- 1Institute of Environmental Sciences (CML), Leiden University, Leiden, Netherlands
- 2Environmental Research and Innovation (ERIN) Department, Luxembourg Institute of Science and Technology, Belvaux, Luxembourg
To support product and technology choices toward a more sustainable future, diverse assessment methods are used, involving life cycle assessment (LCA). This raises the question of their predictive validity. Whereas, many studies focus on quantitative uncertainty, here the main aim is to address the complementary qualitative aspect of the LCA-related model variants. To that end, we first specify three general influential aspects: (1) future conditions, (2) needed predictivity, and (3) mechanism coverage. These have been translated into a more concrete checklist for qualitative predictive validity. Second, we categorized the model variants into a limited number of basic model types, based on five predefined modeling characteristics. These model types show increasingly complex steps for investigating the future, illustrated with energy systems for transport. Different answers to the same questions may result. With increasing model complexity, the relevant questions may change from analysing specific products, to more general product systems, and next to product-technology domain systems. As a third step, the qualitative predictive validity of the nine modeling types is evaluated using the developed checklist. All have limited predictive validity, increasingly so for longer time horizons, as they lack most causal mechanisms, especially the institutional drivers for development and employment of technologies to emerge. Also, the future is only partially determined. For supporting choices, the conclusion is that the comparative analysis regarding long-term also broader product-technology systems has limited predictive validity. As a solution, conditional statements may show directions for explorative analysis resulting in highly tentative advice on potentially attractive directions.
Introduction
There is broad agreement that novel technologies and their extensive application are required for efficiently solving major environmental problems with a long-term horizon. Climate change is the major issue, followed by land use change, eutrophication, and more. The time horizon involved extends to several decades, as in specifying scenarios for 2050 or 2060. A recent example is the IEA net zero scenario study (IEA, 2021), covering the products and technologies to be developed for the future energy sector in detail. The basic question to be answered is: how will future reality and its sustainability characteristics become different long-term if we choose between product and technology options A, B, …X? They will change together as product-technology systems. Several modeling domains have evolved to help guide long-term decision support, see Table 1. Modeling domains here refer to groups of models with either similar features, approaches, or methods, as conventionally defined in the groups involved. All these modeling domains may cover the functioning of emerging technologies in their broader environmental, economic, and social contexts. Any model fit for this purpose may be included, though versions of life cycle assessment (LCA) are starting point here, because this modeling domain aims to realistically model all processes linked with the technology/product of interest, in as much detail as required to make such links. Questions on the long-term sustainability consequences of choices on products and technologies have led to new variants in LCA. New names in LCA include Consequential LCA, Prospective LCA, Ex-ante LCA, Future LCA, Dynamic LCA, and LCA of Emerging Technologies. See the report from the Swiss LCA Discussion Forum on this evolving subject in Adrianto et al. (2021). Consequential LCA (CLCA) is to assess the consequences of product and technology choices, with the product system constructed accordingly (Schaubroeck et al., 2021a). The models from the modeling domains differ in terms of causal mechanisms included, ranging from technical requirements as in LCA, to the motives of agents in ABMs, and to broad market mechanisms as in PEM, GEM, and IAM scenarios. Technology Assessment (TA), on New and Emerging Science and Technology (NEST) and Future-Oriented Technology Assessment (FOTA), also focus on long-term developments. Broader scenario modeling has expanded to futures beyond half a century in the climate policy domain and related energy modeling.
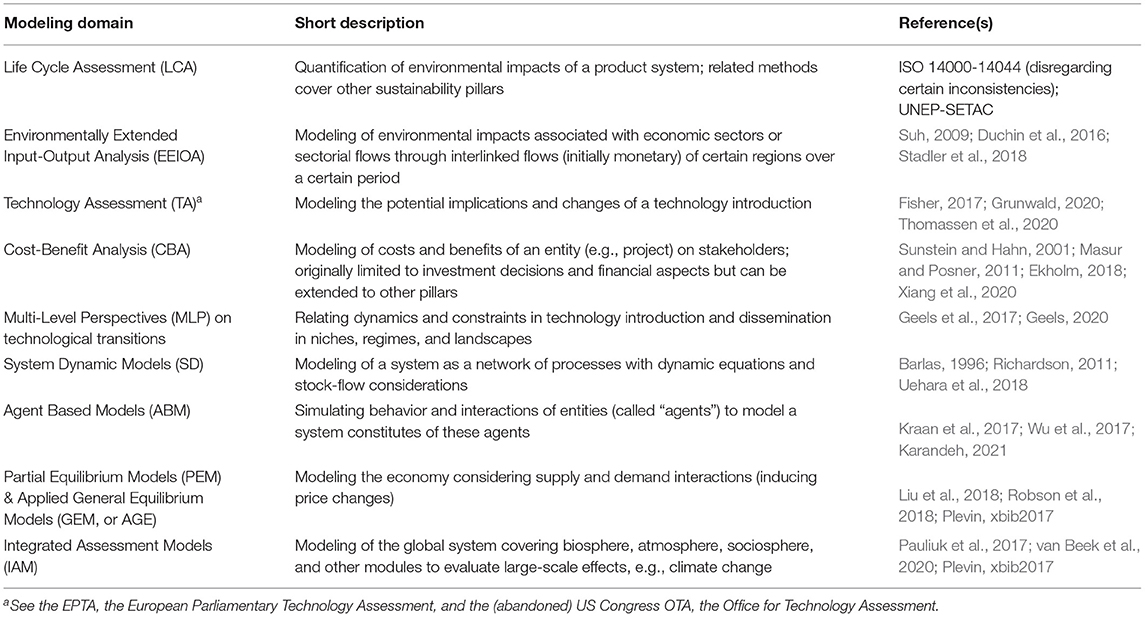
Table 1. A collection of modeling domains that can focus on long-term decision support, and have been linked with LCA.
Approaches from these modeling domains may overlap and support each other. When focusing on LCA, it may be used to refine EEIOA, GEM, and IOA, by adding technology details (Pauliuk et al., 2017). Conversely, these more general models may supply more encompassing background data and time-specified data bases (Mendoza Beltran et al., 2020). ABM and LCA may be coupled (Baustert and Benetto, 2017; Wu et al., 2017; Micolier et al., 2019; Walzberg et al., 2019). LCA may use PEMS to specify induced shifts between technologies.
A first finding is that the lines between the conventional modeling domains are blurring, and it is better to consider certain modeling types as we will elaborate in this work. As we define them here, modeling types are tailored and possibly combined approaches in practice to potentially better answer certain research questions.
A second finding is that these existing models even combined still don't cover all relevant dynamic mechanisms. As far as our knowledge goes, none of the models endogenize institutional developments, basic research outcomes, social preferences changes, and more. Neither does an overarching model exist which combines the partial causal mechanisms of the model types mentioned. Hence, the futures as modeled result from limited and partial causal mechanisms at best. How valid are their outcomes? What is their predictive validity? Given the known and unknown unknowns, this is a qualitative aspect, part of total uncertainty related to modeling inputs and structure. Our focus here is on qualitative predictive validity, as a qualitative aspect of uncertainty.
To evaluate their predictive validity, models are analyzed as to the uncertainties involved. Quantified uncertainty analysis of modeling outcomes is fast developing in the prospective technology domain (Anderson et al., 2014; Baustert and Benetto, 2017; Mariel and Meyerhoff, 2018; Qin et al., 2020; Cucurachi et al., 2021). The boundaries of quantified uncertainty analysis were investigated in the work of Mendoza Beltran et al. (2018). Some use uncertainty in a broader sense to also cover qualitative aspects. See for example Olsen et al. (2018). The uncertainty analyzed in our work also encompasses what has notbeen modeled, and what hence cannot be reckoned with in quantitative uncertainty analysis, partially overlapping with Olsen et al. (2018). It is imperative to consider this qualitative uncertainty especially for sustainability assessment, as pointed out by Schaubroeck T. et al. (2020). They distinguish three types of uncertainty and three strategies to deal with them. In our work we focus in detail on the qualitative uncertainty due to modeling and its aspects, which relates mainly with the specification of the impractical uncertainty type and the strategy to direct model development technically based on reason.
In this context, the overall goal and novelty of our paper is to organize and evaluate the specification of qualitative predictive validity in relation to the characteristics of the different model types used for long term prospective technology analysis. The subobjectives are as follows: (a) identify general influential aspects of models (section Identification of General Aspects That Influence Qualitative Predictivity of Models); (b) specify an evaluation checklist (section Evaluation Checklist of Qualitative Predictive Validity); (c) derive modeling characteristics and types (section Modeling Characteristics and Types) (d) analyze a focused set of modeling types and evaluate their predictive qualitative validity using the checklist (section Nine Main Model Types Described and Analyzed) and (d) conclude and provide an overall message and guidance (section Conclusions).
Identification of General Aspects That Influence Qualitative Predictivity of Models
Before jumping to the validity of specific model quantifications, we need a general framework on what may detract from validity, and what may contribute. In this section, we will identify three key aspects. These are to a certain degree intertwined but are here presented separately for ease of presentation.
First Aspect: Modeled Forecast Conditions and Their Validity
The first aspect concerns the conditions under which the model outcomes might come about. The modeling outcomes are then not necessarily accurate predictions anymore but rather are conditional statements. For example, if the market is demand constrained, which is a condition, then the environmental impact outcome is x, being an outcome that is influenced and restricted in its validity of this condition. The latter is a common condition applied in consequential LCA approaches and databases (Weidema et al., 2013). A second part is to be aware of the validity of these conditions. For example, is a demand-constrained market a representative, and thus a valid, condition also long term? There is broad knowledge on potentially relevant mechanisms, and under which conditions they are valid. Alternatively, these conditions can also be labeled as scenarios or scenario characteristics.
Further examples relate to climate and energy. The currently most detailed model of a future net-zero CO2 emission society (IEA, 2021) gives one roadmap scenario, with many sub-models with possible pathways. The report brings forward several times that outcomes are dependent on institutional adaptations, serving as the conditions. These include new energy markets fit for accommodating intermittent renewables. They do not specify such markets in possible content, but the condition is stated, qualitatively. An example is the use of car batteries for covering day-night shifts in solar cell production. In most current electricity markets, delivering back to the grid gives a lower price than recharging from the grid. Under these circumstances that option for using batteries will not emerge. A market design with real time pricing, equal for all, might create that incentive. That market is then to be designed, within broader constraints. Next, technical assumptions on future technologies are unavoidable. Small-sized nuclear fission energy is an option often mentioned, also in the IEA study. There are currently over 70 different designs at some level of development, see (IAEA, 2020) their Table 1, with different products and product combinations produced. Their future technical status is uncertain as is their timing and economic viability, also depending on several other technical aspects. There are also over 35 private companies developing fusion energy now (FIA and UKAEA, 2021), probably cleaner and long-term possibly less expensive. All fission and fusion developments depend on policy support. Even the sequence of technology introductions may determine outcomes. Betting on a long-term not optimal alternative now may create a lock-in on that technology, see Seto et al. (2016) for examples in climate change. Non-technical constraints are there as well. Substantial resistance to the use of nuclear energy exists now already, possibly fueled by yet unpredictable accidents. Finally: is the IEA 2050 scenario the most relevant one, and is ‘net zero by 2050' the realistic and relevant scenario goal to set?
Second Aspect: The Research Question and Its Predictive Nature
Validity considerably relates to the choice and decision at hand, translated into a research question. For example, which diesel car is best to buy now, economically and environmentally speaking? How do forthcoming battery-electric cars and new Diesel cars compare in terms of impact the coming decade? Which one should one choose to buy for environmental and other reasons, as a responsible consumer? These questions relate to a near future, where surrounding conditions may be assumed not to be much influenced and mainly constant: ceteris paribus. This simplifies modeling tremendously but has deep deficiencies for long-term questions. Which car drives and transport systems might best be developed for use in the 2040's and 2050's, research departments and research funders might ask? Which policies and institutional designs may best reduce climate emissions of person transport the next several decades? This might be a question in the public policy domain. Answers will certainly depend as well on developments in the total energy sector and the energy use sectors. Many specific future technologies will play a key role in answering such questions. LCA started with a focus on product choices and on product improvements “now,” though of course such choices will only work out in the course of time. Which car to buy for climate and broader environmental reasons? Even such short-term decisions in product choice and product development tend to relate to future developments. For example, should I buy a car now or later (Schaubroeck S. et al., 2020)? The answer involves a product improvement question, also considering future background conditions, thus necessitating an expansion of the basic LCA model, which has limited long-term predictive validity. The more the research question relates with future effects, the more challenging it is to ensure this validity and the more the model should take it into account.
Examples of questions. System level questions have come up on new and emerging technology domains. The macro level is what counts ultimately for most sustainability issues, as the aligned sum of all micro level performances. For any specific technology the macro level constitutes its surroundings. Technologies and their products are to be taken broadly, in line with literature on this topic (Thonemann et al., 2020; van der Giesen et al., 2020). The future is open also to fully new technologies, not requiring small-scale production now already, as indicated in the work of Arvidsson et al. (2018). Technology complexes such as “small-scale fusion energy” may be of limited probability but great importance, also for currently developing technologies. What can the role of specific battery-electric and hydrogen fuel cell drives be in CO2 emission reduction toward 2040 and 2060 and how are they best developed and induced? Answers would first require a specification of the possible energy sources, also for low-carbon combustibles. Electric cars will currently run substantially on coal in most countries. Will fuels be made combining renewable energy with CO2 from the air, possibly combined with CCS? Or will non-fossil hydrogen come from new-generation nuclear power plants, fission, or fusion? Answers of course depend on fundamental technology options and limitations. But that technical level, open itself, also results from more specific policies, at niche and landscape level, and from developing institutional frameworks, the regime level (Geels and Schot, 2007; Geels et al., 2017). An example of an ultimate question then might be: What might/could/should be the set-up for our future energy system, technically and institutionally? And which lines of energy-using technologies might follow?
Regarding specific technologies there would only be tentative and conditional answers. A simple example is on small scale secondary electricity production from car batteries to help stabilize a wind and solar dominated electricity grid over the days. Car batteries might well have the required capacity in this respect within a decade. With an adequate electricity market design this may provide owners with substantial income as an incentive, and society with a better option to shift to intermittent solar power. Without such a market, the required technologies—their hardware and software—will not develop, nor their infrastructure. Treating such secondary production options only at a product and technologies level, implicitly assuming the condition that these new markets will come, may well lead to ill-considered long-term advice.
Third Aspect: The Key Mechanisms and Their Coverage
Especially longer term, it is the mechanisms set in motion by the choice which determine developments, as part of the developing future system. Specific choices induce changes, in a world which is also changing due to many other willful choices and autonomous changes. Ideally, high predictive validity however requires the specification of “all relevant mechanisms.” Yet, while some mechanisms may be relatively constant over time, it is these changing key mechanisms that should be included as well. The assessment of validity status also needs the consideration of what has not been included in the model, especially key mechanisms.
Examples of constancy? The propensity to spend additional income on air transport was stable in a broad range of income categories, an OECD survey concluded (Goodwin, 2012), giving a prediction on future volumes with rising incomes. However, Covid-19 might change that picture, not itself, but the public and private reactions to the pandemic. Similarly, there are trends toward exclusions of countries in resources, and other supply, and to at least create protected regional “own” supply of primary resources and sensitive technologies. See on such policy ideas for example COM (2020). How will this work out? Possible mechanisms set in motion may include reduced competition per region and next public policy-based oligopolies, reducing technology development. Supply instability may result instead of increased stability, combined with higher costs, and different product developments.
Evaluation Checklist of Qualitative Predictive Validity
The previous section touched upon three general aspects of qualitative predictive validity evaluation of models, which we translate into a more concrete checklist for evaluation purposes in this section.
Four concrete evaluation questions need to be answered in any modeling study regarding choices on normative sustainability impact of future technology choices, with climate change here as a focus. The prime evaluation question, related to the research question, is if the choice alternatives link to real-world choice alternatives. This relates to what in LCA is the Goal & Scope Definition (ISO 14040-14044). Here, there is the additional restriction that modeling is meant for long-term effects of choices. There are deep consequences, as specific product-technology options now will hardly ever be the ones in place over three or four decades. Somehow the diffuse future must be covered in the modeling for choice support, or the limitations of a simpler choice set are to be made clear, see Table 2.
The second evaluation question relates to a strategic aspect of the choice options investigated. Do they fit in with a general pattern of choices on the agenda or are they deviating, for some reason?
The third evaluation question is if the model is complex enough to handle the necessary complexities of related long-term developments. Any model will have major predictive deficiencies, but they are very different between modeling types.
The fourth evaluation question is if the choice alternatives are well-connected to the model variables driving the model outcomes. Technical characteristics of product-technology systems may not be connected to market mechanisms determining their outcomes. The relevant level of detail may often be difficult to realize.
The details of the checklist may be adjusted, but these evaluation questions will give guidance to qualitative predictive validity evaluation. More detailed applied reasoning is possible for specific modeling set-ups, as in the model types distinguished below.
Modeling Characteristics and Types
Basic Model Characteristics
Given the conditions of interest as defined in the previous section, we distinguish five basic model characteristics relevant for validity analysis in product-technology models, i.e., models that aim to represent product-technology systems, i.e., effects of product-technology choices. They are (1) the treatment of time in linking processes; (2) the period of analysis; (3) the coverage of the background system (e.g., electricity production); (4) the number of future parallel developments considered; and (5) the constant or dynamic nature of the choice options to be compared.
Regarding the treatment of time, a first characteristic concerns the sequential nature of processes. In the real world each input in a process must have been produced before, as an output of a different process at a previous moment in time. Models may simplify reality by leaving out sequential processes time specifications, fully, or partly. The second time characteristic relates to the period chosen for the process data specification. That may be a “current” or some future period of process specifications, a series of periods, or a continuous development of all processes. In the real world there is a continuous development of processes in time. Combining sequential life-cycle specifications with processes development and emerging new processes is the most realistic option, with some research in this direction ongoing in LCA.
The third characteristic relates to the scale for which the choice and its effects are relevant. Also long term, some choices may not much influence the full world. They can be handled at a micro level, under that condition. An example might be the anti-fouling coating options for ocean-going vessels as under development, a micro level. Or it may cover a sectoral meso-scale, like different electric drives in person cars in the transport sector. Alternatively, the choice may cover developments in full society, for global-wide changes in energy use regarding many sectors, i.e., macro-scale. In the real world, any choice, also at micro level, will influence the full society, at a global macro level, to some extent. But this will be more important regarding predictive validity when larger amounts are involved in the choice and hence the study.
The fourth characteristic is on how future developments are covered in the model. The future may be approached as a single development line; as possibly diverging technologies futures (named: Techno-Futures); or broader, as diverging socio-economic and political scenarios (named: Soc-Econ-Pol Scenarios. If outcomes do not depend on the scenario routes, they may have a higher predictive validity.
The fifth characteristic concerns the nature of the choice options. The product-technology options considered in the comparison may be taken as constant, as given already or as assumed to have been developed at some future date. Alternatively, the choice options themselves may develop dynamically in the models, as will usually be the case in reality. Learning curves constitute one mechanisms to specify improved performance in time. With choice options themselves developing dynamically, the choice is not between one or more specific products and technologies anymore but on development lines in broader defined product-technology systems.
See the survey in Table 3.
Combining all five characteristics, 108 types of models are maximally obtainable. However, the classifications are not independent between characteristics. It does not make sense to combine developing choice systems (5); in a single period (2); and without sequential processes specification (1). We select some relevant combinations, resulting in nine modeling types.
For a given choice question regarding products and technologies, several modeling types and variants may be used. The comparison of choice options may be done with-or-without, comparing new situations with the autonomous development or only with each other. Alternatively, it may follow the before-after analysis of alternative options, as is required for the discounted results in Cost-Benefit Analysis. This requires the sequential specification (1) of continuously developing processes (2). Basic choices in these five characteristics determine the structure of models.
Modeling Types Distinguished
All types of product-technology models covered here reckon with a basis of LCA modeling, explicitly or implicitly. We specify only nine modeling types, see Figure 1, but more may be distinguished. Before going deeper into each one in the next section, section Nine Main Model Types Described and Analyzed, we present here an overview of them. In fact each of them can be to some extent considered as an improvement of the other, leading to a stepwise modeling improvement scheme.
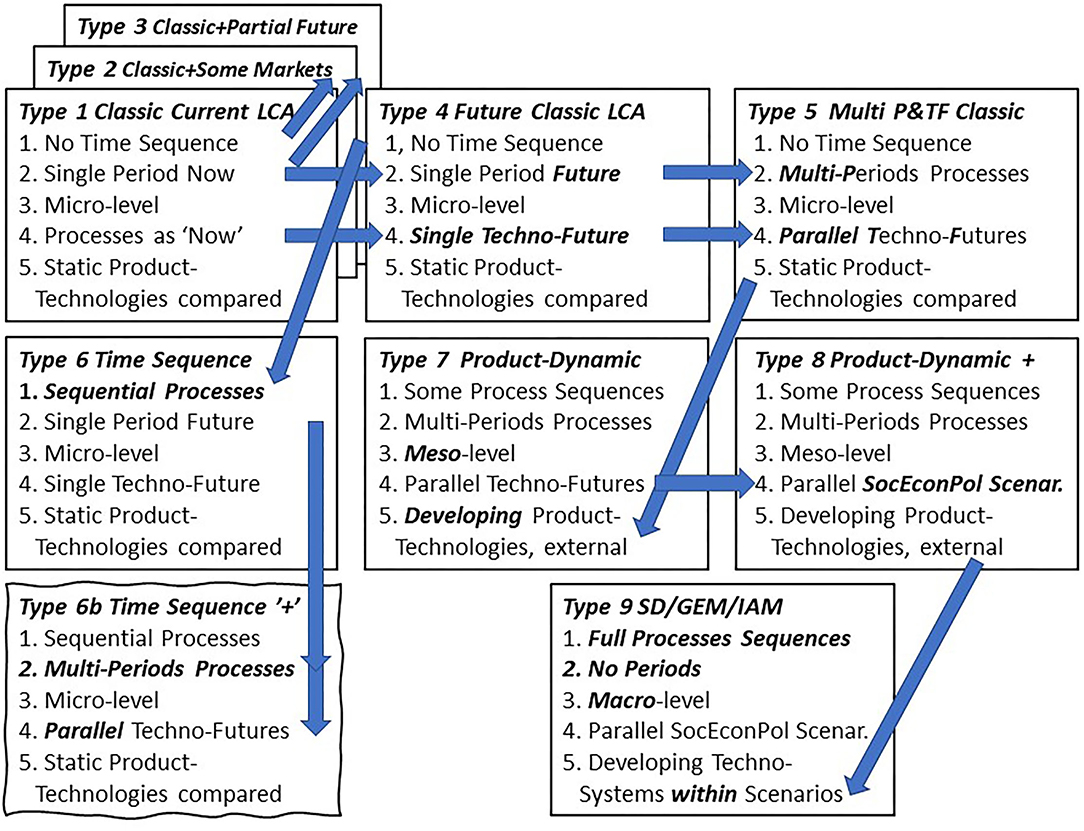
Figure 1. Nine types of product-technology models presented as stepwise evolving (blue arrows) from Classic LCA models. For each type the five basic model characteristics are specified (discussed in section Basic Model Characteristics).
A first model type has no sequential processes specification and covers a single “current” period of processes specification: type 1. A first variant moving toward explicit consequential LCA modeling reckons with induced market shifts, type 2. Next is the specification of some future processes, such as for end-of-life operations, resulting in type 3. Type 4 results when the data used refers to an externally established database for a single future period. These simple conventional LCA-models (Heijungs and Suh, 2002), especially Ch.2, may give answers on how to contribute to a net zero emission future situation, but with substantially lower predictive validity than models including relevant dynamic mechanisms and considering different future development pathways for overall society. However, the computational structure of the conventional LCA-model is simple, with variants easily made, and sensitivity added to quantified uncertainty analysis (Heijungs, 2010).
Starting from type 4, two new variants emerge. Type 5 reckons with several periods of process development and specifies parallel options for the general development of technologies. These are data adjustments, leading to parallel variants in outcomes. Also starting from type 4, type 6 reckons with the fact that inputs in a process have been produced in a previous process in time, specifying processes sequentially. Combining with the type 5 additions this leads to type 6b, with all processes from all periods sequential, and parallel systems for different techno-futures. This constitutes the most complex analysis of constant products variants to be compared.
Type 7, starting from type 5, replaces the constant technologies analyzed by dynamically developing product-technology systems. These are specified externally, as based on learning curves leading to more efficient products, different for the systems to be compared. We combine this type with a move toward the meso level, using sector data for the developing background systems, a simplification relative to type 6b. Though more realistic this complicates the comparison of alternative options. Alternatives then may be defined more generic, therefor indicated as product-technology systems. The more aggregate specifications lose some of their direct relation to environmental impacts, and costs.
In this situation of modeling, the exogenous techno-futures may be endogenized to some extent, by placing them in socio-economic and political scenarios, entailing type 8. The core focus is still the comparison of domains of exogenously defined developing technologies, encompassing also developing broader scenario surroundings. The final step is to endogenize the development of the technologies to be compared, next to all other technologies developing endogenously, type 9. The level of detail of the investigated choice options may then by higher. However, in the steps toward endogenized developments, the role of dynamic mechanisms increases. They are to create the future in the model. The choice options may then shift from specified products and technology domains to incentives regarding specific or broader product-technology systems.
Several further differentiations are possible, in and between types. For example, in the domain of climate policy, the focus has shifted to national policies. It then becomes necessary to specify embodied energy flows in international trade, see the survey paper in Tukker et al. (2018), multi-years data oriented. Applied analysis is for example in Weber et al. (2021) and as an early example (Ackerman et al., 2007). Interestingly, these studies come to quite opposed policy implications: reducing international trade vs. improving technologies internationally to obtain similar low emission levels. The EEIOA based multi-year data may form the basis for specifying background techno-scenarios, in type 5, 6, and 7, then referring to totals in society.
Nine Main Model Types Described and Analyzed
Nine Main Model Types Described
All model types have the same goal: to depict how the future of the analyzed system will change depending on the alternative options chosen. Validity then relates to how good they are for that purpose. Unspecified validity means low validity.
Type 1. Classic Current Period
Single period processes now; no processes time differentiation, given product technologies, full product-technology system coverage.
The short-term question to be answered might be mainly of interest for the product user, namely which consumer-scale product amounts to acquire for environmental reasons, now or later, and for the producer, how to improve it, on short notice. This concerns a micro level.
The simplest life-cycle models, as LCA-models started to be, refer to one period. All process relations take place in this one period, without time specification. The recycled steel from the end-of-life of cars investigated may function as an input in any of the preceding steel-using processes, as a model artifact closed-loop. In reality, closed and open loop may differ substantially (Larrain et al., 2020).
For long-term analysis, the empirical current processes data may be used as a proxy, predictive for the future. Process changes involve only those brought in by the user concerning the to-be-investigated product-technology alternatives. The theoretical structure is like that of micro-economics where a specific price or volume change is assumed not to change the rest of the economy: ceteris paribus. Similarly, the type 1 model is like a partial equilibrium analysis model with all outputs and inputs ultimately adapted in volume to the choice option, “to what ultimately is required.” There is not a future situation depicted, as in type 4.
An example is the comparison of driving a new Battery Electric Vehicle (BEV) with a new Diesel Internal Combustion Engine (ICE) vehicle, using the current electricity mix and all other current processes, including recycling technologies and recycling percentages. The choice relates to the product characteristics and the volume changes in all background processes. The outcomes may favor Diesel, as in regions with now much coal-based electricity, or BEVs where hydro and nuclear generation are dominant, see for example the work of Zheng and Peng (2021). The outcomes are not particularly valid for the longer term if the electricity mix would change, and the ceteris paribus assumption hence becomes less realistic.
Overall, the outcomes are representative for the micro level only (like consumer-amounts), not how the choice would work out for the full economy. At larger scales, if BEV replaces Diesel, considering the choice dynamics, the relative price of fossil fuels will drop, increasing air flight and many other fossil fuel applications. Quantification of such real-life mechanisms is fully absent in this modeling type. Even short-term, substantial differences between the macro-level and the micro-level outcomes are possible. And of course, cars have a lifetime beyond just a few years. Conclusions on which choice to make now remain substantially unfounded. As a proxy for the long-term future, other model types are more relevant.
Type 2. Classic, With Selected Market Shifts
Single period “now,” partial processes development by market shifts for given process alternatives, no time sequence specification differentiation, full product-technology system coverage
The questions are as in Type 1, but with more focus on induced market mechanisms.
Some dynamic mechanisms may be covered externally, to better indicate the consequences of the choice at hand. Key is reckoning with potential substitution mechanisms, as in micro-economic partial equilibrium analysis. There then must be different processes available and specified with the same functional output. For example, reductions in volume demanded may be the costliest, usually older processes, and volume increases result in more of the newest more efficient technologies. The time sequence is not specified but the composition of the background part of the product-technology systems changes. There is though no development of processes considered, as through process innovations.
The time frames involved will increase somewhat, reducing the validity of the ceteris paribus assumption for the process specifications. The dynamic changes introduced by hand will depart from what is usual in substitution processes in economic modeling, where supply and demand reactions are non-linear, tend to be partial only, and develop over time. See (Liu et al., 2018) Chapter 3 for methods and an application related to CO2 emissions from the electricity domain. Real elasticities of supply and demand, time dependent, will however lead to a web of indirectly induced mechanisms. They are beyond this type of modeling.
In the car example, referring to totals sold, BEV cars will use more of new electricity generation technologies, and remaining Diesel cars will use cleaner refineries on average, as the oldest will close. These are estimates of a somewhat open nature. If for more electricity new intermittent renewables are constrained as for materials and administrative reasons and inadequate market design, more coal fired power may come. Such indirect micro-economic and further macro-economic consequences are not covered.
Overall, the effect on the validity of outcomes of moving from Type 1 to Type 2 modeling may be relevant for short to medium terms but can hardly be seen as realistic for long-term analysis. For longer-term questions, neither of the five main characteristics of validity conditions can well be covered.
Type 3. Classic With Partial Future
Single period “now+,” some future processes specifications for long lasting products, full product-technology system coverage
The question is like that for Type 1 but concerns in this case rather choices on product-technology systems with a longer lifetime. To further align with reality, key processes are specified here as assumed to be functioning at future date or dates of their functioning. The modeling structure is that of Type 1 again.
Validity could increase if the added future processes were more realistic, closer to the future reality. An example is the analysis of buildings, with changing energy use during their use period, and future waste processing end-of-life, as in Su et al. (2017). However, the future reality envisioned is one of many technical options and might just be a desired scenario of reality, with unknown predictive probability. Detrimental for validity is the mix of old process data and the newer assumption-based ones, adding some inconsistency, easily half a quarter century apart in practice, with current data usually being old data already.
To conclude, validity of type 3 is limited due to limited nature of the alternatives compared; due to the internal inconsistency of processes regarding different time frames; and due the lacking micro-to-macro relations. For longer-term questions, neither of the five main characteristics of validity can well be covered.
Type 4. Future Classic
No Time Sequence, Single period, single future, full product-technology system coverage
The question here broadens to which product-technology variants and directions are most worthwhile to develop, a question for product developers, producers, researchers, and research funders.
The product-technologies to be compared again are externally given and constant, as with Type 1, but here refer to a future period, with the choice processes and background processes by then to be specified somehow. The single period assumption allows again for what in micro-economics is considered a steady-state equilibrium, resulting if all processes would remain that way forever, only possibly changed by the choices at hand. The product-technology system is fixed as far as possible. Adding market mechanisms as in Type 2 seems difficult at best. Differentiating processes as to times, as done in Type 3, seems open to difficult choices. Predictive elements loose validity the farther away the future chosen is.
Considering the car comparison, ecoinvent apprehends, for example, future electricity mixes based on the “current policies” scenario of the IEA (2016). That would constitute a global disaster climate scenario and is better be avoided. However, will it be? Products-technologies analyzed may be defined broadly. For example, large scale nuclear co-production of electricity and hydrogen, with hydrogen fuel cell car in transport, and H2 for airplanes, in several variants, can be compared to improved fossil fuel applications with zero net emission assuming 100% CCS. Such comparisons have low validity as hardly being predictions. But they may shed light on technology potentials, and the conditions for their possible occurrence. For example, the use of car batteries for grid stabilization under large scale intermittent wind and solar production would require an electricity market design fit for that purpose, as IEA (2021) states. The stationary use of second life car batteries could then add to that role substantially, as Fallah et al. (2021) state for Ireland, with regionalized impact assessment possibly following, see Verones et al. (2020).
Still broader discussions may be opened as well, such as on the role of replacing natural gas for heating buildings by hydrogen or synthesis gas vs. closing off gas and shifting to electric systems. The internal structure of these product-technology systems can be clarified to some extent. Backcasting can give some insight in the requirements to arrive at the options. All choices become broader than just on car fuel source options.
For models of type 4, all scenarios are external, covering options and hardly predictions, now referring to the same future period. Conclusions on specific choices have limited validity. They have low reality value as they become conditional on several non-predictive assumptions. How options or directions might fit into the macro-structure of society is not part of the model type, nor are the incentives required to move toward them.
Type 5. Multi Periods and Futures Classic
No time sequence, multi periods combined with parallel futures, full product-technology system coverage
The questions to be answered become conditional regarding the specified technology background conditions: how would product-technology systems function and compare under different specified technology background conditions?
The product-technology variants to be compared are externally given again, assumed for the year (or broader period) of the analysis. New here is the systematic independent development of background futures. For each of the futures several periods are chosen. The steady-state equilibrium performance for each variant can then be specified. The futures are mostly technical, not encompassing broader full-economy scenarios. The simple model structure still allows for easy variation of combined foreground and background processes.
In the car transport example, several fuel source variants might be investigated, each one placed in several future background conditions, including different electricity grid mixes in each period. Different fuel sources may perform quite differently according to this model type with continued Diesel use but with CCS coming out on top; a well-estabilised wind and solar based electricity supply system; and low-cost nuclear or solar hydrogen production. In addition, material supply constraints (de Koning et al., 2018; Deetman et al., 2021) may influence relative impact scores substantially, mostly favoring fossil fuels with CCS, as non-fossil fuel systems have higher and more complex materials requirements.
For this type 5 models, conclusions on specific choices are mostly hard to make as outcomes are conditional on several combined assumptions. Furthermore, they don't link to the macro-level consequences, nor to the required policies and institutional developments. Comparative results on product-technology options have a low validity, hardly supporting choices. Even if outcomes align over several techno-futures they might still diverge for still different ones. The solution of combining several techno-futures outcomes based on their probability, as a predictive quality (Blanco et al., 2020), presumes that hard-to-produce knowledge.
This analysis might have other functions, as in indicating possible hotspots for research and policy.
Type 6. Time Sequence Single Period and Future
Time sequence specified for a single data period, single techno-future, full product-technology system coverage in time
Core question for specific product-technology choices is on the distribution in time related to the time sequence of processes inputs and outputs. The defining characteristic is that the product-technology system processes are specified sequentially, usually in years but with other options also possible, e.g., months (Beloin-Saint-Pierre et al., 2014), and possibly depending on the nature of the processes involved. The product-technology variants to be compared are constant and externally given again, functioning from a chosen time span onward. An extension could be to reckon with different background Techno-Futures, as Type 6b, in that sense becoming like type 5. Ground-breaking work for this option is in Cardellini and Mutel (2018) and Pigné et al. (2020), also based on methods development by Tiruta-Barna et al. (2016) and with existing alternatives in literature (Beloin-Saint-Pierre et al., 2017). Each process is placed in time, with its inputs received from processes in previous periods, based on their supply chains. This process specification in time is here external, possibly using attributional process data for operational simplification, with software linking the supply chains for each process. For climate change an adapted impact assessment model has been applied, with superseded discounting. Such a method may also be placed in the broader context of the circular economy, see in this sense (Sigüenza et al., 2021), as artificial in-system closed loop is not possible in this model anymore. Their model is broader than common LCA focus, referring to broader business and public strategies.
In the car fuel sources example, climate outcomes will show substantial differences between a single period static and this new dynamic approach. Discounting makes a large difference in absolute outcomes, but maybe less so in relative outcomes. Diesel comes out worst, without CCS, but policies requiring CCS are possible (Jenkins et al., 2021). Upstream emissions are relatively higher in the BEV case, see their Figures 2, 3 (Pigné et al., 2020). Since they focus on an attributional LCA for the case study, these emissions also occur before the start of the functioning of the cars, up to 17 years for the Diesel and nearly 20 years for BEVs. Usage and disposal are forecasted in the future, however.
Yet, they foresee an adaptation to consequential LCA focusing solely on the relative future, which is also covered by this type 6 in general.
Expansions of Type 6 may first involve adding periods and adding parallel futures, as type 6b. Next, developing product-technologies may be considered, like in Type 7, but then having Time Sequences specified for each period-future combinations, see Figure 1.
Type 7. Product-Dynamic Multi-Periods Multi-Futures
Some process sequences, externally specified future periods series, several techno-futures, for developing product-technologies
The questions regard strategies of governments and firms: how may broad technology scenarios develop in time and how would specific product developments fit into these futures? In other words, meso- to macro-scale questions are of interest for type 7 models.
The difference with Type 5 is that it is not a choice between options considered for years Xi placed in dynamically developing background contexts, but an analysis how a future set of developing product-technology systems might function in these different futures. It also reckons with the system span of specific product-technologies, from production to use, to repair, to end-of-life, as in Type 6b. A model including these characteristics has been developed in the work of Sigüenza et al. (2021). See their Module 2 in Figure 1, illustrated there with a single future. Their model is broader. In any specific year there will be a combination of older and newer product-technology versions and their product-technology system processes, the stocks, which together determine the emissions or resource uses in that year. The time inconsistency has been resolved partly by splitting the process system into independent parts, “treating each life cycle stage as an independent system” (Sigüenza et al., 2021, p. 569), with stages developing over the years. Their focus is on material recycling, with different strategies and technologies investigated (Schaubroeck et al., 2021b), and see Schaubroeck et al. (2021c), discuss such loop modeling over time, where loops are propagated sequentially, in the specific context of the LCA study. The distinction between closed loop and open loop recycling here becomes an empirical issue, as indicated by Larrain et al. (2020).
Considering the car example, closed loop recycling of previously produced discarded cars can after all only go into the production processes of newly developed cars, produced before their production. The analysis could give an indication how different developing transport drives could function in broader techno-futures.
This Type 7 has an increased complexity in reasoning relative to Types 5 and 6. There are three basic characteristics to consider: parallel futures to compare, different versions of a developing product-technology systems to compare; different process activities developing in time to envision and to analyze as to their consequences. Their combined relations require extreme clarity in their treatment.
The predictive value of the LCA model part of the total model has all limitations of long-term predictions: the future is developing autonomously but will also be made intentionally. The model validity is very limited as the broader background, linking sectoral to macro level, is not present in the Techno-Futures model. Outcomes have limited validity due to complexity of the interrelated conditions implied in their emergence.
Type 8. Product-Dynamic Periods From Scenarios
Some process sequences, multi-periods, multi-scenarios. Several periods per parallel scenario, with developing product-technology systems placed in Soc-Econ-Pol Scenarios
Research questions concern dynamically developing and broadly defined product-technology systems. How do they fit into the developing surroundings, their background systems? What might be mechanisms leading to their development and to that of the broader technical surroundings?
Broad Soc-Econ-Pol Scenarios have been developed with a focus on covering potential real-life developments. Such scenarios may depict boundaries to a developing world. They are not predictions but socio-economic and political options which to some extent limit what in a technical sense may come about. They tend to cover periods of decades at least, now to 2050 and 2060, and increasingly beyond. Main examples in the normative domain are the IPCC-collected and designed scenarios, now numbering well over 1,0001. The predictive validity of the outcomes remains limited however, as even the most encompassing IAMs hardly cover specific policies and institutions, see the survey in van Beek et al. (2020). They mainly state specific policy goals, with the assumption that these can be reached, supported by some evidence.
The groundbreaking work for their LCA-type application has been prepared in workshops in a multi-national group, resulting in the work of Mendoza Beltran et al. (2020). They used the Integrated Assessment Model (IAM) IMAGE, developed for energy scenarios at the Netherlands Environmental Assessment Agency (PBL), and created detailed processes in the framework of process specifications of the LCA database ecoinvent, where possible. The generic IAM functions as the overall framework for such detailing. The method can be applied to broader scenarios than for energy alone (van Beek et al., 2020). Moreover, the specification of future technologies for a few transport fuel sources considered is by necessity subjective and partial. Only a limited number of detailed options can be included, as otherwise the number of parallel options would explode. The link to climate part of Integrated Assessment Models is more adequate than in terms of CO2 equivalents in an LCA impact assessment, there based on time related climate forcing contributions.
The strength of this type of analysis is that it can link to scenarios deemed fundamental for our future, also in an international context.
Type 9. System-Dynamic, Time Span Lang, Multi-Scenarios. Covering Consecutive Time Spans Independent of Product-Technology Systems With Techno-Systems Developing Endogenously
Research questions here relate to how the incentives and policies in different scenarios may lead to different product-technology systems, and next at the more detailed level which specific product-technology systems form the basis leading (here) to climate emissions.
The future is not split into periods but has continuity over the time spans (e.g., years) covered. The development of new technology systems goes into decades or more so, as with fusion energy, with widespread adoption taking up decades again.
Coming back to the car fuel source example, electric cars started running using fossil fuel-based electricity mainly in many countries. Some Scenarios depicted a solution, but none of them showed how the incentives may create the shift to non-fossil fuel energy systems (Kramer and Haigh, 2009) indicated that market penetration of new energy technologies can be fast up to a modest share of a few percent of total primary energy input. It then slows down due to different constraints: supply restrictions; fixed investments in other technologies; psychological; regulatory; opposition by to-be phased out activities; and budget and other public policy constraints. In the last decade the fast rise of wind and solar has led to a minor fraction of the total energy supply, in absolute terms less than the volume increase of still dominant coal, oil, and natural gas. Which mechanisms might change this situation?
How to resolve such issues is fundamental for our long-term future, and at the same time the most difficult to answer in applied modeling. Such models don't exist and if developed could only be very partial. Key will be how strong enough generic drivers may be developed at primarily the institutional level. If this issue is not actively resolved, macro-level scenarios remain wish lists, or fear lists.
How to include steering mechanisms, which are complex and varied, and difficult to predict? There is no encompassing let alone unified political philosophy, from the local to global scale. There are no general views on public administration at different levels. And certainly, China, India, US, and EU are different, also in their views. Still, this is the level where the future is made, within the physical limitations which constitute the basis of society. Steps linking to our material basis, as have been developed in Type 8, might be followed with development and linkage to this higher order type of long term Soc-Econ-Pol Scenario modeling. Their empirical validity would remain limited. However, they would have a conceptual link to influential institutional driving mechanisms and resulting technology changes, including physical changes like climate emission reductions. This would at least limit their now free-floating nature.
Validity Restrictions per Model Type
Models may have specific validity restrictions, per case study, related to the qualities in their applied modeling, and related to the research questions to be answered. Developments, also in databases and software, are ongoing. Better quality may contribute to reduced quantified uncertainty and somewhat higher overall validity. There are however inherent restrictions for each of the nine modeling types in terms of qualitative predictive validity. We summarize them in terms of a selection of the five main validity evaluation questions from Table 2, here in Table 4. Since we focus on long-term validity, this is given priority in the evaluation of the types. Beware that unknown validity must imply low validity.
Dealing With Limited Validity of Modeling Outcomes for Technology Choices
For all types of model the predictive validity is limited and indicated only qualitatively. IAMs, type 9, are most open to include more relevant mechanisms. Such models will still show deep layers of empirical uncertainty, as Athanasoglou et al. (2021) indicate for scenario studies in the climate domain.
Different strategies have evolved to deal with this limited predictive validity. Hof et al. (2010) aim to reduce the chance on the worst outcomes for the climate domain. Similarly, Athanasoglou et al. (2021) apply this approach to climate policy choices, minimizing the maximum loss. The shift from optimizing to satisficing (Simon, 1956) next to minimax is to avoid the worst outcomes. This does however not improve the predictive validity of the scenario chosen. One direction for resolving this issue is first to define a relevant domain of scenarios where any realistic prediction would fit into, the boundary scenarios. Already the set of scenarios constituting the assumed realistic domain may require including highly improbable scenarios.
There are some checks possible on the feasibility of scenarios, as by backcasting the (desired) results in terms of required changes on the route to that future. Cars driving in 2050 must have been produced in the years before, and their factories and the mines to produce the materials before again. Though possibly increasing subjective probability of scenarios, also backcasting cannot resolve the deep lack of predictive validity in goals-based long-term scenarios.
A strategy shift or scope expansion in choice questions may reduce the lack of validity somewhat. A shift from specific product-technology policies to more generic driver policies is possible in principle for type 9. Such broadened scenarios have been indicated for the climate domain in Huppes (2019)—specific regulations and subsidies in chapter 3 and generic taxes and infrastructure in chapter 4—but have not yet been developed into quantified scenario models.
When focusing on a detailed technology level, there will remain deep technical uncertainties, increasing with time. Will cheap (real) green hydrogen come by 2050 or 2060, as possibly micro-nuclear based (IAEA, 2020)? What would be the most reasonable strategy with several such highly unpredictable options present, in several domains? A mixed strategy might come out, striking a balance between making broad technology choices now, as open as possible, and to create incentives and infrastructure options least linked to specific technologies, with revisions in the process of years. That double-mixed strategy may constitute the most strategic choice level, requiring insight in possible directions and incentive mechanisms. These can then be based among else on current model insights, fit for creating desired developments, while still allowing for flexible changes based on new and future more technical model insights and incentive insights.
Conclusions
• For longer-term questions, modeling extensions to overcome basic-LCA model limitations have developed, as different types. The same questions may well lead to different answers in these different types of analysis. They should be well-distinguished.
• Long-term technology-specific studies, including IAMs, have limited predictive validity, regarding quantified uncertainties, and qualitative considerations. They lack realistic dynamic mechanisms for choice options and background technologies.
• Using well-known multi-scenario models may increase subjective probability of the outcomes but not their basically science-based predictive validity.
• Results of current long-term technology-specific models are by necessity based on unconfirmable policy and technology assumptions. Their unknown but limited validity will make policy advice on specific choices of limited value, even dangerous.
• Advanced types of long-term analysis involving climate effects seem possible in type 8, as a hybrid analysis involving results of Integrated Assessment Models as an encompassing background framework, where more specific technology options are placed into exogenously.
• Incentives and options creation regarding climate, resources, and nature quality seems a priority task in scenario models development. This would be the core addition in type 9 models, to be further developed.
• Ideal fully predictive models cannot exist as the future is substantially open. Main predictive modeling limitations may be specified, using a checklist as in Table 2.
• Finally, technology detailed models may be very useful in ways different from giving predictions. They may give insights in potential system relations, under well-defined assumptions and conditions.
Author Contributions
GH did a main part in design, writing, and editing. TS gave substantial methods input and corrections, and supplied broad links to literature. Both authors contributed to the article and approved the submitted version.
Conflict of Interest
The authors declare that the research was conducted in the absence of any commercial or financial relationships that could be construed as a potential conflict of interest.
Publisher's Note
All claims expressed in this article are solely those of the authors and do not necessarily represent those of their affiliated organizations, or those of the publisher, the editors and the reviewers. Any product that may be evaluated in this article, or claim that may be made by its manufacturer, is not guaranteed or endorsed by the publisher.
Acknowledgments
We would like to thank the editor and the reviewers for their feedback that helped to improve the manuscript, in several rounds.
Footnotes
References
Ackerman, F., Ishikawa, M., and Suga, M. (2007). The carbon content of Japan–US trade. Energy Policy 35, 4455–4462. doi: 10.1016/j.enpol.2007.03.010
Adrianto, L. R., van der Hulst, M. K., Tokaya, J. P., Arvidsson, R., Blanco, C. F., Caldeira, C., et al. (2021). How can LCA include prospective elements to assess emerging technologies and system transitions? The 76th LCA Discussion Forum on Life Cycle Assessment, 19 November 2020. Int. J. Life Cycle Assess. 26, 1541–1544. doi: 10.1007/s11367-021-01934-w
Anderson, B., Borgonovo, E., Galeotti, M., and Roson, R. (2014). Uncertainty in climate change modelling: can global sensitivity analysis be of help? Risk Anal. 34, 271. doi: 10.1111/risa.12117
Arvidsson, R., Tillman, A.-M., Sandén, B. A., Janssen, M., Nordelöf, A., Kushnir, D., et al. (2018). Environmental assessment of emerging technologies: recommendations for prospective LCA. J. Indust. Ecol. 22, 1286–1294. doi: 10.1111/jiec.12690
Athanasoglou, S., Bosetti, V., and Drouet, L. (2021). A satisficing framework for environmental policy under model uncertainty. Environ. Model. Assess. 26, 433–445. doi: 10.1007/s10666-021-09761-x
Barlas, Y. (1996). Formal aspects of model validity and validation in system dynamics. Syst. Dyn. Rev. 12, 183–210. doi: 10.1002/(SICI)1099-1727(199623)12:3<183::AID-SDR103>3.0.CO;2-4
Baustert, P., and Benetto, E. (2017). Uncertainty analysis in agent-based modeling and consequential life cycle assessment coupled models: a critical review. J. Clean. Prod. 156, 378–394. doi: 10.1016/j.jclepro.2017.03.193
Beloin-Saint-Pierre, D., Heijungs, R., and Blanc, I. (2014). The ESPA (Enhanced Structural Path Analysis) method: a solution to an implementation challenge for dynamic life cycle assessment studies. Int. J. Life Cycle Assess. 19, 861–871. doi: 10.1007/s11367-014-0710-9
Beloin-Saint-Pierre, D., Levasseur, A., Margni, M., and Blanc, I. (2017). Implementing a dynamic life cycle assessment methodology with a case study on domestic hot water production. J. Indust. Ecol. 21, 1128–1138. doi: 10.1111/jiec.12499
Blanco, C. F., Cucurachi, S., Guinée, J. B., Vijver, M. G., Peijnenburg, W. J., Trattnig, R., et al. (2020). Assessing the sustainability of emerging technologies: a probabilistic LCA method applied to advanced photovoltaics. J. Clean. Prod. 259, 120968. doi: 10.1016/j.jclepro.2020.120968
Cardellini, G., and Mutel, C. (2018). Temporalis: an open source software for dynamic LCA. J. Open Source Software 3, 612. doi: 10.21105/joss.00612
COM (2020). Critical Raw Materials Resilience: Charting a Path toward greater Security and Sustainability. (COM/2020/474 final). Brussels: European Commission Retrieved from: https://eur-lex.europa.eu/legal-content/EN/TXT/?uri=CELEX:52020DC0474 (accessed February 04, 2022)
Cucurachi, S., Blanco, C. F., Steubing, B., and Heijungs, R. (2021). Implementation of uncertainty analysis and moment-independent global sensitivity analysis for full-scale life cycle assessment models. J. Indust. Ecol. 1–19. doi: 10.1111/jiec.13194
de Koning, A., Kleijn, R., Huppes, G., Sprecher, B., van Engelen, G., and Tukker, A. (2018). Metal supply constraints for a low-carbon economy? Resour. Conserv. Recycl. 129, 202–208. doi: 10.1016/j.resconrec.2017.10.040
Deetman, S., de Boer, H. S., van Engelenburg, M., van der Voet, E., and van Vuuren, D. P. (2021). Projected material requirements for the global electricity infrastructure – generation, transmission and storage. Resour. Conserv. Recycl. 164, 105200. doi: 10.1016/j.resconrec.2020.105200
Duchin, F., Levine, S. H., and Strømman, A. H. (2016). Combining multiregional input-output analysis with a world trade model for evaluating scenarios for sustainable use of global resources, Part I: conceptual framework. J. Ind. Ecol. 20, 775–782. doi: 10.1111/jiec.12302
Ekholm, T. (2018). Climatic cost-benefit analysis under uncertainty and learning on climate sensitivity and damages. Ecol. Econ. 154, 99–106. doi: 10.1016/j.ecolecon.2018.07.024
Fallah, N., Fitzpatrick, C., Killian, S., and Johnson, M. (2021). End-of-life electric vehicle battery stock estimation in ireland through integrated energy and circular economy modelling. Resour. Conserv. Recycl. 174, 105753. doi: 10.1016/j.resconrec.2021.105753
FIA UKAEA (2021). The Global Fusion Industry in 2021. Fusion Companies Survey by the Fusion Industry Association and the UK Atomic Energy Authority. Retrieved from: https://www.fusionindustryassociation.org/about-fusion-industry (accessed February 06, 2022)
Fisher, E. (2017). Entangled futures and responsibilities in technology assessment. J. Responsible Innov. 4, 83–84. doi: 10.1080/23299460.2017.1372061
Geels, F. W. (2020). Micro-foundations of the multi-level perspective on socio-technical transitions: Developing a multi-dimensional model of agency through crossovers between social constructivism, evolutionary economics and neo-institutional theory. Technol. Forecast. Soc. Change. 152:119894. doi: 10.1016/j.techfore.2019.119894
Geels, F. W., and Schot, J. (2007). Typology of sociotechnical transition pathways. Res. Policy 36, 399–417. doi: 10.1016/j.respol.2007.01.003
Geels, F. W., Sovacool, B. K., Schwanen, T., and Sorrell, S. (2017). Sociotechnical transitions for deep decarbonization. Science 357, 1242–1244. doi: 10.1126/science.aao3760
Goodwin, P. (2012). Peak Travel, Peak Car and the Future of Mobility. Evidence, Unresolved Issues, Policy Implications, and a Research Agenda. Paris: OECD/ITF Discussion Paper. doi: 10.1787/9789282105931-3-en
Grunwald, A. (2020). The objects of technology assessment. Hermeneutic extension of consequentialist reasoning. J. Responsible Innov. 7, 96–112. doi: 10.1080/23299460.2019.1647086
Heijungs, R. (2010). Sensitivity coefficients for matrix-based LCA. Int. J. Life Cycle Assess. 15, 511–520. doi: 10.1007/s11367-010-0158-5
Heijungs, R., and Suh, S. (2002). The Computational Structure of Life Cycle Assessment (Vol. 11): Cham: Springer Science & Business Media. doi: 10.1007/978-94-015-9900-9
Hof, A. F., van Vuuren, D. P., and den Elzen, M. G. J. (2010). A quantitative minimax regret approach to climate change: does discounting still matter? Ecol. Econ. 70, 43–51. doi: 10.1016/j.ecolecon.2010.03.023
Huppes, G. (2019). Strategic Designs for Climate Policy Instrumentation, Governance at the Crossroads. Abingdon: Routledge. doi: 10.4324/9781315524818
IAEA (2020). Advances in Small Modular Reactor Technology Developments. A Supplement to: IAEA Advanced Reactors Information System (ARIS) 2020 Edition. Vienna: International Atomic Energy Agency. Retrieved from: https://aris.iaea.org/
IEA (2016). World Energy Outlook 2016. Paris: International Energy Agency. Retrieved from: www.worldenergyoutlook.org (accessed February 06, 2022)
Jenkins, S., Mitchell-Larson, E., Ives, M. C., Haszeldine, S., and Allen, M. (2021). Upstream decarbonization through a carbon takeback obligation: an affordable backstop climate policy. Joule 5, 2777–2796. doi: 10.1016/j.joule.2021.10.012
Karandeh, R. (2021). Decentralized Agent-Based Control of Distributed Energy Resources for Providing Multiple Services in Active Distribution Networks. Charlotte., NC: The University of North Carolina at Charlotte.
Kraan, O., Kramer, G. J., van der Lei, T., and Huppes, G. (2017). “Modelling the energy transition: towards an application of agent based modelling to integrated assessment modelling,” in Advances in Social Simulation 2015, eds W. Jager, R. Verbrugge, A. Flache, G. d. Roo, L. Hoogduin, and C. Hemelrijk (Cham: Springer), 207–216.
Kramer, G. J., and Haigh, M. (2009). No quick switch to low-carbon energy. Nature 462, 568–569. doi: 10.1038/462568a
Larrain, M., Van Passel, S., Thomassen, G., Kresovic, U., Alderweireldt, N., Moerman, E., et al. (2020). Economic performance of pyrolysis of mixed plastic waste: open-loop versus closed-loop recycling. J. Clean. Prod. 270, 122442. doi: 10.1016/j.jclepro.2020.122442
Liu, D., Liu, M., Xu, E., Pang, B., Guo, X., Xiao, B., et al. (2018). Comprehensive effectiveness assessment of renewable energy generation policy: a partial equilibrium analysis in China. Energy Policy 115, 330–341. doi: 10.1016/j.enpol.2018.01.018
Mariel, P., and Meyerhoff, J. (2018). A more flexible model or simply more effort? On the use of correlated random parameters in applied choice studies. Ecol. Econ. 154, 419–429. doi: 10.1016/j.ecolecon.2018.08.020
Masur, J. S., and Posner, E. A. (2011). Climate regulation and the limits of cost-benefit analysis. Cal. Law Rev. 99, 1557–1599. doi: 10.15779/Z38T13X
Mendoza Beltran, A., Cox, B., Mutel, C., van Vuuren, D. P., Font Vivanco, D., Deetman, S., et al. (2020). When the background matters: using scenarios from integrated assessment models in prospective life cycle assessment. J. Indust. Ecol. 24, 64–79. doi: 10.1111/jiec.12825
Mendoza Beltran, A., Prado, V., Font Vivanco, D., Henriksson, P. J. G., Guinée, J. B., and Heijungs, R. (2018). Quantified uncertainties in comparative life cycle assessment: what can be concluded? Environ. Sci. Technol. 52, 2152–2161. doi: 10.1021/acs.est.7b06365
Micolier, A., Loubet, P., Taillandier, F., and Sonnemann, G. (2019). To what extent can agent-based modelling enhance a life cycle assessment? Answers based on a literature review. J. Clean. Product. 239, 118123. doi: 10.1016/j.jclepro.2019.118123
Olsen, S. I., Borup, M., and Andersen, P. D. (2018). “Future-oriented LCA,” in Life Cycle Assessment: Theory and Practice, eds M. Z. Hauschild, R. K. Rosenbaum, and S. I. Olsen (Cham: Springer International Publishing), 499–518. doi: 10.1007/978-3-319-56475-3_21
Pauliuk, S., Arvesen, A., Stadler, K., and Hertwich, E. G. (2017). Industrial ecology in integrated assessment models. Nat. Clim. Change 7, 13–20. doi: 10.1038/nclimate3148
Pigné, Y., Gutiérrez, T. N., Gibon, T., Schaubroeck, T., Popovici, E., Shimako, A. H., et al. (2020). A tool to operationalize dynamic LCA, including time differentiation on the complete background database. Int. J. Life Cycle Assess. 25, 267–279. doi: 10.1007/s11367-019-01696-6
Plevin, R. J. (2017). Assessing the climate effects of biofuels using integrated assessment models, Part I: methodological considerations. J. Ind. Ecol. 21, 1478–1487. doi: 10.1111/jiec.12507
Qin, Y., Cucurachi, S., and Suh, S. (2020). Method to decompose uncertainties in LCA results into contributing factors. Int. J. Life Cycle Assess. 25, 1846–1858. doi: 10.1007/s11367-020-01787-9
Richardson, G. P. (2011). Reflections on the foundations of system dynamics. Syst. Dyn. Rev. 27, 219–243. doi: 10.1002/sdr.462
Robson, E. N., Wijayaratna, K. P., and Dixit, V. V. (2018). A review of computable general equilibrium models for transport and their applications in appraisal. Transp. Res. A: Policy Pract. 116, 31–53. doi: 10.1016/j.tra.2018.06.003
Schaubroeck, S., Schaubroeck, T., Baustert, P., Gibon, T., and Benetto, E. (2020). When to replace a product to decrease environmental impact?—a consequential LCA framework and case study on car replacement. Int. J. Life Cycle Assess. 25, 1500–1521. doi: 10.1007/s11367-020-01758-0
Schaubroeck, T., Baustert, P., Igos, E., and Benetto, E. (2020). Is a sustainability assessment a shot in the dark? How to deal with its nonquantified uncertainty? Environ. Sci. Technol. 54, 2051–2053. doi: 10.1021/acs.est.0c00450
Schaubroeck, T., Gibon, T., Igos, E., and Benetto, E. (2021b). Sustainability assessment of circular economy over time: modelling of finite and variable loops & impact distribution among related products. Resour. Conserv. Recycl. 168, 105319. doi: 10.1016/j.resconrec.2020.105319
Schaubroeck, T., Gibon, T., Igos, E., and Benetto, E. (2021c). CORRECTION – sustainability assessment of circular economy over time: modeling of finite and variable loops & impact distribution among related products. Resour. Conserv. Recycl. 172, 105675. doi: 10.1016/j.resconrec.2021.105675
Schaubroeck, T., Schaubroeck, S., Heijungs, R., Zamagni, A., Brandão, M., and Benetto, E. (2021a). Attributional & consequential life cycle assessment: definitions, conceptual characteristics and modelling restrictions. Sustainability 13, 7386. doi: 10.3390/su13137386
Seto, K. C., Davis, S. J., Mitchell, R. B., Stokes, E. C., Unruh, G., and Ürge-Vorsatz, D. (2016). Carbon lock-in: types, causes, and policy implications. Annu. Rev. Environ. Resour. 41, 425–452. doi: 10.1146/annurev-environ-110615-085934
Sigüenza, C. P., Steubing, B., Tukker, A., and Aguilar-Hernández, G. A. (2021). The environmental and material implications of circular transitions: a diffusion and product-life-cycle-based modeling framework. J. Indust. Ecol. 25, 563–579. doi: 10.1111/jiec.13072
Simon, H. A. (1956). Rational choice and the structure of the environment. Psychol. Rev. 63, 129. doi: 10.1037/h0042769
Stadler, K., Wood, R., Bulavskaya, T., Södersten, C.-J., Simas, M., Schmidt, S., et al. (2018). EXIOBASE 3: Developing a time series of detailed environmentally extended multi-regional input-output tables. J. Ind. Ecol. 22, 502–515. doi: 10.1111/jiec.12715
Su, S., Li, X., Zhu, Y., and Lin, B. (2017). Dynamic LCA framework for environmental impact assessment of buildings. Energy Build. 149, 310–320. doi: 10.1016/j.enbuild.2017.05.042
Suh, S. (2009). Handbook of Input-Output Economics in Industrial Ecology (Vol. 23). Springer Science & Business Media.
Sunstein, C. R., and Hahn, R. W. (2001). A new executive order for improving federal regulation? Deeper and wider cost-benefit analysis. Univ. Pa. Law Rev. 150, 1489–1552. doi: 10.2307/3312946
Thomassen, G., Van Passel, S., and Dewulf, J. (2020). A review on learning effects in prospective technology assessment. Renew. Sust. Energ. Rev. 130:109937. doi: 10.1016/j.rser.2020.109937
Thonemann, N., Schulte, A., and Maga, D. (2020). How to conduct prospective life cycle assessment for emerging technologies? A systematic review and methodological guidance. Sustainability 12, 1192. doi: 10.3390/su12031192
Tiruta-Barna, L., Pign,é, Y., Gutiérrez, T. N., and Benetto, E. (2016). Framework and computational tool for the consideration of time dependency in Life Cycle Inventory: proof of concept. J. Clean. Prod. 116, 198–206. doi: 10.1016/j.jclepro.2015.12.049
Tukker, A., Giljum, S., and Wood, R. (2018). Recent progress in assessment of resource efficiency and environmental impacts embodied in trade: an introduction to this special issue. J. Indust. Ecol. 22, 489–501. doi: 10.1111/jiec.12736
Uehara, T., Cordier, M., and Hamaide, B. (2018). Fully dynamic input-output/system dynamics modeling for ecological-economic system analysis. Sustainability 10:1765. doi: 10.3390/su10061765
van Beek, L., Hajer, M., Pelzer, P., van Vuuren, D., and Cassen, C. (2020). Anticipating futures through models: the rise of Integrated Assessment Modelling in the climate science-policy interface since 1970. Glob. Environ. Change 65, 102191. doi: 10.1016/j.gloenvcha.2020.102191
van der Giesen, C., Cucurachi, S., Guinée, J., Kramer, G. J., and Tukker, A. (2020). A critical view on the current application of LCA for new technologies and recommendations for improved practice. J. Clean. Prod. 259, 120904. doi: 10.1016/j.jclepro.2020.120904
Verones, F., Hellweg, S., Antón, A., Azevedo, L. B., Chaudhary, A., Cosme, N., et al. (2020). LC-IMPACT: a regionalized life cycle damage assessment method. J. Indust. Ecol. 24, 1201–1219. doi: 10.1111/jiec.13018
Walzberg, J., Dandres, T., Merveille, N., Cheriet, M., and Samson, R. (2019). Assessing behavioural change with agent-based life cycle assessment: application to smart homes. Renew. Sustain. Energy Rev. 111, 365–376. doi: 10.1016/j.rser.2019.05.038
Weber, S., Gerlagh, R., Mathys, N. A., and Moran, D. (2021). CO2 embodied in trade: trends and fossil fuel drivers. Environ. Sci. Pollut. Res. 28, 27712–27730. doi: 10.1007/s11356-020-12178-w
Weidema, B. P., Bauer, C., Hischier, R., Mutel, C., Nemecek, T., Reinhard, J., et al. (2013). Data Quality Guidelines for the Ecoinvent Database Version 3 Report 1 Overview and Methodology. Retrieved from: https://ecoinvent.org/wp-content/uploads/2020/10/dataqualityguideline_ecoinvent_3_20130506_.pdf (accessed February 04, 2022)
Wu, S. R., Li, X., Apul, D., Breeze, V., Tang, Y., Fan, Y., et al. (2017). Agent-based modeling of temporal and spatial dynamics in life cycle sustainability assessment. J. Indust. Ecol. 21, 1507–1521. doi: 10.1111/jiec.12666
Xiang, Y., Cai, H., Gu, C., and Shen, X. (2020). Cost-benefit analysis of integrated energy system planning considering demand response. Energy 192:116632. doi: 10.1016/j.energy.2019.116632
Keywords: predictive validity of product-technology models, qualitative validity and uncertainty, quantified uncertainty analysis and sensitivity analysis, sustainability of emerging technologies, prospective LCA, integrated assessment models (IAM), long-term scenario modeling, modeling domains - LCA-EEIOA-TA-CBA-MLP-SD-ABM-GEM-IAM
Citation: Huppes G and Schaubroeck T (2022) Forecasting the Future Sustainability of Technology Choices: Qualitative Predictive Validity of Models as a Complement to Quantitative Uncertainty. Front. Sustain. 3:629653. doi: 10.3389/frsus.2022.629653
Received: 15 November 2020; Accepted: 16 February 2022;
Published: 15 March 2022.
Edited by:
Dingsheng Li, University of Nevada, United StatesReviewed by:
Julien Walzberg, National Renewable Energy Laboratory (DOE), United StatesAnna Björklund, Royal Institute of Technology, Sweden
Copyright © 2022 Huppes and Schaubroeck. This is an open-access article distributed under the terms of the Creative Commons Attribution License (CC BY). The use, distribution or reproduction in other forums is permitted, provided the original author(s) and the copyright owner(s) are credited and that the original publication in this journal is cited, in accordance with accepted academic practice. No use, distribution or reproduction is permitted which does not comply with these terms.
*Correspondence: Gjalt Huppes, aHVwcGVzLmNtbEBnbWFpbC5jb20=
†These authors have contributed equally to this work