- 1Univ Gustave Eiffel, MAST-GPEM, Bouguenais, France
- 2Institut de Recherche en Sciences et Techniques de la Ville (IRSTV) - CNRS, Nantes, France
Transition can be defined as deep-structural changes in interrelated economic sectors. Climate change issue calls for a transition in which all actors can be involved and where the aim is the global interest rather than individual ones. LCA should be used to assess the environmental impacts of transition solutions, however, existing LCAs are not adapted mainly because they are conducted at product-level and rely upon economic assumptions that are unsuitable for a transition context. First typologies of LCAs are redefined, based on general system theory, according to the object under study and their model structures. A new type of LCA, called transition LCA is conceptually described. It aims at studying transition scenarios in a geographical context, and that can be relied to various technological paths. Differences with existing LCAs is that several functional units with varying amounts can be included, and that its results concern the changes of the system itself rather than the individual impacts of products. Transition LCA is also related to a specific geographical context in order to account for existing limits of resources and especially constraint resources such as waste, and to account for the match of flows between production and demand which determines the size of the geographical area. This type of modeling can be reached by coupling LCA with MFA at the suitable geographical scale. Transition LCA also requires specific interpretation steps dedicated to the identification of preferable scenarios and action levers hold by each involved actor. This interpretation requires integrating actors' identification and their decision models as mechanisms inside the system model. It also requires additional methods to systematically generate all actions possibilities and to conduct suitable sensitivity analysis. This method has presently been applied by parts but not as a whole and not as a single numerical tool. These parts are currently being developed in a single computing Python language, in order to become compatible with Brightway 2.
Introduction
Transition can be defined as deep-structural changes in interrelated economic sectors, and because these systemic changes affect the overall configuration of these sectors they can be called socio-technical transitions (Geels, 2011). Today's environmental issues, and particularly climate change, call for a transition in which all actors, such as private companies, policy makers, consumers, civil society, engineers, researchers, as well as their systems of interaction, whether economic, regulatory or social, are questioned in a multi-level perspective (Geels, 2011).
According to (Ceschin and Gaziulusoy, 2016) who defined a typology of transition solutions, there are four general levels of solutions: (i) Product level about changing products' environmental footprint during their life cycle, encouraging an emotional attachment between a product and a consumer to reduce frequent renewal, designing products that facilitate sustainable behavior of consumers, developing biomimetic products, or designing products that only respond to essential needs such as low technology; (ii) product service system level, focusing on services for consumers instead of products like sharing economy solutions; (iii) spatio-social innovation level, such as design for social innovation encouraging collaboration between individuals, or systemic design that favors collaborations between existing industries for reuse or recycling and; (iv) socio-technical system innovation level seen as the collaboration of groups of individuals and economic actors to co-design their desirable future together.
Among general perspectives imagined to respond the challenge, a wide variety of currents of thought coexist for socio-technical and spatio-social levels, which differ in their solutions through very diverse approaches that advocate social changes, or rely essentially on technological developments and/or ruptures, or on changes in economic systems. For example, circular economy is one of these general frames. Although many definitions of circular economy can be found, this current of thoughts generally assumes that its solutions will reduce natural resource extraction in general by extending the life, reusing and recycling the products. Another example concerns digital transition. Some currents of thoughts recommend to rely upon high digital technologies, with many examples such as avoiding transports with teleworking (Guerin, 2021), better control and adapt buildings consumption with connected objects and artificial intelligence (Chauhan and Chauhan, 2019) etc; while others go “against the grain to argue that “high” technology will not solve global problems and envisages a different approach to build a more resilient and sustainable society” by focusing on the essential functionalities and low-tech (Bihouix, 2020). Shared economy is also a common paradigm invocated for ecological transition. In urban planning policies, solutions for active mobility and shared vehicles are often seen as sustainable solutions to cities passenger's transports (Filippova and Buchoud, 2020) but in the same time, the necessary increase of adapted vehicles and infrastructure for shared mobility can drive to considerably lower their environmental benefits (de Bortoli, 2021), or even to a considerable increase of material consumption according to the choice of implementation mode and to consumer's behavior (Chen et al., 2020). Many other examples could be listed for which no clear answers about their environmental performances can be found.
Whatever the school of thought, Life Cycle Assessment (LCA) should be used to assess the environmental impacts of such transition solutions, in order to evaluate their relevancy for that purpose. However, LCAs are not conducted to assess transition solutions. Indeed, the common ground between all these possible transition solutions is that: they intend to significantly and massively replace an existing situation with the aim of reducing environmental impacts, they involve cooperation between different actors (public policies, companies, citizens…) and in order to be successfully implemented each of the involved actor needs to know its role in the general system, several products' life cycle can be involved, their environmental performances may depend on very local constraints and thus implementation conditions are crucial, rebound effects can be important, and they can involve changes in functionalities. The LCA method able to integrate all these characteristics does not exist as will be shown in the first section of this paper. Thus, a new methodological frame named Transition LCA (Tr-LCA), is proposed in the second section, in order to respond these key issues.
Analysis of existing LCA methods
LCA is a general frame inside which different types of methods can be used. The designation of an LCA method can refer either to the object under study such as product, territorial or organizational LCAs or to the structure of the system model such as attributional or consequential. In this section, it is suggested to analyse the methodological characteristics implied by these two aspects (the studied object and the system model) in order to clearly define Tr-LCA compared to other methods.
Types of studied objects with LCA
Characteristics of different objects LCAs
LCAs can be applied to the study of different objects, and the type of object under study determines the functional unit, the number of actors or products concerned, as well as the number and value of the reference flows. This is resumed in Table 1.
Product LCAs (P-LCA) analyse environmental impacts relatively to a unitary functional unit (impacts per one amount of functional unit). Commonly the foreground system of P-LCAs only concerns one actor, although some studies have been conducted with several foreground systems corresponding to different actors (Senga Kiessé et al., 2017).
Territorial LCAs (Te-LCA) have been divided into two types (Loiseau et al., 2018): type A [Te-LCA (A)] study a technology anchored in a known territory of which functions may vary according to that territory, and type B [Te-LCA (B)] study one territory with all its functions and related activities. In both methods, the foreground system is inside the considered territory. Similarly to P-LCA, Te-LCAs (A) assess environmental impacts relatively to a unitary functional unit, but it may include several actors if these are located inside the considered territory. Te-LCAs (B) assess environmental impacts relatively to the total amounts of products that are produced and consumed inside the considered territory and thus all concerned actors inside this territory. Some Te-LCAs (B) define functionalities of the territory according to a territorial diagnosis (Loiseau et al., 2014), thus functionalities are complex and multidimensional and there is no significance in using such Te-LCAs (B) to compare different territories. These Te-LCAs (B) are thus more relevant to analyse and possibly seek for improvements inside the studied territory. Other Te-LCAs (B) simply consider the number of inhabitants as a functional unit, and this can be understood that the main purpose of a territory is to accommodate a population. This type of Te-LCAs (B) are used to compare different territories (Goldstein et al., 2013).
Organizational LCAs (O-LCA) consider a single actor that can be located in different places and produce several products. The O-LCA guidance report (Martinez Blanco et al., 2015) specifies that there is no possible comparison between organizations as activities and products are very different, and thus O-LCA aims at seek for self-improvement by organizations. However, similarly to (Goldstein et al., 2013) with Te-LCAs (B), one could define economic profit as the main function of an organization and thus compare different organizations by calculating the environmental impacts generated per monetary unit of profit. But this type of approach has not been conducted yet, and existing O-LCAs assess environmental impacts relatively to the total amounts of products that are produced by the studied organization.
As explained in the introduction, transitions at spatio-social or socio-technical levels aim at proposing new technical and/or organizational solutions in order to substitute an existing situation assumed to be unsustainable. Although the existing situation may not be specific to a territory, Tr-LCA must consider that solutions to substitute are territorial dependant by default, as their environmental relevance can be highly dependent on local conditions [similarly to Te-LCAs (A)]. Solutions to substitute must also by default be considered multi-products and multi-actors involved in either existing situation and substitution scenarios, thus implying for Tr-LCA [similarly to O-LCAs and Te-LCAs (B)] to consider total amounts of products as reference flows. Furthermore, functionalities can change between existing situation and investigated scenarios, thus Tr-LCA should clearly define existing and investigated functionalities similarly to Te-LCAs (B) of Loiseau et al. (2014).
The question of actors and their relationships
Although Te-LCAs can consider several actors in their foreground systems (see Table 1), the question of possible competition or cooperation is not addressed as such in any type of LCA. The success of a transition solution is a matter of both environmental improvement as a goal, and of cooperation between actors as a mean.
Tr-LCA should thus enable to highlight these possible interactions between actors. What cooperation is necessary to reach the goals of a transition solution? Are there any synergies or antagonisms between actors regarding environmental issues or economic issues? Which actor or group of actors can control a decision?
To address this issue, the structure of the Tr-LCA must allow the integration of actors and specify their control over decisions that will affect either the technological processes at the origin of environmental impacts or the substitution mechanisms between the initial situation and the transition solutions.
Structural integration of actors in LCA models is in fact not specific to Tr-LCA, it has been already been developed on P-LCAs using parametrized inventory models and determining action levers using sensitivity analysis (Andrianandraina et al., 2015; Senga Kiessé et al., 2017; Ta et al., 2018; Duran Quintero et al., 2021). This approach will however be deepened and more generalized in this paper.
System modeling structures in LCA
LCA is a methodological framework derived from the much broader framework of the systems modeling family initiated by Bertalanffy (1968). Systemic modeling is based on the definition of a system according to a purpose with boundaries defining its interior and exterior. The rules defining the system's boundaries are related the purpose of the system model. The relations between the interior and the exterior of a system are defined by the nature of these exchanges as well as rules concerning quantification of these exchanges. In the general system theory, the interior of the system is composed of elements that are linked one another by exchanges also specified by their natures and by quantification rules. System modeling is suitable for complex modeling because: (i) the exchanges between elements of the system may have feedback loops, and (ii) elements inside the system may also be considered as smaller systems included in the larger system, which corresponds to the fact that a system model may have several levels of organization. The structure of a systemic model is defined by the nature of its elements, the nature of the exchanges between the elements, the nature of the exchanges between the inside and the outside of the system, as well as the rules defining the system's boundaries.
The methodological framework of LCA can be re-examined with this perspective, to analyse the existing modeling structures. For all LCAs, basically, the exchanges between the system and its exterior are elementary flows, crossing the boundary between the technosphere and the ecosphere. The elements of the system are named processes (or activities) and they are related by intermediate flows. However, some differences exist between different types of LCAs concerning intermediate flows and system boundaries. These differences are detailed below and resumed in Table 2.
The nature of intermediate flows and the rules to calculate their amounts
One of the main differences between the different types of LCAs concerns the nature of the intermediate flows and the rules defining their amounts. According to this perspective, one can differentiate three types of LCAs that differ in their system modeling structures: attributional LCA (A-LCA), consequential LCA (C-LCA) and input/output LCA (IO LCA).
For A-LCA, the nature of intermediate flow is physical, and their amounts depend on consumed and produced amounts (mostly assumed proportional) relatively to the value of the reference flow.
For C-LCA, one integrates in the system model an economic market of competing objects with identical functionalities that is shifted by the marginal increase of the demand for the object under study. For this particular part of the system, the way to obtain amounts exchanged between processes have changed compared to A-LCA: they depend on a new model that represents the market equilibrium of functional equivalent competing objects and thus requires additional conditions and information. This type of structure also includes the system expansion with substitution models, that can be considered as a very simplified consequential model with only one rule: the functional equivalence between the studied object and the object to substitute, without any market considerations. That is equivalent to assume the maximum possible substitution (100% market shift) in favor of the studied object, i.e., the total replacement of the object to substitute by the studied object.
The IO LCA is based on the so-called Leontief matrix that basically represents monetary exchanges and interdependencies between macroeconomic sectors (Suh, 2005). Although they can be converted into physical flows according to macroeconomic statistics, the nature of the exchanges and their amounts are obtained from monetary flows and based on monetary exchange rates as well as on reference monetary values.
Despite their differences, these three modeling structures have been shown to be consistent within a unified LCA theoretical framework (Suh et al., 2010; Majeau-Bettez et al., 2014), although each of them require different types of data collected at different scales (Ekvall et al., 2016). This compatibility allows to conduct hybrid LCAs that are not another system model structure but combine either A-LCAs or C-LCAs with IO LCAs.
The rules to define the system's boundaries
Rules concerning system boundaries in LCA are related to the different methods that can be used to deal with multi-output processes and waste recovery. In accordance with the general system theory (Bertalanffy, 1968), the rules to define boundaries of a system depend on the purpose of the system model.
For A-LCA of which aim is to describe actual and/or forecasted specific and/or average life cycle of a studied object relatively to its functional unit into a static technosphere (Ekvall et al., 2016), the boundaries are defined by partitioning processes according to different possible rules (cut-off, partitional allocation).
For C-LCA of which aim is to describe expected changes in response to possible decisions or to changes in demand for the functional unit into a dynamic technosphere (Ekvall et al., 2016), the boundaries are expanded compared to A-LCA, to include activities to the extent that they are expected to change as a consequence of the investigated changes (Sonnemann and Vigon, 2011). Let's remark that this system expansion is not a general rule applied to all activities of the entire system, but only to those under study, i.e., those in the foreground system. This implies that for the rest of the system, i.e., background system, boundaries are set according to the rules of A-LCA.
For IO LCA, boundaries definition are not necessary, because all interactions between economic sectors are accounted.
Hybrid LCAs cannot be considered as a new system model structure, in fact they combine either A-LCA or C-LCAs in the foreground system and IO LCA in the background system and thus adopt their rules in for each part of the system.
Which system model for transition LCAs?
According to the above analysis, C-LCA should be more adapted for Tr-LCA of which objective is to assess changes induce by transition solutions in substitution to an existing situation. According to Thomassen et al. (2008), it is required to have a knowledge of physical and market mechanisms in C-LCA. These market mechanisms have been described (Weidema, 2003) for P-LCAs. Substitution mechanisms of a product by the new product introduced are based on several assumptions: (i) the change of market is assumed small compared to the total volume of the considered market; (ii) the time-horizon is long term; (iii) the market segment is narrow (i.e., secondary and substituted products are very similar); (iv) the market trend corresponds to an overall increasing volume; (v) production constraints only apply to by-products with a low-value relatively to other co-products from the same joint production process; (vi) the affected (i.e., substituted technology) is assumed the most competitive and modern technology; (vii) the value to weight ratio determines the geographical market (local market for low ratio, continental for medium and global for high).
However, these basic assumptions cannot always be suitable for transition issues. Whereas transition requires important changes at various spatio-social or socio-technical levels (Geels, 2011), C-LCA is only suitable for small changes at a market level for one studied product. C-LCA forecasts a business-as-usual situation, with overall increasing market volumes in the long term, whereas transition can expect that some market volumes would decrease, and possibly new macro-economic models based on degrowth (Jackson, 2016).
Concerning geographical aspects, hybrid or IO LCAs are not either adapted because IO databases are produced at national scales, in a top-down approach with insufficient details concerning the very studied solutions. The granularity of general economic equilibrium is worldwide, referring to generic activities or industrial sectors. It represents background system in LCA, and compatible with hybrid modeling (Suh et al., 2004) at country, continent or world scales. If they can be useful for background system data, they are not useful for small economic markets. Because many transition initiatives are local, and a bottom-up approach is needed with a definite geographical scale for impact assessment, before it can possibly be evaluated at a larger scale.
In addition, C-LCA only relies upon economic market mechanisms as they are existing today. However, transition solutions at spatio-social or socio-technical levels can concern changes in the economic mechanisms themselves, and rely upon other mechanisms such as behavioral changes, submission to local constraints like physical limits (like maximum resource availability), size of population, etc. Thus, new foreground system models should be proposed for Tr-LCAs where the system should be expanded “to include activities to the extent that they are expected to change as a consequence of the investigated transition solutions” similarly to C-LCA (see Table 2), but the expansion should rely upon different aspects than only existing economic mechanisms. This will be detailed in the next section.
Transition LCA: A new frame for environmental assessment of transition scenarios
According to the analysis of the previous section, the new Tr-LCA method is detailed in this section. It is based on the following principles: inclusion of the geographical context, expanding systems, accepting several and variable functional units and account for total values of flows.
One must keep in mind, that similarly to all other types of LCAs, Tr-LCA is a frame that is implemented iteratively: as the studying process unfolds, the interpretation and critical analysis of the results make it possible to revisit the previous steps and possibly reconsider the objectives.
Goal and scope, functional units
Studying transitions is not about being predictive, it is about examining the range of possibilities to determine different paths toward the objectives of change. The questions to answer when studying transition is more about comparing several possible scenarios to enable choosing a path toward a more sustainable system, rather than predicting effects of changes. This type of approach is today not accounted among classical objectives of LCAs (Schrijvers et al., 2020). As highlighted previously (Zamagni et al., 2012) for studying transitions as quick and drastic changes in an existing system and more focused on possible paths to reach a new socio-economic system rather than modeling the future system itself, only scenario modeling, including possible political, economic, or social breaks, can bring information and guide choices toward possible futures.
In Tr-LCA, it is the existing situation to be substituted under study that is assessed and not individual products inside that system. Thus, the goal of Tr-LCA is to compare an existing situation, modeled by a system, to different possible future situations (modeled by modified systems) and assess if and how some of these futures can lead to improvements regarding environmental impacts. The time scope is thus an important information, it should be specified, and several time steps can be examined.
This also leads to consider that the system under study can contains several products and several functional units that can vary in their nature and their amounts according to the investigated transition solutions. Describing these functional units and their evolution is thus essential. One example among transition currents of thoughts, is the “low tech” (Bihouix, 2020) that considers that functionalities of products can decrease in order to focus on “essential functions.” Another opposite example, issued from circular economy, would be to consider increasing durability of some products, that is an increase in some functional performances, in order to extend their service life.
Another important aspect concerns reference flows. In P-LCAs, the reference flow corresponds to the amount of product necessary to fulfill one unitary functional unit. In Tr-LCA, several reference flows can be defined corresponding to the total amounts produced, stored or consumed in the geographical area under study.
Defining transition scenarios
Transition scenarios are the chore of Tr-LCA. As already mentioned, LCA in general is an iterative approach in which interpretation can lead to revisiting objectives. This is the same for scenarios. As will be described later in this approach, results can reveal possible and unforeseen importance of elements in the background system, and therefore possibly new actors. Indeed, as scenarios are mainly a matter of involved actors, inclusion of new actors can be the result of one LCA iteration, that will lead to consider new or more detailed scenarios. This section is thus providing a guide rather than a strict procedure.
Source and target territories
As mentioned earlier, transition is very much about geographical perimeter because local conditions can have an important influence on environmental performances of transition solutions. However, one must distinguish the geographical area inside which transition solutions are implemented from the geographical area inside which these solutions may induce changes. Let's call them source and target territories respectively.
The source territory is submitted to possible local constraints such as limited locally available resources, the target territory includes the source territory but it can be wider. If the transition solution implies the production of a new or specific product, one must consider the match of flows between the amount of resource necessary for the product issued from transition scenarios (that controls the number of products that can be provided), and the amount necessary to respond the market or population demand. The choice of the size of target geographical perimeter can vary according to the transition solutions under study. If source territories are often small in many transition perspective (with the idea of encouraging localized and short circuits economies for example), they would have to be enlarged if some constraint volumes of new products are larger than the market volume of the source territory. This means that the target geographical perimeter should be defined as the area allowing match between amounts of products out of investigated solutions and size of target markets. The rule based on value to weight ratio used in C-LCA (Weidema, 2003) is rather well adapted to define the size of the target territory at first glance, the value to weight ratio determines the geographical market (local market for low ratio, continental for medium and global for high). It should however be more precisely defined using existing market data on the existing situation to be substituted compared to the maximum production capacities of the investigated transition solutions.
Involved and concerned actors
As transition is generally a multi-actor question, it should be as much as possible, important to distinguish each actor's responsibility in a transition solution. Specifying involved actors, and their action perimeter is thus a necessity. These actors need to be interviewed to understand their choices, and their constraints that can be of economic nature but also of regulatory or social natures. Investigating actor's decision context is a key issue in order to define socioeconomics mechanisms underlying destinations of flows.
The involved actors can be defined as the source territory actors taking an active part in the transition solutions to be investigated. This means that they can take key decisions concerning processes and activities of the transition solutions. This relies to the concept of “foreground system” in LCA defined as the collection of “processes, which are under the control of the decision-maker for which an LCA is carried out” (Frischknecht, 1998). Several actors can cooperate in the foreground system, and their respective action perimeters must thus be clearly defined in the foreground system model as shown in Figure 1. This figure shows a simplified representation: it is possible that action perimeters are not entire processes but could be modeling parameters disseminated in several activities in the foreground process. This is the case of actors that can have a structural role in the transition solution, and not a productive role. As an example, local authorities can put incentives or constraints (such as public grants, taxes, regulation thresholds, etc) that may affect, limit or favor several production activities or locally influence economic equilibrium. This will be later detailed in the system model (Section System model).
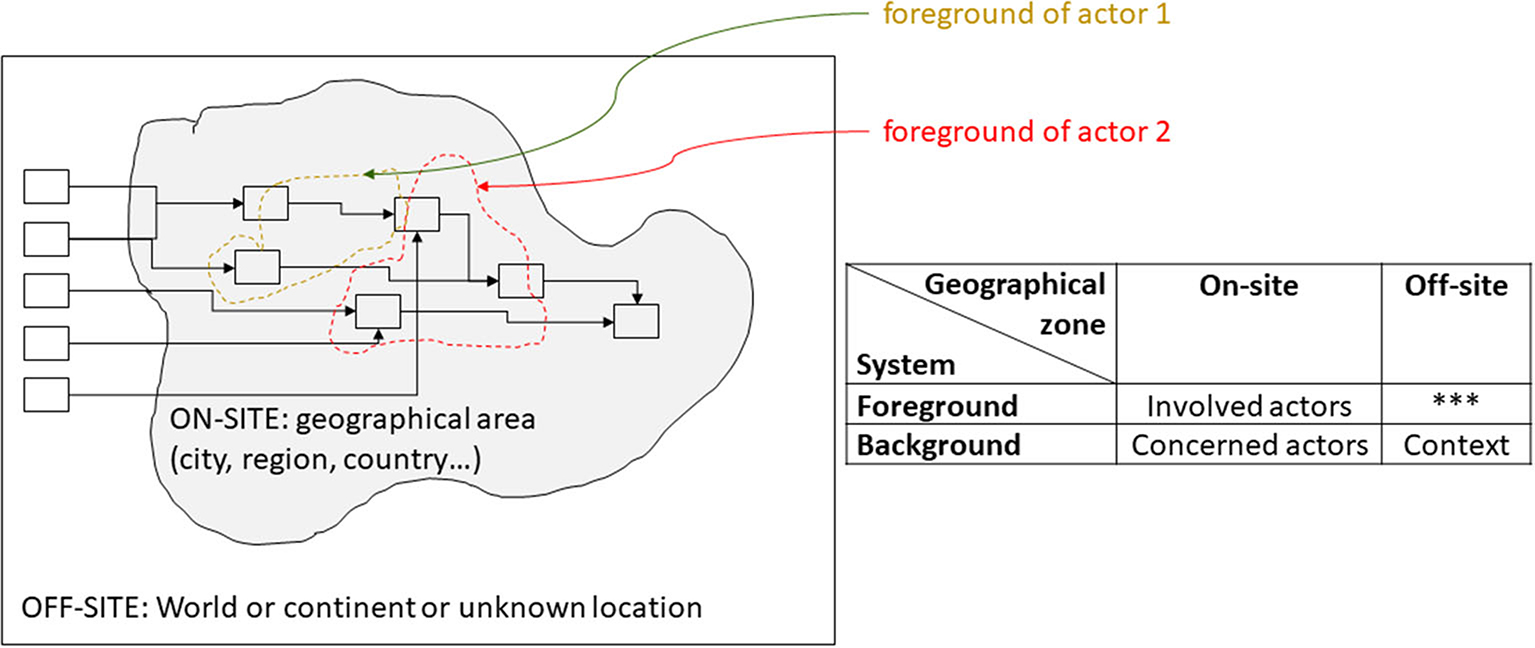
Figure 1. Conceptual representation of foreground and background systems in the source geographical area.
It is possible that during the Tr-LCA processes, preliminary results show that some activities in the background system are found very influent on the results. If these activities concern actors of the source territory they can be defined as concerned actors, and some efforts should be made to involve them in the study in a subsequent iteration.
The territorial MFA system model (see more details in the next Section System model) is useful to identify the set of both concerned and involved actors as defined in Figure 1: each node where an input flow is separated in several output flows is potentially a concerned actor that will make choices in terms of flows' possible destinations that will affect other actors.
Describing transition solutions and data collection
Generally, prospective science is dedicated to defining scenarios, that are “a description of how the future may unfold according to an explicit, coherent and internally consistent set of assumptions about key relationships and driving forces” (GFAR, 2014). Defining scenarios requires “a systematic, participatory and multi-disciplinary approach to explore mid- to long-term futures and drivers of change” (GFAR, 2014). For transition solutions, the use of Participatory Prospective Approach (PPA) should be recommended (Bourgeois et al., 2017; Ianniello et al., 2019), where local scale and bottom-up approaches predominate, scenarios are combinations of trends and alternatives and co-imagined by the “doers” (Bourgeois et al., 2017) defined as the actors who will produce or be affected by the possible futures (Bourgeois et al., 2017).
The involved actors should be able, with the help of the LCA modeler, to describe all possible choices in terms of organization, and resulting processes and activities concerning the transition solutions they want to implement. Similarly to other LCAs, classical data collection can then be conducted using specific data and specific process models when available, completed with literature based information and generic LCA databases. The existing rules applied to inventory data collection in P-LCA concerning representativeness, robustness, completeness, etc can be applied to Tr-LCA and will thus not be detailed here.
Although not required, it is very interesting to integrate flow models inside processes of the transition solutions using the systemic nature of LCA (Bertalanffy, 1968) which allows different levels of organization to be interwoven (Onat et al., 2017). Instead of modeling black box unit processes with values of input and output flows proportional to the reference flow, detailed models can be used related to other parameters and especially parameters related to actors' decision and local specificities. The main interest in that approach is to get models closer to physical, chemical, or economic phenomenon that can intrinsically be nonlinear (Pizzol et al., 2021). This has been conducted on various applications with technological processes such as microalgae cultivation (Duran Quintero et al., 2021), cement concrete design (Ventura et al., 2021), or hemp based thermal insulator (Andrianandraina et al., 2015; Senga Kiessé et al., 2017).
In addition, the process models could also link the quantities and natures of input components as well as operational settings to the properties of the output products, including their functional properties.
Local specificities can determine both constraints affecting the flows, and parameters affecting processes involved in the transition solutions.
As described for Te-LCA (Loiseau et al., 2018), the source territory can be “understood as a geographical system defining opportunities and constraints for organization and development of the human system.” It will determine available local land, mineral, fossil and biotic natural resource, as well as available anthropic resource such as local productions of goods, services and waste. The amounts of reference flows produced by transition solutions can be designed according to the prioritization between two main aspects (offer or demand) and to the consideration of possible limiting factors.
• Prioritizing the offer consists in producing as much as possible from the transition solution, even if it means expanding the target territory beyond the borders of the source territory. With that priority, the constraint is determined by the local available resource on which the transition solution is based. This approach should consider the smallest amount of available resource if several local resources are needed. A typical example is waste recovery, where the objective would be to recover the totality of one waste generated in the source territory and to export the obtained secondary products if the demand of the source territory cannot absorb the production. If waste recovery implies the combination of different types of wastes, then one of these waste flows will be the limiting factor, and one possible scenario would be to import the required missing amount.
• Prioritizing the demand consists in adjusting production according to the demand of the source territory. With that priority, the constraint is determined by the market demand of the source territory. A typical example is active mobility, where the objective is to offer new mobility services according to the population inside the source territory. The case study conducted for Te-LCA (A) (Loiseau et al., 2018) on a collective biogas plant can be included in that category, as the functionalities (either treating organic waste or producing energy) and the resulting sizing characteristics of the technology are driven by the analysis of needs of the target territory.
Apart from offer and demand, some other constraints can exist that may impose constraints on production. Regulation (and possible changes in regulation) can impose maximum annual production thresholds, but also available existing technologies, labor regulations, available infrastructures (for energy, wastewater treatment, transports, etc) can impose minimum or maximum production capacities.
Local specificities are not constraints but local parameters that will affect the processes involved in the transition solutions. They can be issued from standards requiring specific performances of products, from regulation imposing minimum requirements regarding processes, or from contextual characteristics that will influence either the processes themselves (meteorology, traffic, soil, size of population…) or the substitution mechanisms (market prices of competing products, socioeconomic or cultural profiles of the local population, local policies or economic incentives, etc).
Defining the existing reference situation
The existing situation is the model of all activities and processes in the target territory before they are affected by the transition solutions. Although the reference situation to be substituted is always in the mind of actors involved into transition solutions, their own vision can be limited and not account for indirect effects if they are not familiar with system approaches, and more generally it is also possible that not predictable effects can occur.
Concerning waste, actors are generally well aware that waste recovery is a substitution to elimination (either landfill or waste incineration) and that it can save natural resource, but they are not always precisely aware of the competing products that the secondary product could substitute and of which exact natural resource this could save. In fact, during research and developments phases of waste recovery solutions, the future performances of the secondary product are not known and thus uncertainties about the substituted products are important. In that case, several scenarios of the existing reference situation should be investigated.
In other cases, like transition solutions based on shared economy, indirect effects can be difficult to assess because transition solutions can have rebound effects and change other economic sectors that have not been initially identified. In that case, the existing situation may have to be reassessed in the light of this new knowledge.
System model
Territorial Material Flow Analysis (MFA) appears the suitable frame to define the transition system models for the following reasons:
• Territorial MFA considers all flows transiting in and out of a given territory, with their total value, similarly to Te-LCAs (B);
• MFA can be deployed at different geographical scales, from very local to worldwide according to the needs of the study;
• MFA is intrinsically a multi-products, i.e. no unique nor unitary functional unit is required and the system is considered as a whole.
• MFA allows considering resource thresholds in a given geographical area, i.e., the upper limits of a constraint resource either due to local market demands, to resource availability in the considered geographical are, and/or production capacities.
Modeling substitution
Modeling substitution is the key issue of Tr-LCA. As detailed in Table 2, Tr-LCA should describe expected changes in response to possible decisions or to changes generated by transition solutions into a dynamic background system. Thus, from the system corresponding to the initial situation to be substituted, the system boundaries will be expanded according to all possible changes generated by the transition solutions. These changes may be based on economic models like C-LCA, but not only. They can also be related to changes in social behaviors, regulations, and submitted to constraints as detailed in Section Defining transition scenarios (technical, physical…).
Some changes can be modeled whereas others cannot because they are not predictable, and can thus be investigated as scenarios as detailed in Section Involved and concerned actors.
Apart from physical changes that can always be modeled from MFA (see Activities affected by physical laws), other models are not always possible nor necessary. Various market shares can simply be tested using sensitivity analysis, and considering possible constraints (production capacities, geographical availability of resources). They allow providing minimum market share ensuring environmental improvements compared to the reference situation. Some aspects can however be deepened and this section aims at discussing some possible factors influencing the substitution. They are certainly not exhaustive, and should Tr-LCA be applied to different case studies in the future, new substitution models will certainly be developed.
Activities affected by physical laws
Some important indirect effects of changes that are not accounted in P-LCAs, due both the system boundary rules and product scale. These are changes related to physical relationships between co-products issued from the activity and changes due total volumes of activities. Using the MFA method as a system model is perfectly adapted because it considers the physical links between output co-products issued from the same input flow as well as physical consequences of differences between production and consumption.
Changes in multi-outputs activities (with co-products)
A theoretical example is provided in Figure 2. If two coproducts B and B' are issued from the same input flow, the fact that the transition scenario requires an increase of B, will have an effect on B'. This means that possibly, every change on the output of a multi-products process should imply a system expansion.
If B is the main product (the driver of economic benefits), then its increase will provoke an increase of B' as shown in Figure 2. In fact, this relationship between B and B' may actually be negligible when the generated increase on B' is found very small compared to the market demand for B' and equivalent competing products: in that case the side effects of the increase of B on B' can be neglected.
If B' is the main product, then the production of B is constraint by the production of B', and its increase might not be possible or limited. In the same manner, if B >> B', even a drastic change on market demand for B', will not change very much the capacity to produce B, that is not effectively constraint.
Changes in market volume activities
For downstream activities, MFA enables to check the adequation between flows from productions and market needs as shown in Figure 3. This requires that functions and properties of both initial products (A and others) and future product B must be known, and well described, so the market is identified. If they are not known, i.e., for low TRL innovations, they can be defined as plausible or desirable objectives and then tested with a sensitivity analysis to find out the limits of the functions and properties to ensure environmental performances compared to the initial situation.
• If the new offer (product B in Figure 3) is very inferior to market demand in the source geographical area, then prioritizing the offer (see Describing transition solutions and data collection) is a possible scenario and source and target territories can be the same.
• If the new offer (product B in Figure 3) is comparable or superior to market demand in the source geographical area, it may generate stocks as shown in case 2 (Figure 3). In that case, if prioritizing the offer is the chosen approach (see Describing transition solutions and data collection) exportation scenarios should be included, i.e., enlargement of the target geographical area with the corresponding distribution scenarios. If prioritizing the demand is the chosen approach (see Describing transition solutions and data collection), a maximum production threshold is a constraint applied to the production of B.
Activities affected by economic laws
Economic models can be used to calculate market shares that would be obtained by products from the transition solutions. The current existing models are those used in C-LCA but some important assumptions previously detailed (see Which system model for transition LCAs?) are necessary.
If these rules do not apply to transition solutions, then several investigations can be conducted to examine economic plausibility of the substitution and/or determine favorable economic conditions for a successful substitution. An investigation about economic competitivity of the transition solutions can be conducted in order to compare to the initial situation, by comparing production costs of the transition solutions to average market prices.
Similarly to detailed process models as a second level of systems organization (see Describing transition solutions and data collection), the use of a detailed production cost model, detailing various types of operational costs (at least labor, input composing materials and energy, possibly maintenance and other consumables) as well as investment costs can be very useful. Indeed, this can help finding more or less favorable configuration of production facilities and organization.
If production costs are not known, i.e., for low TRL innovations, future production costs and/or market shares can be defined as plausible or desirable objectives and then tested with a sensitivity analysis to find out the market share and cost limits ensuring competitivity (Langhorst et al., 2022).
In a B to B market, price competitivity appears as the main driver, or at least a first plausible basis for a substitution (Zimmerman et al., 2022). However, some existing models show the real substitution can depend on many other factors such as regulation, subjective value given to secondary products, etc. Some existing agent-based models, based on individual value scales of different socio-economic actors can be useful to identify favorable factors to change.
Activities affected by social or behavioral changes
Substitution modeling can be far more complex using various preferences criteria such as environmental or social awareness, that can be important especially in a B to C markets.
The assessment of plausible market shares can be investigated by surveys among consumers' population and possibly modeled using agent-based modeling. This is currently applied to passengers' flows to test changes of behavior affected by new transport solutions (Le Pira et al., 2015).
Establishing simulation plans
According to the previous steps, transition solutions have been modeled within the frame of complex model systems theory (Bertalanffy, 1968), with possibly different levels of organization, numerous parameters, possible retroactive loops, and possible discontinuous effects due to conditional relationships between parameters as well as constraints. Furthermore, this model provides several answers, i.e., different environmental indicators as well as other possible information such as functional changes or production costs. In order to determine most favorable transition solutions, it is important to develop systematic methods to help further interpretation highlighting the roles, possibilities of actions and their environmental consequences for the involved actors. These steps first require to classify variables and then to conduct simulations according to this classification.
Classification of model's variables
During their co-elaboration of transition solutions, involved actors can chose different sets of scenarios represented by a set of discrete possibilities such as locations, type of technologies, maintenance strategies, labor organization, etc. Involved actors can also control some continuous variables (especially if detailed processes, costs or substitution models are used) such as process operational conditions, temperature setpoints, various settings, mix design etc. All these variables are classified as decision variables, because they are within the decision-making perimeter of at least one involved actor.
Some other discrete choices or continuous variables are not within the decision perimeters of involved actors, but may be influent on the results, they are defined as contextual variables, such as local electric mix, regulation thresholds, ambient temperature, market prices, etc.
Finally, some choices can concern modeling options, such as the choice of indicators, reference values, simulation time steps, partition coefficients and they are classified as epistemological variables.
Probability density profile should be collected for all contextual and epistemological continuous variables. Decision continuous variables representing a choice inside a given interval, are always considered with a uniform probability density profile.
The type of simulation according to the type of variable is provided in Table 3 and detailed in the next sections.
Implementing scenarios, conditions and constraints
This section deals with alternative discrete choices (see in Table 3) that can be made by involved actors, imposed by the context or chosen by the modeler.
Whatever the choice, it induces a condition on the model of the type: if such a choice is made, then such a process occurs or does not occur. The fact that a process is activated by a scenario choice results in a non-zero reference flow of this process, and whose value can be known. Furthermore, a discrete choice can also activate incompatibilities between processes, as well as thresholds on some process flows or variables. This can be represented using a table resuming processes (and thus reference flows), variables and constraints with their characteristics (values, unit, thresholds…).
If discrete choices only involve occurrence or non-occurrence of processes, every scenario can be defined as one single and unique combination of all discrete possibilities. It is possible to systematically build the set of all possible scenarios and to test each of them using a suitable simulation plan as schemed in Figure 4.
If discrete choices also imply thresholds or incompatibilities, some scenarios can be eliminated as schemed in Figure 4.
This type of systematic scenario generation and analysis has already been conducted for the case study of nickel slag recovery in New Caledonia (Quéheille et al., 2021).
Sensitivity analysis simulations
In Tr-LCA, sensitivity analysis is used to identify among continuous variables, action levers available to the different actors involved. It requires combining two different sensitivity analysis methods.
The first method, based on Morris indexes (Morris, 1991) aims at ranking influences of variables, and detecting possible interactions between variables, as well as the mathematical shape of their influence (either monotonic increase, monotonic decrease or non-monotonic). If the influence is monotonic, the Morris indexes can also provide the direction of the influence: increasing or decreasing for continuous variables. This is an important information because, with an increasing direction, one needs to decrease the value of the variable in order to decrease indicator, whereas with a decreasing direction, one needs to increase the value of the variable in order to decrease indicator. This provides preferable values of influential variables in order to decrease environmental impacts. If the influence is non-monotonic, this mean some optimal values of variables can exist. They should be further investigated concerning the shape of the relationship in order to determine these possible optimal values. The Morris indexes can also detect non-influential variables.
The Morris indexes should first be calculated on the model that is run on all discrete and continuous variables (contextual, decision and epistemological), especially to identify if non-decision variables are found influential. Then, it can be run a second time, exclusively on decision variables (both discrete and continuous) to determine and rank possible action levers, all the other variables being set to their most probable or default value.
An additional global sensitivity analysis (Sobol, 2001) can be run to quantify influences of continuous variables found influential from the previous steps (all other variables being set to their most probable value) and quantify combined influences of two or more variables (i.e., 2nd or higher Sobol indexes).
These approaches have been fully described in several articles, although names of categories for variables have been changed in the present paper to give them a more general meaning. Contextual variables can also have been named environmental variables previously (Ventura et al., 2021), whereas decision variables have been named technological parameters in previous work (Senga Kiessé et al., 2017; Senga Kiesse et al., 2020; Duran Quintero et al., 2021).
Interpretation
Changes in functionalities
Because Tr-LCA allows for a shift in functionalities between the initial situation and the transition solutions, it is important to incorporate these changes in functionalities as part of the results.
Identification of action levers and their significance
Sensitivity analysis conducted as detailed the above Section Implementing scenarios, conditions and constraints, provides information concerning favorable values of influential continuous decision variables, these are called action levers.
In order to assess significance of actions levers, Monte Carlo simulations should be run using most favorable values for action levers obtained from the previous Section Identification of action levers and their significance and to propagate uncertainties from non-influential and non-controllable variables and determine confidence intervals.
If significance is confirmed, then the list of action levers can be provided as shown in Table 4. In this example, one can read that actor n°1 has one action lever on indicator 1 that is variable vi of which favorable value is mfvi, 1, 1 and two action levers on indicator n that are variables vi and vk of which favorable values are mfvi, 1, p and mfvk, n, p respectively. Actor n° p has one action lever on indicator 1 that is variable vi of which favorable value is mfvi, n, 1 and one action lever on indicator n that is variable vk of which favorable value is mfvk, n, p. One can also see that variable vi is a decision lever for two indicators 1 and n, and that variable vk is controlled by two different actors 1 and p.
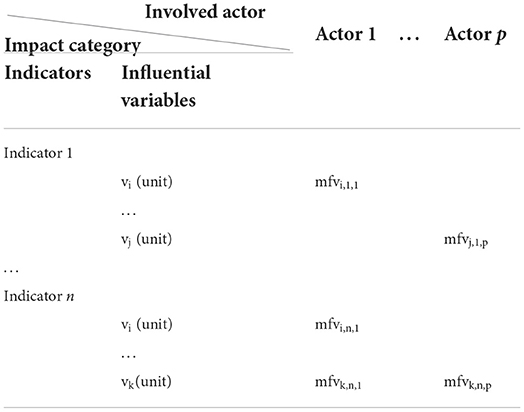
Table 4. Example of results obtained from global sensitivity analysis (mfvk, n, p: most favorable value of variable k for indicator n and actor p).
From Table 4, several cross-analyses are needed to find solutions that decrease all the indicators. Indeed, the variables vi and vk each have two recommendations of favorable values to decrease the indicators. If the recommended values are consistent with each other, they are identical (i.e. if mfvi, 1, 1 = mfvi, n, 1 and mfvk, n, 1 = mfvk, n, p) then there is no conflict, vi and vk each have a single favorable value and using their favorable values generates a synergistic effect: it decreases two indicators and both actors have a converging interest in using the same values.
However, if we find that the variable vi can take two different preferable values (mfvi, 1, 1 ≠ mfvi, n, 1) then there is a conflict between two indicators. This leads to the choice of either setting a priority between indicator 1 and indicator n, or setting vi = mfvi, 1, 1 to improve indicator 1, while setting vk = mfvi, n, 1 to offset the negative influence of vi on indicator n.
Finally, if it is found that the variable vk can take two different preferable values (mfvk,n,1 ≠ mfvk, n, p) then there is a conflict between two actors. This leads to negotiations between these two actors. For example, if mfvk, n, p is chosen for vk, by actor p, actor 1 has the option to compensate by using vi = mfvi, n, 1 to decrease indicator n. This is just one example of a possible outcome to the conflict, and other aspects could intervene in the negotiation, such as the costs generated by the choice of a favorable value by one of the actors.
This type of analysis has not been implemented in existing routines, but provided that numerical tools can identify each variable by a variation interval and a list of controlling actors, it should be possible to easily identify conflicts and further investigation should be necessary to develop routines providing possible resolutions.
Identification of preferable scenarios
Indicators results can be calculated for each single scenario using most favorable values obtained from the previous Section Identification of action levers and their significance. For each indicator, results can be compared to a target, either the initial system or a future target.
Targets should be defined for environmental impact indicators values only, not for other operational indicators. Choosing the indicators resulting from the existing situation as a target enables to assess that some scenarios can provide environmental improvements compared to the initial reference situation. Choosing a future target can also be used when for example political targets are set. This is the case for climate change for which political targets are given for different time frames.
In a first step, all indicators showing no improvement compared to the target should be set aside. Then among the ensemble of remaining indicators and for each of them, scenarios providing results below the target for each indicator can be selected, thus making lists of preferable scenarios for each indicator. These lists are then compared between all indicators in a second step. Ideally, only scenarios improving all indicators are finally selected as shown in Figure 5. If this ensemble of favorable scenarios is empty, i.e., it does not exist on all indicators, then then the indicator for which the smallest number of favorable scenarios and/or those with small improvements over the reference situation as proposed in the literature for P-LCAs (Guérin-Schneider et al., 2018) can be eliminated and the analysis is then repeated.
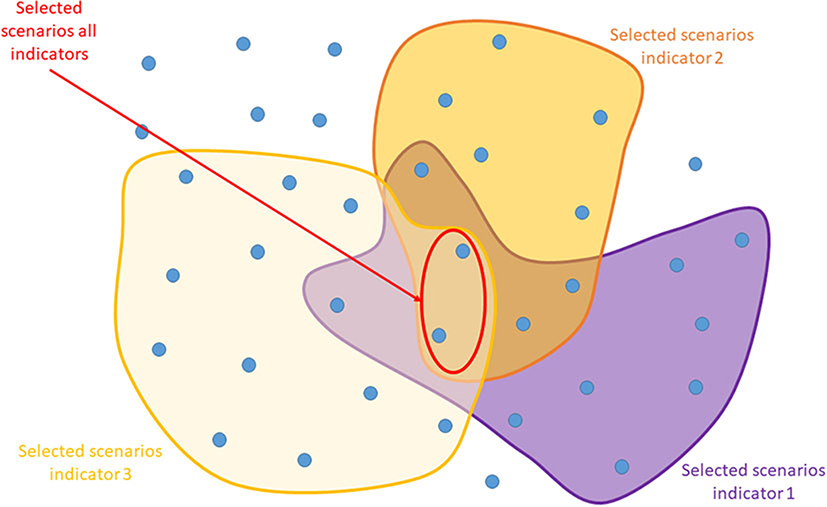
Figure 5. Systematic representation of selection of favorable scenarios: each blue dot represents a scenario; one ensemble represents scenarios complying with the target for one indicator.
Finally, one gets the following results: indicators showing no possible improvements, indicators with very few scenarios with possible improvements, and indicators with common scenarios leading to improvements. At this stage only, priorities between indicators can be discussed among the involved actors, considering if the worsening is due to foreground and/or local background. In the case of worsening due to local background, involvement of background local actors should be considered in order to improve scenarios according to their knowledge. In the case of worsening due to general off-site background scenarios, these are reported to be strong sources of uncertainty, requiring either an additional effort to make them more reliable where possible, or a greater vigilance on the part of the involved actors who will potentially have to reassess and readjust their actions to adapt to a changing context.
Illustrative examples
Three different examples are described in Table 5. They are not exhaustive and no results are presented as no Tr-LCA has been conducted yet. These examples mainly show how Tr-LCA could be conducted. They concern three very different types of transition solutions:
• Example 1 concerns a technological solution that would be largely deployed at a regional scale: the recycling of concrete waste from building's demolition with CO2 capture and possible use of fatal heat from local plants to produce secondary sand used in new concrete mixes;
• Example 2 concerns the large implementation of a low-tech building material a regional scale: earthen walls using soil excavated from public works;
• Example 3 concerns a local policy favoring active mobility: the introduction of shared electric scooters in a city.
Based on the methodological steps described above, we can define the different elements that will allow us to define the objectives, the system, the questions to be asked, and the possible models to be mobilized. Table 5 provides an information that would be suitable to start a first iteration of a Tr-LCA, however, according to first results, new actors, new scenarios and more detailed substitution mechanisms could be developed during the on-going studying process.
Discussion
Transition LCA differs from all other types of LCAs concerning goals, functional unit, reference flow, and system boundaries, leading to important differences in the modeling and the interpretation phases as described all along Section Transition LCA: A new frame for environmental assessment of transition scenarios.
A critical point about transition concerns mass effects. With P-LCAs focused on products, effects of massive production on economic markets cannot be accounted. For example, if a new product issued from a secondary resource is massively produced (because a waste is abundant in the area under study) compared to the size of the same local market demand, the environmental benefits are certainly compromised (Ventura and Antheaume, 2019). Especially in the case of a constraint products such as products obtained from secondary resources, their production capacity is limited by the production of the main product, and this limit is not considered with C-LCA system model. With Tr-LCA using total values (i.e., total amounts of products) and not with relative ones (amount of waste relatively to one unit of main product's amount), it is possible to consider the adequacy between production and consumption, and thus the possible market share that can be taken by the product obtained from secondary resources. In the case of a mismatch between production and consumption, product obtained from secondary resources could have to be stocked, as well as possible substituted products that would themselves be by-products of joint production and thus submitted to constraint production. In a long term, the consequential method recommends to expand again the system to other markets that could absorb the surplus [previously described as the “endless regression problem in system expansion” (Weidema, 2001)], and this expansion can be conducted in a systematic manner using IO models (Suh et al., 2010). However, underlying economic assumptions of these approaches previously described in this article are still not valid for studying systems in transition. In transition LCA, stocks of products obtained from secondary resources have thus to be considered. The implementation of a new multi-output process that is studied with Tr-LCA, can lead to obtain P-LCAs of each output product. Indeed, considering a given allocation rule, it is possible to downscale the results from Tr-LCA system to individual products, but it is very likely that the results obtained will be different from those that would be obtained with classical P-LCA. And this, even if using the same allocation rules because Tr-LCA accounts for changes between two systems (initial and modified), it does not aim at attributing the possible benefits to one or more products that are involved in the transition solutions. This attribution is political (Chen et al., 2010) and can be treated as such by the involved actors and be part of their negotiations for possible attribution of economic benefits linked to the environmental benefits of their cooperation in the transition solution. An example is the implementation of a carbon capture and use technology as a transition solution. Whereas one actor provides CO2 another uses it, and P-LCA always has to deal with the allocation of environmental benefits due to the reduction of CO2 as well as with economic benefits linked with CO2 market. Tr-LCA will provide the total benefits for the whole system, and involved actors have information on both the conditions and action levers to succeed. They can use that information to find suitable business models ensuring that conditions of environmental benefits are possible and reached.
In C-LCA, by-products are considered as low value constraints products. However, strong incentives for climate change or circular economy can lead to higher profitability obtained from by-products and can lead to inversions between current (or initial) ranking between main and by-products. One recent example concerns wood pellets, initially made out of sawdust waste, and now being directly produced from forests to be used as biofuels for power plants (Mouterde, 2021). Indeed, political authorities can provoke rupture effects toward important technological and economic shifts via regulations and public subventions. As a consequence of the recent European green deal (EU, 2019) launched to reach carbon neutrality in 2050, CO2, being currently considered as an undesired emission similarly to a waste, could become a profitable resource in a dozen of years. One could say that the notion of waste, main and co-products are evolving and that it is even desirable that waste of the past become a resource for the future in a transition perspective. However, the inversion between waste and main product also has environmental consequences on the LCA of each of the products by distributing the environmental burdens differently. From this point of view, it seems preferable to consider the whole extended system as a whole in order to evaluate the environmental impacts of the new solution, without having to subjectively define to which product (main or secondary) the environmental credits should be attributed.
This article does not address the question of indicators. Tr-LCA can use all types of existing indicators, and it is obviously also interesting to be able to use regionalized indicators if the objectives and the available data are suitable. In the same way, the interpretation phase can be enlarged to other indicators than environmental impacts, such as costs, or social indicators. The approach would be the same, requiring additional models and increasing the number of indicators. Tr-LCA is thus compatible with Life Cycle Sustainability Assessment in general. In addition to spatial differentiation, another research perspective would be to distinguish the actors impacted by impact categories related to human health such as workers or residents of the considered geographical area, because they could become considered, and possibly involved, actors in a further iteration.
Prospective LCA is generally used to forecast environmental impacts of an innovation at a low Technological Readiness Level (TRL). In that perspective, prospective LCA can in particular integrate evolutions of background processes such as energy production, according to existing scenarios (Beltran et al., 2020). For Tr-LCA, this can also be used if the time perspective is sufficiently long. The possible evolutions of the background processes can be extracted from existing global scenarios. These choices are classified as discrete contextual variables because they are beyond the decision-making perimeter of all considered actors.
The question of the applicability of transition LCA is also important. Although the databases and process models are the same as other LCAs, the coupling with MFA, the possibility of having several functional units in the same system, but especially the different steps of interpretation, require today the use of either several different numerical tools or dedicated programming. The studies or elements of studies already carried out and quoted in this article, were for the most part conducted with Matlab® or in Python language by developing modules coupled with the Brightway software. In the near future, these different modules should be released as open source, but they do not constitute interfaces that can be used by non-experts. The new functionalities required, such as automated scenario generation, sensitivity analysis, or interpretation of results by actor, remain functionalities to be developed and integrated into existing digital tools.
Conclusions
In this article, a new type of LCA, called transition LCA (Tr-LCA) is conceptually described. It aims at studying transition scenarios in a geographical context, and that can be relied to various technological paths. One of the main differences with existing LCAs concern the functional unit: several functional units can be included and they can vary. Additionally, the results of Tr-LCAs concern the changes of the system itself rather than the individual impacts of products. This approach is consistent with the concept of transition that involve new relationships and interdependencies between various actors, where the aim is the global interest rather than individual ones. Tr-LCA is also related to a specific geographical context in order to account for existing limits of resources and especially constraint resources such as waste, and to account for the match of flows between production and demand which determines the size of the geographical area. This type of modeling can be reached by coupling LCA with MFA at the suitable geographical scale.
Tr-LCA also requires specific interpretation steps dedicated to the identification of preferable scenarios and action levers hold by each involved actor. This interpretation requires integrating actors' identification and their decision models as mechanisms inside the system model. It also requires additional methods to generate all actions possibilities and to conduct suitable sensitivity analysis.
This method has presently been applied by parts but not as a whole and not as a single numerical tool. These parts are currently being developed in a single computing Python language, in order to become compatible with Brightway 2. The conceptual frame is also currently applied on a case study concerning recovery of nickel slags in New Caledonia (Carboval research project) that will be the topic for future publications.
Data availability statement
The original contributions presented in the study are included in the article/supplementary material, further inquiries can be directed to the corresponding author.
Author contributions
AV designed, developed the new method, and wrote the whole article.
Funding
The present methodological frame was developed in the context of the Carboval Research Project funded by the French Environmental Agency (ADEME), involving different academic partners (LGC, BRGM, LMDC, LEMNA and ESO), a private partners (CNRT), and Energy Agency of New Caledonia (ACN).
Acknowledgments
The author also wishes to thanks two important networks for exchanging ideas and stimulating research: AgorACV the French regional Western network on LCA, and EcoSD the French National network on eco-design of sustainable systems.
Conflict of interest
The author declares that the research was conducted in the absence of any commercial or financial relationships that could be construed as a potential conflict of interest.
Publisher's note
All claims expressed in this article are solely those of the authors and do not necessarily represent those of their affiliated organizations, or those of the publisher, the editors and the reviewers. Any product that may be evaluated in this article, or claim that may be made by its manufacturer, is not guaranteed or endorsed by the publisher.
References
Andrianandraina Ventura, A., Senga Kiessé, T, Cazacliu, B., Idir, R., and van der Werf, H. M. G. (2015). Sensitivity analysis of environmental process modeling in a life cycle context: a case study of hemp crop production: environmental process modeling in a life cycle context. J. Ind. Ecol. 19, 978–993. doi: 10.1111/jiec.12228
Beltran, A. M., Cox, B., Mutel, C., van Vuuren, D. P., Vivanco, D. F., Deetman, S., et al. (2020). When the Background Matters: Using Scenarios from Integrated Assessment Models in Prospective Life Cycle Assessment. J. Ind. Ecol. 24, 64–79. doi: 10.1111/jiec.12825
Bertalanffy, L. (1968). General System Theory: Foundations, Development, Applications. Ed. by Georges Braziller. revised edition 1976. New York: G. Braziller.
Bihouix, P. (2020). The Age of Low Tech: Towards a Technologically Sustainable Civilization. Trans. by Chris McMahon. Seuil. Bristol (UK): Bristol University Press, October 21. Available online at: https://books.google.fr/books?hl=frandlr=andid=Vfr_DwAAQBAJandoi=fndandpg=PP1anddq=bihouix+low+techandots=PV9zfkefkyandsig=vbaaf8GNgfYljAjsdlPknx62A1I#v=onepageandq=bihouix%20low%20techandf=false (accessed February 10, 2022).
Bourgeois, R., Penunia, E., Bisht, S., and Boruk, D. (2017). Foresight for all. Co-elaborative scenario building and empowerment. Technol. Forecast. Soc. Change 124, 178–188. doi: 10.1016/j.techfore.2017.04.018
Ceschin, F., and Gaziulusoy, I. (2016). Evolution of design for sustainability: from product design to design for system innovations and transitions. Design Stud. 47, 118–163. doi: 10.1016/j.destud.2016.09.002
Chauhan, R. K., and Chauhan, K. (2019). Building automation system for grid-connected home to optimize energy consumption and electricity bill. J. Build. Eng. 21, 409–420. doi: 10.1016/j.jobe.2018.10.032
Chen, C., Habert, G., Bouzidi, Y., Jullien, A., and Ventura, A. (2010). LCA allocation procedure used as an incitative method for waste recycling: An application to mineral additions in concrete. Resour. Conserv. Recycl. 54, 1231–1240. doi: 10.1016/j.resconrec.2010.04.001
Chen, W., Liu, Q., Zhang, C., Mi, Z., Zhu, D., and Liu, G. (2020). Characterizing the stocks, flows, and carbon impact of dockless sharing bikes in China. Resour. Conserv. Recycl. 162, 105038. doi: 10.1016/j.resconrec.2020.105038
de Bortoli, A. (2021). Environmental performance of shared micromobility and personal alternatives using integrated modal LCA. Transp. Res. D Transp. Environ. 93, 102743. doi: 10.1016/j.trd.2021.102743
Duran Quintero, C., Ventura, A., Lépine, O., and Pruvost, J. (2021). Eco-design of spirulina solar cultivation: Key aspects to reduce environmental impacts using Life Cycle Assessment. J. Clean. Prod. 299, 126741. doi: 10.1016/j.jclepro.2021.126741
Ekvall, T., Azapagic, A., Finnveden, G., Rydberg, T., Weidema, B. P., and Zamagni, A. (2016). Attributional and consequential LCA in the ILCD handbook. Int. J. Life Cycle Assess. 21, 293–296. doi: 10.1007/s11367-015-1026-0
EU (2019). EU climate action and the European Green Deal. Text. Climate Action - European Commission. Available online at: https://ec.europa.eu/clima/policies/eu-climate-action_en (accessed April 20, 2021).
Filippova, R., and Buchoud, N. (2020). A Handbook on Sustainable Urban Mobility and Spatial Planning: Promoting Active Mobility. Available online at: https://trid.trb.org/view/1746731 (accessed February 10, 2022).
Frischknecht, R. (1998). Life Cycle Inventory Analysis for Decision-Making: Scope-Dependent Inventory System Models and Context-Specific Joint Product Allocation. SwissFederal Institute of Technology Zurich, March. Available online at: http://link.springer.com/10.1007/BF02978487 (accessed June 13, 2016).
Geels, F.W. (2011). The multi-level perspective on sustainability transitions: responses to seven criticisms. Environ. Innov. Soc. Trans. 1, 24–40. doi: 10.1016/j.eist.2011.02.002
GFAR (2014). A Glossary of Terms Commonly Used in Future Studies. Global Forum on Agricultural Research and Forward Thinking Platform. Available online at: https://www.fao.org/docs/eims/upload/315951/glossary%20of%20terms.pdf (accessed October 7, 2020).
Goldstein, B., Birkved, M., Quitzau, M. B., and Hauschild, M. (2013). Quantification of urban metabolism through coupling with the life cycle assessment framework: concept development and case study. Environ. Res. Lett. 8, 035024. doi: 10.1088/1748-9326/8/3/035024
Guerin, T. F. (2021). Policies to minimise environmental and rebound effects from telework: a study for Australia. Environ. Innov. Soc. Trans. 39, 18–33. doi: 10.1016/j.eist.2021.01.003
Guérin-Schneider, L., Tsanga-Tabi, M., Roux, P., Catel, L., and Biard, Y. (2018). How to better include environmental assessment in public decision-making: Lessons from the use of an LCA-calculator for wastewater systems. J. Clean. Prod. 187, 1057–1068. doi: 10.1016/j.jclepro.2018.03.168
Ianniello, M., Iacuzzi, S., Fedele, P., and Brusati, L. (2019). Obstacles and solutions on the ladder of citizen participation: a systematic review. Public Manag. Rev. 21, 21–46. doi: 10.1080/14719037.2018.1438499
Jackson, T. (2016). Prosperity without Growth: Foundations for the Economy of Tomorrow. Abingdon, VA; Oxfordshire; New York, NY: Taylor and Francis.
Langhorst, T., Winter, B., Roskosch, D., Müller, L., Wang, Y., McCord, S., et al (2022). Low TRL guidelines. In Techno-Economic Assessment and Life-Cycle Assessment Guidelines for CO2 Utilization (Version 2). Global CO2 Initiative, University of Michigan. Available online at: http://hdl.handle.net/2027.42/145436 (accessed March 17, 2022).
Le Pira, M., Inturri, G., Ignaccolo, M., Pluchino, A., and Rapisarda, A. (2015). “Simulating Opinion Dynamics on Stakeholders' Networks through Agent-based Modeling for Collective Transport Decisions. Procedia Computer Science 52,” in The 6th International Conference on Ambient Systems, Networks and Technologies (ANT-2015), the 5th International Conference on Sustainable Energy Information Technology (SEIT-2015) (London). 884–889.
Loiseau, E., Aissani, L., Le Féon, S., Laurent, F., Cerceau, J., Sala, S., and Roux, P. (2018). Territorial Life Cycle Assessment (LCA): What exactly is it about? A proposal towards using a common terminology and a research agenda. J. Clean. Prod. 176, 474–485. doi: 10.1016/j.jclepro.2017.12.169
Loiseau, E., Roux, P., Junqua, G., Maurel, P., and Bellon-Maurel, V. (2014). Implementation of an adapted LCA framework to environmental assessment of a territory: important learning points from a French Mediterranean case study. J. Clean. Prod. 80, 17–29. doi: 10.1016/j.jclepro.2014.05.059
Majeau-Bettez, G., Wood, R., and Strømman, A. H. (2014). Unified Theory of Allocations and Constructs in Life Cycle Assessment and Input-Output Analysis. J. Ind. Ecol. 18, 747–770. doi: 10.1111/jiec.12142
Martinez Blanco, J., Finkbeiner, M., and Inaba, A. (2015). Guidance on Organizational Life Cycle Assessment. United Nations Environment Programme. Berlin, Germany: UNEP SETAC Life Cycle Initiative.
Morris, M. D. (1991). Factorial Sampling Plans for Preliminary Computational Experiments. Technometrics 33, 161–174. doi: 10.1080/00401706.1991.10484804
Mouterde, P. (2021). Le bois, une source d'énergie pas si verte. Le Monde.fr, June 4. Available online at: https://www.lemonde.fr/planete/article/2021/06/04/le-bois-une-source-d-energie-pas-si-verte-dans-un-marche-de-la-biomasse-qui-s-industrialise_6082762_3244.html (accessed October 14, 2021).
Onat, N., Kucukvar, M., Halog, A., and Cloutier, S. (2017). Systems thinking for life cycle sustainability assessment: a review of recent developments, applications, future perspectives. Sustainability 9, 706. doi: 10.3390/su9050706
Pizzol, M., Sacchi, R., Köhler, S., and Anderson Erjavec, A. (2021). Non-linearity in the Life Cycle Assessment of Scalable and Emerging Technologies. Front. Sustain. 1, 611593. doi: 10.3389/frsus.2020.611593
Quéheille, E., Dauvergne, M., and Ventura, A. (2021). Prospective life cycle assessment at early stage of product development: application to nickel slag valorization into cement for the construction sector. Front. Built Environ. 7, 130. doi: 10.3389/fbuil.2021.743948
Schrijvers, D., Loubet, P., and Sonnemann, G. (2020). Archetypes of goal and scope definitions for consistent allocation in LCA. Sustainability 12, 5587. doi: 10.3390/su12145587
Senga Kiesse, T., Bonnet, S., Amiri, O., and Ventura, A. (2020). Analysis of corrosion risk due to chloride diffusion for concrete structures in marine environment. Mar. Struct. 73, 102804. doi: 10.1016/j.marstruc.2020.102804
Senga Kiessé, T., Ventura, A., van der Werf, H. M. G., Cazacliu, B., Idir, R., and Andrianandraina. (2017). Introducing economic actors and their possibilities for action in LCA using sensitivity analysis: Application to hemp-based insulation products for building applications. J. Clean. Prod. 142, 3905–3916. doi: 10.1016/j.jclepro.2016.10.069
Sobol, I. M. (2001). Global sensitivity indices for nonlinear mathematical models and their Monte Carlo estimates. Math. Comput. Simul. 55, 271–280. doi: 10.1016/S0378-4754(00)00270-6
Sonnemann, G., and Vigon, B. (2011). Global Guidance Principles for Life Cycle Assessment Databases - A Basis for Greener Processes and Products. UNEP SETAC Life Cycle Initiative. Available online at: https://lca-net.com/publications/show/global-guidance-principles-life-cycle-assessment-databases/ (accessed April 16, 2021).
Suh, S. (2005). Developing a sectoral environmental database for input–output analysis: the comprehensive environmen. Econ. Syst. Res. 17, 449–469. doi: 10.1080/09535310500284326
Suh, S., Lenzen, M., Treloar, G. J., Hondo, H., Horvath, A., Huppes, G., et al. (2004). System boundary selection in life-cycle inventories using hybrid approaches. Environ. Sci. Technol. 38, 657–664. doi: 10.1021/es0263745
Suh, S., Weidema, B., Schmidt, J. H., and Heijungs, R. (2010). Generalized make and use framework for allocation in life cycle assessment. J. Ind. Ecol. 14, 335–353. doi: 10.1111/j.1530-9290.2010.00235.x
Ta, V. L., Senga Kiesse, T., Bonnet, S., and Ventura, A. (2018). Application of sensitivity analysis in the life cycle design for the durability of reinforced concrete structures in the case of XC4 exposure class. Cem. Concr. Compos. 87, 53–62. doi: 10.1016/j.cemconcomp.2017.11.024
Thomassen, M. A., Dalgaard, R., Heijungs, R., and de Boer, I. (2008). Attributional and consequential LCA of milk production. Int. J. Life Cycle Assess. 13, 339–349. doi: 10.1007/s11367-008-0007-y
Ventura, A., and Antheaume, N. (2019). Environmental assessment of carbon capture and utilization: a new systemic vision – application to valorization of nickel slags. in Proceedings of the International Workshop CO2 Storage in Concrete, eds Djerbi, A., Omikrine-Metalssi, O., and Fen-Chong, T. Marne La Vallée, (France: IFSTTAR.), 73–79.
Ventura, A., Ta, V. L., Kiessé, T. S, and Bonnet, S. (2021). Design of concrete : Setting a new basis for improving both durability and environmental performance. J. Ind. Ecol. 25, 233–247. doi: 10.1111/jiec.13059
Weidema, B. (2001). Avoiding co-product allocation in life-cycle assessment. J. Ind. Ecol. 4, 11–33. doi: 10.1162/108819800300106366
Weidema, B. P. (2003). Market information in life cycle assessment. Environmental Project. Danish Environmental Protection Agency. Available online at: http://www.norlca.org/resources/780.pdf (accessed February 28, 2013).
Zamagni, A., Guinée, J., Heijungs, R., Masoni, P., and Raggi, A. (2012). Lights and shadows in consequential LCA. Int. J. Life Cycle Assess. 17, 904–918. doi: 10.1007/s11367-012-0423-x
Zimmerman, A., Wang, Y., Wunderlich, J., Buchner, G., Armstrong, K., Marxen, A., et al (2022). TEA guidelines. In Techno-Economic Assessment and Life-Cycle Assessment Guidelines for CO2 Utilization (Version 2). Global CO2 Initiative, University of Michigan. Available online at: http://hdl.handle.net/2027.42/145436 (accessed March 17, 2022).
Keywords: circular economy, Material Flow Analysis, waste recovery, carbon capture, urban metabolism, stakeholders, decision, territory
Citation: Ventura A (2022) Transition life cycle assessment: A new method to face ecological transition. Front. Sustain. 3:801668. doi: 10.3389/frsus.2022.801668
Received: 25 October 2021; Accepted: 18 July 2022;
Published: 17 August 2022.
Edited by:
Alessandro Dal Pozzo, University of Bologna, ItalyReviewed by:
Mario Martín Gamboa, Rey Juan Carlos University, SpainChiara Magrini, Oeko Institut, Germany
Copyright © 2022 Ventura. This is an open-access article distributed under the terms of the Creative Commons Attribution License (CC BY). The use, distribution or reproduction in other forums is permitted, provided the original author(s) and the copyright owner(s) are credited and that the original publication in this journal is cited, in accordance with accepted academic practice. No use, distribution or reproduction is permitted which does not comply with these terms.
*Correspondence: Anne Ventura, anne.ventura@univ-eiffel.fr