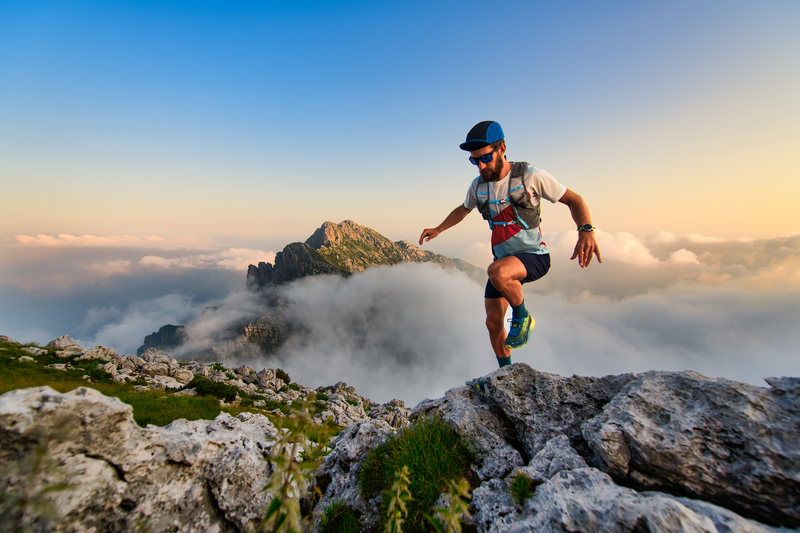
94% of researchers rate our articles as excellent or good
Learn more about the work of our research integrity team to safeguard the quality of each article we publish.
Find out more
ORIGINAL RESEARCH article
Front. Sustain. , 18 September 2023
Sec. Sustainable Consumption
Volume 4 - 2023 | https://doi.org/10.3389/frsus.2023.1178089
This article is part of the Research Topic From an Ethic of Sufficiency to its Policy and Practice in Late Capitalism View all 10 articles
The concept of the rebound effect is important in understanding the limits to how much technological efficiency improvements can reduce energy and resource consumption. However, due to the concept's focus on efficiency, it neglects other environmental implications of technological change. We use the term “induction effect” to grasp additional important mechanisms stemming from new technologies. We define an induction effect as an increase in the level of energy or resource consumption that was caused or enabled by the emergence of “new options” arising from technological change. Our investigation of three cases of new technologies - online consumption, smart homes, and pace of life - shows how including both rebound and induction effects into the analysis helps in understanding the relationship between technological change and energy and resource consumption. Integrating induction effects into the analysis underpins the importance of sufficiency as a strategy for sustainability and helps to develop comprehensive policy measures.
There is consensus in science that the increase in global environmental throughput needs to come to a halt and eventually decline if further transgression of planetary boundaries are to be avoided (Steffen et al., 2015; Rockström et al., 2023). One of the key challenges is to achieve sufficient absolute decoupling of economic activity, i.e., economic growth, from natural resource use and emissions (Parrique et al., 2019; Wiedenhofer et al., 2020). Prominent hope for such decoupling is placed in technological change in order to improve environmental efficiencies and realize circular economy patterns. Currently, particular hope is placed in the potential of digital technologies, including artificial intelligence, to further advance environmentally sound technological change (Lange et al., 2020; Kaack et al., 2022).
However, albeit continuous technological change, including widespread digitalization, key indicators of global resource use and emissions have still increased during the past years and decades (IPCC., 2021; Wolf et al., 2022). There is no evidence for sufficient absolute decoupling (Haberl et al., 2020). One of the reasons discussed in the literature are so-called rebound effects (Herring and Sorrell, 2009; Santarius et al., 2016). Rebound effects are unintended side effects from technological efficiency improvements that spur growth in demand or supply, which cancels out parts or all of the technological savings potential. The argument is that the efficiency improvements of new technologies are partly or even entirely countervailed by additional consumption and/or production of goods and services, shifts in the composition of goods and services, changes in the production methods applied, and behavioral changes of consumers, among others (Lange et al., 2021). Sufficiency is discussed as an effective strategy to prevent rebound effects because it ultimately aims to reduce the resource demands and emissions of individual consumers, organizations, and companies by trying to satisfy needs with less production and consumption (Herring, 2009; Best et al., 2022).
In this article, we develop the concept of the “induction effect” to grasp important mechanisms stemming from introducing new technologies. Efficiency improvements are not the only implication of technological change, and opinion is currently divided on the extent to which mechanisms stemming from efficiency improvements, or rather other mechanisms happening in the wake of technological change, can explain the failure in sufficient absolute decoupling.
We argue that – next to efficiency improvements - new technologies often introduce “new options” regarding production methods, product usage and changed behavior of individuals and firms. These inductions help explain another part of the increase in demand, which has so far countervailed reducing environmental throughput. Introducing the concept of the induction effect further underpins the role of sufficiency in achieving environmental sustainability. We show that sufficiency is an appropriate response not only to ever-increasing efficiencies (to rebound effects) but also to the continuous rise of new options (to induction effects).
The term induction effect has been used occasionally in the literature on the environmental impacts of new technologies, in particular regarding digital technologies (Hilty, 2008; Rattle, 2010). However, as we show in the literature review of this article, the term has never been clearly defined, nor has anyone developed a clear concept of the mechanisms leading to the effect. The first aim of this article, hence, is to develop a definition of the induction effect and of induction mechanisms. Given the body of literature that has used the term so far, and based on observations from our own empirical research on environmental effects of digitalization for absolute decoupling in three consumption domains, we do this by focusing on induction effects from digital technologies.
The second aim of this article is to highlight the relationship of the induction effect to the rebound effect and thereby improve the demarcation of rebound mechanisms from other mechanisms. More specifically, we start the analysis of this article by revisiting definitions of rebound effects and describing various rebound mechanisms arising from digitalization and then, detecting additional induction effects that we empirically observed. This leads us to set the induction effect as a phenomenon of technological change that functions complementary to the rebound effect. This clear definition and conceptualization of the induction effect contributes to rebound research, as it helps disentangle the energy and resource consumption debate on what is a rebound and what is not and helps sharpen the definition and understanding of mechanisms generating rebound effects. And it contributes to sufficiency research, as it helps specify the conditions of frugal consumption, including potential countervailing mechanisms.
Given these two aims, the article is structured as follows. In Section 2, we present our methodological approach before analyzing the literature on the rebound effect in Section 3. We point out that, within different strands of this literature, a debate is evident on whether the concept of the rebound effect should focus narrowly on mechanisms directly following efficiency improvements, or also include mechanisms beyond efficiency. In Section 4, we then introduce the concept of the induction effect. We analyze the few existing references to it in the literature, discuss its relation to the rebound effect and eventually, provide a clear definition of the induction effect. In Section 5, we underscore our conceptual work by use of empirical examples. We discuss environmental implications of digital technologies in three cases – online consumption, smart homes, and pace of life – to show how induction effects emerge, and how rebound and induction mechanisms are to be distinguished. We find that including both effects helps achieve a more comprehensive analysis of the environmental implications of technological change. In the discussion in Section 6, we develop a typology of different induction mechanisms and point out how considering the induction effect helps to improve the analytical basis for sufficiency-oriented policies. We conclude this article with a brief conclusion.
This article is an outcome of the interdisciplinary research group “Digitalization and Sustainability.” We are a group of six researchers who have investigated the relationship between digital technologies and sustainability over a phase of 6 years. We have worked on the relationship between the rebound effect and technologies, using empirical investigations and conceptual and theoretical analyses. In our work, we experienced the strengths but also the limitations of the concept of the rebound effect in understanding how technologies spur energy and resource consumption.
The method of this article combines conceptual work with empirical work in three cases. It follows a two step approach. In the first step of the approach, we combine an analysis of the literature on rebound effects with a nascent stream of literature on induction effects to develop a clear definition of the induction effect and inductions mechanisms that provides a complementary concept to the rebound effect and rebound mechanisms.
In the second step, we put the usefulness of this definition to a test by use of three cases: online-consumption, smart homes and pace of life. The authors of this article have conducted empirical investigations on the sustainability implications of technological change in these three cases. The insights from these empirical investigations are used to indicate rebound and induction mechanisms for these three cases and develop a typology of induction mechanisms.
The literature on the rebound effect and rebound mechanisms contains a controversial debate about which effects of technological change should be considered rebound effects and which are other effects of technology on the environment (Turner, 2013; Madlener and Turner, 2016). The modern debate on the rebound effect has a long tradition, reaching back to the 1970s. The literature started with a focus on economic mechanisms (covered in Sub-section 3.1). It then broadened in various directions. Two important extensions were to take psychological aspects into account (Sub-section 3.2) and to include rebound mechanisms stemming from time efficiency improvements (Sub-section 3.3). We conclude with discussion on what we consider is a rebound effect and what is not (Sub-section 3.4).
We follow Lange et al. (2021) in differentiating between rebound effects and rebound mechanisms: “A rebound effect relates to the quantitative size of a (measurable) impact on energy consumption while a rebound mechanism is a qualitative relation, e.g., a cause-and-effect chain from an energy efficiency improvement to energy consumption” (p. 1). Rebound effects are often divided into direct and indirect rebound effects. Direct effects stem from mechanisms that raise the demand for the goods or services that experienced an efficiency improvement. Indirect effects relate to increases in the consumption of other goods and services. In Sorrell (2007), the sum of the direct and indirect effects makes up the economy-wide effect. Another classification is into rebound effects and mechanisms at the micro, meso, and macro level (Santarius, 2016). In Lange et al. (2021), microeconomic mechanisms refer to those in households and firms, mesoeconomic ones to those in markets and business sectors, macroeconomic ones to mechanisms on the country level. They added a fourth level of global mechanisms between at least two countries.
Most of the rebound mechanisms listed in relevant publications are related to energy efficiency improvements. The literature has indicated numerous such rebound mechanisms (van den Bergh, 2011). Lange et al. (2021) list 18 rebound mechanisms, limiting the list to mechanisms relating to economics. For example, for household appliances, the income mechanism describes how people use money saved by energy efficiency improvements to buy more of the same good or service or others. The substitution mechanism refers to how the consumer is using more of the good or service that experienced the energy efficiency improvement because it becomes relatively cheaper than other goods and services. On the firm side, money saved from energy efficiency improvements can be used to expand production. These initial mechanisms at the household or firm level work their way through the economy, via various additional mechanisms. For example, when many firms experience energy efficiency improvements, this can lead to lower prices of the goods or services they supply, leading to more sales, or higher energy efficiency can lead to lower demand for energy, lowering the price of energy, which in turn induces additional energy consumption elsewhere in the economy.
The debate on what is considered to be a rebound effect or a rebound mechanism and what is not is controversial (Gillingham et al., 2013; Turner, 2013). The central question is whether only such mechanisms that directly and causally follow from an efficiency improvement should be considered rebound mechanisms, or also any associated mechanisms. The literature contains diverse understandings about “the extent to which energy efficiency improvements should be considered independently of any associated improvements in the productivity of labor and capital” (Sorrell and Dimitropoulos, 2007, p. 131). New technologies often go hand-in-hand with energy efficiency improvements, increased labor, capital productivity, or the ability to produce new products. For instance, Holm and Englund (2009) used the term “gross rebound effect” to include such broad effects when comparing energy intensity of GDP and demand at the macro-economic level.
Although the study of rebound effects and mechanisms commenced in the domain of energy economics, rebound research has been extended to other disciplines for more than a decade (Santarius et al., 2016). These studies have resulted in additional rebound mechanisms being identified at the consumer level. A systematic categorization and ordering of interdisciplinary rebound mechanisms are still lacking (Font Vivanco et al., 2022), yet a review on indirect rebound effects at the consumer level contributed insights on psychological mechanisms (Reimers et al., 2021).
Psychological rebound effects - also called “motivational rebound effects” (Santarius and Soland, 2018) or “mental rebound effects” (Girod and de Haan, 2009) rest on the assumption that energy efficiency improvements do not only have a “price content” (Khazzoom, 1980; Girod and de Haan, 2009) but may also have symbolic and behavioral content (Santarius, 2015). Santarius and Soland (2018, p. 415) define a psychological rebound effect as “an increase in energy service demand due to a change in consumer preferences that can be attributed to an increase in technological energy efficiency.” Reimers et al. (2021) delimit psychological mechanisms from economic mechanisms in rebound effects: whereas the economic mechanism describes income and substitution mechanisms that do not require active reflection of one's moral goals, psychological mechanisms include psychological rationalization processes reflecting on the morality or sustainability of one's own behavior.
One such mechanism is referred to as moral licensing: the purchase or use of a more efficient technology is perceived as a good deed that licenses increased preferences for the purchase or use of that technology, or of other technologies. For example, a person that bought a very efficient car or electric vehicle might feel that driving it more than the previous conventional car is morally legitimate. This licensing mechanism is also described under the terms of mental accounting (Hahnel et al., 2020) or negative spillover effects (Truelove et al., 2014; Nilsson et al., 2017).
Additionally, the diffusion of responsibility is a psychological rebound mechanism (Santarius and Soland, 2018). Due to the purchase or use of efficient technologies, consumers may perceive that the responsibility for protecting the environment diffuses to other agents, such as to the engineers, policy makers, or other consumers as potential adopters of those efficient technologies. This diffusion of responsibility, in turn, can give way to a more intensive use of that technology. Another psychological mechanism can stem from consumers' perception that behavioral costs, i.e., the monetary, social, or emotional consequences of using an inefficient technology, shrink when that technology becomes more efficient. Accordingly, taking a ride with a very efficient car or an electric car simply to get bread rolls at the next corner might be perceived as less costly and less frowned upon by neighbors, and may feel better compared to doing so with a dirty combustion engine.
However, it can be controversially debated what part of that demand increase stems from the technological efficiency improvement, i.e., the reduction of behavioral costs, and is therefore to be considered a rebound effect. There may be other (psychological) explanatory mechanisms, for instance, additional rides might be triggered simply by the new design of the car (a “feel good factor”) or by additional functionalities such as driver assistance (new consumption options).
A further distinct research strain investigates time rebound effects. These rebound effects rest on the assumption that technological efficiency improvements may also bring about changes in the time available for consumption. Hence, compared to economic and psychological rebound effects, the input factor considered is not energy or resources, but time.
The aspect of time was introduced by Binswanger (2001) and Jalas (2002). Building on those foundations, Brenčič and Young (2009), Druckman et al. (2012), and Buhl and Acosta (2016) analyze how time efficiency improvements impact energy service demand. For instance, Buhl and Acosta (2016) show that time gains are reinvested in resource-intensive leisure activities and hobbies such as various sports activities or eating out. With a broader scope, Buhl (2016) and Geiger et al. (2021) discuss how time efficiency improvements impact the general pace of life. In that sense, a time rebound effect can be defined as an increase of actions per unit of time that has been caused or at least enabled by an improvement in time efficiency (Santarius and Bergener, 2020).
Four time rebound mechanisms have been identified [see Bergener and Santarius (2021)]. These mechanisms build on explanatory factors found in sociological theories of social acceleration - which is the global phenomenon of an increase in the number of actions per unit of time (Rosa, 2013). First, technologies may be applied to multitasking, i.e. to doing several things at the same time. For instance, media technologies from radio over TV to today's video streaming can be done in parallel to cleaning the house or meeting friends. Second, technologies can be applied to perform activities faster. For instance, the invention of the washing machine most likely led to large rebound effects (backfire) due not only to reductions in energy demand and costs but also to savings in time (see also Davis et al., 2012). Third, technologies can be used to productively fill transfer and waiting times. For instance, the smartphone can be used to do job-related work while being in the bus or metro. And fourth, technologies can be used to replace time-intensive activities with time-saving ones. For instance, time efficiency gains achieved by taking the plane instead of the car or train may result in more trips overall.
However, as with the other two group of rebound effects, the debate continues on whether those four basic mechanisms necessarily all rest on time efficiency improvements and should therefore be considered as rebound mechanisms. If an efficiency improvement is generally understood as optimizing an input-output-ratio, then filling waiting times or multitasking might not result from needing less time to complete an action (i.e. time efficiency) but rather from using time more flexibly to perform such actions. Moreover, media technologies in particular open up new options for flexible time use, such as communicating asynchronously with other people or shopping or watching videos and news anytime, anywhere, rather than complying to infrastructures such as opening hours or a set TV program.
Within the debate on rebound effects and mechanisms, two aspects should be noted. First, the borders of argumentation between economic, psychological, and time rebound mechanisms and effects are blurred. For instance, with reference to Becker (1965), time can be considered an input factor in (household) production functions that is interlinked with, if not substitutable by other inputs such as capital (Jalas, 2002). And Frick (2022) points to the psychological construct of “behavioral costs,” which include not only financial expenses but also the perception of physical, mental, and temporal effort to perform a given behavior (Verhallen and Pieters, 1984).
Second, hotly contested is whether aspects such as time rebound mechanisms should actually be regarded as part of the rebound debate or the term “rebound” should be restricted to energy and resource efficiency improvements. This debate is closely related to the question of whether additional energy consumption due to labor productivity increases, which accompany new technologies that increase energy efficiency, should be counted as part of the rebound effect (Sorrell and Dimitropoulos, 2007).
The important aspect for this article is that there are controversial debates on what to include as the rebound effect. Some scholars keep a narrower focus to clearly define the rebound effect as the increase in energy and resource consumption directly related to efficiency improvements. Others, however, take a wider definition, probably to grasp more of the relevant mechanisms stemming from new technologies. We propose to follow the first option - to restrict the rebound effect to mechanisms stemming from efficiency improvements.
Following a definition of the rebound effect that is limited to mechanisms from increases in efficiency facilitates analytical clarity. But with such a narrower definition, several mechanisms that are important in explaining the limitations of technological change to improve environmental sustainability, are left out. We introduce the term induction in addition to the term rebound in order to achieve a clear and restricted understanding of the rebound effect while at the same time taking into account the major impacts of technological change on energy and resource demand. The next section develops the concept of the induction effect.
The induction effect has been used occasionally in the literature on the environmental impacts of new technologies, in particular regarding digital technologies. The term is often not clearly defined, and its relation to the rebound effects is described differently across articles. In the following, we analyze existing use of the term and descriptions of its relation to rebound effects. We then argue for a definition of the induction effect that focuses on new options brought about by technologies and that sets the induction effect as a phenomenon complementary to the rebound effect.
Early descriptions of the induction effect mainly give examples, while not clearly defining the term. Hilty (2008) is the first to describe the induction effect as increased demand for existing products and goods due to the introduction of digital technologies. As an example, he cites the introduction of (efficient) printers, which resulted in an increase in paper consumption: “[...] today's PC and printer technology enables the user to print out hundreds of pages with just a few mouse clicks” (Hilty, 2008, p. 38). He puts forward a second example regarding more traffic arising from the introduction of digital technologies: “People who get to know each other via the Internet may want to meet some day in person” (Hilty, 2008, p. 133). While Hilty does not clearly define the induction effect, Mickoleit (2010) does provide a definition, referring to an example similar to that of Hilty: “Induction effects can occur if ICT products help to increase demand for other products, e.g., efficient printers may stimulate demand for paper” (p. 9). This definition is broad and does not explain the mechanism behind the induction effect.
Rattle (2010) developed a categorization with two additional effects next to the induction effect. He defines induction effects as “greater use of an existing product, process, or activity” (chapter 6). As an example, he cites “a satellite dish resulting in an increased availability of content [that] might induce increased television viewing” (chapter 6). This description follows the same logic as that of the direct rebound effect, as the use of a technologically changed good or service is increased. Rattle also introduces two further effects: the supplementation effect and the creation effect. In contrast to induction effects, supplementation effects stem from new products related to information and communication technologies (ICT) that complement existing products or services, for example, “a printer [...] would supplement a computer or Internet access, providing an outlet for their increased use” (Chapter 6). Rattle hence distinguishes between the induction effect and the supplementation effect in terms of the product whose consumption is enhanced (an existing product vs. a, for the consumer, new ICT-based product). This distinction is similar to that between the direct and the indirect rebound effect (see Sub-section 2.1). The third effect he identifies is the creation effect, which results from new ICT products being applied in new fields created by ICT. Recent examples of the creation effect include machine learning as an application of artificial intelligence, or cryptocurrencies as an application of blockchain technology. Hence, this third category focuses on the application of a certain product type (ICT products) in the rest of the economy and is therefore only helpful when focusing on the specific set of ICT technologies.
Røpke (2012) gives a first idea of what the mechanism might be that defines an induction effect - convenience and making things easier. She argues that “ICT application stimulates increased use of a product or service” (p. 1634) and provides possible explanations of how the additional demand for other goods and services arises, namely that technologies improve convenience and make it easier for people to consume. Our definition builds on a similar understanding.
The relationship between rebound and induction effects is (often implicitly) displayed differently. Several authors argue that the induction effect is a more general response to technological change than the rebound effect. According to Gossart (2015), the difference between rebound and induction effects lies in the different underlying mechanisms of “pure energy efficiency improvements [i.e. drivers of rebound effects] and technological changes that include energy efficiency improvements [i.e. drivers of induction effects]” (p. 5). Røpke (2012) also describes the induction effect as “more general than the rebound effect” (p. 1634).
In contrast, Rattle (2010) and Aebischer and Hilty (2014) see the induction effect as part of the rebound effect. For Rattle (2010), the rebound effect encompasses all three effects (induction effect, supplementation effect, creation effect) as described above. Aebischer and Hilty (2014) see the induction effect taking place on the micro level, while the rebound effect occurs on the macro level. These understandings of Rattle as well as Hilty and Aebischer are however both unsatisfactory. Rattle's understanding includes the possibility that all mechanisms stemming from technological change could be regarded as rebound effects. Hilty's and Aebischer's view contradicts the literature on the rebound effect, as rebound mechanisms have long been understood to (also) occur at the micro level (Khazzoom, 1980, 1987) and work their way through the economy (Lange et al., 2021).
We propose using an understanding of the concept “induction effect” that can be combined with the concept “rebound effect.” This understanding necessitates that the two effects originate from different types of mechanisms and that one can distinguish and delimit their impact on energy and resource heuristically.
We follow the main line of reasoning in the rebound literature that any rebound mechanism rests on the explanatory factor of changing input-output-ratios; rebound mechanisms stem from technologically facilitated efficiency improvements. As already described, there are controversial debates on whether the rebound effect should be understood in relation to only energy and resource efficiency or also to other efficiencies, such as time, effort, or other types of inputs. We show that, even when a broad understanding is applied, many important impacts of technological change on increased energy or resource consumption cannot be captured by the concept of the rebound effect. And, thus, including the induction effect is expedient.
In contrast to rebound mechanisms, induction mechanisms stem from new options. Several authors highlight the importance of new options leading to consumption increases while not calling the result the induction effect. Walnum and Andrae (2016) argue that, with the introduction of cloud computing, “new consumption (and production) options [emphasis added] that were not available earlier” (p. 237) appeared, ultimately leading to increased energy consumption (the authors do not call this phenomenon the induction effect). This mechanism of increased choice of options leading to increases in electricity consumption is also described by Røpke et al. (2010): “[...] the introduction of the internet [...] opened up a whole new range of possibilities. With the development of laptops, other mobile devices and mobile access to the internet, the number of applications is escalating” (p. 1767).
Several authors have used similar understandings of the induction effect referring to options: Hilty (2008), for example, describes how ICT infrastructure induces “[...] globalization of markets and distributed forms of production [...]” (p. 38). Following this logic, the creation effect described by Rattle (2010) as “a new niche or application for a new ICT product” can also be designated as an induction effect. This can be seen, for example, in the wide range of new application options in various areas such as the home, in production or in the transport sector that have arisen as a result of the introduction of machine to machine communication. Pohl et al. (2019), following Walnum and Andrae (2016), similarly define the induction effect as” changes in user behavior that can be attributed to an increased choice of options” (p. 700).
We build on such understandings and suggest a definition that may serve as a guideline to further investigate the induction effect and related mechanisms both in production and consumption: An induction effect refers to an increase in the level of energy or resource consumption that was caused or enabled by the emergence of new options arising from technological change.
Our understanding of the induction effect is based on investigations on three cases, which we conducted over 6 years. These studies and our results are described in the next section.
We have investigated the relationship between technological change and energy and resource consumption for three cases: online consumption, smart homes and the pace of life. We found that efficiency improvements and the rebound effect were limited in their ability to explain the effects we observed. We ascertain that including the aspect of new options and the induction effect allows a much wider range of mechanisms to be considered and, thereby, improves understanding the interplay between technological change and energy and resource consumption.
Online shopping is becoming increasingly popular. For instance, more than 75% of the population in France, Germany, and Finland ordered or bought goods or services over the internet in 2021, and this share reached at least 80% in Luxembourg, Sweden, Ireland, the Netherlands, and 91% in Denmark (Eurostat, 2022).
Improving the sustainability record of the online/e-commerce sector is a complex process. Most of the sector's CO2 emissions originate from the last mile, shipping, and from returns and the increase in packaging waste, whereas warehouses and distribution centers usually only contribute a small part to the total greenhouse gas emissions of online purchases (Zimmermann et al., 2020). Whether e-commerce is indeed more efficient than in-store commerce depends on a number of factors: the means of transport used to get the product from the seller to the customer (e.g., last-mile delivery), the ICT infrastructures of online shops, the size of shopping baskets, the share of return rates, and the type and quantity of packaging (Zimmermann et al., 2020). Of these factors, transport is the most decisive in any comparison of greenhouse gas emissions from e-commerce or in-store shopping, i.e. whether transport associated with online shopping actually replaces transport for in-store shopping or comes on top. The e-commerce sector's hopes to win in this comparison mainly revolve around the sector's capacity to lower environmental impact by optimizing the shipping process.
In our research (see Box 1), we have found that several mechanisms are involved in how online consumption leads to overall more consumption and, thereby, to more energy and resource use. E-commerce allows users to purchase almost any product or service, from anywhere in the world, at any time. Online shopping means that opening hours, choice restrictions, or product characteristics such as size and weight are no longer a barrier to consumption. This breadth of choice increases the efficiency of purchase behavior (Voropanova, 2015), but it also increases the number of product options and opens up new possibilities for purchasing. Accordingly, some of the associated mechanisms are rebound and some are induction mechanisms.
Box 1. Our studies on online consumption.
In a cross-sectional three-study design, we measured self-reported consumption levels of clothing (N = 883), digital devices (N = 860), and leisure travel (N = 976), purchase intentions and perceived behavioral efficiency gains of online-shopping (Frick and Matthies, 2020). Moderation analyses tested whether purchase intentions and efficiency gains predicted higher consumption levels: Online shopping was perceived to have lower behavioral costs than in-store purchasing, except in searches for transport alternatives (e.g., bus, train). Perceived behavioral efficiency gains of online shopping were not linked to higher clothing consumption levels, but they were linked to higher consumption levels in case of digital devices and travels.
Frick et al. (2020): Another study based on the same sample examined how perception of consumption-promoting online content influences individual consumption levels of clothing, digital devices, and leisure air travel, as mediated by individual aspiration levels and personal and social norms. Structural equation modeling confirmed relationships between perceived consumption-promoting online content and consumption levels, fully mediated through aspiration levels. Sufficiency-promoting online content is associated with higher social and personal norms for sufficiency, but neither of the latter are linked to aspiration or consumption levels.
On the rebound mechanism side, the fear is that online environments make consumption so effortless that they stimulate excessive and unsustainable consumption. For most people, the behavioral costs of online shopping are perceived to be lower than behavioral costs of shopping in-store (Frick and Matthies, 2020). In some cases, the decrease of behavioral costs is also linked to higher consumption levels (Frick and Matthies, 2020). Decreased behavioral costs for shopping online may also lead to more purchases in other areas. For example, even if e-commerce decreased the number of shopping trips [although research by Buldeo Rai (2021) suggests that these trips do not decrease], other passenger trips may increase due to additional time for alternative activities (Smidfelt Rosqvist and Hiselius, 2016). Such decreases in behavioral costs can be covered by the concept of the rebound effect, as they relate to changes in the ratio between effort/time/money and the good or service purchased.
In addition to the rebound mechanisms, online consumption also involves various induction mechanisms. Digital consumption options provide consumers with a wider product collection. New consumer worlds and sales platforms appear, many of which offer almost unlimited product catalogs. If such larger product catalogs lead to more consumption, an induction effect has arisen, an effect due to new options - in this case, the option to buy products that were not available before.
Another mechanism is related to the increasing use of mobile phones for shopping. Mobile phones allow consumers to buy at any time and from anywhere (Lange and Santarius, 2020; Li et al., 2020). In an empirical study, individuals changing from a stationary to a mobile device increased their online shopping level and frequency (Wang et al., 2015). As online purchasing in the middle of the night or while waiting for a bus is a new consumption option, this option can be considered an induction effect. However, this example shows that it is sometimes ambiguous whether a phenomenon is due to efficiency - and hence a rebound - or new options - and hence an induction effect. Does shopping at any time make the shopping process more efficient or is it a new option? In this case, we argue for the new option because the central aspect is not to save time but to consume at a moment in which consumption would otherwise not have been an option.
Further, online marketing strategies can increase consumption levels and accelerate consumption cycles. Retailers are increasingly making use of online marketing. In 2021, 455.30 billion US dollars were spent on digital advertising, 61% of total media advertising spending (Cramer-Flood, 2021), leading to a ubiquity of commercialized messages and high daily exposure to advertising for the average internet user. As a result, not only online sales but also over-the-counter retail sales of clothing were positively linked to online advertising expenditures, bringing greater returns than traditional advertising (Dinner et al., 2014). Additionally, authors of the present article showed in Frick et al. (2020) and Frick et al. (2022) that perceiving online advertising was correlated with individuals' consumption desires and actual consumption levels. The impacts of online advertising by online retailers on increased consumption levels are induction mechanisms, as the internet offers new marketing options that attempt to increase consumption desires and levels.
An increasingly important reason why online marketing may be extremely potent in increasing consumption is personalization, enabled by new data analytics: Advertising can be personalized by showing certain groups of people advertising that fits their sociodemographic and/or psychometric profile, or advertising from retailers located in a viewer's geographic vicinity. It can also be personalized by retargeting (advertising products or shops people recently visited online), a method shown to receive more clicks than non-personalized banner ads (Bleier and Eisenbeiss, 2015). Accordingly, evidence has shown that the perception of personalized advertising is associated with an increased desire to buy (Frick et al., 2022). Personalization is considered to be an induction mechanism as it has arisen from new options to target commercials.
Another increasingly important mechanism is influencer marketing, which is only possible due to social media: The influencers with the strongest reach on social media channels mainly advertise fashion trends, status consumption, and luxurious lifestyles, while sustainable products, ideas, or lifestyles are hardly discussed (Werg et al., 2021). Not surprisingly, this new type of marketing has been shown to stimulate purchase intentions (Jiménez-Castillo and Sánchez-Fernández, 2019). These examples show that new technologies have not only increased the amount of money being spent on commercials but also changed the quality of advertising, leading to more consumption. In addition to the personalization of advertising and influencer marketing, digital advertising strategies such as search engine optimization (SEO), big data, and tracking can also encourage impulsive buying, another main cause of excessive consumption (Zafar et al., 2021).
Overall, the case of online consumption shows that including aspects of additional options and, hence, induction mechanisms allows a much larger set of mechanisms to be incorporated in empirical investigations. The induction mechanisms we encountered - a wider product collection, consumption at any time from anywhere, and various new online marketing possibilities - deliver key explanations for how online consumption can lead to more energy and resource demand.
The second case regarding the relationship between rebound effects, inductions effects, and energy consumption is smart homes. Smart homes contain a variety of networked devices in the home, such as radiator thermostats, windows sensors, smart plugs, smart TV, voice command devices, cameras, smart washing machines, and many more (Berry et al., 2007).
The question of how smart homes may contribute to reducing energy and resource consumption in the house has been discussed in various research disciplines (Marikyan et al., 2019; Sovacool and Furszyfer Del Rio, 2020). The results are ambiguous in terms of absolute energy and resource savings (van Dam et al., 2013; Tirado Herrero et al., 2018). This ambiguity arises from the idea of the automated and connected smart home serving several purposes at the same time, e.g., the potential technical approach to lowering households' energy demands (Balta-Ozkan et al., 2014) and related greenhouse gas emissions (Sintov and Schultz, 2017; Riekstin et al., 2020) while also providing consumer needs such as comfort and convenience (Strengers et al., 2020). Our research shows that smart home users in Germany do not prioritize the overarching goal of environmental and climate protection (Quitzow and Rohde, 2021). This finding is related to our findings that, to justify the appropriateness of smart home technologies, politicians highlight the environmental benefits while the smart home industry emphasizes notions of comfort, convenience, and control (Rohde and Santarius, 2023). And a social media discourse analysis shows that, while critical actors dominate the public online discourse, they do not focus on environmental aspects but rather on issues such as surveillance, privacy and data protection, and cyber security (Rohde et al., under review).
The hope related to smart homes is - as for online consumption - one of efficiency. Most importantly, smart home systems have the potential to save energy by optimizing energy-consuming processes through sensors and intelligent (learning) algorithms (van Dam et al., 2013; Habibi, 2017). These smart applications include regulation of room temperature, e.g., by smart thermostats or smart window control; lighting control depending on room occupancy, e.g., by occupancy based lighting or smart lighting; recommendations for energy savings through visual feedback, e.g., home energy monitoring. Furthermore, the optimization of overall energy consumption by combining different smart home technologies in the smart home is expected to contribute to energy savings (IEA 4E., 2018). Smart home research has found an average heating energy reduction of 4% with smart heating control (Rehm et al., 2018). While some users in the field test achieved energy savings of up to 30%, others had an increase in energy demand of more than 25% (Rehm et al., 2018). Other results from agent-based modeling showed smart energy feedback information could help users reduce their electricity consumption by up to 2% (Walzberg et al., 2017).
In empirical studies (see Box 2), we found that several mechanisms can potentially diminish the energy savings from smart homes. From an environmental perspective, the drivers include the energy and resources from producing smart home technologies (Pohl et al., 2021). From a socio-technical perspective, they include changing lifestyle expectations on comfort, convenience or cleanliness, and related changes in user behavior (Tirado Herrero et al., 2018; Nicholls et al., 2020) due to digital technologies in the home. These mechanisms can again be captured to be either rebound or induction mechanisms.
Box 2. Our studies on smart homes.
We conducted an interdisciplinary smart home study, which integrated concepts and methods from the fields of environmental assessment, environmental psychology, sociology, and science and technology studies. Our empirical insights are based on a quantitative survey with smart home owners in Germany with a smart heating system (N = 375), 12 user interviews, and a life cycle assessment that accounted for differences in user behavior. In addition, we conducted a twitter data analysis and a document analysis (Frick and Nguyen, 2021; Pohl et al., 2021; Rohde and Santarius, 2023).
Major findings are that the energy consumption due to the production and use of smart heating would necessitate at least a 6% reduction in energy consumption in heating in order for it to be environmentally beneficial. But smart home households purchase and use additional smart devices so that the reduction in energy consumption from heating would have to be even bigger. The quantitative survey shows that aspects such as safety, making everyday life easier, practical operation, convenience and financial savings are important motives for using smart home systems (Frick and Nguyen, 2021), which is one explanation for the large number of smart home devices that do not aim to reduce energy demand but to increase controllability and comfort (Strengers et al., 2020; Quitzow and Rohde, 2021). The findings show that four smart home user groups can be identified: enthusiasts, pragmatists, energy savers, and skeptics (Frick and Nguyen, 2021). Through a combination of quantitative network analysis and qualitative content analysis, we were able to reveal five discourse coalitions that form around certain storylines, namely “Threat”,” Hackable”, “Useless”, “Fixable”, and “Opportunity”. It became evident that the most influential actors in the German online discourse were taking a critical stance toward the smart home (Rohde et al., under review).
If smart homes lead to savings in heating energy, people also save on money. This saving can be linked to a rebound mechanism: the income mechanism. People can use the saved money either on heating more or on consuming other goods and services.
The fear that the use of smart homes may lead to people making rooms warmer, or may increase the number of heated rooms in the household, can also be grounded on a psychological rebound mechanism, e.g., on moral licensing or diffusion of responsibility: As smart heating is supposedly energy saving, people might be inclined to heat more, as they think to have done their contribution by buying an automated heating already. And indeed, qualitative results of authors of this article suggest psychological rebound effects, such as pre-heating rooms or turning on heating in rooms that respondents said they would otherwise not heat (Rohde and Santarius, 2023).
However, a quantitative smart home survey did not reveal these rebound effects. It did not reveal any significant differences in heating behavior, with an average room temperature of 19.43 °C for the smart home sample and 19.45 °C for the control group (Pohl et al., 2021). Smart homes did not seem to entice users to increase room temperature.
Instead, a major effect on the environmental impact of smart homes stems from the use of additional smart home devices, new options that do not contribute to energy savings in the home but instead provide other smart home services, such as comfort, security, or control. Based on our definition, these are induction mechanisms, as the application of these devices is due to new technological options not previously available. In our online survey, we found that smart home users with smart heating have, on average, eight additional smart home components, such as smoke detectors, humidity sensors, or cameras (Pohl et al., 2021). We estimated how much heating energy needs to be saved to offset the additional energy consumption due to additional devices in a smart home. The results showed that smart heating can only contribute to overall savings of greenhouse gas emissions and primary energy demand when the associated devices can help save at least 6% of a household's annual heating energy. Hence, the potential overall energy savings due to smart heating are significantly reduced by the environmental impact of producing and operating additional smart home devices that serve other goals.
These induction mechanisms can be rooted in raising expectations and new consumer needs, such as energy-intensive ideas of cleanliness and wellbeing that exacerbate householder anxiety about cleanliness and increase energy consumption (Shove, 2003; Nicholls and Strengers, 2019). Research found that robotic vacuums, for example, could act as a “gateway” appliance to the adoption of other automated home cleaning appliances introduced to the smart home market (Nicholls and Strengers, 2019). With the increasing availability of automated devices in the home (such as shutters, light switches, windows), smart home devices may supplement rather than substitute “manual” household appliances (Nicholls and Strengers, 2019) and thus raise energy demand.
The smart home case shows - as did the online consumption case - that including induction mechanisms helps explain the relation between technological change and energy and resource demand. Indeed, the most important aspect that increases energy and resource demand - i.e., additional smart home devices - cannot be explained by efficiency increases alone but only by taking into account how technologies provide new consumption options.
Technologies can be time-savers. For instance, digital technologies can save time by providing services that might otherwise involve time-consuming journeys – obvious examples being online shopping vs. in-store shopping (see above), or replacing a trip to the cinema by video streaming at home. However, despite the introduction of new technologies, including digital ones, the pace of social life is accelerating (Levine and Norenzayan, 1999). An acceleration of the pace of life is understood as an increasing number of activities performed during a given day (Rosa, 2013). Does a higher “degree of digitalization” (e.g., more digital devices, an intensive use of apps, longer hours on the internet, etc.) lead to a faster pace of life - and thereby also increase energy and resource consumption?
Interestingly, debates around the speeding up of the pace of life have revealed a paradox, i.e., a counter-intuitive driver of social acceleration: “time-saving technology” (Wajcman, 2015). For instance, the introduction of the railroad in the nineteenth century has been identified as a driving force that greatly sped up the pace of life - although the railroad had started out as a time-saving endeavor in comparison to previous modes of transportation, such as walking, horses, carriages, or sailing ships (Schivelbusch, 2014). Against this background, it is interesting to investigate whether it is actually technologies' time-saving nature that increases the pace of life, in other words, whether time rebound mechanisms are a driving force of social acceleration. If this is the case, time-saving technologies are also likely to increase energy and resource consumption, as social acceleration includes more activities per given time frame. In our research (see Box 3) we found not only time rebound mechanisms but also inductions mechanisms.
Box 3. Our studies on the pace of life.
To empirically investigate the relation between digital technologies and the pace of life, we conducted a representative online survey (n = 1,393) in 2019 in Germany (Santarius and Bergener, 2020; Bergener and Santarius, 2021). For the independent variable, we focused on information and communication technologies (ICT), assuming that many ICT applications either intend to or, de facto, serve to “save time.”
Our study finds that any time efficiency improvements arising from applying ICT lead to spending the “saved time” in additional activities. The “degree of digitalization” among participants clearly correlated with the overall number of activities reported, and it was moderated by the time-saving nature of those technologies. Specifically, our data shows that individuals have a denser schedule partially because they use digital technology to (i) eliminate breaks, (ii) engage in increased multitasking, and (iii) replace time-intensive with time-saving activities. Note that our results suggest that time rebound mechanisms are not the only reason, and maybe not the main reason, for the phenomenon of an accelerated pace of life.
The application of digital technologies allows individuals to save time and thereby to conduct additional activities that are accompanied by some type of energy and resource consumption. This mechanism can be defined as a time rebound mechanism. In our empirical study, we do not find evidence for what may be called “direct time rebound effects,” i.e., that the time-saving nature of digital technologies is a causal predictor of longer hours spent on digital devices, or on the internet (Santarius and Bergener, 2020). Yet our analysis suggests an empirical proof of what may be called an “indirect time rebound effect,” i.e., that the time-saving nature of digital technologies is a causal predictor of more overall activities being performed during a day.
Another mechanism at play in the process of digital technologies leading to a faster pace of life is asynchronicity, which is due to several new options for time use. For instance, email, short message sending, and other forms of social media engagement allow quick and asynchronous interpersonal communication; e-commerce allows shopping irrespective of opening hours; and streaming TV allows news and movies to be watched at times different to those of the official broadcasting program. Hassan (2003) argues that time in the light of the internet should be conceptualized as “digitally compressed clock time” that enables “connected asynchronicity.”
Digital technologies bring about new options for asynchronous time use in two manners: First, activities can be performed whenever an individual wants; they become time-independent, e.g., shopping, communicating with friends, or watching news/movies becomes possible at nighttime. And second, individuals can more easily perform activities in parallel, which means they can improve on multitasking; e.g., shopping, communicating with friends, or watching news/movies all become possible at the same time. Note that such tendency toward multitasking is not a sole feature of digital technologies but could already be observed with the rise of previous media technologies, e.g., watching TV while doing the dishes or meeting friends etc. However, digital technologies, and in particular applications based on mobile internet, have both qualitatively and quantitatively increased the multitasking circumstances and fields of application.
In a similar manner, new options for time use arising from digital technologies enable forms of fragmentation. For instance, the act of shopping can be split into several phases, as each phase of the “consumer journey” (researching information, considering product alternatives, the actual purchasing, retention, etc.) can be conducted separately. Likewise, an interpersonal communication to discuss an issue, which may have taken 10 min face-to-face or via the phone, can be split into multiple short messages over an undefined period of time. Again, digital technologies open up two new options for fragmentation with regard to time use. First, they allow for instantaneity as certain “parts” of previously one activity can be conducted quicker and anytime. As Southerton (2020) states, the constant connectivity and instantaneity of the network society offer new opportunities and freedoms for individuals to form their own network-based times of interaction. Second, fragmentation of time use again allows multitasking to be improved as it allows activities to be squeezed into down times, waiting times, or transfer times. For example, one can research a product on the way to work, check on price alternatives on the way home, and conclude the online purchase later in the evening while watching TV.
To sum up, digital technologies bring about new options that allow for both asynchronicity and fragmentation in time use and, hence, allow individuals to increase the number of activities performed in a given day. Yet note that such an acceleration of the pace of life, as well as fragmenting activities or doing them in parallel to other activities, may also lead to feelings of time stress, as well as to alienation. These mechanisms may generate countervailing mechanisms, including a deceleration of the pace of life. More severe impacts of an overwhelming number of options may lead to depression or even burn-out, again decelerating rather than accelerating the pace of life (Rosa, 2013).
Accordingly, as far as a faster pace of life is concerned, increasing time-efficiency and time rebound effects are not the only outcomes of digital technologies. New options due to using digital technologies can lead to induction effects, e.g., by way of asynchronous and fragmented behavior, which also leads to more activities per day. In turn, it can be argued (but yet remains to be empirically investigated) that more activities per day may entail more energy and resource consumption.
The literature on the rebound effect has faced a serious trade-off. In some instances, the rebound effect is conceptualized relatively narrowly so that it can be clearly defined, a definition that places technological efficiency improvements as the premise of any rebound mechanism. But in that definition, important aspects of how technological change increases energy and resource consumption are left unconsidered. Alternatively, the rebound effect is defined broadly, with any development related to technologies' impacts on energy and resource demand efficiency being part of the rebound effect. In that definition, the concept of the rebound effect becomes fuzzy and of little use in the analysis of underlying mechanisms, their empirical investigation, and related policy recommendations on how to reduce the magnitude of rebound effects.
To solve this trade-off, we propose using the concept of the induction effect in order to keep a meaningful definition of the rebound effect while at the same capturing important mechanisms relating to technological change and energy and resource consumption. We suggest placing the emergence of new options as the premise of any induction mechanism. A specific technological change can either include both - efficiency improvements and new options - or only one of these two aspects and thereby also lead to either only one or both of the effects - rebound and induction.
The case studies described in the previous section indicate that a combined analysis of rebound mechanisms and induction mechanisms allows many more technology-related impacts on energy and resource demand to be captured. In fact, in the three cases, induction mechanisms appear to be more important than rebound mechanisms. For the e-commerce case, it remains unclear whether rebound mechanisms take place as the literature is ambiguous on whether online consumption actually increases efficiency. In contrast, induction mechanisms such as a wider product collection, being able to consume at any time and from anywhere, or the consumption-stimulating nature of online marketing clearly tend to raise consumption levels. For the smart home case, energy and resource consumption is increased most by additional digital household devices - which open up new options for action and consumption that would not be available if the home was not smart; in contrast, the empirical evidence on rebound mechanisms related to smart homes is inconclusive. For social acceleration, the increasing pace of life, both time rebound mechanisms and induction mechanisms, such as multitasking or conducting activities at times in the day that were formerly impossible, play important roles.
The case studies also indicate that there are different types of induction mechanisms. We propose differentiating induction mechanisms on two dimensions. First, the dimension related to what the new options are used for: whether they are used to buy, sell or do more of the same (quantity mechanisms - see Table 1) or to buy or sell new products or services or to do new activities (novelty mechanisms). Second, we differentiate whether these mechanisms take place on the production side or the consumption side; with a view to policy recommendations, this may allow to better identify which actors cause these mechanisms, e.g., firms or households/individuals. Table 1 gives an overview of the examples stemming from the cases discussed and some additional examples from digital technologies.
By way of these differentiations, we do not claim to provide an exhaustive collection of induction mechanisms. Rather we intend to spark further debate and scientific analysis on the phenomenon of the induction effect and anticipate that additional induction mechanisms will be identified in the future.
Taking induction mechanisms into account further strengthens the argument that sufficiency is needed, rather than focusing solely on efficiency. The reason is that induction mechanisms lead to more (quantity) and new (novelty) types of production and consumption, which may cause additional energy and resource consumption. The concept of sufficiency is well equipped for responding to both mechanisms as sufficiency takes a holistic view of how to live and produce within environmental limits while satisfying human needs. Implicit in this view is that neither efficiency gains nor new technological options should be used for generating additional consumption desires or activities, which may increase energy and resource use.
Including induction mechanisms also changes the analysis of necessary policy responses. A common policy recommendation from the rebound literature is that the savings from efficiency improvements need to be “taxed away or otherwise removed from further economic circulation” (Wackernagel and Rees, 1997). The idea is that, when the prices of energy and resources increase at the same speed as energy and resource efficiency improvements, the costs for firms and households stay the same and rebound effects can be prevented (von Weizsäcker et al., 2009).
If the induction effect is taken into account, it does not suffice to increase the price of energy and resources in line with efficiency improvements, as this response does not prevent firms from using new technological options to persuade consumers to buy more or from inventing new products that will be bought. Neither would it prevent households from using new options to conduct more or new activities. More appropriate in the induction case would be to think about politically inducing a reduction in the number of options. For instance, as digitalization in the transport sector has enlarged the number of options of how to get from one place to the other, e.g., by way of new forms of ride sharing, free-floating bike sharing or car sharing, e-scooters etc., politicians may find it easier to introduce legislation that reduces the number of options to use the private car, e.g., by way of restraining parking areas or establishing pedestrian zones. Or, for social acceleration, as digital tools now make it possible to work and be reachable anywhere and at any time, some firms have introduced policies that do not deliver emails outside working hours and restrict or prevent the reachability of employees via mobile phones after hours.
On the macroeconomic level, including induction effects into the analysis strengthens the argument for certain sufficiency-oriented policies. According to Callmer and Bradley (2021), a sufficiency orientation in policies for Global North economies and societies could be implemented through a policy of limits, a policy of less, a policy of slower and closer, and a policy of wellbeing. A concrete measure at the macroeconomic level is a reduction in working hours and thus also income (assuming that increases in hourly wages do not entirely compensate for the reduced working hours). Such reductions in income restrict the abilities of households to buy and conduct new options that go along with consumption. Hence, working hours reductions are a reasonable response to induction mechanisms. However, while reduced working hours may lead to a reduction in overall spending, it may not achieve a proportional reduction in energy use and emissions due to the associated changes in spending patterns. This is because households with more time and less money may allocate their financial and time resources differently (Sorrell et al., 2020). A shift toward more energy intensive activities can be addressed by increasing the price of such activities.
Therefore, a combination of policies geared toward addressing efficiency improvements (e.g., increasing prices of energy and resource consumption) and addressing new options (e.g., reduced average working hours) seems most effective in gearing the effects of technological change toward environmental sustainability.
The debate on efficiency, economic growth, environmental sustainability, and the rebound effect today is more timely than ever. The concept of the rebound effect is important in understanding the limitations of efficiency improvements to reduce energy and resource consumption. However, it leaves out important mechanisms via which technological change increases such consumption. Technological change brings with it several mechanisms that arise from the emergence of continuously new options, leading to additional production and consumption. We term the additional energy and resource consumption related to such mechanisms the induction effect.
Including the induction effect facilitates a better understanding of the relation between technological change and environmental sustainability. It broadens the view on necessary steps to reconcile technologies and the environment. It underpins the argument for sufficiency measures and opens up new debates on relevant policies. In this manner, the concept of the induction effect is one step to improving our conceptual toolkit on how to achieve sustainability.
The original contributions presented in the study are included in the article/supplementary material, further inquiries can be directed to the corresponding author.
SL wrote the first draft and finalized the manuscript. VF, MG, JP, FR, and TS wrote specific sections to the manuscript. SL and TS conceptualized the article. All authors contributed to the article and approved the submitted version.
Funding for this research was granted by the Robert Bosch Stiftung (grant number 01000579-001) and the German Federal Ministry of Education and Research (grant number 01UU1607B).
The authors declare that the research was conducted in the absence of any commercial or financial relationships that could be construed as a potential conflict of interest.
All claims expressed in this article are solely those of the authors and do not necessarily represent those of their affiliated organizations, or those of the publisher, the editors and the reviewers. Any product that may be evaluated in this article, or claim that may be made by its manufacturer, is not guaranteed or endorsed by the publisher.
Aebischer, B., and Hilty, L. M. (2014). “The energy demand of ict: A historical perspective and current methodological challenges,” in, ICT Innovations for Sustainability, Advances in Intelligent Systems and Computing (AISC), eds L. M. Hilty and B. Aebischer (Cham: Springer), 71–103.
Balta-Ozkan, N., Boteler, B., and Amerighi, O. (2014). European smart home market development: Public views on technical and economic aspects across the united kingdom, Germany and Italy. Energy Res. Soc. Sci. 3, 65–77. doi: 10.1016/j.erss.2014.07.007
Becker, G. S. (1965). A theory of the allocation of time. Econ. J. 75, 493–517. doi: 10.2307/2228949
Bergener, J., and Santarius, T. (2021). A pace of life indicator. Development and validation of a general acceleration scale. Time Soc. 30, 273–301. doi: 10.1177/0961463X20980645
Berry, M., Gibson, M. N., Nelson, A., and Richardson, I. (2007). “How Smart is ‘Smart'? Smart homes and sustainability,” in Steering Sustainability in an Urbanizing World: Policy, Practice and Performance. Ashgate (Aldershot), eds. A. Nelson (London: CRC Press), 239–252.
Best, B., Christ, M., Santarius, T., and Wiese, F. (2022). Exploring energy sufficiency: New challenges and options in times of crisis. TATuP - Z. Für Tech. Theor. Prax. 31, 11–17. doi: 10.14512/tatup.31.2.10
Binswanger, M. (2001). Technological Progress and Sustainable Development: What about the rebound effect? Ecol. Econ. 36, 119–132. doi: 10.1016/S0921-8009(00)00214-7
Bleier, A., and Eisenbeiss, M. (2015). The Importance of Trust for Personalized Online Advertising. J. Retail. 91, 390–409. doi: 10.1016/j.jretai.2015.04.001
Brenčič, V., and Young, D. (2009). Time-saving innovations, time allocation, and energy use: Evidence from Canadian households. Ecol. Econ. 68, 2859–2867. doi: 10.1016/j.ecolecon.2009.06.005
Buhl, J. (2016). Rebound-Effekte im Steigerungsspiel: Zeit- und Einkommenseffekte in Deutschland, Umweltsoziologie. Baden-Baden: Nomos.
Buhl, J., and Acosta, J. (2016). Work less, do less? Working time reductions and rebound effects. Sustain. Sci. 11, 261–276. doi: 10.1007/s11625-015-0322-8
Buldeo Rai, H. (2021). The net environmental impact of online shopping, beyond the substitution bias. J. Transp. Geogr. 93, 103058. doi: 10.1016/j.jtrangeo.2021.103058
Callmer, A., and Bradley, K. (2021). In search of sufficiency politics: The case of Sweden. Sustain. Sci. Pract. Policy 17, 196–209. doi: 10.1080/15487733.2021.1926684
Cramer-Flood, E. (2021). Worldwide Digital Ad Spending 2021: Surprisingly Resilient 2020 Presages Another Surge [WWW Document]. Insid. Intell. Available online at: https://www.insiderintelligence.com/content/worldwide-digital-ad-spending-2021 (accessed November 11, 2022).
Davis, L. W., Fuchs, A., and Gertler, P. J. (2012). Cash for Coolers (Working Paper No. 230), Energy Institute at Haas (EI @ Haas). Berkeley, CA: Energy Institute at Haas.
Dinner, I. M., van Heerde, H. J., and Neslin, S. A. (2014). Driving online and offline sales: The cross-channel effects of traditional, online display, and paid search advertising. J. Mark. Res. 51, 527–545. doi: 10.1509/jmr.11.0466
Druckman, A., Buck, I., Hayward, B., and Jackson, T. (2012). Time, gender and carbon: A study of the carbon implications of british adults' use of time. Ecol. Econ. Degrowth 84, 153–163. doi: 10.1016/j.ecolecon.2012.09.008
Eurostat (2022). Ordering or Buying Goods and Services [WWW Document]. Eurostat Stat. Explain. Available online at: https://ec.europa.eu/eurostat/statistics-explained/index.php?title=Digital_economy_and_society_statistics_-_households_and_individuals#Ordering_or_buying_goods_and_services (accessed November 18, 2022).
Font Vivanco, D., Freire-González, J., Galvin, R., Santarius, T., Walnum, H. J., and Makov, T. (2022). Rebound effect and sustainability science: A review. J. Ind. Ecol. 26, 1543–1563. doi: 10.1111/jiec.13295
Frick, V. (2022). An Environmental Psychology Perspective on Sufficiency-Oriented Consumption in Online Environments (Doctor Rerum Naturalium). Magdeburg: Otto von Guericke University, Magdeburg.
Frick, V., Gossen, M., and Kettner, S. E. (2022). Does online advertising stimulate overconsumption? ÖkologischesWirtschaften 37, 46–50. doi: 10.14512/OEW370146
Frick, V., and Matthies, E. (2020). Everything is just a click away. Online shopping efficiency and consumption levels in three consumption domains. Sustain. Prod. Consum. 23, 212–223. doi: 10.1016/j.spc.2020.05.002
Frick, V., Matthies, E., Thøgersen, J., and Santarius, T. (2020). Do Online Environments Promote Sufficiency or Overconsumption? Online advertisement and social media effects on clothing, digital devices, and air travel consumption. J. Consum. Behav. 20, 288–308. doi: 10.1002/cb.1855
Frick, V., and Nguyen, T. N. (2021). Energieeinsparung, Sicherheit, Technikbegeisterung und Konsumismus. Eine motivbasierte Typologisierung von Smart-Home NutzerInnen in Deutschland. Umweltpsychologie 25, 19–42.
Geiger, S. J., Brick, C., Nalborczyk, L., Bosshard, A., and Jostmann, N. B. (2021). More Green than Gray? Toward a sustainable overview of environmental spillover effects: A Bayesian meta-analysis. J. Environ. Psychol. 78, 101694. doi: 10.1016/j.jenvp.2021.101694
Gillingham, K., Kotchen, M. J., Rapson, D. S., and Wagner, G. (2013). The rebound effect is overplayed. Nature 493, 475–476. doi: 10.1038/493475a
Girod, B., and de Haan, P. (2009). Mental Rebound (No. EMDM 1522), Rebound Research Report Nr. 3. Zurich: IED-NSSI.
Gossart, C. (2015). “Rebound effects and ICT: A review of the literature,” in ICT Innovations for Sustainability, Advances in Intelligent Systems and Computing (AISC), eds L. M. Hilty and B. Aebischer (Cham: Springer), 435–448.
Haberl, H., Wiedenhofer, D., Virág, D., Kalt, G., Plank, B., Brockway, P., et al. (2020). A systematic review of the evidence on decoupling of GDP, resource use and GHG emissions, part II: Synthesizing the insights. Environ. Res. Lett. 15, 065003. doi: 10.1088/1748-9326/ab842a
Habibi, S. (2017). Micro-climatization and real-time digitalization effects on energy efficiency based on user behavior. Build. Environ. 114, 410–428. doi: 10.1016/j.buildenv.2016.12.039
Hahnel, U. J., Chatelain, G., Conte, B., Piana, V., and Brosch, T. (2020). Mental accounting mechanisms in energy decision-making and behaviour. Nat. Energy 5, 952–958. doi: 10.1038/s41560-020-00704-6
Hassan, R. (2003). Network time and the new knowledge epoch. Time Soc. 12, 226–241. doi: 10.1177/0961463X030122004
Herring, H. (2009). “Sufficiency and the rebound effect,” in Energy Efficiency and Sustainable Consumption: The Rebound Effect, eds H. Herring and S. Sorrell (London: Palgrave Macmillan), 224–239. doi: 10.1057/9780230583108_11
Herring, H., and Sorrell, S. (2009). Energy Efficiency and Sustainable Consumption: The Rebound Effect, Energy, Climate and the Environment Series. London: Palgrave Macmillan.
Hilty, L. M. (2008). Information Technology and Sustainability: Essays on the Relationship Between ICT and Sustainable Development. Norderstedt: Books on Demand.
Holm, S.-O., and Englund, G. (2009). Increased ecoefficiency and gross rebound effect: Evidence from USA and six european countries 1960–2002. Ecol. Econ. 68, 879–887. doi: 10.1016/j.ecolecon.2008.07.006
IEA 4E. (2018). Intelligent Efficiency. A case study of barriers and solutions - Smart Homes. Washington, DC: IEA.
IPCC. (2021). “Summary for policymakers,” in Climate Change 2021: The Physical Science Basis. Contribution of Working Group I to the Sixth Assessment Report of the Intergovernmental Panel on Climate Change, eds V. Masson-Delmotte, P. Zhai, A. Pirani, S.L. Connors, C. Péan, S. Berger, N. Caud, Y. Chen, L. Goldfarb, M. I. Gomis, M. Huang, K. Leitzell, E. Lonnoy, J. B. R. Matthews, T. K. Maycock, T. Waterfield, O. Yelekçi, R. Yu, and B. Zhou (Cambridge; New York, NY: Cambridge University Press), 3–32. doi: 10.1017/9781009157896.001
Jalas, M. (2002). A time use perspective on the materials intensity of consumption. Ecol. Econ. 41, 109–123. doi: 10.1016/S0921-8009(02)00018-6
Jiménez-Castillo, D., and Sánchez-Fernández, R. (2019). The role of digital influencers in brand recommendation: Examining their impact on engagement, expected value and purchase intention. Int. J. Inf. Manag. 49, 366–376. doi: 10.1016/j.ijinfomgt.2019.07.009
Kaack, L. H., Donti, P. L., Strubell, E., Kamiya, G., Creutzig, F., Rolnick, D., et al. (2022). Aligning artificial intelligence with climate change mitigation. Nat. Clim. Change 12, 518–527. doi: 10.1038/s41558-022-01377-7
Khazzoom, J. D. (1980). Economic implications of mandated efficiency in standards for household appliances. Energy J. 1, 21–40. doi: 10.5547/ISSN0195-6574-EJ-Vol1-No4-2
Khazzoom, J. D. (1987). Energy saving resulting from the adoption of more efficient appliances. Energy J. 8, 85–89. doi: 10.5547/ISSN0195-6574-EJ-Vol8-No4-8
Lange, S., Kern, F., Peuckert, J., and Santarius, T. (2021). The Jevons paradox unravelled: A multi-level typology of rebound effects and mechanisms. Energy Res. Soc. Sci. 74. doi: 10.1016/j.erss.2021.101982
Lange, S., Pohl, J., and Santarius, T. (2020). Digitalization and energy consumption. Does ICT reduce energy demand? Ecol. Econ. 176. doi: 10.1016/j.ecolecon.2020.106760
Lange, S., and Santarius, T. (2020). Smart Green World? Making Digitalization Work for Sustainability. London: Routledge.
Levine, R. V., and Norenzayan, A. (1999). The pace of life in 31 countries. J. Cross-Cult. Psychol. 30, 178–205. doi: 10.1177/0022022199030002003
Li, X., Zhao, X., and Xu, W. (2020). Measuring ease of use of mobile applications in e-commerce retailing from the perspective of consumer online shopping behaviour patterns. J. Retail. Consum. Serv. 55, 2093. doi: 10.1016/j.jretconser.2020.102093
Madlener, R., and Turner, K. (2016). “After 35 Years of rebound research in economics: Where do we stand?” in Rethinking Climate and Energy Policies, eds T. Santarius, H. J. Walnum, and C. Aall (Cham: Springer International Publishing), 17–36.
Marikyan, D., Papagiannidis, S., and Alamanos, E. (2019). A systematic review of the smart home literature: A user perspective. Technol. Forecast. Soc. Change 138, 139–154. doi: 10.1016/j.techfore.2018.08.015
Mickoleit, A. (2010). Greener and Smarter: ICTs, The Environment and Climate Change (OECD Green Growth Papers No. 2010/01). Paris: OECD.
Nicholls, L., and Strengers, Y. (2019). Robotic vacuum cleaners save energy? Raising cleanliness conventions and energy demand in Australian households with smart home technologies. Energy Res. Soc. Sci. 50, 73–81. doi: 10.1016/j.erss.2018.11.019
Nicholls, L., Strengers, Y., and Sadowski, J. (2020). Social impacts and control in the smart home. Nat. Energy 5, 180–182. doi: 10.1038/s41560-020-0574-0
Nilsson, A., Bergquist, M., and Schultz, W. P. (2017). Spillover effects in environmental behaviors, across time and context: A review and research agenda. Environ. Educ. Res. 23, 573–589. doi: 10.1080/13504622.2016.1250148
Parrique, T., Barth, J., Briens, F., Kerschner, C., Kraus-Polk, A., Kuokkanen, A., et al. (2019). Decoupling Debunked – Evidence and Arguments Against Green Growth as a Sole Strategy for Sustainability. London: European Environmental Bureau.
Pohl, J., Frick, V., Höfner, A., Santarius, T., and Finkbeiner, M. (2021). Environmental saving potentials of a smart home system from a life cycle perspective: How green is the smart home? J. Clean. Prod. 312, 845. doi: 10.1016/j.jclepro.2021.127845
Pohl, J., Hilty, L. M., and Finkbeiner, M. (2019). How LCA contributes to the environmental assessment of higher order effects of ICT application: A review of different approaches. J. Clean. Prod. 219, 698–712. doi: 10.1016/j.jclepro.2019.02.018
Quitzow, L., and Rohde, F. (2021). Imagining the smart city through smart grids? Urban energy futures between technological experimentation and the imagined low-carbon city. Urban Stud. 59, 341–359. doi: 10.1177/00420980211005946
Rattle, R. (2010). Computing Our Way to Paradise? The Role of Internet and Communication Technologies in Sustainable Consumption and Globalization. Lanham, MD: Rowman and Littlefield
Rehm, T. W., Schneiders, T., Strohm, C., and Deimel, M. (2018). “Smart Home Field Test–Investigation of Heating Energy Savings in Residential Buildings,” in 7th International Energy and Sustainability Conference (IESC). Cologne: IEEE, 1–8.
Reimers, H., Jacksohn, A., Appenfeller, D., Lasarov, W., Hüttel, A., Rehdanz, K., et al. (2021). Indirect rebound effects on the consumer level: A state-of-the-art literature review. Clean. Responsible Consum. 3, 100032. doi: 10.1016/j.clrc.2021.100032
Riekstin, A. C., Langevin, A., Dandres, T., Gagnon, G., and Cheriet, M. (2020). Time series-based GHG emissions prediction for smart homes. IEEE Trans. Sustain. Comput. 5, 134–146. doi: 10.1109/TSUSC.2018.2886164
Rockström, J., Gupta, J., Qin, D., Lade, S. J., Abrams, J. F., Andersen, L. S., et al. (2023). Safe and just Earth system boundaries. Nature 31, 1–10. doi: 10.1038/s41586-023-06083-8
Rohde, F., and Santarius, T. (2023). Emerging Sociotechnical Imaginaries – How the smart home is legitimized in visions from industry, users in homes and policymakers in Germany. Future. 1, 103194. doi: 10.1016/j.futures.2023.103194
Rohde, F., von Andrian, N., and Lange. (under review). Threat Useless or Fixable? Contested Smart Home Futures in the German Social Media Debate. Energy Research & Social Science.
Røpke, I. (2012). The unsustainable directionality of innovation – The example of the broadband transition. Res. Policy 41, 1631–1642. doi: 10.1016/j.respol.2012.04.002
Røpke, I., Christensen, T. H., and Jensen, J. O. (2010). Information and Communication Technologies – A new round of household electrification. Energ. Policy Sec. Conc. Ind. Reg. Papers 38, 1764–1773. doi: 10.1016/j.enpol.2009.11.052
Rosa, H. (2013). Social Acceleration: A New Theory of Modernity, New Directions in Critical Theory. Columbia, NY: Columbia University Press.
Santarius, T. (2015). Der Rebound-Effekt: Ökonomische, psychische und soziale Herausforderungen für die Entkopplung von Wirtschaftswachstum und Energieverbrauch, Wirtschaftswissenschaftliche Nachhaltigkeitsforschung. Marburg: Metropolis-Verlag.
Santarius, T. (2016). Investigating meso-economic rebound effects: Production-side effects and feedback loops between the micro and macro level. J. Clean. Prod. 134, 406–413. doi: 10.1016/j.jclepro.2015.09.055
Santarius, T., and Bergener, J. (2020). “Does the Use of ICT speed up the Pace of Life?” in Proceedings of the 7th International Conference on ICT for Sustainability. Presented at the ICT4S2020, 7th. International Conference on ICT for Sustainability. Bristol: ACM, 107–115. doi: 10.1145/3401335.3401642
Santarius, T., and Soland, M. (2018). How technological efficiency improvements change consumer preferences: Towards a psychological theory of rebound effects. Ecol. Econ. 146, 414–424. doi: 10.1016/j.ecolecon.2017.12.009
Santarius, T., Walnum, H. J., and Aall, C. (2016). Rethinking Climate and Energy Policies: New Perspectives on the Rebound Phenomenon. Cham: Springer International Publishing.
Schivelbusch, W. (2014). The Railway Journey: The Industrialization of Time and Space in the nineteenth century. Oakland, CA: University of California Press.
Shove, E. (2003). Users, technologies and expectations of comfort, cleanliness and convenience. Innov. Eur. J. Soc. Sci. Res. 16, 193–206. doi: 10.1080/13511610304521
Sintov, N. D., and Schultz, P. W. (2017). Adjustable green defaults can help make smart homes more sustainable. Sustainability 9, 622. doi: 10.3390/su9040622
Smidfelt Rosqvist, L., and Hiselius, L. W. (2016). Online shopping habits and the potential for reductions in carbon dioxide emissions from passenger transport. J. Clean. Prod. 131, 163–169. doi: 10.1016/j.jclepro.2016.05.054
Sorrell, S. (2007). The Rebound Effect: An Assessment of the Evidence for Economy-Wide Energy Savings From Improved Energy Efficiency. London: UK Energy Research Centre London.
Sorrell, S., and Dimitropoulos, J. (2007). UKERC Review of Evidence for the Rebound Effect: Technical Report 5. London: UK Energy Research Centre London.
Sorrell, S., Gatersleben, B., and Druckman, A. (2020). The Limits of Energy Sufficiency: A review of the evidence for rebound effects and negative spillovers from behavioural change. Energy Res. Soc. Sci. 64, 101439. doi: 10.1016/j.erss.2020.101439
Southerton, D. (2020). Time, Consumption and the Coordination of Everyday Life. London: Palgrave Macmillan.
Sovacool, B., and Furszyfer Del Rio, D. D. (2020). Smart home technologies in europe: A critical review of concepts, benefits, risks and policies. Renew. Sustain. Energy Rev. 120, 109663. doi: 10.1016/j.rser.2019.109663
Steffen, W., Richardson, K., Rockström, J., Cornell, S. E., Fetzer, I., and Bennett, E. M. (2015). Planetary boundaries: Guiding human development on a changing planet. Sci. Res. Article Summ. 347, 736. doi: 10.1126/science.1259855
Strengers, Y., Hazas, M., Nicholls, L., Kjeldskov, J., and Skov, M. B. (2020). Pursuing pleasance: Interrogating energy-intensive visions for the smart home. Int. J. Hum.-Comput. Stud. 136, 102379. doi: 10.1016/j.ijhcs.2019.102379
Tirado Herrero, S., Nicholls, L., and Strengers, Y. (2018). Smart home technologies in everyday life: Do they address key energy challenges in households? Curr. Opin. Environ. Sustain. 31, 65–70. doi: 10.1016/j.cosust.2017.12.001
Truelove, H. B., Carrico, A. R., Weber, E. U., Raimi, K. T., and Vandenbergh, M. P. (2014). Positive and negative spillover of pro-environmental behavior: An integrative review and theoretical framework. Glob. Environ. Change 29, 127–138. doi: 10.1016/j.gloenvcha.2014.09.004
Turner, K. (2013). “Rebound” effects from increased energy efficiency: A time to pause and reflect. Energy J. 34, 25–34. doi: 10.5547/01956574.34.4.2
van Dam, S. S., Bakker, C. A., and Buiter, J. C. (2013). Do home energy management systems make sense? Assessing their overall lifecycle impact. Energy Policy 63, 398–407. doi: 10.1016/j.enpol.2013.09.041
van den Bergh, J. C. J. M. (2011). Energy Conservation more effective with rebound policy. Environ. Resour. Econ. 48, 43–58. doi: 10.1007/s10640-010-9396-z
Verhallen, T. M. M., and Pieters, R. G. M. (1984). Attitude theory and behavioral costs. J. Econ. Psychol. 5, 223–249. doi: 10.1016/0167-4870(84)90024-2
von Weizsäcker, E. U., Hargroves, K. J., Smith, M. H., Desha, C. J. K., and Stasinopoulos, P. (2009). Factor Five: Transforming the Global Economy Through 80% Improvements in Resource Productivity, Factor Five - Transforming the Global Economy Through a 5X Fold Increase in Energy and Resource Productivity. London: Earthscan Routledge.
Voropanova, E. (2015). Conceptualizing smart shopping with a smartphone: Implications of the use of mobile devices for shopping productivity and value. Int. Rev. Retail Distrib. Consum. Res. 25, 529–550. doi: 10.1080/09593969.2015.1089304
Wackernagel, M., and Rees, W. E. (1997). Perceptual and structural barriers to investing in natural capital: Economics from an ecological footprint perspective. Ecol. Econ. 20, 3–24. doi: 10.1016/S0921-8009(96)00077-8
Wajcman, J. (2015). Pressed for Time: The acceleration of life in digital capitalism. Chicago: The University of Chicago Press.
Walnum, H. J., and Andrae, A. S. G. (2016). “The Internet: Explaining ICT service demand in light of cloud computing technologies,” in Rethinking Climate and Energy Policies: New Perspectives on the Rebound Phenomenon, eds T. Santarius, J. H. Walnum, and C. Aall (Cham: Springer International Publishing),227–241.
Walzberg, J., Dandres, T., Samson, R., Merveille, N., and Cheriet, M. (2017).” An Agent-Based Model to Evaluate Smart Homes Sustainability Potential”, in Personal, Indoor, and Mobile Radio Communications (PIMRC). Presented at the 2017 IEEE 28th Annual International Symposium on Personal, Indoor, and Mobile Radio Communications (PIMRC), Manhattan, NY: IEEE,1–7.
Wang, R. J. H., Malthouse, E. C., and Krishnamurthi, L. (2015). On the go: How mobile shopping affects customer purchase behavior. J. Retail. 91, 217–234. doi: 10.1016/j.jretai.2015.01.002
Werg, J., Scheffler, D., and Hilscher, M. (2021). Jugend und Konsum: Entwicklung von Aktivierungsstrategien für Nachhaltigen Konsum mit Hilfe des Design Thinking Ansatzes (Abschlussbericht No. TEXTE 42/2021). Dessau-Roßlau: Umweltbundesamt.
Wiedenhofer, D., Virág, D., Kalt, G., Plank, B., Streeck, J., Pichler, M., et al. (2020). A systematic review of the evidence on decoupling of GDP, resource use and GHG emissions, part I: Bibliometric and conceptual mapping. Environ. Res. Lett. 15, 063002. doi: 10.1088/1748-9326/ab8429
Wolf, M., Emerson, J., Esty, D., De Sherbinin, D, and Wendling, A. Z. (2022). Environmental Performance Index (Environmental Performance Index). New Haven, CT: Yale Center for Environmental Law and Policy, New Haven.
Zafar, A. U., Shen, J., Shahzad, M., and Islam, T. (2021). Relation of impulsive urges and sustainable purchase decisions in the personalized environment of social media. Sustain. Prod. Consum. 25, 591–603. doi: 10.1016/j.spc.2020.11.020
Keywords: rebound effect, technological change, sustainability, induction effect, online consumption, smart home, acceleration
Citation: Lange S, Frick V, Gossen M, Pohl J, Rohde F and Santarius T (2023) The induction effect: why the rebound effect is only half the story of technology's failure to achieve sustainability. Front. Sustain. 4:1178089. doi: 10.3389/frsus.2023.1178089
Received: 02 March 2023; Accepted: 16 August 2023;
Published: 18 September 2023.
Edited by:
Manu V. Mathai, Azim Premji University, IndiaReviewed by:
Sylvia Lorek, Sustainable Europe Reserch Institute, GermanyCopyright © 2023 Lange, Frick, Gossen, Pohl, Rohde and Santarius. This is an open-access article distributed under the terms of the Creative Commons Attribution License (CC BY). The use, distribution or reproduction in other forums is permitted, provided the original author(s) and the copyright owner(s) are credited and that the original publication in this journal is cited, in accordance with accepted academic practice. No use, distribution or reproduction is permitted which does not comply with these terms.
*Correspondence: Steffen Lange, bGFuZ2VAd2l3aS51bmktc2llZ2VuLmRl
Disclaimer: All claims expressed in this article are solely those of the authors and do not necessarily represent those of their affiliated organizations, or those of the publisher, the editors and the reviewers. Any product that may be evaluated in this article or claim that may be made by its manufacturer is not guaranteed or endorsed by the publisher.
Research integrity at Frontiers
Learn more about the work of our research integrity team to safeguard the quality of each article we publish.