- 1Circular Economy Research Programme, African Leadership University, Kigali, Rwanda
- 2African Centre of Excellence in Data Science, University of Rwanda, Kigali, Rwanda
Food security remains a challenge for Africa and regenerative agricultural practices can play an important role in building resilience while sustaining agricultural production and preserving diversity of ingredients and rich biodiversity. This study conducted surveys in eight regions in East Africa, four in Rwanda and four in Kenya, to understand how circular principles are applied in agriculture, potential drivers of the adoption of regenerative agriculture and its contribution to household food security. A binary logistic regression model was used to capture the influence of independent variables on the adoption of regenerative agricultural practices and determining factors of household food security. The findings suggest that farming households engage in less than four different practices, mainly in less labor-intensive practices such as crop rotation and intercropping. Household food security increased with an increased number of regenerative agricultural practices applied by the household and increased frequency of farm visits by extension agents. Regenerative agriculture practices, when applied in combinations, have the potential to increase household food security. This study recommends designing scaling-up pathways to accelerate the transition to regenerative agriculture and build a more resilient food system in East Africa.
1. Introduction
Agriculture remains an important source of livelihood for most Africans (Shimeles et al., 2018). The growing food demand is exacerbated by environmental challenges such as climate change, land degradation, biodiversity loss, and resource scarcity that are increasingly pressurizing the agricultural system, and impacting food security (Godfrey, 2021).
Over the last decades, Africa's population growth, among other factors, has made it a net food importer (FAO, 2012). A default response has been the use of intensive farming practices and synthetic inputs, which are associated with short-term productivity gains but long-term negative impacts on farmer health and the environment, in particular soil, biodiversity, and climate (EMF, 2019). The Food and Agricultural Organization of the United Nations predicts that the decline in agricultural production is likely to affect food availability in sub-Saharan Africa and South Asia where the majority of the population is already vulnerable due to poverty and has low coping strategies to environmental stresses (FAO, 2009). By 2030, food demand is projected to increase by 55 % (van Dijk et al., 2021). Such demand will not match climate change and the frequency of extreme weather events (EMF, 2021).
Food insecurity will remain a challenge to Africa if the continent continues to follow a resource-intensive, linear path, focused on short-term efficiency gains. Crop production and food security systems need to become more adaptive as uncertainties of projected climate variability and change unfold (Kogo et al., 2021).
A model shift in the agricultural sector is required from a take-make-dispose system toward one in which resources are maintained at their highest value possible, i.e., circular economy (CE) (Preston and Lehne, 2017). The CE is increasingly recognized as a key driver of a post-pandemic economic recovery, and a new source of more sustained and resilient economic growth, particularly in developing countries (Godfrey, 2021). It also provides an opportunity to build more resilient, sustainable economies that are better able to withstand future shocks–not only pandemics; but climate-induced natural disasters such as droughts and floods, and resource shortages, all of which are predicted to increase in both frequency and intensity in future (Newton et al., 2020). When applied to the agriculture sector, the CE principles of designing out waste, closing resource loops, and regenerating natural systems, provide a framework to address the food security challenge (Godfrey, 2021). For instance, a CE for food strives to have a positive impact on people, planet, and business through the application of three principles: (1) eliminating waste through food loss reduction and low dependence on external inputs, (2) keeping materials in use through food waste valorization, and (3) regenerating natural systems through regenerative food production (Boon and Anuga, 2020; Godfrey, 2021). These principles suggest that regenerative agriculture circular in nature. It centers on a regenerative system, with the production of agricultural commodities using a minimal amount of external inputs; decoupling production and processing from resource utilization; closing nutrient loops; restoring soil fertility; and reducing discharges to the environment (Godfrey, 2021). Regenerative agriculture is defined as an approach to farming that uses soil conservation as the entry point to regenerate and contribute to multiple provisioning, regulating and supporting ecosystem services, with the objective that this will enhance not only the environmental, but also the social and economic dimensions of sustainable food production (Schreefel et al., 2020). If practiced on a wide scale, regenerative agriculture can reduce resource requirements (e.g., water, energy), land use, chemical fertilizers, synthetic pesticides, greenhouse gas (GHG) emissions, and ultimately the ecological footprint of agriculture.
Transitioning to a more regenerative agricultural system supports the circular economy concept for regenerative agriculture reduces agricultural waste outputs and environmental pollution, keeps agricultural materials in use for the longest possible time, and focuses on restoring soil health to regenerate natural systems. Thus, creating very real social, economic and environmental benefits (Newton et al., 2020; Schreefel et al., 2020). These include improved food security, resilience, livelihoods, biodiversity conservation, global competitiveness; economic development, job creation, decarbonizing the sector, sustainable resource utilization, and sustainable food systems (Boon and Anuga, 2020; Godfrey, 2021; Winowiecki et al., 2021). Transitioning the sector from a linear to a circular one is a necessity to support food security and to unlock new economic opportunities (Preston and Lehne, 2017; Abad-Segura et al., 2020; Whyte et al., 2020). It is clear that the principles of a CE are not new to the agricultural sector in Africa. For instance, a study on the potential of a CE in Rwanda revealed opportunities that apply CE principles and are not necessarily termed ‘circular economy' initiatives (Whyte et al., 2020). Another study concluded that some circular economy practices are already implemented in Rwanda's food system through practices such as using organic waste as an organic fertilizer or as animal feed (Nijman, 2020). Elsewhere, farmers in Zambia, Malawi, and Ghana have been applying CE principles such as mixed farming, agroforestry, and crop-livestock integrated farming to enhance their farm productivity (Sosola et al., 2011; Boon and Anuga, 2020).
Eastern Africa presents a unique opportunity to transition to regenerative agriculture as its population largely depends on agriculture (AGRA, 2017; Nakawuka et al., 2018). Countries in Eastern Africa have developed plans that focus on economic development, which already hint at CE principles. The endorsement of the East African Organic Product Standards (EAOPS) by the East African Community is a step toward circularity (UNEP, 2010). A recent report indicated that Rwanda has an opportunity to focus on regenerative agriculture and support farmers who rely on organic fertilizers (Whyte et al., 2020). The Strategic Plan for Agricultural transformation for Rwanda supports the increase in organic fertilizer production and utilization as part of integrated soil fertility management practices in conjunction with the gradual liberalization of the mineral fertilizer supply (MINAGRI., 2018). In Kenya, there is a strong presence of private sector engagements in the development of the ecological organic agriculture sub-sector that applies circular principles (Ozor and Nyambane, 2021). The Kenya Organic Agriculture Network (KOAN), the Participatory Ecological Land Use Management (PELUM) Association, and many non-governmental organizations (NGOs) among others have played critical roles in the development of the sub-sector and particularly in the development of the draft National Organic Policy, which is yet to be gazetted (Ozor and Nyambane, 2021).
Despite numerous efforts to identify and promote “sustainable” agricultural innovations including farming practices among smallholders, outcomes have been often disappointing with low adoption. To date, there are rather only a few studies from Eastern Africa which examine how agricultural innovations are adopted. Furthermore, information is still lacking on the drivers that have made some countries succeed in applying circular agriculture practices, and the constraints they face to scale up such practices to larger impacts (Meijer et al., 2015; Liyama et al., 2018). However, such studies have often focused on the adoption of specific regenerative agriculture practices, not a set of practices, and they do not present the role of these practices in alleviating food insecurity. Additionally, little is known about the technical know-how of farming communities and actors along agricultural value chains with regard to regenerative agriculture. A recent study in Rwanda recommended research to generate information and disseminate knowledge on regenerative agriculture, agricultural waste valorization, food waste, and organic fertilizers to inform policy and practices of circular agriculture (Whyte et al., 2020).
The general objective of this study is to assess agricultural practices in Rwanda and Kenya. Practices that are aligned with three CE principles: designing out waste and pollution, keeping products and materials in use, and regenerating natural systems, in the framework of addressing food and nutrition security (EMF, 2021). Accelerating regenerative agriculture requires the best information available on previous and current experiences with regenerative agricultural practices. The two specific objectives of this study are: (1) to assess factors that determine the adoption of regenerative agricultural practices, and (2) to assess factors of food security among households that apply regenerative agricultural practices. The full potential of regenerative agricultural practices in improving food security is possible if factors enhancing the adoption of circular practices are well understood and considered in designing an appropriate scaling-up pathway.
2. Materials and methods
2.1. Study area
The project was implemented in two East African countries, Rwanda and Kenya. Kenyan and Rwandan populations depend on agriculture for livelihoods, contributing 22 and 23% to national GDP, respectively (CBK, 2022; MINAGRI, 2022). Both countries have taken considerable steps toward CE and demonstrate readiness for transition. Kenya is one of the leading CE hotspots in East and Central Africa (NEA, 2021) and Rwanda is the first country in Africa to develop a national circular economy action plan with agriculture as one of four priority sectors (Mo, 2020). Respondents were selected from eight sites, four in Rwanda and four in Kenya. They were contrasted according to agroecological conditions, two sites in each country were in low-altitude semi-arid areas. This study selected Ngarama and Rwimbogo sectors of Gatsibo District as low-attitude semi-areas for Rwanda, and in Makueni and Kilome sub-counties in Makueni County for Kenya. The other two sites in each country were selected from higher altitude areas. The Kibumbwe and Buruhukiro Sectors of Nyamagabe District in Rwanda have been selected as higher altitudes, and Molo and Njoro sub-counties in Nakuru County in Kenya. This study made the assumption that farmers who practice agriculture near protected areas would develop more understanding of principles of regeneration than farmers who practice agriculture far from protected areas (FAO, 2014; Farkas and Kovács, 2020). Therefore, one group of sites was also selected according to their nearness distance to protected areas, while other sides were chosen far away from protected areas with the purpose to compare the results of the different geographical sites. In Rwanda, data was collected in Rwimbogo sector near Akagera National Park (ANP), Ngarama Sector far from ANP, Buruhukiro sector near Nyungwe National Park (NNP), and Kibumbwe sector far from NNP. In Kenya, data was collected in Molo near Mariashoni Reserve Forest (MRF), Njoro far from MRF in Nakuru county, Kilome landscapes surrounding Kilungu Forest Reserve, and Makueni far from KFR in Makueni county.
2.2. Survey approach and data collection
Data were collected in two phases. The first phase was secondary data mining through a comprehensive literature review to collect relevant information from reports, manuals, research papers, theses, textbooks, and internet sources. A structured questionnaire was used to facilitate the collection of information from smallholder farmers through a household survey. Questions in the questionnaire were designed on the respondent's profile, farm plot profile, agricultural inputs, applied regenerative agricultural practices, marketing and income, food and nutritional security, and knowledge and capacity building on regenerative agriculture. Data was collected using tablets with Open Data Kit (Carl et al., 2010). A random sample of 30 farmers in each site was selected and snowball sampling was used in this study (Noy, 2008). There are sites that had slightly less or more than 30 farmers, making 245 farming households in total that participated in the survey.
2.3. Data analysis
The adoption of CE practices in regenerative agriculture can be measured at three different levels according to Saidani et al. (2019): (i) the macro-level refers to an investigation at the scale of the city, province, region or nation; (ii) the meso-level fits with eco-industrial parks; and (iii) the micro-level corresponds to a single company or consumer investigations. This study focused on the micro-level where CE principles application were assessed from farm level. Data are from one agricultural season. Data were analyzed using explorative methods (descriptive, correlation and non-parametric). Interest variables were food security and the adoption of regenerative agricultural practices. Hypothesis testing was done using Chi-Square and Independent sample t-test (Agresti, 2007; Field, 2018). A Chi-square test was used for categorical variables, t-test was used for quantitative variables to test the difference between two groups for both food security and adoption as interest variables. Since the outcome variable was binary, we used binary logistics regression to build two models that assess determining factors of food security and adoption for all respondents (Kleinbaum and Klein, 2002). Since the outcome variable for food security model was being food secure, the reference category was being food insecure. The same applies for adoption of regenerative practices. The developed model was as follows:
Where: P: probability of success in our case being food secure or being an adopter, and 1-P : probability of failure in our case being food insecure or being a non-adopter. Data management was done with Microsoft Excel while other tests were conducted with Python (McKinney, 2012).
For this model, we tested multicollinearity on quantitative variables of food security and adoption by assessing the Variance Inflation Factor (VIF). The VIF was 4.62, 3.2, and 4.61 for the number of farm plots, the number of regenerative practices, and the number of household members, respectively. They had no multicollinearity problem because VIF was less than five (O'Brien, 2007; Hair et al., 2010). Secondly, multi-collinearity was tested for categorical variables with a chi-square test. The relationship between livestock owners and another source of income and between livestock owners and not trained but practicing farmers, and between other sources of income and not trained practicing farmers were not statistically significant, p-values were 0.35, 0.58, 0.83, respectively. Thus, there was no multicollinearity among categorical variables. Thirdly, we compared categorical variables with quantitative variables, using an independent sample t-test. Comparing livestock owners with the number of practices, household members, and the number of plots (p-values 0.0018, 0.005, and 0.58, respectively), livestock owners were related to the number of practices and household members but not correlated with the number of plots. Comparing other sources of income with the number of practices, household members, and the number of plots (p-values 0.0070, 0.17, and 0.06, respectively), having another source of income was related to the number of practices but not to the number of plots and household members. Comparing the practitioners that had not been trained with the number of practices, household members, and the number (p-values 0.19, 0.56, and 0.45, respectively), farmers who had not been trained but practiced regenerative agriculture were not correlated with the number of plots and household members. Even though there are some categorical variables that have significant relationships with some quantitative variables, the same variables were not statistically significant in relation to other similar variables used in the models. Therefore, the three methods show that the variables used in the models had no multicollinearity problems.
3. Results
The findings of this study were from 245 farming households (Table 1). The respondents were generally smallholder farmers growing subsistence crops such as maize, beans and Irish potatoes, owning livestock such as cows (139), goats (65), sheep (16), chicken (42), and pigs (10). About 45 % of respondents were female and 75.2 % of respondents were married living with spouses while 11.6% were widows or widowers, mostly male-headed households. The average age of respondents was 46 years. When this study asked about the status of their farm fertility, only 23.9% of respondents thought their farm fertility was high, 61.4% reported moderate fertility.
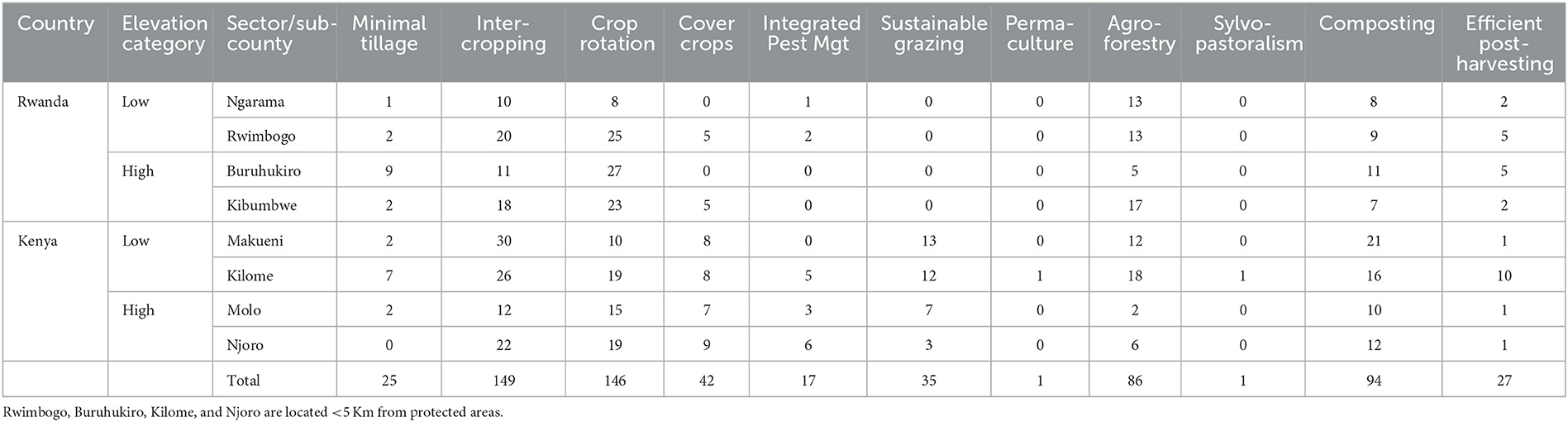
Table 1. Regenerative agricultural practices disaggregated according to sectors and sub-counties in Rwanda and Kenya.
The survey investigated the application of 11 different regenerative agricultural practices including: crop rotation, minimal or zero tillage including fallowing, intercropping, agroforestry, cover crops and mulching, composting, post-harvest loss practices, sustainable grazing, permaculture, sylvopastoralism, and integrated pest management. The most dominant practices were mainly practices with low-cost implications such as crop rotation and intercropping (60.8 and 61.6%, respectively).
Apart from Makueni and Kilome sub-counties, the average number of regenerative practices applied by farming households was two (Figure 1). Households in Rwanda apply between four and six regenerative agricultural practices in most cases, crop rotation, intercropping, agroforestry and composting (68.8, 48.8, 39.2, and 28%, respectively). Farming households in Makueni in Kenya had been more exposed to regenerative agriculture than households in other study sites and farmers could be applying more than six different practices on the same farm plot.
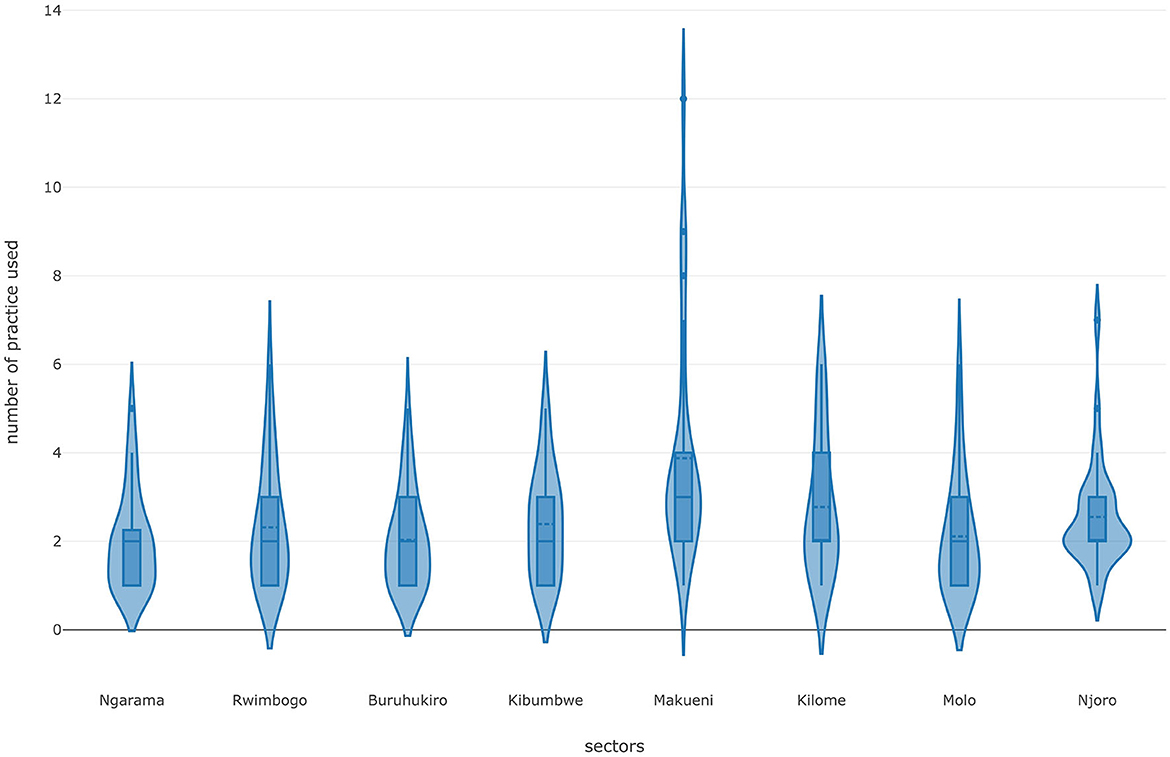
Figure 1. Number of regenerative agricultural practices applied in Njoro, Molo, Kilome, and Makueni sub-counties in Kenya and Kibumbwe, Buruhukiro, Rwimbogo, and Ngarama sectors in Rwanda.
3.1. Determining factors of adoption of regenerative practices
Given the number of regenerative agricultural practices that were being applied by farming households, this study classified them into three different categories, the lowest was applying at least two practices, the medium could apply up to three practices while the highest category was applying more than four different practices on combined farm plots. Farmers were also asked how confident they were in applying different practices without external support. Farmers' readiness was ranked on a scale from one to ten. The farmers were thereafter classified into three categories, too. This includes the categories low readiness from zero to five, medium readiness from 5 to 7.5 and high readiness from 7.5 to 10. Farmers who have received training on regenerative agricultural practices in the past and were still applying the practices on their farms were recorded as “trained practicing.” A category of farmers who have not received any training on regenerative agricultural practices but who applied the practices on their farms was recorded as “untrained practicing.” Both ‘trained practicing' and “untrained practicing” were classified as “continued practitioner.” To understand to what extent farmers have applied regenerative agricultural practices on their farms, this study calculated the practice over farm area ratio. This research divided the practice area by total farm size and converted the quotient into percentages. The practice over farm area ratio was therefore classified as low between 0 and 50%, medium between 50 and 75%, and high between 75 and 100%. For comparability and scalability, the reported farm area size was converted from square meters to hectares (m2 to Ha). Therefore, non-adopters of regenerative agricultural practices were farmers who applied two or less than two practices on a low practice over farm size ratio, i.e., <50% of the total farm size. Adopters were farmers who applied more than two regenerative agricultural practices on a medium or high practice over farm size ratio, i.e., more than 50% of the total farm size.
This study built the following model to assess determining factors for the adoption of regenerative agricultural practices (see outputs in Table 2):
Where γ is farmer participation in training on regenerative agricultural practices, σ indicates the frequent visit of extension agents to farmers, μ indicates farmer's skills readiness, ϵ indicates the use of inorganic fertilizers, π indicates household food security status, α indicates nearness to a protected area, β indicates farmers who apply regenerative agricultural practices with no training.
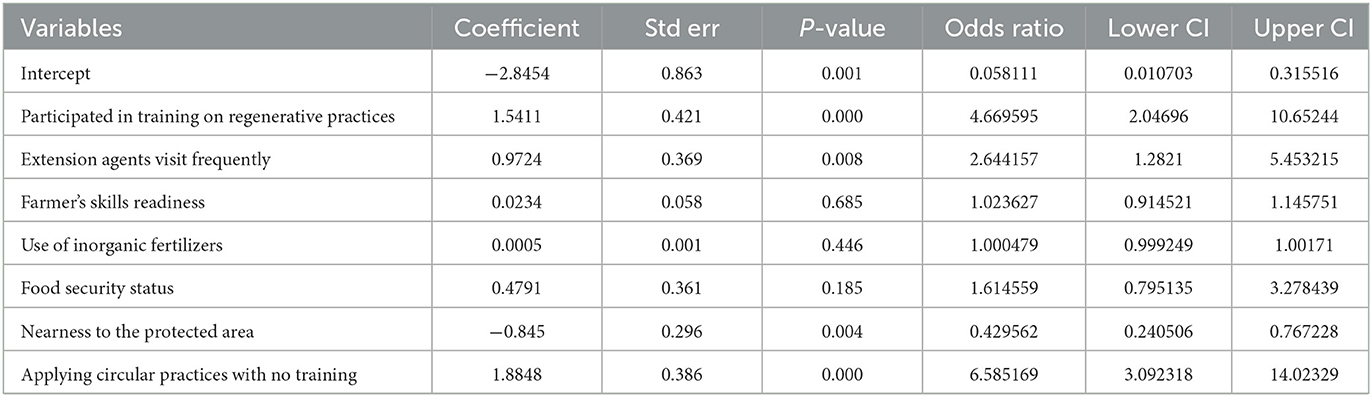
Table 2. Statistical results on factors that can influence the adoption of regenerative agricultural practices.
The model outputs have revealed that farmers who participated in training on regenerative agricultural practices were 4.67 times more likely to adopt regenerative agricultural practices than the farmers who have not participated in any training (P = 0.000) (Table 2). The adoption was not only associated with training but also with the frequency of visits of extension agents to farming households. The odds of a farmer who occasionally received extension services adopting regenerative agricultural practices is 2.65 times more than a farmer who received no extension services (P = 0.008). However, this research found no significant direct association between a farmer's skills readiness and their adoption of the practices. The odds of becoming an adopter were 1.02 and were not statistically different (P = 0.68). For farming households that buy and use inorganic fertilizers, this study could not find enough evidence of the influence on adoption. Their odds to be adopters were multiplied by 1.0005 and were not statistically different (P = 0.446). Moreover, household food security status could not explain household adoption of regenerative agricultural practices for they were not statistically different (P = 0.185). The odds were 1.61. Contrary to our assumption, living near protected areas did not translate into adopting regenerative practices in farming. A farmer who lives near a protected area was 0.43 less likely to adopt regenerative agricultural practices than farmers who live far from the protected area (P = 0.01). Interestingly, farming households who have not received training on regenerative agricultural practices were 6.6 times more likely to apply the practices than farmers who were trained (P = 0.000) (Table 2).
3.2. Determining factors of food security
Conscious of the definition of food security by the Food and Agriculture Organization (FAO, 2008), households were asked to indicate their food security status over a period of 12 months in three categories: (1) not having enough food for the family, (2) having enough food but not enough variety, and (3) having enough food and enough variety. Responses were coded as binary to support statistical computations, thus combining categories 2 and 3 into one category that was ranked 1 to indicate food secure household. Category 1 was thereafter coded as 0 to indicate food-insecure households. The household food security status was considered as the average of all 12 months. About 78.9% of surveyed households were ranked as food secure and 21.1% ranked as food insecure. This study tested variables that could influence household food security, using a binary logistic regression model (see outputs in Table 3):
Where α represents owning livestock, β represents other income sources, γis the number of regenerative agricultural practices, σ represents the number of household members, μ represents the number of farm plots, and ϵ is for farmers who apply regenerative agriculture practices with no training.
The number of regenerative agriculture practices applied by a farmer had a significant positive influence on household food security status (Table 3). For each added practice, a household became 1.36 more likely to be food secure (P = 0.048). Food security increased with the increased number of farm plots. For a unit increase in the number of plots owned by a farmer, their household was 1.89 times more likely to be food secure (P = 0.01). Having another source of income and being food secure were not statistically different (P = 0.081). Therefore, having another source of income could not explain the food security status of a household. The odds were 0.50. From the findings, this study has little evidence that farming households that apply regenerative agricultural practices without training on the practices could be more food secure. They were not statistically different (P = 0.572). The odds of such households being food secure was 0.81. The number of household members had a significant negative influence on food security. For a unit increase in the number of household members, the household becomes 0.80 times more likely not to be food secure (P = 0.01). Owning livestock had a significant positive influence on household food security. A household with livestock is 2.77 times more likely to be food secure than a household with no livestock (P = 0.007).
4. Discussion
Household surveys were conducted to assess how the principles of CE are applied in the agricultural sector and understand the farm-level adoption of regenerative agriculture as well as its contribution to household food security, with the ultimate aim of offering recommendations to accelerate adoption.
4.1. Drivers of adoption
The decision of farmers to adopt or not to adopt a new technology depends on their perceptions of the relative advantages of the adoption such as extra income, goods, and services, against costs including extra resources and trade-offs to procure inputs and managing agronomic complexities within local farming systems and market conditions (Miller et al., 2016). There is a need to identify factors beyond just farm finances that explain the adoption of regenerative agriculture (Knowler and Bradshaw, 2006; Noeldeke et al., 2022).
An effective extension system was an important driver of the adoption of regenerative agricultural practices. Farming households that were visited by agricultural extension agents had nearly three times the chance of adopting regenerative practices than households that did not receive extension agents. This is in agreement with findings that factors influencing farmers' decisions on choice of options are access to agricultural extension services and availability of resources (Hassan and Nhemachena, 2008; Deressa et al., 2009; Mugwe et al., 2009; Bryan et al., 2013; Kichamu et al., 2018). This research also found a positive correlation between farmers who are not frequently visited by extension agents and farmers who apply regenerative practices with no training. A possible explanation could be that information and skills are passed on from farmer to farmer through informal exchange visits and seeing tangible realities and benefits of regenerative agriculture. This was observed in Makueni sub-county and could be one adoption mechanism to establish demonstration farms for best regenerative agriculture practices to accelerate their wide adoption by farming communities led by champion farmers. However, this farmer-to-farmer learning could not be expected in communities like Kilome that have had limited exposure to regenerative agricultural practices. Their limited exposure is mainly the reason for the low adoption of the practices. Farmers' exposure to new technologies may be potentially an important intermediary factor before farmers perceive their advantages (Liyama et al., 2018). Even though Kilome farming community lives adjacent to a protected forest, their adoption of regenerative practices was low, contrary to our initial assumption. This is partly because the area is not frequently reached by extension agents as indicated by respondents. Additionally, the area has not received agricultural development partners in a long time. Farming households in such areas lack adequate participatory farmer-led extension activities to drive the successful uptake of locally feasible practices toward improved agricultural production (Brown et al., 2018).
The present findings indicated that farmers who participated in training on regenerative agricultural practices were 4.67 times more likely to adopt regenerative agricultural practices than the farmers who have not participated in any training. This concurs well with Murindangabo et al. (2021) who reported that the adoption of such practices was related to the knowledge acquired during training and hands-on work on demonstration plots. This highlights the critical role of capacity building for farming communities to adopt regenerative agricultural practices and partly explains why Makueni sub-county applied more regenerative agricultural practices than other sites. Through surveys, this study found that some individual farmers in Makueni had been trained in regenerative agricultural practices including crop rotation, intercropping, integrated pest management, crop cover and mulching, agroforestry, composting, and minimal tillage. Farmers in Makueni who have not participated in formal training were informally introduced to regenerative practices by their fellow farmers.
4.2. Drivers of household food security
The Food and Agriculture Organization of the United Nations defines food security as a “situation that exists when all people, at all times, have physical, social and economic access to sufficient, safe and nutritious food that meets their dietary needs and food preferences for an active and healthy life” (FAO, 2009). Knowler and Bradshaw (2006) reported that the benefits of regenerative agricultural practices include the increase in soil fertility and moisture retention, resulting in long-term yield increase, decreasing yield variations, and greater food security. In this study, the number of regenerative agricultural practices positively influenced household food security. Not only do regenerative practices produce quantities of food but they uniquely offer diverse varieties of food from agroforestry fruit trees to rotation grains. Coulibaly et al. (2017) found that farming households were selling both agroforestry tree products and crops in Malawi, which contributed to their food security. This provides enough alternatives for a household to create a daily menu and ensures there is the provision of food all year round because of maturing at different times of the year. Having multiple regenerative agricultural practices also contributes to soil health maintenance with ongoing nutrient recycling (Schreefel et al., 2020).
Both livestock income and farm size are the common wealth indicators for farming households (Ndoli et al., 2021). Owning livestock for farming communities meant more chances to be food secure. With livestock, farmers can access manure for soil health restoration and subsequent higher crop yields. Livestock could be both a source of protein and manure for farm plots to grow more food crops. Livestock is at the interface of integrated farming where the potentials of trees, crops, and livestock are enhanced to create a more circular system. Livestock is also a source of cash income for rural households, with which they can afford a variety of food (Ndoli et al., 2021). Large or multiple farm plots had a positive impact on household food security. This is in line with Ndoli et al. (2021) who found food security increases with increasing farm size in rural Rwanda. Farmers with large or multiple farm plots have more flexibility to harvest quantities and grow various crops on the same piece of land. Availability of land provides the opportunity for farmers to experiment with such regenerative practices, thus influencing the usage of large-scale practices such as cover crops, crop rotation, agroforestry, use of organic fertilizers, and mulching (Kirimi et al., 2013; Wekesa et al., 2018). Unexpectedly, off-farm income did not have a positive influence on household food security. This could be because households probably invested their income in other activities rather than agriculture, for example, small-scale commercial business (Nahayo et al., 2016).
Our analysis is based on self-reported sufficiency in the quantity and diversity of food but the model we built to assess driving factors helps to minimize the bias (Tadesse et al., 2020). There are few if any universal variables that regularly explain the adoption of regenerative agriculture practices (Knowler and Bradshaw, 2006). We also note that our samples were taken from a micro-level scale with smallholder farming systems. Future research should explore how household income generation and food security of smallholders are impacted by the adoption of regenerative agricultural practices.
5. Conclusion
Revisiting the objective of this study, determining factors for the adoption of regenerative agricultural practices were investigated as well as determining factors of food security in relation to the application of regenerative agricultural practices. The results indicate that the adoption rate of regenerative agricultural practices was still low with many farmers implementing low capital and labor-requiring practices such as crop rotation and intercropping. It was clear that farmers who participated in training on regenerative agricultural practices were more likely to adopt regenerative agricultural practices. Thus, highlighting the critical role of capacity building for farming communities for skills and knowledge transfer through hands-on work training and demonstration plots. The study, therefore, recommends pilot studies that will further investigate different regenerative agricultural practices in relation to provided skillsets as well as their role to improve household food security status. It was also observed that the number of regenerative agriculture practices applied by a farmer had a significant positive influence on household food security status, thus highlighting the potential of regenerative agricultural practices to alleviate food insecurity among smallholder farmers if used in combinations and to a larger extent. This study recommends further pilot studies on specific regenerative agricultural practices and their adoption imperatives. The findings of this study can help design scaling pathways, and enhance practical training and extension services to accelerate the adoption of regenerative agriculture to build a more resilient food system.
Data availability statement
The original contributions presented in the study are included in the article/supplementary material, further inquiries can be directed to the authors.
Ethics statement
The research involving human participants has been approved by the Ethical Committee of the Circular Economy Research Programme at African Leadership University. Written informed consent from the participants was not required to participate in this study in accordance with the national legislation and the institutional requirements.
Author contributions
Conceiving, designing, writing, and carrying out the fieldwork were done by EN. EN-R, DN, and TG reviewed the manuscript in the design and final stages. SN contributed to data analysis. All authors have read and agreed to the published version of the manuscript.
Funding
This study was possible due to funding from MAVA Foundation.
Acknowledgments
We thank Charite Abijuru, Christophe Nsengiyumva, Valentine Nyirahabimana, Deborah Mukundwa, Daniella Uwase, Cedrick Igiraneza, Marube Annah, Joy Imo, Caren Rotich, Nyaruai Pierra, Joshua Kioko, George Musyoki, Brigid Wambua, and Jacinta Ndinda for their support in the data collection. We also thank MAVA Foundation for supporting the Circular Economy Programme at African Leadership University, and to fund the circular economy programme.
Conflict of interest
The authors declare that the research was conducted in the absence of any commercial or financial relationships that could be construed as a potential conflict of interest.
Publisher's note
All claims expressed in this article are solely those of the authors and do not necessarily represent those of their affiliated organizations, or those of the publisher, the editors and the reviewers. Any product that may be evaluated in this article, or claim that may be made by its manufacturer, is not guaranteed or endorsed by the publisher.
References
Abad-Segura, E., González-Zamar, M. D., Infante-Moro, J. C., and García, G. R. (2020). Sustainable management of digital transformation in higher education: global research trends. Sustainability 12:2107. doi: 10.3390/su12052107
AGRA (2017). Africa Agriculture Status Report: The Business of Smallholder Agriculture in Sub-Saharan Africa (Issue 5). Nairobi, Kenya: Alliance for a Green Revolution in Africa (AGRA). Issue No. 5
Agresti, A. (2007). An Introduction to Categorical Data Analysis (2nd ed.). Hoboken, NJ: John Wiley and Sons. 34–40. doi: 10.1002/0470114754
Boon, E. K., and Anuga, S. W. (2020). Circular economy and its relevance for improving food and nutrition security in Sub-Saharan Africa: the Case of Ghana. Mater Circ Econ 2, 5. doi: 10.1007/s42824-020-00005-z
Brown, B., Llewellyn, R., and Nuberg, I. (2018). Global learnings to inform the local adaptation of conservation agriculture in Eastern and Southern Africa. Global Food Security 17, 213–220. doi: 10.1016/j.gfs.2017.10.002
Bryan, E., Ringler, C., Okoba, B., Roncoli, C., Silvestri, S., and Herrero, M. (2013). Adapting agriculture to climate change in Kenya: household strategies and determinants. J. Environ. Manage. 114, 26–35. doi: 10.1016/j.jenvman.2012.10.036
Carl, H., Adam, L., Yaw, A., Clint, T., Waylon, B., and Gaetano, B. (2010). Open Data Kit: Tools to Build Information Services for Developing Regions. Available online at: https://dl.acm.org/citation.cfm?id=2369236
CBK (2022). Monetary Policy Committee Agriculture Sector Survey. Central Bank of Kenya. Nairobi Kenya.
Coulibaly, J. Y., Chiputwa, B., Nakelse, T., and Kundhlande, G. (2017). Adoption of agroforestry and the impact on household food security among farmers in Malawi. Agric. Syst. 155, 52–69. doi: 10.1016/j.agsy.2017.03.017
Deressa, T. T., Hassan, R. M., Ringler, C., Alemu, T., and Yesuf, M. (2009). Determinants of farmers' choice of adaptation methods to climate change in the Nile Basin of Ethiopia. Glob. Environ. Change 19, 248–255. doi: 10.1016/j.gloenvcha.2009.01.002
EMF (2019). Cities and Circular Economy for Food. Environmental, Health, and Economic Impacts Due to Global Food Production Equivalent to About USD 6 Trillion.
FAO (2008). An Introduction to the Basic Concepts of Food Security. Food Security Information for Action-Practical Guides. EC - FAO Food Security Programme. Available online at: https://www.fao.org/3/al936e/al936e00.pdf
FAO (2009). Declaration of the World Summit on Food Security. World Summit on Food Security Rome, 1–7.
FAO (2012). Why has Africa Become a Net Food Importer? Explaining Africa Agricultural and Food Trade Deficits. Rome
Farkas, J. Z., and Kovács, A. D. (2020). Nature conservation versus agriculture in the light of socio-economic changes over the last half-century–Case study from a Hungarian national park. Land Use Policy 101:105131. doi: 10.1016/j.landusepol.2020.105131
Hair, J. F., Black, W. C., Babin, B. J., and Anderson, R. E. (2010). Multivariate Data Analysis (7th ed.). New York, NY: Prentice Hall.
Hassan, R. M., and Nhemachena, C. (2008). Determinants of African farmers' strategies for adapting to climate change: multinomial choice analysis. Afr. J. Agric. Resour. Econ. 2, 83–104. doi: 10.22004/ag.econ.56969
Kichamu, E. A., Ziro, J. S., Palaniappan, G., and Ross, H. (2018). Climate change perceptions and adaptations of smallholder farmers in Eastern Kenya. Environ. Dev. Sustain. 20, 2663–2680. doi: 10.1007/s10668-017-0010-1
Kirimi, L., Gitau, R., and Olunga, M. (2013). “Household food security and commercialization among smallholder farmers in Kenya,” in Fourth International Conference of the African Association of Agricultural Economists (African Association of Agricultural Economists).
Kleinbaum, D. G., and Klein, M. (2002). Logistic Regression: A Self-Learning Text. Springer Science and Business Media.
Knowler, D., and Bradshaw, B. (2006). Farmers' adoption of conservation agriculture: a review and synthesis of recent research. Food Policy 32, 25–48. doi: 10.1016/j.foodpol.2006.01.003
Kogo, B. K., Kumar, L., and Koech, R. (2021). Climate change and variability in Kenya: a review of impacts on agriculture and food security. Environ. Dev. Sustain. 23, 23–43. doi: 10.1007/s10668-020-00589-1
Liyama, M., Mukuralinda, A., Ndayambaje, J. D., Musana, B. S., Ndoli, A., Mowo, J. G., et al. (2018). Addressing the paradox – the divergence between smallholders' preference and actual adoption of agricultural innovations. Int. J. Agric. Sustain. 16, 472–485. doi: 10.1080/14735903.2018.1539384
McKinney, W. (2012). Python for Data Analysis. O'Reilly Media, Gravenstein Highway North, Sebastopol, United States.
Meijer, S. S., Catacutan, D., Ajayi, O. C., Sileshi, G. W., and Nieuwenhuis, M. (2015). The role of knowledge, attitudes and perceptions in the uptake of agricultural and agroforestry innovations among smallholder farmers in sub-Saharan Africa. Int. J. Agri. Sustain. 13, 40–54. doi: 10.1080/14735903.2014.912493
Miller, D. C., Muñoz-Mora, J. C., and Christiaensen, L. (2016). Prevalence, economic contribution, and determinants of trees on farms across Sub-Saharan Africa. (Policy Research Working Paper 7802). Washington, DC: World Bank. doi: 10.1596/1813-9450-7802
MINAGRI (2022). Annual Report for 2021/2022 Fiscal Year. Kigali Rwanda: Ministry of Agriculture and Animal Resources.
MINAGRI. (2018). Strategic Plan for Agricultural Transformation 2018-2024. Kigali Rwanda: Ministry of Agriculture and Animal Resources.
Mo, E. (2020). WCEF22 invites the world to Africa on 6-8 December 2022 to build circular economy solutions. Available online at: www.environment.gov.rw, http://bit.ly/3WW1AEI (accessed November 11, 2022).
Mugwe, J., Mugendi, D., Mucheru-Muna, M., Merckx, R., Chianu, J., and Vanlauwe, B. (2009). Determinants of the decision to adopt integrated soil fertility management practices by smallholder farmers in the central highlands of Kenya. Exp. Agric. 45, 61–75 doi: 10.1017/S0014479708007072
Murindangabo, Y. T., Kopeck,ý, M., and Konvalina, P. (2021). Adoption of conservation agriculture in rwanda: a case study of gicumbi district region. Agronomy. 11:1732. doi: 10.3390/agronomy11091732
Nahayo, A., Pan, G., and Joseph, S. (2016). Factors influencing the adoption of soil conservation techniques in Northern Rwanda. J. Plant Nutr. Soil Sci. 179, 367–375. doi: 10.1002/jpln.201500403
Nakawuka, P., Langan, S., Schmitter, P., and Barron, J. (2018). A review of trends, constraints and opportunities of smallholder irrigation in East Africa. Global Food Security 17, 196–212. doi: 10.1016/j.gfs.2017.10.003
Ndoli, A., Mukuralinda, A., Schut, A. G. T., Liyama, M., Ndayambaje, J. D., Mowo, J. G., et al. (2021). On-farm trees are a safety net for the poorest households rather than a major contributor to food security in Rwanda. Food Sec. 13, 685–699. doi: 10.1007/s12571-020-01138-4
Newton, P., Civita, N., Frankel-Goldwater, L., Bartel, K., and Johns, C. (2020). What is regenerative agriculture? a review of scholar and practitioner definitions based on processes and outcomes. Front. Sustain. Food Syst. 194:577723. doi: 10.3389/fsufs.2020.577723
Nijman, E. (2020). Towards Circular Food Production Systems in East Africa. MSc Thesis. Radboud University.
Noeldeke, B., Winter, E., and Ntawuhiganayo, E. B. (2022). Representing human decision-making in agent-based simulation models: agroforestry adoption in rural Rwanda. Ecol. Econ. 200:107529. doi: 10.1016/j.ecolecon.2022.107529
Noy (2008). Sampling knowledge: the hermeneutics of snowball sampling in qualitative research. Int. J. Soc. Res. Methodol. 11, 327–344. doi: 10.1080/13645570701401305
O'Brien, R. M. (2007). A caution regarding rules of thumb for variance inflation factors. Qual. Quant. 41, 673–690. doi: 10.1007/s11135-006-9018-6
Ozor, N., and Nyambane, A. (2021). Institutional Framework for Ecological Organic Agriculture in Kenya. African Technology Policy Studies Network (ATPS).
Preston, F., and Lehne, J. (2017). A Wider Circle? The Circular Economy in Developing Countries. Briefing. Energy, Environment and Resources Department. Chatham House.
Saidani, M., Yannou, B., Leroy, Y., Cluzel, F., and Kendall, A. (2019). A taxonomy of circular economy indicators. J. Clean. Prod. 207, 542–559. doi: 10.1016/j.jclepro.2018.10.014
Schreefel, L., Schulte, R. P. O., de Boer, I. J. M., Pas Schrijver, A., and van Zanten, H. H. E. (2020). Regenerative agriculture – the soil is the base. Glob. Food Sec. 26:100404. doi: 10.1016/j.gfs.2020.100404
Shimeles, A., Verdier-Chouchane, A., and Boly, A. (2018). Introduction: Understanding the Challenges of the Agricultural Sector in Sub-Saharan Africa. Palgrave Macmillan, Cham: Building a Resilient and Sustainable Agriculture in Sub-Saharan Africa. doi: 10.1007/978-3-319-76222-7_1
Sosola, B. G., Akinnifesi, F. K., Sileshi, G., and Ajayi, O. C. (2011). Evergreen Agriculture: Extension Manual for Farmers and Extension Workers. Lilongwe, Malawi: World Agroforestry Centre.
Tadesse, G., Abate, G. T., and Zewdie, T. (2020). Biases in self-reported food insecurity measurement: a list experiment approach. Food Policy 92:101862, doi: 10.1016/j.foodpol.2020.101862
UNEP (2010). Aid for Trade Case Story: The East African Organic Products Standard. Available online at: https://www.oecd.org/aidfortrade/47719232.pdf
van Dijk, M., Morley, T., Rau, M. L., et al. (2021). A meta-analysis of projected global food demand and population at risk of hunger for the period 2010–2050. Nat. Food. 2, 494–501. doi: 10.1038/s43016-021-00322-9
Wekesa, B. M., Ayuya, O. I., and Lagat, J. K. (2018). Effect of climate-smart agricultural practices on household food security in smallholder production systems: micro-level evidence from Kenya. Agric. Food Secur. 7, 80. doi: 10.1186/s40066-018-0230-0
Whyte, C. R., Irakoze, G., Katanisa, P., and Desmond, P. (2020). Circular economy in the Africa. EU cooperation - Country Report for Rwanda. Country report under EC Contract ENV.F.2./ETU/2018/004 Project: “Circular Economy in Africa-EU cooperation,” Trinomics B.V., ACEN, adelphi Consult GmbH and Cambridge Econometrics Ltd.
Keywords: circular economy, regenerative agriculture, food systems, Kenya, Rwanda
Citation: Ntawuhiganayo EB, Nijman-Ross E, Geme T, Negesa D and Nahimana S (2023) Assessing the adoption of regenerative agricultural practices in Eastern Africa. Front. Sustain. 4:1105846. doi: 10.3389/frsus.2023.1105846
Received: 23 November 2022; Accepted: 22 May 2023;
Published: 29 June 2023.
Edited by:
Dimitrios Paparas, Harper Adams University, United KingdomReviewed by:
Amos Ncube, University of Naples Parthenope, ItalyAlbert Novas Somanje, Ministry of Agriculture, Zambia
Fanya Qin, The University of Tokyo, Japan
Copyright © 2023 Ntawuhiganayo, Nijman-Ross, Geme, Negesa and Nahimana. This is an open-access article distributed under the terms of the Creative Commons Attribution License (CC BY). The use, distribution or reproduction in other forums is permitted, provided the original author(s) and the copyright owner(s) are credited and that the original publication in this journal is cited, in accordance with accepted academic practice. No use, distribution or reproduction is permitted which does not comply with these terms.
*Correspondence: Elisée Bahati Ntawuhiganayo, ntawebahati@gmail.com