojevic V, et al. Next-generation serology by mass spectrometry: readout of the SARS-CoV-2 antibody repertoire. J Proteome Res. (2022) 21(1):274–88. doi: 10.1021/acs.jproteome.1c00882
99. Forte E, Des Soye BJ, Melani RD, Hollas MAR, Kafader JO, Sha BE, et al. Divergent antibody repertoires found for omicron versus Wuhan SARS-CoV-2 strains using ig-MS. J Proteome Res. (2022) 21(12):2987–97. doi: 10.1021/acs.jproteome.2c00514
100. Li JSY, Raghubar AM, Matigian NA, Ng MSY, Rogers NM, Mallett AJ. The utility of spatial transcriptomics for solid organ transplantation. Transplantation. (2023) 107(7):1463–71. doi: 10.1097/TP.0000000000004466
101. Tang Y, Wang J, Zhang Y, Li J, Chen M, Gao Y, et al. Single-cell RNA sequencing identifies intra-graft population heterogeneity in acute heart allograft rejection in mouse. Front Immunol. (2022) 13:832573. doi: 10.3389/fimmu.2022.832573
102. Doan Ngoc TM, Tilly G, Danger R, Bonizec O, Masset C, Guerif P, et al. Effector memory-expressing CD45RA (TEMRA) CD8(+) T cells from kidney transplant recipients exhibit enhanced purinergic P2X4 receptor-dependent proinflammatory and migratory responses. J Am Soc Nephrol. (2022) 33(12):2211–31. doi: 10.1681/ASN.2022030286
103. Peereboom ETM, Matern BM, Spierings E, Geneugelijk K. The value of single-cell technologies in solid organ transplantation studies. Transplantation. (2022) 106(12):2325–37. doi: 10.1097/TP.0000000000004237
104. Callemeyn J, Lamarthee B, Koenig A, Koshy P, Thaunat O, Naesens M. Allorecognition and the spectrum of kidney transplant rejection. Kidney Int. (2022) 101(4):692–710. doi: 10.1016/j.kint.2021.11.029
105. Budnik B, Levy E, Harmange G, Slavov N. SCoPE-MS: mass spectrometry of single mammalian cells quantifies proteome heterogeneity during cell differentiation. Genome Biol. (2018) 19(1):161. doi: 10.1186/s13059-018-1547-5
106. Woo J, Williams SM, Markillie LM, Feng S, Tsai CF, Aguilera-Vazquez V, et al. High-throughput and high-efficiency sample preparation for single-cell proteomics using a nested nanowell chip. Nat Commun. (2021) 12(1):6246. doi: 10.1038/s41467-021-26514-2
107. Zhu Y, Clair G, Chrisler WB, Shen Y, Zhao R, Shukla AK, et al. Proteomic analysis of single mammalian cells enabled by microfluidic nanodroplet sample preparation and ultrasensitive NanoLC-MS. Angew Chem Int Ed Engl. (2018) 57(38):12370–4. doi: 10.1002/anie.201802843
108. Leduc A, Huffman RG, Cantlon J, Khan S, Slavov N. Exploring functional protein covariation across single cells using nPOP. Genome Biol. (2022) 23(1):261. doi: 10.1186/s13059-022-02817-5
109. Cong Y, Liang Y, Motamedchaboki K, Huguet R, Truong T, Zhao R, et al. Improved single-cell proteome coverage using narrow-bore packed NanoLC columns and ultrasensitive mass spectrometry. Anal Chem. (2020) 92(3):2665–71. doi: 10.1021/acs.analchem.9b04631
110. Xiang P, Zhu Y, Yang Y, Zhao Z, Williams SM, Moore RJ, et al. Picoflow liquid chromatography-mass spectrometry for ultrasensitive bottom-up proteomics using 2-mum-i.d. Open tubular columns. Anal Chem. (2020) 92(7):4711–5. doi: 10.1021/acs.analchem.9b05639
111. Petelski AA, Emmott E, Leduc A, Huffman RG, Specht H, Perlman DH, et al. Multiplexed single-cell proteomics using SCoPE2. Nat Protoc. (2021) 16(12):5398–425. doi: 10.1038/s41596-021-00616-z
112. Stejskal K, Jeff OB, Matzinger M, Durnberger G, Boychenko A, Jacobs P, et al. Deep proteome profiling with reduced carryover using superficially porous microfabricated nanoLC columns. Anal Chem. (2022) 94(46):15930–8. doi: 10.1021/acs.analchem.2c01196
113. Melby JA, Brown KA, Gregorich ZR, Roberts DS, Chapman EA, Ehlers LE, et al. High sensitivity top-down proteomics captures single muscle cell heterogeneity in large proteoforms. Proc Natl Acad Sci U S A. (2023) 120(19):e2222081120. doi: 10.1073/pnas.2222081120
114. Pei Su MARH, Rubakhin S, Butun FA, Greer JB, Early BP, Fellers RT, et al. Top-down proteomics of 10,000 single brain cells. bioRxiv. (2023). doi: 10.1101/2023.05.31.543176
115. Clotet-Freixas S, McEvoy CM, Batruch I, Pastrello C, Kotlyar M, Van JAD, et al. Extracellular matrix injury of kidney allografts in antibody-mediated rejection: a proteomics study. J Am Soc Nephrol. (2020) 31(11):2705–24. doi: 10.1681/ASN.2020030286
116. Lundberg E, Borner GHH. Spatial proteomics: a powerful discovery tool for cell biology. Nat Rev Mol Cell Biol. (2019) 20(5):285–302. doi: 10.1038/s41580-018-0094-y
117. Giesen C, Wang HA, Schapiro D, Zivanovic N, Jacobs A, Hattendorf B, et al. Highly multiplexed imaging of tumor tissues with subcellular resolution by mass cytometry. Nat Methods. (2014) 11(4):417–22. doi: 10.1038/nmeth.2869
118. Singh N, Avigan ZM, Kliegel JA, Shuch BM, Montgomery RR, Moeckel GW, et al. Development of a 2-dimensional atlas of the human kidney with imaging mass cytometry. JCI Insight. (2019) 4(12). doi: 10.1172/jci.insight.129477
119. Piehowski PD, Zhu Y, Bramer LM, Stratton KG, Zhao R, Orton DJ, et al. Automated mass spectrometry imaging of over 2000 proteins from tissue sections at 100-mum spatial resolution. Nat Commun. (2020) 11(1):8. doi: 10.1038/s41467-019-13858-z
120. Seeley EH, Caprioli RM. Molecular imaging of proteins in tissues by mass spectrometry. Proc Natl Acad Sci U S A. (2008) 105(47):18126–31. doi: 10.1073/pnas.0801374105
121. Shrestha B, Javonillo R, Burns JR, Pirger Z, Vertes A. Comparative local analysis of metabolites, lipids and proteins in intact fish tissues by LAESI mass spectrometry. Analyst. (2013) 138(12):3444–9. doi: 10.1039/c3an00631j
122. Kiss A, Smith DF, Reschke BR, Powell MJ, Heeren RM. Top-down mass spectrometry imaging of intact proteins by laser ablation ESI FT-ICR MS. Proteomics. (2014) 14(10):1283–9. doi: 10.1002/pmic.201300306
123. Su P, McGee JP, Durbin KR, Hollas MAR, Yang M, Neumann EK, et al. Highly multiplexed, label-free proteoform imaging of tissues by individual ion mass spectrometry. Sci Adv. (2022) 8(32):eabp9929. doi: 10.1126/sciadv.abp9929
124. Hale OJ, Cooper HJ. Native mass spectrometry imaging of proteins and protein complexes by nano-DESI. Anal Chem. (2021) 93(10):4619–27. doi: 10.1021/acs.analchem.0c05277
125. Yang M, Hu H, Su P, Thomas PM, Camarillo JM, Greer JB, et al. Proteoform-selective imaging of tissues using mass spectrometry. Angew Chem Int Ed Engl. (2022) 61(29):e202200721. doi: 10.1002/anie.202200721
126. Laskin J, Heath BS, Roach PJ, Cazares L, Semmes OJ. Tissue imaging using nanospray desorption electrospray ionization mass spectrometry. Anal Chem. (2012) 84(1):141–8. doi: 10.1021/ac2021322
127. McGee JP, Su P, Durbin KR, Hollas MAR, Bateman NW, Maxwell GL, et al. Automated imaging and identification of proteoforms directly from ovarian cancer tissue. Nat Commun. (2023) 14(1):6478. doi: 10.1038/s41467-023-42208-3
128. Khachatoorian Y, Khachadourian V, Chang E, Sernas ER, Reed EF, Deng M, et al. Noninvasive biomarkers for prediction and diagnosis of heart transplantation rejection. Transplant Rev (Orlando). (2021) 35(1):100590. doi: 10.1016/j.trre.2020.100590
129. Mezzolla V, Pontrelli P, Fiorentino M, Stasi A, Pesce F, Franzin R, et al. Emerging biomarkers of delayed graft function in kidney transplantation. Transplant Rev (Orlando). (2021) 35(4):100629. doi: 10.1016/j.trre.2021.100629
130. Kienzl-Wagner K, Pratschke J, Brandacher G. Biomarker discovery in transplantation–proteomic adventure or mission impossible? Clin Biochem. (2013) 46(6):497–505. doi: 10.1016/j.clinbiochem.2012.10.010
Keywords: proteomics, proteoforms, bottom-up mass spectrometry, top-down mass spectrometry, biomarkers, solid organ transplantation
Citation: Huang C-F, Su P, Fisher TD, Levitsky J, Kelleher NL and Forte E (2023) Mass spectrometry-based proteomics for advancing solid organ transplantation research. Front. Transplant. 2:1286881. doi: 10.3389/frtra.2023.1286881
Received: 31 August 2023; Accepted: 13 November 2023;
Published: 24 November 2023.
Edited by:
Carl Atkinson, University of Florida, United StatesReviewed by:
Abhijit Naik, University of Michigan, United States© 2023 Huang, Su, Fisher, Levitsky, Kelleher and Forte. This is an open-access article distributed under the terms of the Creative Commons Attribution License (CC BY). The use, distribution or reproduction in other forums is permitted, provided the original author(s) and the copyright owner(s) are credited and that the original publication in this journal is cited, in accordance with accepted academic practice. No use, distribution or reproduction is permitted which does not comply with these terms.
*Correspondence: Eleonora Forte ZS1mb3J0ZUBub3J0aHdlc3Rlcm4uZWR1
†These authors have contributed equally to this work and share first authorship
Disclaimer: All claims expressed in this article are solely those of the authors and do not necessarily represent those of their affiliated organizations, or those of the publisher, the editors and the reviewers. Any product that may be evaluated in this article or claim that may be made by its manufacturer is not guaranteed or endorsed by the publisher.
Research integrity at Frontiers
Learn more about the work of our research integrity team to safeguard the quality of each article we publish.