- College of Science and Engineering, Hamad Bin Khalifa University, Qatar Foundation, Doha, Qatar
Introduction: While promoting sustainable industrial cluster development using the circular economy perspective, the increased use of underutilized materials results in compromised profitability in the cooperative network. The focus is to evaluate the external financial support required in industrial clusters against specific objectives and highlight the potential beneficiaries and losers in financial terms because of industrial symbiosis while closing material loops.
Method: This study provides an agent-based approach to record the system response based on three case studies to demonstrate the potential cost variations: The first case is about utilizing only naturally available resources by all the industrial entities and is taken as a base case. The second case is about targeting the lowest cost for each product, and the third one provides a sustainable and flexible solution by targeting the best transformation methods and materials.
Conclusion: The study concludes with valuable insights to identify the economically compromised entities in an industrial cluster network by considering economic deviations beyond a critical value. It can help take concrete measures in the form of incentives or investors subsidies by governmental organizations, regulators, and policymakers to intervene and stimulate markets through targeted financial support/policies to the compromised entities. This results in improved materials loop closing, essentially promoting sustainable production systems in industrial clusters. Additionally, such financial support/incentives also influence the scarcity or accumulation of by-products or low-value materials, ultimately improving the industrial network's environmental and economic performance.
1. Introduction
While closing underutilized material loops, symbiosis as a strategy in industrial clusters plays a vital role in sustainable industrial development with increased regional economic benefits (Baldassarre et al., 2019; Laybourn, 2021). However, the restrictions on cooperation and material exchange among entities strongly impact the collective benefits and resource management. While closing material loops in an industrial network, a common hurdle is the non-utilization of waste materials due to the infeasible economic component or high capital cost (Frosch, 1997). The factors contributing to the increased cost include spatially and temporally distributed under-utilized materials, transformation technology used for a process, and the generation and handling of hazardous materials, leading to higher product costs. Generally, the collective contribution of these factors disrupts the economic component among the cooperating entities exchanging materials.
Furthermore, depending upon the industrial cluster network plan, the materials involved therein, and the generation of multiple types of wastes/emissions at an alarming rate harming nature and human health, the uncontrolled use of limited natural resources (raw materials, water, and energy) influences the sustainability of existing production and consumption systems (OECD, 2007; UNEP, 2015). Additionally, the enormous amount of generated waste threatens the integrity of regional ecosystems necessary for human life (Zommers et al., 2016; UN, 2022). To slow down these impacts, strategies related to selecting and converting low-value raw materials through economic manufacturing processes to valuable products are critical and in line with circular economy (CE) principles. Adopting sustainable resource management within industrial zones can alleviate environmental burdens from production and manufacturing (Simonis and Ayres, 1995). However, considering external financial compensation for critical entities closing material loops can catalyze and promote the CE perspective in industrial cluster networks. In this study, critical entities refer to those product manufacturing entities having compromised profitability to the extent that affects feasibility. While reducing cluster impacts, the first step for financial intervention would be to find those critical entities that help reduce material leakages and have compromised economic components.
Ideally, a cooperative industrial cluster network exchanging information and materials per requirements without any restriction has the competitive advantage of increased collective economic benefits. The loop closing through this exchange makes the system more sustainable overall; however, at the level of an individual entity striving for sustainability, it may disrupt the economic component, which limits this cooperation either by affecting the profit or incurring economic losses. The financial impacts caused by material exchange beyond an objective-based critical point lead to infeasibility that needs to be analyzed and compensated by governmental bodies to promote materials loop closing. The transformation cost of converting raw materials to finished products and co-products includes energy and labor, which in return produces by-products (most likely of lower economic value), waste (zero economic value), and emissions, which have economic, social, and environmental impacts. Therefore, it is crucial to analyze the secondary pollution impacts of further management required for the generated by-products and their transformation as this impedes the economic and environmental benefits. Such associated transformations and underutilized materials management are challenging and affect the economic component.
The challenging part is the decision to operate a specific material transformation as a bridge, utilizing materials/residues/by-products from other entities as inputs and generating by-products for others to provide a more holistic eco-efficient solution. For a feasible material transformation to be acceptable, it is crucial to assess (1) the economic aspect while reducing the waste in terms of variety, value, and quantity against impacts and (2) the generation of valuable by-products/waste after analyzing their hazardous/non-hazardous nature (EPA, 2000; Bourguignon, 2015). Additionally, the network may cause scarcity/accumulation of underutilized materials depending on factors such as the input material quality, type, cost, competition level and market value of the generated wastes, regional price/demand, transformation techniques or processes involved therein, and end product's cost and demand. Therefore, increasingly considering waste as a resource adds to the vulnerability to supply chain risks due to these factors. This risk impacts economic sustainability and therefore needs analysis, optimization, and technology use (Choi, 2022).
This work contributes to sustainable industrial cluster development by providing insights to help policymakers and governmental organizations identify the potential areas where targeted external financial support is needed. Although external financial support is one of the most critical aspects for sustainable materials loop closing, the literature lacks. In this study, the objective of providing insights for identifying potential areas where targeted financial support is needed is accomplished by examining the role of individual industrial entities in the regional clusters for variations in the cost of manufacturing a product and the resulting quantity of waste or emissions generated. The methodology presented in this study can be used to examine the cluster entities holistically to identify critical stages regarding the affected economic aspect in the operating phase. Such analysis helps governmental organizations (1) develop strategies and policies to reduce hurdles in materials loop closing and (2) provide targeted financial support to promote material flows for sustainability.
The remaining sections of this article are structured as follows: after presenting the related work from literature in the “Literature review” section, the system model and methodology are discussed in the “Problem description” section, where the proposed approach is demonstrated to promote sustainable industrial cluster development by finding the economically compromised entities within industrial cooperative networks for targeted financial support, and finally, results are presented in the “Results and discussion” section, before concluding in the “Conclusion” section.
2. Literature review
Industrial cluster entities that promote the exchange and use of underutilized materials significantly affect production systems' economic and environmental performance (Worrell et al., 2016; Castiglione et al., 2022). For instance, the economic and environmental-related benefits derived from exchanges of water and steam for reuse are illustrated by Brings Jacobsen (2006), who concluded that IS must be understood in terms of individual and collective approaches toward the economic and environmental performance of industrial sustainability. The collaborative development of various system components can effectively promote regional economic performance (Feng and Bai, 2021). The role of knowledge coordination strategy and relationship capacity is explored by Zheng and Jia (2017) using an ABM model, highlighting implications for IS-promoting agencies. Similarly, the contribution of information exchange in business making and IS networks' economic/environmental performance is illustrated by Fraccascia and Yazan (2018). The positive contribution of IS in reducing climatic changes is claimed in a manufacturing cluster by Daddi et al. (2017) using a life cycle assessment approach.
Moreover, Hu et al. (2020) claimed significant cost savings and emission reduction while treating wastewater in industrial parks to reuse organic matter in food wastewater. While studying the facts about regional economic performance, Porter (2003) indicated that the strength of local clusters and the level of innovation strongly influence the performance of regional economies. However, the strength of local clusters cannot be attained without determining the critical entities based on the CE perspective to compensate for the economic factor supporting the closing of material loops in industrial clusters. Moreover, Delgado et al. (2014) suggested that industries in a strong cluster tend to positively affect employment, patenting growth, and favoring new regional sectors.
Realizing objective-based functional exchanges to favor loop closing for improving materials value depends on (1) the relative geographical proximity of other entities (Ghisellini et al., 2016), (2) by-products type and quantity generated/available in the network, (3) the optimal selection of drains for underutilized materials based on end products' demands and the transformation cost, to name a few. In addition, for greater efficiency, the value of the by-products/materials can be improved by adding value through engaging multiple actors/stakeholders (suppliers, manufacturers, retailers, and consumers) in the CE value chain (World Economic Forum, 2019). For instance, Guirlanda et al. (2021), in the cocoa production chain, aimed to raise production by adding value to residues such as cocoa honey (~80% of fruit) through the pharmaceutical and cosmetic industries. Better decisions about underutilized materials through cooperation in IC enhance productivity and income. The factors that promote the use of underutilized materials include (1) the demand for high-quality products and modern infrastructure availability (Mehdiyeva et al., 2020); (2) cooperation, collective actions, higher density of clusters, and higher diversity, which improve the average income level in clusters (Wardhana et al., 2020b); (3) effects of competitive pressure from neighboring entities' economic activity (Wardhana et al., 2020a); and (4) increase in production through sustainable and innovative developments.
Regarding tools/techniques for practicing the CE concept through symbiosis for sustainable industrial development, Yu et al. (2021) proposed an ABM approach integrating geographic information systems (GIS) to explore IS for recycling concrete aggregates to tackle the CE challenge while lacking economic incentives. Similarly, for optimal design and location of bioeconomic clusters, multicriteria decision analysis (MCDA) integrated with GIS is used to consider the quantitative analysis of the flow of resources based on economic input–output analysis (Perez-Valdes et al., 2019; Nørstebø et al., 2020). For waste material recycling and exchange decisions, Huang et al. (2020) proposed a mixed-integer programming method, where the raw material price and by-product recycling are the two main cost elements of the waste material. Considering the environmental aspect of a carbon-constrained industrial network, Bechara and Alnouri (2022) formulated a mixed integer non-linear program (MINLP) to select the optimal allocation of the different fuels using source-to-sink mapping, meeting the mentioned targets. A game model in a textile cluster aiming to optimize wastewater systems for sustainability under stringent wastewater discharge limits illustrated the economic and environmental trade-offs amongst the stakeholders (Lyu et al., 2021). Generally, in such systems, loss of performance and reliability concerns are challenging due to economic hotspots with unbalanced inter-industrial raw materials demands.
For analysis of clusters, the agents/actors of the distributed method in an ABM cooperate based on some constraints/rules that reflect the complex system's evolving nature (Farmer and Foley, 2009). Some example studies that utilize ABM to analyze IS include understanding the complex interactions in microbial communities (Koshy-Chenthittayil et al., 2021), coal industrial symbiosis systems (Wang et al., 2017), the interactions of exchange between manure suppliers and biogas producers (Yazan et al., 2018), agro-industrial ecosystems (Huang et al., 2015), and agro-food supply chains (Taghikhah et al., 2021), to name a few. The agent-based method can realize potential cooperating entities of an industrial network to obtain eco-efficient outcomes. For instance, the advantages of an agent-based method in producing cost-efficient energy futures were explained by Batten (2009), where sets of entities perform more eco-efficiently by introducing strategically located clusters of renewables, low emissions, and distributed generation. Moreover, only a few studies demonstrated the application of agent-based models at the life cycle inventory (LCI) stage of life cycle assessment (Davis et al., 2009; Wu et al., 2017), where the latter addressed temporal and spatial dynamics. Also, the method was used to model the operation of eco-industrial parks (Romero and Ruiz, 2013).
From the reviewed articles, only a few research studies incorporated multi-objective approaches in industrial clusters and these are even rarer when it comes to (i) investing in the use of underutilized materials to stimulate economic growth and (ii) quantitatively evaluating the profitability of industrial entities while considering symbiosis. To address these gaps in the literature, this study primarily proposed an agent-based approach for production in an industrial cluster where the dynamics of underutilized materials and the product cost under three different cases are considered: manufacturing using natural resources only, targeting for lowest cost options, and achieving sustainability through considering multiple transformation methods. The mentioned cases are considered while determining sustainably improved sinks to extract near-optimal value from underutilized intra-cluster industrial materials. The focus is to provide insights for governmental intervention and policies to promote closing the materials loop by addressing financial problems. Due to the unfavorable proportion of broader types of wastes/by-products, the benefits between any two entities vary in addition to other management challenges. Therefore, a holistic response is needed to assess the economic aspect while enhancing better management of the by-products detrimental to the environment through information and material exchange among entities. Briefly, the contributions that differentiate this study from other studies include the following:
• Investigation of the critical loser entities resulting from IS while exploiting the underutilized materials going to landfills using the ABM approach.
• Provide valuable insights for the targeted financial intervention of governmental agencies to assist the loser entities, to investigate the level of external economic support needed, and to promote symbiosis to reduce underutilized materials going to landfills.
• Based on the above insights, governments can control the utilization of low-value materials and regulate the market by imposing taxes or providing subsidies. This can help provide an improved investment environment while targeting sustainability.
3. Problem description
In this study, information sharing is used to bring transparency to industrial by-products management by observing the underutilized materials in the network. An agent-based approach uses this information for material exchange decisions among actors, with applicability irrespective of size and geographic location achieved by setting a few parameters according to the geographic region. The holistic gains of the industrial network may vary from region to region based on the set parameters. Generally, distributed approaches are preferred over centralized ones as the latter inversely affect the scalability and reliability of industrial clusters (Moss, 2007; Chanfreut et al., 2021). The proposed closed-loop agent-based system, managed in a grid-like structure, having multiple entities, is a distributed waste/by-product management approach that deals with the complexity of waste management by identifying suitable sources and sinks for materials. As such, it improves materials management, diverts significant material flows from landfills, and favors economic and environmental aspects. Each industrial entity is assigned its agent to exchange information with its immediate neighbors and to exploit its locality when making decisions. Since each agent can only exchange information with its neighbors, they needs multiple information exchanges at specific time instants to propagate attributes throughout the cluster for a near-optimal state. Performing only the local exchange of information makes the model scalable and reliable. Moreover, information exchange would only be triggered at specific timestamps conditioned to observed change.
In general, production systems are demand-driven, where every product has an independent demand, thus generating unfavorable proportions of a wide variety of wastes/by-products. The tendency to use the waste from other IC entities links multiple products' production systems in the CE loop/value chain, leading to a finished product's dependency upon the preceding generated/collected underutilized materials in the IC network, introducing uncertainties in the raw material availability. Uncertainty in the availability of a specific proportion of raw inputs required to manufacture a specific, relatively high-value product may become a bottleneck. This inhibits economies of scale and can be the reason for underutilized material scarcity/accumulation, which changes the economics of the whole cluster. The factors influencing the sustainability of IC include the scarcity or accumulation of raw material/waste, underutilized material quality, type, cost, market value of the generated wastes, transformation techniques and processes involved, end product's cost, regional price, and demand. Socioeconomic factors and the response of the regional population to a specific product price in the CE value chain impact multiple entities in the cluster. All these factors derail the sustainable transition of materials and increase the complexity of the model. Even the most optimized systems, if not analyzed correctly, may accumulate vast amounts of particular industrial waste that is generally landfilled. Uncertainties in raw materials availability due to this linking generally reflect low service levels and therefore affects the profit, leading to infeasibility in the worst situation. Generally, the service level in such cases can improve through other possible raw material substitutes and manufacturing processes that may require additional technical support, adding an overhead.
It is assumed that each industry produces a single product in the industrial cluster network and has an agent that shares the attributes, information, and availability of underutilized materials/resources. An agent is a communicational and computational entity that acts on behalf of other autonomous and flexible entities (Wooldridge and Jennings, 1995). All the agents are considered identical, cooperative, and at the same level, making the manufacturing cluster scalable and reliable. Each agent reacts independently based on the actions of others without any higher instance to control these agents. All agents share node-specific attribute information stored in local memory, such as wastes/by-products availability and raw materials requirement of neighbors, which is further propagated elsewhere through a predefined scheme as indicated in Figure 1. Through these shared attributes, the common goal for each agent here is to find suitable sinks. Detection of any change in attribute values at an entity causes the sharing of attributes. Based on the shared information processing, appropriate sinks for all underutilized surplus materials are determined. The word “material” here refers to natural resources and by-products/wastes, while underutilized materials are the wastes/by-products generated intra-cluster. The model can be modified based on implementation, where the agents can disseminate information either with neighbors, first-tier suppliers, or all entities in the ICs. The time required to disseminate critical information to the farthest agent is directly related to the network size and inversely related to the number of directly communicating agents affecting the objective.
The consumer demand for a particular product is the driver for operating entities, and this model uses it as an initiation trigger for the system. However, the regional demand for a specific product or its substitute varies and depends on many factors, such as a society's socioeconomic conditions, culture, and living standards. However, for analysis purposes, the probability distribution for a product's regional demand can be considered Gaussian with a fixed mean and variance. While meeting demands, industrial by-products/wastes are generated, which may become raw inputs for other industrial products within the cluster. This connection/dependency of preceding products' waste to multiple succeeding products makes it a linked system. Also, the demands for all products are independent of the wastes/by-products generated, leading to uncertainties in required raw inputs availability, restricting economies of scale and causing disruptions in the supply chain. Each entity deals with the uncertainty by allowing the use of multiple options of potential inputs and material transformation processes to produce a product. The reasons for the inclusion of three different feasible material transformation methods to choose from when making a product or its substitute are to (1) ascertain whether the loop is closing for most of the underutilized materials, (2) improve the economic factor, and (3) tackle the unfavorable proportions of wastes/by-products generated after fulfilling the end product market demands. However, depending upon the objective, the cost/economic component (fixed or variable) of making a sustainable finished product at an entity may be disrupted, and sometimes may become infeasible, requiring external intervention. Also, the viability and economics of materials flow networks may vary from region to region. The equations in the following section indicate the sustainability-based assessment of a transformation process to determine the usefulness of transforming materials along the value paths that support the loop closing in line with the CE.
3.1. Mathematical analyzes for transformation methods
While optimizing a material flow network (MFN), cost analysis of the materials exchange among processes and the process itself is crucial while integrating it into loop-closing strategies in industrial clusters. Figure 2 represents a generalized transformation process, transforming multiple input materials into valuable products, by-products (with a lower market value), and some waste of multiple types considered to have no market value.
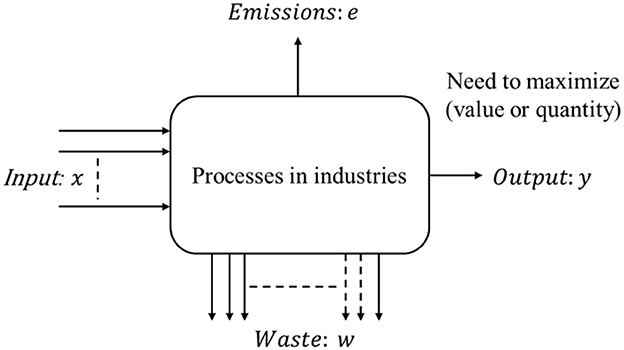
Figure 2. Illustration of a transformation process converting materials into high-value consumer products.
According to the law of conservation of mass, Figure 2 can be expressed by Equation 1 as follows:
where the left side is the summation of average quantities of all inputs xi required to produce a unit of output y. The right side represents the summation of j different average quantities of emissions ej and average quantities of k different wastes wk. Furthermore, Equation 1 can be modified in all forms in accordance with the transformation processes considered to make a particular product in an entity but must not violate the law. For cost-benefit analysis and evaluation, only feasible and relatively sustainable transformations must be shortlisted based on a set of costs where the value of the inputs is smaller than the product's value, as given in the following equation:
where ai, bj, and ck represent the sustainability costs based on the value/usefulness of the inputs, emissions, and waste, respectively. Every transformation method that converts raw materials to finished goods has different labor and energy overheads, incorporated into Equation 2 with regional/global environmental impacts of emissions and waste. Equation 2 is also applicable and can be modified according to any transformation process in an entity.
A valuable product can be produced using a combination of raw materials or equivalent alternates through different transformation methods, thus incurring different costs. The equivalent alternate intra-cluster by-products can replace naturally existing raw materials while closing material loops for possible sustainability enhancement. Material transformations result in one or more types of wastes/by-products (solids, liquids, or emissions) that are added to the environment if not utilized in other industries. Mentioned are the two illustration cases regarding materials use and competition in industrial clusters. First, more than one product may compete for a specific raw material, having independent demands with different market values and costs. A fixed proportion of input raw materials is required to produce a particular finished product, where the raw materials include natural sources and by-products/industrial wastes. Second, while producing a product or its equivalent alternate, a specific raw material (natural/waste) may compete with other intra-cluster materials/substitutes.
3.2. Case description and model
A set of N industrial entities (agents) is given, where each entity is exchanging information and producing a single unique product {A1, A2, …, AN} using natural resources and industrial wastes R = {1, …, n} available in the region of the industrial cluster. Initially, the model is set to have abundant natural resources, while all underutilized materials are zero. The cost of materials is fixed initially and kept constant throughout the time horizon. For the underutilized materials, the effects of dynamic cost due to competition among entities are not considered for simplicity. Fulfilling demands for a specific period T = {1, …, p}, an entity uses only one of the possible transformation methods among the set J = {1,..., m}. Table 1 provides all mathematical notations used in this study.
Both materials and transformation processes need to be reviewed for impacts to enhance sustainability. Sometimes, the transformation generates hazardous waste; therefore, considering collective impacts in terms of cost is crucial. For producing a product at an entity, three sustainable industrial transformation methods are included with assumed costs for producing a product or its substitute. In this study, a transformation method may constitute more than one process. The costs must be adjusted according to whether the facilities/resources are shared or not. Moreover, this study assumes that each entity could perform all required processes. Occasionally when reflecting on the CE perspective while transforming, hazardous materials are produced, which must be investigated for positive and negative aspects, with the inclusion of comparatively less hazardous industrial entities.
However, to avoid switching among transformation methods as they have overheads, only one among the three is adopted within a period. For this study, while manufacturing a product at an entity, the total cost is calculated by Equation 3, which comprises raw materials + transportation cost + transformation/value addition cost, specified as follows:
where is a [1 × m] matrix representing the total cost incurred while producing a unit product A1 for the m methods from the set J = {1,..., m}. The details of the model formulation are discussed below.
3.3. Mathematical formulation
For simplicity, only the set of parameters and variables for agent A1 is used in the description below. The same set of parameters and variables can be replicated for each agent of the set {A1, A2, …, AN}. Below is the stated objective function to evaluate and record the holistic response of the whole cluster. The net objective function value and the required attribute values must be communicated as per the scheme with direct neighbors to propagate across the cluster entities, which are then used to determine the net objective value of the cluster, as given in the following equation:
The entity A1 produces a product named A1, and so on. The objective value at an individual entity of the cluster is evaluated, as shown in Equation 5, representing the net gains/benefits at entity A1, which are then exchanged with the direct neighbors only. Similarly, X2 are the net gains at entity A2, and so on.
In this equation, the first part represents profit, the second represents the total waste consumed, the third represents waste generated, the fourth represents the loss of opportunity cost, and the last is the inventory holding cost, where is the total inventory holding cost for product A1 in a period t. Equations 6–18 are the mathematical constraints applied to each industrial cluster entity. Equations 6 and 7 together indicate how many units are produced (capped by 500 units/period) using a specific transformation method adopted from the set in a period at an entity:
where the Boolean indicates whether a particular method is used among the multiple transformation methods.
The total profit earned can be calculated as per the following equation:
where A1M.price is the assumed market price for product A1 and is constant. As , the cost of producing product A1, depends on the applied transformation method j, the total profit of an entity A1 is evaluated as a product of the summation of profit/unit and served demands of product A1 over all the periods for each transformation process j. is calculated using Equation 3.
To restrict the model to only one transformation process within a period, avoiding intra-period switching can be represented in mathematical form as the summation of the Boolean decision variable to ≤1 along the j dimension, and can be expressed as follows:
This inequality nine confirms that, at most, one of the transformations is valid in a specific period. The restriction of using one transformation method within a period is to reduce overheads. Inequality 10 forces the served demand to be less than the summation of the current production and inventory, brought forward from the previous period for all the periods:
Inequality 11 ensures that the demand served in a period cannot exceed the market demand:
Equation 12 represents the total waste units generated against the products produced using a method j in a period t:
Equation 13 represents the total waste units consumed against the products produced using a method j in a period t:
Due to the concept of IS and underutilized materials circulation, the generated wastes can be consumed anywhere in the cluster when required. In this regard, information and material exchange play crucial roles while producing the products. Equation 14 keeps track of the raw materials inventory at A1 in a period p, by relating to the waste generated and consumed using a method j.
Inequality 15 ensures that the underutilized materials can be consumed only when they are available in abundance:
where and for Equations 14 and 15.
Finally, Equation 16 tracks the end-product inventory from period to period by relating to the production in the current period and the demand served:
Equation 17 ensures that the demand can only be served when the product is available in the inventory or produced:
where the initial inventory is for both Equations 16 and 17.
While we aim to reduce the generated underutilized materials quantities, the mentioned objective function produces nothing at any entity in return, which is logical but does not make sense. Therefore, we set the model to produce at least 50% of the regional demands locally. The following equation represents the constraint applied in this regard:
To demonstrate the functionality of the proposed formulation, demand over the periods was assumed to be Gaussian for the five products manufactured at five entities in the industrial cluster, namely, N (500, 40), N (300, 70), N (600, 80), N (450, 90), and N (400, 100). The production capacity of each product per period is capped at 500, 450, 700, 650, and 600 units, respectively. The finished product inventory storage facility is capped at 190, 200, 150, 120, and 100 units, respectively.
4. Results and discussion
For the model's applicability, various parameters mentioned in Equation 3 are reasonably assumed to evaluate the total cost of the product/substitute using the transformation methods J = {1...3} and raw materials R = {1…11}. The cost using any method is kept constant over periods T = {1...20} for analysis purposes for each agent A = [1...5]. As only five agents are considered, they can be arranged as one agent surrounded by four to share attributes information. The assumptions regarding the costs of transforming materials and regional demands for all products are justifiable as they are subjective and region-dependent. Each entity has only one agent where the information regarding the material availability and requirements is recorded and then shared with the neighbors to produce a unique product. The identical and distributed nature of agents improves reliability and scalability. Also, for simplicity, the same type of intra-cluster underutilized materials from any entity are brought together in a joint stocking facility where the average cost of materials needs to be determined, as illustrated in Figure 3. Afterwards, as per requirements from agents, these materials are relocated to the optimal sinks with a mean sustainable transportation cost evaluated in Equation 3. Without the joint stocking facility, different sources incur different costs for the same type of underutilized material.
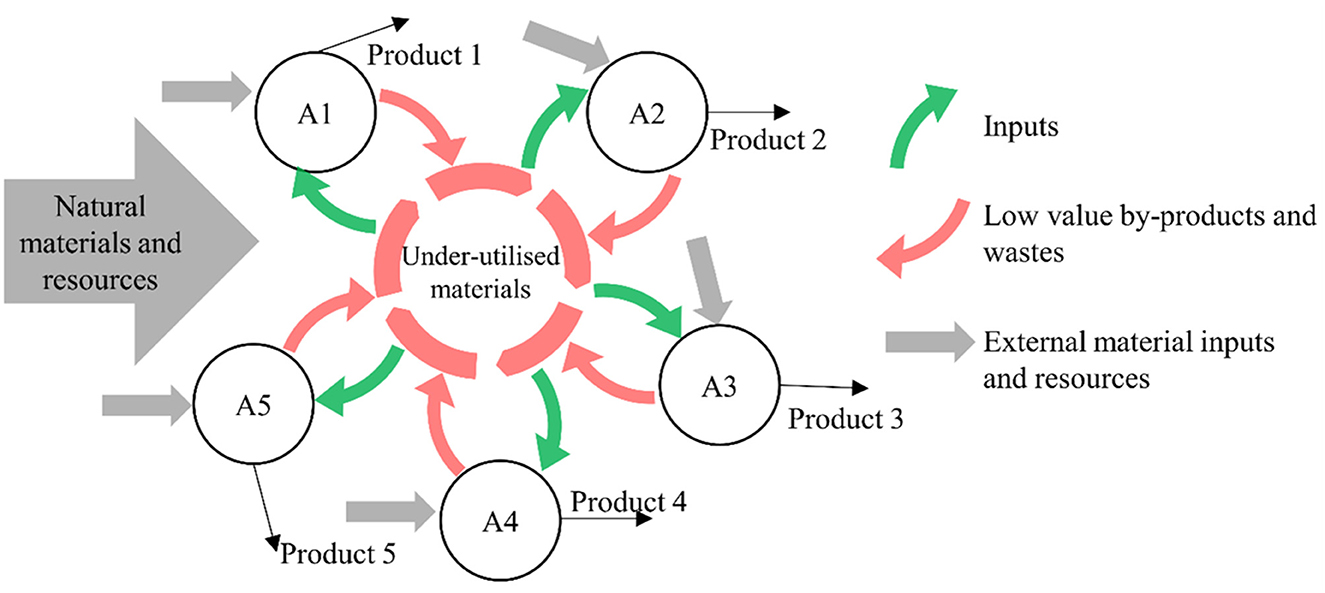
Figure 3. Illustration of an industrial cluster with entities A1...A5 using a joint stocking facility for intra-cluster underutilized materials.
To begin with, the system was initialized as no underutilized materials existed at any of the entities, and the natural resources were abundant. Afterwards, every agent keeps track of modifications in attributes according to the requirements of the transformations while fulfilling product demands. The costs for three best transformation methods to manufacture a product at an entity are assumed for analysis purposes based on a sustainability criteria by the governmental organizations based on the limitations of emissions with economical factors and standards of living considerations. Each feasible method utilizes natural resources or underutilized material or their combinations in specific proportions for producing products of comparable qualities to enhance the CE perspective while considering the economic aspect. Switching to a relatively better transformation method at an entity is subject to the abundance of the concerned underutilized materials availability to fulfill a product's regional demand for a specific period. No storage capacities are capped for materials in order to observe the actual model response based on an objective regarding accumulated/less competitive materials.
Allowing multiple transformation possibilities for a product or its substitute at each entity promotes the usage of the underutilized materials and therefore improves sustainability, which is evident from the results in this section. Also, considering different possible raw inputs in the feasible transformations for manufacturing a specific product or its substitutes can support selecting better raw materials and suppliers. Moreover, this improves the overall industrial cluster performance and fill rate. Three scenarios, namely, targeting natural resource utilization, the lowest cost option, and choosing sustainable options among multiple transformation methods, are considered for demonstration purposes, where the applied objective function is the same for all three cases. It is worth mentioning that the choice among transformation methods and enough available input materials or substitutes are vital to increase the resilience. In addition, the proactive nature of waste utilization as soon as it is generated and the uncertainty of the availability of enough raw materials all together control the transformational switching.
The performance or response of the model for all scenarios is elaborated on below, based on the produced low-value materials or waste, loss of opportunity, fill rate, and average profit percentage returned from an industry in the cluster, which can support governmental agencies to regulate markets by providing targeted financial support while promoting the CE perspective in an industrial cluster.
Tables 2–4 illustrates details of performance indicators against assumed parameters at the end of time horizon for specific objectives elaborated in the following subsections at each entity.
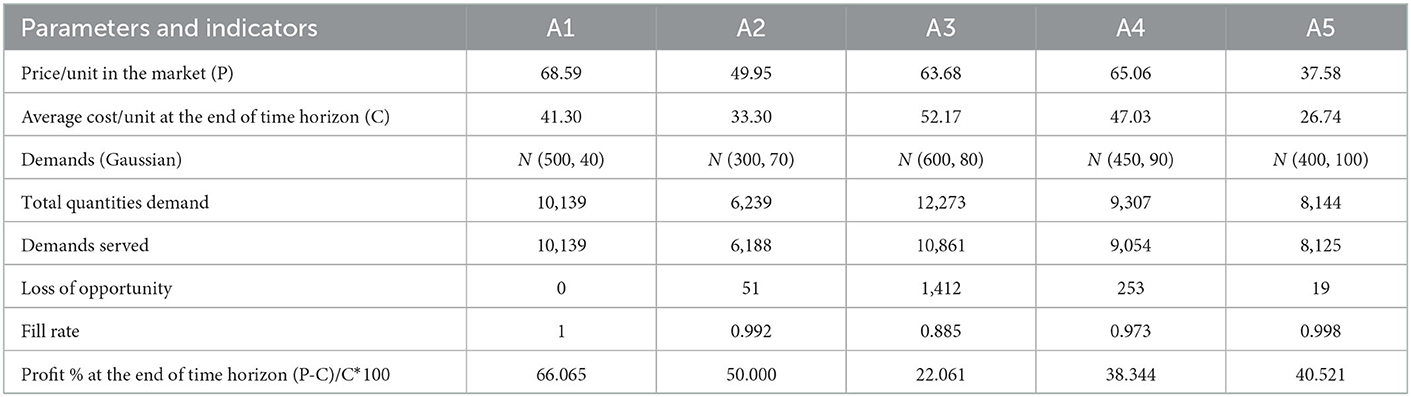
Table 4. Parameters and performance indicators when targeting sustainability using multiple transformation techniques.
4.1. When using natural resources only
In this study, the entities of the industrial system target the manufacture of products from natural resources only, causing their depletion without caring for the type and quantity of waste generated from any entities; therefore, the underutilized materials accumulate, as indicated in Figure 4. Also, a compromise on fill rate is observed for some products, for example, A3 and A5 are severely affected, while A2 and A4 are mildly affected. This case is considered a base case, where the consumers' demand for multiple products or substitutes is fulfilled irrespective of waste generation or consumption and profit consideration. The waste storage facility is not capped anywhere to observe the actual response of the model for approximating product cost convergence by the end of the time horizon. The response recorded in Figure 4 is that when there is no dependency on other producers for underutilized materials. It is noteworthy to mention that, in this scenario, the manufacturing cost of the end product may be more or less than the cost of producing using underutilized materials when evaluated using Equation 3. Generally, in an eco-industrial system, industrial entities tend more toward using natural resources; therefore, this is considered a business-as-usual case (Aggestam et al., 2021). It is assumed that every entity earns a fixed 50% of the cost incurred as profit when manufacturing a unit product utilizing natural resources, referred to as the price/unit in the market and denoted as P. The maximum value of the fill rate can reach one, which indicates fulfillment of all the requirements.
4.2. Targeting the lowest costs irrespective of waste generated (maximum profit case)
Every individual entity here independently strives for the lowest total cost evaluated according to Equation 3. Figure 5 indicates the model's response without switching among transformation methods options and considering waste accumulation. In this study, the production of the lowest cost product is independent of the type of material used as feedstock; therefore, the possibility exists of using underutilized materials generated in intra-clusters, along with their possible combinations for manufacturing a product. The underutilized materials may accumulate or become scarce. Therefore, while targeting the lowest cost option, the dependency on underutilized raw inputs is at the preceding stages of the material flow network, which badly affects the fill rate and therefore compromises profit. Also, being demand-driven, sometimes, due to less demand, the underutilized material accumulates, and loops cannot be closed. Moreover, while producing products with less cost from wastes/by-products, sometimes the demand is not fulfilled due to the scarcity of underutilized materials. Although the percentage profits are all improved in this case, the fill rate is badly affected except for product A2.
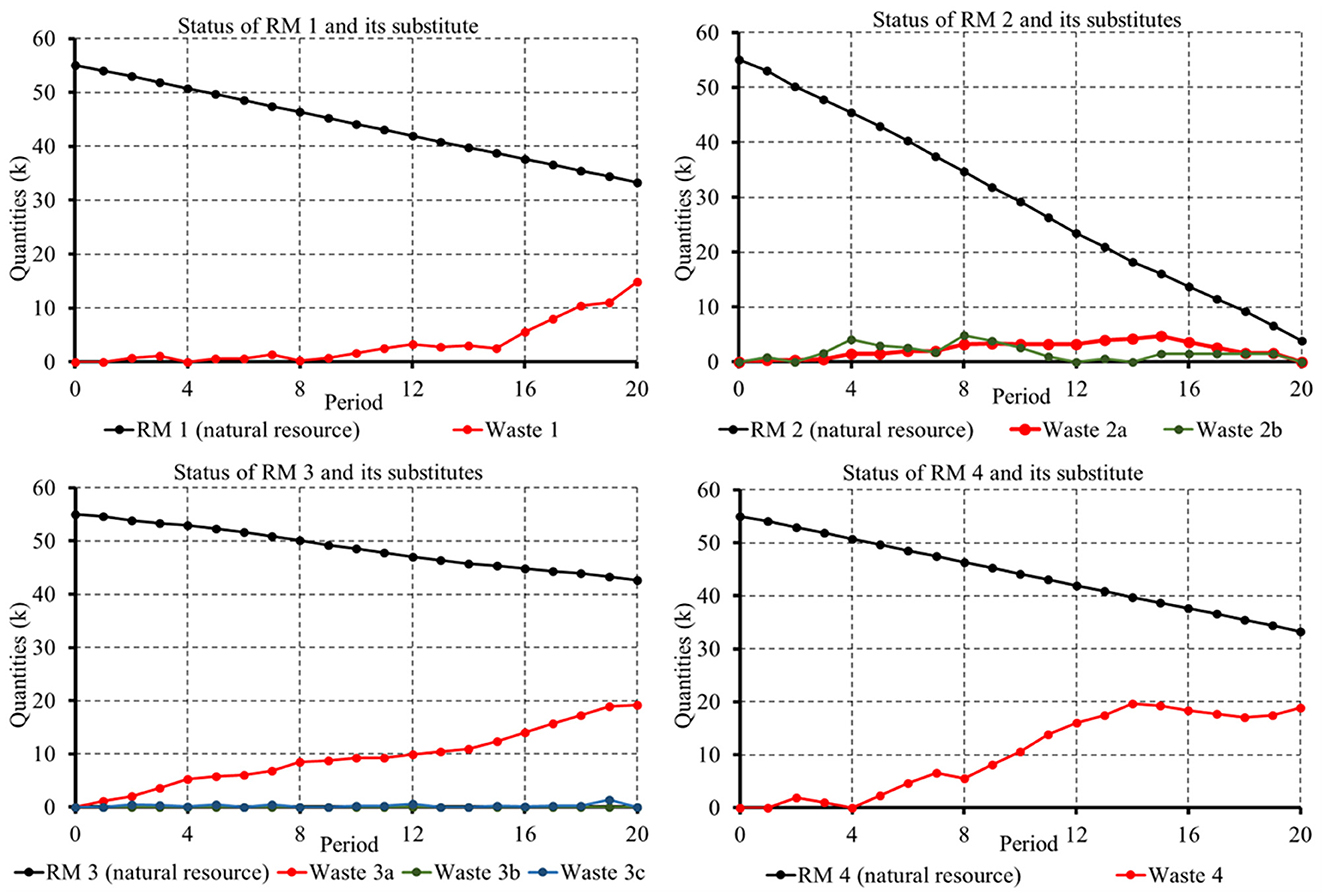
Figure 5. Intra-cluster materials' status over the time horizon, when using the lowest cost transformation technique (both underutilized materials and natural resources as feedstock are feasible).
4.3. Targeting flexible solutions using multiple transformation methods
Unlike the two scenarios above, switching to a relatively better way of making a product, depending on the objective, is allowed as soon as enough of the required underutilized materials are available to manufacture against demands in a period. The costs of utilizing materials are evaluated as per Equation 3. The underutilized materials availability depends on the other preceding product demands in the materials flow network, leading to uncertainty in the cost and availability of underutilized materials. The proposed model targets the maximum profit and waste utilization in the industrial network. An increase in the costs per unit of individual products is evident from the results while balancing profit and the maximum use of underutilized materials from other entities. In this study, the fill rate and the profit percentages are highest but at the cost of higher CAPEX due to multiple transformation methods for manufacturing a product from natural sources or intra-cluster by-products. The use of numerous transformation methods favors the fill rate, and frequent switching among the transformation methods helps avoid the accumulation of underutilized materials. The results indicate a relatively better balance between the profit, loss of opportunity, and underutilized materials while ensuring the highest fill rate. In terms of profitability, the results suggest that A3 is the most compromised, while A4 and A5 are mildly compromised economic entities (<50%: profit compared with the base case); therefore, external financing is required according to the compromise observed. In contrast, product A1 generates high profits. Furthermore, the combined gain of all entities in a holistic sense is also reduced to a great extent. Such insights can help governmental organizations to tax and subsidize for regulating the market.
Since the model uses multiple transformation options while targeting sustainability in the industrial cluster, Figure 6 indicates the variations in the cost of all five products in the industrial cluster, when allowing the use of three distinct feasible transformation methods for producing each product or its equivalent substitute. The cost convergence to a specific value after a few periods and the stability afterwards are valuable to investors and governments in predicting critical economic entities and assessing the effects of cost and product price for taking improved decisions. Furthermore, adopting the most expensive methods is crucial for some entities to (1) avoid material accumulation, increasing the average cost per unit product and, as such, converging to higher costs at the end of the time horizon; and (2) generate by-product materials that are highly valuable to another entity within the cluster. Hence, the optimized cluster approaches opt for the expensive manufacturing mode at some entities and, as such, affect the economic component. However, this profit compromise leads to a much higher profit for another entity in the cluster.
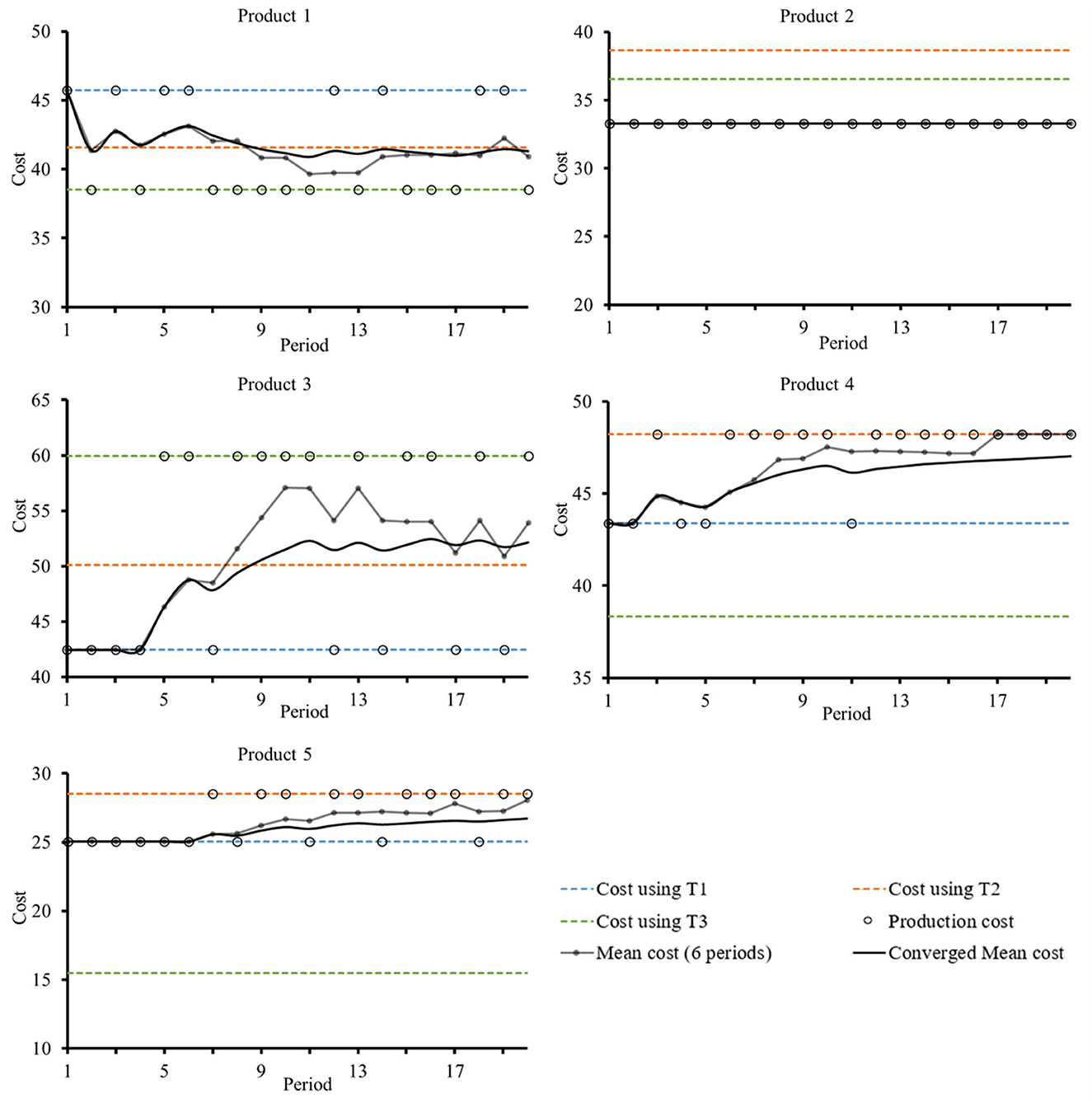
Figure 6. Details of cost convergence over the time horizon with the choice among the three transformation methods.
The material trends in Figures 4, 5, and 7 indicate uncertainty regarding the availability of raw materials and their competition level, providing valuable insights to managers and operators. Collectively, it is evident that effective utilization of by-products reduces the dependency on natural resources as input materials. A higher positive slope indicates rapid materials accumulation, and a higher negative slope indicates rapid depletion of materials. Furthermore, the scarcity of required underutilized material forces entities to adopt expensive materials and production methods in later periods, which impacts the average cost of some products produced within the cluster. In some scenarios, large amounts of waste accumulation are due to a lower waste utilization rate or high demand fluctuations of the preceding and succeeding product along the material flow network.
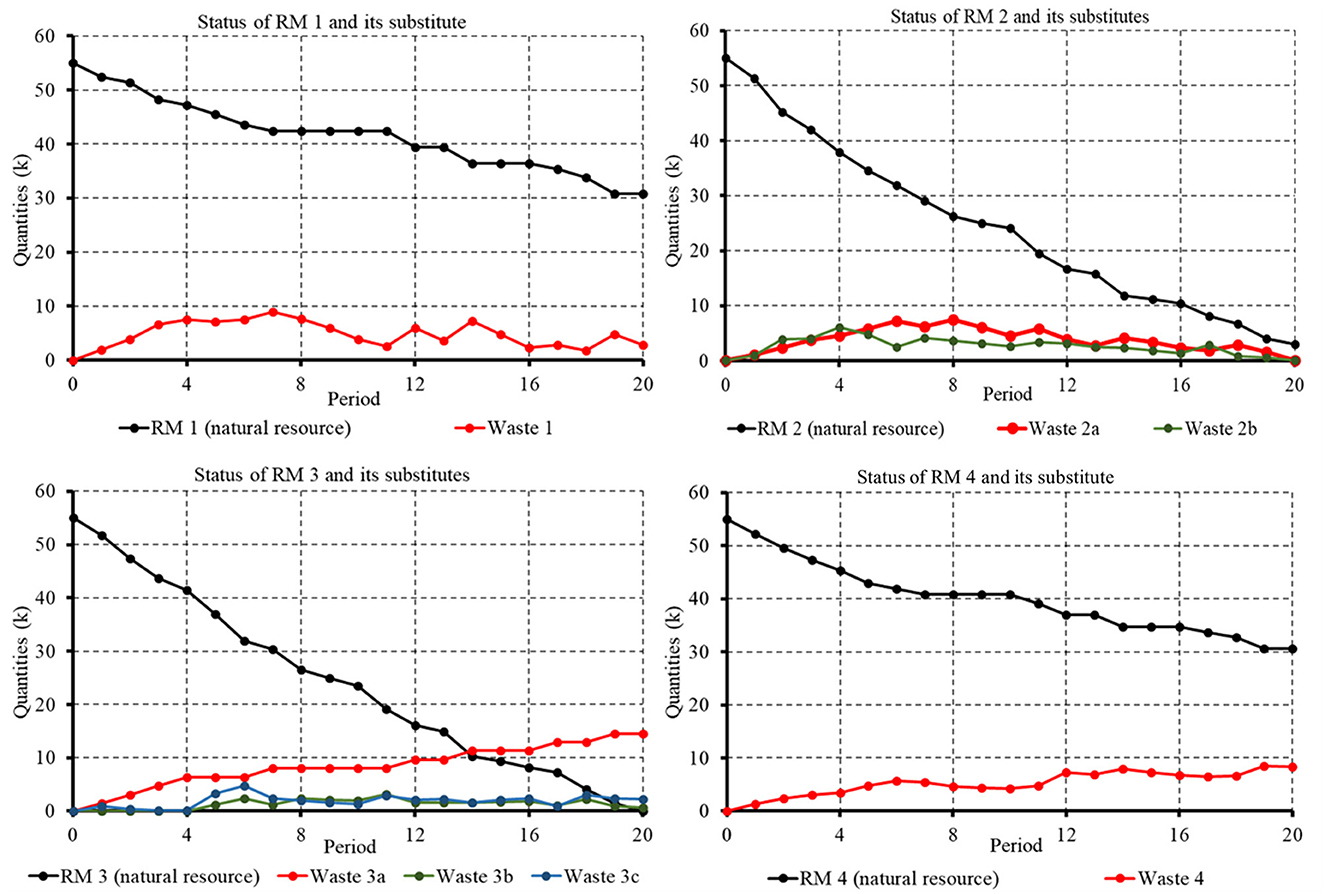
Figure 7. Response to the status of all materials in the cluster while targeting sustainability under multiple methods.
Generally, sustainable systems lead to improved ecology, but the end products may cost relatively more as they may need higher CAPEX or OPEX. Most often, while striving for sustainability, governments impose taxes on wastes/emissions producers, increasing product costs, affecting purchasing power, and eventually causing a low standard of living. Being part of market competition shifts the tendency to produce relatively low-cost products that may compromise quality or may have shorter lifetimes. Such taxes may influence the waste generation and utilization of by-products, providing a short-term solution to tackle climate change. When a solution compromises durability/quality, in the long term, this urges producers to produce more, which means the generation of more waste/emissions; therefore, the system may perform poorly in terms of sustainability. A better solution would be to explore more options for using underutilized materials. In addition, from region to region, the source and choice of raw materials vary, which implicitly concerns the viability of products or substitutes and the transformation processes to be applied. When closing the feasible connecting links based on CE, multiple factors must be considered to assess how a process or value addition affects sustainability.
5. Limitations
The limitation of this model is the cooperation through extensive communication mechanisms to share critical information with other entities, which is risky, and therefore, businesses usually do not agree. Also, moving the available intra-cluster raw materials based on type and quantity to suitable sinks may disrupt the financial benefits for some entities. Moreover, uncertainties regarding the continuous supply of enough raw materials due to the CE-based linked system may disrupt the supply chain. Furthermore, the customers must use an equivalent product substitute produced in the region instead of importing from other regions, affecting sustainability. Also, in this model, the dynamic cost variation for the underutilized materials is not considered based on competition level. The product cost in this model for each transformation method is considered constant throughout the time horizon; however, in practice, it may vary from period to period due to raw materials and transportation costs. For analysis to observe the convergence of cost, it is applicable.
6. Conclusion
In this study, the economic aspect of an individual entity in an industrial cluster is investigated, as its evaluation plays the most significant role in encouraging investors to promote sustainable development. This study aims to provide valuable insights into an industrial cluster's complex, linked entities to help progressively modify the cluster based on raw material availability and the assessment of economic impacts while closing material loops. Although, in practice, opportunities utilizing the generated wastes exist, regulating them through specific sustainability objectives from a CE perspective with incentives/financial support for investors, which is a must for maximum value extraction, lacks. Moreover, this aspect is highly neglected in the literature; therefore, this study aims to close the materials loop by addressing this aspect.
While targeting sustainability, the agent-based approach applied in this study helps identify the economically compromised entities due to any of the interdependent factors in an industrial manufacturing network. The provided insights can help evaluate the required level of financial support to enhance ecological efficiency based on the design of the cluster and the assessment of regional demands for a product based on intra-cluster raw materials availability. As such, identifying economically compromised entities using the presented approach is of value to governmental organizations, operators, and regulators for the arrangement of targeted financial support to incentivize investors, especially when enhancing sustainability and adapting to varying regional demands from adjacent industrial networks due to socioeconomic factors. Moreover, governments may intervene, where needed, by imposing taxes or providing subsidies to stimulate and regulate the price competitiveness of markets. Furthermore, this study also indicates that governmental financial intervention can play a vital role in improving the sustainability of industrial parks. Finally, promoting underutilized materials utilization through such an external intervention will also help preserve regional ecosystems and reduce the potentially unexpected environmental impacts of industrial production.
From an application perspective, besides the discussed scenarios, this approach is equally applicable to utilizing both technical and biological materials, irrespective of the region, which can be achieved through appropriate adjustments of the parameters in the presented model.
Data availability statement
The original contributions presented in the study are included in the article/supplementary material, further inquiries can be directed to the corresponding author.
Author contributions
MI: conceptualization, methodology, formal analysis, modeling, and writing and drafting original draft. TA-A: conceptualization, methodology, writing, review and editing, and supervision. All authors contributed to the article and approved the submitted version.
Funding
This research was supported by Hamad Bin Khalifa University, a member of the Qatar Foundation, Doha, Qatar.
Conflict of interest
The authors declare that the research was conducted in the absence of any commercial or financial relationships that could be construed as a potential conflict of interest.
Publisher's note
All claims expressed in this article are solely those of the authors and do not necessarily represent those of their affiliated organizations, or those of the publisher, the editors and the reviewers. Any product that may be evaluated in this article, or claim that may be made by its manufacturer, is not guaranteed or endorsed by the publisher.
References
Aggestam, F., Prins, K., and Tulsidas, H. (2021). Natural Resource Nexuses in the ECE Region. New York, NY: United Nations Publications.
Baldassarre, B., Schepers, M., Bocken, N., Cuppen, E., Korevaar, G., and Calabretta, G. (2019). Industrial symbiosis: Towards a design process for eco-industrial clusters by integrating Circular Economy and Industrial Ecology perspectives. J. Clean. Prod. 216, 446–460. doi: 10.1016/j.jclepro.2019.01.091
Batten, D. F. (2009). Fostering industrial symbiosis with agent-based simulation and participatory modeling. J. Indus. Ecol. 13, 197–213. doi: 10.1111/j.1530-9290.2009.00115.x
Bechara, C. A., and Alnouri, S. Y. (2022). Energy assessment strategies in carbon-constrained industrial clusters. Energy Conv. Manag. 254, 115204. doi: 10.1016/j.enconman.2021.115204
Bourguignon, D. (2015). “Understanding waste streams,” in EPRS. European Parliamentary Research Service, 1–12. Available online at: http://www.europarl.europa.eu/EPRS/EPRS-Briefing-564398-Understanding-waste-streams-FINAL.pdf (accessed September 2022).
Brings Jacobsen, N. (2006). Industrial symbiosis in Kalumdborg, Denmark, a quantitative assessment of economic and environmental aspects. J. Indus. Ecol. 10, 18. doi: 10.1162/108819806775545411
Castiglione, C., Pastore, E., and Alfieri, A. (2022). Technical, economic, and environmental performance assessment of manufacturing systems: The multi-layer enterprise input-output formalisation method. Prod. Plan. Control 2022, 1–18. doi: 10.1080/09537287.2022.2054743
Chanfreut, P., Maestre, J. M., and Camacho, E. F. (2021). A survey on clustering methods for distributed and networked control systems. Ann. Rev. Control 52, 75–90. doi: 10.1016/j.arcontrol.2021.08.002
Choi, T.-M. (2022). Achieving economic sustainability: Operations research for risk analysis and optimisation problems in the blockchain era. Ann. Operat. Res. 21, 5. doi: 10.1007/s10479-021-04394-5
Daddi, T., Nucci, B., and Iraldo, F. (2017). Using Life Cycle Assessment (LCA) to measure the environmental benefits of industrial symbiosis in an industrial cluster of SMEs. J. Clean. Prod. 147, 157–164. doi: 10.1016/j.jclepro.2017.01.090
Davis, C., Nikolíc, I., and Dijkema, G. P. J. (2009). Integration of life cycle assessment into agent-based modeling toward informed decisions on evolving infrastructure systems. J. Indus. Ecol. 13, 306–325. doi: 10.1111/j.1530-9290.2009.00122.x
Delgado, M., Porter, M. E., and Stern, S. (2014). Clusters, convergence, and economic performance. Res. Pol. 43, 1785–1799. doi: 10.1016/j.respol.2014.05.007
EPA. (2000). Guide for Industrial Waste Management: Protecting Land, Ground Water, Surface Water, Air. Industrial Waste Management. p. 1–478.
Farmer, J. D., and Foley, D. (2009). The economy needs agent-based modelling. Nature 460, 685–686. doi: 10.1038/460685a
Feng, S., and Bai, Y. (2021). Coordinated development of airport economy in China: Impact of “Airport-Industry-City” coordination on regional economic performance. Ann. Operat. Res. 21, 5. doi: 10.1007/s10479-021-04340-5
Fraccascia, L., and Yazan, D. M. (2018). The role of online information-sharing platforms on the performance of industrial symbiosis networks. Resour. Conserv. Recycl. 136, 473–485. doi: 10.1016/j.resconrec.2018.03.009
Ghisellini, P., Cialani, C., and Ulgiati, S. (2016). A review on circular economy: The expected transition to a balanced interplay of environmental and economic systems. J. Clean. Prod. 114, 11–32. doi: 10.1016/j.jclepro.2015.09.007
Guirlanda, C. P., da Silva, G. G., and Takahashi, J. A. (2021). Cocoa honey: Agro-industrial waste or underutilised cocoa by-product? Fut. Foods 4, 100061. doi: 10.1016/j.fufo.2021.100061
Hu, W., Tian, J., Li, X., and Chen, L. (2020). Wastewater treatment system optimisation with an industrial symbiosis model: A case study of a Chinese eco-industrial park. J. Indus. Ecol. 24, 1338–1351. doi: 10.1111/jiec.13020
Huang, L., Zhen, L., and Yin, L. (2020). Waste material recycling and exchanging decisions for industrial symbiosis network optimisation. J. Clean. Prod. 276, 124073. doi: 10.1016/j.jclepro.2020.124073
Huang, Y. Q., Liu, J. R., and Wang, X. H. (2015). Agent-based modeling and simulation of agro-industrial compound eco-industrial park. J. Ecol. Rural Environ. 31, 301–307. doi: 10.11934/j.issn.1673-4831.2015.03.005
Koshy-Chenthittayil, S., Archambault, L., Senthilkumar, D., Laubenbacher, R., Mendes, P., and Dongari-Bagtzoglou, A. (2021). Agent based models of polymicrobial biofilms and the micro-biome—A review. Microorganisms 9, 1–23. doi: 10.3390/microorganisms9020417
Laybourn, P. (2021). How Symbiosis Can Help Industrial Clusters Reach Net-Zero. World Economic Forum. Available online at: https://www.weforum.org/agenda/2021/03/industrial-symbiosis-clusters/ (accessed September 2022).
Lyu, Y., Liu, Y., Guo, Y., Tian, J., and Chen, L. (2021). Managing water sustainability in textile industry through adaptive multiple stakeholder collaboration. Water Res. 205, 117655. doi: 10.1016/j.watres.2021.117655
Mehdiyeva, I., Kerimli, V., Gafarov, N., Sultanova, N., Heydarova, K., and Taghiyev, A. (2020). Barriers and drivers of the implementation and management of green agri-food supply chains in Azerbaijan. Int. J. Supply Chain Manag. 9, 527–535.
Moss, M. B. (2007). “Comparing centralized and distributed approaches for operational impact analysis in enterprise systems,” in 2007 IEEE International Conference on Granular Computing (San Jose, CA: IEEE), 765–765. doi: 10.1109/GrC.2007.130
Nørstebø, V., Krøgli, S. O., Debella-Gilo, M., Perez-Valdes, G. A., Uggen, K. T., and Dramstad, W. E. (2020). Identifying suitable bioeconomic cluster sites—Combining GIS-MCDA and operational research methods. Environ. Model. Assess. 25, 689–703. doi: 10.1007/s10666-020-09694-x
OECD. (2007). Material Resources, Productivity and the Environment: Key Findings. Paris: OECD. p. 1–14.
Perez-Valdes, G. A., Nørstebø, V. S., Ellingsen, M. B., Teräs, J., and Werner, A. T. (2019). Bioeconomic clusters-background, emergence, localisation and modelling. Sustainability 11, 174611. doi: 10.3390/su11174611
Porter, M. E. (2003). The economic performance of regions. Region. Stud. 37, 545–546. doi: 10.1080/0034340032000108688
Romero, E., and Ruiz, M. C. (2013). Framework for applying a complex adaptive system approach to model the operation of eco-industrial parks. J. Indus. Ecol. 17, 731–741. doi: 10.1111/jiec.12032
Simonis, U. E., and Ayres, R. U. (1995). Industrial metabolism: Restructuring for sustainable development. Glob. Environ. Change 5, 157. doi: 10.1016/0959-3780(95)90041-1
Taghikhah, F., Voinov, A., Shukla, N., Filatova, T., and Anufriev, M. (2021). Integrated modeling of extended agro-food supply chains: A systems approach. Eur. J. Operat. Res. 288, 852–868. doi: 10.1016/j.ejor.2020.06.036
UN. (2022). The Sustainable Development Goals Report 2022. United Nations Publication Issued by the Department of Economic and Social Affairs, United Nations. p. 64.
UNEP. (2015). Sustainable Consumption and Production. In Earth Summit 2002: A New Deal. Nairobi: UNEP.
Wang, D., Li, J., Wang, Y., Wan, K., Song, X., and Liu, Y. (2017). Comparing the vulnerability of different coal industrial symbiosis networks under economic fluctuations. J. Clean. Prod. 149, 636–652. doi: 10.1016/j.jclepro.2017.02.137
Wardhana, D., Ihle, R., and Heijman, W. (2020a). Effects of peer pressure in agro-clusters of west JAVA. Bullet. Indones. Econ. Stud. 2020, 1–34. doi: 10.1080/00074918.2020.1780197
Wardhana, D., Ihle, R., and Heijman, W. (2020b). Farmer cooperation in agro-clusters: Evidence from Indonesia. Agribusiness 36, 725–750. doi: 10.1002/agr.21637
Wooldridge, M., and Jennings, N. R. (1995). Intelligent agents: Theory and practice. Knowl. Eng. Rev. 10, 115–152. doi: 10.1017/S0269888900008122
World Economic Forum (2019). WEF Supply Chain 4.0 2019 Report. White Paper, January. Available online at: http://www3.weforum.org/docs/WEF_Supply_Chain_4.0_2019_Report.pdf (accessed September 2022).
Worrell, E., Allwood, J., and Gutowski, T. (2016). The role of material efficiency in environmental stewardship. Ann. Rev. 41, 575–598. doi: 10.1146/annurev-environ-110615-085737
Wu, S. R., Li, X., Apul, D., Breeze, V., Tang, Y., Fan, Y., et al. (2017). Agent-based modeling of temporal and spatial dynamics in life cycle sustainability assessment. J. Indus. Ecol. 21, 1507–1521. doi: 10.1111/jiec.12666
Yazan, D. M., Fraccascia, L., Mes, M., and Zijm, H. (2018). Cooperation in manure-based biogas production networks: An agent-based modeling approach. Appl. Energy 212, 820–833. doi: 10.1016/j.apenergy.2017.12.074
Yu, Y., Yazan, D. M., Bhochhibhoya, S., and Volker, L. (2021). Towards circular economy through industrial symbiosis in the Dutch construction industry: A case of recycled concrete aggregates. J. Clean. Prod. 293, 126083. doi: 10.1016/j.jclepro.2021.126083
Zheng, K., and Jia, S. (2017). Promoting the opportunity identification of industrial symbiosis: Agent-based modeling inspired by innovation diffusion theory. Sustainability 9, 50765. doi: 10.3390/su9050765
Zommers, Z., van der Geest, K., de Sherbinin, A., Kienberger, S., Roberts, E., Harootunian, G., et al. (2016). Loss and Damage: The Role of Ecosystem Services. Available online at: http://collections.unu.edu/view/UNU:5614 (accessed September 2022).
Keywords: decision support systems, waste materials utilization, industrial ecology, agent based modeling, circular economy, industrial production, sustainable development
Citation: Ismail M and Al-Ansari T (2023) Enhancing sustainability within industrial cooperative networks through the evaluation of economically compromised entities. Front. Sustain. 4:1089450. doi: 10.3389/frsus.2023.1089450
Received: 04 November 2022; Accepted: 02 January 2023;
Published: 27 January 2023.
Edited by:
Fausto Cavallaro, University of Molise, ItalyReviewed by:
Miguel Sellitto, University of the Rio dos Sinos Valley, BrazilMing Lang Tseng, Asia University, Taiwan
Copyright © 2023 Ismail and Al-Ansari. This is an open-access article distributed under the terms of the Creative Commons Attribution License (CC BY). The use, distribution or reproduction in other forums is permitted, provided the original author(s) and the copyright owner(s) are credited and that the original publication in this journal is cited, in accordance with accepted academic practice. No use, distribution or reproduction is permitted which does not comply with these terms.
*Correspondence: Tareq Al-Ansari, dGFsYW5zYXJpQGhia3UuZWR1LnFh